DOI:
10.1039/D0RA01484B
(Review Article)
RSC Adv., 2020,
10, 18451-18468
Antibiotic resistance: bioinformatics-based understanding as a functional strategy for drug design
Received
15th February 2020
, Accepted 1st May 2020
First published on 14th May 2020
Abstract
The use of antibiotics to manage infectious diseases dates back to ancient civilization, but the lack of a clear distinction between the therapeutic and toxic dose has been a major challenge. This precipitates the notion that antibiotic resistance was from time immemorial, principally because of a lack of adequate knowledge of therapeutic doses and continuous exposure of these bacteria to suboptimal plasma concentration of antibiotics. With the discovery of penicillin by Alexander Fleming in 1924, a milestone in bacterial infections' treatment was achieved. This forms the foundation for the modern era of antibiotic drugs. Antibiotics such as penicillins, cephalosporins, quinolones, tetracycline, macrolides, sulphonamides, aminoglycosides and glycopeptides are the mainstay in managing severe bacterial infections, but resistant strains of bacteria have emerged and hampered the progress of research in this field. Recently, new approaches to research involving bacteria resistance to antibiotics have appeared; these involve combining the molecular understanding of bacteria systems with the knowledge of bioinformatics. Consequently, many molecules have been developed to curb resistance associated with different bacterial infections. However, because of increased emphasis on the clinical relevance of antibiotics, the synergy between in silico study and in vivo study is well cemented and this facilitates the discovery of potent antibiotics. In this review, we seek to give an overview of earlier reviews and molecular and structural understanding of bacteria resistance to antibiotics, while focusing on the recent bioinformatics approach to antibacterial drug discovery.
1. Introduction
The use of antimicrobial entities dates back to ancient civilization1 during which a variety of naturally available healing methods were used in curbing infections.1 Some of these methods require the use of moulds and plant extracts with characteristics antibiotic properties. This is true for compounds that were accidentally discovered (such as penicillin by Alexander Fleming in 1924) and even for those that are linked to the knowledge handed down by successive generations; Egyptians applied mouldy bread to infected wounds.2
However, until the 20th century, bacterial infections were the number one cause of human death in the developed world.2 This is as a result of dose-related issues, the knowledge of the amount required to clear the organism from the system being inadequate. Therefore, marked symptoms of bacterial infection persist until such time that a significant amount is reached, and symptoms begin to disappear. Exposure of bacteria to the inadequate dose of these agents is presumed as the number one cause of bacterial resistance, which could be a contributing factor to bacterial evolution and perhaps the origin of bacteria evolution.3
In the 1940s, antibiotics were major lifelines for treating serious infections,4 this was re-established by the successful use of penicillin in controlling bacterial infections among military personnel during the Second World War.5 Luria and Delbrück in the year 1943, conducted a fluctuation test6 and acknowledged that bacterial resistance arises from heritable changes in the bacterial cell (mutation),6 which is independent of factors such as co-habitation of the bacterial colony with the virus. Despite the advantages derived from the use of penicillin, resistance to these drugs emerged,7 such that in the 1950s, the giant strides made in antibiotics development in the prior decade were threatened.8 In response to this, new beta (β)-lactam antibiotics were discovered and eventually upheld, thereby re-establishing the initial confidence of “magic-use and magic result” antibiotics are being portrayed.5,8 Within the same decade, methicillin-resistant Staphylococcus aureus (MRSA) was first identified in the United Kingdom in 1962 and the United States in 1968.4,5 Unfortunately, resistance to most antibiotics emerged in time-course4 to the extent that even the most powerful antibiotics developed in the early 1970s like vancomycin used in managing methicillin resistance in both Staphylococcus aureus (S. aureus) and coagulase-negative staphylococci had its share of resistance4,5 in the late 1970s.
To understand the key aspect of bacterial survival, Baym et al. (2016) introduced microbial evolution and growth arena (MEGA) – plate,9 typically used to study microbial evolution. Their work suggests that evolution is not always led by the most resistant mutants; highly resistant mutant can be trapped behind more sensitive lineages.9 Baym concept of Petri dish offers an unprecedented insight into the phenomenon of antibiotic resistance.10
Resistance to antibiotics is eroding the scientific effort in keeping up the pace of antibiotics' discovery in the 21st century.11 In the past (the golden era of antibiotics drug discovery), resistance to antibiotics' was not too challenging,11 this was because scientific research was coping with the challenges of antibiotic resistance.11 However, recent events in the antibiotic resistance profile have pushed most pharmaceutical industries to rededicate their efforts in developing novel molecules used in managing chronic illnesses, this approach provides more financial benefits that guaranty their survival in monetary times. Thus, leading to drying up in the antibiotics pipeline and leaving behind a few potent molecules that may limit the choice of antibiotics in curing certain disease conditions. The emergence and widespread of antibiotic-resistant pathogens is an emerging challenge in the public health domain, this requires global action with a multi-dimensional approach that would address the fundamental challenges inherent in curbing drug-resistance and fine-tune the lead way to minimise drug-resistance.12
The most recent World Economic Forum Global Risks reports have listed antibiotic resistance as one of the greatest threats that put the health system under new pressure.13 Similarly, the United Nations (UN) ad hoc interagency coordinating group on antimicrobial resistance in one of its recent report warned that drug-resistant diseases could account for up to 10 million deaths each year by 2050 and damage to the economy as catastrophic as the 2008–2009 global financial crisis. By 2030, it is likely that antimicrobial resistance could drag up to 24 million people into extreme poverty.14 Currently, at least 700
000 people die each year due to drug-resistant diseases, including 230
000 people who die from multidrug-resistant tuberculosis.14 United State (U.S.) Centers for Disease, Control and Prevention in its 2019 report, states that more than 2.8 million antibiotic-resistant infections occur in the U.S. each year,15 and more than 35
000 death result from this menace.15 Also, 223
900 cases of Clostridioides difficile occurred in 2017 and at least 12
800 people died. Relative to this, there has been an upsurge in antibiotic resistance profile and relative lack of new antibiotics in development.15
Nevertheless, bacteria resistant to antibiotics might be acquired through gene mutation and by horizontal gene transfer; it can also be due to intrinsic activities resulting from inherent structural or functional characteristics (Fig. 1). Unavailability of the susceptible target of a specific antibiotic is a typical example of intrinsic resistance, this is well represented in gram stain organism, with Gram-negative bacteria having a lower proportion of anionic phospholipids in the cytoplasmic membrane than do Gram-positive bacteria.16 This reduces the efficiency of the Ca2+ – mediated insertion of daptomycin into the cytoplasmic membrane that is required for its antibacterial activity.17 The intrinsic properties of some Gram-negative bacteria give them protective measures that allow them to resist antibiotic effects such as antibiotic transport across the outer membrane. For example, the glycopeptide antibiotic vancomycin inhibits peptidoglycan cross-linking by binding to target D-Ala-D-Ala peptides, this effect is more pronounced in Gram-positive bacteria, whereas in Gram-negative organisms, the drug transport across the outer membrane is hindered and access to the peptides in the periplasm is shutdown.18 The word antibiotic has become synonymous with antibacterial drugs therefore, in this article; the term antibacterial refers to the antibiotic.
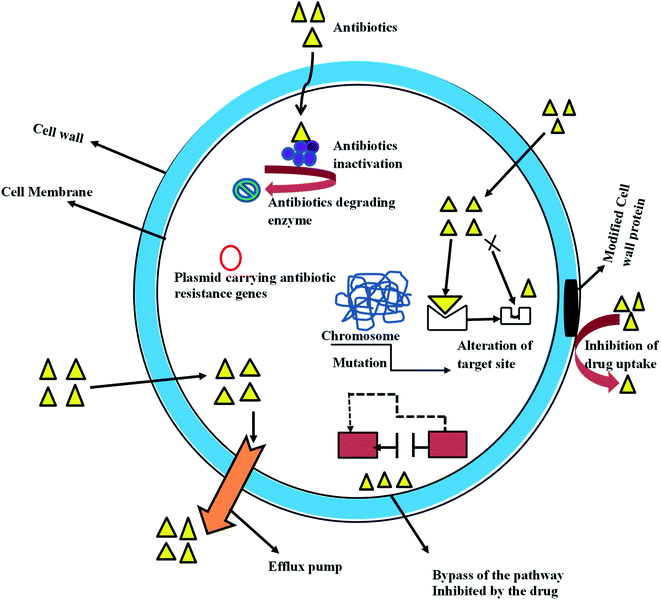 |
| Fig. 1 Evolution of antibiotic resistance in bacteria cell. | |
Bioinformatics is a practical discipline that employs a range of computation techniques including sequence and structural alignment, analyses of large collections of biological data, such as genetic sequences, cell populations or protein samples, to make new predictions or discover new biology.19 The application of bioinformatics tools and techniques in analysing the increasing data generated in molecular biology, genomics, transcriptomics, and proteomics is gaining momentum.20 Furthermore, the quantum of information aggregated in the form of databases and literature for generating molecular profiles and for collecting data related epidemiology of pathogens has significantly increased.21 Therefore, the use of bioinformatics tools and techniques in curbing bacteria resistance, pathogen identification and typing, identifying markers for early diagnosis and treatment, enabling personalized interventions and predicting patient outcomes, is crucial.22 Herein, we provide an overview of previous reviews, molecular and structural understanding of bacteria resistance to antibiotics while focusing on the recent bioinformatics approach to antibacterial drug discovery.
1.1 Antibiotic resistance; what we need to know
Bacteria resistance to antibiotics is relative in terms of the dose of exposure to antibiotic,23 this is so because even the most resistant bacterium could be inhibited or killed by a sufficiently high concentration of antibiotics.23 However, many patients might be unable to tolerate the high concentration required to kill/inhibit the bacteria, this is further complicated by variation in the susceptibility profile of different bacteria. For example, most strains of Streptococcus pneumoniae in Britain have a minimum inhibitory concentration (MIC) of 0.01 mg L−1 with benzylpenicillin.23 Whereas for Escherichia coli, 32–64 mg L−1 is ideal to inhibit bacteria growth;23 a concentration that is likely difficult to meet in human plasma, which would prevent thriving drug-resistance.23 Hence, resistance to antibiotics can be better considered as clinical resistance as opposed to drug resistance. Similarly, the rapid spread of the transposon (jumping DNA) drives bacterial resistance. This DNA is a vehicle for spreading of bacteria resistance and characterise with the autonomous switch of locations in the genome.24
Antibiotics resistance is best understood using two conceptual models;25 first, resistance to antibiotics could be considered as a result of microorganisms (bacteria) interaction with their immediate environment.25 The presence of these molecules, some of which are natural compounds, within the bacteria milieu exert the survival pressure that allows the bacteria to adapt to a certain concentration, hence, co-resident bacteria evolved mechanisms to overcome these molecules' actions to survive. Therefore, these organisms are often considered as “intrinsically” resistant to one or more antibiotics.25 However, in clinical settings, the focus is more on “acquired resistance” in a bacterial population that was originally susceptible to the antibiotics.25 Usually, acquired resistance results from mutations in chromosomal genes or perhaps due to acquired external genetic determinants of resistance,25 which is likely obtained from intrinsically resistant organisms present in the environment.25
In the second model, antibiotics resistance/susceptibility in clinical practice could be considered as a relative phenomenon with different strata.25 The establishment of clinical resistance or susceptibility endpoints (susceptible, intermediate and resistant) depends on the in vitro activity of antibiotics against a particular bacterial population, combined with some pharmacodynamics parameters.25 Thus, when treating antibiotic-resistant bacteria, susceptibility patterns interpretations may vary according to the clinical scenario and treatment options availability.25 For instance, the plasma concentration of cefuroxime achieved in the lungs could be sufficiently high to treat upper respiratory tract infection caused by an organism reported as cefuroxime-resistant.25 However, the in vivo susceptibility of an organism to a particular antibiotic depends on the dose of bacterial inoculum.25 As noted from research, some cephalosporins (like cefazolin) may fail in the setting of high-inocula (deep-seated infections) caused by cephalosporin-susceptible S. aureus. This situation has been well documented in S. aureus infections with some cephalosporins.26
2. Molecular basis of antibiotics resistance
Bacteria genome flexibility allows them to respond to a range of environmental challenges,25 including when antibiotic molecules that may distort the strength of their survival are present. Just like other organisms, bacteria share atomic (chemical structure) similarities with antibacterial agents and hence ease adaptability to the harmful effect of antibiotics.27 Two major genetic strategies define bacteria adaptability to antibiotics: (1) gene mutation and (2) horizontal transfer of resistance gene.
