DOI:
10.1039/C9LC01045A
(Paper)
Lab Chip, 2020,
20, 84-92
Soft, skin-interfaced microfluidic systems with integrated enzymatic assays for measuring the concentration of ammonia and ethanol in sweat†
Received
22nd October 2019
, Accepted 20th November 2019
First published on 20th November 2019
Abstract
Eccrine sweat is a rich and largely unexplored biofluid that contains a range of important biomarkers, from electrolytes, metabolites, micronutrients and hormones to exogenous agents, each of which can change in concentration with diet, stress level, hydration status and physiologic or metabolic state. Traditionally, clinicians and researchers have used absorbent pads and benchtop analyzers to collect and analyze the biochemical constituents of sweat in controlled, laboratory settings. Recently reported wearable microfluidic and electrochemical sensing devices represent significant advances in this context, with capabilities for rapid, in situ evaluations, in many cases with improved repeatability and accuracy. A limitation is that assays performed in these platforms offer limited control of reaction kinetics and mixing of different reagents and samples. Here, we present a multi-layered microfluidic device platform with designs that eliminate these constraints, to enable integrated enzymatic assays with demonstrations of in situ analysis of the concentrations of ammonia and ethanol in microliter volumes of sweat. Careful characterization of the reaction kinetics and their optimization using statistical techniques yield robust analysis protocols. Human subject studies with sweat initiated by warm-water bathing highlight the operational features of these systems.
Introduction
Eccrine sweat, a biofluid that contains a rich mixture of metabolites (e.g. ammonia, lactate, glucose, urea, creatinine), micronutrients (e.g. ascorbic acid), hormones (e.g. cortisol), proteins (e.g. cytokines), and exogenous agents (e.g. alcohol and drugs),1–5 is of interest as a potential non-invasive alternative to blood and interstitial fluid for monitoring human physiology and biochemistry. Traditional methods for sweat collection and analysis based on absorbent pads and benchtop diagnostic equipment are being supplanted by wearable, real-time platforms that enable monitoring in practical, real-world settings.6–9
Many of the components of sweat can be analyzed passively using colorimetric assays deployed in skin-interfaced soft microfluidic systems.8,10 Related assays can measure species at exceptionally low concentrations through fluorescence imaging.11 Established device designs of these types typically require, however, the chemical reactions to reach equilibrium, thereby precluding the use of enzymatic and other types of assays that develop continuously with time. One solution relies on active, powered electronics to measure elapsed time,12–14 although with associated disadvantages in cost and form factor. As a simple alternative, colorimetric assays have the potential to be used in such contexts via techniques that passively normalize the effective reaction time. This paper introduces skin-interfaced soft microfluidic systems with capacity to exploit enzymatic assays via collections of microchannels, reservoirs, valves and other components cast in low modulus elastomers using the techniques of soft lithography. The result is a class of skin-compatible device with capabilities in on-body sweat collection, rate/volume measurements, and enzymatic biomarker analysis in real-time without any supporting electronics. Here, super-absorbent polymer valves respond at the time of sweat production such that the enzymatic reactions in sweat samples can be compared to those of multiple control samples, triggered simultaneously. Statistical analysis of the reaction kinetics allows reliable extraction of reaction rates and parameters for analysis of ammonia and ethanol in sweat. Ammonia levels, in particular, could serve as an index for hepatic encephalopathy diagnosis in subjects who are experiencing alcohol abuse.15 Studies in healthy volunteers, with sweat induced by warm water bathing, demonstrate the performance and practical utility of these systems.
Results and discussion
Capillary burst valves and superabsorbent polymer pumps for enzymatic analysis of ethanol and ammonia
Fig. 1a shows an exploded view of a microfluidic device designed to collect sweat from the surface of the skin. The layout includes a network of microchannels connected to micro-reservoirs, standard solution wells, and super absorbent polymer (SAP) layers located subjacent to individual standard solution wells. The SAP layer expands upon contact with sweat, thereby serving as an actively triggered mechanical pump that injects pre-loaded standard solution buffers into micro-reservoirs. Fig. S1† shows the SAP swelling phenomena compared to other absorbent pads. The SAP swelling is significantly greater than cellulose and cotton absorbent pads. Enzymatic reagents for ammonia and alcohol, pre-injected within a microfiber matrix and embedded within the micro-reservoirs, make direct contact with reference alcohol and ammonia buffers. Capillary burst valves10 connect the standard solution wells to each micro-reservoir, creating a vent for air to escape as sweat flows into the system.
