DOI:
10.1039/C7RA04032F
(Paper)
RSC Adv., 2017,
7, 29860-29870
Fast profiling of metabolite mixtures using chemometric analysis of a speeded-up 2D heteronuclear correlation NMR experiment†
Received
9th April 2017
, Accepted 1st June 2017
First published on 8th June 2017
Abstract
One-dimensional (1D) NMR spectra of mixtures of metabolites suffer from severe overlap of spectral resonances and hence recent research in NMR-based metabolomics focuses on using two-dimensional (2D) NMR experiments for metabolite fingerprinting. While standard 2D NMR experiments offer an attractive alternative to the problem of overlapping resonances, they suffer from the disadvantages of long experimental time and poor sensitivity. This work uses a fast 2D NMR experiment namely the 2D ASAP-HSQC (acceleration by sharing adjacent polarization heteronuclear single quantum correlation spectroscopy) scheme, which achieves good spectral resolution in a fraction of the experimental time taken by the standard 2D NMR pulse sequences. The experiment is easy to implement on standard NMR spectrometers and does not require specialized hardware, complicated software routines or expensive isotope labeling. The entire metabolomics study, including metabolite identification and preparing input data for multivariate statistical analysis, is performed using the 2D NMR dataset. Integrated 2D cross-peak intensities are used directly as input variables for statistical analysis. The results of the statistical analysis obtained using the 2D ASAP-HSQC spectra were validated by comparing with those obtained by using 1D proton NMR and the standard 2D HSQC NMR datasets, and a good match was obtained.
1 Introduction
NMR spectroscopy is rapidly becoming the method of choice for metabolomics-based studies, due to ease of sample preparation and rigorous spectral analysis leading to accurate metabolite identification and quantification, as discussed extensively in several topical review articles.1–4 While one-dimensional (1D) 1H NMR spectra can be easily acquired with high sensitivity in just a few minutes, the spectra of complex metabolite mixtures contain tens to hundreds of metabolites with severely overlapping peaks, which can hinder unambiguous identification and accurate quantitation of these metabolites. Further, NMR peaks from less abundant metabolites are often not quantifiable in 1D proton spectra as they are obscured by peaks from more concentrated metabolites.
Two-dimensional (2D) NMR spectroscopy has several advantages over 1D NMR and can provide detailed information about molecular structure and topology via a multitude of correlation maps between atoms; 2D NMR can also resolve the overlap problem present in 1D NMR to a certain extent, by spreading out cross-peaks (and hence connectivity information) along the indirect 13C (F1) dimension in the NMR spectrum.5 However, while 1D 1H NMR peaks are easy to quantify as signal intensity is directly proportional to metabolite molar concentrations, 2D NMR resonance cross-peak intensities are difficult to quantify as resonance-specific signal attenuation occurs during the coherence transfer periods due to spin relaxation, pulse imperfections and other experimental artifacts.6–9 The relative concentrations obtained from 2D cross-peak intensities are typically converted to absolute concentrations by referencing to an internal standard of known concentration, and the accuracy of metabolite concentration measurement can be improved by averaging intensities of several non-overlapping 2D cross peaks assigned to that particular metabolite.10 Several techniques such as matrix factorization, maximum likelihood estimation and pattern recognition have been designed to overcome problems of peak intensity quantification (and hence estimation of metabolite concentration) from 2D NMR spectra.11–16 The various multidimensional strategies in current use and the advantages and disadvantages of using 2D NMR for quantitative metabolomics are extensively discussed in several recent review articles.17–19 One versatile and widely-used method to accurately quantify 2D peak intensities is the time-zero HSQC method (HSQC0), wherein several HSQC spectra with incremented repetition times are recorded and the 2D cross-peak intensities are extrapolated back to zero time, giving signal intensities which are proportional to the concentrations of the individual metabolites.20–22 The 2D HSQC NMR experiment has thus far proved most popular for metabolomics investigations,23,24 although there have been a few studies using the 2D TOCSY,25,26 2D COSY,27,28 and 2D INADEQUATE NMR pulse sequences.29–31
Most metabolomics investigations use 2D NMR spectroscopy predominantly as an aid to peak assignment (and hence metabolite fingerprinting), however recent studies have used 2D NMR experiments as a stand-alone method for chemometrics.32–35 A major problem with 2D NMR experiments, which precludes their usage in high-throughput metabolomic studies (typically involving several dozen replicate samples), is that of long experimental times. Several techniques to circumvent this problem in NMR-based metabolomics studies include using non-uniform sampling to accelerate 2D HSQC experiments,36 as well as using “ultrafast” versions of standard 2D experiments, where data acquisition occurs in a single scan using spatial encoding and detection is based on echo planar imaging.37–39 All these fast 2D NMR techniques as tools for metabolomics studies are extensively discussed in a recent review article.40 In a another direction to reduce 2D experiment time, a novel sensitivity-enhanced 2D HSQC-type NMR experiment was recently designed called the ASAP-HSQC scheme (acceleration by sharing adjacent polarization heteronuclear single quantum correlation spectroscopy).41 The scheme records very fast 2D 13C–1H correlation spectra without compromising on spectral quality or resolution and uses much shorter relaxation delays as compared to conventional 2D HSQC experiments. While the interscan delay can be very short in an ASAP scheme, it is limited to heteronuclear experiments on samples at natural abundance.40 Further, since ASAP schemes incorporate very short relaxation delays and apply rapid high-power pulses for quite long times,42 they place a heavy stress on the rf probe and could lead to sample heating and subsequent line-broadening and resolution loss in the NMR spectrum.43,44 Such problems can be avoided by performing an EXACT ASAP-HSQC experiment, which introduces delays into the acquisition periods and later recovers the missing data points in the recorded FIDs via algorithmic reconstruction methods.45
This work evaluates the utility of the 2D ASAP-HSQC experiment in performing a fully 2D NMR-based metabolomics study, wherein the entire study from metabolite identification to preparing input data for multivariate statistical analysis is performed using 2D NMR datasets alone. Our results demonstrate the benefits of directly using 2D NMR spectral peak intensities instead of the more traditional 1D 1H NMR peaks as inputs for multivariate statistical analysis. We used tea as a model system and performed non-targeted metabolite fingerprinting. Metabolites were identified solely from the 2D NMR spectra, and the NMR analysis was combined with multivariate pattern recognition to identify metabolites that contribute significantly to the metabolic differences between green tea and black tea. We used the HSQC0 approach20 for accurate quantification of 2D cross-peak intensities in both the standard 2D HSQC and the 2D ASAP-HSQC experiments. We show that the novel 2D ASAP-HSQC experiment is able to obtain the same two-dimensional carbon–proton correlation information as the standard 2D HSQC experiment, in a fraction of the time. We compared the results of performing multivariate data analysis on 2D NMR datasets with those obtained by using 1D NMR datasets and found a good agreement between the two methods. We also performed a multivariate analysis to identify metabolites that vary in concentration in Bougainvillea plant leaves collected at two different times during the day. For this study, we had no prior information about metabolites identified from 1D 1H NMR data, and using 2D NMR data alone we were able to perform the entire analysis and identify the significant metabolites. Our work is a useful addition to the current arsenal of fast 2D NMR experiments utilized in metabolomics research.