2.1 Resistance resulting from a gene mutation
Mutation is one of the useful means of survival of bacterial amidst antibiotics threat; it usually results from exposure of bacteria to the sub-optimal concentration of antibiotics. Once a resistant mutant emerges,27 the antibiotic eliminates the susceptible population and the resistant bacterial predominate.27 From a molecular perspective, mutation undermines the network of interaction between the atoms of the drug and atoms of the amino acid from the bacterial enzyme. Consequently, drug-receptor resident-time would be shortened and potency of the drug molecule could be affected. In many instances, mutational changes leading to resistance affects cell homeostasis27 with an irreversible decrease in drug fitness. Several mechanisms of bacteria resistance are available in the literature, the most common ones include; (1) changes in bacteria drug target site (2) reduction in drug uptake (3) activation of efflux mechanism to exclude drug molecule and (4) changes in essential metabolic pathways.
2.2 Horizontal gene transfer (HGT)
Transfer of genetic trait through DNA has been known as the most common method of bacteria resistance,25 it involves acquiring foreign DNA material through horizontal gene transfer. It is a well-known fact that bacteria share the same environmental characteristic with most pharmaceuticals, thereby developing an intrinsic resistance trait required to facilitate the growth of antimicrobial resistance. This genetic exchange could be associated with the widespread resistance to many frequently used antibiotics.27 Acquisition and transfer of genetic materials by bacterial could be achieved via three basic mechanisms, this includes transformation (incorporation of available DNA), transduction (microphage mediated) and conjugation (bacterial mating).25 Transformation is the simplest mechanism of horizontal gene transfer with only a few clinically relevant bacterial proceeds with this method. However, conjugation is an efficient mechanism of gene transfer that involves contact between two or more cells driving by free genetic elements which transfer valuable genetic information.25 Plasmid and transposons (jumping DNA) are critical to bacterial in the development and transfer of resistance genetic materials in the clinical relevant organism. Similarly, integrons have been identified as one of the most effective mechanisms of antimicrobial resistance gene assemble.27 It played a major role in the acquisition, expression, and dissemination of antibiotic resistance genes. Its mechanism is simple and efficient in adding new genes into bacterial chromosomes, along with the necessary machinery to ensure their expression25,28 (Fig. 1).
It is important to note that bacteria can develop resistance to antibiotics using multiple biochemical pathways, this is true for some bacterial that gain exposure to different types of antibiotics. They adopt these pathways to resist the effect of antibiotics and for continuous survival, this is targeted at some antibiotics perhaps because of their structural peculiarities, for example, bacteria resistance to fluoroquinolone comes with three different mechanisms.29 These are (1) alterations in the target enzymes (DNA gyrase and topoisomerase) (2) change in drug entry and efflux mechanism (3) plasmid-mediated Qnr-protein which protects quinolones targets from inhibition.29 These mechanisms may co-exist in some bacterial and can augment resistance effects of one mechanism from the others. Similarly, a selective mechanism of resistance is adequate for some bacterial to resist certain antibiotics effect, this is common with the β-lactamase producing organisms. In this case, the Gram-negative bacterial produce β-lactamases while Gram-positive bacteria adopt target-site adjustment or modification. This might be partly due to major differences in the cell envelope between Gram-negative and Gram-positive bacteria.
Generally, bacterial resistance to β-lactam antibiotics is mainly due to similarity in the structural feature of beta-lactam ring30 which is partly responsible for inhibiting the synthesis of the bacterial cell wall.30 These antibiotics target peptidoglycan cross-linked enzymes particularly transpeptidase and carboxypeptidase (penicillin-binding proteins, PBP) which are highly susceptible to autolytic effects of the beta-lactam antibiotics in Gram-negative bacteria.30 However, in Gram-positive bacteria that lack outer membrane, resistance could be attributed to penicillin-binding protein modification30 or porins (permeability barrier and low affinity of PBP to the drugs). Hence, producing inactivating enzymes (beta-lactamases) and inhibition of release of autolytic enzymes is more pronounced.30 The commonest mechanism of adaptability among bacteria is therefore presented.
3. Mechanism of antibiotics resistance
3.1 Structural modification of antibiotics molecules
Structural modification of the drug molecule is one of the adaptive mechanisms of bacterial resistance to antibiotics.31 These organisms produce enzymes that inactivate the drug by adding specific chemical moieties to the molecule itself thereby changing the molecular orientation of the drug at the target site.31 This mechanism involves the chemical replacement of a certain group or moiety by bacterial enzyme limiting the antibacterial profile and reducing the potency of antibiotics. Most antibiotics that inhibit the bacteria ribosomal subunit32 fall prey to this mechanism. Typically, aminoglycoside modifying enzymes (AMEs) presence which covalently modify the hydroxyl (–OH) or amino groups (–NH2) of the 2-deoxystreptamine nucleus or the sugar moieties could be nucleotidyltransferase, phosphotransferases, or acetyltransferases.31 This phenomenal of aminoglycoside modification is responsible for most clinical resistance to these groups of antibiotics and is the predominant mechanism of aminoglycoside resistance worldwide.33 Many AMEs have been discovered and are usually found in mobile genetic elements (MGEs).33 However, the genes responsible for AMEs coding have been found as integral of chromosomes in some bacterial species.31 This is a typical representation of different types of amino-acid modifying enzymes as seen in Fig. 2.
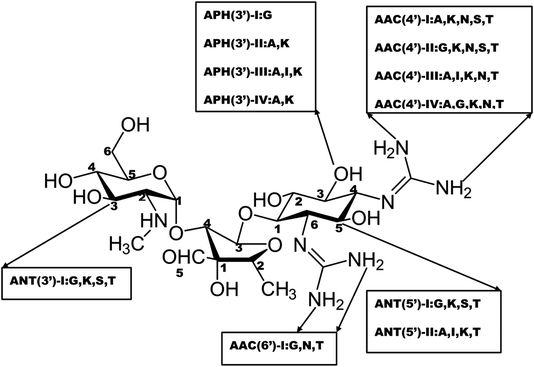 |
| Fig. 2 Nomenclatures of different types of aminoglycoside-modifying enzymes. | |
The modified entity as depicted in Fig. 2 represents different biochemical activity as acetyltransferase (AAC), adenyltransferase (ANT) and phosphotransferase (APH).34 The distribution of these enzymes varies among the bacteria and differs among aminoglycosides.34 This could be exemplified by APH family presence in Gram-positive and Gram-negative bacteria which alter the structure of kanamycin and streptomycin but spares gentamycin and tobramycin.34 In a related development, AAC is found mainly in Gram-negative clinical isolates like Enterobacteriaceae, Pseudomonas and Acinetobacter and affects most aminoglycosides including amikacin and gentamicin.33 Besides, there is a variation in the distribution and AMEs activity from the same family.33
Within the same context, the chloramphenicol is also a subject of enzyme modification; the chemical modification of chloramphenicol is mainly driven by chloramphenicol acetyltransferases (CATs) expression. These CATs genes are of different types in both Gram-positive and Gram-negative bacteria, they exist mainly as Type A which promote high-level resistance and Type B that only allows for low-level chloramphenicol resistance.35
3.2 Decreased in antibiotic penetration (an efflux mechanism)
Antibiotics targets for many bacteria are located within the cytoplasm of the bacteria cell.36 Therefore, these compounds must penetrate through the outer to the inner layer of the bacterial cytoplasm to exert its effect. Bacteria have developed a protective mechanism that prevents antibiotics from reaching their target sites. This mechanism is most common in Gram-negative bacterial where it limits the outer membrane from adequate exposure to antibiotics.37 Antibiotics with hydrophilic properties such as β-lactams, some fluoroquinolones and tetracyclines could be affected by the changes in the bacterial outer membrane permeability. They often used porin (water-filled diffusion channel) to cross the barrier,37 other antibiotics such as vancomycin has reduced effect in some Gram-positive bacterial due to antibiotics restricted mechanism (lack of penetration through the outer membrane).37 Similarly, decrease in Pseudomonas and Acinetobacter baumanii susceptibility to β-lactams (compared to Enterobacteriaceae) is partly due to decrease in number and variation in porins expression.38
3.3 Efflux pump
Bacteria resistance to antibiotics could be predicated when a mechanism of expelling drugs out of bacteria cells is available through a pump known as an efflux pump.39 This mechanism was first described in the 1980s when an efflux pump was responsible for ejecting tetracycline out of the cytoplasm of E. coli.39 A wide range of bacterial operates their resistance mechanism in this manner, this includes Gram-positive and Gram-negative organism. The mechanism is often highly specific for some substrate or may have a broad spectrum of substrate specificity which are widely found MDR bacteria.40 Because of this, a good number of antibiotics would be affected by this mechanism, this includes carbapenems, polymyxins, β-lactams, fluoroquinolones and protein synthesis inhibitors.40,41
Another important clinical relevance antibiotic resistance mediated by the efflux mechanism is that of resistance to macrolides.42 Efflux pumps are typically encoded by the macrolide efflux (mef) genes [mef(A) and mef(E)] that are responsible for extruding the macrolides class of antibiotics (such as erythromycin).42 The mef genes are mainly found in S. pyogenes, S. pneumoniae, streptococci and Gram-positive organisms.42,43 Mef(A) is usually carried in a transposon (Tn1207) within the chromosome, Mef(E) is found in the “MEGA-element” (macrolide efflux genetic assembly), which is a DNA fragment that is naturally associated with different regions of the bacterial chromosome.42 It is important to note that, macrolide resistance caused by this mechanism does not result in cross-resistance to lincosamides and streptogramins (the so-called MLSB group).42
3.4 Target site interference
Some bacteria can interfere with their target site to negate the effects of antibiotics. This is usually achieved by preventing the antibiotic from reaching its binding site or modifications of the target site resulting in a decrease in affinity to the antibiotic molecule.31
3.5 Target site protection
Some of the genetic determinants coding for the proteins that mediate target protection have been found in the bacterial chromosome, most of which are clinically relevant genes and are involved in target site protection mechanism of resistance which is often carried by MGEs.44 Examples of drugs affected by this mechanism include tetracycline [Tet(M) and Tet(O)], fluoroquinolones (Qnr) and fusidic acid (FusB and FusC).44 Tetracycline resistance is well-studied in this regard, during this period tetracycline resistance determinants Tet(M) and Tet(O) were considered. Tet(M) was only described in Streptococcus spp and Tet(O) in Campylobacter jejuni. However, their presence in other bacteria species has been also established.44
These proteins are a sub-family member of GTPases (translation factor superfamily) and act as homologues of elongation factors (EF-G and EF-Tu) used in protein synthesis.31,45,46 Interaction of Tet(O) and Tet(M) with the ribosomes dislodges the tetracycline from its binding site in a GTP-dependent manner.45,46 To understand tetracycline resistance, Dönhöfer et al. (2012) showed that Tet(M) directly dislodges and releases tetracycline from the ribosome.46 This interaction alters the ribosomal conformational space thereby preventing the rebinding of the antibiotic.46 Bacterial can adopt this mechanism of resistance to other antibiotics like quinolones.
3.6 Mutations of the target sites
Mutation is one of the major and commonest mechanisms of bacterial resistance to antibiotics,47 it involves changes in amino acid residue resulting in a defective network of amino acid interaction which eventually affects drug binding. A good example of this mechanism is in rifampin (RIF) resistance.47 Rifampin (a rifamycin) is used as a first-line antibiotic treatment for tuberculosis (TB), and it remains the cornerstone of current short-term TB treatment.47 Rifamycin-resistant (RIFR) TB,47 results from the RpoB S531L mutation in RNA polymerase (RNAP), this has become a growing problem worldwide.47 Molodtsov et al. (2017) determined the X-ray crystal structures of the E. coli RNAPs containing the most clinically important S531L mutation and two other frequently observed RIFR mutants, RpoB D516V and RpoB H526Y47 have been subjected to molecular analysis. The structures show that the S531L mutation impacts subtle if any structural or functional impact on RNAP occurs when RIF is absent,47 this could be illustrated in Fig. 3 with the binding pocket of rifampicin monooxygenase (RIFMO).
