DOI:
10.1039/C9RA09386A
(Paper)
RSC Adv., 2019,
9, 39858-39870
Ultra-performance liquid chromatography-mass spectrometry-based metabolomics reveals Huangqiliuyi decoction attenuates abnormal metabolism as a novel therapeutic opportunity for type 2 diabetes†
Received
11th November 2019
, Accepted 15th November 2019
First published on 2nd December 2019
Abstract
Background: As a typical chronic metabolic disease, type 2 diabetes mellitus causes a heavy health-care burden to society. In this study, we applied the metabolomics strategy to explore the potential molecular mechanism of the Huangqiliuyi decoction (HQLYD) for type-2 diabetes (T2D). Ultra-performance liquid chromatography-mass spectrometry (UPLC-MS) combined with pattern recognition methods was utilized to select specific metabolites closely associated with HQLYD. Biomarker pathway analysis and biological network were utilized to uncover the therapeutic effect and action mechanism related to HQLYD. A total of twenty-five biomarkers were identified in the animal model, in which sixteen biomarkers are associated with HQLYD treatment for T2D. They attenuated the abnormalities of metabolic pathways such as phenylalanine, tyrosine and tryptophan biosynthesis, phenylalanine metabolism, and the citrate cycle. HQLYD also significantly elevated the serum FINS and SOD, GSP-x level in the liver and kidney, and reduced the serum TC, TG, HDL, LDL, urea, Scr, AST, ALT, FBG, IRS, MDA, and CAT level. We found that the therapeutic mechanism of HQLYD against T2D affected amino acid metabolism, glucose metabolism and lipid metabolism. Metabolomics revealed that the Huangqiliuyi decoction attenuates abnormal metabolism as a novel therapeutic opportunity for type 2 diabetes.
1. Introduction
Type 2 diabetes mellitus is a typical chronic metabolic disease, and it was reported that more than four million people suffered from it globally in 2014. Based on the latest research of the Diabetes Atlas from the International Diabetes Federation, this figure is likely to reach 642 million by 2040, and the amazing growth rate undoubtedly brings a heavy health-care burden to society.1 Because of the intricate and incomplete understanding of the reciprocity between the natural environmental and inheritance factors, type 2 diabetes mellitus invalids the present feature of insulin sensitivity decline, impaired β-cell physiological function and hyperinsulinaemia.2–4 In recent years, diabetes defense and treatment progress have failed to achieve good results, where the main problem is that patients are in the pathophysiological state of type 2 diabetes mellitus for many years before the onset of clinical symptoms, and in addition, patients are easily prone to complications such as nephropathy, retinopathy and residual foot issues at the time of diagnosis.5,6 In clinic, the available and routine methods such as detecting BMI, fasting blood glucose and HbA 1c content have limitations and are imprecise.7–9 Currently, the commonly used drugs in clinic are sulfonylureas combined with biguanide hypoglycemic agents and insulin injections; however, their therapeutic effect is limited and they even result in distinct side effects such as severe liver dysfunction, kidney dysfunction, severer infections, and pregnancy contraindication.10,11 Thus, it is imperative to seek advanced diagnostic methods and effective therapies for distinguishing high-risk populations, maximizing therapeutic efficiency and minimizing adverse reactions to remedy the strained situation of type 2 diabetes mellitus.
Mounting evidence suggests that drug combinations containing multiple drugs targets are more conducive to the treatment of chronic diseases. As long ago as 400 years ago, a combinative therapeutic method called ‘prescription’, which takes advantage of medicinal herbs by interactive relationship in the therapy of diabetes mellitus, was recorded in the General Questions and Answers from Huang Di's Inner Classie. As an indispensable factor of the modern medical setup in comparison with Western medicine, traditional Chinese medicine (TCM) prescriptions consist of some particular herbs containing multiple active ingredients and multiple disease targets are characterized with accessibility, hypotoxicity, prominent efficacy and fewer serious side effects in resisting various types of diseases by synergistic effects in organisms.12,13 The Huangqiliuyi decoction (HQLYD) is a formula consisting of a boiled water solution of Astragalus and Glycyrrhiza with a proportion of 6
:
1, which has been applied for the treatment of verruca acuminata, prostatitis, and diabetes mellitus in clinical practice, especially for type 2 diabetes mellitus-related diseases. According to the chemical ingredient analysis, the main medicinal substances of Astragalus membranaceus are Astragalus polysaccharides, Astragalus saponins, flavonoids, amino acids and trace elements.14 Many studies found that it markedly perfects insulin sensitivity by adjusting PKB/GLUT4 signaling in skeletal muscle and declines the expression of PTP1B medicated by removing ER stress-induced ATF6 activation in model animals.15,16 In addition, astragaloside IV has the ability to weaken lipolysis and heighten insulin resistance through the hepatic glucose-regulating enzyme pathway.17 Licorice contains a large amount of flavonoids, such as isoflavones, dihydroflavones, flavonols, chalcones, and dihydrochalcone.18 These compounds exhibit activity for restoring tyrosine phosphorylation of insulin receptors and insulin receptor substrates, improving insulin signaling and enhancing glucose tolerance and insulin sensitivity.19,20 These facts indicate that HQLYD has excellent therapeutic effects on type 2 diabetes mellitus; however, the therapeutic mechanism of HQLYD on type 2 diabetes mellitus is still not fully understood.
Metabolomics focuses on small endogenous metabolites with a molecular weight less than 1 kDa in biofluids and tissues, and aims to explore multi-parametric metabolic reactions to pathological provocative or medicinal therapy, which supplies an integral metabolic profiling analysis of biological systems.21,22 It aims to screen potential biomarkers that are highly sensitive to disorder and contribute to changes. There are two major analytical techniques, including nuclear magnetic resonance (NMR) and mass spectrometry (MS), widely applied in measurement at a rapidly increasing rate. Comparing the MS and NMR technology platforms, the sample detection requirement of MS is low, it has high sensitivity and a wide linear range, and metabolites with a large difference in concentration can also achieve good detection results.23,24 The metabolome as the final outcome during the biological process contains the total set of metabolites in a living organism. Compared with the large number of genes or mRNA, it has significant capacity to select biomarkers and seek disrupted pathways of HQLYD in protecting against type 2 diabetes mellitus by detecting metabolome changes.25 In this research, a comprehensive strategy based on serum metabolomics was applied to perform an overall determination of the mechanism of HQLYD in treating type 2 diabetes mellitus. The differentiated biomarkers identified in a rat model induced by the dietary model of high sugar and high fat were further confirmed by a targeted quantitative analysis. Pathway analysis and relational network construction were conducted to disclose the corresponding pathways associated with HQLYD treatment and to aid in evaluating unknown treatments, which may increase the comprehension of the therapeutic mechanism of HQLYD and support the expansion of emerging drugs.
2. Materials and methods
2.1 Materials
Liquid chromatography-grade methanol, acetonitrile and formic acid were purchased from Merck (Darmstadt, Germany). Standard leucine enkephalin (purity ≥ 99%) was obtained from the National Institute for the Control of Pharmaceutical and Biological Products (Beijing, China). Citric acid and sodium citrate were obtained from Sinopharm Chemical Reagent Co. Ltd (Beijing, China). Streptozotocin (STZ) was obtained from the National Institute for the Control of Biological and Pharmaceutical Products of China. 0.9% sodium chloride injection and pentobarbital sodium were obtained from Sanling Pharmaceutical Co., Ltd (Jiangsu, China). The fasting blood glucose (FBG), total cholesterol (TC), triglyceride (TG), high-density lipoprotein cholesterol (HDL) and low-density lipoprotein cholesterol (LDL) kits were obtained from Shanghai Source Leaf Biological Technology Co. Ltd (Shanghai, China). The iodine [125I] insulin radioimmunoassay kit was obtained from MREDA Technology Inc. (Palo Alto, CA, USA). The superoxide dismutase (SOD), malonic dialdehyde (MDA), glutathione peroxidase (GSP-x), and catalase (CAT) kits were obtained from Weifang SanWei Biotechnology Institute (Beijing, China). The serum creatinine (Scr), aspartate aminotransferase (AST), alanine aminotransferase (ALT), and urea (Urea) kits were obtained from Pierce (Rockford IL USA). Astragalus root slices and licorice slices were purchased from Tongrentang Drug Store (Beijing, China).