2 Experimental
2.1 Model system & sample preparation
There has been much interest in the anti-oxidant activity and medicinal properties of green tea46 and in the metabolites that contribute significantly to the differences in green tea and black tea.47,48 We hence used green and black tea as a model system to demonstrate the utility of the 2D ASAP-HSQC experiment for fast and accurate profiling of metabolite mixtures. The chemical shifts and metabolite lists for green tea and black tea obtained from NMR data and reference databases is summarized in Table ST1 (ESI†). The green tea and black tea samples were prepared from commercially procured tea leaves. To prepare the sample for NMR experiments, 0.1 g of tea was stirred with 1200 μl of extraction solvent at 60 °C for 30 min. The tea was allowed to cool and then centrifuged at 10
000 rpm for 10 min and the supernatant was used for the NMR experiments. The extraction solvent was made by using 840 μl of methanol-d4 and 5.1 mg of Na2HPO4 (30 mM) in 340 μl of D2O (with 20 μl of TMS added as an internal NMR reference standard). A total of 20 samples (10 for green tea and 10 for black tea) were prepared as replicates for statistical analysis. Similar procedures were used to prepare the set of Bougainvillea plant samples for NMR metabolomics experiments. Briefly, fresh leaves were first dried and crushed using a pestle and mortar; 0.1 g of dried leaf powder was then stirred with 1200 μl of extraction solvent for 30 min at 60 °C to extract the metabolites. The solvent was then cooled and centrifuged at 10
000 rpm for 10 min and the supernatant was used for the NMR experiments.
2.2 1D and 2D NMR spectroscopy
All the NMR experiments were recorded at 298 K on Bruker Biospin 600 MHz Avance-III NMR spectrometers operating at a 1H NMR frequency of 600.13 MHz equipped with a QXI probe, with gradient shimming being performed prior to signal acquisition. For each sample, a set of three NMR experiments: 1D 1H, 2D 1H–13C HSQC and 2D 1H–13C ASAP-HSQC were recorded.
1D 1H NMR spectra were acquired with a 90° pulse width of 10.1 μs, a relaxation delay of 2 s, 8 scans, 16 K data points and a spectral width of 10 ppm. Water suppression of the residual water peak was achieved with a pre-saturation pulse sequence with low power irradiation during the recycle delay. Data were zero-filled by a factor of 2 and the FIDs were multiplied by an exponential weighting function equivalent to a line broadening of 1 Hz prior to Fourier transformation. The spectra were phase and baseline corrected and referenced to the TMS reference peak (at δ = 0.00 ppm).
2D 1H–13C HSQC NMR spectra were recorded via a standard Bruker phase sensitive pulse sequence, using a double-INEPT transfer for polarization enhancement and with gradients for coherence selection. Decoupling during acquisition was achieved by a GARP sequence on the carbon channel. The HSQC spectra were recorded with a spectral width of 10 ppm and 160 ppm in the proton and carbon dimensions respectively, with a total of 12 scans, 1024 data points along the proton dimension, and 128t1 increments along the indirect carbon dimension. Data were zero-filled by a factor of 2 and a QSINE window function was used prior to Fourier transformation. Using a recycle delay of 2 s, the total measurement time for each sample was around 60 min.
2D 1H–13C ASAP-HSQC NMR spectra were recorded using a new pulse sequence proposed by Schulze-Sünninghausen et al.41 Most experimental parameters, including the spectral width and data points in the proton and carbon dimensions, were kept the same as for the standard 2D HSQC experiment. A DIPSI-2 mixing interval of 40 ms was used for ASAP polarization transfer. The delay Δ′ (inversely proportional to CNST3 in the pulse program) was set to 0.7 ms to optimize the Ernst angle. This parameter is a delay in the INEPT transfer step which achieves Ernst angle-type excitation and is optimized for each sample. Non-optimal delays or wrong estimation of the JCH coupling constant could lead to a loss in sensitivity. Ernst angle-type optimization uses non-90° flip angle excitation pulses (typically 120–130°) and has been implemented in fast pulsed 2D HMQC-type experiments to help circumvent the loss in signal intensity.49 Numerically optimized shaped pulses were used for excitation, inversion and refocusing with the proton inversion and proton excitation pulses being a BURBOP-180 pulse of length 600 μs and a BEBOP-180x pulse of length 550 μs, respectively. The 13C refocusing pulse used was a BURBOP-180y pulse of duration 1.1 ms. Using a recycle delay of 200 ms and 12 scans, the total measurement time for each sample was around 8 min.
2.3 2D NMR data processing
2D cross-peak intensities were quantified using the HSQC0 approach of Hu et al.20 A series of HSQCi spectra were recorded with different number of repetitions of the basic HSQC building block. The time-zero 2D HSQC spectrum was obtained by linear regression extrapolation of all cross-peak intensities to zero repetitions (zero time) using the relation: |
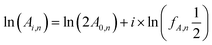 | (1) |
where fA,n is the amplitude attenuation factor in each HSQC block for the peak labeled n; Ai,n and A0,n are the peak intensities of the peak labeled n in the HSQCi spectra and the extrapolated virtual HSQC0 spectra, respectively; and i is the number of times the basic HSQC building block is repeated. The 2D ASAP-HSQC pulse sequence was modified and the ASAP cross-peaks were quantified using the same HSQC0 approach as described above. The plots of extrapolated peak intensities for various peaks in the 2D HSQC and 2D ASAP-HSQC spectra are given in the ESI (Fig. S6 and S7†).
All the 2D NMR spectra were processed in MATLAB50 by using the script provided by Hedenström et al.,32 prior to subjecting to multivariate statistical analysis. Each spectrum was converted to a row vector and normalized to a constant sum to remove differences in spectral peak intensities due to variations in the amounts of sample. Data points with intensity below a set threshold were considered to be noise. This threshold was determined by calculating the column-wise variance of a noise region in the unfolded data matrix such that the noise levels were considered for all the spectra. All the data points with intensities lower than two times the mean variance from such a noise region were considered to be noise and were subsequently excluded. To remove this noise and peaks from solvents and other additives, which otherwise do not contain any useful information and can result in biased models, data points from these additives were set to zero within the regions selected to contain only useful peaks.
To reduce the number of variables in the data matrix, bucketing and wavelet transformation of spectra is a useful approach.51 However, we followed the procedure used by Hedenström et al.32 to remove the unwanted signals by including only the selected regions of the spectra in the analysis. Such selected regions containing only the useful information were merged to one vector in the data matrix. Since the covariance and correlation patterns between the individual variables are retained, no spectral information was lost in this unfolding procedure. The resulting new data matrix, X, with reduced number of variables, was then used for multivariate analysis methods of PCA and OPLS-DA by importing in Metaboanalyst52 and generating the scores plot for both PCA and OPLS-DA. The PCA calculations were also performed in MATLAB to obtain the loadings vector. The loadings, which were present as columns in the original data matrix, were converted to 2D loading spectra by reversing the unfolding procedure in which each variable was inserted at its original position in a vector of length K and then folded to a matrix with the same dimensions as the original spectrum and visualized and analyzed in the same way as a typical 2D spectrum.
2.4 Metabolite identification
Metabolites in the 1D and 2D NMR spectra of green tea and black tea were identified based on comparison with NMR metabolite data deposited in reference databases such as the Biological Magnetic Resonance Data Bank (BMRB), www.bmrb.wise.edu, the Madison Metabolomics Consortium Database (MMCD), http://mmcd.nmrfam.wise.edu and the Human Metabolome Database (HMDB), http://www.hmdb.ca. To circumvent the problem associated with a slight shifting of chemical shifts of proton and carbon resonances in the 2D NMR spectra, a leeway of 0.5 ppm and 1 ppm was given along the proton and carbon dimensions respectively, while identifying metabolites from the corresponding 2D HSQC NMR data extracted from the reference databases.
