DOI:
10.1039/D2RA02645G
(Paper)
RSC Adv., 2022,
12, 18834-18847
Virtual screening of natural products against 5-enolpyruvylshikimate-3-phosphate synthase using the Anagreen herbicide-like natural compound library†
Received
26th April 2022
, Accepted 14th June 2022
First published on 29th June 2022
Abstract
The shikimate pathway enzyme 5-enolpyruvylshikimate-3-phosphate synthase (EPSPS) catalyzes a reaction involved in the production of amino acids essential for plant growth and survival. EPSPS is the main target of glyphosate, a broad-spectrum herbicide that acts as a competitive inhibitor concerning phosphoenolpyruvate (PEP), which is the natural substrate of EPSPS. In the present study, we introduce a natural compound library, named Anagreen, which is a compendium of herbicide-like compounds obtained from different natural product databases. Herein, we combined the structure- and ligand-based virtual screening strategies to explore Anagreen against EPSPS using the structure of glyphosate complexed with a T102I/P106S mutant of EPSPS from Eleusine indica (EiEPSPS) as a starting point. First, ligand-based pharmacophore screening was performed to select compounds with a similar pharmacophore to glyphosate. Then, structure-based pharmacophore modeling was applied to build a model which represents the molecular features of glyphosate. Then, consensus docking was performed to rank the best poses of the natural compounds against the PEP binding site, and then molecular dynamics simulations were performed to analyze the stability of EPSPS complexed with the selected ligands. Finally, we have investigated the binding affinity of the complexes using free energy calculations. The selected hit compound, namely AG332841, showed a stable conformation and binding affinity to the EPSPS structure and showed no structural similarity to the already known weed EPSPS inhibitors. Our computational study aims to clarify the inhibition of the mutant EiEPSPS, which is resistant to glyphosate, and identify new potential herbicides from natural products.
Introduction
Glyphosate (N-(phosphonomethyl)glycine) is the most relevant and widely used broad-spectrum organophosphate herbicide in agriculture, owing to its low cost and high efficiency.1,2 Glyphosate inhibits 5-enolpyruvylshikimate-3-phosphate synthase (EPSPS), a transferase family enzyme that converts phosphoenolpyruvate (PEP) and shikimate-3-phosphate (S3P) to 5-enolpyruvylshikimate-3-phosphate (EPSP) in the penultimate step of the shikimate pathway leading to the biosynthesis of aromatic amino acids.3–5 Glyphosate acts as a competitive inhibitor of PEP, mimicking an intermediate state of the EPSPS–substrate complex, thus inhibiting enzyme catalysis.6
The application of glyphosate for a wide range of industrial crops has led to the emergence of new resistant weeds worldwide.7 According to the International Survey of Herbicide Resistant Weeds website,8 51 weed species have acquired resistance to EPSPS-targeting herbicides, and in most cases, the resistance is caused by 2 to 5 molecular mechanisms at the same time. Different EPSPS mutations have been reported to confer a target-site resistance to glyphosate in weeds,9–16 including the double substitution Pro106Leu and Thr102Ile observed in several weed species.17–21
Eleusine indica (E. indica) is a highly invasive diploid grass (also known as goosegrass or yard-grass) originating from Asia that has adapted to a wide range of climate conditions, and currently, it is a common weed in tropical, subtropical, and temperate regions of the world that has evolved resistance to multiple herbicides.22 Glyphosate is known to be one of the main herbicides for E. indica control.23 The first single resistance mutation P106S was identified in E. indica EPSPS (EiEPSPS) two decades ago by Baerson et al.24 The double mutation T102I + P106S was first characterized also in E. indica.18 It confers a higher level of glyphosate resistance than single mutations at position 106 (such as P106L, P106T, P106A, and T102I). Thus, there is an urgent need for new herbicide discoveries to overcome the glyphosate resistance in E. indica.
Computational methods have been widely applied to perform virtual screening of bioactive compounds against specific molecular targets.25–27 Up to date, there are dozens of reports about the successful application of molecular docking, pharmacophore modeling, QSAR modeling in drug and pesticide discovery.28–32 However, studies reporting the discovery of novel compounds with herbicidal activity by rational in silico modeling are rather scarce.
Considering that natural products act as an interesting resource of novel bioactive compounds with industrial applications,33–35 we constructed currently the largest library of herbicide-like small organic compounds named Anagreen Herbicide-Like (AHL) comprising more than 62 thousand unique compounds of natural origin. In this work, we present a hierarchical ligand- and structure-based virtual screening approach following an in-depth validation to identify novel E. indica EPSPS-targeting compounds based on a herbicide-like compound library AHL. This study explores the molecular chemo-structural diversity of natural products and their potential bioactivity against the native (EiEPSPSwt) and T102I/P106S mutant (EiEPSPSmut) of EPSPS structures obtained from Eleusine indica.
Results and discussion
Anagreen herbicide-like natural products library
A set of predefined filters suggested by Avram et al.28 have been applied to the initially constructed core dataset (395
742 compounds), which allowed us to construct a targeted library, entitled Anagreen herbicide-like, comprising 62
706 nature-derived compounds. To the best of our knowledge, it is the largest natural product-based library aimed at discovering new compounds with herbicide-like properties available currently.
Using Canvas,36 we performed a cheminformatics analysis of the prepared dataset. The median molecular weight of the dataset (SD) is 337.5 ± 62.5 Da, while the median Alog
P is 3.19 ± 1.25 units. The number of H-bond acceptors varies from 2 to 7 (the most typical value is 4), whereas the number of H-bond donors – from 0 to 2 (the mode is 1). The total count of rotatable bonds in the dataset varies between 1 and 11 (the mode is 5), chiral centers – from 0 to 11 (the most typical value is 0), rings – from 0 to 3 (the mode is 3). The median polar surface area is 69.64 ± 27.80 Å2.
Validation of the screening protocols
First, two validation datasets were generated using the same input – known actives against EPSPS using a total of 25 compounds (ESI Table S1†). Since there are Ki values only for compounds targeting E. coli K12 EPSPS, they were selected as positive controls in validation screening. The datasets were prepared using different decoy-generating tools, namely DUD-E (Directory of Useful Decoys, Enhanced)37 and RADER (RApid DEcoy Retriever),38 and had a 1
:
60 vs. 1
:
35 ratio of actives to decoys, respectively. Since two different chemical classes of compounds, the phosphonomethylglycines (e.g., glyphosate) and phosphonooxycyclohexenes, are known to inhibit EPSPS in vitro and in vivo (based on the compounds available in ChEMBL39), we explored not only glyphosate/glyphosate-bound complexes (PDB IDs: 3FJZ, 2QFU, 2GGA, 2AAY, 1G6S, 3NVS, 3SLH, 1RF6) but also an intermediate state-like inhibitor (ISLI)/ISLI-bound complexes (PDB IDs: 2PQC, 2PQD).
First, we tested the screening power of glyphosate-based pharmacophore modeling protocols. The pharmacophore models constructed from the glyphosate–EPSPS interactions (PDB IDs: 3FJZ) or overlayed multiple (PDB IDs: 3FJZ, 2QFU, 2GGA, 2AAY, 1G6S, 3NVS, 3SLH, and 1RF6) active conformations of glyphosate contained three pharmacophore features – two negative ionic groups and one H-bond donor or a positive ionic group (Fig. S1, panels (A) and (B)†). Both of these models showed satisfactory screening power with high enrichment factor (EF) values and efficient early recognition for top-0.5% compounds, especially in the case of RADER-generated validation set, but moderate performance for top-1% and top-2% compounds (Table 1, Fig. S2, panels (A)–(D)†). However, the interaction-based model had higher ROC AUC (area under the receiver operating characteristic curve) values and, thus, was selected as the first filter for the initial screening of the Anagreen herbicide-like dataset.