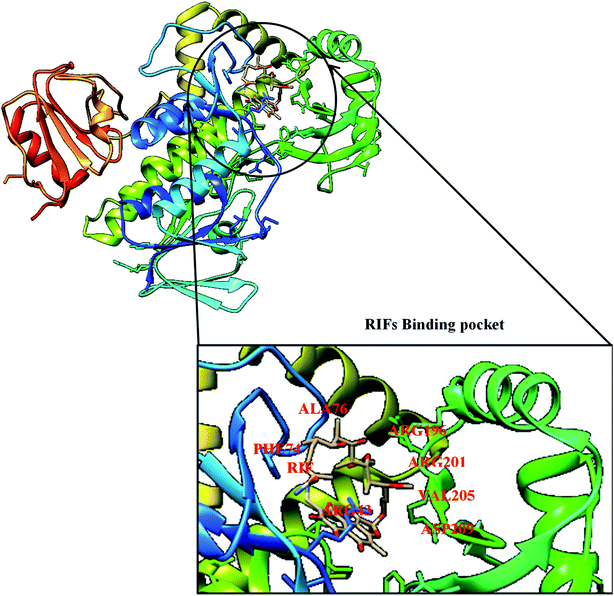 |
| Fig. 3 Crystal structure of rifampicin monooxygenase (RIFMO) with mutant residues. | |
However, upon RIF binding, the S531L mutant exhibits a disordering of the RIFMO binding interface,47 which effectively reduces the RIF affinity. In contrast, the H526Y mutation reshapes the RIFMO binding pocket, generating significant steric conflicts that essentially prevent any RIF binding.47 While the D516V mutant does not exhibit any such gross structural changes, certainly the electrostatic surface of the RIFMO binding pocket is dramatically changed, likely resulting in the decreased affinity for RIFs.47 In a related development, conversion of RIF to oxidative products causes a decrease in the potency of RIFMO.48 Further decomposition of RIF was observed in bacterial producing RIFMO and contributes to RIFMO-mediated drug resistance.48
3.7 Enzyme alteration of target sites
Alteration in the target sites of antibiotics is one of the commonest mechanism of bacterial resistance49 attracting a lot of significance, even the clinical strains showing resistance could be found in every class of antibiotic irrespective of the mechanism of action.49 Alteration at the target site often results from continuous mutation of a bacterial gene on the chromosome and selection when the antibiotics are present.49 For instance, mutations in RNA polymerase and DNA gyrase of susceptible bacterial result in rifamycins and quinolones resistance, respectively.49 Similarly, acquisition of resistance may involve the transfer of resistance genes from other organisms through the genetic exchange (conjugation, transduction, or transformation). Examples of these mechanisms include acquisition of the mecA genes encoding methicillin resistance in S. aureus and the various van genes in enterococci encoding resistance to glycopeptides.49
In a related development, ribosomal methylation of some antibiotics is catalysed by an enzyme encoded by the erm genes, this includes methylation of erythromycin ribosomes which results in macrolide resistance.50 These enzymes are capable of mono- or demethylation of an adenine residue in position A2058 of the domain V of the 23rRNA of the 50S ribosomal subunit.50 Changes resulting from this biochemical process are directly responsible for the distortion and re-orientation of the binding site that affects the drug fitness. All the antibiotics with overlapping binding sites tend to cross-resistance, this is true with macrolides, lincosamide and streptogramin B with overlapping binding sites in the 23S rRNA.50
Enzymatic alteration of the target is also involved in Cfr-mediated linezolid resistance.51,52 The plasmid-borne determinant gene (cfr gene) was initially described in the year 2000 from a bovine isolate of Staphylococcus sciuri,51 it was first reported in a human in 2005 in an S. aureus isolated from a patient in Colombia.51 Since then, it has been found in several species of human pathogens, including S. aureus, E. faecalis, E. faecium and some Gram-negative bacteria.51 This gene encodes the Cfr enzyme, which is a member of the S-adenosyl-L-methionine (SAM) methylase family that also confers resistance to phenicols, lincosamides, pleuromutilins and streptogramin A.51 Also, cfr has been found to enhance the activities of the various mobile genetic elements (MGEs) suggesting the relative capacity of transmission of linezolid resistance soon.51
3.8 Target site replacement or circumvention
In applying this strategy, bacterial evolve new targets with similar biochemical functions like the original target but are not susceptible to inhibition of antibiotics.25 This includes methicillin resistance in S. aureus due to the acquired exogenous penicillin-binding protein (PBP) and vancomycin resistance in enterococci through modifications of the peptidoglycan structure mediated by the van gene clusters.25 The microorganisms can also avoid antimicrobial action by “circumventing” the susceptible metabolic pathway resulting in an overproduced antibiotic target.25,53 A relevant example of this mechanism includes resistance to trimethoprim–sulfamethoxazole (TMP–SMX) by a “clever” bypass strategy from dihydropteroic acid synthase (DHPS) overproduction. DHPS forms dihydrofolate from para-aminobenzoic acid (inhibited by SMX), and dihydrofolate reductase (DHFR) through mutations in the promoter region of the DNA encoding these enzymes.53,54 These mutations result in excessive production of enzymes, thus, “overwhelming” the ability of TMP–SMX to inhibit the folate production thereby opening the door for bacterial survival.53,54
4. Pharmacodynamics drug resistance
Pharmacodynamics (PD) of the antibiotic is essentially a matter of the impact of antibiotics on the targeted pathogen.55 It is a complex relationship and very much affiliated with the pathogen susceptibility to a given antibiotic, it relates the ability of the antibiotic to reach the targeted tissue, achieving optimum plasma concentrations at the target site, and patient or host factors.55 Patients' underlying comorbidities, immune function status, renal and liver function status, and concomitant administration of drugs have a major impact on antibiotic PD—in part by affecting pharmacokinetics (PK) and in part by increasing susceptibility to colonization and bacterial infection,55 decreasing the ability to fight the bacterial infection.55
Toxicity is also an important issue. Therefore, it is important to balance the need to administer a high enough antibiotic dose to eradicate the pathogen keeping in mind patient safety and the ability to tolerate the drug. Because antibiotic PD depends on these various interconnected factors, delivering an effective dose is more complex than simply choosing a dose that is effective in well-controlled clinical trials.55 An antibiotic's ability to resolve an infection depends on a critical drug concentration being reached or exceeded at the infection site and exposure of the bacterial to this concentration for a time long enough for organism eradication to occur from the infection site. Therefore, a decrease or lack of antibiotic minimum inhibitory concentration (MIC) required to eradicate bacterial can cause bacterial resistance. MIC, defined as the lowest concentration of an antimicrobial agent that inhibits the growth of the wild type population, assuming no mutations, by 99% (ref. 56 and 57) has been used extensively to classify bacteria as resistant to an antibiotic.57 With an increase in antibiotic concentrations, the first steep decline in colony numbers, representing an approximately 1% recovery, corresponds to the MIC.57 After exposing cells to antibiotics at MIC levels, there will often still exist resistant mutants population due to spontaneous mutations, usually called single-step resistant mutants.57 As concentrations increase beyond the MIC, these single-step mutants will remain until a concentration that reduces colony recovery to 0% is achieved.57 Above this concentration, no single-step mutants can exist. This concentration is the second metric of resistance, the mutant prevention concentration (MPC),57 which could be defined as an interesting tool used in minimising resistance emergence by modifying antibiotic drug concentrations.55
Based on the experimental result, 3 PD parameters are commonly used to predict antibiotic efficacy (1) the ratio of maximum serum concentration to the minimum inhibitory concentration (Cmax/MIC); (2) the ratio of the area under the plasma concentration versus time curve to the minimum inhibitory concentration (AUC/MIC) and (3) the dosing time interval that plasma concentrations exceed the MIC (T·MIC).55 The Cmax/MIC ratio showed to predict aminoglycoside efficacy, AUC/MIC best describes fluoroquinolone, glycopeptide, and ketolide efficacy, and T·MIC best describes the efficacy of β-lactams and macrolides as presented in Fig. 4.
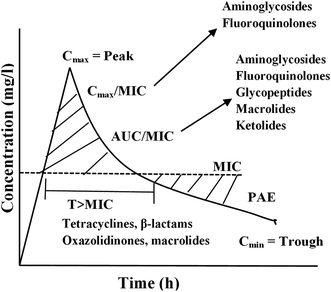 |
| Fig. 4 Important pharmacodynamics parameters describing the efficacy of different antibiotics. AUC, area under the concentration–time curve; Cmax, maximum concentration; Cmin, minimum concentration; MIC, minimum inhibitory concentration; PAE, postantibiotic effect; T, time. | |
Generally, PK and PD (PK/PD) parameters are used to predict antibiotic efficacy with an increasing interest in the use of these parameters to minimise resistance development.55 Bacterial resistance to fluoroquinolone is well described by the ‘‘mutant selection window’’, a developed hypothesis to describe how drug exposures below the mutant prevention concentration may create conditions for resistant bacterial strains selection.55 The AUC/MIC ratio has also been used to describe fluoroquinolone drug exposures associated with either increased or decreased risk of resistance emergence.