2.5 Statistical analysis
Both 1D 1H and 2D NMR data from green tea and black tea samples were used for univariate and multivariate statistical analysis. 1D data was bucketed using the “optimized bucketing algorithm” (OBA) that optimizes bucket sizes, by setting their boundaries at the local minima determined via an average NMR spectrum, and generates variable sized buckets. The script for OBA is accessible as a MATLAB code at http://lqta.iqm.unicamp.br.53 Statistical analysis was performed using Metaboanalyst software.52 The binned data was first analyzed by the unsupervised method of principal component analysis (PCA) to identify and remove any outliers (located outside the 95% confidence region of the model) which could wrongly influence data analysis. The data was then analyzed by the supervised pattern recognition method of orthogonal projections to latent structure-discriminant analysis (OPLS-DA), which maximizes the class discrimination. The scores plot obtained for OPLS-DA analysis gives the Hotelling's T2 region, which is shown as an ellipse in the scores plot, and defines the 95% confidence interval of the modeled variation. The loadings plot gives variables which are responsible for the maximum separation between the groups. The quality of the model was evaluated by R2X and Q2 values, which give the variance explained and predicted for the model, respectively. Permutation analysis was also performed on the best model using 100 permutation tests with a p-value threshold of 0.05 to indicate that none of the results are better than the original one. Multivariate analysis was followed by univariate analysis. A t-test (p-value < 0.05) was performed to determine the significance of the metabolites identified to be responsible for group separation between green and black tea. To further cross-check that all the identified metabolites were statistically significant, a multiple hypothesis test correction using the method of Benjamini–Hochberg was also performed with a level of significance of 0.05 for the p-value.54
3 Results & discussion
3.1 Metabolite identification from NMR spectra
A detailed analysis of 1D and 2D NMR spectra of both green tea and black tea samples revealed the presence of a wide variety of metabolites in both the samples. Fig. 1(a) shows the 1H NMR spectrum of green tea while Fig. 1(b) shows the 1H NMR spectrum of black tea, both recorded at 600 MHz. A comparative preliminary analysis of both the spectra reveals the presence of similar peaks in the high-field region from 0.8–3.5 ppm (amino acids and lipids peaks) and in the mid to low-field region from 3.5–5.5 ppm (carbohydrate peaks). The peaks differ in their intensities, indicating the differences in concentrations of these metabolites in green versus black tea. The low-field region beyond 6 ppm shows the presence of a wide variety of aromatic compounds in both green tea and black tea. While both green and black tea are produced from the leaves of the same plant Camellia sinensis, green tea is prepared without significant fermentation, and hence contains several phenols and polyphenols such as flavanols and catechins.55 On the other hand, black tea undergoes a fermentation process, during which the polyphenols undergo a series of metabolic changes to produce other new polyphenols such as theaflavin and thearubigin.56 Fig. 1(a) shows the peaks identified for phenolic compounds such as flavanols and different catechins in green tea, while Fig. 1(b) shows the peaks identified for theaflavin and thearubigin present in black tea. A full list of metabolites identified both in green and black tea from the NMR spectra is given in ESI Table ST1.† The NMR chemical shift assignments of significant metabolites were further confirmed by spiking with individual metabolites and by cross-checking with reference databases and with previously published data.57,58
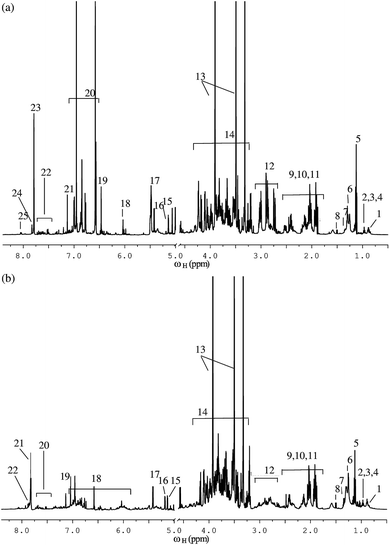 |
| Fig. 1 1D 1H NMR spectrum recorded at 600 MHz by using the water pre-saturation pulse sequence, showing specific resonances of identified metabolites of (a) green tea peaks labeled: 1, fatty acids; 2, leucine; 3, isoleucine; 4, valine; 5, theanine; 6, fatty acids; 7, lactate; 8, alanine; 9–11, fatty acids, amino acids, quinic acid; 12, EGCG, EGC, ECG and other catechins; 13, caffeine; 14, sugars; 15, theaflavin 16, glucose; 17, sucrose; 18, EGC; 19, EGCG; 20, EC, ECG, EGCG, EGC; 21, gallic acid; 22, cinnamate, chlorogenate and other phenolics; 23, caffeine; 24, theobromine; 25, flavanols; and (b) black tea peaks labeled: 1, fatty acid; 2, leucine; 3, isoleucine; 4, valine; 5, theanine; 6, fatty acids; 7, lactate; 8, alanine; 9–11, fatty acids, amino acids, quinic acid; 12, EGCG, EGC, ECG and other catechins; 13, caffeine; 14, sugars; 15; theaflavin 16, glucose; 17, sucrose; 18; thearubigins & theaflavin; 19, gallic acid; 20, cinnamate, chlorogenate and other phenolics; 21, caffeine; 22, theobromine. | |
3.2 Statistical analysis using 1D NMR
Clustering and significant metabolite differentiation between green tea and black tea was performed using PCA and OPLS-DA multivariate methods, as reported previously.54 Fig. 2 shows the results of the multivariate analysis for green tea and black tea, performed using binned data obtained from 1D 1H NMR experiments. Fig. 2(a) shows the PCA scores plot, with component 1 explaining 81.4% variation and component 2 explaining 4.9% variation. PCA analysis was performed to identify and remove outlier samples, which could otherwise incorrectly influence the data analysis.59 This was followed by OPLS-DA analysis as shown in the score plot in Fig. 2(b), obtained with one predictive and one orthogonal component. The OPLS-DA score plot shows a clear separation between green tea and black tea samples. Fig. 2(c) shows the loading plot for OPLS-DA analysis showing metabolites responsible for separation between the two types of tea samples. The metabolites marked above the baseline in the figure are present in higher quantities in green tea, while those marked below the baseline in the figure are present in higher quantities in black tea. The variables identified to be responsible for separation were also confirmed by the VIP score parameter; R2X, the variance explained by the model was 63.7% and Q2, the variance predicted by the model was 96%, while R2Y was 96.7%. The model was therefore an effective model and had a good predictive accuracy. Statistical significance of the model was tested using CV-ANOVA (p-value < 0.01) and the model was validated using a permutation test (p-value < 0.05). The multivariate analysis was followed by univariate analysis. The variables identified by multivariate analysis were subjected to a t-test for statistical significance. The metabolites identified to be responsible for differentiation between green and black tea were fatty acids, amino acids including alanine, leucine, isoleucine, valine, cysteine, aspartate, hesperidin, sugars including sucrose and glucose, phenolic compounds including caffeine, EC, ECG, EGCG, theobromine, theanine, theaflavin and thearubigins. The statistical significance of the metabolites was also confirmed by the multiple hypothesis test correction method of Benjamini–Hochberg. ESI Table ST2† lists the metabolites responsible for separation between green tea and black tea, with their p-values and Benjamini–Hochberg corrected p-values.