Table 1 Results of the pharmacophore modeling protocols validation
Validation dataset |
Enrichment metric |
ROC AUC |
EF0.5%a |
EF1% |
EF2% |
BEDROC (α = 321.9) |
BEDROC (α = 161.9) |
BEDROC (α = 80.4) |
Due to the small sample size, EF0.5% should be treated with caution. |
Glyphosate interaction-based model |
DUD-E |
0.839 |
20.2 |
9.3 |
3.9 |
0.399 |
0.245 |
0.181 |
RADER |
0.843 |
27.2 |
13.6 |
3.7 |
0.719 |
0.475 |
0.305 |
![[thin space (1/6-em)]](https://www.rsc.org/images/entities/char_2009.gif) |
Glyphosate active conformations-based model |
DUD-E |
0.799 |
20.2 |
9.3 |
5.9 |
0.407 |
0.265 |
0.198 |
RADER |
0.726 |
27.2 |
13.6 |
4.9 |
0.722 |
0.496 |
0.340 |
![[thin space (1/6-em)]](https://www.rsc.org/images/entities/char_2009.gif) |
ISLI interaction-based model |
DUD-E |
0.828 |
42.6 |
28.0 |
16.3 |
0.668 |
0.527 |
0.465 |
RADER |
0.879 |
15.7 |
9.1 |
12.1 |
0.579 |
0.410 |
0.379 |
![[thin space (1/6-em)]](https://www.rsc.org/images/entities/char_2009.gif) |
ISLI active conformations-based model |
DUD-E |
0.620 |
51.2 |
32.7 |
16.3 |
0.833 |
0.631 |
0.486 |
RADER |
0.793 |
31.4 |
36.2 |
19.3 |
0.966 |
0.825 |
0.640 |
Then, we evaluated the ISLI-based pharmacophore modeling protocols. The pharmacophore hypotheses built from the ISLI–EPSPS interactions (PDB IDs: 2PQC) or overlayed multiple (PDB IDs: 2PQC, 2PQD) active conformations of ISLI (named [3R-[3A,4A,5B(R*)]]-5-(1-carboxy-1-phosphonoethoxy)-4-hydroxy-3-(phosphonooxy)-1-cyclohexene-1-carboxylic acid) contained a different number of pharmacophore features, i.e., the interaction-based model comprised five features (three negative ionic groups, one H-bond acceptor and one H-bond donor), while the active conformations-based – only three (one negative ionic group and two H-bond acceptors) (Fig. S1, panels (C) and (D)†). Both models showed excellent screening power (Table 1, Fig. S2, panels (E)–(H)†) for top-0.5% and top-1% of the list, mainly when the RADER-generated dataset was used as a target. In the case of the top-2% of the results, the enrichment factor (EF) and Boltzmann-enhanced discrimination of ROC (BEDROC) values were moderate. Since the early recognition capability and enrichment values in the active conformations-based model were much better, it was selected as the second filter for the initial screening step.
Pharmacophore-based screening of Anagreen herbicide-like library
Two separate pharmacophore-based screening runs were performed to filter the Anagreen herbicide-like dataset and maintain only the pharmacophore-compliant compounds. Firstly, we screened the target library using glyphosate–EPSPS interactions-based pharmacophore (GEIB-Ph), comprising two negative ionic points and one H-bond donor. Top-0.5% of the hit-list (314 compounds in total) were retrieved and inspected. The Phase screen score in this selection varied from 0.11 (lowest) to 1.38 (highest), with only 109 compounds having a score higher than 1.00. The most IB-Ph-compliant compound was AG327841 (2-amino-3-(2,4-dibromo-3-hydroxyphenyl)propanoic acid).
Secondly, we filtered the target library using ISLI multiple active conformations-based pharmacophores (IMACB-Ph) composed of one negative ionic group and two H-bond acceptors. This time, the output list of IMACB-Ph-compliant compounds was less than 0.5% (in sum, 165 compounds) of the whole dataset, so all hits were retrieved and analyzed. The Phase screen score in this selection varied from 0.51 (lowest) to 1.44 (highest), with 138 compounds having a score higher than 1.00. AG328959 (2-(2-hydroxyphenyl)-4-methyl-5H-1,3-thiazole-4-carboxylic acid) was shown to be the most IMACB-Ph-compliant compound.
Since both pharmacophore models overlayed in part (via a negative ionic feature), we hypothesized that hits from GEIB-Ph and IMACB-Ph runs would also overlay to some extent. Therefore, after merging both lists and removing duplicates, 35% of all compounds were discarded, and the final list of unique hits compliant with at least one of the used pharmacophore models contained 309 compounds, which were promoted to the docking-based screening stage.
Consensus docking analysis
The rapid evolution of weed resistance against the herbicide glyphosate has been considered from several perspectives, and the search for new herbicides has become urgent. Herein, we conceived a consensus docking that integrates the predictions of independent scoring functions to rank potential leads. The 309 pharmacophore-compliant hits were first docked against the wild-type and mutant variants of EiEPSPS using Glide SP (standard precision) protocol. Unfortunately, less than 10% of the compounds were successfully docked either to wild-type or mutant EPSPS. Then, the successfully docked compounds were docked and scored again using GOLD to increase the robustness of the results. Table 2 shows the two scoring functions of the consensus docking of the best hit compounds and considers the binding energies and the intermolecular interactions into the binding sites (root mean square deviation (RMSD) < 2 Å).
Table 2 Results of consensus docking exhibiting the seven best hit compounds obtained against the EiEPSPS structures
Ligand ID |
Glide energies (kcal mol−1) |
GOLD energies (kcal mol−1) |
X (esc) |
Consensus docking |
Glide energies |
GOLD energies |
EiEPSPSwt |
AG332841 |
−6.28 |
74.3319 |
1 |
1 |
2 |
AG322323 |
−5.56 |
58.3528 |
0.78904 |
0.67624 |
1.46528 |
AG325666 |
−6.33 |
25.5606 |
1.00000 |
0.01181 |
1.01181 |
AG351308 |
−2.68 |
72.1308 |
0 |
0.95540 |
0.95540 |
AG334346 |
−4.98 |
24.9777 |
0.63014 |
0.00000 |
0.63014 |
AG327871 |
−3.41 |
34.7255 |
0.20000 |
0.19751 |
0.39751 |
AG327875 |
−3.96 |
26.0414 |
0.35068 |
0.02155 |
0.37224 |
![[thin space (1/6-em)]](https://www.rsc.org/images/entities/char_2009.gif) |
EiEPSPSmut |
AG332841 |
−6.43 |
74.6396 |
1 |
1 |
2 |
AG351308 |
−3.24 |
72.5991 |
0.22573 |
0.96373 |
1.18946 |
AG327871 |
−3.98 |
33.4005 |
0.40534 |
0.26693 |
0.67227 |
AG351307 |
−2.73 |
38.6039 |
0.10194 |
0.35943 |
0.46137 |
AG327875 |
−3.32 |
26.5210 |
0.24515 |
0.14464 |
0.38979 |
AG334346 |
−2.31 |
39.8455 |
0.00000 |
0.38150 |
0.38150 |
AG324178 |
−3.04 |
18.3840 |
0.17718 |
0.00000 |
0.17718 |
The best consensus docking results were observed for the ligands AG332841 and AG351308 EiEPSPSmut which showed similar patterns of the hydrogen-bond interactions mainly with the residues of Arg131, Thr102, Asp50, Gly101, Arg404, Arg105, and Lys23. Among these residues, we can highlight Lys23, Asp50, Gly101, Thr102, Arg105, Arg131, and Arg404, which are responsible for essential interactions with PEP and glyphosate in the PEP-binding site.40,41 Additionally, for EiEPSPSwt, AG322323 and AG325666 appear with a consensus value higher than 1 and presented a similar pattern of interactions at the active site. Based on these results, the ligands AG332841 and AG351308 were selected for subsequent analyses against EiEPSPSmut while AG332841, AG351308, AG322323 and AG325666 were selected for subsequent analyses against EiEPSPSwt.
Binding affinities and dynamic analyses of natural products complexed with EiEPSPS
Some reports revealed that docking methods have several limitations in sampling ligand conformational space and correlating docking scores with experimental binding affinities.42,43 End-point binding free energy calculations using MM/GBSA (Molecular Mechanics energies combined with the Generalized Born and Surface Area) methods have improved accuracy in protein–ligand binding free energy calculations.44 Thus, we have employed MM/GBSA calculations to evaluate the binding energies of the compounds that presented scores higher than 0.9. Binding to EiEPSPSwt and EiEPSPSmut both ligands AG332841 and AG351308 presented great affinity energies (Table 3). On the other hand, for EiEPSPSwt, ligands AG322323 and AG325666 showed binding energies of −37.92 (±3.99) and −30.68 (±5.10), respectively.