5. Pharmacokinetics drug resistance
The efficiency of antimicrobial treatment could be determined by both pharmacokinetics and pharmacodynamics.58 Pharmacokinetics is the study of the bodily absorption, distribution, metabolism and excretion of drugs.59 Antibiotic resistance is relatively associated with the various mechanisms of drug absorption, distribution (transport) and elimination (metabolism and excretion). Antibiotic PK is often considered in terms of body's effect on the drug, but physicochemical properties of these drugs must also be considered to predict its disposition.60 Most importantly is the relative solubility of the antimicrobial, which can impact significantly on its volume of distribution, and thus might be vital in selecting agents expected to attain adequate penetration to the site of infection.60 PK parameters that affect antibiotic efficacy includes;
5.1 Absorption
The ingestion of orally administered antibiotics goes through a normal route like any other oral formulation. However, this could change when permeability glycol-protein (p-gp), also known as multidrug-resistant (MDR) protein, found in the gastrointestinal tract (GIT)61 and small intestines (primary site for epithelial absorption), is present.61 Co-administration of antibiotics and anticancer drugs could lead to over-expression of p-gp thereby decreasing some of these agents' bioavailability including azithromycin61 hence, suboptimal plasma concentration. Therefore, antibiotics concentration within bacteria milieu might be quite low, hence encourages bacterial resistance. Genetic polymorphism and change in the body physiological state also play an important role in expressing p-gp in the GIT.62 Thus, causing erratic absorption of some antibiotics including actinomycin D, erythromycin, gramicidin D, rifampin, salinomycin, sparfloxacin and valinomycin.62
Generally, drugs are better absorbed in the small intestine (presence of larger surface area) than in the stomach. Therefore, gastric (stomach) emptying plays a major role in plasma drug concentrations,63 while eating stimulates gastric acid secretion into the gastric lumen. Food, especially fatty food, delays gastric emptying, slows and lowers peak plasma concentration, and may affect the drug's bioavailability and efficacy.63 Eating is a physiological process that stimulates gastric acid secretion into the gastric lumen. Antibiotics such as penicillin G, which is unstable in gastric acid, is best administered in the fasting state (i.e., a half-hour before or 2 hours after a meal). Penicillin V is also better absorbed in the fasting state.63 Amoxicillin is equally well absorbed with food or in the fasting state.63 However, when amoxicillin combines with clavulanate, enhanced absorption of clavulanate potassium would be observed when it is administered at the start of a meal. Similarly, macrolides such as azithromycin and erythromycin have low bioavailability of about 40% that could be further lowered by food availability, these drugs are best administered in fasting state for optimum plasma concentration and to avoid drug resistance.63
5.2 Distribution
To optimise dosing regimens of antibiotics, it is imperative to have a good understanding of pharmacokinetics (PK) and pharmacodynamics (PD). Drug efficacy is usually related to unbound concentrations of drug at the site of action. For antibiotics, the infection site is typically located outside plasma, and a drug should be transported across the capillary membranes to reach its target site.64 Disease- and drug-related factors can contribute to differential tissue distribution of these drugs, therefore, the impact of protein binding is of prime interest because the free, unbound drug concentrations at the site of action/infection are responsible for the drug's effect.64 Drug binding to protein can occur in intra- and extravascular spaces and is an important determinant of a drug's pharmacokinetics (PK), as it will impact distribution and elimination processes. Tissue binding increases the drug fraction outside plasma and away from systemic drug elimination organs.64 The impact of protein binding on drug efficacy will depend on the binding limit, PK properties, and intrinsic activity of the drug.65 Despite adequate total plasma levels of highly protein-bound drugs, free (i.e., active) drug concentration might be less than the MIC of the pathogen, which will require the use of higher doses.66 The clinical significance of this phenomenon showed in vitro failure of cefonicid against S. aureus but was highly protein-bound in vivo to cure endocarditis caused by S. aureus.63 When the drug reaches the site of infection, local factors determine its antibacterial activity.67 For example, the aminoglycosides and erythromycin have decreased activity at an acidic pH, such a situation occurs in an abscess.67 The aminoglycosides are also less active against facultative organisms in an anaerobic environment because aminoglycosides penetration into the bacterial cells depends on an oxygen-dependent reaction.67 Other substances such as beta-lactamases and those that may cause enzymes deactivation, might be at the site.68
5.3 Elimination
The vital organs responsible for drug elimination in the human system include; liver, kidney, bile, lung, and skin. However, the liver and the kidney are responsible for the major elimination process of the drug in the body. Drugs could be eliminated by converting them to metabolites (mainly in the liver); unchanged drugs or their metabolites are often eliminated in faeces or urine by the excretory organs, mainly the kidneys, liver, and gut.63 Some drugs or their metabolites that are usually excreted in bile would be reabsorbed into the bloodstream and recycled by a process called enterohepatic circulation.63 The aminoglycosides, tetracycline, and vancomycin are primarily excreted by glomerular filtration. More than 80% to 90% of vancomycin is often recovered unchanged in urine within 24 hours after administration of a single dose.69 Only free drugs in the plasma can pass through the glomerular filter, so drugs such as ceftriaxone that are highly bound to plasma protein stays longer in the body.69 Most fluoroquinolones are primarily eliminated by renal mechanisms and to a lesser extent, by nonrenal mechanisms, such as hepatic metabolism and transepithelial intestinal elimination. Fluoroquinolone moxifloxacin, however, is mainly eliminated by nonrenal mechanisms such as hepatic metabolism and transepithelial intestinal elimination.69 Tubular secretion occurs via two active transport mechanisms: one for anions (weak organic acids) and one for cations (weak organic bases). Competition between drugs for the carriers can occur within each transport system.69 The organic acid transport mechanism contributes to eliminating many beta-lactam antibiotics, fluoroquinolones, and some sulfonamides. Competition between probenecid and these beta-lactams, fluoroquinolones, and sulfonamides for the organic acid transport carriers can prolong the (half-life) duration of these antimicrobial drugs in the body.69
Polar substances elimination is via the renal system because they are not freely diffusible across the tubular membrane and so, remain in the urine despite the concentration gradient that favours back-diffusion into the interstitial fluid.69 While nonpolar drugs are usually eliminated after the metabolic conversion of the drugs in the liver to more polar metabolites, which cannot diffuse out of the tubular lumen and are then excreted in the urine.69 Erythromycin, azithromycin, moxifloxacin, clindamycin, rifampin, nafcillin, and cefoperazone are mainly excreted by the liver into bile; about 40% dose of ceftriaxone is often eliminated by the liver bile, but when renal failure occurs, hepatic excretion increases. Doxycycline is normally eliminated by the gut.69
6. Prevention of resistance
Studies show that drug levels should exceed at least 8 to 10 times the MIC to prevent potential antibiotic resistance, this could be accomplished by using single daily dosing of an aminoglycoside, using the most potent fluoroquinolone, or using high doses of a beta-lactam.63 In vitro and animal models of infection have identified the peak MIC ratios and free-drug 24 hour AUC/MIC ratios for fluoroquinolones capable to prevent emerging resistant subpopulations.63 The minimal prevention dose, which varies among bacterial species, is higher for denser bacterial populations and is often higher than the ratios required for efficacy.70,70
6.1 Quorum sensing
With bacterial antimicrobial resistance on the rise, the search for alternative therapeutic agents remained sustainable. One such alternative is the use of anti-virulence strategies such as quorum-sensing inhibition (QSI), which is a potentially viable treatment option for pathogenic microorganisms such as MDR S. aureus and Pseudomonas aeruginosa (P. aeruginosa).71 Quorum sensing (QS) simply means bacterial cell–cell communication process that involves the production, detection, and response to extracellular signalling molecules called autoinducers (AIs).72 Increase in bacteria population density reflects an increase in AIs in the bacteria milieu. These track changes are then monitored by microorganisms that use such information to change their cell numbers and collectively alter gene expression.72 QS monitors the genes that direct activities that are important when performed by groups of bacteria acting in synchrony.72 This controls a range of processes, that includes bioluminescence, sporulation, competence, antibiotic production, biofilm formation, and virulence factor secretion.72 QS inhibition focuses on targeting bacterial virulence and not survival, thus less likely to spur evolutionary moves towards resistance by bacteria.73
In more than 50 Gram-negative bacterial species, QS was mediated by producing N-acyl L-homoserine lactones (AHLs) synthesized from S-adenosylmethionine (SAM) and fatty acid intermediates.74–76 Acyl-homoserine lactones could be formed using acyl carrier proteins that donate the acyl chain and S-adenosyl methionine to form the homoserine lactone component.77 Produced by LuxI-type synthase genes,78 these AHL signals consist of a core N-acylated homoserine-lactone ring with an acyl side chain between 4 and 18 carbons,75 which can then be modified with simple acyl, 3-oxoacyl or 3-hydroxyacyl groups.79 N-acyl L-homoserine lactone signals are membrane-diffusible; accumulating such that they are directly proportional to cell density.75 A LuxR-type receptor found either within the cytoplasm or bound within the membrane binds the signals and then activated once a threshold AHL concentration has been reached. Thereafter acting as a transcriptional regulator for the previously mentioned QS-mediated genes80 as well as for the further production of signalling molecules, creating a positive feedback loop.75,81
The AHL-based QS systems of Gram-negative bacteria have been the most widely considered viable targets for QSI due to their important role in regulating proteobacterial virulence factor production.82 In general, these agents aim to reduce bacterial virulence in common pathogens such as P. aeruginosa without decreasing bacterial growth, thereby minimising the selection for resistance.74,82,83 The host immune system should then theoretically stave off the weakened pathogens,83 or in the case of immune-compromised patients, a dual-therapy approach pairing QSI agents and antibiotics could be used. QS pathways in Gram-negative bacteria usually consist of three important steps: production of signals (autoinducer molecules), diffusion of signals, and recognition of signals by receptor molecules which then activate the target genes.84 Thus, any of these three steps, as well as QS genes transcription,85 may potentially be targeted to inhibit the QS pathway, either through binding or interfering with the biosynthesis of AHLs or degradation of the QS molecules themselves to reduce their concentration.79,83,84
An unprecedented infection by several bacteria species associated with pulmonary infections in Cystic Fibrosis (CF) patients was reportedly shown in the literature.86,87 These include S. aureus, P. aeruginosa, Burkholderia cepacia, Pandoraea species, and a spectrum of other Gram-negative bacteria. Members of genus Pandoraea had shown QS activity that can communicate via AHLs production.88 Bioinformatics prediction of QS genes in the genomes of proteobacteria has revealed the presence of many additional orphan LuxR homologs with no LuxI homologs nearby.89 In addition to their widespread distributions in proteobacteria, some of these LuxR solos are phylogenetically related and several reports have provided evidence on the clustering of LuxR solos into different functionally relevant groups.90–92 An in silico systemic bioinformatics prediction workflow development, which is useful for LuxI and LuxR genes identification of bacterial species, has thus laid down the foundation for the use of bioinformatics as an important tool in the study of QS systems in various pathogenic organisms.87
7. Bioinformatics strategies to limit antibiotic resistance
Bacteria resistance to an antibiotic is a serious public health concern worldwide,93 and has imposed selective pressure on the spread of resistance genes through the exchange of genetic material among bacterial isolates.93 Also, excessive use of antibiotics has changed the microbial populations of the soil, water, and microbiota.93 Because of relentless effort to subdue the bacteria adaptability to the effect of antibiotics, the need to cement the knowledge of antibiotic resistance and bioinformatics becomes very important. Bioinformatics is a discipline that employs a lot of computation techniques including sequence and structural alignment, analyses of large collections of biological data, such as genetic sequences, cell populations, or protein samples, to make new predictions or discover new biology.19 Nowadays bioinformatics techniques such as molecular docking have been used to evaluate ligand–protein interaction and also to estimate binding energy during the docking process. Bioinformatics revolutionises molecular studies to determine the protein structure, gene structure or sequence, molecular markers and relate them to other previously known structures.93 Bioinformatics studies have provided an important system of modelling a biological living cell (with proven DNA sequence) and docking proteins that enabled scientists to discover effective drug strategies to combat the diversifying problem of antibiotic resistance that is becoming one of the major public health challenges.93 Bioinformatics can be used to explore the connections between classical mathematical modelling (at different scales) and predictions of omic scope along with specific aspects of the immune system.94 Some commonly used software in bioinformatics include; Swiss-model (online software for homology modelling), Autodock Vina (for ligand-protein docking) Avogadro (for ligand energy minimisation) and Chimera (to prepare and view 3D docked complex), among others. In the recent time, bioinformatics strategy at preventing antibiotic resistance has evolved, this includes;
7.1 Whole genome sequencing bioinformatics strategies of curbing antibiotics resistance
MDR bacteria is one of the major threats to vulnerable patient populations in hospitals around the world.95,96 This is particularly true for the patients in the intensive care unit (ICU) because of their high level of susceptibility to nosocomial infections perhaps due to indwelling devices such as intravascular catheters, drains, and intratracheal tubes for mechanical ventilation.95 This is why clinicians advocate for effective outbreak management protocols in place, for proper management of these conditions.95 A good understanding of pathogens transmission via genotyping methods could serve as an important tool for outbreak management.95
In recent times, whole-genome sequencing (WGS) of pathogens has become a more accessible and affordable tool for genotyping95 and plays an important role in human and agricultural research.97 Analysis of the entire bacteria genome using WGS could provide insight into related lineages of bacterial98 and can revolutionize outbreak analysis in hospitals.95 The recent development in bioinformatics has changed the understanding of clinicians and perhaps financial burden been perceived on the patients.95 Sequencing technologies and analysis tools have rapidly increased the output and analysis speed as well as reduced the overall costs of WGS.95 Genome sequencing provide a good regime for improvement in scientific research, particularly in biomolecular modelling and drug design with an emphasis on antibiotics resistance.95,99 In addition to identifying pathogens more rapidly and precisely than traditional methods, high-throughput technologies and bioinformatics can provide new insights into disease transmission, virulence, and antimicrobial resistance.99
