DOI:
10.1039/D1MO00132A
(Research Article)
Mol. Omics, 2021,
17, 939-947
Extensive heterogeneity of glycopeptides in plasma revealed by deep glycoproteomic analysis using size-exclusion chromatography†
Received
27th April 2021
, Accepted 27th July 2021
First published on 4th August 2021
Abstract
Several plasma glycoproteins are clinically useful as biomarkers in a variety of diseases. Although thousands of proteins are present in plasma, >95% of the plasma proteome by mass is represented by only 22 proteins. This necessitates strategies to deplete the abundant proteins and enrich other subsets of proteins. Although glycoproteins are abundant in plasma, in routine proteomic analyses, glycopeptides are not often investigated. Traditional methods such as lectin-based enrichment of glycopeptides followed by deglycosylation have helped understand the glycoproteome, but they lack any information about the attached glycans. Here, we apply size-exclusion chromatography (SEC) as a simple strategy to enrich intact N-glycopeptides based on their larger size which achieves broad selectivity regardless of the nature of attached glycans. Using this approach, we identified 1317 N-glycopeptides derived from 266 glycosylation sites on 154 plasma glycoproteins. The deep coverage achieved by this approach was evidenced by extensive heterogeneity that was observed. For instance, 20–100 glycopeptides were observed per protein for the 15 most-glycosylated glycoproteins. Notably, we discovered 615 novel glycopeptides of which 39 glycosylation sites (from 38 glycoproteins) were not included in protein databases such as Uniprot and GlyConnectDB. Finally, we also identified 12 novel glycopeptides containing di-sialic acid, which is a rare glycan epitope. Our results demonstrate the utility of SEC for efficient LC-MS/MS-based deep glycoproteomics analysis of human plasma. Overall, the SEC-based method described here is a simple, rapid and high-throughput strategy for characterization of any glycoproteome.
Introduction
Human blood plasma is a routinely available clinical sample and is an excellent source for the discovery of biomarkers in disease states. Though plasma contains >10
000 proteins,1,2 99% of the total plasma protein mass is accounted for by only 22 of them, thus complicating proteomic analysis.3 Alternative approaches have been adopted such as study of hemodialysis fluid because it is depleted in highly abundant proteins.4 However, studies of whole plasma are preferred, and potential biomarkers can be proteins of lower abundance. Biomarker discovery is greatly impacted by the dynamic range of protein abundance. Plasma protein levels are known to be affected by heritability, age and diet.5–8 Several possible protein biomarkers are, in fact, N-linked glycoproteins such as thyroglobulin and alpha-fetoprotein, which have markedly higher sensitivity and specificity compared to non-glycosylated proteins.9 Glycoproteomics is developing at a rapid pace and glycoprotein biomarkers discovered through quantitative analysis of intact glycopeptides hold immense potential for clinical translation.10–12 Depletion of abundant proteins for detection of low abundance ones for biomarker discovery, although attractive for several reasons, is not easily translated clinically and may generate additional variability. Moreover, depletion of proteins makes assays difficult to deploy in clinical laboratories.
Analysis of glycopeptides by mass spectrometry requires their enrichment as non-glycosylated peptides are more abundant and they dominate the spectra owing to their higher proton affinity.13 We sought to establish an intact N-glycopeptide profiling workflow for mass spectrometry-based analysis which can be performed in a short time from smaller amounts of undepleted human plasma. Deeper profiling experiments of intact glycopeptides require enrichment as well as fractionation of glycopeptides. Enrichment is traditionally done by lectin-affinity chromatography (LAC), although this requires larger amounts of starting material. Hydrophilic interaction chromatography (HILIC)14,15 and chemical enrichment strategies16 are also popular alternatives. LAC further requires fractionation of enriched glycopeptides by orthogonal methods such as basic pH reversed phase liquid chromatography for deeper coverage. Size-exclusion chromatography (SEC) has previously been proposed for enrichment of intact N-glycopeptides followed by PNGase-F digestion for their identification.17 However, it has not evaluated for deep profiling and simultaneous enrichment/fractionation of intact glycopeptides. We present here an optimized intact N-glycopeptide workflow for undepleted human plasma which combines enrichment and simultaneous fractionation by SEC from very small amounts of human plasma (1 μl per LC-MS/MS injection). It was followed by intact glycopeptide analysis to identify peptide sequences, glycosylation sites, glycan composition and plausible glycan structures. We compared the results with LAC-enriched glycopeptides coupled to fractionation into 12 fractions.
Methods
Samples and ethical approval
Fifty individual lithium-heparin anticoagulated control blood samples were used to obtain plasma by double centrifugation and a pool was made by combining equal volumes. This study was approved by the Institutional Review Board at Mayo Clinic (approval number IRB19-004317). This pooled sample was used for all experiments described in this study.
