DOI:
10.1039/D0RA07969C
(Paper)
RSC Adv., 2020,
10, 43213-43224
Adsorption of dicamba and MCPA onto MIL-53(Al) metal–organic framework: response surface methodology and artificial neural network model studies†
Received
17th September 2020
, Accepted 2nd November 2020
First published on 27th November 2020
Abstract
An aluminium-based metal–organic framework ((MOF), MIL-53(Al)), was hydrothermally synthesized, characterized and applied for the remediation of the herbicides dicamba (3,6-dichloro-2-methoxy benzoic acid) and 4-chloro-2-methylphenoxyacetic acid (MCPA) in aqueous medium. Response surface methodology (RSM) and artificial neural network (ANN) were used to design, optimize and predict the non-linear relationships between the independent and dependent variables. The shared interaction of the effects of key response parameters on the adsorption capacity were assessed using the central composite design-RSM and ANN optimization models. The optimum adsorption capacities for dicamba and MCPA are 228.5 and 231.9 mg g−1, respectively. The RSM ANOVA results showed significant p-values, with coefficients of determination (R2) = 0.988 and 0.987 and R2 adjusted = 0.974 and 0.976 for dicamba and MCPA, respectively. The ANN prediction model gave R2 = 0.999 and 0.999, R2 adjusted = 0.997 and 0.995 and root mean square errors (RMSEs) of 0.001 and 0.004 for dicamba and MCPA, respectively. In each set of experimental conditions used for the study, the ANN gave better prediction than the RSM, with high accuracy and minimal error. The rapid removal (∼25 min), reusability (5 times) and good agreement between the experimental findings and simulation results suggest the great potential of MIL-53(Al) for the remediation of dicamba and MCPA from water matrices.
Introduction
Dicamba (3,6-dichloro-2-methoxy benzoic acid) and MCPA (4-chloro-2-methylphenoxyacetic) (Table 1) are widely used as post-emergence herbicides to selectively kill broadleaf weeds that infest farmlands.1 They act by altering the metabolism, physiology and cell wall plasticity of the weeds.2,3 The excess use of dicamba and MCPA deteriorates the quality of water resources due to their toxicity, slow biodegradability and persistence in aqueous media.4 This has led to uncontrollable non-point source effluent in the form of agricultural run-off and leaching, which pollutes surface and ground waters.5 Studies have indicated that dicamba and MCPA are easily biomagnified and accumulate in living organisms through the food chain, causing negative impacts such as endocrine disruption of aquatic animals and humans.6 Dicamba and MCPA have been listed as priority pollutants by the European Union (EU) and are considered to have possible carcinogenic and mutagenic effects by the International Agency for Research on Cancer.7 Due to adverse environmental and health impact of these compounds, European Union (EU) directives have set 0.1 μg L−1 as the maximum concentration allowed in drinking water.8 Hence, it is crucial to apply appropriate treatment techniques that can efficiently and effectively remove these contaminants from water.9,10
Table 1 Some properties of the studied herbicides
Common name |
Chemical name |
Molecular formula |
Structure |
Solubility (mg L−1) |
pKa |
log P |
Dicamba |
3,6-Dichloro-2-methoxybenzoic |
C8H6Cl2O3 |
 |
6100 |
1.87 |
2.21 |
MCPA |
4-Chloro-2-methylphenoxyacetic acid |
C9H9ClO3 |
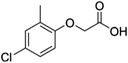 |
825 |
3.13 |
2.80 |
Over the years, methods such as adsorption, coagulation, flocculation, ion exchange, membrane filtration, and chemical oxidation11 have been applied to remove recalcitrant herbicides from water. Of these methods, adsorption has been singled out to be the most practical due to its cost-effectiveness, simple operations, convenient recycling and availability.12,13 The most frequently used adsorbent for the removal of dicamba and MCPA is activated carbon;14 however, other adsorbents, such as organic materials,4 graphene oxide,15 goethite,16 biochar,17,18 and clay materials,19 have recently been proposed. It has been recognised that potential adsorbents must have high surface areas, large pore volumes, and good thermal and water stabilities.20
Metal–organic frameworks (MOFs) typify the substantial progress that has been made in the development of the new generation of porous materials over the years.21 MOFs have quickly attracted the attention of researchers for the removal of different environmental pollutants.22,23 They are a class of highly porous, crystalline materials with high surface areas, consisting of metal clusters and multifunctional organic linkers.24 Recently, the application of MOFs in the remediation of toxic contaminants in wastewater such as pharmaceuticals, dyes, and heavy metals has been gaining attention.25
Aluminium-based MOFs such as MIL-53(Al), MIL-68(Al), MIL-100(Al), and MIL-101(Al) (Materials Institute Lavoisier) have been used for various applications, such as CO2 capture, gas storage, separation, sensing, catalysis and wastewater remediation, due to their potential and versatile properties.26,27 One unique feature of MIL-53(Al) is that it has high thermal stability and structural flexibility, which is also referred to as the “breathing effect”,28 due to its ability to expand and contract by adjusting the pore size to accommodate different molecule sizes and still retain its crystallinity. MIL-53(Al), with a surface area of 500 m2 g−1, was recently applied by Gao et al.29 for the removal of sulfonamide antibiotics from water. Equilibrium adsorption was reached within 60 min. Guan et al.30 observed rapid removal and high adsorption capacity of methyl blue (93.1 mg g−1) and amaranth dyes (40.2 mg g−1) using MIL-53(Al). Li et al.31 recorded a high adsorption capacity (228.2 mg g−1) of Cu(II) onto MIL-53(Al). Jia et al.32 also applied MIL-53(Al) for the adsorption of triclosan with high removal efficiency of ∼99.2% within 120 min, and the adsorbent was used repeatedly.
In most earlier studies, only one parameter was varied at a time, which does not take into consideration the interaction and influence of other parameters simultaneously affecting the adsorption process. This may be time-consuming and costly for industrial applications.33 To remediate this challenge, this study employs computational intelligent algorithms, i.e., response surface methodology (RSM) and an artificial neural network (ANN), to design, optimize, predict and validate the non-linear relationships between the independent and dependent variables as well as to study the shared interactions of the adsorption parameters. This technique was seen to improve process efficiency, determine the impact of combining several parameters at a time, minimize the number of experimental runs, and reduce the cost and time of process optimization.
RSM is used to construct mathematical models based on a specific experimental design with linear, quadratic and optimum interaction terms for predicting the effects of certain observed variables on a response output.34 ANN mimics the behaviour of the human brain in processing information, and it can learn, predict and correlate the pattern of experimental data when subjected to training.35 The ANN technique provides a platform that can determine the impact of some optimized adsorption parameters on the behaviour of a target output.36 RSM was used to optimize the design of the ANN architecture to obtain the best input combination for training, testing and validation. Although the superiority of the integration of RSM and ANN for wastewater remediation has been demonstrated,37,38 scant studies by researchers can be found in the literature. Here, the predictive, sensitivity and generalization capabilities of RSM and ANN in the removal of dicamba and MCPA by MIL-53(Al) were compared, and this has not been reported before.