2.2 Preparation of Huangqiliuyi decoction and other solutions
After soaking for 2 h, Astragalus root slices (600 g) and licorice slices (100 g) were boiled one time in 2 L distilled water, and then the decoction liquid was immediately filtered. The residual medicinal slices were put in 2 L distilled water again and boiled for 0.5 h. The filtrate obtained by boiling twice was combined and then concentrated to 1 g mL−1 crude drug density. The decoction was transferred to a brown bottle, sealed and stored at 4 °C in a refrigerator until use. Citric acid-sodium citrate buffer was prepared as follows: citric acid (2.1 g) and sodium citrate (2.94 g) were each dissolved in 100 mL distilled water to obtain 21 mg mL−1 citric acid and 29.4 mg mL−1 sodium citrate solutions. A mixture containing 28 mL citric acid solution and 22 mL sodium citrate solution was diluted to 100 mL using distilled water and the pH was adjusted to 4.5. Streptozotocin solution (STZ) was obtained by dissolving 30 mg of STZ in 1 mL of citric acid-sodium citrate buffer, which was subsequently filtered to remove impurities.
2.3 Animals model establishment
50 Sprague-Dawley (SD) male rats weighing 200 ± 20 g and six weeks of age were purchased from the Drug Safety Evaluation Hub of Tianjin University of Traditional Chinese Medicine. Animal care and experiments were carried out according to the Guide for the Care and Use of Laboratory Animals (US National Research Council, 1996) and were approved by the Animal Experimental Ethics Committee of Heilongjiang University of Traditional Chinese Medicine. All SD rats were supported in a chamber with 12 h light/dark cycles at 22–24 °C and 45–55% humidity.
After seven days acclimatization, 12 rats were given a normal diet randomly as the control group and the rest of the rats were given the high-sugar and high-fat diet for 21 days. After fasting for 12 h, the rats in the control group were intraperitoneally injected with citric acid-sodium citrate buffer (pH = 4.5) and the rats with the special diet were intraperitoneally injected with an equal dose of STZ (40 mg kg−1) dissolved in citric acid-sodium citrate buffer (pH = 4.5). At three days after the last drug administration, rats with blood glucose ≥16.7 mmol L−1 were considered as diabetic rats, and a total of 32 successful model rats were obtained. Twenty-four diabetes rats were randomly divided into two groups, including the model group and HQLTD group. On the 7th day after modeling, the animals in the HQLTD group were given 12.60 g kg−1 HQLTD once daily, other rats in the control and model group were administered with the identical bulk of saline solution in gavage way. The intragastric dose was altered considering body weight and successively treated for one month.
2.4 Biochemical index detection
All the rats from each group were anesthetized by pentobarbital sodium, blood was collected in Eppendorf tubes from the aorta abdominalis at the end of the treatment period and then immediately centrifuged at 4000 rpm for 10 min at 4 °C. The obtained biomedical samples for biochemical index detection and metabolomics analysis were stored at −80 °C until use. Meanwhile, the kidney and liver tissues of the rats were collected and quickly frozen in liquid nitrogen gas before the kit tests. The serum levels of TC, TG, HDL, LDL, Scr, AST, ALT, and urea were assessed using the appropriate kit according to the manufacturer's protocols and a TBA-30FR fully automatic biochemical analyzer (Toshiba, Tokyo, Japan). The serum FBG was detected every week using a Roche blood glucose meter equipped with blood glucose test paper, where the tail tip was cut to 5 to 10 mm for blood collection. The content of fasting insulin (FINS) was determined using a radioimmunoanalyzer, and the resistance index (IRS) was calculated as follows: (fasting insulin × fasting glucose)/22.5. The thawed kidney and liver tissues were homogenated in ice physiological saline at a ratio of 1
:
9 to monitor the activity of SOD, MDA, GSP-x, and CAT using ELISA kits.
2.5 Metabolomic study
On the eve of UPLC-MS analysis, the serum samples were thawed on ice at 4 °C. 200 μL of each serum sample from each group was deproteinized with 700 μL of methanol, and then centrifuged at 4000 rpm, 4 °C for 15 min. The supernatant liquor was hollowed via nitrogen gas and dissolved again in 120 μL of methanol. The quality control (QC) sample ensured the reliability of the data during the whole analysis process, which was mixed 20 μL of solution from each serum sample and injected three times at the beginning of the instrument operation, then every six samples were tested.
A UPLC system (Waters Corp., Milford, MA/USA) coupled to a Micromass Q-TOF Micro™ system (Waters Corporation, Milfold, MA, USA) was employed for metabolomic analysis of the serum samples. A 100 nm × 2.1 mm ACQUITY UPLC HSS T3 1.8 μm analytical column was utilized to detach metabolites, and the column and sample manager temperatures were set at 40 °C and 4 °C, respectively, and the flow rate was maintained at 0.3 mL min−1. After the screening, the optimal mobile phase was made up of 0.15% formic acid in acetonitrile (A) and 0.15% formic acid in water (B). The linear gradient was set as follows: 0–1 min, 1–10% A; 1–3 min, 10–45% A; 3–7 min, 45–73% A; 7–9.5 min, 73–99% A; 9.5–11.5 min, maintain 99%A; 11.5–12 min, and linear decrease from 99% to 1% A. Zevo G1 QTOFMS high-definition spectrometry (Waters Corp., Manchester, UK) was conducted for the comprehensive detection of changes in biological information, which possesses positive and negative electrostatic ionization modes. The detailed parameters are as follows: In ESI+ mode, capillary voltage: 3200 V, sample cone voltage: 35 V, and source temperature: 100 °C were set; in ESI− mode, capillary voltage: 2800 kV, sample cone voltage: 40 V, and source temperature: 110 °C were employed; and lock mass calibration of leucine enkephalin at m/z 556.2771 and 554.2615 was used to ensure stable and precise scanning, respectively.
2.6 Data processing
Raw spectral data of UPLC-MS was gained from MarkerLynx Applications Manager Version 4.1 (Waters), which contains main parameters such as retention time (Rt), mass range, mass tolerance, intensity threshold, retention time tolerance and noise liquidation level. Then, the ion strength of the investigated peaks with relevant Rt and mass data was tabulated. Each peak ion intensity for all samples was further picked and renormalised to the summation of the peak intensities in that sample. The processed data eliminated peaks with a loss value of over 60% sample and then imported into the SIMCA-P 12.0.1+ (Umetrics, Umea, Sweden) software for multivariate data analyses, including unsupervised component analysis (principal component analysis PCA) and supervised component analysis (partial least-squared discriminant analysis OPLS-DA). OPLS-DA is a supervised analysis model for better distinguishing different groups, in which the separation quality of a model is appraised by Q2 and R2Y. Q2 is an assessment of how fine the model forecasts Y and R2Y represents how fine the model fits the Y figures. Variable importance in the projection (VIP) was performed to explore latent biomarkers in accordance with the sill VIP > 1. Ions which met the condition of VIP > 1 and P < 0.05 in the t-test were screened for the discriminative analysis of endogenous biomarkers using the Human Metabolome Database (HMDB) and other databases, such as METLIN. The chemical structure of potential biomarkers was determined by corresponding fragment ion information adapted with the probable cleavage patterns of the gained precursor ions and fragment ions based on databases such as HMDB and MassFragment manager (Waters Corp., Milford, USA). Pathway analysis was carried out using the Metabolomics Pathway Analysis (MetPA) database and KEGG pathway database.
Experimental data is expressed as mean ± standard deviation (SD). Statistical analysis was performed using the SPSS software v19.0 (IBM Corp., Chicago, IL, USA) for the Student's t-test for contradistinction between two groups and one-way analysis of variance (ANOVA), where p values < 0.05 were premeditated statistically significant and p values < 0.01 were premeditated significantly significance.
3. Results
3.1 Biochemical index analysis
The animal model was established by the dietary model of high-sugar and high-fat. The animal model of type 2 diabetes mellitus was made successfully and examined for biochemical indicators of oxidative stress response, liver and kidney function, lipid metabolism and glucose metabolism measurement. Compared with the control group, the level of MDA in the liver and the level of MDA and CAT in the kidney prominently increased with p values of more than 0.01 (Fig. 1A–D). The level of SOD and GSP-x in the liver and kidney prominently decreased with p values of more than 0.01. The serum level of TG, LDL, Scr, AST and ALT prominently increased with p values of more than 0.01, and the level of TC, HDL and urea in the model rats is increased with p values of more than 0.01(Fig. 1E and F). With time, the serum level of FBG was always elevated in the model group beyond the normal range in comparison with that of the control group. The animals with type 2 diabetes mellitus presented a lower content of FINS and higher content IRS (Fig. 1G and H). The distinct content changes between the control and the type 2 diabetes mellitus rats demonstrate that biological alteration occurred in the model group. After HQLYD treatment for four weeks, the metabolic status of the model rats significantly improved in the content SOD, MDA, GSP-x and CAT in the liver, and serum FBG, TC, TG, HDL, Scr, ALT, FINS and IRS. Subsequently, potential biomarker discovery by metabolite analysis in serum metabolomics was carried out to determine the mechanism of type 2 diabetes mellitus and HQLYD therapeutic action.