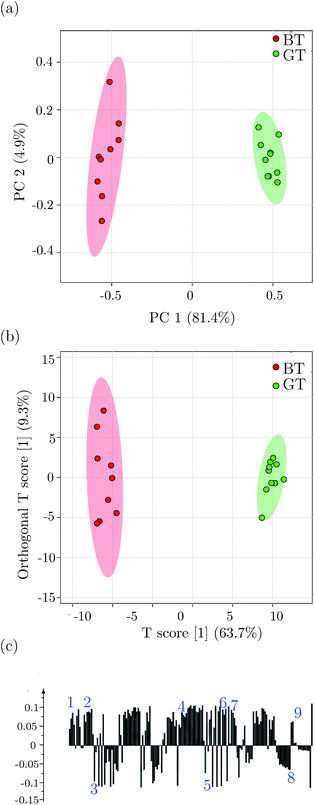 |
| Fig. 2 (a) PCA score plot and (b) OPLS-DA score plot obtained from 1D 1H NMR data of green tea and black tea. (c) Loadings plot showing the significant metabolites differing in concentration in green tea and black tea. The labels correspond to: 1. Leucine, valine 2. Theanine 3. Fatty acids, amino acids and quinic acid 4. Sugars 5. Sucrose 6. Glucose 7. ECG, EGCG and other catechins 8. Gallic acid and 9. Flavanols. | |
3.3 ASAP-HSQC versus standard HSQC
The savings in time for 2D ASAP-HSQC experiments as compared to conventional HSQC-type experiments arises from much shorter recycle times. The magnetization loss due to incomplete relaxation is compensated for by transferring magnetization from nearby 12C-attached protons (which are kept along the z direction during the pulse sequence), to the selectively excited 13C-attached protons during a short homonuclear isotropic mixing period.41 Fig. 3(a) shows different plotted regions of a standard 2D 1H–13C HSQC spectrum obtained for green tea, while Fig. 3(b) shows the corresponding 2D ASAP-HSQC spectrum, acquired with nearly the same experimental conditions as the standard HSQC experiment and a much shorter relaxation delay. The right, middle and left plots in the figure contains peaks resonating in the (proton) regions 0.8–4.3 ppm (amino acids and fatty acids region), 2.9–5.7 ppm (carbohydrate region) and 4.9–8.2 ppm (aromatics region), respectively. As is evident from Fig. 3, the ASAP-HSQC experiment is able to obtain the same carbon-proton connectivity information with nearly the same signal sensitivity and resolution, but in a fraction of the time as compared to a standard HSQC experiment. Table 1 shows a comparison of signal-to-noise ratios (SNR), for peaks spanning across the whole spectrum, obtained from both HSQC and ASAP-HSQC experiments. As can be seen in Fig. 3, the intensities of the cross-peaks differ in both ASAP-HSQC and standard HSQC spectra due to the limited availability of 1H–13C pairs for magnetization transfer in different spectral regions. The intensities of the peaks in the amino acid and fatty acid region and in the aromatic region (Fig. 3(a) and (c)) are higher in ASAP-HSQC spectra as compared to the standard HSQC (as evidenced by the corresponding higher SNR of the ASAP-HSQC peak intensities in Table 1). The carbohydrate region shows slightly less intensity for peaks in the ASAP-HSQC spectrum than in the HSQC spectrum as shown in Fig. 3(b). However, it should be noted that we are here comparing ASAP-HSQC and HSQC spectra recorded with the equivalent number of scans, resulting in ASAP-HSQC being recorded in a much shorter time than the standard HSQC experiment. Overall, the ASAP-HSQC shows a moderate increase in SNR per unit time when compared to the standard HSQC recorded for the same experimental time for peaks from all the regions of the spectra, including the carbohydrate region.60 In this work, we used an optimized mixing time of 40 ms and a recycle delay of 200 ms, so that the 2D ASAP-HSQC spectrum of one sample can be acquired in approximately 8 minutes (see Fig. S1–S5 in ESI† for details of spectra acquired with different number of scans). However, as has been discussed in the literature, experiment time can be drastically reduced to just a few hundred seconds (comparable to the time taken to perform a 1D 1H NMR experiment) by reducing the recycle delay even further.41 Furthermore, for metabolite mixtures with severe spectral overlap issues, high-resolution data can be obtained by increasing the number of t1 increments in the ASAP-HSQC scheme, by measuring at higher magnetic field strengths which increases the splitting between the spin quantum energy levels, by increasing the dimensionality of the NMR experiment, or by maximizing resolution using non-uniform data sampling strategies.61
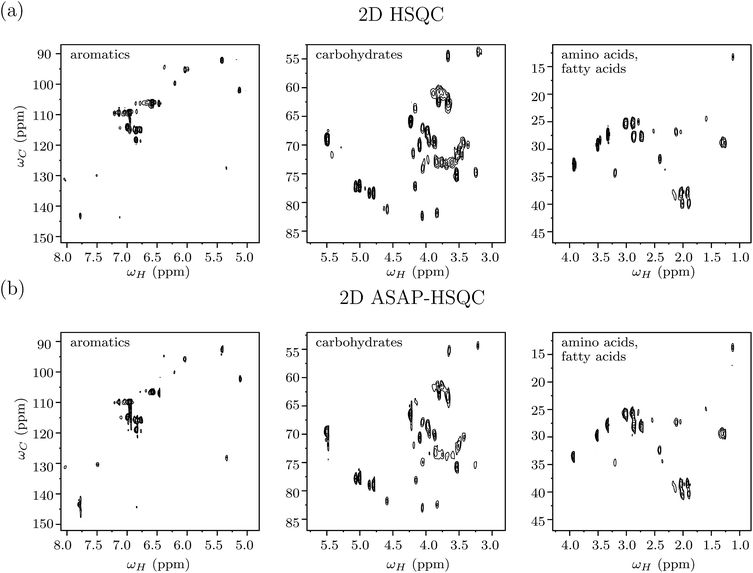 |
| Fig. 3 Regions of the (a) 2D 1H–13C HSQC NMR spectrum and (b) 2D 1H–13C ASAP-HSQC NMR spectrum of green tea recorded at 600 MHz. The right, middle and left plots contains peaks resonating in the (proton) regions 0.8–4.3 ppm (amino acids and fatty acids region), 2.9–5.7 ppm (carbohydrate region) and 4.9–8.2 ppm (aromatics region), respectively. A few peaks marked with an asterisk symbol (*) are present only in the 2D HSQC spectrum. | |
Table 1 Signal-to-noise ratios (SNR) of various peaks in 2D ASAP-HSQC and 2D HSQC NMR spectra; R denotes the ratio of ASAP-HSQC to HSQC peak intensities
Peak position (1H, 13C) ppm |
ASAP-HSQC |
HSQC |
R |
(1.11, 13.63) |
14.39 |
13.81 |
1.04 |
(1.28, 29.33) |
31.46 |
26.7 |
1.18 |
(1.59, 24.86) |
39.81 |
34.18 |
1.16 |
(1.89, 40.31) |
25.49 |
17.19 |
1.48 |
(2.15, 27.17) |
20.52 |
19.12 |
1.07 |
(2.41, 32.44) |
32.4 |
20.7 |
1.56 |
(3.31, 27.68) |
61.05 |
49.25 |
1.24 |
(3.9, 33.33) |
91.01 |
118.03 |
0.77 |
(3.19, 54.13) |
9.02 |
14.74 |
0.61 |
(5.49, 69.4) |
75.66 |
109.8 |
0.69 |
(4.99, 77.61) |
61.11 |
81.19 |
0.75 |
(5.41, 92.56) |
14.83 |
24.27 |
0.61 |
(5.12, 102.1) |
11.74 |
16.43 |
0.71 |
(6.03, 95.6) |
12.43 |
18.52 |
0.67 |
(6.57, 106.6) |
141.4 |
167.7 |
0.84 |
(6.77, 115.7) |
30.05 |
29.24 |
1.03 |
(6.99, 114.7) |
38.43 |
40.39 |
0.95 |
(7.15, 109.6) |
175.3 |
207.7 |
0.85 |
(7.48, 130.3) |
5.49 |
4.89 |
1.12 |
(7.77, 143.4) |
22.22 |
18.99 |
1.17 |
3.4 Statistical analysis using 2D NMR datasets
