DOI:
10.1039/C0AY00302F
(Paper)
Anal. Methods, 2010,
2, 1263-1269
Received
7th May 2010
, Accepted 25th May 2010
First published on
8th July 2010
Abstract
A new method for simultaneous determination of dopamine (DA) and ascorbic acid (ASC) is proposed. The method is based on the reaction of dopamine and ascorbic acid with the oxidizing agent (silver nitrate) in the presence of PVP (as a stabilizer) and the formation of silver nanoparticles in a slightly basic medium. Spectrophotometry is used to monitor the changes of the surface plasmon resonance (SPR) band at a maximum wavelength of silver nanoparticles (440 nm) vs. time. Three-layered feed-forward artificial neural networks (ANN) trained by back propagation learning algorithm is used to model the relationship between absorbance and concentration to quantify analyte in mixtures under optimum conditions. Sigmoidal and linear transfer functions were used in the hidden and output layers, respectively. Linear calibration graphs were obtained in the concentration range of (3.2 × 10−6 – 2.0 × 10−5 mol L−1) for DA and (2.0 × 10−6 – 4.8 × 10−5mol L−1) for ASC. The detection limits were 8.0 × 10−7 and 6.2 × 10−7 mol L−1 for DA and ASC, respectively, which are comparable by different spectrophotometric and colorimetric methods. The method was applied successfully to the determination of dopamine (DA) and ascorbic acid in human serum samples.
1. Introduction
Dopamine (DA) chemically known as 4-(2-aminoethyl) benzene-1,2-diol, is one of the crucial catecholamine neurotransmitter molecules distributed in brain tissue.1,2 It plays an important role in regulating activity of hormonal, cardiovascular, renal and central nervous systems.3–5 Thus, shortage of DA, particularly the death of DA neurons in the nigrostriatal pathway is a cause of Parkinson's disease, in which a person loses the ability to execute smooth and controlled movements.6 Similarly, ascorbic acid (ASC), a water-soluble vitamin widely required for metabolism and consumed on a large scale, is electroactive and has been studied extensively.7–10 ASC has been used for the prevention and treatment of the common cold, mental illness, infertility, cancer and AIDS (Arrigoni and Tullio11). It is present in many biological systems11 and multivitamin preparations, which are commonly used to supplement inadequate dietary intake. Also, it is widely used in foods as an antioxidant for the stabilization of color and aroma with subsequent extension of the storage life of the products. Thus, the determination of ASC content is particularly important in the pharmaceutical and food industry. Since these two species usually coexist in real biological matrices, the development of a selective and sensitive method for simultaneous determination of DA and ASC is highly desirable for analytical applications and diagnostic research.
Kinetic methods based on difference in reaction rates are effective ways for simultaneous determination of mixtures especially in cases where the analytes react with a common reagent and create similar or identical spectra that cannot be resolved by equilibrium-based methods.12–16 These methods have been widely used for the simultaneous determination of multi-component mixtures due to the availability of computerized data acquisition systems and the development of powerful mathematical treatments for processing the recorded information. Principal component regression (PCR) and partial least squares regression (PLS) which are well-known multivariate calibration procedures are widely used in recent years for the simultaneous determination of analytes in mixtures by means of kinetic-spectrophotometric procedures.17–20 Also, chemometric methods based on artificial neural networks (ANN)21,22 have found increasing applications for multicomponent kinetic determinations. Use of analytical methods combined with multivariate calibration can be considered a promising, faster, direct and relatively less expensive alternative for multicomponent analysis of mixtures.23