Deoxyribonucleic acid (DNA) sequencing provides a good platform for protein modelling and drug design. Also, remarkable progress made in genome sequencing, protein expression, high-throughput crystallography, and nuclear magnetic resonance (NMR) has radically transformed the opportunities to use protein three-dimensional structures to accelerate drug discovery.100 Coping with eminent bacteria resistance in our environment, structural biology, and bioinformatics have assisted in lead optimisation and target identification where they have well-established roles.100 They can now contribute to lead discovery,100 exploiting high-throughput methods of structure determination that provide powerful approaches to the screening complex bacteria proteins.100 This is one of the major strategies of curbing bacteria resistance, it allows for putative relatives identification the sequences aligned, and the three-dimensional structures modelled.100 Bioinformatics is also helpful in proposing binding sites and molecular functions if key residues are nicely conserved.100
7.2 In silico analysis of serovar, serogroup, and antigenic profile
Development of whole-genome sequencing technology takes centre stage in genotyping,101 as serovar prediction by traditional serotyping got replaced by molecular serotyping.101 An existing in silico serovar prediction approaches utilise surface antigen encoding genes101 with core genome multilocus sequence typing (MLST) and serovar-specific gene markers or DNA fragments for serotyping. However, these serovar-specific gene markers or DNA fragments can only distinguish a few serovars.101 A recent study by Zhang et al. (2019)101 designed and evaluated an in silico serovar prediction approach by screening 1089 genomes representing 106 serovars against a set of 131 serovar-specific gene markers.101 This approach was reportedly a useful diagnostic tool for culture-independent and metagenomic methods101 as well as providing the best alternative of confirming other genome-based analyses.101 This set of bioinformatics procedure is useful in identifying a specific type of gene markers and may also be useful in developing more cost-effective molecular assays designed to detect specific gene markers of the all major serovars.101
7.3 In silico plasmid identification
Plasmids are extrachromosomal genetic elements which are also known as mobile genetic elements102 (MGEs), they play an important role in horizontal gene transfer and characterised by their ability to self-replicate and transfer between bacterial.103–105 They exist in different sizes and often are carriers of genes with selective advantage to the host under specific conditions.103–105 Plasmids are essential MGEs for the acquisition and spread of antibiotic resistance and are important in transmitting virulence traits.102
Different types of tools are readily available for use in plasmid extraction and assembling from high throughput sequencing (HTS) data, these include; cBar,106 PLACNET,107 plasmidSPAdes,108 and Recycler.109 These tools could be used to accessed plasmid data by searching for specific markers or exploring the distinctive nature of plasmid sequence.102 The tools are assumptions based on relative failure because plasmid can exist in single-copy in the cell110 or linear DNA molecules.103–105 Other tools such as plasmidFinder,111 MOB-suite112 Plasmid Profiler,113 regardless of any of these methods used to reconstruct or find plasmids in HTS data,102 the user will have to struggle to interpret the list of hits and to evaluate the impact of these possible plasmids on the host bacteria.102 There appears about 13
924 entries in the National Centre for Biotechnology Information (NCBI) reference sequence (RefSeq) plasmid database114 with a relative lack of essential tools for accessing these huge data of plasmid sequences.102
However, Jesus et al. (2019) described Plasmid Atlas (pATLAS)102 as intending to offer an easily accessible visual analytics tool for users to explore the existing plasmids in the NCBI's RefSeq database and to aid in identifying plasmids from HTS data.102 pATLAS enables the visualization and exploration of the metadata associated with all plasmids available in NCBI's RefSeq database,102 as well as their putative antibiotic resistance and virulence genes,102 and plasmid families,102 through de novo annotations based on CARD,115 ResFinder,116 Virulence Factors Database (VFDB)117 and PlasmidFinder.111 By offering a representational state transfer application programming interface (REST API), pATLAS can easily be integrated into systems that aim to identify plasmids,102 with leverage on the advantage of the database and visualization tools available in it.102
7.4 In silico identification of antibiotic resistance genes and their association with plasmids
Bacteria resistance to antibiotics is constantly evolving118 and horizontal gene transfer through plasmids plays a crucial role.98,118 Assessment of plasmids characteristics and their association with different bacterial hosts provides a good understanding of plasmids' contribution in transmitting antibiotics resistant (ABR) determinants.118 Molecular identification of plasmids and strain genotypes elicits a distinction between the spread of ABR genes by plasmids and the dissemination of these genes by the spread of bacterial clones.118
In one of the successful studies conducted by Kudirkiene et al. (2018),119 they have selected 16 plasmids carrying antimicrobial resistance genes of Salmonella enterica using whole-genome sequencing (WGS).119 The combination of PlasmidFinder,118,120 ResFinder,118,120 plasmidSPAdes, and BLAST genomic analysis tools118 were used to predict plasmids and resistance genes sites. S1-PFGE was then employed for analysis of plasmids profiles,118 whole genome sequencing confirmed antimicrobial resistance genes present in Salmonella isolates118 showing multidrug resistance phenotypically.119
In S. enteritidis,119 resistance genes were predominantly carried on plasmids of IncN type, in S. Typhimurium on plasmids of IncFII(S)/IncFIB(S)/IncQ1 type. Likewise, resistance genes in S. Virchow and S. Poona were carefully detected on plasmids of IncX1 and TrfA/IncHI2/IncHI2A type, respectively. These two plasmids were therefore described for the first time in these serovars.119 The combination of genomic analytical tools allowed full mapping of the resistance plasmids in all Salmonella strains analyzed.119 Their results suggest that the improved analytical approach used in the study could be used to identify plasmids that are specifically associated with resistance phenotypes in whole-genome sequences.119 Such knowledge would allow the development of rapid multidrug resistance tracking tools in Salmonella populations using world genome sequencing.119
7.5 Metagenomics for antimicrobial surveillance
Antimicrobial resistance (AMR) surveillance has focused mainly on a few pathogens based on passive reporting of certain phenotypes from laboratory results.20 This limits the spectrum to a few selected pathogens as in the Danish Monitoring System (DANMAP) https://www.danmap.org, leading to a narrow pathogen spectrum that does not capture all relevant AMR genes.20 The majority of AMR genes might be found in the commensal bacterial flora of healthy humans and animals or the environment.20
Metagenomics techniques use short-read next-generation sequencing data,20 with the capacity to quantify thousands of transmissible resistance genes in a single sample without any predetermined genes.20 Therefore, it can provide more information about bacterial species presence,20 pathogens, and virulence genes,20 the generated data can then be re-analysed, if novel genes of interest are identified.20 Recently, metagenomics is superior to conventional methods of AMR surveillance in pig herds,121 successfully been used for comparing AMR in livestock in Europe,122 also used in the investigations related to epidemiological data.123
The prospect of metagenomics as a tool for AMR surveillance in the future is quite bright because of its enormous advantage,20 one of which involves direct application on samples from healthy and clinically ill individuals and animals as well as the potential reservoir.20 This might result in the ultimate one goal surveillance of AMR allowing determination of all resistance genes and their context in all reservoirs.20
7.6 Use of comprehensive antibiotic resistance database
The Comprehensive Antibiotic Resistance Database (CARD; https://card.mcmaster.ca) is a rigorously curated resource providing reference DNA and protein sequences, detection models, and bioinformatics tools on the molecular basis of bacterial antimicrobial resistance (AMR).124,125 CARD is used to focus on providing high-quality reference data and molecular sequences within a controlled vocabulary. The Antibiotic Resistance Ontology (ARO), designed by the CARD biocuration team to integrate with software development efforts for resistome analysis and prediction includes CARD's Resistance Gene Identifier (RGI) software.124 In 2017, the use of CARD expanded through extensive curation of reference sequences, revision of the ontological structure, curation of over 500 new AMR detection models, development of a new classification paradigm and expansion of analytical tools.124 Most importantly is the available new module (Resistomes & Variants) that provides analysis and statistical summary of in silico predicted resistance variants from 82 pathogens and over 100
000 genomes.124 By adding these resistance variants to CARD, predicted resistance could be summarized using the information included in CARD, identify trends in AMR mobility and determine previously undescribed and novel resistance variants.124
A good number of resistance gene prediction tools are available, this includes Resfams126 and ARG-ANNOT,127 relative to RGI these provide a certain level of detection of individual components of resistance.127 This approach has proven to have several limitations, however, bioinformatics approaches are often required for analysis of genome sequence that is tolerant of missing data such as unsampled sequence while simultaneously replacing simple sequence similarity with total evidence when predicting antibiogram.125 One possible way is the development of Probabilistic Graphic Models (PGMs), which are a mathematical framework for incorporating uncertainty and probability when making predictions from limited or noisy data128 and that has proven very successful in genomics research, particularly in the analysis of regulatory networks, genetic association studies, and genetic architecture of complex diseases.125
7.7 Use of machine learning tools to predict antibiotic resistance
In a study conducted by Moradigaravand et al. (2018), a machine learning tool was intentionally used to predict antibiotic resistance in Escherichia coli from large-scale pan-genome data.120 In this study, antibiotic resistance detection was typically made by measuring a few known determinants previously identified from genome sequencing, this requires the prior knowledge of its biological mechanisms.120 To overcome this limitation, machine learning models were then used to predict resistance to 11 compounds across four classes of antibiotics from existing and novel whole-genome sequences of 1936 E. coli strains.120 They considered a range of methods and examined population structure, isolation year, gene content, and polymorphism information as predictors.120 In the end, decision trees with gradient boosted provides better results compared to alternative models with an average accuracy of 0.91 on held-out data (range 0.81–0.97).120 While the best models most frequently employed gene content, an average accuracy score of 0.79 could be obtained using population structure information alone.120 Although, single nucleotide variation data were less useful, and significantly improved prediction only for two antibiotics, including ciprofloxacin.120 The result from the study demonstrate that whole-genome sequences could be used to predict antibiotic resistance in E. coli without a priori knowledge of the mechanisms and that both genomic and epidemiological data could be informative.120 This paves the way for integrating machine learning approaches into diagnostic tools in the clinic.120
8. Conclusion
Since the discovery of penicillin by Alexander Fleming in 1924, a great deal of research is available from in silico (in vitro) to in vivo attempting to improve on the therapeutic outcome and perhaps reduce the incidences of bacterial resistance to antibiotics. Most bacteria were initially assumed as commensal organisms and were consider not offending to the human system. This is contrary to the current scientific evidence where disease-causing bacteria are identified alongside the use of drugs in treating disease conditions. Despite the challenges emanating from the clinical use of antibiotics, there is a growing use of these molecules in bacterial infection treatment. However, several resistance strains have evolved and threatened the effectiveness of most antibiotics in the clinical management of disease conditions.
Molecular understanding of bacterial existence and its activities is key in preventing bacterial resistance. In recent times, bioinformatics has played a vital role in drug discovery, gene sequencing, gene alignment, and genera proteomics study. Adequate knowledge on the process of bacterial resistance can precipitate the bioinformatics approach needed to unravel such resistance cases. Investigation of which is in continuum to explore different bioinformatics tools leveraging on the available fundamental understanding of the bacterial system at the molecular level. Hopefully, this method would enable to curb bacteria resistance and potentially lead to an improved therapeutic outcome in treating bacterial infections.
Abbreviations and meanings
AAC | Acetyltransferase |
ABR | Antibiotics resistant |
AHLs | Acyl L-homoserine lactones |
AMEs | Aminoglycoside modifying enzymes |
ANT | Adenyltransferase |
APH | Phosphotransferase |
ARO | Antibiotic resistance ontology |
AUC | Area under curve |
CARD | Comprehensive antibiotic resistance database |
CATs | Chloramphenicol acetyltransferases |
CF | Cystic fibrosis |
cfr gene | Plasmid-borne determinant gene |
DANMAP | Danish monitoring system |
DHFR | Dihydrofolate reductase |
DHPS | Dihydropteroic acid synthase |
DNA | Deoxyribonucleic acid |
E. coli | Escherichia coli |
EF-G | Elongation factor G |
EF-Tu | Elongation factor Tu |
FusB | Fusidic acid B |
FusC | Fusidic acid C |
GTPases | Guanosine triphosphatase |
HTS | High throughput sequencing |
ICU | Intensive care unit |
MDR | Methicillin-resistant |
MDR | Multidrug-resistant |
mecA | Staphylococcal cassette chromosome |
mef | Macrolide efflux |
MEGA | Macrolide efflux genetic assembly |
MGEs | Mobile genetic elements |
MIC | Minimum inhibitory concentration |
MIC | Minimum inhibitory concentration |
MLSB | Macrolides, lincosamides, streptogramines B-resistance |
MLST | Multilocus sequence typing |
MPC | Mutant prevention concentration |
MRSA | Methicillin-resistant Staphylococcus aureus |
NCBI's | National centre for biotechnology information's |
NMR | Nuclear magnetic resonance |
p-gp | Permeability glycol-protein |
PAE | Post antibiotic effect |
pATLAS | Plasmid atlas |
PBP | Penicillin-binding proteins |
PD | Pharmacodynamics |
pH | Potentiality of hydrogen ion |
PK | Pharmacokinetics |
Qnr | A plasmid-encoded and chromosomally determined protein that protects DNA gyrase and topoisomerase |
QS | Quorum-sensing |
QSI | Quorum-sensing inhibition |
RefSeq | Reference sequence |
REST API | Representational state transfer application programming interface |
RIF | Rifampin |
RIFMO | Rifampicin monooxygenase |
RNAPs | Ribonucleic acid proteins |
RpoB | β subunit of bacterial RNA polymerase |
rRNA | Ribosomal ribonucleic acid |
S. aureus | Staphylococcus aureus |
S. Poona | Salmonella Poona |
S. Virchow | Salmonella Virchow |
S1-PFGE | S1-nuclease pulsed-field gel electrophoresis |
SAM | S-Adenosyl-L-methionine |
SAM | S-Adenosylmethionine |
SMX | Trimethoprim–sulfamethoxazole |
TB | Tuberculosis |
Tet | Tetracycline |
Tn1207 | Transposon (1207) |
U.S. | United States |
UN | United Nations |
VFDB | Virulence factors database |
WGS | Whole-genome sequencing |
β-lactamases | Beta-lactamases; enzymes produced by bacteria that provide multi-resistance to β-lactam antibiotics |
Conflict of interest
Authors declare no financial and intellectual conflict of interests.