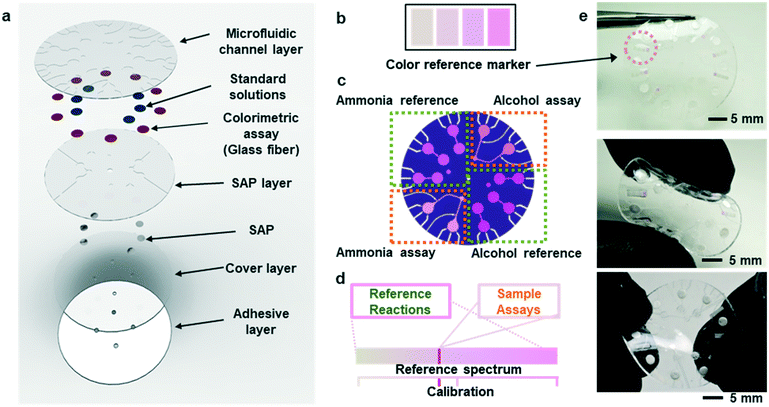 |
| Fig. 1 Schematic illustration and digital images of an epidermal microfluidic device for enzymatic analysis of sweat biomarkers. (a) Schematic drawing of the device in an exploded view format to illustrate the various layers. (b) Color reference marker layer on the top surface. (c) Schematic drawing of the microchannels and colorimetric assays associated with an enzymatic analysis for the concentration of ammonia and ethanol. (d) The scheme for sample assay calibration using a reference reaction. (e) Optical images of a device held with a pair of tweezers and while manually bent and twisted. | |
During normal modes of operation, sweat excreted from the skin pores travels through inlet ports in the microfluidic substrate. One set of inlets connects to the SAPs, which expand upon contact causing standard solution transport and mixing with the reference assays in the micro-reservoirs. A separate set of inlets connect to ammonia and ethanol assays allowing measurement of these species in sweat. The patterns of microchannels, reservoirs, and capillary burst valves allow sequential filling of individual reservoirs before sweat exits the device at a relatively low rate of efflux.
Fig. S2a† illustrates the process by which sweat fills into the device, first through the inlet region, then into the microreservoirs, and eventually out through the capillary burst valves. The passive valves have lateral channel dimensions (50 μm) that are significantly smaller (∼3×) than those of the microchannels leading into the microreservoirs (150 μm). The burst pressure mechanism follows the Laplace–Young equation according to:
|  | (1) |
where
σ is the surface tension of liquid,
θA is the contact angle of the channel,

is the min [
θA +
β; 180°],
β is the diverging angle of the channel,
b and
h are the width and the height of the diverging section, respectively.
10,16 Fig. S2b
† shows designs where ‘Valve#1’ and ‘Valve#2’ have diverging angles of 90° and 120°, respectively. The different bursting pressures of different valves enable precise control of the filling pathways. Glass fiber mats with large pore sizes (Fig. S3a
†) and fast wetting rates (Fig. S3b
†) serve as matrices for enzymes and colorimetric assay reagents. The soft, ultrathin elastomeric construction and multilayered geometry support passive and active modes of operation, with levels of mechanical flexibility and stretchability that facilitate mounting on the skin at various anatomical locations across the body.
Colorimetric analysis of multiplexed enzymatic assays
Fig. S4(a and b)† summarizes the formulations of the enzymatic reactions for the ammonia and ethanol assays (ammonia monooxigenase; AMO, hydroxylamine oxidoreductase; HAO for ammonia and alcohol oxidase; AOx for ethanol). The oxidation reactions for both species generate hydrogen peroxide with horseradish peroxidase (HRP) as a by-product, which in turn, reduces a colorimetric/fluorometric probe (OxiRed™) to form resorufin. The developed color associated with resorufin relates directly to the concentration of ammonia (0–10 mM) and ethanol (0–20 mM) in sweat. The depth of each microreservoir defines the dynamic range of color analysis, as it determines the optical path length according to the Beer–Lambert law. Ammonia and ethanol reference micro-reservoirs (Fig. 1b and c) provide calibration of enzymatic reactions under various conditions (e.g. changes in temperature and pH; Fig. 1d). The depths of the micro-reservoirs and SAP layers are ∼200 μm and ∼300 μm, respectively, to provide sufficient color intensity and contrast across a physiologically relevant range of concentrations, while retaining favorable bending and twisting mechanics for mounting on the skin (Fig. 1e). Color reference bars adjacent to the reaction wells simplify colorimetric image-based analysis of biomarkers in real-time, across various lighting conditions. Fig. 1e shows such reference bars formed in a range of colors that correspond to those expected to result from concentrations of ammonia and ethanol in sweat, as determined by systematic benchtop testing.
Kinetics of the enzymatic reactions
The enzymatic assays consist of a series of chain reactions that oxidize the substrates into H2O2, which in turn, acts as a stimulating agent to yield a colorimetric response. Such reactions generally exhibit a transient time response before the rate of formation of the product reaches its maximum.17,18 Fig. S5† shows the time dependence of color changes of the assays exposed to different concentrations of ammonia and ethanol in artificial sweat perfused through a microfluidic device on the bench. The reaction rate in the initial phase is rapid (25–40 min) and then plateaus at later phases. These temporal changes in color intensity provide important kinetic information, dependent on substrate concentrations.
Controlled reaction conditions with excess reagents and optimized enzyme loading quickly drive the reaction rate to its maximum, consistent with first-order kinetics behavior.19,20 Derivation of the first-order kinetics parameters allows extrapolation of substrate concentrations in situ. Here, the Michaelis–Menten equation captures the behavior of the enzymatic reactions, yielding an expression for the inverse of the reaction rate (1/r) for use in Lineweaver–Burk plots, according to:
| 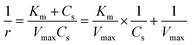 | (2) |
where
Km is the Michaelis constant,
Vmax is maximum reaction rate with original concentration of enzyme, and
Cs is the concentration of substrate (ammonia or ethanol).