Silver and gold nanoparticles have been the subject of intensive research because of their unique and tunable surface plasmon resonance (SPR) properties.24–26 When dispersed in liquid media, these nanoparticles exhibit a strong UV-Vis extinction band that is not present in the spectrum of the bulk metal. This extinction band results when the incident photon frequency is resonant with the collective excitation of the conduction electrons and is known as the surface plasmon resonance (SPR).26,27 SPR excitation results in wavelength-selective absorption with extremely large molar extinction coefficients (3 × 1011 mol L−1 cm−1).28 It is well established that the peak wavelength, λmax, and intensity of the SPR spectrum is dependent upon the size, shape and interparticle spacing of the nanoparticle as well as its own dielectric properties and those of its local environment including the substrate, solvent and adsorbates.24,29,30 For these reasons, gold nanoparticles (Au NPs) have attracted much attention as a unique and advantageous platform for highly sensitive colorimetric detection. Recently colorimetric nanoprobes have been developed for sensitive and selective detection of alkali metal ions,31,32 fluoride ion,33 heavy metal ions,34 lectine,35 neurotransmitters,36 thiol-containing amino acids37 and DNA.38 Also, silver nanoparticles (AgNPs) have found wide applications for analytical purposes owing to their unique optical properties in the field of surface plasmon resonance spectroscopy, colorimetric detection and scattering detection in recent years.39,40
In this work a new method for simultaneous determination of the DA and ASC is proposed. The method is based on the reaction of dopamine and ascorbic acid with an oxidizing agent (silver nitrate) in the presence of PVP and preparation of silver nanoparticles in slightly basic medium and spectrophotometrically monitoring the changes of SPR band at maximum wavelength of silver nanoparticles (440 nm) vs. time. Three-layer ANN with back-propagation of error algorithm for the model quantify the considered analyte in mixtures under optimum conditions. The method was applied to simultaneous determination of the DA and ASC in their mixtures and satisfactory results were obtained.
2. Experimental
2.1 Apparatus and software
The UV-Vis absorbance spectra were recorded on a PerkinElmer (Lambda25) spectrophotometer with a 1.0 cm glass cell. Measurements of pH were made with a Denver Instrument Model 270 pH meter equipped with a Metrohm glass electrode. The size of the Ag-NPs were characterized by transmission electron microscopy (TEM) using a Zeiss transmission electron microscope operated at an accelerating voltage of 80 kV. The ANN program was performed with Winnn32 software running under Windows NT. The Principal Component Analysis (PCA) was done using MATLAB for windows (Mathworks, version 6.1).
2.2 Chemicals and reagents
All chemicals used were of analytical reagent grade and the solutions were prepared with deionized water. Dopamine (DA), ascorbic acid (ASC), silver nitrate and polyvinylpyrrolidone (PVP) by average mol wt 10,000 were purchased from Merck. All other common laboratory chemicals were of the best grade available and were used without further purification. All solutions were used within 1 h after preparation, and the experiments were performed at ambient temperature (25 ± 2 °C). Stock solutions of AgNO3 (0.01 mol L−1) were prepared by dissolving 0.085 g AgNO3 in deionized water and diluting to 50 ml. A stock solution of polyvinylpyrrolidone (PVP) (0.4 g L−1) was prepared daily by dissolving 0.01 g of PVP in water and diluting to 25 ml. Fresh 0.05 mol L−1 solution of DA and ASC were prepared daily by dissolving the reagent in deionized water.
2.3 General procedure
In 5 ml volumetric flasks, 1 ml of AgNO3 0.01 M, 0.7 ml of PVP 0.4 (g L−1), different concentrations of the catecholamines and 1 ml of NaOH 0.001M were added, respectively, to obtain a reasonable solution. Then this solution was mixed slowly and transferred into a 1 cm spectrophotometric cell to record the absorbance vs. time. It should be noted that the order of the addition of the reagents is very important. Alteration of the order of reagent addition caused the silver nanoparticles to be not stabilized by PVP and caused a decrease in colloidal stability and rapid formation of silver or silver oxide precipitate. The absorbances were measured at 440 nm that is λmax of silver nanoparticles surface plasmon resonance peak at this condition, against a reagent blank. In this study binary mixtures of the dopamine and ascorbic acid were analyzed. In Table 1, the concentrations of standard solutions in these mixtures of the analytes are presented. The concentrations of DA and ASC in the mixtures were in the micromolar range and selected randomly. In order to evaluate the performance of the ANN model, 12 standard solutions were considered in the prediction set as shown in Table 2 and in order to complete our investigation of the performance of the optimized model, the proposed ANN model was used to predict the concentration of 8 standard solutions in the validation set.