Table 3 Binding free energy values (kcal mol−1) were obtained for the complexes formed with the wild-type and mutant EiEPSPS structures
MM/GBSA: binding free energy |
Energy (kcal mol−1) |
EiEPSPSwt |
EiEPSPSmut |
Glyphosate |
AG351308 |
AG332841 |
Glyphosate |
AG351308 |
AG332841 |
vdW |
−3.78 (±4.45) |
−33.23 (±3.90) |
−22.68 (±3.68) |
−3.39 (±4.30) |
−35.07 (±2.48) |
−27.89 (±3.29) |
EEL |
−178.63 (±37.15) |
9.87 (±16.31) |
3.95 (±8.84) |
−88.16 (±42.53) |
67.04 (±10.67) |
−7.56 (±12.20) |
ΔGgas |
−182.41 (±36.82) |
−23.36 (±16.77) |
−18.73 (±8.33) |
−91.56 (±41.15) |
31.97 (±10.22) |
−35.45 (±11.45) |
EGB |
74.38 (±28.75) |
−34.43 (±12.39) |
−47.71 (±7.26) |
16.41 (±31.37) |
−67.96 (±9.53) |
−23.54 (±8.72) |
ESURF |
−3.31 (±0.14) |
−5.36 (±0.14) |
−4.13 (±0.06) |
−3.48 (±0.17) |
−4.62 (±0.17) |
−4.08 (±0.09) |
ΔGsolv |
71.06 (±28.71) |
−39.79 (±12.33) |
−51.84 (±7.26) |
12.93 (±31.26) |
−72.59 (±9.52) |
−27.63 (±8.71) |
ΔGtotal |
−111.34 (±15.98) |
−63.16 (±8.32) |
−70.58 (±4.01) |
−78.62 (±15.51) |
−40.62 (±3.42) |
−63.08 (±6.03) |
As can be seen in Table 3, our simulation of the EPSPS structures complexed with the glyphosate inhibitor presented the lowest binding energy. However, despite the importance of hydrophobic interactions for the lead optimization, we also noted that vdW analysis satisfactorily represented the EiEPSPSmut–ligand affinity. The energy values obtained from MM/GBSA method showed the importance of considering the solvation energy in the herbicide design against EiEPSPS structure. Energy values of −70.58 and −63.08 kcal mol−1 for wild-type and mutant structures, respectively, showed to be determinants for AG332841 affinity against EiEPSPSwt binding pocket. We also noticed the high electrostatic contribution related to glyphosate binding. Other studies have considered that glyphosate forms at least seven electrostatic interactions.40,41 These findings ratify the importance of the electrostatic interactions in the lead optimization for EiEPSPS structure.
We compared the binding affinities of the ligand AG332841 with glyphosate performing the per-residue energy decomposition analysis in the EiEPSPSwt and EiEPSPSmut structures (Fig. 1). The residues Lys23, Arg28, Gln101, Thr/Ile102, Arg105, Gln180, Lys358, Glu359, Arg362, His403, Arg404, and Lys429 formed favorable interactions and contributed to the overall affinity of the AG332841 complex. Similarly, glyphosate interacts with the residues of the EiEPSPS binding pocket, such as Lys23, Arg28, Gly101, Thr/Ile102, Arg105, Arg 131, Gln180, Lys358, His403, Arg404, and Lys429. The main favorable residue difference between systems with glyphosate and AG332841 is Arg131 which has a favorable energy value closer to −30 kcal mol−1 in the EiEPSPSwt and closer to −20 kcal mol−1 in the EiEPSPSmut system while for AG332841 it is −0.4 kcal mol−1 in EiEPSPSmut and −0.13 kcal mol−1. The main residues that contributed to the value of binding free energy obtained from the AG351308–EiEPSPSwt and AG351308–EiEPSPSmut complexes did not present values better than the systems complexed to glyphosate and AG332841 (Fig. S8†).
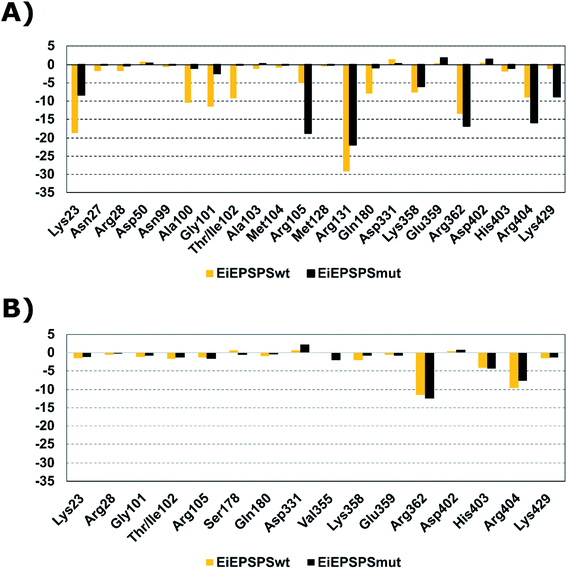 |
| Fig. 1 Per-residue energy decomposition analysis of main residues with energy decomposition value ≤ or ≥0.5 kcal mol−1 of (A) glyphosate and (B) AG332841 complexed with EiEPSPSwt and EiEPSPSmut structures. | |
To evaluate the dynamics of the interactions between EiEPSPS and the best-ranked ligands, we first plotted the RMSD values of the EiEPSPS–ligand complexes obtained during 150 ns of molecular dynamics (MD) simulations (Fig. 2). The selected ligands AG332841 and AG351308 were shown to be stable in both wild-type (EiEPSPSwt) and mutant (EiEPSPSmut) complexes.
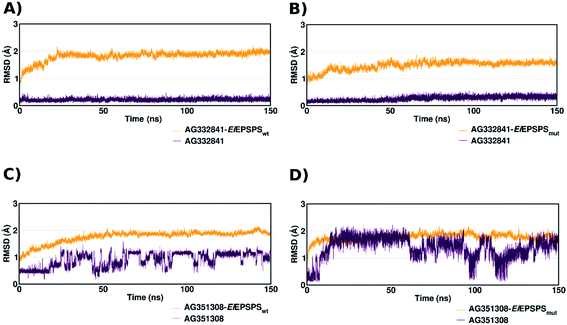 |
| Fig. 2 RMSD plots of the heavy atoms in the EiEPSPSwt– and EiEPSPSmut–ligand complexes (orange) and the ligands (maroon) were analyzed over 150 ns of MD simulation. (A) Complex of EiEPSPSwt and AG332841; (B) complex of EiEPSPSmut and AG332841; (C) complex of EiEPSPSwt and AG351308; (D) complex of EiEPSPSmut and AG351308. | |
The ligand AG332841 complexed with EiEPSPSwt and EiEPSPSmut showed higher stability in the binding pocket, evidenced by RMSD values obtained during the MD simulation (Fig. 2, panels (A) and (B)). In contrast, the ligand AG351308 showed higher fluctuations. The movement of the ligand in the active site of EiEPSPSwt during the simulation caused the loss of some H-bond interactions with the residues of the binding pocket, such as Arg105. RMSD analysis for this ligand shows local fluctuations, which demonstrates the instability of the ligand structure in the active site (Fig. S3 and S4†).
Root-Mean-Square Fluctuations (RMSF) plot was performed in the last 50 ns of the system to identify the fluctuation of the protein amino acids. In our simulations, EiEPSPS has mostly low fluctuations (between 1 to 2 Å) with only 3 loop regions reaching values greater than 3 Å (Fig. 3, panels (A)–(C)). It is important to note that region III refers to the C-terminal region and it has high movement during the simulation. However, we observed that in both regions I and II the system complexed with AG332841 showed much lower fluctuation than the system complexed with AG351308 and glyphosate in either wild-type or mutant protein. Considering only the binding pocket region, we noticed lower fluctuations in both EiEPSPSwt and EiEPSPSmut residues when complexed with AG332841 in comparison with AG351308 and glyphosate (Fig. 3, panels (D) and (E)).