21,22 Graphing the data in this manner yields a double-reciprocal plot as a straight line (1/
r vs. 1/
Cs) with a slope and
y-intercept that define
Km/
Vmax and 1/
Vmax, respectively, as shown in
Fig. 2(a and b). This relationship follows from the Lineweaver–Burk equation (
eqn (2)), which defines the initial rate of reaction at various substrate concentrations based on calculation of the color indexes extracted from images. The reactions shown in
Fig. 2(a and b) have slopes (
Km/
Vmax) that depend on substrate concentrations, where the
y-intercept (1/
Vmax) remains nearly constant. The
Km value derived from color analysis and the mean value of
Vmax for ammonia and ethanol serve as the basis for computing their concentrations. Table S1
† summarizes the list of parameters derived in this manner, and
Fig. 2(c and d) shows the
Km calibration curves for these assays.
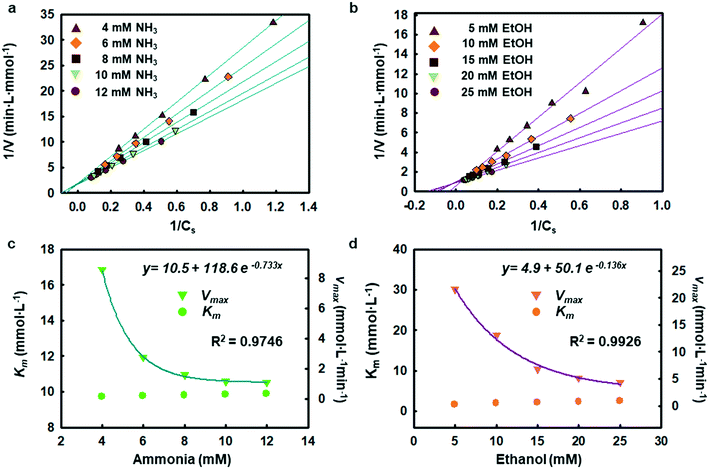 |
| Fig. 2 Quantitative analysis of ammonia and ethanol using enzyme kinetics. (a) Lineweaver–Burk plot of the ammonia assay reaction at different concentrations of ammonia (4 mM, 6 mM, 8 mM, 10 mM, and 12 mM). (b) Lineweaver–Burk plot of the alcohol assay reaction at different ethanol concentrations (5 mM, 10 mM, 15 mM, 20 mM and 25 mM). (c) Km and Vmax of the ammonia assay reaction at different ammonia concentrations. (d) Km and Vmax of alcohol assay reaction at different concentrations of ethanol. | |
Integrated standards and super-absorbent polymer pumps
Although measurement of the time course of color development allows quantification of ammonia and ethanol concentrations in sweat, such a process requires capture and analysis of multiple images at different times. Such operation can be cumbersome in practical, non-laboratory settings. The use of integrated standards with known concentrations, stored in microreservoirs on the device and introduced into assay chambers at the onset of sweating through the use of super-absorbent polymers, provides an alternative. Fig. 3a illustrates a microreservoir filled with pre-loaded standard solutions. As sweat encounters the SAP layer, the polymer expands. This expansion leads to pressure changes and transport of the pre-loaded reagent23 as shown in Fig. 3(b and c). Fig. 3d shows optical images of the SAP in the device, in situ. A reinforcing layer of a photocurable polyurethane or poly(styreneisoprene–styrene)24 helps to reduce evaporation of the buffer reagent through the polydimethylsiloxane (PDMS) layer. A commercial photocured polyurethane (Norland Optical Adhesive, NOA 61) cast with the PDMS above the microreservoir (Fig. S6†) provides this impermeable layer.
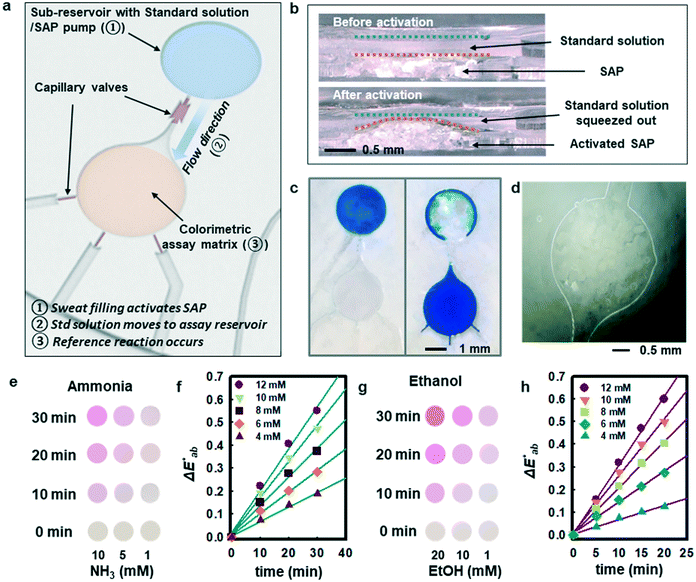 |
| Fig. 3 Quantitative analysis of the concentrations of ammonia and ethanol using reference reservoirs activated by super absorbent polymer pumps. (a) Illustration of the SAP pump structure and location of standard solutions in reference reservoirs. (b) Cross-sectional view of the SAP pump as the pump undergoes mechanical activation. (c) Optical image of the SAP in the device. (d) Optical images showing the mechanism for expansion of the SAP pump. (e) Colorimetric analysis of the ammonia assay as a function of time. (f) Color index plot for the ammonia assay after CIE color normalization. (g) Colorimetric analysis of the ethanol assay as a function of time. (h) Color index plot for the ethanol assay after CIE color normalization. | |
Fig. 3e shows observed colors at three different concentrations of ammonia (1, 5, and 10 mM, respectively). Analysis of the normalized color indexes
highlight quantitative differences in concentration as a function of time (Fig. 3f). Fig. 3(g and h) show similar colorimetric responses across three different concentrations of ethanol (1, 10, and 20 mM, respectively) and their calibration plots. Since the time course of the reference enzymatic assay matches that of the unknown sweat sample and the color changes systematically with concentration, the concentration of the unknown sample can be determined by interpolating between the colors of reference standards at different concentrations.