Table 1 Concentrations of DA and ACS in standard solutions of calibration set
Sample |
DA/μM |
AA/μM |
Sample |
DA/μM |
AA/μM |
1 |
4.0 |
20.0 |
16 |
16.0 |
20.0 |
2 |
4.0 |
40.8 |
17 |
4.8 |
3.6 |
3 |
2.4 |
20.0 |
18 |
8.0 |
3.6 |
4 |
2.4 |
40.8 |
19 |
8.0 |
44.0 |
5 |
5.6 |
20.0 |
20 |
1.6 |
4.0 |
6 |
5.6 |
40.8 |
21 |
6.4 |
4.0 |
7 |
7.2 |
20.0 |
22 |
9.6 |
4.0 |
8 |
7.2 |
40.8 |
23 |
6.4 |
40.0 |
9 |
1.1 |
20.0 |
24 |
8.8 |
40.0 |
10 |
1.1 |
40.8 |
25 |
8.8 |
16.0 |
11 |
4.8 |
40.8 |
26 |
9.6 |
16.0 |
12 |
8.0 |
10.2 |
27 |
12.8 |
4.0 |
13 |
1.6 |
10.2 |
28 |
4.8 |
4.0 |
14 |
8.0 |
8.0 |
29 |
4.8 |
20.0 |
15 |
8.0 |
20.0 |
30 |
6.4 |
8.0 |
Table 2 Concentrations of DA and ACS in standard solutions of prediction set
Sample |
DA/μM |
AA/μM |
1 |
2.4 |
32.0 |
2 |
7.2 |
32.0 |
3 |
8.8 |
32.0 |
4 |
4.8 |
8.0 |
5 |
16.0 |
8.0 |
6 |
5.2 |
20.0 |
7 |
16.0 |
16.0 |
8 |
4.8 |
44.0 |
9 |
8.8 |
4.0 |
10 |
4.0 |
16.0 |
11 |
4.8 |
1.2 |
12 |
5.6 |
4.0 |
2.4 Data processing
The kinetic data were processed by ANN, which was trained with the back-propagation algorithm. In this work, the SPR absorbance data vs. time were treated with ANN, and the output of the network was the calculated concentrations related to the SPR absorbance data. The structure of the network is comprised of three node layers: an input layer, a hidden layer and output layer. Since the large number of nodes in the input layer of the network (i.e. the number of absorbance readings for each solution) increases the CPU time for ANN modeling, the data matrix was factor analyzed before its introduction into the network and the PC-ANN model was run.41 Consider that the data matrix D has a dimension of n × m, where n and m are the number of standard solutions and the number of absorbance readings for each solution, respectively. The score and loading of this matrix were calculated by the singular value decomposition (SVD). Through the ANN modeling, the numbers of input nodes (i.e. the number of principal components f) and the number of nodes in the hidden layer were optimized. The weights of hidden and output layers were adjusted by back-propagation of error algorithm through an iterative procedure until a minimum value for standard error (S.E.) of prediction was obtained. The momentum and learning rate of the network were also optimized for minimum S.E an also during training (calibration) of the network, the weights are iteratively calculated in order to minimize the sum of the squared difference between the known concentration and the calculated concentration. Overfitting is avoided by using two sample sets; thus, weights are calculated from a training set while the concentration of another sample set (the prediction set) is being simultaneously predicted.
3. Result and discussion
3.1 Preliminary study of the system
Nanoparticles made of silver and gold have been the focus of research for many decades as a result of their intriguing optical properties. The systems in this study consist of an aqueous AgNO3 solution that includes polyvinylpyrrolidone (PVP), as stabilizer, in an alkaline medium. DA and ASC act as effective reducing agents for reduction of silver ions (Ag+) to the Ag-NPs without adding seeds (Scheme 1). In the absence of reducing agents, there is no absorption peak in visible region (380–700 nm). Upon addition of analyte which acts as a reducing agent silver ions are reduced to silver nanoparticles, then the absorbance characteristic to the plasmon of the Ag-NPs is observed. Fig. 1 shows the absorption spectra of the Ag nanoparticles plasmon that was produced by the DA analyte against reagent blank. TEM images show the as prepared Ag-NPs are highly dispersed and uniform in aqueous solution, with an average diameter about 10 nm (Fig. 2). Fig. 3 shows change of absorbance vs. time for the reaction of DA and ASC with silver ions. As it is obvious the reaction of ASC with silver ions is faster than it is with DA and is almost completed within 30 min.