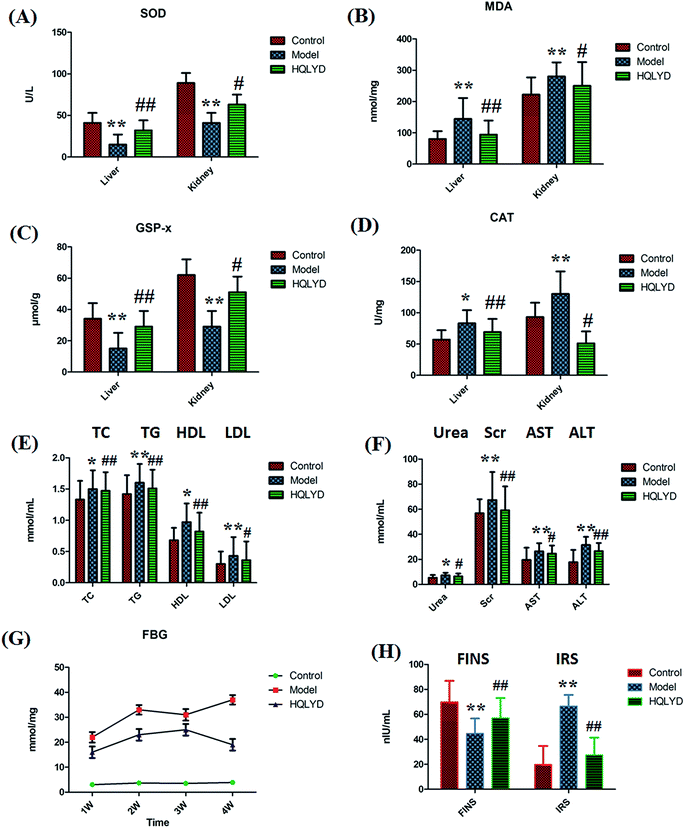 |
| Fig. 1 Therapeutic effect of HQLYD on type 2 diabetes mellitus was displayed in clinical biochemistry indexes of glucose metabolism, lipid metabolism, oxidative stress response, liver and kidney function compared with the control groups. *p < 0.05; **p < 0.01; compared with the model groups. #p < 0.05; ##p < 0.01. | |
3.2 Biomarker selection and identification
The metabolic characteristics of serum samples among the control, model and HQLYD group are displayed in Fig. 2A and B. The unsupervised PCA method suggests the tendency difference that every symbol embraces when they possess higher a resemblance of metabolomic compositions and have distinct metabolome in antagonistic circumstances. Preliminary separation between three groups was noticed from the PCA score plot, in which the scatter of the model group is distinctly away from the control group and the scatter of the HQLYD-treated group is close to the control group. This indicates that the animal model was prepared smoothly and HQLYD can regulate the metabolic disorder of type 2 diabetes mellitus. As a more sophisticated analysis, the subsequent OPLS-DA score plot (two-component model, R2X = 0.45, R2Y = 0.94, and Q2Y = 0.86 in positive ion mode; R2X = 0.29, R2Y = 0.83, and Q2Y = 0.59 in negative ion mode) was applied to confirm the above results in Fig. 3A and Fig. 4A, in which the animals in the model group were well-separated from those in the control group. Then wed explored whether the differentially delivered metabolites were liable for the separation among the three groups. As shown in Fig. 3B and 4B, the ions furthest away from the beginning appear as the distinguished metabolites in the corresponding loading-plots. The S-plots (Fig. 3C and 4C) and VIP-plots (Fig. 3D and 4D) were plotted following the OPLS analysis, which exhibit the variation and correlation of the metabolites, respectively. Potential biomarkers were screened considering VIP values more than 1 and p value less than 0.05 in the Student's t-test analysis.
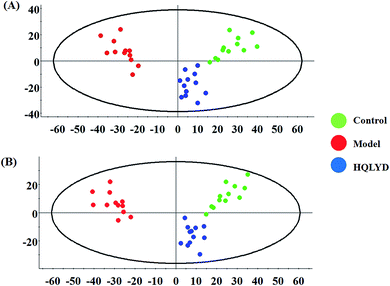 |
| Fig. 2 Principal component analysis score plots of the model rats with type 2 diabetes mellitus after HQLYD treatment in the positive and negative modes. | |
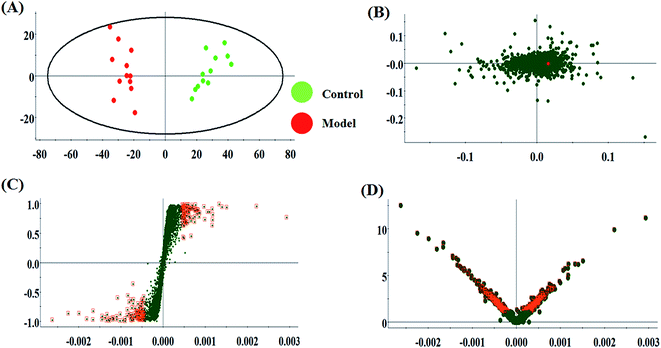 |
| Fig. 3 Multivariate analysis from metabolites in type 2 diabetes mellitus rats. (A) OPLS-DA score plot of serum metabolites for clustering the control and model groups in positive ion mode. (B) Loading plot of OPLS-DA model of serum metabolites for clustering the control and model groups in positive ion mode. (C) S-plot of OPLS-DA model of serum metabolites for clustering the control and model groups in positive ion mode. (D) VIP-plot of OPLS-DA model of serum metabolites for clustering the control and model groups in positive ion mode. | |
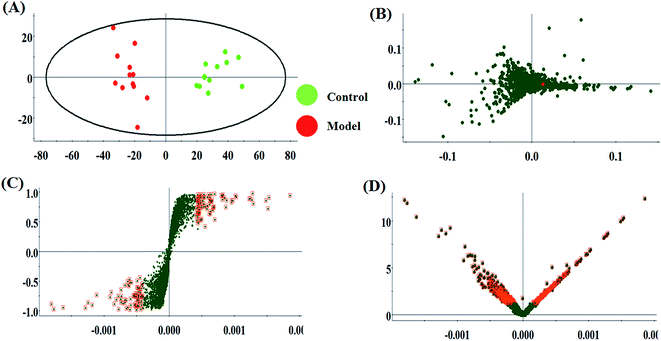 |
| Fig. 4 Multivariate analysis from metabolites in type 2 diabetes mellitus rat. (A) OPLS-DA score plot of serum metabolites for clustering the control and model groups in negative ion mode. (B) Loading plot of OPLS-DA model of serum metabolites for clustering the control and model groups in negative ion mode. (C) S-plot of OPLS-DA model of serum metabolites for clustering the control and model groups in negative ion mode. (D) VIP-plot of OPLS-DA model of serum metabolites for clustering the control and model groups in negative ion mode. | |
As presented in ESI Table 1,† 25 differentially expressed small molecule metabolites were identified using the consistency between the MS and MS/MS signal data and screening in network databases such as Mass-Bank, ChemSpider, KEGG and LIPIDMAPS. After HQLYD treatment, 16 of the serum metabolites changed significantly compared with the model rats, including galactose, citric acid, L-leucine, PE(14:0/24:1(15Z)), isocitric acid, acetylglycine, 3-hydroxybutyric acid, homocysteine, PC(18:1(9Z)/15:0), palmitic amide, PC(15:0/20:3(8Z, 11Z, 14Z)), uric acid, D-glucose, L-phenylalanine, D-glutamine, and L-tyrosine. As shown in Fig. 5, the clustering heatmap analysis of sixteen metabolites disclosed the differences in their relative contents among the control, model and HQLYD groups, in which the luminance fluctuation of the color displays the content alteration of the biomarker. The comparable signal intensities of the serum metabolites, as shown in Fig. 6, mirror the activity of HQLYD regulating type 2 diabetes mellitus, in which the level of isocitric acid, acetylglycine, 3-hydroxybutyric acid, PC(18:1(9Z)/15:0), palmitic amide, PC(15:0/20:3(8Z, 11Z, 14Z)), citric acid, L-leucine, uric acid, D-glucose, L-phenylalanine, D-glutamine and L-tyrosine observably changed after HQLYD treatment compared with the control group (P < 0.01).