Benchtop tests using a liquid chromatography-mass spectrometry (LCMS) system (Waters Synapt G2-Si ESI, MA, USA) provide points of comparison to assess the accuracy of these assays. The measurements include ∼100 μL samples of sweat collected using absorbent pads placed on the forehead region of three subjects during rest in a warm water bath (∼48 °C) after consumption of an alcoholic beverage. Fig. S7(a and b)† show the results of LCMS (storage of samples at −20 °C between the time of collection and analysis) and colorimetric enzymatic analysis (samples applied immediately on assay coupons) across 27 measurements for the ammonia assay and 20 for the ethanol assay. Fig. S7† shows concentration analysis results with 95% confidence and accuracy estimates for the colorimetric assays (R2: 0.671 and S: 1.22 for ammonia; R2: 0.884 and S: 2.02 for ethanol; where S is standard error of estimate).
Control of enzymatic reaction kinetics
Chemical reactions catalyzed by enzymes exhibit reaction rates that depend on various factors such as the concentration of product and substrate, the pH, and the temperature. Statistical optimization and modelling techniques allow for characterization of enzymatic reaction times and the effects of different variables.25,26 Tables S2 and S3† capture the response to changes in enzyme loading (X1), reaction temperature (X2), pH (X3), and salt concentration (X4). Fig. S8† shows how the ratio of AOx and HRP affects the reactivity of the alcohol assay. Similarly, the ammonia assay relies on enzyme cocktails, characterized using loading tests and simulations.
Tables S4 and S5† summarize the fitting results and analysis of variance (ANOVA) using the data shown in Table S2.† The findings indicate that both ammonia and alcohol reaction rates are relatively insensitive to pH and salt concentration (pH 6–8 and 40–100 mM sodium chloride) with low F-values (Table S5†).27,28 The reaction rates depend mostly on enzyme loading and temperature. The contour plots in Fig. S9 and S10 and Table S6† highlight the competing interactions between the different factors and identify an optimal set of parameters (∼14.5% v/v enzyme loading, 32–38 °C temperature 6.5–7.8 pH and 40–80 mM chloride concentration for ammonia and ∼23 mg mL−1 enzyme loading, 38–39 °C temperature, pH 6–7, and 40–80 mM chloride concentration for alcohol). This statistical analysis defines the enzyme loading and relevant reaction conditions needed for analysis of sweat flowing through the microfluidic device structures.
Multiplexed, colorimetric, and enzymatic analysis of sweat in human subject studies
Field testing on three healthy volunteers demonstrates the performance of ammonia and ethanol sweat assays in response to sweating induced passively in a warm water bath (∼48 °C; Fig. S11a†). Each subject wore a sweat microfluidic device on their forehead where the sweating is abundant, while being immersed in a warm bath. The measurements were recorded within 1 h after alcohol consumption, as ethanol levels tend to decrease after 1–1.5 h of intake (Fig. S11b†).29Fig. 4 shows results of sweat ammonia and ethanol concentrations determined based on colorimetric measurements and kinetic calculations, with comparisons to conventional laboratory analysis (LCMS; Waters Synapt G2-Si ESI, MA, USA) using separately collected samples of sweat. Fig. 4(a, c and e) highlights colorimetric analysis results for sweat ammonia concentrations that rely on reference calibration curves shown in Fig. S12† as obtained from reference chambers for subject #1 (5.0 ± 0.6 mM and 4.6 ± 0.3 mM), subject #2 (5.6 ± 0.2 mM and 9.6 ± 0.3 mM), and subject #3 (3.1 ± 0.4 mM and 3.8 ± 0.1 mM) with and without alcohol consumption, respectively. Kinetics estimates in Fig. 4(a, c and e) using color indexes at different snapshots in time for subject #1 (5.9 mM and 9.0 mM), subject #2 (4.9 mM and 10.3 mM), and subject #3 (3.3 mM and 5.5 mM) support the colorimetric ammonia measurement results. The benchtop LCMS results for subject #1 (5.2 ± 0.2 mM and 6.2 ± 0.1 mM), subject #2 (6.1 ± 0.3 mM and 8.8 ± 0.3 mM), subject #3 (3.7 ± 0.2 mM and 6.4 ± 0.3 mM) are comparable to the colorimetric and kinetic measurements. Ammonia is meta-stable in biofluids leading to the formation of ammonium,31 which could account for some of the scatter across the different measurement techniques. Fig. 4(b, d and f) shows colorimetric measurements of sweat ethanol across subject #1 (2.6 ± 0.1 mM), subject #2 (6.27 ± 0.02 mM), and subject #3 (3.0 ± 0.1 mM) after alcoholic beverage consumption. Kinetic estimates for these three subjects (subject #1: 2.7 mM, subject #2: 7.3 mM, subject #3: 3.2 mM) have similar trends as the colorimetric analysis. The colorimetric and kinetic results are both comparable to benchtop analysis of ethanol (3.25 ± 0.05 mM, 8.5 ± 0.3 mM and 5.66 ± 0.02 mM). In contrast, sweat measurements taken without alcohol ingestion show only trace amounts of ethanol (subject #1: 0.05 ± 0.004 mM, subject #2: 0.03 ± 0.03 mM, subject #3: 0.05 ± 0.03 mM), consistent with previous sweat and blood-based studies.12,29,30 Benchtop tests in Fig. S7† show similar trends for ammonia and ethanol measurements. The results may have statistical limitation due to the accuracy of the colorimetric analysis, which relies on the two duplication reservoirs.