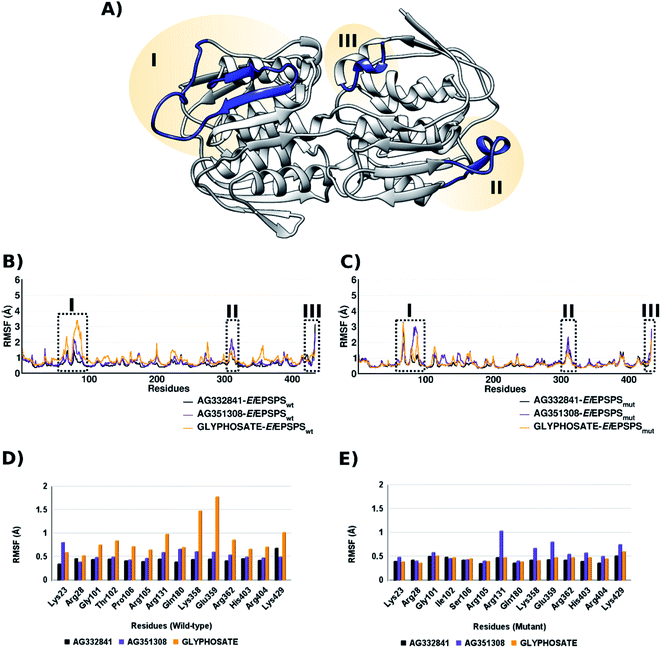 |
| Fig. 3 (A) Regions of EiEPSPS with fluctuation values ≥3.0 Å. RMSF plot, in Å, of the backbones atoms in the (B) EiEPSPSwt– and (C) EiEPSPSmut–ligand complexes analyzed over the last 50 ns of MD simulation. Panels (D) and (E) show the fluctuations of the main binding pocket residues in EiEPSPSwt– and EiEPSPSmut–ligand complexes, respectively. | |
We noticed that the ligand AG332841 formed similar H-bond interactions to those verified by glyphosate in the EiEPSPSwt binding site as described in the literature.41 Besides that, additional H-bonds were formed with the residue His403 in the binding site (Fig. 4, panels (A) and (B)).
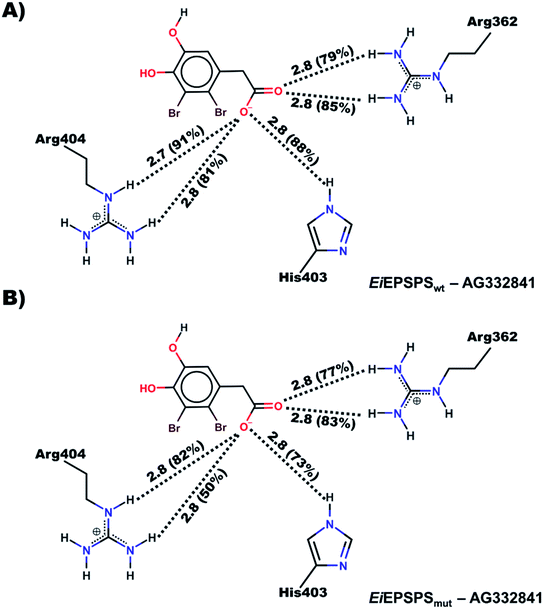 |
| Fig. 4 The 2D diagram of the (A) EiEPSPSwt and (B) EiEPSPSmut complexed with AG332841 shows the average distances of the main intermolecular interactions with occupancy value ≥50% obtained over the MD simulation. | |
Similarly, those interactions were also observed in the AG332841–EiEPSPSmut complex. The ligand AG332841 formed interactions with residues Arg362 and Arg404 similar to those verified for glyphosate in the EiEPSPSmut binding site (Fig. S7†) and additional hydrogen interaction formed with His403 (Fig. 4, panel (B)). Besides that, we verified that the interactions between Ile102 and bromine atoms of the ligand suggest better stability due to the formation of van der Waals interactions.
The electrostatic potential map is a widely used coloring scheme of protein surfaces that indicates the overall charge distribution.45–48 Meanwhile, electrostatic maps are predictive of the chemical reactivity of ligands and their types of intermolecular interactions. We analyzed the electrostatic potential map of EiEPSPSwt and EiEPSPSmut to investigate potential affinity sites of the bromine interface of AG332841. The electrostatic map of AG332841 showed a neutral charge distribution. Red regions indicate negative potential, and blue ones show positive potential (see Fig. 5).
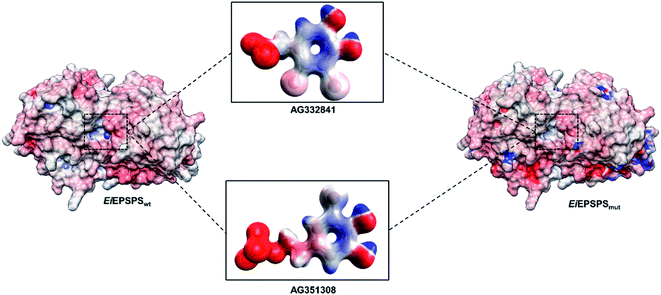 |
| Fig. 5 The electrostatic potential maps of EiEPSPSwt and EiEPSPSmut enzymes and AG332841 and AG351308 ligands. | |
Ile102 has a sizeable hydrophobic interface at the active site of the wild-type enzyme when compared with the Thr102 present in the mutant structure. Based on these results, we conjecture that hydrophobic interactions play a vital role in the formation of EiEPSPS–ligand complexes, and these interactions should be further considered for ligand optimization.
Estimation of AG332841 scaffold novelty
The compound named AG332841 (2,3-dibromo-4,5-dihydroxyphenyl)acetic acid has shown to be the most promising hit against EiEPSPS and its structure novelty was estimated based on the 2D similarity to the known compounds deposited in the ChEMBL database that inhibit plant or bacterial EPSPS structures. AG332841 scaffold belongs to the chemical family of hydroxylated phenylacetates, while the majority of known EPSPS inhibitors are phosphonomethylglycines (like glyphosate) and phosphonooxycyclohexenes. The median Tc value between AG332841 and the list of known inhibitors of EPSPS was 0.11, suggesting a lack of structural similarity. Even the highest Tc value (0.44), which was obtained for compound CHEMBL98618, showed a low similarity. Moreover, the most similar compound, CHEMBL98618, inhibits E. coli EPSPS, but there is no evidence of its action against plant EPSPS. Thus, we conjecture that AG332841 has a new chemical scaffold not seen earlier for other potential inhibitors of plant EPSPS structures.
This compound was taken to Anagreen herbicide-like dataset from the COlleCtion of Open Natural Products (COCONUT), where it has ID CNP0289943. Originally, AG332841 was isolated from a marine red algae Rhodomela confervoides in 2011.49
Final considerations
In the present study, we applied cheminformatics and molecular modeling methods involving a combination of structure- and ligand-based virtual screening approaches to identify natural product-derived compounds with the potential to inhibit the wild-type (sensitive to glyphosate) and mutant structures (resistant to glyphosate) of EiEPSPS. Our approach intended to increase the success of finding novel herbicides using the prefiltered dataset of compounds with the physicochemical properties common to commercial herbicides. Moreover, molecular docking and MD simulations of both wild-type and mutant T102I/P106S EiEPSPS structures were carried out for the best compounds to verify the stability of the predicted binding poses, which, according to Lagarias et al.,50 correlates with the bioactivity. The final hit compound, namely AG332841, showed to be stable over the MD simulation and it also demonstrated a satisfactory binding affinity to EiEPSPS structures. In addition, it has no structural similarity to the already known EPSPS inhibitors, which could allow further exploration of this compound as a potential herbicide against plant EPSPS structures. As for now, it is speculative to say that this compound is a better EPSPS inhibitor than glyphosate. However, its novel chemical scaffold and dissimilar pattern of interactions together with a comparable predicted affinity towards a mutant EiEPSPS make AG332841 a promising candidate for further exploration.
Computational methods
A general overview of the computational workflow applied in the present study to filter the herbicide-like natural compounds with high affinity to EiEPSPS is shown in Fig. 6.