In order to demonstrate the utility of 2D NMR datasets as a viable alternative to 1D NMR datasets for multivariate analysis, we repeated the multivariate statistical analysis for green tea and black tea, on the 2D NMR data sets. The 2D data set was prepared for OPLS-DA analysis, following the protocol outlined in Hedenström et al.32 The 2D spectral region was first unfolded into a row vector and the data were UV scaled (unit variance), giving the peaks the same variance irrespective of their intensities, which helped to detect metabolites with low abundance and directly related the peak intensities in the loadings to their significance for sample discrimination. The resultant data matrix contains the spectral data of each sample as a row vector, which is given as input data for multivariate analysis. The peaks with maximum correlation in discriminating samples and therefore being significant for separating the green and black tea samples were identified by choosing a cut-off value of 0.5. Fig. 4(a) shows the OPLS-DA score plot (top panel) with both orthogonal and predictive components and the corresponding loadings plot obtained from the analysis of the 2D HSQC NMR spectra of green tea and black tea. The variance explained and variance predicted by the model, R2X and Q2 were 79.4% and 96.6% respectively (R2Y = 97.2%). This model was hence an effective model with good predictability accuracy. The model was tested for statistical significance using CV-ANOVA (p-value < 0.01) and validated using a permutation test (p-value < 0.05) as before. The loading vectors obtained from the statistical model were transferred to the MATLAB script and the loading values were arranged in their original positions in a vector of length corresponding to the original unfolded spectrum. This vector was then folded into a matrix of the same dimensions of the original 2D spectra, in order to visualize the loading vectors. The variables obtained from the loadings plot were subjected to t-test and multiple hypothesis test correction method of Benjamini–Hochberg for their statistical significance. We next repeated the entire multivariate analysis described above, using the 2D ASAP-HSQC peak intensities. Fig. 4(b) shows the OPLS-DA score plot (top panel) with one orthogonal and one predictive component and the corresponding 2D loadings plot (bottom panel) obtained for the OPLS-DA analysis. The variance explained and variance predicted by the model R2X and Q2, were 81.7% and 97.4% respectively (R2Y = 97.7%) proving that the model had good predictive accuracy. The metabolites identified were then subjected to the univariate analysis of t-test and Benjamini–Hochberg as before. The variables (i.e. significant metabolites) identified using the 2D ASAP-HSQC data were the same as those identified from the standard HSQC and from the 1D NMR datasets. Significant metabolites have been marked on the loadings plots obtained from both the HSQC and ASAP-HSQC data analysis in Fig. 4, to show that the list of significant metabolites obtained from both the spectra match well and hence the standard HSQC and the ASAP-HSQC data led to comparable results.
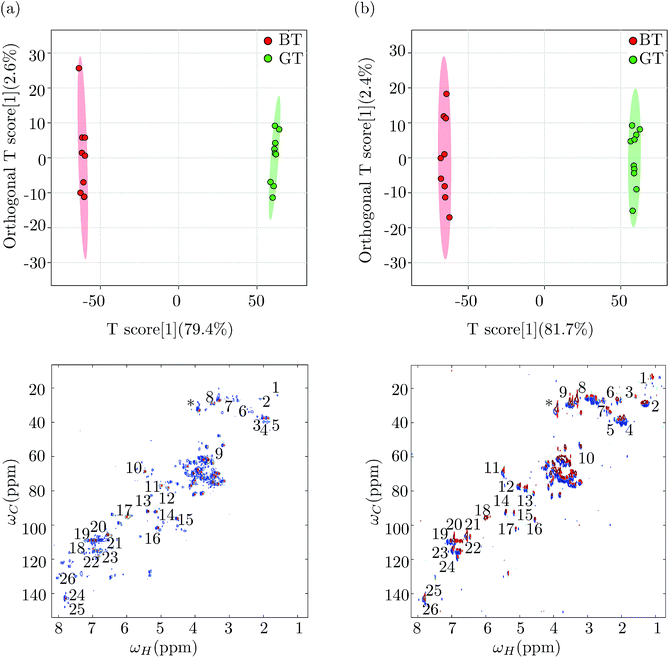 |
| Fig. 4 (a) OPLS-DA score plot (upper panel) and corresponding loadings plot (lower panel) obtained from the multivariate analysis of 2D HSQC NMR spectra of green and black tea (R2X = 0.794, R2Y = 0.972 and Q2 = 0.966). Significant peaks are marked on loadings plot as 1, alanine; 2, valine; 3, lipid; 4, isoleucine; 5, leucine; 6, theanine; 7, aspartate; 8, cysteine; 9, choline; 10, hesperidin; 11, GC; 12, EC; 13, sucrose; 14 and 15, glucose; 16, catechins; 17, naringin; 18–23, EC, EGC, EGCG, theaflavin, thearubigin; 24, theobromine; 25, caffeine; 26, phenols, and (b) OPLS-DA score plot (upper panel) and corresponding loadings plot (lower panel) obtained from the multivariate analysis of 2D ASAP-HSQC NMR spectra of green and black tea (R2X = 0.817, R2Y = 0.977 and Q2 = 0.974). Significant peaks are marked on loadings plot as 1, lipids; 2, lipids; 3, alanine; 4, leucine; 5, isoleucine; 6, valine; 7, theanine; 8, aspartate; 9, cysteine; 10, choline; 11, hesperidin; 12, GC; 13, EC; 14, sucrose; 15 and 16, glucose; 17, catechins; 18, naringin; 19–24, EC, EGC, EGCG, theaflavin, thearubigin; 25, theobromine; 26, caffeine. Differences in peak color in both the loadings plots depict the differences in metabolite concentrations, with the red color signifying metabolites present in higher quantities in green tea, while the blue color signifies those present in higher quantities in black tea. The peak marked with an asterisk (*) labels caffeine at (3.95, 33.74) ppm, which is taken as the reference peak. | |
Table 2 shows the list of significant metabolites responsible for the differences between green and black tea, using all the three NMR datasets (from the 1D and the 2D experiments). Since the NMR reference standard TMS does not give a peak in the 2D NMR spectrum, we used the peak for caffeine at (3.95, 33.74) ppm as our reference peak and calculated the relative integrals of significant metabolites in both green tea and black tea using the integral for caffeine as 1 in the green tea spectrum. Caffeine is present as a clearly identifiable and non-overlapping peak in both green and black tea NMR spectra, and was hence chosen as our reference. The metabolite concentrations obtained from all three datasets were similar, corroborating our assertion that the 2D ASAP-HSQC NMR data set is a good candidate for metabolite fingerprinting as well as multivariate statistical analysis as it combines the twin advantages of faster experimental times of 1D NMR experiments and better resolution of standard 2D NMR experiments. Furthermore, from the multivariate analysis of 2D NMR data, we were able to identify three more metabolites, namely choline, naringin and hesperidin, that contribute significantly to the metabolic differences between green tea and black tea. Their relative amounts present in green tea and black tea are shown in Table 3. We note here that these metabolites do not show up as significant from the statistical analysis of 1D 1H NMR data, demonstrating that using 2D NMR data for metabolomics studies can indeed lead to better metabolite fingerprinting.