Sample processing and trypsin digestion
Protein concentration in pooled plasma was estimated by BCA assay. Plasma containing 2 mg protein was aliquoted into a microcentrifuge tube and diluted 10 times with ice cold acetone. After vortexing vigorously for 10 seconds, the sample was incubated at −20 °C for 2 hours and centrifuged at 14
000 × g for 20 minutes. The supernatant was discarded, and the pellet was dissolved in 100 μl of 8 M urea in 50 mM triethylammonium bicarbonate (TEAB), pH 8.5. Dithiothreitol (Sigma) was added to the sample at a final concentration of 10 mM and incubated at 37 °C for 45 minutes with mild shaking. The sample was cooled to room temperature (RT) and iodoacetamide (Sigma) was added at a final concentration of 40 mM and incubated for 15 minutes in the dark at RT. The sample was subsequently diluted 10 times with TEAB buffer, pH 8.5 and sequencing-grade trypsin was added to a final amount of 1
:
50 (trypsin
:
total protein), and the mixture was incubated overnight at 37 °C with mild shaking. Next day, digested peptide mixture was dried as such in speed vacuum drier at 35 °C. In a separate tube 8 mg of total plasma protein was digested in the same way. Digested peptides were acidified with 1% trifluoroacetic acid and cleaned up with C18 tips (TopTip, Glygen) according to manufacturer's instructions. The eluate from C18 tips (40% acetonitrile) was dried at 35 °C in speed vacuum system.
Size-exclusion chromatography
Dried peptide mixture without cleanup (2 mg) was dissolved in 100 μl of 0.1% formic acid by vortexing and water bath sonication for 1 minute. The resulting 100 μl was put in the sample manager of Agilent Infinity 1260 II HPLC system. Superdex peptide 10/300 column (GE Healthcare) was equilibrated for 2 hours with 0.1% formic acid as solvent. A flow rate of 0.2 ml min−1 was used throughout the isocratic run using 0.1% formic acid. A total of 48 fractions were collected starting at 10 minutes after injection until the end of the run (total run time of 130 minutes). 20 μl of each fraction was analyzed by LC-MS/MS as described in following sections.
Multi-lectin affinity chromatography
Lectin-affinity chromatography was performed parallelly for glycopeptide enrichment using four agarose-bound lectins: Concanavalin A (ConA, binds high mannose glycans), Sambucus nigra agglutinin (SNA, binds α2,6 linked N-acetyl neuraminic acid), Lens culinaris agglutinin (LCA, binds core fucosylated bi- and triantennary glycans) and Aleuria alantia Lectin (AAL, binds core and branch fucose). We selected four different lectins according to their distinct binding specificities to cover a broad range of N-glycopeptides.12 200 ml of 50% slurry of each of these lectins was separately washed with 400 ml of binding/wash buffer (10 mM HEPES buffer, pH 7.4 with 150 mM NaCl, 0.1 mM CaCl2, 0.1 mM MgCl2 and 0.1 mM MnCl2) three times before combining in one tube. After removing residual binding/wash buffer, 8 mg of digested plasma peptides, which were cleaned up with C18 tips as described, dried, and dissolved in 400 μl of binding/wash buffer, were incubated with this multi-lectin column overnight at 4 °C with rotation. The next day, the non-bound fraction was collected by centrifuging the spin columns at 1000 × g for 1 min, and lectin beads were washed 2 times with binding/wash buffer at the same speed. The resulting beads were used for eluting bound glycopeptides with 100 ml of a mixture of four sugars (200 mM α-methyl mannopyranoside, 200 mM α-methyl glucopyranoside, 500 mM lactose, and 100 mM L-fucose) followed by 100 ml of 2% formic acid. Both elutions were combined in one tube and cleaned up with C18 tips and dried as described above.
Basic pH reversed phase liquid chromatography
The one-pot eluted glycopeptides from multi-lectin affinity chromatography were fractionated by bRPLC on a reversed phase C18 column (4.6 × 100 mm column) using an Ultimate 3000 UHPLC System. The solvent A used was 5 mM ammonium formate, pH 9 and solvent B was 5 mM ammonium formate, pH 9, in 90% acetonitrile. Ninety-six fractions were collected for a total run time of 120 min in a time-based manner. The fractions were then concatenated into 12 fractions by combining 8 fractions each of which were 12 fractions apart. These concatenated 12 fractions were dried down at 35 °C in a Speedvac system and resuspended in 0.1% formic acid for LC-MS/MS analysis. One third of each fraction was analyzed by LC-MS/MS in each run as described below.
Liquid chromatography tandem mass spectrometry (LC-MS/MS)
LC-MS/MS parameters used have been published previously by our group18 and were used with the following modifications for the current study. 20 early fractions from SEC selected based on the UV profile (214 nm) of the earliest eluting peptides, and 12 fractions of LAC eluate fractionated by bRPLC were analyzed by Q Exactive HF mass spectrometer (Thermo Fisher Scientific). Before MS analysis peptides were separated by LC using an Ultimate 3000 liquid chromatography system (Thermo Fisher Scientific). An EASY-Spray column (75 μm × 50 cm, PepMap RSCL C18, Thermo Fisher Scientific) packed with 2 μm C18 particles was used as a separating device and the column temperature was maintained at 50 °C. Solvent A was 0.1% formic acid in water and solvent B 0.1% formic acid in acetonitrile. Injected peptides were trapped on a trap column (100 mm × 2 cm, Acclaim PepMap100 Nano-Trap, Thermo Fisher Scientific) at a flow rate of 10 ml min−1. All runs were performed in triplicates with single run being 130 minutes and flow rate 300 nl min−1. The gradient used for separation was as follows: equilibration at 3% solvent B from 0 to 4 min, 3% to 25% solvent B from 4 to 100 min, 25% to 40% solvent B from 100 to 115 min, 40% to 95% sol B from 115 to 124 minutes followed by equilibration for next run at 3% sol B for 5 min. Ionization of eluting peptides was performed using an EASY-Spray source kept at an electric potential of 2.2 kV. All experiments were done in DDA mode with top 15 ions isolated at a window of 1.2 m/z and default charge state of +2. Only precursors with charge states ranging from +2 to +7 were considered for MS/MS events. Stepped collision energy was applied to fragment precursors at normalized collision energies of 15, 25, 40. MS precursor mass range was set to 375 to 2000 m/z and 100 to 2000 for MS/MS. Automatic gain control for MS and MS/MS were 106 and 5 × 105 and injection time to reach AGC were 50 ms and 100 ms, respectively. Exclude isotopes feature was set to “ON” and 30 s dynamic exclusion was applied. Data acquisition was performed with option of Lock mass (441.1200025 m/z) for all data.