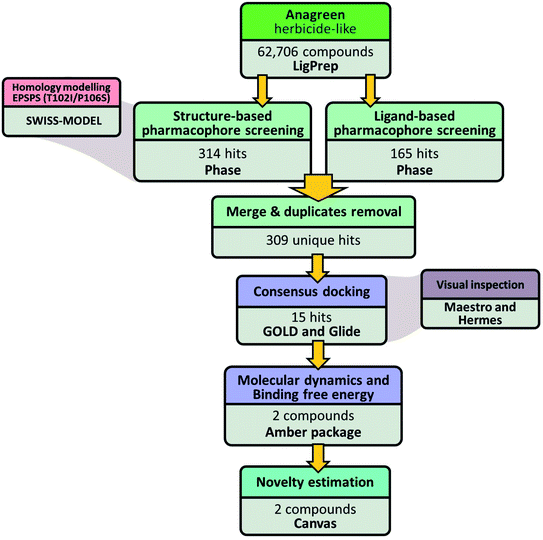 |
| Fig. 6 Computational workflow applied in the virtual screening strategy. | |
Anagreen herbicide-like dataset construction and preparation
First, we collected the available structural data from several different open natural compound databases, including TCM Database@Taiwan,51 Central African medicinal plants natural product database (ConMedNP),25 Cameroonian medicinal natural products database (CamMedNP),52 NuBBEDB,53,54 Seaweed Metabolites Database (SWMD),55 Dictionary of Marine Natural Products (CMNPD),56 Indonesian Herbal Constituents Database (IHD),57 Vietnamese Herbal Medicine Database (VIETHERB),58 Indofine Natural Products (INDOFINE Chemical Company, Inc.) and InterBioScreen Natural Products library (IBSDB, InterBioScreen Ltd). Then, we manually collected the names of metabolites from a few other databases lacking structural data, including well-known Dr Duke's ethnobotanical and phytochemical database59 and some other libraries. JChem and ChemOffice were used to translate names into 2D structures. Those compounds that failed to be translated were translated manually via search in PubChem, ChEMBL, and ChemSpider. Then, all compounds were consolidated and sorted, duplicates were removed, and standardized names (AG******, where * is a digit from 0 to 9) were assigned to all compounds using Canvas. In sum, 395
742 compounds with 2D structures have been accumulated (this dataset was named “Anagreen Core 1.0”). Then, we applied a set of cheminformatics criteria suggested by Avram et al.28 to create a target dataset called Anagreen Herbicide-Like (AHL; 62
706 compounds): molecular weight: 150–500, clog
P ≤ 3.5, hydrogen bond donors: ≤3, hydrogen bond acceptor: 2–12, and rotatable bonds: <12 (Fig. 7).
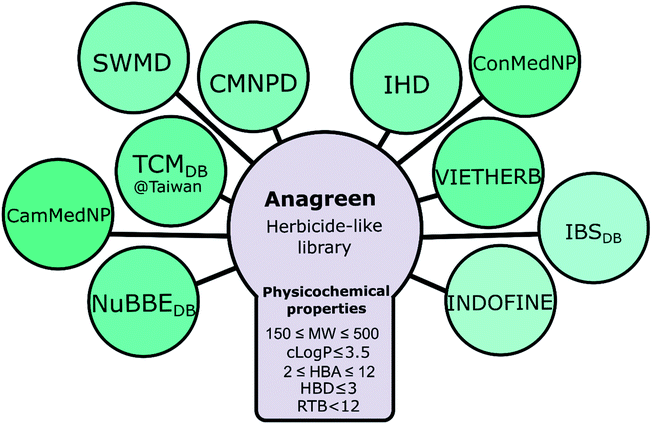 |
| Fig. 7 Construction of the Anagreen herbicide-like natural compound library. | |
Validation of compound datasets and libraries
The datasets consisting of experimentally validated antagonists obtained from the ChEMBL database39 and putative decoys of the plant PPO were created with DUD-E decoy generating tool37 and RADER web-server.38 Both datasets were used to assess the compound screening using different tools. To avoid bias towards particular scaffolds, all compounds were clustered based upon Tc values using Canvas, and only the most diverse of them were saved. In all cases, ≈1
:
50 ratio between inhibitors and decoys was explicitly set, according to the following two datasets: (1) 25 EPSPS antagonists and 1254 decoys (DUD-E dataset); (2) 25 EPSPS antagonists and 761 decoys (RADER dataset).
Enrichment calculations
All ranked lists were preprocessed in Microsoft® Excel® for Office 365 MSO (Microsoft Inc., Albuquerque, NM, USA) and analyzed by the Screening Explorer tool60 available online. The following metrics have been calculated both for all tautomers (full list) and the best tautomers (original list without duplicates): enrichment factor (EF), receiver operating characteristic (ROC), and Boltzmann-enhanced discrimination of ROC (BEDROC). The ROC curves and their AUC are known to provide a common scale to compare the performances of virtual screening methods. However, they suffer from some intrinsic limitations, such as an early recognition problem, that can be overcome with other metrics, such as BEDROC.61
The BEDROC scores using the three α values were calculated for both protein targets based on the test screening results obtained from two docking programs, Glide and GOLD. BEDROC (α = 321.9) corresponds to docking enrichments at 0.5%, BEDROC (α = 161.9) – to EF at 1%, and BEDROC (α = 80.4) – to EF at 2%, requiring that 80% of the score comes from the respective selections.62
The ROC curves were built, and the area under these curves was calculated with Rocker.63 In the best-case scenario, an ideal model ROC curve has a steep slope and a high area under the curve (AUC). It means that the model finds all the active molecules and no inactive hits, sensitivity, and specificity are 1, and the EF is high.
Structure-based pharmacophore modeling
Ligand-based pharmacophore modeling. To predict the pharmacophore, we used as a starting point the structure of glyphosate complexed with EiEPSPS. Then, we selected three receptor–ligand pharmacophore hypotheses, and the top-ranked model was selected according to the most relevant interactions formed by glyphosate with the EPSPS binding site.
Interaction-based pharmacophore modeling. To generate a pharmacophore hypothesis, we based upon the EPSPS structure complexed with shikimate and glyphosate (PDB ID: 2AAY).64 The models were generated using the ‘Develop Pharmacophore Model’ module of Phase software incorporated in the Schrodinger Small Molecule Suite 2020-4.65 The following parameters were used for hypotheses generation: scoring function – Phase Hypo Score, number of features in the hypotheses – from 2 to 7, donors as projection points, create receptor-based excluded volume shell, limit excluded volume shell thickness to 5.00 Å, pharmacophore generation method – Auto/E-pharmacophore. All other parameters were used with default values. Validation datasets were screened with the following options: generate conformers during search – true, target number of conformers – 250, return all hits, scoring function – Phase Screen Score. Then, the results were sorted according to the Screen Score and exported for enrichment calculations. The actual pharmacophore-based screening was carried out using Phase, and the target number of conformers was set to 500. The hit-list was sorted based upon the Phase Screen Score, and the top-0.5% of hits (this sample size was previously shown to have the highest enrichment values) were isolated for further analysis.
Consensus molecular docking
Two molecular docking tools, CSD GOLD66 and Schrodinger Glide,67 were used in parallel according to a consensus docking approach to increase the robustness of a screening protocol.
The CSD GOLD program was used to perform molecular docking simulations to analyze the binding mode of five selected natural products complexed to EiEPSPS that obtained the best pharmacophore-like similarity with the selected pharmacophore model. First, to validate the docking protocol, redocking simulations were performed with the glyphosate structure against EPSPS. Root Mean Square Deviation (RMSD) values less than or equal to 1.0 Å were considered satisfactory for replicating the ligand binding mode in the crystallographic structure. Next, for the docking simulations, the docking grids with a radius of 8 to 15 Å, depending on the selected natural products, were positioned in the EiEPSPS active site using the spatial coordinates of crystallographic glyphosate as a reference. Water molecules, ions, and the glyphosate structure were removed from the EiEPSPS structure before the docking simulations. Then, we performed the flexible docking protocol of GOLD, which considers the flexibility of the ligand and residues side chains.66 All poses obtained were ranked according to the best superposition with the crystallographic structure of glyphosate and lower docking energy.