Table 2 Relative amounts of significant metabolites present in green tea (GT) and black tea (BT) obtained using 1D, 2D HSQC and 2D ASAP-HSQC NMR data. Data are represented as mean ± SD, where the integral of the caffeine peak was taken as the reference and set to value 1.0. Statistical significance was confirmed by a t-test (p value < 0.05)
Metabolite |
1D data |
2D HSQC |
2D ASAP-HSQC |
GT |
BT |
GT |
BT |
GT |
BT |
Lipids |
0.76 ± 0.02 |
1.02 ± 0.05 |
0.70 ± 0.03 |
0.97 ± 0.04 |
0.74 ± 0.05 |
0.96 ± 0.03 |
Alanine |
0.13 ± 0.04 |
0.18 ± 0.05 |
0.17 ± 0.05 |
0.22 ± 0.04 |
0.15 ± 0.04 |
0.23 ± 0.04 |
Leucine |
0.42 ± 0.05 |
0.63 ± 0.03 |
0.38 ± 0.02 |
0.58 ± 0.04 |
0.44 ± 0.06 |
0.64 ± 0.07 |
Isoleucine |
0.36 ± 0.01 |
0.72 ± 0.05 |
0.40 ± 0.04 |
0.67 ± 0.05 |
0.37 ± 0.06 |
0.71 ± 0.04 |
Valine |
0.44 ± 0.04 |
0.65 ± 0.04 |
0.39 ± 0.03 |
0.64 ± 0.05 |
0.41 ± 0.04 |
0.67 ± 0.03 |
Cysteine |
0.29 ± 0.07 |
0.63 ± 0.04 |
0.26 ± 0.03 |
0.59 ± 0.04 |
0.30 ± 0.05 |
0.56 ± 0.05 |
Aspartate |
0.63 ± 0.03 |
0.75 ± 0.03 |
0.59 ± 0.06 |
0.70 ± 0.05 |
0.65 ± 0.04 |
0.72 ± 0.08 |
Sucrose |
0.77 ± 0.06 |
0.39 ± 0.07 |
0.71 ± 0.08 |
0.38 ± 0.06 |
0.70 ± 0.08 |
0.35 ± 0.04 |
Glucose |
0.64 ± 0.03 |
0.39 ± 0.03 |
0.58 ± 0.05 |
0.36 ± 0.03 |
0.57 ± 0.06 |
0.32 ± 0.02 |
Caffeine |
1.00 |
0.86 ± 0.03 |
1.00 |
0.89 ± 0.04 |
1.00 |
0.88 ± 0.02 |
EC |
1.42 ± 0.09 |
0.68 ± 0.06 |
1.50 ± 0.10 |
0.65 ± 0.05 |
1.49 ± 0.05 |
0.62 ± 0.07 |
EGCG |
1.44 ± 0.04 |
0.37 ± 0.05 |
1.43 ± 0.05 |
0.44 ± 0.06 |
1.38 ± 0.06 |
0.42 ± 0.08 |
Theobromine |
0.42 ± 0.09 |
0.15 ± 0.03 |
0.45 ± 0.04 |
0.18 ± 0.03 |
0.44 ± 0.03 |
0.19 ± 0.04 |
Theanine |
0.29 ± 0.08 |
0.12 ± 0.02 |
0.23 ± 0.04 |
0.10 ± 0.03 |
0.27 ± 0.05 |
0.14 ± 0.04 |
Theaflavin |
0.21 ± 0.04 |
0.96 ± 0.08 |
0.16 ± 0.06 |
1.02 ± 0.08 |
0.18 ± 0.04 |
0.99 ± 0.06 |
Table 3 Relative amounts of significant metabolites present in green tea (GT) and black tea (BT) obtained from multivariate analysis of 2D HSQC and 2D ASAP-HSQC NMR data
Metabolite |
2D HSQC |
2D ASAP-HSQC |
GT |
BT |
GT |
BT |
Choline |
0.22 ± 0.03 |
0.29 ± 0.06 |
0.16 ± 0.05 |
0.28 ± 0.04 |
Naringin |
0.44 ± 0.05 |
0.38 ± 0.06 |
0.47 ± 0.06 |
0.40 ± 0.05 |
Hesperidin |
0.73 ± 0.04 |
0.64 ± 0.05 |
0.70 ± 0.05 |
0.66 ± 0.04 |
To validate the 2D HSQC experiment as a viable alternative to 1D 1H NMR experiments for NMR-based metabolomics studies, we next performed NMR metabolomic analysis on a different sample, of Bougainvillea plant leaves with a crowded 1D 1H NMR spectrum and several overlapping resonances. The motivation was to use 2D HSQC NMR data alone for the multivariate statistical analysis, without taking recourse to 1D 1H NMR data. Fig. 5(a) shows the 1D 1H NMR spectrum for the Bougainvillea plant, recorded at 400 MHz. Fig. 5(b) shows the corresponding 2D 1H–13C HSQC NMR spectrum. We performed multivariate statistical analysis of the plant leaves collected at two time points (12 hours apart) during the day, with an aim of identifying the “cycling” metabolites associated with the circadian rhythm of the plant i.e. those that undergo changes in their concentration during different times of the day. Five replicates were selected for each time point for a total of ten samples and PCA analysis done using 2D HSQC data to identify the outliers. This was followed by OPLS-DA analysis to identify metabolites that significantly differ at the two time points. Fig. 5(c) depicts the OLPS-DA scores plot with one orthogonal component and one predictive component. The variance explained and predicted by the model, R2X and Q2 were 48.9% and 87.8% respectively (with R2Y = 94.4%), confirming that the model was an effective one with good predictable accuracy. Statistical significance of the model was tested using CV-ANOVA (p-value < 0.01) and the model was validated using a permutation test (p-value < 0.05). The loading vectors obtained from the statistical model were transferred to the MATLAB script and the loading values were arranged in their original positions in a vector of length corresponding to the original unfolded spectrum as explained previously. Similarly, the loading vectors were visualized by folding the vector into a matrix with the same dimensions as the original 2D spectra. Fig. 5(d) shows the resultant 2D loadings spectrum obtained for the OPLS-DA analysis via the MATLAB script. As can be seen from the loadings plot, the metabolites undergoing changes in their concentrations during the day are lipids, the amino acids alanine, valine and phenylalanine, glucose, and phenolic compounds hesperidin, choline and trigonelline. Table 4 shows the list of significant metabolites identified to be responsible for the differences between the samples at two time points, along with their relative peak integrals. The metabolite peak integrals were calculated relative to the integral for the peak of fatty acid at 1.29 ppm, which was set to the value 1.0. Of these metabolites, lipids, amino acids alanine and valine, glucose are known to undergo cyclic changes in their concentrations during the day in fruitfly Drosophila melanogaster.54 Trigonelline, a compound from nicotinamide, is known to play many significant roles in plant metabolism including its role in cell cycle regulation, nodulation and oxidative and UV-stresses.62 Choline is known to be produced in plants during conditions of stress.63 The changes in the levels of trigonelline and choline in the plant during the day shows the changes in their levels in response to changes in the environment faced by plants during different times of the day.