Materials and methods
All chemicals and solvents used were of analytical grade and were used without further purification. Aluminium nitrate nonahydrate (Al(NO3)3·9H2O, ≥98%), terephthalic acid (TPA, 99%), N,N-dimethyl formamide (DMF, 99%), methanol (99.5%), hydrochloric acid and sodium hydroxide were supplied by Avantis Laboratory (Ipoh Perak, Malaysia), while dicamba and MCPA were purchased from Sigma-Aldrich (St. Louis, MO, USA).
Preparation of MIL-53(Al) adsorbent
MIL-53(Al) was hydrothermally synthesized according to a previous procedure.39 Thus, aluminium nitrate (Al(NO3)3) (13 g) and terephthalic acid (TPA) (2.88 g) were dissolved in deionized water (50 mL). The mixture was homogenized for 30 min with a magnetic stirrer and the suspension was placed in a Teflon-lined stainless-steel autoclave, sealed and heated in an oven for 72 h at 493 K. Next, the reactor was allowed to cool to room temperature and the product was filtered, then washed with DMF and distilled water several times to remove possible impurities and unreacted (1,4-benzenedicarboxylate) materials. The final product was dried for 2 h at 353 K and finally activated at 473 K for 8 h.
Characterization of MIL-53(Al) adsorbent
The thermal stability of the adsorbent was assessed by thermogravimetric analysis (TGA) under N2 atmosphere using a Shimadzu TGA-50 Analyzer and was heated from 30–800 °C at a heating rate of 10 °C min−1. A Bruker D8 Advance X-ray diffractometer (XRD) was used to determine the crystallinity of the material. The functional group of the MIL-53(Al) was determined by scanning from 400 to 4000 cm−1 on a PerkinElmer FTIR spectrometer. Field emission scanning electron microscopy (FESEM) was used to determine the morphology using a Zeiss Supra 55 VP instrument, while the BET surface area and pore size were analysed using N2 adsorption–desorption with a Micrometric ASAP 2020.
Batch adsorption studies
Stock solutions of dicamba and MCPA (1000 mg L−1) were prepared by dissolving the analyte standard (0.1 g L−1) in a 1000 mL volumetric flask and maintaining it in a refrigerator (0 °C) prior to usage. Adsorptions were carried out at different concentrations by subsequent dilution of the stock solution with distilled water. MIL-53(Al) adsorbent (0.01 g L−1) was suspended in a conical flask (100 mL) of a solution containing 5–50 mg L−1 initial concentrations of dicamba and MCPA. The flask was then placed inside a temperature-regulated incubator shaker (incubator ES 20/60, Biosan) and agitated at 150 rpm for 5–60 min. At every 5 min interval, the sample solution (2 mL) was collected and filtered with a nylon syringe membrane (0.45 μm). The absorbances of the dicamba and MCPA solutions were analysed at λmax of 275 and 279 nm with a UV-vis spectrophotometer (Shimadzu, Lamda 25), respectively. The effects of pH and temperature were also studied by adjusting the initial pH from 2 to 12 using either 0.1 M HCl or 0.1 M NaOH, while the temperature was varied from 25 °C to 50 °C. The dosage was varied from 0.005 to 0.05 g L−1. All the adsorption data were recorded in triplicate, from which the average values were calculated. The optimum conditions used for the batch experiment were set as pH: 4; concentration: 20 mg L−1; dosage: 0.01 g L−1; temperature: 40 °C; and contact time: 25 min.
The quantities of dicamba and MCPA adsorbed at equilibrium (qe), percentage removal (%R) and quantities adsorbed at a time interval (qt) were calculated using the following equations:
|
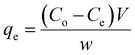 | (1) |
|
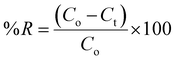 | (2) |
|
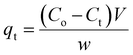 | (3) |
where
Co,
Ct and
Ce are the initial, time and equilibrium concentrations of dicamba and MCPA (mg g
−1),
v is the volume of the solution (L), and
w is the weight of the adsorbent (g).
Model design and optimization by response surface methodology (RSM)
RSM uses mathematical and statistical modelling techniques for the approximation and optimization of critical parameters that affect the behaviour of a given response.40 Using this model, central composite design (CCD) was applied to determine the best experimental data combination to be obtained from the preliminary one-factor-at-a-time experiments. The CCD was selected because of its ability to accommodate a range of factors and give a precise prediction.41 In this study, the CCD was fitted using the second-order polynomial quadratic equation (eqn (4)) with five independent variables comprising contact time, initial concentration, adsorbent dosage, pH, and temperature, while the removal capacities of dicamba and MCPA were the response variables. The accuracy and significance of the fitted model were ascertained by the analysis of variance (ANOVA) statistic. At a confidence level of 95%, the model with the highest F-value and lowest P-value suggests a better combination of the parameters used.42 |
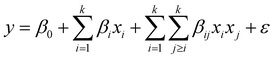 | (4) |
where i is the linear coefficient, j is the quadratic coefficient, k is the number of factors, xi and xj are independent variables, y is the predicted response, β0 is the constant, βi is the linear coefficient, βij is the interactive coefficient, and ε is the noise or error detected in the reply.
Artificial neural network (ANN)
ANN is an intelligent modelling technique that mimics the behaviour of the biological nervous system (brain) in processing information.43,44 ANN can learn and predict the pattern of experimental data when subjected to training, which allows it to model the complex non-linear relationship between the independent and dependent variables.45 ANN has the ability to predict, cluster, optimize and apportion the impact of certain optimization parameters in the behaviour of an expected output. In this study, a multilayer-perceptron feed-forward-neural network (MLP-FF-ANN) with a back-propagation algorithm and log-sigmoid activation function46 was used to predict the adsorption capacities of dicamba and MCPA onto MIL-53(Al) in comparison with the experimental results.
The network structure of the MLP-FF-ANN consisted of multiple neurons that are organized in layers. The input neuron with five parameters (contact time, initial concentration adsorbent dosage, pH and temperature) fed the network with the required information. Signals were disseminated to the hidden neuron via a system of weighted connections where the actual processing was performed and were finally disseminated to the output layer.47 The number of hidden neurons was determined on the basis of trial and error, which forms the training process.48 Two neurons that show the adsorption capacities of dicamba and MCPA were predicted as the output layer. The dataset was divided into training (60%), testing (20%) and validation (20%) sets. The network was trained by adjusting the weight to learn the data pattern, while the validation datasets were used to evaluate the network efficiency. The R2, R2adj and RMSE values were selected as the criteria to assess the model performance. The following equations were used:
|
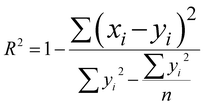 | (5) |
|
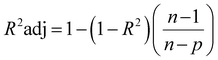 | (6) |
|
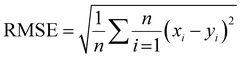 | (7) |
|
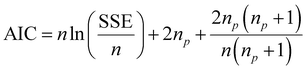 | (8) |
where
xi represents the observed data that were determined experimentally,
yi is the predicted data,
n is the number of observations and
p denotes the number of parameters.