Acknowledgements
The authors acknowledge the School of Health Science, University of KwaZulu-Natal for technical support.
References
- K. Gould, Antibiotics: from prehistory to the present day, J. Antimicrob. Chemother., 2016, 71, 572–575 CrossRef CAS PubMed
. - M. Wainwright, Moulds in ancient and more recent medicine, Mycologist, 1989, 3, 21–23 CrossRef
. - J. Davies and D. Davies, Origins and evolution of antibiotic resistance, Microbiol. Mol. Biol. Rev., 2010, 74, 417–433 CrossRef CAS PubMed
. - Biggest Threats and Data|Antibiotic/Antimicrobial Resistance, CDC Search PubMed.
- S. Sengupta, M. K. Chattopadhyay and H. P. Grossart, The multifaceted roles of antibiotics and antibiotic resistance in nature, Frontiers in Microbiology, 2013, 4, 47 CrossRef PubMed
. - S. E. Luria and M. Delbrück, Mutations of Bacteria from Virus Sensitivity to Virus Resistance, Genetics, 1943, 28, 491–511 CAS
. - C. L. Ventola, The antibiotic resistance crisis: part 1: causes and threats, P & T, 2015, vol. 40, pp. 277–283 Search PubMed
. - B. Spellberg and D. N. Gilbert, The future of antibiotics and resistance: a tribute to a career of leadership by John Bartlett, Clin. Infect. Dis., 2014, 59(suppl. 2), S71–S75 CrossRef CAS PubMed
. - M. Baym, T. D. Lieberman, E. D. Kelsic, R. Chait, R. Gross, I. Yelin and R. Kishony, Spatiotemporal microbial evolution on antibiotic landscapes, Science, 2016, 353, 1147–1151 CrossRef CAS PubMed
. - Scientists Build Giant Petri Dish to Film Bacteria Resistance, American Association for the Advancement of Science Search PubMed.
- D. Lyddiard, G. L. Jones and B. W. Greatrex, Keeping it simple: lessons from the golden era of antibiotic discovery, FEMS Microbiol. Lett., 2016, 363, fnw084 CrossRef PubMed
. - N. Jackson, L. Czaplewski and L. J. V. Piddock, Discovery and development of new antibacterial drugs: learning from experience?, J. Antimicrob. Chemother., 2018, 73, 1452–1459 CrossRef CAS PubMed
. - The Global Risks Report 2020, World Economic Forum Search PubMed.
- New report calls for urgent action to avert antimicrobial resistance crisis Search PubMed.
- Biggest Threats and Data|Antibiotic/Antimicrobial Resistance, CDC Search PubMed.
- J. M. A. Blair, M. A. Webber, A. J. Baylay, D. O. Ogbolu and L. J. V. Piddock, Molecular mechanisms of antibiotic resistance, Nat. Rev. Microbiol., 2015, 13(1), 42–51 CrossRef CAS PubMed
. - C. P. Randall, K. R. Mariner, I. Chopra and A. J. O'Neill, The target of daptomycin is absent from Escherichia coli and other gram-negative pathogens, Antimicrob. Agents Chemother., 2013, 57, 637–639 CrossRef CAS PubMed
. - T. Tsuchidon and M. Takano, Sensitization by heat treatment of Escherichia coli K-12 cells to hydrophobic antibacterial compounds, Antimicrob. Agents Chemother., 1988, 32, 1680–1683 CrossRef PubMed
. - N. M. Luscombe, D. Greenbaum and M. Gerstein, What is bioinformatics? A proposed definition and overview of the field, Methods Inf. Med., 2001, 40, 346–358 CrossRef CAS PubMed
. - R. S. Hendriksen, V. Bortolaia, H. Tate, G. H. Tyson, F. M. Aarestrup and P. F. McDermott, Using Genomics to Track Global Antimicrobial Resistance, Frontiers in Public Health, 2019, 7, 242 CrossRef PubMed
. - J. A. Carriço, A. J. Sabat, A. W. Friedrich and M. Ramirez, Bioinformatics in bacterial molecular epidemiology and public health: databases, tools and the next-generation sequencing revolution, Eurosurveillance, 2013, 14, 4 Search PubMed
. - A. T. M. Saeb, M. Abouelhoda, M. Selvaraju, S. I. Althawadi, M. Mutabagani, M. Adil, A. Al Hokail and H. T. Tayeb, The use of next-generation sequencing in the identification of a fastidious pathogen: a lesson from a clinical setup, Evol. Bioinf. Online, 2017, 13, 1176934316686072 Search PubMed
. - P. M. Hawkey, The origins and molecular basis of antibiotic resistance, BMJ, 1998, 317, 657–660 CrossRef CAS PubMed
. - Transposon|genetics|Britannica.com.
- J. M. Munita and C. A. Arias, Mechanisms of Antibiotic Resistance, Microbiol. Spectrum, 2016, 4, 2 Search PubMed
. - E. C. Nannini, K. V. Singh, C. A. Arias and B. E. Murray, In Vivo Effects of Cefazolin, Daptomycin, and Nafcillin in Experimental Endocarditis with a Methicillin-Susceptible Staphylococcus aureus Strain Showing an Inoculum Effect against Cefazolin, Antimicrob. Agents Chemother., 2013, 57, 4276–4281 CrossRef CAS PubMed
. - I. Sultan, S. Rahman, A. T. Jan, M. T. Siddiqui, A. H. Mondal and Q. M. R. Haq, Antibiotics, Resistome and Resistance Mechanisms: A Bacterial Perspective, Frontiers in Microbiology, 2018, 9, 2066 CrossRef PubMed
. - M. R. Gillings, Integrons: Past, Present, and Future, Microbiol. Mol. Biol. Rev., 2014, 78, 257–277 CrossRef PubMed
. - E. Cambau and L. Gutmann, Mechanisms of Resistance to Quinolones, Drugs, 1993, 45, 15–23 CrossRef CAS PubMed
. - J. Heesemann, Mechanisms of resistance to beta-lactam antibiotics, Infection, 1993, 21(suppl. 1), S4–S9 CrossRef PubMed
. - J. M. Munita and C. Arias, Mechanisms of Antibiotic Resistance, Univ. Oklahoma Heal. Sci. Cent., 2016, vol. 23, pp. 1–45 Search PubMed
. - D. N. Wilson, Ribosome-targeting antibiotics and mechanisms of bacterial resistance, Nat. Rev. Microbiol., 2014, 12, 35–48 CrossRef CAS PubMed
. - M. S. Ramirez and M. E. Tolmasky, Aminoglycoside modifying enzymes, Drug Resist. Updates, 2010, 13, 151–171 CrossRef CAS PubMed
. - C. A. DiazGranados, S. M. Zimmer, K. Mitchel and J. A. Jernigan, Comparison of Mortality Associated with Vancomycin-Resistant and Vancomycin-Susceptible Enterococcal Bloodstream Infections: A Meta-analysis, Clin. Infect. Dis., 2005, 41, 327–333 CrossRef PubMed
. - S. Schwarz, C. Kehrenberg, B. Doublet and A. Cloeckaert, Molecular basis of bacterial resistance to chloramphenicol and florfenicol, FEMS Microbiol. Rev., 2004, 28, 519–542 CrossRef CAS PubMed
. - B. Samuel, Structure – Medical Microbiology – NCBI Bookshelf, 1996 Search PubMed
. - J. M. Pagès, C. E. James and M. Winterhalter, The porin and the permeating antibiotic: a selective diffusion barrier in Gram-negative bacteria, Nat. Rev. Microbiol., 2008, 6, 893–903 CrossRef PubMed
. - H. Nikaido, Molecular Basis of Bacterial Outer Membrane Permeability Revisited, Microbiol. Mol. Biol. Rev., 2003, 67, 593–656 CrossRef CAS PubMed
. - L. McMurry, R. E. Petrucci and S. B. Levy, Active efflux of tetracycline encoded by four genetically different tetracycline resistance determinants in Escherichia coli, Proc. Natl. Acad. Sci. U. S. A., 1980, 77, 3974–3977 CrossRef CAS PubMed
. - X. Z. Li and H. Nikaido, Efflux-mediated antimicrobial resistance, Drugs, 2004, 64, 159–204 CrossRef CAS PubMed
. - K. V. Singh, G. M. Weinstock and B. E. Murray, An Enterococcus faecalis ABC homologue (Lsa) is required for the resistance of this species to clindamycin and quinupristin–dalfopristin, Antimicrob. Agents Chemother., 2002, 46, 1845–1850 CrossRef CAS PubMed
. - J. I. Ross, E. A. Eady, J. H. Cove, W. J. Cunliffe, S. Baumberg and J. C. Wootton, Inducible erythromycin resistance in staphlyococci is encoded by a member of the ATP-binding transport super-gene family, Mol. Microbiol., 1990, 4, 1207–1214 CrossRef CAS PubMed
. - S. Pal, A journey across the sequential development of macrolides and ketolides related to erythromycin, Tetrahedron, 2006, 14, 3171–3200 CrossRef
. - S. R. Connell, D. M. Tracz, K. H. Nierhaus and D. E. Taylor, Ribosomal Protection Proteins and Their Mechanism of Tetracycline Resistance, Antimicrob. Agents Chemother., 2003, 47, 3675–3681 CrossRef CAS PubMed
. - L. K. R. Sharkey and A. J. O'Neill, Antibiotic Resistance ABC-F Proteins: Bringing Target Protection into the Limelight, ACS Infect. Dis., 2018, 4, 239–246 CrossRef CAS PubMed
. - A. Dönhöfer, S. Franckenberg, S. Wickles, O. Berninghausen, R. Beckmann and D. N. Wilson, Structural basis for TetM-mediated tetracycline resistance, Proc. Natl. Acad. Sci. U. S. A., 2012, 109, 16900–16905 CrossRef PubMed
. - V. Molodtsov, N. T. Scharf, M. A. Stefan, G. A. Garcia and K. S. Murakami, Structural basis for rifamycin resistance of bacterial RNA polymerase by the three most clinically important RpoB mutations found in Mycobacterium tuberculosis, Mol. Microbiol., 2017, 103, 1034–1045 CrossRef CAS PubMed
. - L. K. Liu, Y. Dai, H. Abdelwahab, P. Sobrado and J. J. Tanner, Structural Evidence for Rifampicin Monooxygenase Inactivating Rifampicin by Cleaving Its Ansa-Bridge, Biochemistry, 2018, 57, 2065–2068 CrossRef CAS PubMed
. - P. A. Lambert, Bacterial resistance to antibiotics: modified target sites, Adv. Drug Delivery Rev., 2005, 57, 1471–1485 CrossRef CAS PubMed
. - R. Leclercq, Mechanisms of Resistance to Macrolides and Lincosamides: Nature of the Resistance Elements and Their Clinical Implications, Clin. Infect. Dis., 2002, 34, 482–492 CrossRef CAS PubMed
. - S. M. Toh, L. Xiong, C. A. Arias, M. V. Villegas, K. Lolans, J. Quinn and A. S. Mankin, Acquisition of a natural resistance gene renders a clinical strain of methicillin-resistant Staphylococcus aureus resistant to the synthetic antibiotic linezolid, Mol. Microbiol., 2007, 64, 1506–1514 CrossRef CAS PubMed
. - Y. Song, Y. Lv, L. Cui, Y. Li, Q. Ke and Y. Zhao, cfr-mediated linezolid-resistant clinical isolates of methicillin-resistant coagulase-negative staphylococci from China, Journal of Global Antimicrobial Resistance, 2017, 8, 1–5 CrossRef PubMed