Database searching and analysis
All database searching was performed using publicly available software pGlyco Version 2.2.0.19,20 A glycan database containing 8092 entries, which is automatically available with the software was used and Uniprot human reviewed protein sequences (20
432 entries) were used as a proteins sequence file for all searches. Trypsin specificity was set to fully tryptic with 3 missed cleavages allowed. Precursor tolerance was set to 5 ppm and fragment tolerance to 20 ppm. Cysteine carbamidomethylation was set as fixed modification and oxidation of methionine, protein N-terminal acetylation, deamidation of glutamine and conversion of Gln to pyro-Gln were set as variable modifications. The results were filtered at 1% FDR at peptide, glycan and glycopeptide levels. Glycopeptide PSM lists were reduced to unique glycopeptides per search for further manual analysis. Individual spectra were manually verified for quality and oxonium ions. For example, all sialic acid containing spectra were manually verified for presence of sialic acid-specific glycan oxonium ions; 274.09, 292.1 and 657.23. All core fucosylated glycopeptides spectra were checked for presence of at least one peptide + HexNAc + Fuc ion.
Immunoprecipitation of ORM1 from pooled plasma
Anti-ORM1 antibody (Novus Biologicals) was biotinylated with Sulfo-NHS biotin (Thermo Fisher) and reaction was quenched with 100 mM Tris pH 7.5 after one hour on ice. Biotinylated antibody incubated with 100 μl of plasma diluted with modified RIPA buffer (1× final concentration, No SDS) overnight at 4 °C with end-over-end rotation. Next day, protein-bound antibody was captured with streptavidin-agarose (Merck-Millipore) for 1 h and washed once with modified RIPA buffer, once with PBS and bound protein was eluted with 2% formic acid. Dried eluted protein was digested with trypsin as described above for whole plasma and glycopeptides were enriched using SEC as described above. They were analyzed by LC-MS/MS and database searching was performed essentially as described above.
Results and discussion
Unlike shotgun proteomics, glycoproteomics necessitates separation and enrichment of glycopeptides from their non-glycosylated counterparts following protease digestion to enable efficient detection. Several methods have been developed for enrichment of intact glycopeptides, prominent ones being HILIC and lectin affinity chromatography. While lectin affinity chromatography is a more direct method of glycopeptide enrichment, it is not a comprehensive enrichment technique when performing global glycoproteomics owing to narrow specificity of lectins. Despite the use of multiple lectins for broad application, extensive fractionation following enrichment becomes necessary to increase depth and coverage, increasing turnaround time of the workflow. HILIC, on the other hand, suffers from non-selectivity and co-enrichment of hydrophilic peptides such as serine/threonine/tyrosine containing non-glycosylated peptides presents a challenge when analyzing very complex mixtures such as plasma. For enrichment, we focused on size as a distinct feature of glycopeptides compared to non-glycosylated peptides. It is known that tryptic N-glycopeptides are typically larger than most non-glycosylated peptides. For deep glycopeptide profiling, we adapted SEC by carefully selecting the fractionation range of SEC column and optimized the flow rate and run times for very complex mixtures (undepleted plasma) for simultaneous enrichment and fractionation.
Size-exclusion chromatography for enriching intact glycopeptides
We developed a generalized workflow to analyze glycopeptides from small volumes of plasma (Fig. 1) while avoiding the technical variability introduced by depletion of abundant proteins. We started with 25 μl of whole pooled plasma (2 mg of total protein from pooled plasma), and the resulting tryptic peptides were fractionated using a size-exclusion column (fractionation range 3000–7000 Da), and 20 early fractions containing glycopeptides (Fig. 2A) were collected.