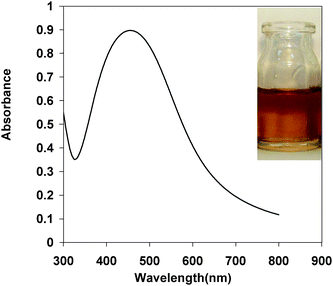 |
| Fig. 1 Absorbance spectra of Ag-NPs formed by DA under the optimum conditions. (The inset is an optical image of Ag-NPs formed by 1.0 × 10−5 M concentration of DA). | |
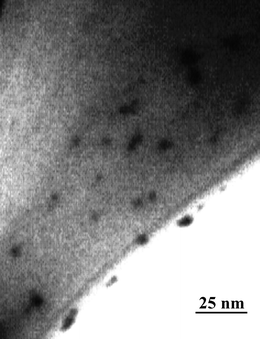 |
| Fig. 2 TEM images of Ag NPs formed by 1.0 × 10−5 M of DA. | |
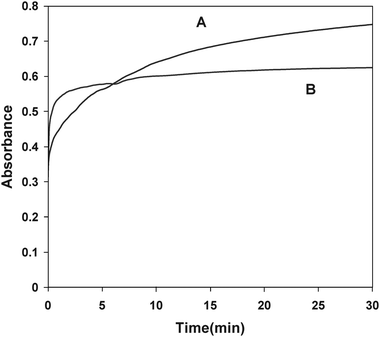 |
| Fig. 3 Kinetic curves corresponding to the absorbance changes of the DA and ASC stimulated growth of Ag-NPs. Conditions; AgNO3 (2 × 10−3 M), PVP (0.06 g L−1), NaOH (0.2 mM) ASC (A) and DA (B). | |
3.2 Effect of stabilizer type and concentration
Protective agents or stabilizers play a crucial role in stabilizing the nanoparticle colloidal metals from agglomeration according to the two basic modes: electrostatic and steric stabilization.42 Electrostatic stabilization is caused by the Coulombic repulsion between particles, caused by the electrical double layer formed by ions adsorbed at the particle surface (e.g., sodium citrate) and the corresponding counter ions. Steric stabilization is achieved because of the coordination of sterically demanding organic molecules and polymers that act as protective shields on the metallic surface (e.g. PVP).43 In this study we selected PVP and sodium citrate as stabilizers for preventing silver nanoparticles agglomeration. Further studies show that sensitivity and linear range of analytes were better when we used PVP compared to sodium citrate. Since H+ was produced from the reaction between silver ions and analytes, consumption of H+ can promote silver ion reduction. When PVP was introduced, it would coordinate with Ag+ and H+ which can produce complex compounds, Ag(PVP)+ and H(PVP)+ then Ag+ and H+ were stabilized.44 The stabilization of Ag+ reserved the silver nanoparticles formation reaction, but the stabilization of H+ facilitated it. The latter affection may be stronger than the former one, and the reaction was accelerated. The variation of absorbance at 440 nm that is λmax surface plasmon peak of the Ag-NPs as a function of the concentration of PVP is shown in Fig. 4. The results show that the maximum intensity was obtained at 0.06 g L−1 PVP for different analytes.
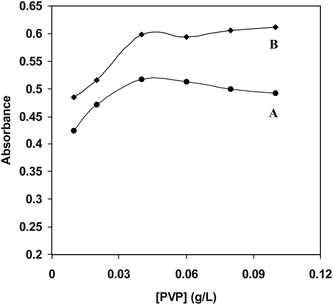 |
| Fig. 4 Effect of PVP concentration on the absorbances plasmon of Ag-NPs AgNO3 (2 × 10−3 M), PVP (0.01-0.1 g L−1), NaOH (0.2 mM), DA (B) and ASC (A). | |
3.3 Effect of NaOH concentration
The influence of NaOH concentration on Ag+ reduction by analyte is expected since the analyte has a dihydroxyphenyl group which can lose H+ during oxidation (Scheme 1). Because buffered conditions failed to obtain silver nanoparticles NaOH was added to provide enough alkalinity. Fig. 5 shows the effect of NaOH concentration on silver nanoparticles SPR peak intensity. As it is shown, the signal increases up to a known concentration of NaOH then decreases, this might be due to Ag2O formation. Thus, a concentration of 0.2 mM NaOH was selected as the optimum NaOH concentration.