. - J. Flensburg and O. Sköld, Massive overproduction of dihydrofolate reductase in bacteria as a response to the use of trimethoprim, Eur. J. Biochem., 1987, 162, 473–476 CrossRef CAS PubMed
. - G. M. Eliopoulos and P. Huovinen, Resistance to Trimethoprim-Sulfamethoxazole, Clin. Infect. Dis., 2001, 32, 1608–1614 CrossRef PubMed
. - M. J. Rybak, Pharmacodynamics: relation to antimicrobial resistance, Am. J. Infect. Control, 2006, 34, S38–S45 CrossRef PubMed
. - T. H. Haight and M. Finland, Resistance of Bacteria to Erythromycin, Exp. Biol. Med., 1952, 81, 183–188 CrossRef CAS PubMed
. - C. Gianvecchio, N. A. Lozano, C. Henderson, P. Kalhori, A. Bullivant, A. Valencia, L. Su, G. Bello, M. Wong, E. Cook, L. Fuller, J. B. Neal and P. J. Yeh, Variation in Mutant Prevention Concentrations, Frontiers in Microbiology, 2019, 10, 42 CrossRef PubMed
. - Ç. Yılmaz and G. Özcengiz, Antibiotics: pharmacokinetics, toxicity, resistance and multidrug efflux pumps, Biochem. Pharmacol., 2017, 133, 43–62 CrossRef PubMed
. - M. Boffito, Pharmacokinetic implications of resistance, Antiretrovir. Resist. Clin. Pract, 2006 Search PubMed
. - N. J. Onufrak, A. Forrest and D. Gonzalez, Pharmacokinetic and Pharmacodynamic Principles of Anti-infective Dosing, Clin. Ther., 2016, 39, 1930–1947 CrossRef PubMed
. - G. T. Ho, F. M. Moodie and J. Satsangi, Multidrug resistance 1 gene (P-glycoprotein 170): an important determinant in gastrointestinal disease?, Gut, 2003, 52, 759–766 CrossRef CAS PubMed
. - M. L. Amin, P-glycoprotein inhibition for optimal drug delivery, Drug Target Insights, 2013, 7, 27–32 CrossRef PubMed
. - M. E. Levison and J. H. Levison, Pharmacokinetics and Pharmacodynamics of Antibacterial Agents, Infectious Disease Clinics of North America, 2009, 23, 791–815 CrossRef PubMed
. - D. Gonzalez, S. Schmidt and H. Derendorf, Importance of relating efficacy measures to unbound drug concentrations for anti-infective agents, Clin. Microbiol. Rev., 2013, 26, 274–288 CrossRef CAS PubMed
. - L. M. Berezhkovskiy, On the influence of protein binding on pharmacological activity of drugs, J. Pharm. Sci., 2010, 99, 2153–2165 CrossRef CAS PubMed
. - M. W. Garrison, K. Vance-Bryan, T. A. Larson, J. P. Toscano and J. C. Rotschafer, Assessments of effects of protein binding on daptomycin and vancomycin killing of Staphylococcus aureus by using an in vitro pharmacodynamic model, Antimicrob. Agents Chemother., 1990, 34, 1925–1931 CrossRef CAS PubMed
. - L. E. Bryan and H. M. Van Den Elzen, Streptomycin accumulation in susceptible and resistant strains of Escherichia coli and Pseudomonas aeruginosa, Antimicrob. Agents Chemother., 1976, 9, 928–938 CrossRef CAS PubMed
. - D. M. Bamberger, B. L. Herndon and P. R. Suvarna, The effect of zinc on microbial growth and bacterial killing by cefazolin in a Staphylococcus aureus abscess milieu, J. Infect. Dis., 1993, 168, 893–896 CrossRef CAS PubMed
. - O. Álvarez, J. C. Plaza-Plaza, M. Ramirez, A. Peralta, C. A. Amador and R. Amador, Pharmacokinetic assessment of vancomycin loading dose in critically ill patients, Antimicrob. Agents Chemother., 2017, 61, e00280-17 CrossRef PubMed
. - P. G. Ambrose, S. M. Bhavnani and R. C. Owens, Clinical pharmacodynamics of quinolones, Infectious Disease Clinics of North America, 2003, 17, 529–543 CrossRef PubMed
. - K. Saurav, R. Bar-Shalom, M. Haber, I. Burgsdorf, G. Oliviero, V. Costantino, D. Morgenstern and L. Steindler, In search of alternative antibiotic drugs: Quorum-quenching activity in sponges and their bacterial isolates, Frontiers in Microbiology, 2016, 7, 416 CrossRef PubMed
. - S. T. Rutherford and B. L. Bassler, Bacterial quorum sensing: its role in virulence and possibilities for its control, Cold Spring Harbor Perspect. Med., 2012, 2, 12427 CrossRef PubMed
. - A. R. Padmavathi, D. Bakkiyaraj, N. Thajuddin and S. K. Pandian, Effect of 2,4-di-tert-butylphenol on growth and biofilm formation by an opportunistic fungus Candida albicans, Biofouling, 2015, 31, 565–574 CrossRef CAS PubMed
. - R. Hassan, M. I. Shaaban, F. M. Abdel Bar, A. M. El-Mahdy and S. Shokralla, Quorum sensing inhibiting activity of Streptomyces coelicoflavus isolated from soil, Frontiers in Microbiology, 2016, 7, 659 CrossRef PubMed
. - K. Papenfort and B. L. Bassler, Quorum sensing signal-response systems in Gram-negative bacteria, Nat. Rev. Microbiol., 2016, 14, 576–588 CrossRef CAS PubMed
. - K. Lee and S. S. Yoon, Pseudomonas aeruginosa Biofilm, a Programmed Bacterial Life for Fitness, J. Microbiol. Biotechnol., 2017, 27, 1053–1064 CrossRef CAS PubMed
. - D. Safari, N. Kurniati, L. Waslia, M. M. Khoeri, T. Putri, D. Bogaert and K. Trzciński, Serotype Distribution and Antibiotic Susceptibility of Streptococcus pneumoniae Strains Carried by Children Infected with Human Immunodeficiency Virus, PLoS One, 2014, 9, e110526 CrossRef PubMed
. - M. Cooley, S. R. Chhabra and P. Williams, N-Acylhomoserine Lactone-Mediated Quorum Sensing: A Twist in the Tail and a Blow for Host Immunity, Chem. Biol., 2008, 15, 1141–1147 CrossRef CAS PubMed
. - W. Zhang and C. Li, Exploiting quorum sensing interfering strategies in Gram-negative bacteria for the enhancement of environmental applications, Frontiers in Microbiology, 2016, 6, 1535 Search PubMed
. - X. Gao, X. Wang, Q. Mao, R. Xu, X. Zhou, Y. Ma, Q. Liu, Y. Zhang and Q. Wang, VqsA, a novel LysR-type transcriptional regulator, coordinates quorum sensing (QS) and is controlled by QS to regulate virulence in the pathogen Vibrio alginolyticus, Appl. Environ. Microbiol., 2018, 84, e00444-18 CrossRef PubMed
. - I. Castillo-Juarez, L. E. Lopez-Jacome, G. Soberon-Chavez, M. Tomas, J. Lee, P. Castaneda-Tamez, I. A. Hernandez-Barragan, M. Cruz-Muniz, T. Maeda, T. K. Wood and R. Garcia-Contreras, Exploiting Quorum Sensing Inhibition for the Control of Pseudomonas aeruginosa and Acinetobacter baumannii Biofilms, Curr. Top. Med. Chem., 2017, 17, 1915–1927 CrossRef CAS
. - P. D. Rekha, H. S. Vasavi, C. Vipin, K. Saptami and A. B. Arun, A medicinal herb Cassia alata attenuates quorum sensing in Chromobacterium violaceum and Pseudomonas aeruginosa, Lett. Appl. Microbiol., 2017, 64, 231–238 CrossRef CAS
. - R. García-Contreras, Is Quorum Sensing Interference a Viable Alternative to Treat Pseudomonas aeruginosa Infections?, Frontiers in Microbiology, 2016, 7, 1454 CrossRef PubMed
. - K. Tang and X. H. Zhang, Quorum quenching agents: resources for antivirulence therapy, Mar. Drugs, 2014, 12, 3245–3282 CrossRef PubMed
. - B. Subhadra, M. Hwan Oh and C. Hee Choi, Quorum sensing in Acinetobacter: with special emphasis on antibiotic resistance, biofilm formation and quorum quenching, AIMS Microbiol., 2016, 2, 27–41 CAS
. - J. C. Davies and B. K. Rubin, Emerging and unusual Gram-negative infections in cystic fibrosis, Seminars in Respiratory and Critical Care Medicine, 2007, 28, 312–321 CrossRef PubMed
. - K.-O. Chua, W.-S. See-Too, R. Ee, Y.-L. Lim, W.-F. Yin and K.-G. Chan, In silico Analysis Reveals Distribution of Quorum Sensing Genes and Consistent Presence of LuxR Solos in the Pandoraea Species, Frontiers in Microbiology, 2019, 10, 1758 CrossRef PubMed
. - R. Ee, Y. L. Lim, L. X. Kin, W. F. Yin and K. G. Chan, Quorum sensing activity in Pandoraea pnomenusa RB38, Sensors, 2014, 14, 10177–10186 CrossRef
. - C. Fuqua, The QscR quorum-sensing regulon of Pseudomonas aeruginosa: an orphan claims its identity, J. Bacteriol., 2006, 9, 3169–3171 CrossRef
. - S. Brameyer, D. Kresovic, H. B. Bode and R. Heermann, LuxR solos in Photorhabdus species, Front. Cell. Infect. Microbiol., 2014, 4, 166 Search PubMed
. - H. M. Gan, H. Y. Gan, N. H. Ahmad, N. A. Aziz, A. O. Hudson and M. A. Savka, Whole genome sequencing and analysis reveal insights into the genetic structure, diversity and evolutionary relatedness of luxI and luxR homologs in bacteria belonging to the Sphingomonadaceae family, Front. Cell. Infect. Microbiol., 2014, 4, 188 Search PubMed
. - S. Subramoni, D. V. F. Salcedo and Z. R. Suarez-Moreno, A bioinformatic survey of distribution, conservation, and probable functions of LuxR solo regulators in bacteria, Front. Cell. Infect. Microbiol., 2015, 5, 16 Search PubMed
. - H. Hemlata and A. Tiwari, Applications of bioinformatics tools to combat the antibiotic resistance, in 2015 International Conference on Soft Computing Techniques and Implementations, ICSCTI, 2016, pp. 96–98 Search PubMed
. - N. Rapin, O. Lund, M. Bernaschi and F. Castiglione, Computational immunology meets bioinformatics: the use of prediction tools for molecular binding in the simulation of the immune system, PLoS One, 2010, 4, e9862 CrossRef PubMed
. - S. Quainoo, J. P. M. Coolen, S. A. F. T. van Hijum, M. A. Huynen, W. J. G. Melchers, W. van Schaik and H. F. L. Wertheim, Whole-genome sequencing of bacterial pathogens: the future of nosocomial outbreak analysis, Clin. Microbiol. Rev., 2017, 4, 1015–1063 CrossRef
. - H. Wong, K. Eso, A. Ip, J. Jones, Y. Kwon, S. Powelson, J. de Grood, R. Geransar, M. Santana, A. M. Joffe, G. Taylor, B. Missaghi, C. Pearce, W. A. Ghali and J. Conly, Use of ward closure to control outbreaks among hospitalized patients in acute care settings: a systematic review, Syst. Rev., 2015, 4, 152 CrossRef PubMed