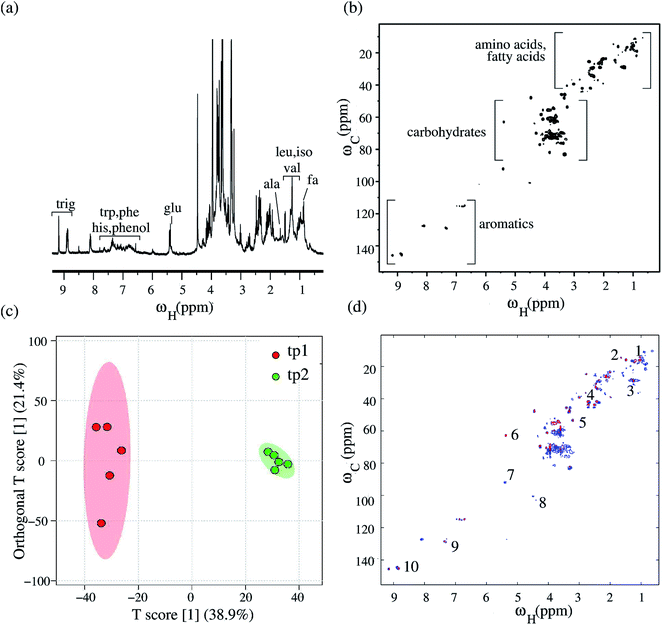 |
| Fig. 5 (a) 1D 1H NMR spectrum of and (b) 2D 1H–13C HSQC NMR spectrum of Bougainvillea leaves, recorded at 400 MHz. (c) OPLS-DA score plot obtained from multivariate analysis of 2D HSQC NMR spectra, showing a clear separation between Bougainvillea leaf samples collected at two different times during the day (R2X = 0.489, R2Y = 0.944 and Q2 = 0.878). (d) 2D loadings plot showing significant metabolites responsible for differentiation between leaf samples collected at two different time points. Peaks numbering: 1, lipid; 2, alanine; 3, fatty acid; 4, valine; 5, choline; 6, hesperidin; 7, 8 glucose; 9, phenylalanine; 10, trigonelline. | |
Table 4 Relative amounts of significant metabolites in Bougainvillea leaves collected at two different times in a day, obtained from multivariate analysis of 2D HSQC NMR data
Metabolite |
Time point 1 |
Time point 2 |
Lipid |
0.67 ± 0.03 |
0.45 ± 0.02 |
Alanine |
0.61 ± 0.04 |
0.49 ± 0.02 |
Fatty acid |
1 |
1.16 ± 0.05 |
Valine |
0.27 ± 0.03 |
0.20 ± 0.04 |
Choline |
0.92 ± 0.02 |
0.77 ± 0.05 |
Hesperidin |
0.44 ± 0.01 |
0.55 ± 0.04 |
Glucose |
0.42 ± 0.06 |
0.35 ± 0.05 |
Phenyl alanine |
0.77 ± 0.04 |
0.85 ± 0.05 |
Trigonelline |
0.56 ± 0.03 |
0.40 ± 0.01 |
4 Conclusions
Multivariate statistical analysis has been used to reduce the high dimensionality of the experimental NMR spectral data and hence to obtain discriminatory features of the biological classes being studied. Although 2D NMR experiments offer a feasible solution to the problem of spectral resolution in 1D 1H spectra, they have not yet been fully exploited for chemometric investigations. Nevertheless, the number of 2D NMR metabolite spectra available in standard online databases is rapidly increasing, though care must be taken during peak quantification to account for chemical shift variations between standard deposited spectra and actual experimental spectra (due to pH, temperature and solvent experimental factors). We demonstrate the utility of a speeded-up version of the standard 2D HSQC experiment, namely the 2D ASAP-HSQC scheme, as a viable alternative to 1D 1H NMR experiments, in performing a full NMR-based metabolomics investigation. Our work is a step forward in the direction of integrating usage of 2D HSQC NMR experimental data into a routine protocol for non-targeted, high-throughput metabolomics studies.
Acknowledgements
The NMR experiments were performed on Bruker Avance-III 600 MHz and 400 MHz FT-NMR spectrometers at the NMR Research Facility at IISER Mohali. HS acknowledges financial support from CSIR India. We thank Dr Mattias Hedenström for providing the MATLAB scripts for 2D NMR data processing and Johanna Becker for providing the Bruker code for the 2D ASAP-HSQC pulse sequence.
References
- J. S. McKenzie, J. A. Donarski, J. C. Wilson and A. J. Charlton, Prog. Nucl. Magn. Reson. Spectrosc., 2011, 59, 336–359 CrossRef CAS PubMed.
- K. Bingol and R. Bruschweiler, Anal. Chem., 2013, 86, 47 CrossRef PubMed.
- S. L. Robinette, R. Bruschweiler, F. C. Schroeder and A. S. Edison, Acc. Chem. Res., 2012, 45, 288 CrossRef CAS PubMed.
- C. K. L. G. A. Barding and M. M. Dinges, Anal. Chem., 2015, 87, 133 CrossRef PubMed.
- J. S. McKenzie, A. J. Charlton, J. A. Donarski, A. D. MacNicoll and J. C. Wilson, Metabolomics, 2010, 6, 574 CrossRef CAS.
- K. Bingol, D. Li, L. B. Li, O. A. Cabrera, T. Megraw, F. Zhang and R. Bruschweiler, ACS Chem. Biol., 2015, 10, 452 CrossRef CAS PubMed.
- V. Makela, J. Helminen, I. Kilpelainen and S. Heikkinen, J. Magn. Reson., 2016, 271, 34–39 CrossRef CAS PubMed.
- F. Fardus-Reid, J. Warren and A. L. Gresley, Anal. Chem., 2016, 8, 2013–2019 CAS.
- C. Mauve, S. Khlifi, F. Gilard, G. Mouille and J. Farjon, Chem. Commun., 2016, 52, 6142 RSC.
- J. Xia, T. C. Bjorndahl, P. Tang and D. S. Wishart, Bioinformatics, 2008, 9, 507 Search PubMed.
- D. A. Snyder, F. Zhang, S. L. Robinette, L. Bruschweiler-Li and R. Bruschweiler, J. Chem. Phys., 2008, 128, 052313 CrossRef PubMed.
- R. K. Rai, P. Tripathi and N. Sinha, Anal. Chem., 2009, 81, 10232–10238 CrossRef CAS PubMed.
- R. A. Chylla, K. Hu, J. J. Ellinger and J. L. Markley, Anal. Chem., 2011, 83, 4871 CrossRef CAS PubMed.
- S. L. Robinette, R. Ajredini, H. Rasheed, A. Zeinomar, F. C. S. A. T. Dossey and A. S. Edison, Anal. Chem., 2011, 83, 1649 CrossRef CAS PubMed.
- E. Holmes and H. Antti, Analyst, 2002, 127, 1549 RSC.
- A. Smolinska, L. Blanchet, L. M. Buydens and S. S. Wijmenga, Anal. Chim. Acta, 2012, 750, 82 CrossRef CAS PubMed.
- P. Giraudeau, Magn. Reson. Chem., 2014, 52, 259–272 CrossRef CAS PubMed.
- P. Giraudeau, Magn. Reson. Chem., 2017, 55, 61–69 CrossRef CAS PubMed.
- J. Marchand, E. Martineau, Y. Guitton, G. D. Pinel and P. Giraudeau, Curr. Opin. Biotechnol., 2017, 43, 49–55 CrossRef CAS PubMed.
- K. Hu, W. M. Westler and J. L. Markley, J. Am. Chem. Soc., 2011, 133, 1662 CrossRef CAS PubMed.
- K. Hu, J. J. Ellinger, R. A. Chylla and J. L. Markley, Anal. Chem., 2011, 83, 9352 CrossRef CAS PubMed.
- K. Hu, T. P. Wyche, T. S. Bugni and J. L. Markley, J. Nat. Prod., 2011, 74, 2295–2298 CrossRef CAS PubMed.