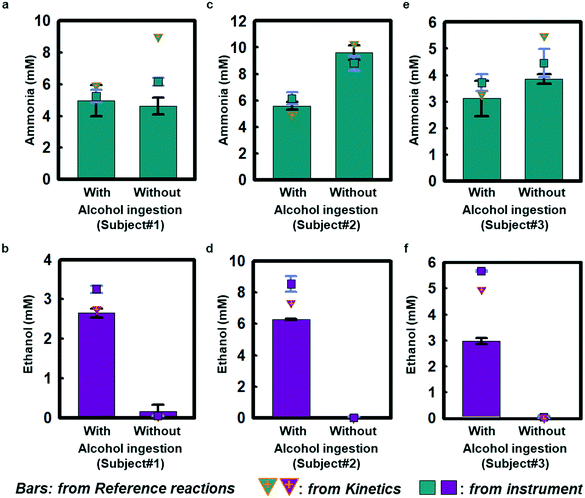 |
| Fig. 4 Human study and calculation of kinetics. Ammonia and ethanol results for subject #1 (a and b), #2 (c and d) and #3 (e and f), respectively, based on reference color development, kinetics calculation and instrument analysis (liquid chromatography; LC-MS; Waters Synapt G2-Si ESI, MA, USA) of ammonia and ethanol assays under conditions of with/without ingestion of alcoholic beverage. | |
Taken together, these findings indicate that sweat ethanol concentrations change significantly with alcohol consumption. In contrast, sweat ammonia concentrations do not change significantly with alcohol consumption under normal conditions.32 Sweat ammonia concentration could be affected by other factors, including fatigue, exercise activity, and heavy alcohol consumption in subjects with abnormal liver function.33–35 Furthermore, alcohol abuse may cause an increase in ammonia level and give rise to the accumulation of ammonia in the liver, whereby hepatic encephalopathy could occur.15 Although alcohol intake can cause vasodilation and, in some cases, small associated changes in sweat rate,36 such effects were not explored in this study and are still being investigated.32,37
Conclusions
The integration of multiplexed, rate-dependent enzymatic assays into multi-layered soft microfluidic devices creates many additional possibilities for real-time analysis of sweat biomarkers beyond those previously reported. Pre-loaded standard solutions corresponding to physiological concentrations of ammonia and ethanol serve as integrated time-matched references, allowing for simple and reliable mode of operation. This approach and associated microfluidic device designs yield key temporal features of enzymatic reactions over physiological concentrations, enabling in situ deployment in the field, with direct implications for sweat biomarker research, health monitoring at home, and drug/alcohol testing in daily living.
Experimental
Fabrication of components for soft microfluidic systems
Fabrication of the molds began with photolithographically defined patterns of photoresist (photoresist, KMPR1010, MicroChem, Westborough, MA) formed by spin casting at 3000 rpm for 30 s on silicon wafers, followed by baking on a hot plate at 110 °C for 3 min, UV irradiance for 300 mJ cm−1, exposure to developer (MF917) for 2 min. Deep-reactive-ion etching (STS Pegasus ICP-DRIE, SPTS Technologies Ltd, UK) formed relief structures in the silicon to depths of 200 ± 10 μm and 300 ± 10 μm for the microchannel/micro-reservoir layer and the SAP pump layer, respectively. Layers of polymethylmethacrylate (PMMA; 3000 rpm for 30 s, curing at 180 °C for 10 min) spin cast on these molds facilitated release of PDMS after casting and curing. Soft lithographic processing formed PDMS (Sylgard 184, Dow Corning, Midlan, MI) microfluidic substrates (20
:
1 PDMS in an oven at 70 °C for 4 h) from a channel layer, formed by spin casting at 250 rpm for 30 s (∼500 μm) and curing on the mold, a valve layer by spin casting at 400 rpm for 30 s on a different mold (∼300 μm) and a cover layer by spin casting at 400 rpm for 30 s (∼300 μm) on an unprocessed silicon wafer.