Reusability studies
The reusability of an adsorbent is studied to assess its potential for regeneration. After the adsorption experiments, the supernatant was decanted, and the MOF residue was soaked in 100 mL of acetone for 24 h. Subsequently, the adsorbent was filtered and washed with distilled water several times. The resulting MOF was vacuum-dried for 4 h at 80 °C and maintained in the desiccator prior to reuse. For the reusability study, the regenerated MOF adsorbent was used for the adsorption experiment based on the optimized conditions, similar to the batch adsorption studies. The MOF was used for 5 cycles.
Results and discussion
Characterization of MIL-53(Al)
The XRD pattern indicates peaks that match those of a previous study32 (Fig. 1(a)), which implies that MIL-53(Al) possesses high crystallinity and that the structure was successfully formed. TGA was used to assess the thermal stability of the MIL-53(Al) (Fig. 1(b)). The first weight loss is around 282 °C, attributed to the desorption of adsorbed guest molecules from the pores. The most significant weight loss was observed from 350 °C to 555 °C, denoting complete decomposition of terephthalic acid in the framework. The MOF was completely decomposed at 800 °C, in agreement with an earlier study.49 The FTIR spectrum of MIL-53(Al) is displayed in Fig. 1(c). The strong and sharp peaks at 3679 cm−1 are due to the O–H stretching vibration.39 The peaks at 2879 cm−1 and 2993 cm−1 are assigned to the C–H stretching vibration.50 The peak at 1703 cm−1 shows the absorption band that corresponds to the C
O stretching of the free 1,4-benzenedicarboxylate encapsulated inside the pores of the MOF.51 Intense peaks were observed at 1601, 1510, and 1416 cm−1; these are attributed to the asymmetric and symmetric stretching and bending vibrations of the carboxylate group, which can be adsorption sites for the herbicides.51 The morphology of the MOF as seen from the FESEM image (Fig. 1(d)) corresponds to a hexagonal pyramid structure of well-formed MIL-53(Al). The elemental composition on the surface of the adsorbent was also verified using EDX (Fig. 1(d)), indicating the presence of aluminium, oxygen and carbon. The nitrogen adsorption–desorption isotherm of MIL-53(Al) was calculated at 77 K (Fig. 1(e)) with no hysteresis; it exhibits a type I isotherm curve, which is typical of highly porous materials. The BET surface area of MIL-53(Al) is about 1104 m2 g−1, and the micropore volume is 0.63 cm3 g−1.
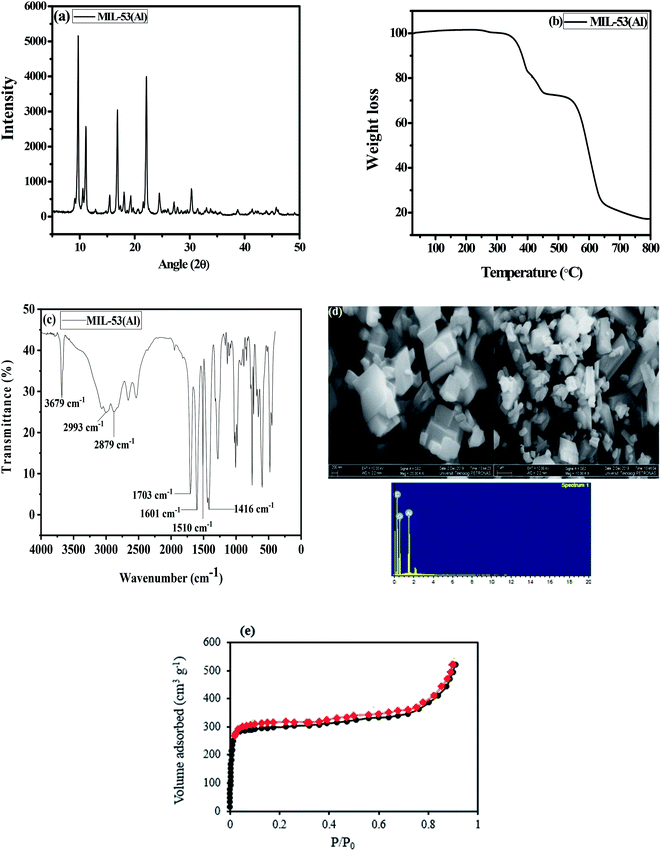 |
| Fig. 1 (a) XRD pattern (b), TGA thermogram (c), FTIR spectrum (d), FESEM-EDX spectrum and (e) N2 adsorption–desorption isotherm of MIL-53(Al). | |
Adsorption of dicamba and MCPA in water based on experimental findings
Effects of adsorbent dosage. The effectiveness of the adsorbent (MIL-53(Al)) for the removal of the analytes in water was studied by varying the loading from 0.005 to 0.05 g L−1 in 50 mL solution containing 20 mg L−1 of dicamba and MCPA. The results obtained are presented in Fig. 2(a). High removal efficiency was observed even at the smaller dosages of 0.005 g L−1 (98.2% and 97.7%) and 0.01 g L−1 (99.2% and 98.5%) for dicamba and MCPA, respectively, within the first 5–25 min of contact due to the availability of vacant and active adsorption sites in the adsorbent. Further increase in the dosage from 0.02–0.05 g L−1 had little impact on the removal efficiency. Hence, 0.01 g L−1 adsorbent was used for all the subsequent experiments.
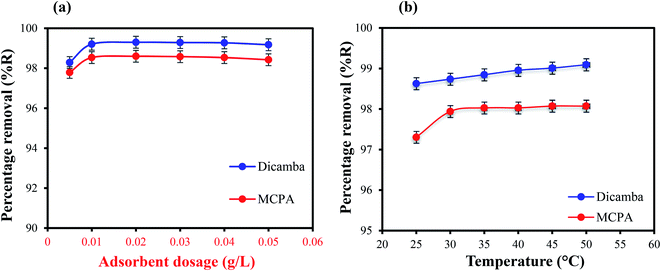 |
| Fig. 2 (a) Effects of adsorbent dosage the red colour highlights in (a) should be black and (b) temperature on the removal of dicamba and MCPA (herbicide concentration, 20 mg L−1; equilibrium time, 25 min; rpm, 150). | |
Effects of temperature. The temperature at which adsorption takes place plays an important role in the removal process. It is used to study the thermodynamics behaviour and spontaneity of the adsorption system. Thus, the effects of temperature (25 °C–50 °C) on the adsorption efficiency were studied, and the results are depicted in Fig. 2(b). An increase in the temperature leads to an increase in the adsorption capacity for both the herbicides.