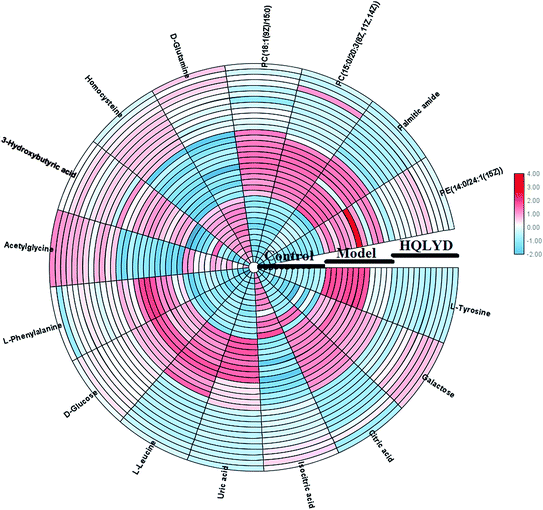 |
| Fig. 5 Hierarchical clustering heatmap of the sixteen differential metabolites regulated by HQLYD after one month of treatment with the degree of change marked with colors including up-regulation (red) and down-regulation (blue). | |
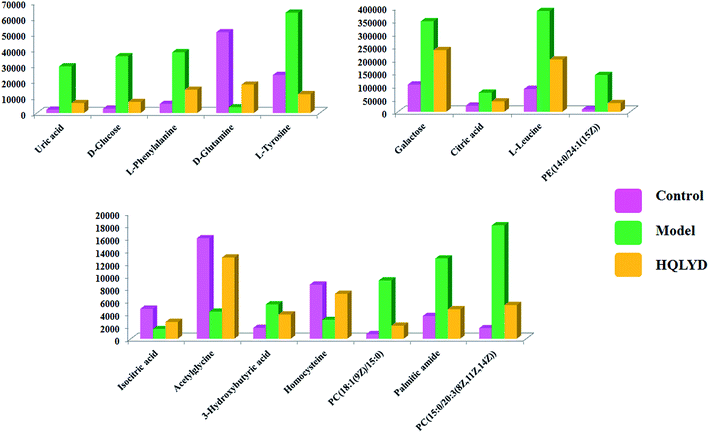 |
| Fig. 6 Relative signal intensities of metabolic biomarkers effectively changed by HQLYD treatment in serum sample selected from the control model and HQLYD-treated groups, in which the level of isocitric acid, acetylglycine, 3-hydroxybutyric acid, PC(18:1(9Z)/15:0), palmitic amide, PC(15:0/20:3(8Z,11Z, 14Z)), citric acid, L-leucine, uric acid, D-glucose, L-phenylalanine, D-glutamine and L-tyrosine observably changed after HQLYD compared with the control group (P < 0.01). | |
3.3 Pathway analysis
MetPA analysis is a free web-based pathway topology tool, which showed that the differentiated biomarkers were mainly involved in phenylalanine, tyrosine and tryptophan biosynthesis (impact value 1), phenylalanine metabolism (impact value 0.40741), valine, leucine and isoleucine biosynthesis (impact value 0.33333), glyoxylate and dicarboxylate metabolism (impact value 0.2963), glycerophospholipid metabolism (impact value 0.23056), tyrosine metabolism (impact value 0.14045), cysteine and methionine metabolism (impact value 0.1388), citrate cycle (TCA cycle) (impact value 0.09488), glycosylphosphatidylinositol (GPI)-anchor biosynthesis (impact value 0.0439), starch and sucrose metabolism (impact value 0.03778), galactose metabolism (impact value 0.03644), and purine metabolism (impact value 0.02077) in Fig. 7. The metabolite-metabolic pathway interaction network from KEGG in conformity with the reactions from indistinguishable chemical structures and molecular activities is presented in Fig. 8A, which mainly refers to citric acid, L-phenylalanine, L-leucine, L-tyrosine, uric acid, homocysteine, D-galactose, D-glucose, isocitric acid, and D-glutamine. The gene–metabolite interaction network enables exploration and visualization of interactions between functionally related metabolites and genes, which mainly involved citric acid, homocysteine, uric acid, L-leucine, L-phenylalanine, D-glucose, isocitric acid, L-tyrosine, PC(16:0/16:0), and D-galactose (Fig. 8).
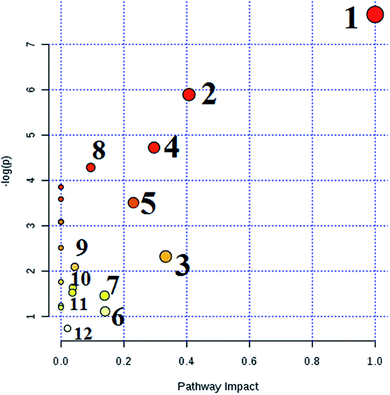 |
| Fig. 7 Pathway analysis of HQLYD therapeutic effect on type 2 diabetes mellitus rats by serum metabolomics. 1. Phenylalanine, tyrosine and tryptophan biosynthesis; 2. Phenylalanine metabolism; 3. Valine, leucine and isoleucine biosynthesis; 4. Glyoxylate and dicarboxylate metabolism; 5. Glycerophospholipid metabolism; 6. Tyrosine metabolism; 7. Cysteine and methionine metabolism; 8. Citrate cycle (TCA cycle); 9. Glycosylphosphatidylinositol(GPI)-anchor biosynthesis; 10. Starch and sucrose metabolism; 11. Galactose metabolism; and 12. Purine metabolism. | |
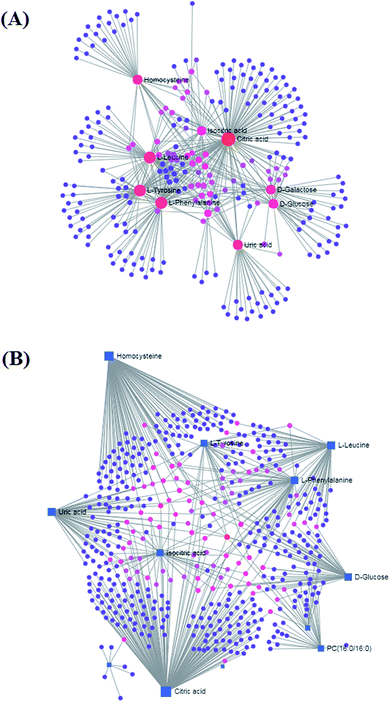 |
| Fig. 8 (A) Metabolite–metabolite interaction network related with HQLYD therapeutic effects resisting type 2 diabetes mellitus on the model animal. (B) Gene-metabolite interaction network of HQLYD therapeutic effects enables exploration and visualization of interactions between functionally related metabolites and genes in type 2 diabetes mellitus treatment. | |
4. Discussion
Type 2 diabetes is a multifactorial disease that is mainly affected by genetic factors and environmental factors. Insulin resistance (IR) is defined by the WHO as a common ground for T2DM and various complications caused by it.26,27 Currently, the “second strike” doctrine of oxidative stress has occupied the core position of IR research. Studies have shown that oxidative stress not only originates from IR, but also damages islet β cells and aggravates lipid metabolism disorder.28,29 Therefore, it is necessary to conduct reasonable external intervention on the diabetic oxidative stress state to observe whether it can reduce insulin by improving oxidative stress. After 4 weeks, the effects of HQLYD on blood glucose, blood lipids, liver and kidney tissues facing oxidative stress were explored in diabetic rats. After treatment with HQLYD, the SOD, MDA, GSP-x and CAT indexes of the liver and kidney tissues changed to the normal trend in contrast to the control group. The results show that HQLYD improved the peroxide level in body, which reflects that HQLYD has an anti-oxidation effect and can improve the oxidative stress level of diabetic rats. Simultaneously, it was found that HQLYD effectively reduced the abnormal levels of urea, Scr, AST and ALT in the diabetic rats. Studies have shown that ALT may be an important reference index reflecting insulin resistance and metabolic disorders in sufferers with newly diagnosed diabetes.30,31 In sufferers with type 2 diabetes mellitus, the severity of retinopathy increases and serum urea nitrogen levels are negatively correlated with retinal fiber layer thickness.32,33 It can be seen that HQLYD effectively improved liver and kidney dysfunction in the diabetic rats. In the regulation of blood lipids, blood lipids TC, TG, HDL (p < 0.01) and LDL (p < 0.05) were notably lower in the diabetic rats after HQLYD treatment. In terms of insulin, HQLYD can raise insulin and lower blood sugar. In recent years, IRS has become an important indicator for assessing insulin resistance.34 The IRS in the serum of the model group increased in contrast to that of the normal group (P < 0.01), suggesting that the insulin sensitivity in diabetes was reduced and insulin resistance occurred. Compared with the model group, HQLYD significantly relieved the IRS level (P < 0.01). Thus, the biochemical indicators show that HQLYD can treat diabetes by perfecting glucose and lipid metabolism and oxidative stress in diabetic rats.