Preparation of enzymatic bioassays
Enzyme cocktails for the alcohol assay were prepared by mixing 70% v/v alcohol oxidase (AOx; 23 mg mL−1) from Candida boidinii and 30% v/v horse radish peroxidase (HRP; 23 mg mL−1; Sigma Aldrich, St. Louis, MO). An optimized ratio of ∼14.5% (v/v; mixed in 0.1 sodium phosphate buffer, pH 7.0) of a commercial enzyme cocktail for the ammonia assay included ammonia monooxigenase (AMO), hydroxylamine oxidoreductase (HAO), β-nicotinamide adenine dinucleotide 2′-phosphate (NADPH) oxidase (NOx), superoxide dismutase (SD) and horseradish peroxidase (HRP; BioVision Inc., Milpitas, CA). Response surface methodology (RSM) determined the enzyme loading amount. Glass fiber sheets (Advanced Microdevices, Ambala Cantt, India) served as supporting matrices for the assays, hole-punched into circular shapes with diameters of ∼2.5 mm. Small volumes of enzyme solutions (∼2 μL) were placed onto each piece of glass fiber and dried in an oven at 30 °C for 2 h to complete the active sensing platforms. OxiRed™ (BioVision Inc.) served as the color development agent for the alcohol assay, dispensed into each reservoir (volume ∼1 mL), followed by drying in a desiccator at room temperature for 4 h.
Preparation of the SAP material
Sodium polycarbonate served as the super absorbent polymer. Addition of 55% KOH to glacial acrylic acid monomer (Sigma-Aldrich, St. Louis, MO) neutralized a solution that included 2 mL ammonium sulfate (37.5 g L−1) and 4 g sodium bicarbonate. Mixing 2 mL of N,N′-methylenebisacrylamide as a fast-swelling agent (Sigma-Aldrich, St. Louis, MO), with 2 mL of sodium metabisulfite (31.5 g L−1) as a cross linker increased the viscosity necessary for gelation. The gel was spread evenly over a tray and allowed to dry at 70 °C for 24 h. The resulting gel-like material was ground using a mortar and then passed through a no. 120 sieve (100 μm mesh size) to yield the final SAP material.
Fabrication and packaging of the microfluidic device
The sweat microfluidic devices consisted of soft microfluidic structures, glass fiber assay coupons, SAP materials and standard solutions of NH4Cl and ethanol (1, 5, 10 mM of NH4Cl and 1, 10, 20 mM of ethanol; Sigma-Aldrich, St. Louis, MO). Both the SAP material and assay coupon were manually loaded onto the locations of the valves and reservoirs, respectively. Bonding of the channel layers, valve layers and cover layers occurred via contact after wetting the surfaces with uncured PDMS. Pre-loading of standard solutions was manually conducted using a 30-gauge (∼300 μm outer diameter) blunt needle and a 1 mL syringe after device assembly (Fig. S13†), directly through the channel structure of the PDMS microfluidics. A double-sided medical adhesive layer (Scapa Soft-Pro, Scapa Healthcare, Windsor, CT) with an opening at the position of an inlet port on the bottom side of the microfluidic platform served as an interface layer to join the device with the skin.
Enzyme kinetics
The oxidation of ammonia (ammonium) based on reactions shown in Fig. S4† produced hydrogen peroxide, which subsequently reduced OxiRed to resorufin. The colorimetric assay for alcohol relied on reactions associated with this oxidation and color development by means of AOx and HRP. Because the reagents were in excess, the amounts of the substrates (ammonia and ethanol) determined the reaction rates and therefore the color intensities directly.
Color assay and normalization
CIE (Commission Internationale de l'Eclairage) color normalization allowed assessment of color index from RGB values extracted from digital images of the reaction reservoirs using commercial software (Adobe Photoshop). The white back cover layer of the device served a white reference, and yielded CIE L*a*b* values.21,38 The following equation defined the color differences |  | (3) |
where
,
, and
denote the values at the nth assay location and
,
, and
are values for the white reference region.
Instrumental analysis
A liquid chromatography-mass spectrometry system (LC-MS; Waters Synapt G2-Si ESI, MA, USA) provided assays for ammonia and ethanol samples. Waters ACQUITY UPLC BEH C18 column (130 Å, 1.7 μm, 2.1 mm × 50 mm) served as the ammonia separation column at a 0.2 mL min−1 flow rate of eluents that included solvent A (5% v/v acetonitrile, and 0.1% v/v formic acid) and solvent B (95% acetonitrile and 0.1% formic acid). An Aminex HPX-87H ion exclusion column (Bio-Rad, Hercules, CA) and a refractive index detector enabled evaluations of ethanol at a 0.8 mL min−1 flow rate of 0.005 N H2SO4 eluent and a 50 °C column temperature. The total sample volume was 100 μL, with at least 1 μL of sweat. Here, color analyses of liquid samples were conducted with a Varian Cary5G UV-vis-NIR spectrophotometer (Agilent, Santa Clara, CA).