Effects of contact time. Variation of the contact time of the adsorption was used to study the kinetics of the adsorption process. Thus, the removal of dicamba and MCPA was studied at different concentrations (5–50 mg L−1), with a contact time of 5–60 min, under the optimized pH, adsorbent dose and temperature conditions (pH 4, 0.01 g L−1 and 40 °C). The results obtained are plotted in Fig. 3(a) and (b), indicating rapid and high removal of the herbicides. Between 5 and 10 min, rapid removals of both dicamba and MPCA were recorded due to the favourable interactions between the pollutants and the MOF. The MIL-53(Al) MOF has large numbers of vacant and active adsorption sites due to its porous nature. This is corroborated by the high BET surface area of the adsorbent (1104 m2 g−1). Hence, equilibrium was attained within 25 min for both dicamba and MCPA. The contact time was extended until 60 min to ensure better interactions of the MOF with the analytes after the equilibrium was established.
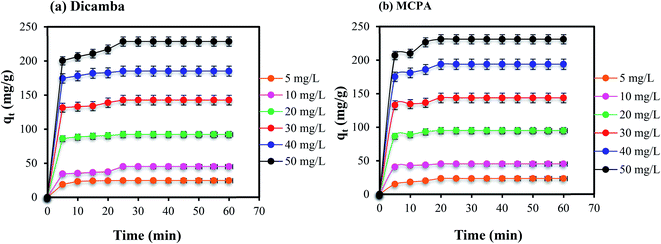 |
| Fig. 3 Effects of contact time on the removal of (a) dicamba and (b) MCPA (dosage, 0.01 g L−1; equilibrium time, 25 min; temperature, 40 °C; rpm, 150). | |
RSM modelling and optimization. The factor levels and experimental data matrix designed by RSM in Tables S1 and S2† show a combination of five input parameters and levels used to predict the adsorption capacity of dicamba and MCPA. The analysis of variance (ANOVA) results presented in Table S3† show a significant data combination, with model F-values of 73.4 and 70.8 and p-values less than 0.05 for dicamba and MCPA, respectively. This model implies that there is only a 0.01% chance that an F-value this large could occur due to noise. The small p-values obtained indicate that the quadratic model is statistically significant to track the design domain and can be used to predict the adsorption capacity of dicamba and MCPA. Model reduction was applied to eliminate some of the noisy terms that are insignificant and to improve the model performance.33 In this case, the following combinations that impact the adsorption capacity are described below.Dicamba adsorption capacity (mg g−1) =
|
10.4 − 0.5A + 4.8B + 0.4C − 0.2D − 0.3E + 0.5AB − 0.8AC + 0.7AD − 0.6BC + 0.5BD + 0.4BE − 0.7CD − 0.5CE + 0.5DE − 1.1B2 + 0.9C2 + 0.7E2
| (9) |
MCPA adsorption capacity (mg g−1) =
|
10.5 − 0.6A + 4.9B + 0.4C − 0.2D − 0.3E + 0.5AB − 0.7AC + 0.7D − 0.6BC + 0.5BD + 0.4BE − 0.7CD − 0.5CE + 0.5DE − 1.2B2 + 1.1C2 + 0.7E2
| (10) |
The results of the RSM model show significant coefficients of determination of R2 = 0.988 and 0.987; R2adj = 0.974 and 0.976; and R2pred = 0.866 and 0.870 for dicamba and MCPA, respectively. These results imply a good correlation between the actual and predicted values of the response. Adequate precision was also observed in the model (AP = 24.5 and 24.7, respectively), which represents the signal-to-noise ratio for the model adequacy. This is better described by the scatter plots in Fig. S1(a) and (b),† which show a significant relationship and adequate estimate between the actual and predicted variables. The optimum conditions for the dicamba and MCPA adsorption capacity based on the shared interaction between contact time (25 min), initial concentration (50 mg L−1), adsorbent dosage (0.01 g L−1), pH (4) and temperature (40 °C) are presented in Fig. 4. The three-dimensional graphs of the RSM plots are in strong agreement with the experimental findings. Fig. 4(a) depicts an increase in the adsorption capacity when the initial concentrations of dicamba and MCPA are varied from 10–50 mg L−1 within a minimal contact time. The equilibrium adsorption time is attained within ∼25 min, and no change is observed when the contact time is extended to 60 min. Higher concentration may result in an increase in the mass transfer driving force on the available vacant and active porous sites of the adsorbent. In addition, MIL-53(Al) has a flexible lattice, which allows the MOF to expand and contract its pore sizes to adsorb more molecules.29 The effects of pH and contact time on the dicamba and MCPA adsorption capacity are shown in Fig. 4(b). An inverse relationship is observed, resulting in a decrease in the adsorption capacity as the pH becomes more alkaline. When the pH of the solution increases from 7 to 12, the surface charge of the MIL-53(Al) adsorbent becomes negative, thereby retarding the possible electrostatic interactions and resulting in low adsorption capacity. This may be due to the strong competition for the available active sites between the –OH and the herbicide molecules.52 At a higher pH level, several functional groups, such as hydroxyl and carbonyl, are in the protonated cationic form, causing a repulsion in the adoption process. Thus, at low pH (2–6), the herbicide solution will be predominantly in the anionic form (negatively charged) due to deprotonation, while the surface of MIL-53(Al) is positively charged. This encourages possible electrostatic interactions, leading to high adsorption capacity. The influence of the adsorbent dose and initial concentration on the adsorption capacities is described in Fig. 4(c). At a minimal dose of 0.01 g L−1, the adsorption capacities of dicamba and MCPA are very high due to the porous nature of the adsorbent material.29 Further increase in the adsorbent did not change the adsorption capacities. This indicates that high adsorption capacities can be achieved with a very small dosage of MIL-53(Al). The impact of temperature change (25 °C–50 °C) and dosage on the adsorption capacities was also observed, and the resultant effects are described in Fig. 4(d). Increasing the temperature range improves the surface activities of the adsorbent material as well as the kinetic energy of the solution. This indicates that temperature plays an important role in determining the adsorption capacity of MIL-53(Al).