- I. A. Lewis, S. C. Schommer, B. Hodis, K. A. Robb, M. Tonelli, W. M. Westler, M. R. Sussman and J. L. Markley, Anal. Chem., 2007, 79, 9385–9390 CrossRef CAS PubMed.
- T. Oman, M. Tessem, T. F. Bathen, H. Bertilsson, A. Angelsen, M. Hedenström and T. Andreassen, Bioinformatics, 2014, 15, 413 Search PubMed.
- K. Bingol, F. Zhang, L. Bruschweiler-Li and R. Bruschweiler, Anal. Chem., 2013, 85, 6414 CrossRef CAS PubMed.
- K. Bingol, L. Bruschweiler-Li, D. W. Li and R. Bruschweiler, Anal. Chem., 2014, 86, 5494 CrossRef CAS PubMed.
- F. C. Schroeder, D. M. Gibson, A. C. L. Churchill, P. Sojikul, E. J. Wursthorn, S. B. Krasnoff and J. Clardy, Angew. Chem., Int. Ed., 2007, 46, 901 CrossRef CAS PubMed.
- B. Feraud, B. Govaerts, M. Verleysen and P. D. Tullio, Metabolomics, 2015, 11, 1756 CrossRef CAS.
- E. Martineau, P. Giraudeau, I. Tea and S. Akoka, J. Pharm. Biomed. Anal., 2011, 54, 252 CrossRef CAS PubMed.
- E. Martineau, I. Tea, S. Akoka and P. Giraudeau, NMR Biomed., 2012, 25, 985 CrossRef CAS PubMed.
- C. S. Clendinen, C. Pasquel, R. Ajredini and A. S. Edison, Anal. Chem., 2015, 87, 5698 CrossRef CAS PubMed.
- M. Hedenström, S. W. Lindstrom, T. Omana, F. Lu, L. Gerber, P. Schatz, B. Sundberg and J. Ralph, Mol. Plant., 2009, 2, 933 CrossRef PubMed.
- M. A. Farag, E. A. Mahrous, T. Lubken, A. Porzel and L. Wessjohann, Metabolomics, 2014, 10, 21 CrossRef CAS.
- E. A. Mahrous and M. A. Farag, J. Adv. Res., 2015, 6, 3 CrossRef CAS PubMed.
- Y. Izrayelit, S. L. Robinette, N. Bose, S. H. von Reuss and F. C. Schroeder, ACS Chem. Biol., 2013, 8, 314 CrossRef CAS PubMed.
- R. K. Rai and N. Sinha, Anal. Chem., 2012, 84, 10005–10011 CrossRef CAS PubMed.
- A. L. Guennec, I. Tea, I. Antheaume, E. Martineau, B. Charrier, M. Pathan, S. Akoka and P. Giraudeau, Anal. Chem., 2012, 84, 10831 CrossRef PubMed.
- P. Giraudeau, S. Massou, Y. Robin, E. Cahoreau, J. Portais and S. Akoka, Anal. Chem., 2011, 83, 3112 CrossRef CAS PubMed.
- T. Jezequel, C. Deborde, M. Maucourt, V. Zhendre, A. Moing and P. Giraudeau, Metabolomics, 2015, 128, 0780 Search PubMed.
- A. L. Guennec, P. Giraudeau and S. Caldarelli, Anal. Chem., 2014, 86, 5914 CrossRef PubMed.
- D. Schulze-Sünninghausen, J. Becker and B. Luy, J. Am. Chem. Soc., 2014, 136, 1242 CrossRef PubMed.
- E. Kupce and R. Freeman, Magn. Reson. Chem., 2007, 45, 2–4 CrossRef CAS PubMed.
- R. C. Breton and W. F. Reynolds, Nat. Prod. Rep., 2013, 30, 501 RSC.
- J. Becker and B. Luy, Magn. Reson. Chem., 2015, 53, 878–885 CrossRef CAS PubMed.
- I. E. Ndukwe, A. Shchukina, K. Kazimierczuk and C. P. Butts, Chem. Commun., 2016, 52, 12769 RSC.
- G. L. Gall, I. J. Colquhoun and M. Defernez, J. Agric. Food Chem., 2004, 52, 692 CrossRef PubMed.
- C. A. Daykin, J. P. M. Vanduynhoven, A. Groenewegen, J. M. M. V. A. M. Dachtler and T. P. J. Mulder, J. Agric. Food Chem., 2005, 53, 1428 CrossRef CAS PubMed.
- S. Balayssac, M. A. Delsuc, V. Gilard, Y. Prigent and M. M. Martino, J. Magn. Reson., 2009, 196, 78 CrossRef CAS PubMed.
- A. Ross, M. Salzmann and H. Senn, J. Biomol. NMR, 1997, 10, 389–396 CrossRef CAS PubMed.
- MATLAB, Version 7.10 (R2010a), MathWorks Inc., Natick, Massachusetts USA, 2010 Search PubMed.
- N. Trbovic, F. Dancea, T. Danger and U. Gunther, J. Magn. Reson., 2015, 173, 280–287 CrossRef PubMed.
- J. Xia, N. Psychogios, N. Young and D. S. Wishart, Nucleic Acids Res., 2009, 37, 652 CrossRef PubMed.
- S. Sousa, A. Magalhaes and M. M. C. Ferreira, Chemom. Intell. Lab. Syst., 2013, 122, 93–102 CrossRef CAS.
- N. Gogna, V. J. Singh, V. Sheeba and K. Dorai, Mol. BioSyst., 2015, 11, 3305 RSC.
- L. K. Leung, Y. Su, R. Chen, Z. Zhang, Y. Huang and Z. Chen, J. Nutr., 2001, 131, 2248 CAS.
- E. A. H. Roberts and R. F. Smith, Analyst, 1961, 86, 94–98 RSC.
- G. L. Gall, I. Colquhoun and M. Defernez, J. Agric. Food Chem., 2004, 52, 692 CrossRef PubMed.
- A. Ohno, K. Oka, C. Sakuma, H. Okudaand and K. Fukuhara, J. Agric. Food Chem., 2011, 59, 5181 CrossRef CAS PubMed.
- N. Gogna, N. Hamid and K. Dorai, J. Pharm. Biomed. Anal., 2015, 115, 74 CrossRef CAS PubMed.
- L. Castanar and T. Parella, Annual Reports on NMR Spectroscopy, Academic Press, Orlando, Florida, USA, 2015, vol. 84, pp. 163–232 Search PubMed.
- D. P. Frueh, Prog. Nucl. Magn. Reson. Spectrosc., 2014, 78, 47–75 CrossRef CAS PubMed.
- P. V. Minorsky, Plant Physiol., 2002, 128, 7–8 CrossRef CAS.
- K. Shirasawa, T. Takabe, T. Takabe and S. Kishitani, Ann. Bot., 2006, 93, 565–571 CrossRef PubMed.
Footnote |
† Electronic supplementary information (ESI) available: Table ST1 shows a full list of metabolites identified from the NMR spectra of green tea and black tea. Table ST2 displays the results of post hoc analysis of significant metabolites responsible for the separation between green and black tea. Fig. S1–S5 display 2D ASAP-HSQC NMR spectra of green tea, for different values of the number of scans. Fig. S6 and S7 show the extrapolation of peak heights measured from 2D HSQC and 2D ASAP-HSQC spectra used for 2D peak quantification. See DOI: 10.1039/c7ra04032f |
|
This journal is © The Royal Society of Chemistry 2017 |
Click here to see how this site uses Cookies. View our privacy policy here.