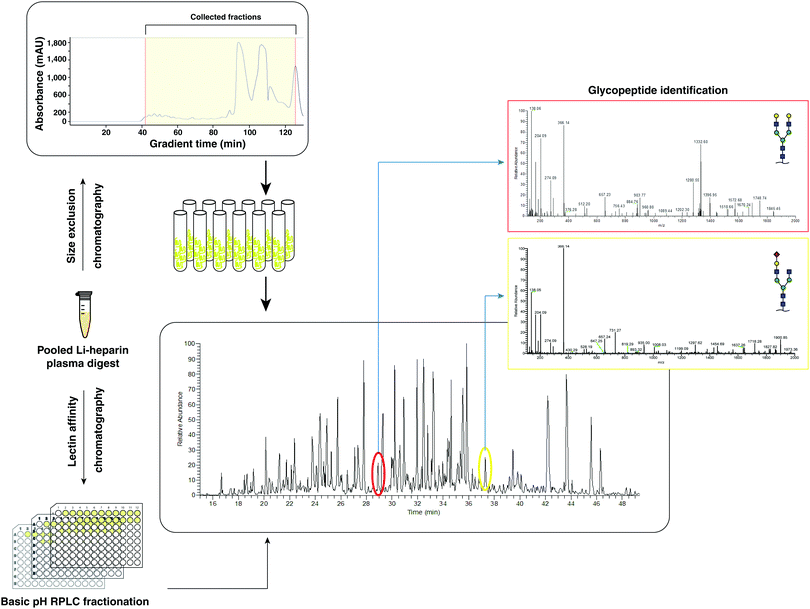 |
| Fig. 1
N-Glycoproteomics workflow. A schematic workflow of size-exclusion chromatography, SEC-LC-MS/MS and lectin affinity chromatography-basic pH reversed phase liquid chromatography, LAC-bRPLC-LC-MS/MS that was employed is shown. | |
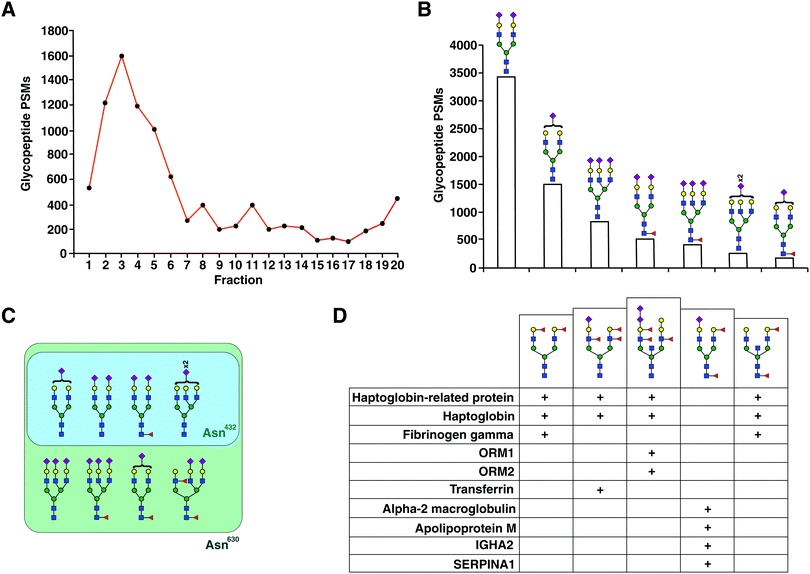 |
| Fig. 2 Distribution of glycopeptides. (A) N-Glycopeptide PSMs identified per fraction of size-exclusion chromatography in early fractions as indicated. (B) The number of N-glycopeptide PSMs based on the category glycan structures as shown. (C) Different plausible glycan structures identified at two different sites of serotransferrin (Asn432 and Asn630). All structures identified at Asn630 were also identified at Asn432. (D) Selected branch fucosylated structures and corresponding proteins from which they were derived are shown. | |
LC-MS/MS analysis for identifying glycopeptides
An aliquot of each fraction was analyzed by LC-MS/MS in a data dependent mode. We identified 1317 non-redundant N-glycopeptides from 266 glycosylation sites belonging to 154 plasma glycoproteins (Table S1, ESI†). These glycopeptides harbored 103 unique glycan compositions and 243 unique proposed glycan structures. 317 were isobaric glycopeptides with the same composition but different retention times, leaving 1042 peptide–glycan composition combinations. The glycopeptides contained all classes of N-glycans whose distribution was in agreement with recent reviews of the literature,14,15 thus reducing concerns of any kind of glycan structure bias in enrichment. Significantly smaller number of steps without cleanup and higher throughput by using the automated sampling and fractionation modules in HPLC systems (2 h per sample) are the strengths of the current workflow. 97 glycoproteins contained at least 2 glycopeptides, while some had a much higher number attributed to individual glycoprotein (Fig. S1B, ESI†). The top 15 contributing glycoproteins had glycopeptide numbers ranging from 23 (haptoglobin-related protein) to 101 glycopeptides (alpha-1-acid glycoprotein 1) per protein (Fig. 3). Out of the 266 glycosylation sites, 207 glycosylation sites from 104 proteins were annotated in Uniprot and/or GlyConnect databases, while 39 sites from 38 proteins were novel.
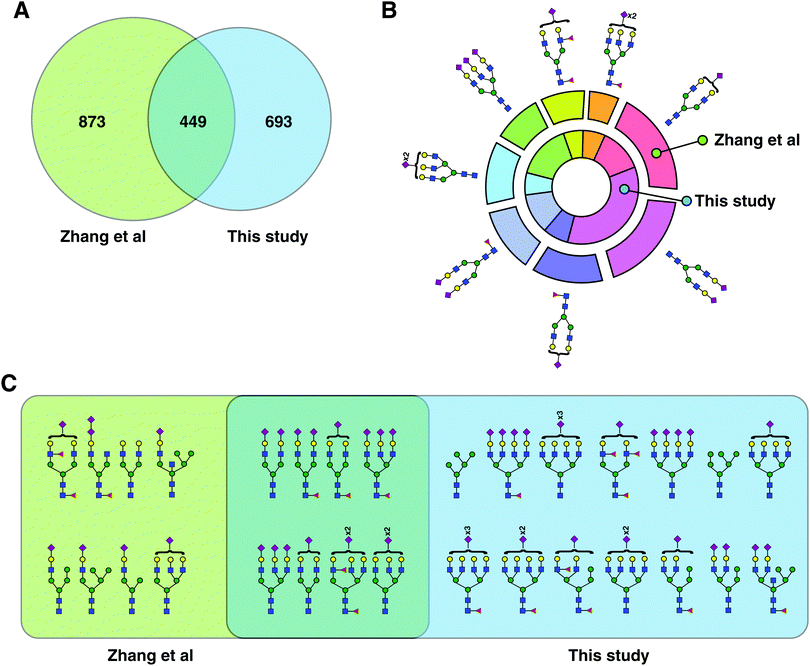 |
| Fig. 3 Comparison with previous glycopeptide studies. (A) Venn diagram comparing the identified glycopeptides in this study with those by Zhang et al.14 (B) From the glycopeptides unique to Zhang et al. and this study, eight common glycans structures were chosen, and their frequency distribution is shown. (C) All plausible glycan structures found on the unusual glycosylation motif, NXC, were extracted and compared. Eight plausible structures were common to both datasets while 14 were unique to this study and eight to Zhang et al. | |
Of the 59 sites not confirmed by Uniprot or GlyConnect DB, 13 glycosylation sites (derived from 13 proteins) are predicted to be glycosylated based on automated sequence annotation in Uniprot database. Thus, our study confirms the presence of glycans on these glycosylation sites from 13 proteins. These proteins include APO-B100 (Asn4431), lumican (Asn127), low-density lipoprotein receptor-related protein 1B (Asn1209) and pregnancy zone protein (Asn54) among others. 46 glycosylation sites from 44 proteins were further searched in database of Asian Community of Glycoscience and Glycotechnology (ACGG-DB) out of which, 39 glycosylation sites from 38 proteins were found to be neither known nor predicted to be glycosylated. The data were further searched with semi-trypic specificity and 348 additional glycopeptides (82 additional glycosylation sites) were identified (1387 unique glycopeptides, Table S2, ESI†).