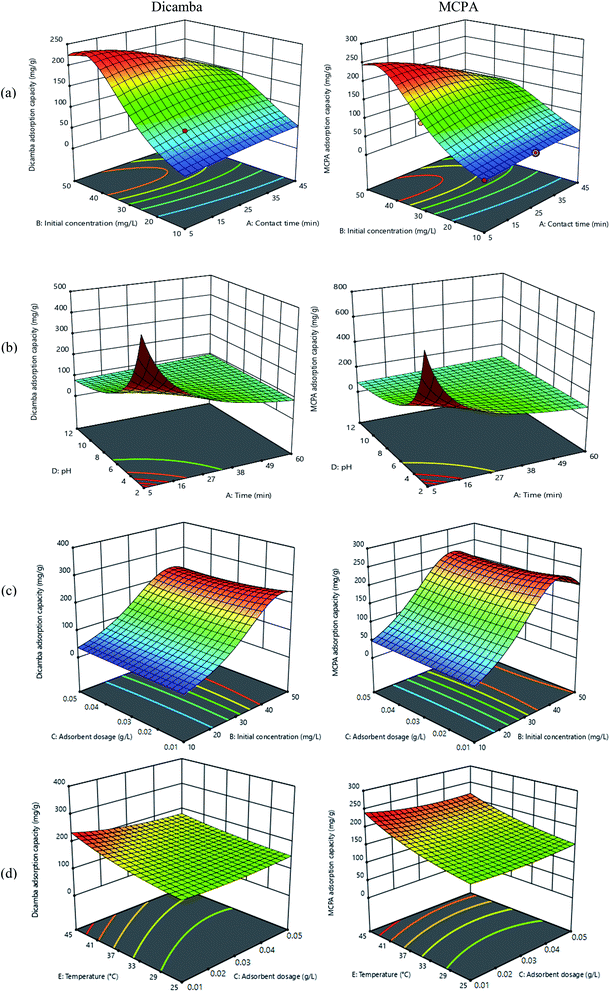 |
| Fig. 4 Shared interaction plots of (a) initial concentration and contact time, (b) pH and contact time, (c) adsorbent dose *9 + 7 and initial concentration (d) with the temperature and adsorbent dose. | |
Artificial neural network model
The most crucial step to develop an ANN network with high accuracy and minimal error is to determine the best input combination, network architecture and model uncertainty.36 RSM was used to optimize the design matrix of the ANN architecture to obtain the best data combination for training, testing and validation. A total of 308 experimental data sets obtained through the CCD were used for the prediction model. The best network architecture was obtained by varying the number of neurons in the hidden layer. This gave rise to a three-structural layer with a 5–7–2 topology (Fig. 5). The first layer has five input neurons (contact time, initial concentration, adsorbent dosage, pH and temperature), the second makes up the hidden layer with seven neurons, and the third layer consists of two neurons as the predicted response (dicamba and MCPA adsorption capacities). The results show a significant prediction by the ANN model with high accuracy. The coefficients of determination for the dicamba and MCPA adsorption capacities are R2 = 0.999 and 0.999; R2adj = 0.997 and 0.995; and RMSE = 0.001 and 0.004, respectively. Table 2 shows the prediction levels of the ANN model, from which the best architecture was obtained with seven neurons. Fig. S1(c) and (d)† show the scatter plots of the ANN model, which depict the relationship between the actual and predicted response values.
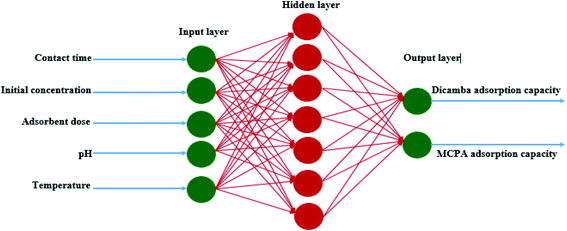 |
| Fig. 5 Artificial neural network topology. | |
Table 2 Optimum ANN architecture for the prediction of the dicamba and MCPA adsorption capacities
No. |
Architectures |
Dicamba removal |
MCPA removal |
R2 |
R2adj |
RMSE |
R2 |
R2adj |
RMSE |
1 |
[3] |
0.999 |
0.932 |
1.133 |
0.996 |
0.862 |
3.341 |
2 |
[4] |
0.997 |
0.988 |
0.788 |
0.998 |
0.994 |
1.769 |
3 |
[5] |
0.998 |
0.992 |
0.474 |
0.990 |
0.993 |
1.917 |
4 |
[6] |
0.996 |
0.994 |
1.182 |
0.993 |
0.991 |
0.520 |
5 |
[7] |
0.999 |
0.997 |
0.001 |
0.999 |
0.995 |
0.004 |
6 |
[8] |
0.921 |
0.901 |
0.485 |
0.934 |
0.906 |
0.553 |
7 |
[9] |
0.998 |
0.993 |
1.902 |
0.998 |
0.994 |
1.795 |
8 |
[10] |
0.998 |
0.997 |
1.907 |
0.997 |
0.995 |
2.248 |
9 |
[5 5] |
0.941 |
0.922 |
1.668 |
0.961 |
0.931 |
0.884 |
10 |
[5 7] |
0.975 |
0.964 |
0.392 |
0.941 |
0.920 |
0.221 |
11 |
[7 6] |
0.966 |
0.942 |
1.866 |
0.972 |
0.954 |
1.022 |
Comparison of RSM and ANN prediction performance
The capability of RSM and ANN was assessed to determine the model that best predicts the experimental adsorption capacities of dicamba and MCPA. Although both models are statistically significant with good performance, the results presented in Table 3 show that the predicted values of the ANN model are in better agreement with the experimental findings when compared with RSM. At each data combination used for the adsorption studies, ANN was able to predict the experimental results with a high level of significance and also validate them. The coefficients of determination of the ANN model are higher than those of RSM. The ANN has R2 = 0.999 and 0.999; R2adj = 0.983 and 0.988; and RMSE = 0.001 and 0.004, while RSM has R2 = 0.988 and 0.987 and R2adj = 0.974 and 0.976 for dicamba and MCPA, respectively. Also, the ANN model displays minimal error response compared to the RSM. This is because the ANN mimics the human brain by learning the pattern of the datasets, and it can generalize the nonlinear relationship between the actual and the predicted results.