Field testing of device on healthy volunteers
Field tests were conducted on three healthy subject volunteers with due process of law. All subjects were informed and provided signed consent. Evaluations involved sweat induction at rest in a warm water bath (∼48 °C; Fig. 4a) for 40 min with/without alcohol ingestion.27 The subjects consumed approximately 20 mmol per kg body weight of ethanol (alcohol beverage at ∼15% alcohol content) within 30 min without food and water intake. Prior to mounting the devices, the skin was cleaned with soapy water, rinsed, and dried thoroughly.
Conflicts of interest
There are no conflicts to declare.
Acknowledgements
SBK, JK, JY contributed equally to this work. CP acknowledges National Research Foundation (NRF) of Ministry of Science in Information Technology (MSIT), South Korea government supported this work (The Bio & Medical Technology Development Program; NRF-2018M3A9H3020459). This work utilized Northwestern University Micro/Nano Fabrication Facility (NUFAB), which is partially supported by Soft and Hybrid Nanotechnology Experimental (SHyNE) Resource (NSF ECCS-1542205), the Materials Research Science and Engineering Center (DMR-1720139), the State of Illinois, and Northwestern University.
Notes and references
- K. Sato, Rev. Physiol., Biochem. Pharmacol., 1977, 79, 51–131 CrossRef CAS PubMed.
- A. P. Jones, L. M. C. Webb, A. O. Anderson, E. J. Leonardo and A. Rot, J. Leukocyte Biol., 1995, 57, 434–437 CrossRef CAS PubMed.
- G. Cizza, A. H. Marques, F. Eskandari, I. C. Christie, S. Torvik, M. N. Silverman, T. M. Phillips and E. N. Sternberg, Biol. Psychiatry, 2008, 64, 907–911 CrossRef CAS PubMed.
- A. Mena-Bravo and L. de Castro, J. Pharm. Biomed. Anal., 2014, 90, 139–147 CrossRef CAS PubMed.
- M. D. Hladek, S. L. Szanton, Y. E. Cho, C. Lai, C. Sacko, L. Roberts and J. Gill, J. Pharm. Biomed. Anal., 2018, 454, 1–5 CAS.
- W. Gao, S. Emaminejad, H. Y. Y. Nyein, S. Challa, K. Chen, A. Peck, H. M. Fahad, H. Ota, H. Shiraki, D. Kiriya, D. Lien, G. A. Brooks, R. W. Davis and A. Javey, Nature, 2016, 529, 509 CrossRef CAS PubMed.
- H. Y. Y. Nyein, W. Gao, Z. Shahpa, S. Emaminejad, S. Challa, K. Chen, H. M. Fahad, L. Tai, H. Ota, R. W. Davis and A. Javey, ACS Nano, 2016, 10, 7216 CrossRef CAS PubMed.
- A. Koh, D. Kang, Y. Xue, S. Lee, R. M. Pielak, J. Kim, T. Hwang, S. Min, A. Banks, P. Bastien, M. C. Manco, L. Wang, K. R. Ammann, K. I. Jang, P. Won, S. Han, R. Ghaffari, U. Paik, M. J. Slepain, G. Balooch, Y. Huang and J. A. Rogers, Sci. Transl. Med., 2016, 8, 366ra165 CrossRef PubMed.
- A. J. Bandodkar and J. Wang, Trends Biotechnol., 2014, 32, 363 CrossRef CAS PubMed.
- J. Choi, D. Kang, S. Han, S. B. Kim and J. A. Rogers, Adv. Healthcare Mater., 2017, 6, 1601355 CrossRef PubMed.
- Y. Sekine, S. B. Kim, Y. Zhang, A. J. Bandodkar, S. Xu, J. Choi, M. Irie, T. R. Ray, P. Kohli, N. Kozai, T. Sugita, Y. Wu, K. Lee, K. T. Kee, R. Ghaffari and J. A. Rogers, Lab Chip, 2018, 18, 2178–2186 RSC.
- A. J. Bandodkar, P. Gutruf, J. Choi, K. Lee, Y. Sekine, J. T. Reeder, W. J. Jeang, A. J. Aranyosi, S. P. Lee, J. B. Model, R. Ghaffari, C. J. Su, J. P. Leshock, T. Ray, A. Verrillo, K. Thomas, V. Krishnamurthi, S. Han, J. Kim, S. Krishnan, T. Hang and J. A. Rogers, Sci. Adv., 2019, 5, eaav3294 CrossRef PubMed.
- A. Hauke, P. Simmers, Y. R. Ojha, B. D. Cameron, R. Ballweg, T. Zhang, N. Twine, M. Brothers, E. Gomez and J. Heikenfeld, Lab Chip, 2018, 18, 3750–3759 RSC.
- L. C. Tai, W. Gao, M. Chao, M. Bariya, Q. P. Ngo, Z. Shahpar, H. Y. Y. Nyein, H. Park, J. Sun, Y. Jung, E. Wu, H. M. Fahad, D. H. Lien, H. Ota, G. Cho and A. Javey, Adv. Mater., 2018, 30, 1707442 CrossRef PubMed.