The distribution of the principal types of N-glycans identified as part of 1042 glycopeptides (based on glycan compositions) was 89% complex glycans, 8% hybrid glycans and 2% high mannose (Fig. S1A, ESI†). One glycopeptide from fibrinogen gamma (Asn78) was occupied by the N-glycan core structure. 91% of all glycopeptides were sialylated, of which 92% were complex type glycans and 8% hybrid. Of all the glycopeptides 38% were fucosylated out of which 94% were both sialylated and fucosylated. Out of all fucosylated glycopeptides, 79% were found to be core fucosylated only, while 21% were both core and branch fucosylated. One glycopeptide of alpha-1-antichymotrypsin (Asn271) had four fucoses (Lewis b/y structure). Bisecting GlcNAc was found in 8% of all glycopeptides. 12 glycopeptides belonging to 8 glycoproteins including alpha-1-acid glycoprotein and haptoglobin were found to have a di-sialic acid terminal motif.
Comparison of SEC with LAC-bRPLC and other published methods on plasma
In a separate experiment, peptides obtained from 100 ml of plasma were loaded onto spin columns containing equal amounts of four different immobilized lectins. These lectins comprise most possible glycan binding capabilities that are required to enrich major N-glycan types. After elution, these intact glycopeptides were fractionated by basic pH RPLC and 12 fractions were analyzed by LC-MS/MS.
478 glycopeptides were identified (Table S3, ESI†) and 164 were occupied by isobaric glycans leaving 314 non-redundant peptide–glycan composition combinations occupying 84 glycosylation sites on 53 proteins. Of these, 4 sites identified by LAC had no evidence of glycosylation on Uniprot, ACGG-DB and Glyconnect. These inlcuded integrator subunit complex 1 (Asn345), dynamin-3 (Asn674), peptide N-glycanse 1 (Asn117) and zinc finger protein 678 (Asn251). One novel glycosylation site, Asn3336 of apolipoprotein B-100 was uniquely found in LAC-bRPLC analysis. Out of the 313 glycopeptides identified by LAC-bRPLC, 72% contained complex type glycans and 15% were hybrid type and 12% were occupied by high-mannose type of gycans. This was in contarst to 89%, 8% and 2% respectively found by SEC. Out of all the complex type glycopeptides identified by both SEC and LAC-bRPLC, 77% were unique to SEC while 6% were unique to LAC-bRPLC while 17% were common. Of the identified high-mannose glycan carrying glycopeptides, 43% were unique to LAC-bRPLC method and 18% were unique to SEC while 39% were common to both the methods. In hybrid type glycan-bearingglycopeptides, 57% were unique to SEC, 22% to LAC-bRPLC and 21% were common to both the methods. In a composite analysis, considering all glycopeptides identified by both the methods rergardless of glycan class, SEC clearly outperfromed LAC-bRPLC as 73% glycopeptides were unique to SEC while only 9% were unique to LAC-bRPLC (Fig. S2A, ESI†) with a 19% overlap between the two methods. In other words, 66% glycopeptides identified by LAC-bRPLC were also identified by SEC, while LAC-bRPLC could identify only 20% of those identified by SEC demontsrating superiority of SEC. Out of the 57 unique glycan compositions found by LAC-bRPLC, 51 were also found by SEC in addition to the 52 unique to SEC. Of 6 compositions not found by SEC, 5 were hybrid type structures with 4 of them fucosylated, 1 linear, also fucosylated (Fig. S2B, ESI†).