Table 3 Comparison of prediction capabilities by RSM and ANN
Dicamba adsorption capacity (mg g−1) |
MCPA adsorption capacity (mg g−1) |
Actual |
RSM |
ANN |
Actual |
RSM |
ANN |
Predicted |
Error |
Predicted |
Error |
Predicted |
Error |
Predicted |
Error |
84.3 |
80.3 |
4.0 |
84.1 |
0.2 |
92.4 |
89.1 |
3.3 |
90.7 |
1.8 |
34.3 |
34.6 |
0.2 |
34.3 |
0.0 |
40.7 |
40.8 |
0.1 |
40.8 |
0.1 |
89.7 |
82.5 |
7.2 |
89.7 |
0.0 |
93.2 |
86.0 |
7.2 |
92.1 |
1.1 |
89.1 |
82.3 |
6.8 |
90.1 |
1.0 |
93.2 |
85.9 |
7.3 |
93.0 |
0.1 |
185.1 |
186.5 |
1.4 |
185.1 |
0.0 |
193.8 |
190.4 |
3.5 |
193.8 |
0.0 |
45.1 |
44.4 |
0.7 |
44.3 |
0.8 |
45.2 |
45.4 |
0.2 |
46.5 |
1.2 |
89.7 |
85.9 |
3.8 |
89.7 |
0.0 |
93.2 |
89.9 |
3.3 |
92.0 |
1.2 |
131.5 |
137.4 |
5.8 |
131.4 |
0.1 |
131.5 |
136.8 |
5.3 |
133.0 |
1.5 |
131.5 |
132.2 |
0.8 |
131.0 |
0.5 |
132.9 |
132.4 |
0.5 |
132.8 |
0.1 |
131.5 |
131.1 |
0.4 |
131.5 |
0.0 |
132.1 |
131.4 |
0.7 |
134.5 |
2.4 |
34.3 |
34.9 |
0.5 |
34.5 |
0.1 |
40.7 |
41.3 |
0.6 |
41.4 |
0.7 |
45.1 |
45.8 |
0.7 |
45.1 |
0.0 |
45.2 |
46.0 |
0.8 |
45.0 |
0.2 |
142.6 |
155.9 |
13.4 |
142.6 |
0.0 |
143.6 |
150.3 |
6.7 |
144.1 |
0.4 |
141.6 |
150.2 |
8.7 |
142.2 |
0.7 |
143.6 |
152.4 |
8.7 |
142.1 |
1.6 |
34.0 |
33.0 |
1.0 |
34.3 |
0.0 |
40.7 |
40.6 |
0.1 |
41.1 |
0.4 |
131.5 |
139.8 |
8.4 |
131.5 |
0.0 |
132.9 |
140.0 |
7.1 |
132.9 |
0.0 |
92.1 |
89.3 |
2.8 |
94.7 |
2.5 |
94.9 |
89.8 |
5.1 |
95.8 |
0.9 |
131.0 |
134.2 |
3.2 |
131.9 |
0.9 |
132.4 |
139.0 |
6.6 |
132.4 |
0.0 |
131.5 |
133.4 |
1.9 |
131.5 |
0.0 |
132.9 |
134.4 |
1.5 |
132.3 |
0.6 |
45.1 |
45.2 |
0.1 |
44.7 |
0.4 |
45.2 |
46.0 |
0.8 |
46.9 |
1.6 |
142.6 |
152.3 |
9.7 |
142.6 |
0.0 |
143.6 |
151.5 |
7.9 |
143.8 |
0.2 |
89.7 |
86.0 |
3.7 |
89.7 |
0.0 |
93.2 |
88.9 |
4.2 |
93.1 |
0.1 |
44.1 |
46.6 |
2.5 |
45.1 |
1.0 |
45.2 |
46.6 |
1.4 |
46.2 |
1.0 |
45.3 |
46.4 |
1.1 |
45.1 |
0.2 |
45.0 |
46.4 |
1.4 |
45.9 |
0.8 |
92.1 |
87.7 |
4.5 |
92.1 |
0.0 |
94.9 |
90.4 |
4.4 |
94.7 |
0.2 |
92.1 |
92.0 |
0.2 |
94.2 |
2.1 |
94.9 |
92.3 |
2.6 |
94.8 |
0.0 |
34.3 |
34.4 |
0.1 |
34.3 |
0.0 |
40.7 |
41.1 |
0.4 |
41.1 |
0.4 |
34.3 |
34.9 |
0.5 |
34.6 |
0.2 |
40.7 |
41.3 |
0.6 |
41.7 |
1.0 |
142.6 |
144.5 |
1.9 |
142.6 |
0.0 |
143.6 |
145.7 |
2.1 |
144.0 |
0.3 |
131.5 |
129.9 |
1.5 |
131.5 |
0.0 |
132.9 |
131.7 |
1.2 |
133.5 |
0.6 |
142.6 |
150.1 |
7.6 |
142.8 |
0.2 |
143.6 |
151.0 |
7.3 |
144.6 |
0.9 |
34.3 |
33.8 |
0.6 |
34.3 |
0.0 |
40.7 |
40.2 |
0.5 |
40.8 |
0.1 |
131.5 |
135.1 |
3.6 |
131.4 |
0.0 |
132.9 |
135.6 |
2.7 |
134.0 |
1.1 |
142.6 |
147.7 |
5.2 |
142.6 |
0.0 |
143.6 |
148.7 |
5.1 |
144.0 |
0.4 |
34.3 |
35.0 |
0.6 |
34.3 |
0.0 |
40.7 |
41.7 |
1.0 |
41.1 |
0.4 |
45.1 |
44.8 |
0.4 |
45.1 |
0.0 |
45.2 |
45.6 |
0.4 |
45.3 |
0.1 |
88.1 |
86.7 |
1.4 |
89.7 |
1.6 |
92.6 |
89.6 |
3.0 |
91.9 |
0.7 |
89.7 |
87.3 |
2.3 |
89.7 |
0.0 |
93.2 |
92.7 |
0.5 |
92.4 |
0.7 |
228.5 |
228.5 |
0.0 |
228.3 |
0.2 |
231.1 |
232.0 |
0.8 |
231.9 |
0.8 |
34.3 |
34.5 |
0.2 |
34.3 |
0.0 |
40.7 |
40.7 |
0.0 |
40.8 |
0.1 |
36.3 |
38.4 |
2.0 |
36.4 |
0.0 |
43.6 |
43.1 |
0.5 |
42.1 |
1.6 |
142.6 |
148.9 |
6.3 |
142.6 |
0.0 |
143.6 |
149.3 |
5.7 |
144.2 |
0.6 |
141.3 |
139.9 |
1.4 |
142.6 |
1.2 |
142.0 |
145.4 |
3.4 |
142.7 |
0.7 |
45.1 |
45.6 |
0.5 |
45.1 |
0.0 |
45.2 |
45.8 |
0.6 |
46.9 |
1.7 |
Reusability studies and re-characterisation
The prospects of the MOF for repeated removal of the pollutants were studied in view of its regeneration possibility. The removal efficiency of the material was ∼90% even after the fifth cycle (Fig. 6), which is better compared with the materials presented in Table 4. A slight decrease in the percentage removal of the herbicides after the fourth cycle (∼3%) and fifth cycle (∼8%) was found. The adsorbent was re-characterised by FTIR, FESEM and EDX to determine the stability of the MOF (Fig. 7). The result show the good similarity with the pristine MIL-53(Al) and stabilility after several uses.
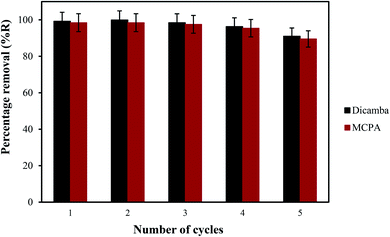 |
| Fig. 6 Regeneration and reusability potential of MIL 53(Al). | |
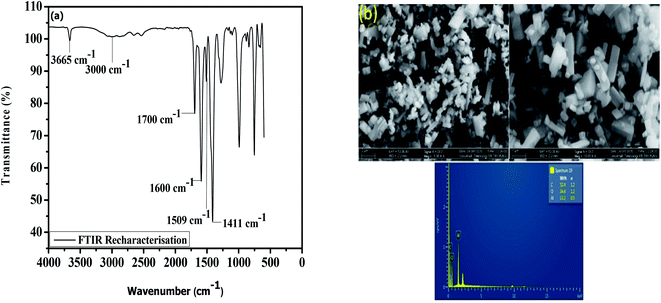 |
| Fig. 7 Re-characterisation of the adsorbent after use by (a) FTIR and (b) FESEM-EDX. | |
Comparison with other adsorbent materials
The performance of different adsorbents that have been reported for the removal of dicamba and MCPA in water is summarised in Table 4. The superiority of the MIL-53(Al) adsorbent is readily seen, especially in terms of high surface area, adsorption capacity, %removal, fast equilibration time and prospects for regeneration.