Glide incorporated in Schrodinger Small Molecule Suite 2020-4 was used as a second docking tool. Before grid generation, all protein structures were prepared using the Protein Preparation module68 regarding the correct bond order, with the addition of missing hydrogens and removal of crystallographic waters. Then, a restrained energy minimization under the OPLS-3e force field was applied. The active site of the prepared protein was defined using default parameters of receptor-grid generation (using a scaling factor of 0.8 for the van der Waals radii of protein atoms) present in the Glide module. The ligands were prepared with the LigPrep module,69 and all possible tautomers were generated at pH 7.0 ± 2.0 using Epik, the specified chiralities were retained. The in-depth conformational search for each ligand was carried out with the ConfGen module using the default options. Different docking calculations were performed for each grid by using a ligand van der Waals scaling factor of 0.8 or 1.0, and sampling was performed by either standard (SP) or extra (XP) precision algorithms using the default GlideScore scoring function.
Molecular dynamics simulations
The modeled structures of wild-type and mutant EPSPS structures were obtained from our previous study.41 To analyze the variant structures of EPSPS (mutant and wild-type) complexed with the substrate shikimate-3-phosphate (S3P), the inhibitor (glyphosate), and the two best ligand poses selected from the pharmacophore-based virtual screening, we performed 150 ns of MD simulations in the Amber18 package.70 The coordinates of glyphosate and shikimate-3-phosphate were obtained from the crystallographic structure (PDB ID: 3NVS). First, the protonation state of the ionizable residues was analyzed by pKa calculations using the PDB2PQR server.71 Second, the ligand charges were calculated using the restrained electrostatic potentials (RESP) approach with the Hartree–Fock method and the 6-31G* basis set in the Gaussian09 program.72 Third, the tLeap module of Amber18 was used to build the receptor–ligand complex parameters where the ff14SB force field73 describes the protein atoms, and the general amber force field74 treats the ligand atoms. Finally, these complexes were solvated in a cubic water box using the TIP3P explicit solvation model75 with a radius of 12.0 Å between the box and the protein surface. Counterions were added to neutralize the protein–ligand systems. Before performing the MD simulation, each protein system was minimized to reduce the overall energy using the steepest-descent and conjugate gradient algorithms.76 The minimization was performed in four steps: the first step corresponds to the minimization of the solvation waters and counterions of the system, the second minimization included hydrogen atoms of the protein, in the third step, all hydrogens and water molecules were minimized, and finally, the whole system.
After minimization, the heating of the systems was started at 0 K and gradually increased to 300 K over 100 ps with constant volume constraints. We carried out 200 ps of density equilibration with weak restraints on the EPSPS–ligand complexes followed by 700 ps of constant pressure equilibration at 300 K. A Langevin thermostat was used to maintain the temperature of the system at 300 K. The SHAKE algorithm77 was used to maintain all of the H-bonds at their equilibrium distances, which allowed the use of an integration time step of 2 fs. Then, we performed 150 ns of MD simulation for each analyzed system.
Binding free energy calculations
The trajectories of each MD simulation were obtained to calculate the binding free energy of EiEPSPS complexed with glyphosate and the two best ligand poses of natural products according to the selected pharmacophore modeling. Then, the energy calculations were performed using molecular mechanics generalized Born surface area continuum solvation (MM/GBSA)78 method available in the Amber18 package. Using the CPPTRAJ program implemented in Amber18, the non-complexed ions and waters were removed before performing the binding free energy calculations. For both mutant and wild-type complexes, 5000 trajectory snapshots were used to compute the binding free energy values.
Estimation of selected scaffolds novelty
The novelty of selected hits was estimated using the Schrödinger Canvas software.36 We calculated the maximum pairwise Tanimoto similarity of each hit regarding the 29 in vitro validated plant EiEPSPS inhibitors in the ChEMBL28 database using the extended chemical fingerprints for four atoms (ECFP4). Those compounds with the lowest Tc values were treated as carriers of novel chemical scaffolds for the development of EPSPS-targeting herbicides.
Conflicts of interest
There are no conflicts to declare.
Acknowledgements
S. V. is grateful to the bioinformatics (J. V. Lehtonen), translational activities, and structural biology (FINStruct) infrastructure support from Biocenter Finland and CSC IT Center for Science for computational infrastructure support at the Structural Bioinformatics Laboratory (SBL), Åbo Akademi University. SBL is part of the NordForsk Nordic POP (Patient Oriented Products), the Solutions for Health strategic area of Åbo Akademi University, and the InFlames Flagship program of the Academy of Finland on inflammation, cancer, and infection, University of Turku and Åbo Akademi University. A. H. L. and K. S. da C. are grateful to Conselho Nacional de Desenvolvimento Científico e Tecnológico (CNPq, Brazil) for the financial support of the research and to Laboratório Nacional de Computação Científica (LNCC/MCTI/Brazil) for the supercomputer facilities; and M. V. D. O. and G. B. are grateful to Coordenação de Aperfeiçoamento de Pessoal de Nível Superior (CAPES, Brazil) for the scholarship.
References
- S. O. Duke and S. B. Powles, Glyphosate: a once-in-a-century herbicide, Pest Manage. Sci., 2008, 64, 319–325 CrossRef CAS PubMed.
- S. O. Duke, The history and current status of glyphosate, Pest Manage. Sci., 2018, 74, 1027–1034 CrossRef CAS PubMed.
- H. Maeda and N. Dudareva, The shikimate pathway and aromatic amino acid biosynthesis in plants, Annu. Rev. Plant Biol., 2012, 63, 73–105 CrossRef CAS PubMed.
- S. O. Duke, Glyphosate degradation in glyphosate-resistant and -susceptible crops and weeds, J. Agric. Food Chem., 2011, 59, 5835–5841 CrossRef CAS PubMed.
- A. M. dos Santos, A. H. Lima, C. N. Alves and J. Lameira, Unraveling the addition–elimination mechanism of EPSP synthase through computer modeling, J. Phys. Chem. B, 2017, 121, 8626–8637 CrossRef CAS PubMed.
- E. Schönbrunn, et al., Interaction of the herbicide glyphosate with its target enzyme 5-enolpyruvylshikimate 3-phosphate synthase in atomic detail, Proc. Natl. Acad. Sci. U. S. A., 2001, 98, 1376–1380 CrossRef PubMed.
- E. Bracamonte, P. T. Fernández-Moreno, F. Barro and R. de Prado, Glyphosate-resistant Parthenium hysterophorus in the Caribbean Islands: non target site resistance and target site resistance in relation to resistance levels, Front. Plant Sci., 2016, 7, 1845 Search PubMed.
- I. Heap, The International Herbicide-Resistant Weed Database, 2022 Search PubMed.
- I. Heap and S. O. Duke, Overview of glyphosate-resistant weeds worldwide, Pest Manage. Sci., 2018, 74, 1040–1049 CrossRef CAS PubMed.
- V. E. Perotti, et al., A novel triple amino acid substitution in the EPSPS found in a high-level glyphosate-resistant Amaranthus hybridus population from Argentina, Pest Manage. Sci., 2019, 75, 1242–1251 CrossRef CAS PubMed.
- T. A. Gaines, et al., Gene amplification confers glyphosate resistance in Amaranthus palmeri, Proc. Natl. Acad. Sci. U. S. A., 2010, 107, 1029–1034 CrossRef CAS PubMed.
- M. J. García, et al., Multiple mutations in the EPSPS and ALS genes of Amaranthus hybridus underlie resistance to glyphosate and ALS inhibitors, Sci. Rep., 2020, 10, 17681 CrossRef PubMed.
- S. Nasr Ramzi, M. M. Sohani, R. Shirzadian-Khorramabad, J. Asghari and M. Amininasab, Enhancement of glyphosate tolerance in rice (Oryza sativa L.) through mutation induction in EPSPS (5-enolpyruvylshikimate-3-phosphate synthase), Plant Gene, 2020, 22, 100225 CrossRef CAS.