A comparison of our study with a recently published study by Zhang et al. revealed that the total number of identified glycopeptides and glycosylation sites from both was comparable despite lack of any depletion or additional fractionation steps.14 Zhang et al. reported 1330 glycopeptides (based on glycan composition at given sites) from control plasma, starting from 200 μl of plasma. This study looked at employing combinatorial peptide ligand libraries followed by HILIC enrichemnt of intact glycopeptides and reported the biggest intact glycopeptide dataset. The authors concentrated low-abundance glycoproteins by washing off excess of high-abundance proteins, thereby reducing the dynamic range of the proteins, potentially leading to more identifications. In comparison, our study reports 1042 intact glycopeptides at the glycan composition level, from low amounts of plasma employing simultaneous enrichment/fractionation of glycopeptides using size-exclusion chromatography. LAC-bRPLC, on the other hand, was performed on 100 μl of starting amount of plasma and led to identification of 313 non-redundant glycopeptides based on glycan compositions. Reflecting on intact glycopeptide levels, CPLL, identified 238 glycosylation sites, while our SEC data identified 266 sites with 140 overlapping sites between the two datasets. Ninety eight were unique to CPLL while 126 sites were unique to SEC dataset. Comparing CPLL's 1332 glycopeptides with 1042 of our SEC dataset, 915 glycopeptides are unique to CPLL and 635 unique to this study but when LAC-bRPLC glycopeptides were also considered, 873 were unique to CPLL and 693 to this study with an overlap of 449 glycopeptides (Fig. 3A). When we examined glycopeptides with a relatively rare NXC motif, our study had 14 unique glycan structures compared to 8 unique to CPLL (Fig. 3C). However, if we look at the unique glycan compositions, our study had a much bigger overlap (46%) between the two datasets. Out of all glycopeptides, the distribution of complex/hybrid type glycan containing glycopeptides and high mannose glycopeptides were similar between CPLL and our study. The second largest previous study on human serum glycoproteomics used a spectral library-like database to find 1359 unique glycopeptides.15 When compared to our SEC dataset, only 18% overlap was found with 49% being unique to their study and 34% to this study (Fig. S3, ESI†). Once again, the enrichment method was HILIC again confirming that these two methods are complementary and future studies should consider applying them in tandem to go further into complex samples such as plasma/serum.
Unusal glycosylation motifs found in plasma glycoproteins
Canonical N-glycosylation sequence motif NXS/T was found in 98% of glycopeptides (36% NXS and 62% NXT) in our SEC dataset while the relatively rare non-canonical sequence motif NXC, which has also been described, was observed in 2% of glycopeptides. This follows the trend and distribution of N-glycosylation motifs reported from previous studies on plamsa/serum.14,15 An unsual motif of NXV has also been reported15 and we wished to determine whether our workflow could detect the glycans occupying this motif. pGlyco 2.0 is programmed to search NXS/T/C motifs for potential glycosylation by converting N to symbol J. To search our data for NXV sequences, we manually converted the NXV motif conatining sequences to JXV in our fasta files and another search was conducted. Using this approach, we found 18 glycopeptides containing glycans at the sites in NXV motif in 14 sites of 13 proteins (Table S4, ESI†). These proteins inlcuded serum albumin (Asn68), apolipoprotein B-100 (Asn2973), emilin-2 (Asn739), alpha-2-macroglobulin (Asn1413), dynamin-3 (Asn664), Complement C1q subcomponent subunit A (Asn129), coagulation factor V (Asn817), alpha-1-B glycoprotein (Asn63 and Asn267) and inter-alpha-trypsin inhibitor heavy chain H4 (Asn274). Four instances were high mannose glycan (Man6, Man5) while the remaining were complex type glycans with Hex5HexNAc4Sia2 being the major glycan type on these non-canonical sites. The database search for our data looking particularly at NXV sequon containing glycopeptides found 18 glycopeptides as confidently identified. Only a handful of such glycoproteins have been identified by our study and two previous studies including serum albumin (Asn68).14,15 The effect of glycosylated albumin on its biological properties as transporter/carrier in plasma has not yet been studied. However, parallels can be drawn from studies on glycated albumin, which changes the conformation upon glycation and is catabolized faster.23 One more study reported yet another non-canonical sequon (NXG) in CH1 constant domain of IgG1 and IgG2 recombinant human antibodies produced in mammalian cells.24
Extenstive heterogeneity in ORM1 glycosylation
We identified 101 unique glycopeptides at four glycosylation sites of orosomucoid (ORM1 or alpha-1-acid glycoprotein 1) in our SEC workflow. Appreciating this large microheterogeneity and to validate these results, we immunoprecipitated ORM1 from pooled plasma of 50 controls. We analyzed six early fractions of SEC and found a total of 140 unique glycopeptides validating 45 preciously identified and identifying 95 more glycopeptides. These structures are shown in Fig. 4 mapped to their corresponding glycosylation sites. Ninety one out of these 95 glycopeptides were sialylated including seven diasialic acid containing glycopeptides. This distribution followed the same trend for ORM1 glycopeptides as seen in the SEC datatset.