Table 4 Adsorbents reported for the removal of dicamba and MCPA from water
Adsorbent |
Surface area (m2 g−1) |
Analytes |
Concentrations (mg L−1) |
(%) removal |
Adsorption capacity (mg g−1) |
Equilibrium time (min) |
Reuse |
Ref. |
Activated carbon |
592 |
MCPA |
50 |
70 |
417 |
210 |
— |
53 |
Bentonite |
20 |
MCPA |
1 |
80–95 |
|
1440 |
— |
54 |
Biochar |
1.1 |
MCPA |
100 |
90 |
28.1 |
360 |
— |
55 |
Metal hydroxide |
NIL |
MCPA |
50 |
84 |
42 |
240 |
NIL |
56 |
MIL-53(Al) |
1104 |
MCPA |
50 |
98 |
231.1 |
25 |
5 |
This work |
Mesoporous carbon |
876 |
Dicamba |
250 |
NIL |
222.2 |
60 |
— |
57 |
Carbon nanotubes |
600 |
Dicamba |
50 |
85.5 |
20.7 |
— |
— |
58 |
MIL-53(Al) |
1104 |
Dicamba |
50 |
99 |
228.4 |
25 |
5 |
This work |
Conclusion
A detailed study outlining the adsorption and removal of the herbicides dicamba and MCPA from aqueous medium was carried out using MIL-53(Al). The hydrothermally synthesized MOF was characterised by a high surface area (1104 m2 g−1) and pore volume (0.63 cm3 g−1) as determined by the BET analysis. XRD analysis reveals its high crystallinity and well-formed hexagonal pyramid structure. The adsorption of dicamba and MCPA reached equilibrium within ∼25 min using a small dose of adsorbent (0.01 g L−1). Adsorption kinetics such as pseudo-first-order, pseudo-second-order and intraparticle diffusion were studied, and the adsorption process best fit the pseudo-second-order model, with R2 > 0.99% and maximum qe values of 227.2 and 232.5 mg g−1 for dicamba and MCPA, respectively. Different isotherm models were also studied, and the results were best fitted by the Freundlich isotherm, with the highest values of R2 = 0.974 and 0.962 for dicamba and MCPA, respectively. The thermodynamic studies suggest that the adsorption is endothermic and spontaneous in nature, with increased randomness at the liquid–solid interface. CCD-RSM was used to design the data matrix and optimum conditions for the adsorption process. The data matrix designed by CCD-RSM were used for the ANN prediction. ANN gave better prediction than the RSM model with minimal error. In short, MIL-53(Al) shows superior characteristics as an adsorbent for the removal of dicamba and MCPA compared to previously reported adsorbents, especially in terms of rapid equilibration, which was contributed by its large surface area and pore volume and its prospects for regeneration.
Conflicts of interest
The authors declare no conflict of interest.
Acknowledgements
This project was supported by YUTP Grant provided by Universiti Teknologi PETRONAS (cost centre 015LCO-211) and UTM CRG Collaborative Reseach Grant (015MD0-044).
References
- A. López-piñeiro, D. Peña, Á. Albarrán, J. Sánchez-llerena, D. Becerra, D. Fernández and S. Gómez, J. Environ. Manage., 2019, 237, 44–53 CrossRef.
- L. Yao, X. Jia, J. Zhao, Q. Cao, X. Xie, L. Yu and J. He, Int. Biodeterior. Biodegrad., 2015, 104, 324–332 CrossRef CAS.
- A. Spaltro, M. Pila, S. Simonetti, S. Álvarez-Torrellas, J. G. Rodríguez, D. Ruiz, A. D. Compañy, A. Juan and P. Allegretti, J. Contam. Hydrol., 2018, 218, 84–93 CrossRef CAS.
- D. Wu, Y. Yun, L. Jiang and C. Wu, Sci. Total Environ., 2018, 616–617, 1449–1456 CrossRef CAS.
- M. Turek, B. Pawłowska, E. Różycka-Sokołowska, R. Biczak, J. Skalik, K. Owsianik, B. Marciniak and P. Bałczewski, J. Hazard. Mater., 2020, 382, 121086 CrossRef CAS.
- C. Ruiz de Arcaute, M. L. Larramendy and S. Soloneski, Environ. Pollut., 2018, 243, 670–678 CrossRef CAS.
- P. K. Gupta, Toxicity of Herbicides, Elsevier Inc., 3rd edn, 2018 Search PubMed.
- J. Kelly, G. Morrison, N. Skillen, P. Manesiotis and P. K. J. Robertson, Chem. Eng. J., 2019, 359, 112–118 CrossRef CAS.
- W. Fawcett-hirst, T. J. Temple, M. K. Ladyman and F. Coulon, Chemosphere, 2020, 255, 126848 CrossRef CAS.
- M. Watson, J. M. Jazić, T. Đurkić, B. Bašić, T. Apostolović, A. Tubić and J. Agbaba, Environ. Sci.: Water Res. Technol., 2020, 6, 2800–2815 RSC.
- A. M. Awad, S. M. R. Shaikh, R. Jalab, M. H. Gulied, M. S. Nasser, A. Benamor and S. Adham, Sep. Purif. Technol., 2019, 228, 115719 CrossRef CAS.
- S. Dhaka, R. Kumar, A. Deep, M. B. Kurade, S. W. Ji and B. H. Jeon, Coord. Chem. Rev., 2019, 380, 330–352 CrossRef CAS.
- Z. Noorimotlagh, M. Ravanbakhsh, M. R. Valizadeh, B. Bayati, G. Z. Kyzas, M. Ahmadi, N. Rahbar and N. Jaafarzadeh, Polyhedron, 2020, 179, 114354 CrossRef CAS.
- I. P. P. Cansado, P. A. M. Mourão, J. A. F. L. Gomes and V. Almodôvar, Cienc. Tecnol. Mater., 2017, 29, e224–e228 Search PubMed.
- X. Hou, S. Tang, X. Guo, L. Wang, X. Liu, X. Lu and Y. Guo, J. Chromatogr. A, 2018, 1571, 65–75 CrossRef CAS.
- A. Iglesias, R. López, D. Gondar, J. Antelo, S. Fiol and F. Arce, J. Hazard. Mater., 2010, 183, 664–668 CrossRef CAS.
- Y. Dai, N. Zhang, C. Xing, Q. Cui and Q. Sun, Chemosphere, 2019, 223, 12–27 CrossRef CAS.
- Y. Tong, P. J. Mcnamara and B. K. Mayer, Environ. Sci.: Water Res. Technol., 2019, 5, 821 RSC.
- H. Azejjel, C. Hoyo, K. Draoui, M. S. Rodríguez-cruz and M. J. Sánchez-martín, Desalination, 2009, 249, 1151–1158 CrossRef CAS.