- Y. Jung and K. Namkoong, Handb. Clin. Neurol., 2014, 125, 115–121 Search PubMed.
- H. Cho, H. Y. Kim, J. Y. Kang and T. S. Kim, J. Colloid Interface Sci., 2007, 306, 379 CrossRef CAS PubMed.
- J. S. Easterby, Biochim. Biophys. Acta, 1973, 293, 552–558 CrossRef CAS.
- W. W. Cleland, Anal. Biochem., 1979, 99, 142–145 CrossRef CAS PubMed.
- S. Schnell and C. Mendoza, Biophys. Chem., 2004, 107, 165–174 CrossRef CAS PubMed.
- M. G. Pedersen and A. M. Bersani, J. Math. Biol., 2010, 60, 267–283 CrossRef PubMed.
- S. B. Kim, Y. Zhang, S. M. Won, A. J. Bandodkar, Y. Sekine, Y. G. Xue, J. Koo, S. W. Harshman, J. A. Martin, J. M. Park, T. R. Ray, K. E. Crawford, K. T. Lee, J. Choi, R. L. Pitsch, C. C. Grigsby, A. J. Strang, Y. Y. Chen, S. Xu, J. Kim, A. Koh, J. S. Ha, Y. G. Huang, S. W. Kim and J. A. Rogers, Small, 2018, 14, 1703334 CrossRef PubMed.
- R. P. Lana, R. H. T. B. Goes, L. M. Moreira, A. B. Mâncio, D. M. Fonseca and L. O. Tedeschi, Livest. Prod. Sci., 2005, 98, 219–224 CrossRef.
- B. K. Hamilton, C. R. Gardner and C. K. Colton, AIChE J., 1974, 20, 503–510 CrossRef CAS.
- J. T. Reeder, J. Choi, Y. Xue, P. Gutruf, J. Hanson, M. Liu, T. Ray, A. J. Bondodkar, R. Avila, W. Xia, S. Krishnan, S. Xu, K. Barnes, M. Pahnke, R. Ghaffari, Y. Huang and J. A. Rogers, Sci. Adv., 2019, 5, eaau6356 CrossRef PubMed.
- S. B. Kim, S. J. Lee, J. H. Lee, Y. R. Jung, L. P. Thapa, J. S. Kim, Y. Um, C. Park and S. W. Kim, Biotechnol. Biofuels, 2013, 6, 109 CrossRef CAS PubMed.
- Y. Lee, S. B. Kim, T. Lee, M. Jang, A. Shin, S. J. Park, J. R. Kim, Y. E. Choi, J. G. Na and C. Park, J. Cleaner Prod., 2019, 218, 985–992 CrossRef CAS.
- L. Y. Stein and D. J. Arp, Appl. Environ. Microbiol., 1998, 64, 4098–4102 CAS.
- T. Kondo, Y. Morikawa and N. Hayashi, Appl. Microbiol. Biotechnol., 2008, 77, 995–1002 CrossRef CAS PubMed.
- M. J. Buono, Exp. Physiol., 1999, 84, 401–404 CrossRef CAS PubMed.
- B. Lansdorp, W. Ramsay, R. Hamid and E. Strenk, Sensors, 2019, 19, 2380 CrossRef PubMed.
- J. R. Huizenga, A. Tangerman and C. H. Gips, Ann. Clin. Biochem., 1994, 31, 529–543 CrossRef CAS PubMed.
- F. Meyer, O. Laitano, O. Bar-Or, D. McDougall and G. J. F. Heigenhauser, Braz. J. Med. Biol. Res., 2007, 40, 135–143 CrossRef CAS PubMed.
- S. Kant, G. Davuluri, K. A. Alchirazi, N. Welch, C. Heit, A. Kumar, M. Gangadhariah, A. Kim, M. R. McMullen, B. Willard, D. S. Luse, L. E. Nagy, V. Vasiliou, A. M. Marini, D. Weiner and S. Dasarathy, J. Biol. Chem., 2019, 294, 7231–7244 CrossRef CAS PubMed.
- A. M. Dawson, Gut, 1978, 19, 504–509 CrossRef CAS.
- D. J. Willkinson, N. J. Smeeton and P. W. Watt, Prog. Neurobiol., 2010, 91, 200–219 CrossRef PubMed.
- T. Yoda, L. I. Crawshaw, M. Nakamura, K. Saito, A. Konishi, K. Nagashima, S. Uchida and K. Kanosue, Alcohol, 2005, 36, 195–200 CrossRef CAS PubMed.
- S. W. Brusilow and E. H. Gordes, Am. J. Physiol., 1968, 214, 513–517 CrossRef CAS PubMed.
- H. Araki, J. Kim, S. Zhang, A. Banks, K. E. Crawford, X. Sheng, P. Gutruf, Y. Shi, R. M. Pielak and J. A. Rogers, Adv. Funct. Mater., 2017, 27, 1604465 CrossRef.
Footnotes |
† Electronic supplementary information (ESI) available. See DOI: 10.1039/c9lc01045a |
‡ SBK, JK, JY contributed equally to this work. |
|
This journal is © The Royal Society of Chemistry 2020 |