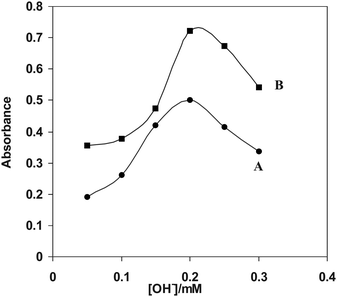 |
| Fig. 5 Dependence of the surface plasmon band intensity on the concentrations of NaOH, AgNO3 (2 × 10−3 M), PVP (0.06 g L−1), NaOH, (0.05-0.3 mM) DA (B) and ASC (A). | |
3.4 Analytical parameters
Under the optimum conditions the calibration curves were linear in the ranges 3.2 × 10−6 – 2.0 × 10−5 mol L−1, 2.0 × 10−6 – 48 × 10−6 mol L−1 for DA and ASC, respectively. Limits of detection were obtained at 1.2 × 10−6mol L−1 for DA and 1.1 × 10−6 mol L−1 for ASC and features of the analytical characteristics of the method for the individual analyte is shown in Table 3.
Table 3 Features the analytical characteristics of the method
Parameters |
DA |
ASC |
Unit of concentration in calibration equations are μM.
Theoretical detection limit (blank plus three times its S.D.)
|
Linear range/mol L−1 |
3.2 × 10−6 – 2.0 × 10−5 |
2.0 × 10−6 – 4.8 × 10−5 |
Calibration equation |
A = 0.0483Ca + 0.1192 (R2 = 0.997) |
A = 0.0156C + 0.1183 (R2 = 0.992) |
LODb (mol L−1) |
1.2 × 10−6 |
1.1 × 10−6 |
%RSD |
3.2–4.5 |
2.5– 3.8 |
3.5 Synergistic effect
Application of ANN in multivariate calibrations was proposed when significant nonlinearity is observed in the data. The nonlinearity due to synergistic effect, which is the main factor in the error of the kinetic analysis, has attracted much attention, and some work has been done to solve this problem in nonlinear systems with the aid of chemometrics.45 As Fig. 6 shows the kinetic curve of Ag+ after reaction with the binary mixture of the analyte (curve A) has a lower overall intensity than the one computed from the sum of the kinetic curve of Ag+ after reaction with the individual analyte (curve B). Thus, a factor(s), which is not accounted for by the simple Beer's and absorbance additivity laws and ANN models can be used for such nonlinear systems to resolve mixtures.
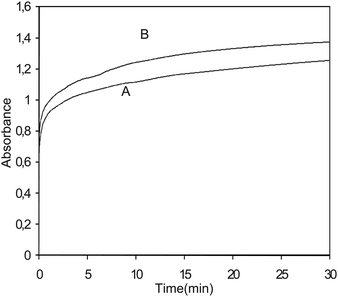 |
| Fig. 6 Kinetic curve of Ag+ after reaction with the binary mixture of the analyte (A) and sum of the kinetic curve of Ag+ after reaction with the individual analyte (B). | |
3.6 ANN modeling
A set of sample solutions, Table 1 and Table 2, with different concentrations of DA and ASC were prepared and measurements were carried out under the optimum conditions described in Section 3.3. According to the analytical procedure described in Section 3.3, the measured kinetic data and two calibration and prediction matrices used to establish calibration models with ANN. These models were then used for prediction of unknown samples. Studies on the network structure include the selection of the number of layers and the number of nodes in each layer. The network was confined to three layers, because learning of a network with higher layers is very complex and is more time consuming. Since the high number of nodes in the input layer will increase the learning time, the data were subjected to principal component analysis before introducing to the network and, therefore, the input of the ANN model was the extracted principle components of the absorbance data matrix. Since the number of nodes in the hidden layer is dependent on the number of input nodes, the number of nodes in the input and hidden layers were optimized simultaneously. The Eigen-vectors were entered step-by-step, and in each step the number of nodes in the hidden layer was varied to reach the minimum value of SE. The network parameters that should be optimized include the transfer functions in the layers, the momentum and the learning rate. The most versatile transfer function that can be used to model a variety of non-liner relationships is a sigmoid transfer function. In our network, it was found that the sigmoid transfer function in the hidden layer has a better performance than the other transfer functions (i.e., tangent, Gaussian and linear functions). The characteristics of the optimized networks used to model the titration data are summarized in Table 4.