- T. C. Ramalho, M. S. Caetano, E. F. F. da Cunha, T. C. S. Souza and M. V. J. Rocha, Construction and assessment of reaction models of class I EPSP synthase: molecular docking and density functional theoretical calculations, J. Biomol. Struct. Dyn., 2009, 27, 195–207 CrossRef CAS PubMed.
- M. Yanniccari, et al., Mechanism of resistance to glyphosate in Lolium perenne from Argentina, Front. Ecol. Evol., 2017, 5, 123, DOI:10.3389/fevo.2017.00123.
- R. Alcántara-de la Cruz, et al., First resistance mechanisms characterization in glyphosate-resistant Leptochloa virgata, Front. Plant Sci., 2016, 7, 1742, DOI:10.3389/fpls.2016.01742.
- J. Chen, et al., Mutations and amplification of EPSPS gene confer resistance to glyphosate in goosegrass (Eleusine indica), Planta, 2015, 242, 859–868 CrossRef CAS PubMed.
- Q. Yu, et al., Evolution of a double amino acid substitution in the 5-enolpyruvylshikimate-3-phosphate synthase in Eleusine indica conferring high-level glyphosate resistance, Plant Physiol., 2015, 167, 1440–1447 CrossRef CAS PubMed.
- R. Alcántara-de la Cruz, et al., Target and non-target site mechanisms developed by glyphosate-resistant hairy beggarticks (Bidens pilosa L.) populations from Mexico, Front. Plant Sci., 2016, 7, 1492 Search PubMed.
- M. S. Bell, A. G. Hager and P. J. Tranel, Multiple resistance to herbicides from four site-of-action groups in waterhemp (Amaranthus tuberculatus), Weed Sci., 2013, 61, 460–468 CrossRef CAS.
- J. Gherekhloo, et al., Pro-106-Ser mutation and EPSPS overexpression acting together simultaneously in glyphosate-resistant goosegrass (Eleusine indica), Sci. Rep., 2017, 7, 6702 CrossRef PubMed.
- R. K. Nishimoto and L. B. McCarty, Fluctuating temperature and light influence seed germination of goosegrass (Eleusine indica), Weed Sci., 1997, 45, 426–429 CrossRef CAS.
- C. Zhang, et al., Evolution of multiple target-site resistance mechanisms in individual plants of glyphosate-resistant Eleusine indica from China, Pest Manage. Sci., 2021, 77, 4810–4817 CrossRef CAS PubMed.
- S. R. Baerson, et al., Glyphosate-resistant goosegrass. Identification
of a mutation in the target enzyme 5-enolpyruvylshikimate-3-phosphate synthase, Plant Physiol., 2002, 129, 1265–1275 CrossRef CAS PubMed.
- M. D. De Oliveira, J. d. O. Araújo, J. M. P. Galúcio, K. Santana and A. H. Lima, Targeting shikimate pathway: in silico analysis of phosphoenolpyruvate derivatives as inhibitors of EPSP synthase and DAHP synthase, J. Mol. Graphics Modell., 2020, 101, 107735 CrossRef CAS PubMed.
- K. S. da Costa, et al., Targeting peptidyl-prolyl cis-trans isomerase NIMA-interacting 1: a structure-based virtual screening approach to find novel inhibitors, Curr. Comput.-Aided Drug Des., 2020, 16, 605–617, DOI:10.2174/1573409915666191025114009.
- K. Santana, et al., Applications of virtual screening in bioprospecting: facts, shifts, and perspectives to explore the chemo-structural diversity of natural products, Front. Chem., 2021, 9, 662688, DOI:10.3389/fchem.2021.662688.
- S. Avram, et al., Quantitative estimation of pesticide-likeness for agrochemical discovery, J. Cheminf., 2014, 6, 42, DOI:10.1186/s13321-014-0042-6.
- Y. Fu, et al., 3D pharmacophore-based virtual screening and docking approaches toward the discovery of novel HPPD inhibitors, Molecules, 2017, 22, 959 CrossRef PubMed.
- G.-F. Hao, Y. Tan, N.-X. Yu and G.-F. Yang, Structure–activity relationships of diphenyl-ether as protoporphyrinogen oxidase inhibitors: insights from computational simulations, J. Comput.-Aided Mol. Des., 2011, 25, 213–222 CrossRef CAS PubMed.
- Y. Fu, et al., Combination of virtual screening protocol by in silico toward the discovery of novel 4-hydroxyphenylpyruvate dioxygenase inhibitors, Front. Chem., 2018, 6, 14, DOI:10.3389/fchem.2018.00014.
- T. P. P. Ribeiro, F. G. Manarin and E. Borges de Melo, In silico study toward the identification of new and safe potential inhibitors of photosynthetic electron transport, Ecotoxicol. Environ. Saf., 2018, 153, 175–180 CrossRef CAS PubMed.
- J. M. Galúcio, et al., In silico identification of natural products with anticancer activity using a chemo-structural database of Brazilian biodiversity, Comput. Biol. Chem., 2019, 83, 107102 CrossRef PubMed.
- K. S. da Costa, et al., Exploring the potentiality of natural products from essential oils as inhibitors of odorant-binding proteins: a structure- and ligand-based virtual screening approach to find novel mosquito repellents, ACS Omega, 2019, 4, 22475–22486 CrossRef CAS PubMed.
- L. D. Do Nascimento, et al., Bioactive natural compounds and antioxidant activity of essential oils from spice plants: new findings and potential applications, Biomolecules, 2020, 10, 1–37 Search PubMed.
- Canvas, Schrödinger, LLC, New York, NY, 2019 Search PubMed.
- M. M. Mysinger, M. Carchia, J. J. Irwin and B. K. Shoichet, Directory of useful decoys, enhanced (DUD-E): better ligands and decoys for better benchmarking, J. Med. Chem., 2012, 55, 6582–6594 CrossRef CAS PubMed.
- L. Wang, X. Pang, Y. Li, Z. Zhang and W. Tan, RADER: a RApid DEcoy Retriever to facilitate decoy based assessment of virtual screening, Bioinformatics, 2017, 33, 1235–1237 CAS.
- A. Gaulton, et al., The ChEMBL database in 2017, Nucleic Acids Res., 2017, 45, D945–D954 CrossRef CAS PubMed.
- J. Li, et al., Glyphosate resistance in Tridax procumbens via a novel EPSPS Thr-102-Ser substitution, J. Agric. Food Chem., 2018, 66, 7880–7888, DOI:10.1021/acs.jafc.8b01651.
- E. C. M. Fonseca, K. S. da Costa, J. Lameira, C. N. Alves and A. H. Lima, Investigation of the target-site resistance of EPSP synthase mutants P106T and T102I/P106S against glyphosate, RSC Adv., 2020, 10, 44352–44360 RSC.
- G. L. Warren, et al., A critical assessment of docking programs and scoring functions, J. Med. Chem., 2006, 49, 5912–5931 CrossRef CAS PubMed.
- X. He, et al., Fast, accurate, and reliable protocols for routine calculations of protein–ligand binding affinities in drug design projects using AMBER GPU-TI with ff14SB/GAFF, ACS Omega, 2020, 5, 4611–4619 CrossRef CAS PubMed.
- E. Wang, et al., End-point binding free energy calculation with MM/PBSA and MM/GBSA: strategies and applications in drug design, Chem. Rev., 2019, 119, 9478–9508 CrossRef CAS PubMed.
- J. Neves Cruz, K. S. da Costa, T. A. A. de Carvalho and N. A. N. de Alencar, Measuring the structural impact of mutations on cytochrome P450 21A2, the major steroid 21-hydroxylase related to congenital adrenal hyperplasia, J. Biomol. Struct. Dyn., 2020, 38, 1425–1434 CrossRef CAS PubMed.
- C. H. S. d. Costa, et al.,
Unraveling the conformational dynamics of glycerol 3-phosphate dehydrogenase, a nicotinamide adenine dinucleotide-dependent enzyme of Leishmania mexicana, J. Biomol. Struct. Dyn., 2021, 39, 2044–2055, DOI:10.1080/07391102.2020.1742206.
- C. H. S. Costa, et al., Assessment of the PETase conformational changes induced by poly(ethylene terephthalate) binding, Proteins: Struct., Funct., Bioinf., 2021, 89, 1340–1352 CrossRef PubMed.