. - J. L. Jones, L. Wang, O. Ceric, S. M. Nemser, D. S. Rotstein, D. A. Jurkovic, Y. Rosa, B. Byrum, J. Cui, Y. Zhang, C. A. Brown, A. L. Burnum, S. Sanchez and R. Reimschuessel, Whole genome sequencing confirms source of pathogens associated with bacterial foodborne illness in pets fed raw pet food, J. Vet. Diagn. Invest., 2019, 31, 235–240 CrossRef PubMed
. - S. Biswas, D. Raoult and J. M. Rolain, A bioinformatic approach to understanding antibiotic resistance in intracellular bacteria through whole genome analysis, Int. J. Antimicrob. Agents, 2008, 32, 207–220 CrossRef CAS PubMed
. - M. Gwinn, D. Maccannell and G. L. Armstrong, Next-Generation Sequencing of Infectious Pathogens, JAMA, 2019, 321, 893–894 CrossRef PubMed
. - T. L. Blundell, B. L. Sibanda, R. W. Montalvão, S. Brewerton, V. Chelliah, C. L. Worth, N. J. Harmer, O. Davies and D. Burke, Structural biology and bioinformatics in drug design: opportunities and challenges for target identification and lead discovery, Philos. Trans. R. Soc., B, 2006, 361, 413–423 CrossRef CAS PubMed
. - X. Zhang, M. Payne and R. Lan, In silico Identification of Serovar-Specific Genes for Salmonella Serotyping, Frontiers in Microbiology, 2019, 10, 835 CrossRef PubMed
. - T. F. Jesus, B. Ribeiro-Gonçalves, D. N. Silva, V. Bortolaia, M. Ramirez and J. A. Carriço, Plasmid ATLAS: plasmid visual analytics and identification in high-throughput sequencing data, Nucleic Acids Res., 2019, 47, D188–D194 CrossRef CAS PubMed
. - L. S. Frost, R. Leplae, A. O. Summers and A. Toussaint, Mobile genetic elements: the agents of open source evolution, Nat. Rev. Microbiol., 2005, 3, 722–732 CrossRef CAS PubMed
. - M. Shintani, Z. K. Sanchez and K. Kimbara, Genomics of microbial plasmids: classification and identification based on replication and transfer systems and host taxonomy, Frontiers in Microbiology, 2015, 6, 242 CrossRef PubMed
. - J. P. J. Hall, M. A. Brockhurst and E. Harrison, Sampling the mobile gene pool: Innovation via horizontal gene transfer in bacteria, Philos. Trans. R. Soc., B, 2017, 372, 20160424 CrossRef PubMed
. - F. Zhou and Y. Xu, cBar: a computer program to distinguish plasmid-derived from chromosome-derived sequence fragments in metagenomics data, Bioinformatics, 2010, 26, 2051–2052 CrossRef CAS PubMed
. - V. F. Lanza, M. de Toro, M. P. Garcillán-Barcia, A. Mora, J. Blanco, T. M. Coque and F. de la Cruz, Plasmid Flux in Escherichia coli ST131 Sublineages, Analyzed by Plasmid Constellation Network (PLACNET), a New Method for Plasmid Reconstruction from Whole Genome Sequences, PLoS Genet., 2014, 10, e1004766 CrossRef PubMed
. - D. Antipov, N. Hartwick, M. Shen, M. Raiko, A. Lapidus and P. A. Pevzner, PlasmidSPAdes: assembling plasmids from whole genome sequencing data, Bioinformatics, 2016, 32, 3380–3387 CrossRef CAS PubMed
. - R. Rozov, A. B. Kav, D. Bogumil, N. Shterzer, E. Halperin, I. Mizrahi and R. Shamir, Recycler: an algorithm for detecting plasmids from de novo assembly graphs, Bioinformatics, 2017, 33, 475–482 CAS
. - A. Orlek, N. Stoesser, M. F. Anjum, M. Doumith, M. J. Ellington, T. Peto, D. Crook, N. Woodford, A. Sarah Walker, H. Phan and A. E. Sheppard, Plasmid classification in an era of whole-genome sequencing: application in studies of antibiotic resistance epidemiology, Frontiers in Microbiology, 2017, 8, 182 CrossRef PubMed
. - A. Carattoli, E. Zankari, A. Garciá-Fernández, M. V. Larsen, O. Lund, L. Villa, F. M. Aarestrup and H. Hasman, In Silico detection and typing of plasmids using PlasmidFinder and plasmid multilocus sequence typing, Antimicrob. Agents Chemother., 2014, 58, 3895–3903 CrossRef PubMed
. - J. Robertson and J. H. E. Nash, MOB-suite: software tools for clustering, reconstruction and typing of plasmids from draft assemblies, Microb. Genomics, 2018, 4, 1–7 Search PubMed
. - A. Zetner, J. Cabral, L. Mataseje, N. C. Knox, P. Mabon, M. Mulvey and G. Van Domselaar, Plasmid Profiler: Comparative Analysis of Plasmid Content in WGS Data, 2017, bioRxiv 121350 Search PubMed.
- N. A. O'Leary, M. W. Wright, J. R. Brister, S. Ciufo, D. Haddad, R. McVeigh, B. Rajput, B. Robbertse, B. Smith-White, D. Ako-Adjei, A. Astashyn, A. Badretdin, Y. Bao, O. Blinkova, V. Brover, V. Chetvernin, J. Choi, E. Cox, O. Ermolaeva, C. M. Farrell, T. Goldfarb, T. Gupta, D. Haft, E. Hatcher, W. Hlavina, V. S. Joardar, V. K. Kodali, W. Li, D. Maglott, P. Masterson, K. M. McGarvey, R. Murphy, K. O'Neill, S. Pujar, S. H. Rangwala, D. Rausch, L. D. Riddick, C. Schoch, A. Shkeda, M. S. S. Storz, H. Sun, F. Thibaud-Nissen, I. Tolstoy, R. E. Tully, A. R. Vatsan, C. Wallin, D. Webb, W. Wu, M. J. Landrum, A. Kimchi, T. Tatusova, M. DiCuccio, P. Kitts, T. D. Murphy and K. D. Pruitt, Reference sequence (RefSeq) database at NCBI: current status, taxonomic expansion and functional annotation, Nucleic Acids Res., 2016, 44, D733–D745 CrossRef PubMed
. - D. Wang, J. Zhu, K. Zhou, J. Chen, Z. Yin, J. Feng, L. Ma and D. Zhou, Genetic characterization of novel class 1 Integrons In0, In1069 and In1287 to In1290, and the inference of In1069-associated integron evolution in Enterobacteriaceae, Antimicrobial Resistance & Infection Control, 2017, 6, 84 Search PubMed
. - E. Zankari, H. Hasman, S. Cosentino, M. Vestergaard, S. Rasmussen, O. Lund, F. M. Aarestrup and M. V. Larsen, Identification of acquired antimicrobial resistance genes, J. Antimicrob. Chemother., 2012, 67, 2640–2644 CrossRef CAS PubMed
. - L. Chen, J. Yang, J. Yu, Z. Yao, L. Sun, Y. Shen and Q. Jin, VFDB: a reference database for bacterial virulence factors, Nucleic Acids Res., 2005, 33, 325–328 CrossRef PubMed
. - M. Rozwandowicz, M. S. M. Brouwer, J. Fischer, J. A. Wagenaar, B. Gonzalez-Zorn, B. Guerra, D. J. Mevius and J. Hordijk, Plasmids carrying antimicrobial resistance genes in Enterobacteriaceae, J. Antimicrob. Chemother., 2018, 73, 1121–1137 CrossRef CAS PubMed
. - E. Kudirkiene, L. A. Andoh, S. Ahmed, A. Herrero-Fresno, A. Dalsgaard, K. Obiri-Danso and J. E. Olsen, The use of a combined bioinformatics approach to locate antibiotic resistance genes on plasmids from whole genome sequences of Salmonella enterica serovars from humans in Ghana, Frontiers in Microbiology, 2018, 9, 1010 CrossRef PubMed
. - D. Moradigaravand, M. Palm, A. Farewell, V. Mustonen, J. Warringeri, L. Partsid and A. E. Darling, Prediction of antibiotic resistance in Escherichia coli from large-scale pan-genome data, PLoS Comput. Biol., 2018, 14, e1006258 CrossRef PubMed
. - P. Munk, V. D. Andersen, L. de Knegt, M. S. Jensen, B. E. Knudsen, O. Lukjancenko, H. Mordhorst, J. Clasen, Y. Agersø, A. Folkesson, S. J. Pamp, H. Vigre and F. M. Aarestrup, A sampling and metagenomic sequencing-based methodology for monitoring antimicrobial resistance in swine herds, J. Antimicrob. Chemother., 2017, 72, 385–392 CrossRef CAS PubMed
. - P. Munk, B. E. Knudsen, O. Lukjacenko, A. S. R. Duarte, L. Van Gompel, R. E. C. Luiken, L. A. M. Smit, H. Schmitt, A. D. Garcia, R. B. Hansen, T. N. Petersen, A. Bossers, E. Ruppé, H. Graveland, A. van Essen, B. Gonzalez-Zorn, G. Moyano, P. Sanders, C. Chauvin, J. David, A. Battisti, A. Caprioli, J. Dewulf, T. Blaha, K. Wadepohl, M. Brandt, D. Wasyl, M. Skarzyńska, M. Zajac, H. Daskalov, H. W. Saatkamp, K. D. C. Stärk, O. Lund, T. Hald, S. J. Pamp, H. Vigre, D. Heederik, J. A. Wagenaar, D. Mevius and F. M. Aarestrup, Abundance and diversity of the faecal resistome in slaughter pigs and broilers in nine European countries, Nat. Microbiol., 2018, 3, 898–908 CrossRef CAS PubMed
. - L. Van Gompel, R. E. C. Luiken, S. Sarrazin, P. Munk, B. E. Knudsen, R. B. Hansen, A. Bossers, F. M. Aarestrup, J. Dewulf, J. A. Wagenaar, D. J. Mevius, H. Schmitt, D. J. J. Heederik, A. Dorado-García and L. A. M. Smit, The antimicrobial resistome in relation to antimicrobial use and biosecurity in pig farming, a metagenome-wide association study in nine European countries, J. Antimicrob. Chemother., 2019, 74, 865–876 CrossRef CAS PubMed
. - P. A. Brian, R. R. Amogelang, T. Y. L. Tammy, K. T. Kara, B. Mégane, E. Arman, H. William, V. N. Anna-Lisa, A. C. Annie, L. Sihan, Y. M. Sally, M. Anatoly, T. Hiu-Ki, E. W. Rafik, A. N. Jalees, M. Oloni, D. J. Speicher, A. Florescu, B. Singh, F. Mateusz, H.-K. Anastasia, N. S. Arjun, B. Emily, C. P. Andrew, L. Z. Haley, D. Damion, G. Emma, M. Finlay, W. Geoff, L. G. B. Robert, S. L. B. Fiona, W. L. H. William, V. D. Gary and G. McAndrew, CARD 2020: antibiotic resistome surveillance with the comprehensive antibiotic resistance database, Nucleic Acids Res., 2019, 48, D1 Search PubMed
. - A. G. McArthur and G. D. Wright, Bioinformatics of antimicrobial resistance in the age of molecular epidemiology, Curr. Opin. Microbiol., 2015, 27, 45–50 CrossRef PubMed
. - M. K. Gibson, K. J. Forsberg and G. Dantas, Improved annotation of antibiotic resistance determinants reveals microbial resistomes cluster by ecology, ISME J., 2015, 9, 207–216 CrossRef CAS PubMed
. - S. K. Gupta, B. R. Padmanabhan, S. M. Diene, R. Lopez-Rojas, M. Kempf, L. Landraud and J. M. Rolain, ARG-annot, a new bioinformatic tool to discover antibiotic resistance genes in bacterial genomes, Antimicrob. Agents Chemother., 2014, 58, 212–220 CrossRef PubMed
. - N. A. Sakhanenko and D. J. Galas, Probabilistic logic methods and some applications to biology and medicine, J. Comput. Biol., 2012, 19, 316–336 CrossRef CAS PubMed
.
|
This journal is © The Royal Society of Chemistry 2020 |