Table 4 Optimized parameters used for construction of ANN models
Parameter |
DA |
ASC |
Input nodes |
5 |
5 |
Output nodes |
1 |
1 |
Hidden nodes |
8 |
7 |
Learning rate |
0.85 |
0.9 |
Momentum |
0.04 |
0.03 |
Number of iteration |
16.000 |
10.000 |
Hidden layer transfer function |
Sigmoid |
Sigmoid |
Output layer transfer function |
Sigmoid |
Sigmoid |
(PCS) |
5 |
5 |
3.7 Prediction ability of the calibration models
In order to complete our investigation about the performance of the optimized model, the proposed ANN model was used to predict the concentration of DA and ASC in some other mixtures not used in the training and prediction sets and the results are given in Table 5. It is immediately obvious that the ANN model possesses a high ability to predict the concentration of these analytes with low error levels. The prediction ability of a single component and total components in the mixtures were evaluated in terms of the percent relative error of the predicted concentrations, relative error of prediction (REP), root mean square error (RMSE) and square of correlation coefficient (r2) of the results. These results are summarized in Table 6. | 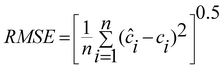 | (1) |
| 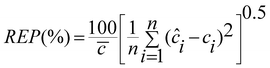 | (2) |
Where n is the number of samples, ci is the concentration of the component in the jth mixture and ĉi is the estimated concentration. The low values of REP and RMSE indicate that the networks used can properly process the relationship between the concentration of DA and ASC in the mixture and the absorbance at different mixtures. The plot of the predicted concentrations of each analyte in the mixtures versus their actual concentrations showed a nice fit of data to a straight line (with r2 > 0.990). This confirms the excellent predictive ability of the networks used in this study.
Table 5 Statistical parameters calculated for the prediction set using optimized neural network model
Analyte Parameter |
DA |
ASC |
RMSD |
0.0234 |
0.0577 |
REP(%) |
0.7217 |
1.0289 |
SEP |
0.0603 |
0.0905 |
R2 |
0.9948 |
0.9957 |
Table 6 Prediction results for determination of DA and ACS using the optimized neural network model
Sample |
Added |
Found |
Added |
Found |
DA/μM |
DA/μM |
ASC/μM |
ASC/μM |
1 |
4.00 |
4.13 |
20.00 |
20.70 |
2 |
4.00 |
3.92 |
40.80 |
40.82 |
3 |
2.40 |
2.40 |
20.00 |
20.00 |
4 |
2.40 |
2.54 |
40.80 |
4.65 |
5 |
5.60 |
5.85 |
20.00 |
19.32 |
6 |
5.60 |
5.60 |
40.80 |
41.20 |
7 |
7.20 |
7.21 |
20.00 |
17.90 |
RSE (%) |
|
6.3% |
|
8.1% |
3.8 Analytical application
The proposed method was successfully applied to the simultaneous determination of DA and ASC in human serum. For the determination of analyte in human serum, a certain amount of each the compounds was spiked to the samples without further treatment and their concentrations were determined by the proposed methods. The results are given in Table 7. The results show that there is a satisfactory agreement between the declared analyte content and the determined value. Therefore, the proposed method could be effectively used for the determination of DA and AA in real samples such as human serum.