Mass spectrometry-based metabolomics has enabled the discovery of many biomarkers and provides insight into pathogenesis,35–55 disease diagnosis,56–75 treatment prognosis,76–80 and monitoring testing.81–87 Some researchers have found that amino acids are the final metabolites of proteins. The amino acid spectrum of diabetic patients undergoes characteristic changes. It has also been reported by the Framingham Offspring Study in 2011 that five types of amino acids in blood, including isoleucine, leucine, valine, tyrosine and phenylalanine, increase abnormally in the early stage of type 2 diabetes.88 As one of the essential amino acids for extrahepatic oxidation, phenylalanine in the free state and other amino acids will be the main source of energy supply when insulin secretion is insufficient and the body cannot make full use of glucose and fatty acids, which leads to a large number of phenylalanine appearing in the serum.89 L-leucine is a branched-chain amino acid (BCAAs) together with isoleucine and valine, which play a key role during the insulin secernment, protein turnover and synthesis adjustment. The increased fasting level of circulating BCAAs is connected with in increase in the threat of type 2 diabetes mellitus, insulin resistance and other metabolic diseases in humans on account of the lowered physiological activity of branched-chain α-keto acid dehydrogenase.90 L-tyrosine, an aromatic amino acid, is a glucogenic and ketogenic amino acid. Studies have found that L-tyrosine has resistive effects on the pathological development of diabetes.91 Compared with the control group, the content of serum phenylalanine, tyrosine and leucine increased in the model group, which indicates that diabetic rats had insufficient blood sugar utilization, increased protein catabolism and weakened anabolism. Sugar-producing amino acids were consumed in large quantities and their content decreased. HQLYD therapy effectively regulated the metabolic activities of phenylalanine, tyrosine and tryptophan biosynthesis, phenylalanine metabolism, and valine, leucine and isoleucine biosynthesis. Homocysteine (HCY) is a sulfur-containing amino acid generated by methionine metabolism. 80% of HCY binds to protein through the disulfide bond in the blood, and only a small amount of free homocysteine participates in circulation.92 Compared with the control group, the content of HCY in the model rats was lower than normal. HQLYD regulated the metabolism of cysteine and methionine to make the content of HCY trend to normal in blood. Lipids are the most abundant metabolites in human serum. After the body ingests a large amount of glucose and fat, the synthesis of cholesterol and triglyceride increases under the action of insulin, and the storage of lipids in peripheral tissues also increases. A long-term increase in lipid metabolites leads to insulin resistance in peripheral tissues in the initial stage, which is still in the early stage of onset for developing diabetes.93 The serum levels of cholesterol esters, free fatty acids, phospholipids and triglycerides in diabetic patients are higher than that in the normal glucose tolerance group. It has been found that the fatty acid composition of serum lipids and skeletal muscle phospholipids can affect insulin sensitivity in elderly diabetic patients, which further confirms the relationship between lipid metabolism and type 2 diabetes mellitus.94 In our investigation, HQLYD had an intense influence on glycerophospholipids, such as PC and PE, which were observably elevated in the model rats. PC and PE as the glycerophospholipids with the highest contents in mammals are vital elements in biomemebranes and transport the majority of membrane lipids within cells. Glycerophospholipid species are synthesized downstream of DG, resulting in many metabolic diseases. The synthesis of both PC and PE occurs through DG in most cells, and an increase in PC and PE is closely associated with obesity, insulin resistance and other metabolic syndromes.95 PC forms lipid droplets and lipoproteins accumulation during the lipid storage process, which are both increased risks of obesity. It was suggested that the incremental increase in PC in liver-derived microsomes in vitro or the extra PC level in the membrane restrains calcium homeostasis in organelles regulated by the calcium transport activity of sarcoendoplasmic reticulum Ca2+-ATPase (SERCA), which results in protein misfolding and endoplasmic reticulum (ER) stress, and finally stimulates insulin resistance.96,97 HQLYD validly reduced the contents of PC(18:1(9Z)/15:0), PC(15:0/20:3(8Z,11Z, 14Z)) and PE(14:0/24:1(15Z)) by regulating glycerophospholipid metabolism and glycosylphosphatidylinositol(GPI)-anchor biosynthesis. D-glucose exists in the blood, spinal fluid and lymph of animals, and is used as a nutrient for diuretic, detoxification and cardiotonic. HQLYD directly regulated D-glucose by promoting starch, sucrose and galactose metabolism to reduce the abnormal level of D-glucose in the model rats.
Proteins, lipids, and sugars can be directly or indirectly converted through the tricarboxylic acid cycle (TCA). In our study, citric acid and isocitrate as differential metabolites of type 2 diabetes were involved in the metabolic pathway of TCA cycle. Insulin is a citrate synthase activator, which causes the TCA cycle to be blocked when it is insufficient. It is also an inhibitor of lipolytic enzyme, and it causes fat decomposition to accelerate, and acetyl COA production and ketone bodies increase when it is insufficient in diabetic patients. The increased content of citric acid and the decreased content of isocitric acid in the model group indicated that disorder in the TCA occurred in the rats.98,99 After HQLYD treatment, the content of citric acid and isocitrate acid trended to the level of control group, which suggests that HQLYD promoted the TCA cycle and ensured the normal metabolism of proteins, lipids and sugars in the diabetic rats. Studies have reported that a high level of uric acid (UA) is an independent risk factor for type 2 diabetes. With an increase in age, the blood UA of diabetic patients gradually increases, which is more prominent in males than females.100 There is still no clear answer to the mechanism of diabetes caused by hyperuricemia. However, hyperuricemia is an inevitable result of purine metabolism disorder. Abnormal purine metabolism due to any cause and/or abnormal renal excretion of UA can lead to elevated blood UA levels. Relevant animal experiments have shown that insulin relies on an NO-mediated mechanism for the uptake of glucose; however, high levels of blood UA can inhibit the biological activity of NO, and then destroy endothelial cells, leading to endothelial cell dysfunction. Ultimately insulin resistance can arise due to the inhibition of glucose uptake leading to the development of type 2 diabetes. UA also has the potential to increase the risk of systemic inflammatory responses and oxidative stress, which are closely related to type 2 diabetes.101 HQLYD can effectively control the abnormal increase of UA level and regulate the purine metabolism to be balanced. 3-Hydroxybutyrate is a type of ketone body in the body, which is mainly produced by the oxidative metabolism of fatty acids in the liver to produce acetyl-CoA. Muscle tissue can utilize ketone bodies to prevent excessive consumption of protein in the body. 3-Hydroxybutyric acid is closely related to the occurrence of diseases, such as energy metabolism disorder and diabetes. It has been reported that 3-hydroxybutyrate is catalyzed by 3-hydroxybutyrate dehydrogenase to produce acetoacetate, which provides energy for the activities of cells such as brain and heart muscle when the body is starved or insulin is below normal. The abnormal increase in the content of 3-hydroxybutyrate in the model group may be the result of the lack of insulin in the diabetic rats, which led to the compensatory increase to supply the energy required for physiological activities in the body.102
5. Conclusion
The indispensable power of this research is its global plan to reveal insight into the mechanism of HQLYD on type 2 diabetes mellitus using a serum metabolomic strategy. It searched for and identified meaningful representative metabolite markers associated with HQLYD treatment, and the obtained biomarkers were verified by serum metabolic profiling and quantitative analysis. Furthermore, biochemical indicators such as blood lipid, blood glucose, oxidative stress and liver and kidney function as an auxiliary tool were applied to explore the pharmacological mechanism of HQLYD. The therapeutic effect of HQLYD on type 2 diabetes mellitus ameliorating glucose and lipid metabolism and oxidative stress reaction occurred based on multiple elements acting on various aims in different pathways, which regulated sixteen serum biomarkers and mainly affected amino acid metabolism, lipid metabolism and glucose metabolism, especially, the phenylalanine, tyrosine and tryptophan biosynthesis pathway was affected the most. This study provides a systemic novel way to disclose the scientific foundation of traditional Chinese medicine formulas.
Conflicts of interest
There are no conflicts to declare.
Acknowledgements
This work was financially supported by the National Natural Science Foundation of China (No. 31770688), the National Public Welfare Industry Special (No. 201404718), the Harbin Research and Development Fund of Applied Technology (No. 2017RAQXJ129) and the Scientific Research Fund of the Health Planning Committee of Heilongjiang (No. 2017-579).
References
- B. Carstensen, M. E. Jørgensen and S. Friis, The epidemiology of diabetes and cancer, Curr. Diabetes Rep., 2014, 14(10), 535 CrossRef PubMed.
- R. A. DeFronzo, E. Ferrannini and L. Groop, et al., Type 2 diabetes mellitus, Nat Rev Dis Primers, 2015, 1, 15019 CrossRef PubMed.