- K. S. Da Costa, et al., Structural analysis of viral infectivity factor of HIV type 1 and its interaction with A3G, EloC and EloB, PLoS One, 2014, 9, e89116 CrossRef PubMed.
- K. Li, X.-M. Li, J. B. Gloer and B.-G. Wang, Isolation, characterization, and antioxidant activity of bromophenols of the marine red alga Rhodomela confervoides, J. Agric. Food Chem., 2011, 59, 9916–9921 CrossRef CAS PubMed.
- P. Lagarias, et al., Discovery of novel adenosine receptor antagonists through a combined structure- and ligand-based approach followed by molecular dynamics investigation of ligand binding mode, J. Chem. Inf. Model., 2018, 58, 794–815 CrossRef CAS PubMed.
- C. Y. C. Chen, TCM Database@Taiwan: the world's largest traditional Chinese medicine database for drug screening in silico, PLoS One, 2011, 6, 1–5 Search PubMed.
- F. Ntie-Kang, et al., CamMedNP: building the Cameroonian 3D structural natural products database for virtual screening, BMC Complementary Altern. Med., 2013, 13, 88, DOI:10.1186/1472-6882-13-88.
- A. C. Pilon, et al., NuBBEDB: an updated database to uncover chemical and biological information from Brazilian biodiversity, Sci. Rep., 2017, 7, 7215, DOI:10.1038/s41598-017-07451-x.
- M. Valli, et al., Development of a natural products database from the biodiversity of Brazil, J. Nat. Prod., 2013, 76, 439–444 CrossRef CAS PubMed.
- G. D. J. Davis and A. H. R. Vasanthi, Seaweed metabolite database (SWMD): a database of natural compounds from marine algae, Bioinformation, 2011, 5, 361–364, DOI:10.6026/97320630005361.
- J. Lei and J. Zhou, A marine natural product database, J. Chem. Inf. Comput. Sci., 2002, 42, 742–748, DOI:10.1021/ci010111x.
- S. Naeem, P. Hylands and D. Barlow, Construction of an Indonesian herbal constituents database and its use in Random Forest modelling in a search for inhibitors of aldose reductase, Bioorg. Med. Chem., 2012, 20, 1251–1258, DOI:10.1016/j.bmc.2011.12.033.
- T. H. Nguyen-Vo, et al., VIETHERB: a database for Vietnamese herbal species, J. Chem. Inf. Model., 2019, 59, 1–9, DOI:10.1021/acs.jcim.8b00399.
- U.S. Department of Agriculture, Agricultural Research Service, Dr. Duke's Phytochemical and Ethnobotanical Databases, 1992–2016, DOI:10.15482/USDA.ADC/1239279, https://phytochem.nal.usda.gov/.
- C. Empereur-Mot, J. F. Zagury and M. Montes, Screening Explorer-an interactive tool for the analysis of screening results, J. Chem. Inf. Model., 2016, 56, 2281–2286 CrossRef CAS PubMed.
- C. Empereur-mot, et al., Predictiveness curves in virtual screening, J. Cheminf., 2015, 7, 52 Search PubMed.
- J. F. Truchon and C. I. Bayly, Evaluating virtual screening methods: good and bad metrics for the ‘early recognition’ problem, J. Chem. Inf. Model., 2007, 47, 488–508, DOI:10.1021/ci600426e.
- S. Lätti, S. Niinivehmas and O. T. Pentikäinen, Rocker: open source, easy-to-use tool for AUC and enrichment calculations and ROC visualization, J. Cheminf., 2016, 8, 45 Search PubMed.
- M. A. Priestman, M. L. Healy, T. Funke, A. Becker and E. Schönbrunn, Molecular basis for the glyphosate-insensitivity of the reaction of 5-enolpyruvylshikimate 3-phosphate synthase with shikimate, FEBS Lett., 2005, 579, 5773–5780 CrossRef CAS PubMed.
- Schrödinger Release 2020-4: Phase, Schrödinger, LLC, New York, NY, 2020 Search PubMed.
- M. L. Verdonk, J. C. Cole, M. J. Hartshorn, C. W. Murray and R. D. Taylor, Improved protein-ligand docking using GOLD, Proteins: Struct., Funct., Bioinf., 2003, 52, 609–623 CrossRef CAS PubMed.
- R. A. Friesner, et al., Glide: a new approach for rapid, accurate docking and scoring. 1. Method and assessment of docking accuracy, J. Med. Chem., 2004, 47, 1739–1749 CrossRef CAS PubMed.
- Schrödinger Release 2022-2: Maestro, Schrödinger, LLC, New York, NY, 2021 Search PubMed.
- LigPrep, Schrödinger, LLC, New York, NY, USA, 2019 Search PubMed.
- D. A. Case, et al., Amber 2018, University of California, San Francisco, 2018 Search PubMed.
- T. J. Dolinsky, J. E. Nielsen, J. A. McCammon and N. A. Baker, PDB2PQR: an automated pipeline for the setup of Poisson-Boltzmann electrostatics calculations, Nucleic Acids Res., 2004, 32, W665–W667 CrossRef CAS PubMed.
- M. J. Frisch, G. W. Trucks, H. B. Schlegel, G. E. Scuseria, M. A. Robb, J. R. Cheeseman, G. Scalmani, V. Barone, G. A. Petersson, H. Nakatsuji, X. Li, M. Caricato, A. Marenich, J. Bloino, B. G. Janesko, R. Gomperts, B. Mennucci, H. P. Hratchian, J. V. Ortiz, A. F. Izmaylov, J. L. Sonnenberg, D. Williams-Young, F. Ding, F. Lipparini, F. Egidi, J. Goings, B. Peng, A. Petrone, T. Henderson, D. Ranasinghe, V. G. Zakrzewski, J. Gao, N. Rega, G. Zheng, W. Liang, M. Hada, M. Ehara, K. Toyota, R. Fukuda, J. Hasegawa, M. Ishida, T. Nakajima, Y. Honda, O. Kitao, H. Nakai, T. Vreven, K. Throssell, J. A. Montgomery Jr, J. E. Peralta, F. Ogliaro, M. Bearpark, J. J. Heyd, E. Brothers, K. N. Kudin, V. N. Staroverov, T. Keith, R. Kobayashi, J. Normand, K. Raghavachari, A. Rendell, J. C. Burant, S. S. Iyengar, J. Tomasi, M. Cossi, J. M. Millam, M. Klene, C. Adamo, R. Cammi, J. W. Ochterski, R. L. Martin, K. Morokuma, O. Farkas, J. B. Foresman, and D. J. Fox, Gaussian 09, Revision A.02, Gaussian, Inc., Wallingford CT, 2016 Search PubMed.
- J. A. Maier, et al., ff14SB: improving the accuracy of protein side chain and backbone parameters from ff99SB, J. Chem. Theory Comput., 2015, 11, 3696–3713 CrossRef CAS PubMed.
- J. Wang, R. M. Wolf, J. W. Caldwell, P. A. Kollman and D. A. Case, Development and testing of a general Amber force field, J. Comput. Chem., 2004, 25, 1157–1174, DOI:10.1002/jcc.20035.
- W. L. Jorgensen, Transferable intermolecular potential functions for water, alcohols, and ethers. Application to liquid water, J. Am. Chem. Soc., 1981, 103, 335–340 CrossRef CAS.
- M. R. Hestenes and E. Stiefel, Methods of conjugate gradients for solving linear systems, J. Res. Natl. Bur. Stand., 1952, 49, 409 CrossRef.
- J.-P. Ryckaert, G. Ciccotti and H. J. Berendsen, Numerical integration of the Cartesian equations of motion of a system with constraints: molecular dynamics of n-alkanes, J. Comput. Phys., 1977, 23, 327–341 CrossRef CAS.
- T. Hou, J. Wang, Y. Li and W. Wang, Assessing the performance of the MM/PBSA and MM/GBSA methods. 1. The accuracy of binding free energy calculations based on molecular dynamics simulations, J. Chem. Inf. Model., 2011, 51, 69–82 CrossRef CAS PubMed.
|
This journal is © The Royal Society of Chemistry 2022 |
Click here to see how this site uses Cookies. View our privacy policy here.