- S. Wadhawan, A. Jain, J. Nayyar and S. K. Mehta, J. Water Process. Eng., 2020, 33, 101038 CrossRef.
- Y. Y. Cui, J. Zhang, L. L. Ren, A. L. Cheng and E. Q. Gao, Polyhedron, 2019, 161, 71–77 CrossRef CAS.
- Q. Pang, B. Tu and Q. Li, Coord. Chem. Rev., 2019, 388, 107–125 CrossRef CAS.
- D. Chen, J. Zhao, P. Zhang and S. Dai, Polyhedron, 2019, 162, 59–64 CrossRef CAS.
- T. Ghanbari, F. Abnisa and W. M. A. Wan Daud, Sci. Total Environ., 2020, 707, 135090 CrossRef CAS.
- L. Joseph, B. M. Jun, M. Jang, C. M. Park, J. C. Muñoz-Senmache, A. J. Hernández-Maldonado, A. Heyden, M. Yu and Y. Yoon, Chem. Eng. J., 2019, 369, 928–946 CrossRef CAS.
- A. Karami, R. Sabouni and J. Ghommem, J. Mol. Liq., 2020, 101283 Search PubMed.
- A. Samokhvalov, Coord. Chem. Rev., 2018, 374, 236–253 CrossRef CAS.
- B. Seoane, C. Téllez, J. Coronas and C. Staudt, Sep. Purif. Technol., 2013, 111, 72–81 CrossRef CAS.
- Y. Gao, R. Kang, J. Xia, G. Yu and S. Deng, J. Colloid Interface Sci., 2019, 535, 159–168 CrossRef CAS.
- Y. Guan, M. Xia, X. Wang, W. Cao and A. Marchetti, Inorg. Chim. Acta, 2019, 484, 180–184 CrossRef CAS.
- B. Li, J. Q. Zheng, J. Z. Guo and C. Q. Dai, Chem. Eng. J., 2020, 383, 123174 CrossRef CAS.
- Y. Jia, Y. Chen, J. Luo and Y. Hu, Ecotoxicol. Environ. Saf., 2019, 184, 109670 CrossRef CAS.
- M. R. Gadekar and M. M. Ahammed, J. Environ. Manage., 2019, 231, 241–248 CrossRef CAS.
- R. Bagheri, M. Ghaedi, A. Asfaram, E. Alipanahpour Dil and H. Javadian, Polyhedron, 2019, 171, 464–472 CrossRef CAS.
- M. Dolatabadi, M. Mehrabpour, M. Esfandyari and H. Alidadi, Chemom. Intell. Lab. Syst., 2018, 181, 72–78 CrossRef CAS.
- Z. Uddin, L. Yao, Q. Lian, F. Islam, M. E. Zappi and D. Dianchen, Chemosphere, 2020, 256, 127081 CrossRef.
- M. Hadi, R. Rao, Z. Tafaroji and A. Hossein, J. Mol. Liq., 2020, 302, 112526 CrossRef.
- A. Samadi-Maybodi and M. Nikou, Polyhedron, 2020, 179, 114342 CrossRef CAS.
- T. Loiseau, C. Serre, C. Huguenard, G. Fink, F. Taulelle, M. Henry, T. Bataille and G. Fÿrey, Chem. – Eur. J., 2004, 10, 1373–1382 CrossRef CAS.
- S. Maleki and A. Karimi-Jashni, Chemosphere, 2020, 246, 125710 CrossRef CAS.
- E. Mossavi, M. H. Sabzevari, M. Ghaedi, M. H. A. Azqhandi and S. J. Hosseini, Polyhedron, 2019, 171, 65–76 CrossRef CAS.
- H. Soleimanzadeh, A. Niaei, D. Salari, A. Tarjomannejad, S. Penner, M. Grünbacher, S. Ali and S. Mahdi, J. Environ. Manage., 2019, 238, 360–367 CrossRef CAS.
- H. A. Isiyaka, H. Juahir and P. Phil-, Model. Earth Syst. Environ., 2019, 5, 583–593 CrossRef.
- R. Karimi, F. Yousefi, M. Ghaedi and Z. Rezaee, Polyhedron, 2019, 170, 60–69 CrossRef CAS.
- A. M. Ghaedi and A. Vafaei, Adv. Colloid Interface Sci., 2017, 245, 20–39 CrossRef CAS.
- T. Severo, M. De Freitas, T. Maria, M. Silva, M. Alice, F. Porto, C. Malala, M. Souza and A. F. Mota, Chemosphere, 2019, 236, 1–15 Search PubMed.
- P. S. Pauletto, J. O. Gonçalves, L. A. A. Pinto, G. L. Dotto and N. P. G. Salau, J. Colloid Interface Sci., 2020, 560, 722–729 CrossRef CAS.
- X. Wu, S. Wang, R. Zhang and Z. Gao, J. Chromatogr. A, 2014, 1332, 14–20 CrossRef CAS.
- A. Taheri, E. Ganji and J. Tow, J. Nat. Gas Sci. Eng., 2017, 38, 272–282 CrossRef CAS.
- H. Reinsch, R. S. Pillai, R. Siegel, J. Senker, A. Lieb, G. Maurin and N. Stock, Dalton Trans., 2016, 4179–4186 RSC.
- J. O. Hsieh, K. J. B. Jr, J. P. Ferraris and I. H. Musselman, Microporous Mesoporous Mater., 2014, 196, 165–174 CrossRef CAS.
- M.- Al, M. Zhou, Y. Wu, J. Qiao, J. Zhang, A. Mcdonald, G. Li and F. Li, J. Colloid Interface Sci., 2013, 405, 157–163 CrossRef.
- A. Pandiarajan, R. Kamaraj and S. Vasudevan, Bioresour. Technol., 2018, 261, 329–341 CrossRef CAS.
- E. Durán, S. Bueno, M. C. Hermosín, L. Cox and B. Gámiz, Sci. Total Environ., 2019, 672, 743–751 CrossRef.
- M. Essandoh, D. Wolgemuth, C. U. Pittman, D. Mohan and T. Mlsna, Chemosphere, 2017, 174, 49–57 CrossRef CAS.
- R. Kamaraj, D. J. Davidson, G. Sozhan and S. Vasudevan, J. Environ. Chem. Eng., 2014, 2, 2068–2077 CrossRef CAS.
- M. De Carvalho Eufrásio Pinto, R. G. L. Gonçalves, R. M. M. Dos Santos, E. A. Araújo, G. F. Perotti, R. Dos Santos Macedo, M. A. Bizeto, V. R. L. Constantino, F. G. Pinto and J. Tronto, Microporous Mesoporous Mater., 2016, 225, 342–354 CrossRef.
- K. Pyrzynska, A. Stafiej and M. Biesaga, Microchim. Acta, 2007, 159, 293–298 CrossRef CAS.
Footnote |
† Electronic supplementary information (ESI) available. See DOI: 10.1039/d0ra07969c |
|
This journal is © The Royal Society of Chemistry 2020 |
Click here to see how this site uses Cookies. View our privacy policy here.