- K. L. Way, D. A. Hackett and M. K. Baker, et al., The Effect of Regular Exercise on Insulin Sensitivity in Type 2 Diabetes Mellitus: A Systematic Review and Meta-Analysis, Diabetes Metab J, 2016, 40(4), 253–271 CrossRef PubMed.
- N. Zhang, X. Yang and X. Zhu, et al., Type 2 diabetes mellitus unawareness, prevalence, trends and risk factors: National Health and Nutrition Examination Survey (NHANES) 1999–2010, J. Int. Med. Res., 2017, 45(2), 594–609 CrossRef PubMed.
- P. Kotlarsky, A. Bolotin and K. Dorfman, et al., Link between retinopathy and nephropathy caused by complications of diabetes mellitus type 2, Int. Ophthalmol., 2015, 35(1), 59–66 CrossRef PubMed.
- S. Spiller, Y. Li and M. Blüher, et al., Diagnostic Accuracy of Protein Glycation Sites in Long-Term Controlled Patients with Type 2 Diabetes Mellitus and Their Prognostic Potential for Early Diagnosis, Pharmaceuticals, 2018, 11(2) DOI:10.3390/ph11020038.
- N. Gray, G. Picone and F. Sloan, et al., Relation between BMI and diabetes mellitus and its complications among US older adults, South. Med. J., 2015, 108(1), 29–36 CrossRef PubMed.
- M. Riant, A. Meirhaeghe and J. Giovannelli, et al., Associations between long-term exposure to air pollution, glycosylated hemoglobin, fasting blood glucose and diabetes mellitus in Northern France, Environ. Int., 2018, 120, 121–129 CrossRef CAS PubMed.
- D. Jakubowicz, J. Wainstein and Z. Landau, et al., High-energy breakfast based on whey protein reduces body weight, postprandial glycemia and HbA1C in Type 2 diabetes, J. Nutr. Biochem., 2017, 49, 1–7 CrossRef CAS PubMed.
- C. Meehan and J. Silverstein, Treatment Options for Type 2 Diabetes in Youth Remain Limited, J. Pediatr., 2016, 170, 20–27 CrossRef PubMed.
- G. Grunberger, Should Side Effects Influence the Selection of Antidiabetic Therapies in Type 2 Diabetes?, Curr. Diabetes Rep., 2017, 17(4), 21 CrossRef PubMed.
- L. J. Xiao and R. Tao, Traditional Chinese Medicine (TCM) Therapy, Adv. Exp. Med. Biol., 2017, 1010, 261–280 CrossRef PubMed.
- J. Zhu, L. Shen and X. Lin, et al., Clinical Research on Traditional Chinese Medicine compounds and their preparations for Amyotrophic Lateral Sclerosis, Biomed. Pharmacother., 2017, 96, 854–864 CrossRef CAS PubMed.
- P. K. Lai, J. Y. Chan and L. Cheng, et al., Isolation of anti-inflammatory fractions and compounds from the root of Astragalus membranaceus, Phytother. Res., 2013, 27(4), 581–587 CrossRef CAS PubMed.
- M. Liu, K. Wu and X. Mao, et al., Astragalus polysaccharide improves insulin sensitivity in KKAy mice: regulation of PKB/GLUT4 signaling in skeletal muscle, J. Ethnopharmacol., 2010, 127(1), 32–37 CrossRef CAS PubMed.
- N. Wang, D. L. Zhang and X. Q. Mao, et al., Astragalus polysaccharides decreased the expression of PTP1B through relieving ER stress induced activation of ATF6 in a rat model of type 2 diabetes, Mol. Cell. Endocrinol., 2009, 307(1), 89–98 CrossRef CAS PubMed.
- L. Lv, S. Y. Wu and G. F. Wang, et al., Effect of astragaloside IV on hepatic glucose-regulating enzymes in diabetic mice induced by a high-fat diet and streptozotocin, Phytother. Res., 2010, 24(2), 219–224 CAS.
- M. Kuroda, Y. Mimaki and S. Honda, et al., Phenolics from Glycyrrhiza glabra roots and their PPAR-γ ligand-binding activity, Bioorg. Med. Chem., 2010, 18(2), 962–970 CrossRef CAS PubMed.
- K. Honda, H. Kamisoyama and Y. Tominaga, et al., The molecular mechanism underlying the reduction in abdominal fat accumulation by licorice flavonoid oil in high fat diet-induced obese rats, Anim. Sci. J., 2009, 80(5), 562–569 CrossRef CAS PubMed.
- S. Dal Rhee, C. H. Kim and J. S. Park, et al., Carbenoxolone prevents the development of fatty liver in C57BL/6-Lep ob/ob mice via the inhibition of sterol regulatory element binding protein-1c activity and apoptosis, Eur. J. Pharmacol., 2012, 691(1), 9–18 CrossRef CAS PubMed.
- X. Wang, X. Gao and A. Zhang, et al., High-throughput metabolomics for evaluating the efficacy and discovering the metabolic mechanism of Luozhen capsules from the excessive liver-fire syndrome of hypertension, RSC Adv., 2019, 9(55), 32141–32153 RSC.
- A. Zhang, Z. Ma and H. Sun, et al., High-Throughput Metabolomics Evaluate the Efficacy of Total Lignans From Acanthophanax Senticosus Stem Against Ovariectomized Osteoporosis Rat, Front. Pharmacol., 2019, 10, 553 CrossRef PubMed.
- A. Zhang, H. Sun and G. Yan, et al., Mass spectrometry-based metabolomics: applications to biomarker and metabolic pathway research, Biomed. Chromatogr., 2016, 30(1), 7–12 CrossRef.
- H. Dong, A. Zhang and H. Sun, et al., Ingenuity pathways analysis of urine metabolomics phenotypes toxicity of Chuanwu in Wistar rats by UPLC-Q-TOF-HDMS coupled with pattern recognition methods, Mol. BioSyst., 2012, 8(4), 1206–1221 RSC.
- A. Zhang, H. Sun and P. Wang, et al., Modern analytical techniques in metabolomics analysis, Analyst, 2012, 137(2), 293–300 RSC.
- I. Kanazawa, M. Notsu and A. Takeno, et al., Overweight and underweight are risk factors for vertebral fractures in patients with type 2 diabetes mellitus, J. Bone Miner. Metab., 2019, 37(4), 703–710 CrossRef CAS PubMed.
- Y. Xu, W. Shi and R. Song, et al., Divergent patterns of genic copy number variation in KCNIP1 gene reveal risk locus of type 2 diabetes in Chinese population, Endocr. J., 2018, 65(5), 537–545 CrossRef CAS PubMed.
- R. C. R. Meex, E. E. Blaak and L. J. C. van Loon, et al., Lipotoxicity plays a key role in the development of both insulin resistance and muscle atrophy in patients with type 2 diabetes, Obes. Rev., 2019, 20(9), 1205–1217 CrossRef CAS PubMed.
- M. L. Zuo, A. P. Wang and Y. Tian, et al., Oxymatrine ameliorates insulin resistance in rats with type 2 diabetes by regulating the expression of KSRP, PETN, and AKT in the liver, J. Cell. Biochem., 2019, 120(9), 16185–16194 CrossRef CAS PubMed.
- M. U. R. Abro, A. Butt and K. Baqa, et al., Association of serum liver enzyme Alanine Aminotransferase (ALT) in patients with type 2 diabetes, Pak J Med Sci, 2018 Jul-Aug, 34(4), 839–843 Search PubMed.
- V. Kuzhandai velu, B. Jyothirmayi and J. S. Kumar, Insulin resistance and alanine amino transaminase (ALT) levels in first degree relatives of type 2 diabetes mellitus, Diabetes Metab Syndr, 2011, 5(3), 143–147 CrossRef CAS PubMed.
- Y. Y. Xiao, M. K. Yuan and P. Xi, et al., Relationship between carotid plaque and diabetic retinopathy in type 2 diabetes mellitus patients, Zhonghua Yi Xue Za Zhi, 2017, 97(24), 1871–1874 CAS.
- Y. H. Chong, Q. Fan and Y. C. Tham, et al., Type 2 Diabetes Genetic Variants and Risk of Diabetic Retinopathy, Ophthalmology, 2017, 124(3), 336–342 CrossRef PubMed.
- M. Pourfarzam, F. Zadhoush and M. Sadeghi, et al., The difference in correlation between insulin resistance index and chronic inflammation in type 2 diabetes with and without metabolic syndrome, Adv. Biomed. Res., 2016, 5, 153 CrossRef PubMed.
- A. Zhang, H. Sun and S. Qiu, et al., Metabolomics in noninvasive breast cancer, Clin. Chim. Acta, 2013, 424, 3–7 CrossRef CAS PubMed.