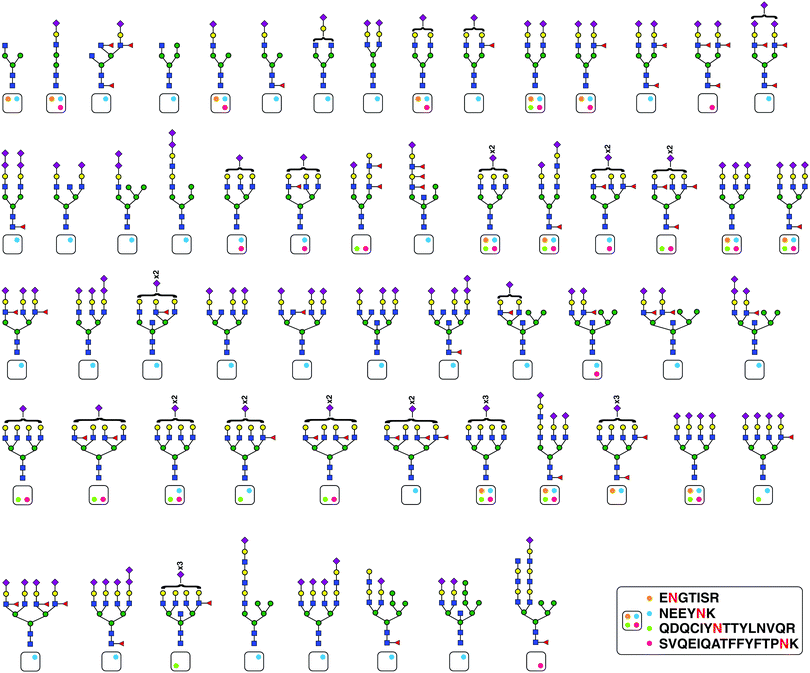 |
| Fig. 4 ORM1 (alpha-1-acid glycoprotein) exhibits extensive glycan structure microheterogeneity. ORM1 protein was immunoprecipitated form plasma and glycans structures found at four different sites are shown. The four glycosylation sites on which these structures were found are shown as color-coded circles in a box and for every glycan plausible structure, the glycosylation site is indicated. | |
Conclusions
In summary, we report a large number of previously unreported glycopeptides including those previously undiscovered to be modified by di-sialic acids. Further, SEC enriches and fractionates a previously not reported set of glycopeptides. HILIC and SEC complement each other as enrichment techniques with only modest overlap in identified set of glycopeptides which warrants their use in tandem in future studies for ultra-deep coverage. In conclusion, our workflow is ideally suited to simultaneously enrich/fractionate plasma glycopeptides for deep glycoproteomics of very-low amounts of plasma. This is particularly helpful for conditions where sample amount is a limitation such as neonatal diseases including congenital disorders of glycosylation. Clinically and biologically important hypothesis generation and validation studies employing this workflow to utilize glycoproteomics for discovery and mechanistic studies can be envisaged and are planned in our laboratory. Although SEC is a robust method for simultaneous enrichment/fractionation of N-glycopeptides from complex biological samples, it enables a qualitative assessment of the glycoproteome as described in this study. As many biological studies involve multiple samples that are compared to each other, development of quantitative workflows in the context of SEC enrichment and fractionation will greatly enhance the application of this workflow to a broad variety of biomedical applications.
Funding
This work was supported by DBT/Wellcome Trust India Alliance Margdarshi Fellowship grant (IA/M/15/1/502023) awarded to Akhilesh Pandey.
Data availability
The mass spectrometry proteomics data have been deposited to the ProteomeXchange Consortium21via the PRIDE22 partner repository with the dataset identifier PXD025414.
Conflicts of interest
There are no conflicts to declare.
References
- B. Muthusamy, G. Hanumanthu, S. Suresh, B. Rekha, D. Srinivas, L. Karthick, B. M. Vrushabendra, S. Sharma, G. Mishra, P. Chatterjee, K. S. Mangala, H. N. Shivashankar, K. N. Chandrika, N. Deshpande, M. Suresh, N. Kannabiran, V. Niranjan, A. Nalli, T. S. Prasad, K. S. Arun, R. Reddy, S. Chandran, T. Jadhav, D. Julie, M. Mahesh, S. L. John, K. Palvankar, D. Sudhir, P. Bala, N. S. Rashmi, G. Vishnupriya, K. Dhar, S. Reshma, R. Chaerkady, T. K. Gandhi, H. C. Harsha, S. S. Mohan, K. S. Deshpande, M. Sarker and A. Pandey, Proteomics, 2005, 5, 3531–3536 CrossRef CAS PubMed.
- P. Ping, T. M. Vondriska, C. J. Creighton, T. K. Gandhi, Z. Yang, R. Menon, M. S. Kwon, S. Y. Cho, G. Drwal, M. Kellmann, S. Peri, S. Suresh, M. Gronborg, H. Molina, R. Chaerkady, B. Rekha, A. S. Shet, R. E. Gerszten, H. Wu, M. Raftery, V. Wasinger, P. Schulz-Knappe, S. M. Hanash, Y. K. Paik, W. S. Hancock, D. J. States, G. S. Omenn and A. Pandey, Proteomics, 2005, 5, 3506–3519 CrossRef CAS PubMed.
- N. L. Anderson and N. G. Anderson, Mol. Cell. Proteomics, 2002, 1, 845–867 CrossRef CAS PubMed.
- H. Molina, J. Bunkenborg, G. H. Reddy, B. Muthusamy, P. J. Scheel and A. Pandey, Mol. Cell. Proteomics, 2005, 4, 637–650 CrossRef CAS PubMed.
- A. Johansson, S. Enroth, M. Palmblad, A. M. Deelder, J. Bergquist and U. Gyllensten, Proc. Natl. Acad. Sci. U. S. A., 2013, 110, 4673–4678 CrossRef CAS.
- M. F. te Pas, S. J. Koopmans, L. Kruijt, M. P. Calus and M. A. Smits, PLoS One, 2013, 8, e73087 CrossRef CAS.
- D. J. Harney, A. T. Hutchison, L. Hatchwell, S. J. Humphrey, D. E. James, S. Hocking, L. K. Heilbronn and M. Larance, J. Proteome Res., 2019, 18, 2228–2240 CrossRef CAS PubMed.