- A. H. Zhang, J. B. Yu and H. Sun, et al., Identifying quality-markers from Shengmai San protects against transgenic mouse model of Alzheimer's disease using chinmedomics approach, Phytomedicine, 2018, 45, 84–92 CrossRef CAS PubMed.
- A. Zhang, H. Sun and H. Xu, et al., Cell metabolomics, OMICS: J. Integr. Biol., 2013, 17(10), 495–501 CrossRef CAS PubMed.
- X. Wang, A. Zhang and X. Zhou, et al., An integrated chinmedomics strategy for discovery of effective constituents from traditional herbal medicine, Sci. Rep., 2016, 6, 18997 CrossRef CAS PubMed.
- Y. F. Li, S. Qiu and L. J. Gao, et al., Metabolomic estimation of the diagnosis of hepatocellular carcinoma based on ultrahigh performance liquid chromatography coupled with time-of-flight mass spectrometry, RSC Adv., 2018, 8(17), 9375–9382 RSC.
- X. Wang, J. Li and A. H. Zhang, Urine metabolic phenotypes analysis of extrahepatic cholangiocarcinoma disease using ultra-high performance liquid chromatography-mass spectrometry, RSC Adv., 2016, 6(67), 63049–63057 RSC.
- A. Zhang, H. Sun and G. Yan, et al., Metabolomics study of type 2 diabetes using ultra-performance LC-ESI/quadrupole-TOF high-definition MS coupled with pattern recognition methods, J. Physiol. Biochem., 2014, 70(1), 117–128 CrossRef CAS PubMed.
- H. Wang, G. Yan and A. Zhang, et al., Rapid discovery and global characterization of chemical constituents and rats metabolites of Phellodendri amurensis cortex by ultra-performance liquid chromatography-electrospray ionization/quadrupole-time-of-flight mass spectrometry coupled with pattern recognition approach, Analyst, 2013, 138(11), 3303–3312 RSC.
- A. Zhang, H. Sun and S. Qiu, et al., Metabolomics insights into pathophysiological mechanisms of nephrology, Int. Urol. Nephrol., 2014, 46(5), 1025–1030 CrossRef CAS PubMed.
- H. Sun, A. H. Zhang and Q. Song, et al., Functional metabolomics discover pentose and glucuronate interconversion pathways as promising targets for Yang Huang syndrome treatment with Yinchenhao Tang, RSC Adv., 2018, 8, 36831–36839 RSC.
- Y. Zhao, H. Lv and S. Qiu, et al., Plasma metabolic profiling and novel metabolite biomarkers for diagnosing prostate cancer, RSC Adv., 2017, 7(48), 30060–30069 RSC.
- X. N. Li, A. Zhang and M. Wang, et al., Screening the active compounds of Phellodendri Amurensis cortex for treating prostate cancer by high-throughput chinmedomics, Sci. Rep., 2017, 7, 46234 CrossRef CAS PubMed.
- A. Zhang, H. Sun and Y. Han, et al., Urinary metabolic biomarker and pathway study of hepatitis B virus infected patients based on UPLC-MS system, PLoS One, 2013, 8(5), e64381 CrossRef CAS PubMed.
- H. Sun, A. Zhang and X. Wang, Potential role of metabolomic approaches for Chinese medicine syndromes and herbal medicine, Phytother. Res., 2012, 26(10), 1466–1471 CAS.
- A. Zhang, H. Sun and P. Wang, et al., Salivary proteomics in biomedical research, Clin. Chim. Acta, 2013, 415, 261–265 CrossRef CAS PubMed.
- H. Fang, A. H. Zhang and H. Sun, et al., High-throughput metabolomics screen coupled with multivariate statistical analysis identifies therapeutic targets in alcoholic liver disease rats using liquid chromatography-mass spectrometry, J. Chromatogr. B: Anal. Technol. Biomed. Life Sci., 2019, 1109, 112–120 CrossRef CAS.
- H. Cao, A. Zhang and H. Zhang, et al., The application of metabolomics in traditional Chinese medicine opens up a dialogue between Chinese and Western medicine, Phytother. Res., 2015, 29(2), 159–166 CrossRef PubMed.
- X. Liu, A. Zhang and H. Fang, et al., Serum metabolomics strategy for understanding the therapeutic effects of Yin-Chen-Hao-Tang against Yanghuang syndrome, RSC Adv., 2018, 8, 7403–7413 RSC.
- A. Zhang, H. Sun and G. Yan, et al., Metabolomics in diagnosis and biomarker discovery of colorectal cancer, Cancer Lett., 2014, 345(1), 17–20 CrossRef CAS PubMed.
- A. Zhang, H. Sun and Y. Han, et al., Exploratory urinary metabolic biomarkers and pathways using UPLC-Q-TOF-HDMS coupled with pattern recognition approach, Analyst, 2012, 137(18), 4200–4208 RSC.
- X. Li, A. Zhang and H. Sun, et al., Metabolic characterization and pathway analysis of berberine protects against prostate cancer, Oncotarget, 2017, 8, 65022–65041 Search PubMed.
- H. Fang, A. Zhang and J. Yu, et al., Insight into the metabolic mechanism of scoparone on biomarkers for inhibiting Yanghuang syndrome, Sci. Rep., 2016, 6, 37519 CrossRef CAS PubMed.
- A. Zhang, H. Sun and G. Yan, et al., Mass spectrometry-based metabolomics: applications to biomarker and metabolic pathway research, Biomed. Chromatogr., 2016, 30(1), 7–12 CrossRef PubMed.
- H. Chu, A. Zhang and Y. Han, et al., Metabolomics approach to explore the effects of Kai-Xin-San on Alzheimer's disease using UPLC/ESI-Q-TOF mass spectrometry, J. Chromatogr. B, 2016, 1015, 50–61 CrossRef PubMed.
- A. Zhang, H. Sun and G. Yan, et al., Metabolomics for biomarker discovery: moving to the clinic, BioMed Res. Int., 2015, 2015, 354671 Search PubMed.
- H. Sun, H. Wang and A. Zhang, et al., Berberine ameliorates nonbacterial prostatitis via multi-target metabolic network regulation, OMICS: J. Integr. Biol., 2015, 19(3), 186–195 CrossRef CAS PubMed.
- A. Zhang, H. Sun and X. Wang, Emerging role and recent applications of metabolomics biomarkers in obesity disease research, RSC Adv., 2017, 7(25), 14966–14973 RSC.
- H. Xiong, A. H. Zhang and Q. Q. Zhao, et al., Discovery and screening quality-marker ingredients of Panax quinquefolius using chinmedomics approach, Phytomedicine, 2019, 152928 CrossRef PubMed.
- A. Zhang, H. Wang and H. Sun, et al., Metabolomics strategy reveals therapeutical assessment of limonin on nonbacterial prostatitis, Food Funct., 2015, 6(11), 3540–3549 RSC.
- H. L. Zhang, A. H. Zhang and X. H. Zhou, et al., High-throughput lipidomics reveal mirabilite regulating lipid metabolism as anticancer therapeutics, RSC Adv., 2018, 8(62), 35600–35610 RSC.
- H. Sun, A. Zhang and L. Yang, et al., High-throughput chinmedomics strategy for discovering the quality-markers and potential targets for Yinchenhao decoction, Phytomedicine, 2019, 54, 328–338 CrossRef CAS PubMed.
- H. Sun, A. Zhang and G. Yan, et al., Metabolomic analysis of key regulatory metabolites in hepatitis C virus–infected tree shrews, Mol. Cell. Proteomics, 2013, 12(3), 710–719 CrossRef CAS PubMed.
- A. Zhang, H. Sun and X. Wang, Potentiating therapeutic effects by enhancing synergism based on active constituents from traditional medicine, Phytother. Res., 2014, 28(4), 526–533 CrossRef PubMed.
- Y. Nan, X. Zhou and Q. Liu, et al., Serum metabolomics strategy for understanding pharmacological effects of ShenQi pill acting on kidney yang deficiency syndrome, J. Chromatogr. B, 2016, 1026, 217–226 CrossRef CAS PubMed.
- J. Xie, A. H. Zhang and S. Qiu, Identification of the perturbed metabolic pathways associating with prostate cancer cells and anticancer affects of obacunone, J. Proteomics, 2019, 206, 103447 CrossRef CAS PubMed.
- A. Zhang, H. Sun and P. Wang, et al., Future perspectives of personalized medicine in traditional Chinese medicine: a systems biology approach, Complement Ther Med, 2012, 20(1–2), 93–99 CrossRef PubMed.
- Y. Zhang, P. Liu and Y. Li, et al., Exploration of metabolite signatures using high-throughput mass spectrometry coupled with multivariate data analysis, RSC Adv., 2017, 7, 6780–6787 RSC.