- B. Lehallier, D. Gate, N. Schaum, T. Nanasi, S. E. Lee, H. Yousef, P. Moran Losada, D. Berdnik, A. Keller, J. Verghese, S. Sathyan, C. Franceschi, S. Milman, N. Barzilai and T. Wyss-Coray, Nat. Med., 2019, 25, 1843–1850 CrossRef CAS PubMed.
- A. Kirwan, M. Utratna, M. E. O'Dwyer, L. Joshi and M. Kilcoyne, BioMed. Res. Int., 2015, 2015, 490531 Search PubMed.
- I. Belczacka, M. Pejchinovski, M. Krochmal, P. Magalhaes, M. Frantzi, W. Mullen, A. Vlahou, H. Mischak and V. Jankowski, Proteomics: Clin. Appl., 2019, 13, e1800111 Search PubMed.
- M. Saraswat, A. Makitie, T. Tohmola, A. Dickinson, S. Saraswat, S. Joenvaara and S. Renkonen, Proteomics: Clin. Appl., 2018, 12, e1800061 Search PubMed.
- S. Joenvaara, M. Saraswat, P. Kuusela, S. Saraswat, R. Agarwal, J. Kaartinen, A. Jarvinen and R. Renkonen, PLoS One, 2018, 13, e0195006 CrossRef PubMed.
- H. Jiang, H. Desaire, V. Y. Butnev and G. R. Bousfield, J. Am. Soc. Mass Spectrom., 2004, 15, 750–758 CrossRef CAS PubMed.
- Y. Zhang, Y. Mao, W. Zhao, T. Su, Y. Zhong, L. Fu, J. Zhu, J. Cheng and H. Yang, J. Proteome Res., 2020, 19, 655–666 CrossRef CAS PubMed.
- S. Sun, Y. Hu, L. Jia, S. T. Eshghi, Y. Liu, P. Shah and H. Zhang, Anal. Chem., 2018, 90, 6292–6299 CrossRef CAS PubMed.
- J. Nilsson, U. Ruetschi, A. Halim, C. Hesse, E. Carlsohn, G. Brinkmalm and G. Larson, Nat. Methods, 2009, 6, 809–811 CrossRef CAS.
- G. Alvarez-Manilla, J. Atwood, 3rd, Y. Guo, N. L. Warren, R. Orlando and M. Pierce, J. Proteome Res., 2006, 5, 701–708 CrossRef CAS PubMed.
- D. G. Mun, S. Renuse, M. Saraswat, A. Madugundu, S. Udainiya, H. Kim, S. R. Park, H. Zhao, R. S. Nirujogi, C. H. Na, N. Kannan, J. R. Yates, 3rd, S. W. Lee and A. Pandey, Anal. Chem., 2020, 92, 14466–14475 CrossRef PubMed.
- W. F. Zeng, M. Q. Liu, Y. Zhang, J. Q. Wu, P. Fang, C. Peng, A. Nie, G. Yan, W. Cao, C. Liu, H. Chi, R. X. Sun, C. C. Wong, S. M. He and P. Yang, Sci. Rep., 2016, 6, 25102 CrossRef CAS PubMed.
- M. Q. Liu, W. F. Zeng, P. Fang, W. Q. Cao, C. Liu, G. Q. Yan, Y. Zhang, C. Peng, J. Q. Wu, X. J. Zhang, H. J. Tu, H. Chi, R. X. Sun, Y. Cao, M. Q. Dong, B. Y. Jiang, J. M. Huang, H. L. Shen, C. C. L. Wong, S. M. He and P. Y. Yang, Nat. Commun., 2017, 8, 438 CrossRef PubMed.
- E. W. Deutsch, N. Bandeira, V. Sharma, Y. Perez-Riverol, J. J. Carver, D. J. Kundu, D. Garcia-Seisdedos, A. F. Jarnuczak, S. Hewapathirana, B. S. Pullman, J. Wertz, Z. Sun, S. Kawano, S. Okuda, Y. Watanabe, H. Hermjakob, B. MacLean, M. J. MacCoss, Y. Zhu, Y. Ishihama and J. A. Vizcaino, Nucleic Acids Res., 2020, 48, D1145–D1152 CAS.
- Y. Perez-Riverol, A. Csordas, J. Bai, M. Bernal-Llinares, S. Hewapathirana, D. J. Kundu, A. Inuganti, J. Griss, G. Mayer, M. Eisenacher, E. Perez, J. Uszkoreit, J. Pfeuffer, T. Sachsenberg, S. Yilmaz, S. Tiwary, J. Cox, E. Audain, M. Walzer, A. F. Jarnuczak, T. Ternent, A. Brazma and J. A. Vizcaino, Nucleic Acids Res., 2019, 47, D442–D450 CrossRef CAS PubMed.
- M. C. Wagner, J. Myslinski, S. Pratap, B. Flores, G. Rhodes, S. B. Campos-Bilderback, R. M. Sandoval, S. Kumar, M. Patel, Ashish and B. A. Molitoris, Am. J. Physiol. Renal. Physiol., 2016, 310, F1089–1102 CrossRef CAS PubMed.
- J. F. Valliere-Douglass, P. Kodama, M. Mujacic, L. J. Brady, W. Wang, A. Wallace, B. Yan, P. Reddy, M. J. Treuheit and A. Balland, J. Biol. Chem., 2009, 284, 32493–32506 CrossRef CAS PubMed.
Footnote |
† Electronic supplementary information (ESI) available. See DOI: 10.1039/d1mo00132a |
|
This journal is © The Royal Society of Chemistry 2021 |
Click here to see how this site uses Cookies. View our privacy policy here.