- L. Wang, H. Dong and A. H. Zhang, et al., Exploring the detoxification effects and mechanism of Caowu in prescription using liquid chromatography-high-resolution mass spectrometry-based metabolomics, Open Journal of Proteomics and Genomics, 2018, 3(1), 011–023 Search PubMed.
- A. H. Zhang, H. Sun and G. L. Yan, et al., Chinmedomics: A Powerful Approach Integrating Metabolomics with Serum Pharmacochemistry to Evaluate the Efficacy of Traditional Chinese Medicine, Engineering, 2019, 5, 60–68 CrossRef CAS.
- H. Sun, H. L. Zhang and A. H. Zhang, et al., Network pharmacology combined with functional metabolomics discover bile acid metabolism as a promising target for mirabilite against colorectal cancer, RSC Adv., 2018, 8, 30061–30070 RSC.
- Q. Liang, H. Liu and X. Li, et al., High-throughput metabolomics analysis discovers salivary biomarkers for predicting mild cognitive impairment and Alzheimer's disease, RSC Adv., 2016, 6, 75499–75504 RSC.
- X. J. Wang, J. L. Ren and A. H. Zhang, et al., Novel applications of mass spectrometry-based metabolomics in herbal medicines and its active ingredients: current evidence, Mass Spectrom. Rev., 2019, 38(4–5), 380–402 CrossRef PubMed.
- H. Sun, A. H. Zhang and S. B. Liu, et al., Cell metabolomics identify regulatory pathways and targets of magnoline against prostate cancer, J. Chromatogr. B: Anal. Technol. Biomed. Life Sci., 2018, 1102–1103, 143–151 CrossRef CAS PubMed.
- H. Zhang, A. Zhang and J. Miao, et al., Targeting regulation of tryptophan metabolism for colorectal cancer therapy: a systematic review, RSC Adv., 2019, 9, 3072–3080 RSC.
- A. Zhang, H. Sun and S. Qiu, et al., NMR-based metabolomics coupled with pattern recognition methods in biomarker discovery and disease diagnosis, Magn. Reson. Chem., 2013, 51(9), 549–556 CrossRef CAS PubMed.
- C. C. Feng, A. H. Zhang and J. H. Miao, et al., Recent advances in understanding cross-talk between Bile Acids and Gut Microbiota, Open Journal of Proteomics and Genomics, 2018, 3(1), 024–034 Search PubMed.
- A. Zhang, S. Qiu and H. Xu, et al., Metabolomics in diabetes, Clin. Chim. Acta, 2014, 429, 106–110 CrossRef CAS PubMed.
- H. Sun, X. Li and A. Zhang, et al., Exploring potential biomarkers of coronary heart disease treated by Jing Zhi Guan Xin Pian using high-throughput metabolomics, RSC Adv., 2019, 9(20), 11420–11432 RSC.
- A. Zhang, H. Sun and G. Yan, et al., Serum proteomics in biomedical research: a systematic review, Appl. Biochem. Biotechnol., 2013, 170(4), 774–786 CrossRef CAS PubMed.
- X. Li, Y. Han and A. Zhang, et al., Mechanistic and Therapeutic Advances in Colon Cancer: A Systematic Review, Open Journal of Proteomics and Genomics, 2019, 4(1), 001–012 CrossRef.
- H. Sun, M. Wang and A. Zhang, et al., UPLC-Q-TOF-HDMS Analysis of Constituents in the Root of Two Kinds of Aconitum Using a Metabolomics Approach, Phytochem. Anal., 2013, 24(3), 263–276 CrossRef CAS PubMed.
- A. Zhang, H. Sun and X. Wang, Potentiating therapeutic effects by enhancing synergism based on active constituents from traditional medicine, Phytother. Res., 2014, 28(4), 526–533 CrossRef PubMed.
- G. Yan, A. Zhang and H. Sun, et al., An effective method for determining the ingredients of S huanghuanglian formula in blood samples using high-resolution LC-MS coupled with background subtraction and a multiple data processing approach, J. Sep. Sci., 2013, 36(19), 3191–3199 CAS.
- P. W. Wilson, R. B. D'Agostino and C. S. Fox, et al., Type 2 diabetes risk in persons with dysglycemia: the Framingham Offspring Study, Diabetes Res. Clin. Pract., 2011, 92(1), 124–147 CrossRef PubMed.
- J. F. Iverson, M. C. Gannon and F. Q. Nuttall, Ingestion of leucine + phenylalanine with glucose produces an additive effect on serum insulin but less than additive effect on plasma glucose, J. Amino Acids, 2013, 2013, 964637 Search PubMed.
- J. Zhou, Y. Wang and J. Chen, et al., Rational Engineering of Bacillus cereus Leucine Dehydrogenase Towards α-keto Acid Reduction for Improving Unnatural Amino Acid Production, Biotechnol. J., 2019, 14(3), e1800253 CrossRef PubMed.
- J. Li, Y. F. Cao and X. Y. Sun, et al., Plasma tyrosine and its interaction with low high-density lipoprotein cholesterol and the risk of type 2 diabetes mellitus in Chinese, J. Diabetes Invest., 2019, 10(2), 491–498 CrossRef CAS PubMed.
- A. Schaffer, M. Verdoia and L. Barbieri, et al., Impact of Diabetes on Homocysteine Levels and Its Relationship with Coronary Artery Disease: A Single-Centre Cohort Study, Ann. Nutr. Metab., 2016, 68(3), 180–188 CrossRef CAS PubMed.
- A. Zare Javid, R. Hormoznejad and H. A. Yousefimanesh, et al., The Impact of Resveratrol Supplementation on Blood Glucose, Insulin, Insulin Resistance, Triglyceride, and Periodontal Markers in Type 2 Diabetic Patients with Chronic Periodontitis, Phytother. Res., 2017, 31(1), 108–114 CrossRef CAS PubMed.
- J. Wohlrab, A. Gabel and M. Wolfram, et al., Age- and Diabetes-Related Changes in the Free Fatty Acid Composition of the Human Stratum Corneum, Skin Pharmacol. Physiol., 2018, 31(6), 283–291 CrossRef CAS PubMed.
- A. J. Pantophlet, H. Roelofsen and M. P. de Vries, et al., The use of metabolic profiling to identify insulin resistance in veal calves, PLoS One, 2017, 12(6), e0179612 CrossRef PubMed.
- M. O. Frégeau, M. Carrier and G. Guillemette, Mechanism of dopamine D2 receptor-induced Ca2+ release in PC-12 cells, Cell. Signalling, 2013, 25(12), 2871–2877 CrossRef PubMed.
- A. A. Albegali, M. Shahzad and M. I. Ullah, et al., Association of genetic polymorphism of PC-1 gene (rs1044498 Lys121Gln) with insulin-resistant type 2 diabetes mellitus in Punjabi Population of Pakistan, Mol. Genet. Genomic Med., 2019, 7(8), e775 Search PubMed.
- A. Villaseñor, D. Aedo-Martín and D. Obeso, et al., Metabolomics reveals citric acid secretion in mechanically-stimulated osteocytes is inhibited by high glucose, Sci. Rep., 2019, 9(1), 2295 CrossRef PubMed.
- A. V. Mathew, M. Jaiswal and L. Ang, et al., Impaired Amino Acid and TCA Metabolism and Cardiovascular Autonomic Neuropathy Progression in Type 1 Diabetes, Diabetes, 2019, db190145 Search PubMed.
- P. Bjornstad, L. Laffel and J. Lynch, et al., Elevated Serum Uric Acid Is Associated With Greater Risk for Hypertension and Diabetic Kidney Diseases in Obese Adolescents With Type 2 Diabetes: An Observational Analysis From the Treatment Options for Type 2 Diabetes in Adolescents and Youth (TODAY) Study, Diabetes Care, 2019, 42(6), 1120–1128 CrossRef CAS PubMed.
- Y. Shao, H. Shao and M. S. Sawhney, et al., Serum uric acid as a risk factor of all-cause mortality and cardiovascular events among type 2 diabetes population: Meta-analysis of correlational evidence, J Diabetes Complications, 2019, 107409 CrossRef PubMed.
- S. Vigili de Kreutzenberg and A. Avogaro, The role of point-of-care 3-hydroxybutyrate testing in patients with type 2 diabetes undergoing coronary angiography, J. Endocrinol. Invest., 2017, 40(6), 627–634 CrossRef CAS PubMed.
Footnotes |
† Electronic supplementary information (ESI) available. See DOI: 10.1039/c9ra09386a |
‡ These authors contributed equally. |
|
This journal is © The Royal Society of Chemistry 2019 |
Click here to see how this site uses Cookies. View our privacy policy here.