Table 7 Determination of DA and AA in human serum as a real sample
Sample |
Added |
Found |
Added |
Found |
DA/μM |
DA/μM |
ASC/μM |
ASC/μM |
1 |
4.50 |
4.65 |
6.40 |
6.32 |
2 |
4.50 |
4.64 |
42.00 |
42.11 |
3 |
6.80 |
6.83 |
32.00 |
32.16 |
4 |
12.40 |
12.41 |
6.40 |
6.40 |
5 |
3.20 |
3.20 |
24.00 |
24.00 |
6 |
20.00 |
20.17 |
48.00 |
48.32 |
7 |
15.60 |
15.35 |
48.00 |
47.58 |
8 |
8.40 |
8.45 |
12.60 |
12.55 |
RSE (%) |
|
8.3% |
|
7.1% |
4. Conclusion
A new kinetic spectrophotometric method for the simultaneous analysis of DA and ASC has been successfully researched. The proposed method was dependent on the reduction of Ag+ by the analyte to make silver nanoparticles. The kinetics of the formation of silver nanoparticles by DA and ASC and followed by spectrophotometry indicate that the proposed method is satisfactory for the simultaneous determination of DA and ASC in mixtures.
Acknowledgements
Financial support for this work by the research councils of Persian Gulf University and Sharif University of Technology are highly appreciated.
References
- P. Damier, E. C. Hirsch, Y. Agid and A. M. Graybiel, Brain, 1999, 122, 1437–1448 CrossRef.
- T. Selvaraju and R. Ramaraj, Electrochem. Commun., 2003, 5, 667–672 CrossRef CAS.
- T. Hussain and M. F. Lokhandwala, Exp. Biol. Med., 2003, 228, 134–142 Search PubMed.
- P. A. Jose, G. M. Eisner and R. A. Felder, Pharmacol. Ther., 1998, 80, 149–182 CrossRef CAS.
- G. Ivan, N. Szigeti, M. Olah, G. M. Nagy and M. I. Goth, Endocrine, 2005, 28, 101–110 CrossRef CAS.
- T. Kienast and A. Heinz, CNS Neurol. Disord.: Drug Targets, 2006, 5, 109–131 CrossRef CAS.
- P. Karabinas and D. Jannakoudakis, J. Electroanal. Chem., 1984, 160, 159–167 CrossRef CAS.
- M. Dominguez, A. Aldaz and F. Sanchez-Burgos, J. Electroanal. Chem., 1976, 68, 345–354 CrossRef CAS.
- S. P. Perone and W. J. Kretlow, Anal. Chem., 1966, 38, 1760–1770 CrossRef CAS.
- H. I. Feng and K. Theodore, Anal. Chem., 1986, 58, 3235–3239 CrossRef CAS.
-
B. A. Fox, A. G. Cameron, Nutrition and Health, fifth ed., Edward Arnold, London, 1989. p. 261 Search PubMed.
- M. Bahram and A. Afkhami, J. Iran. Chem. Soc., 2008, 5, 352–366 Search PubMed.
- A. Afkhami and A. R. Zarei, Talanta, 2001, 53, 815–821 CrossRef CAS.
- A. Afkhami, T. Madrakian and A. R. Zarei, Anal. Sci., 2001, 17, 1199–1202 CAS.
- A. Afkhami and A. R. Zarei, Talanta, 2003, 60, 63–71 CrossRef CAS.
- E. Furusjo and L. G. Danielson, Anal. Chim. Acta, 1998, 373, 83–94 CrossRef CAS.
-
B. G. M. Vandeginste, D. L. Massart, L. M. C. Buydens, S. De Jong, P. J. Lewi, J. Smeyers-Verbeke, Elsevier, Amsterdam, 1998. Handbook of Chemometrics and Qualimetrics Search PubMed.
- G. López-Cueto, J. Lizarreta, M. Ostra and C. Ubide, Talanta, 2002, 58, 569–578 CrossRef CAS.
- Y. Ni, Y. Wang and S. Kokot, Food Chem., 2008, 109, 431–438 CrossRef CAS.
- A. Afkhami, N. Sarlak and A. R. Zarei, Talanta, 2007, 71, 893–899 CrossRef CAS.
- M. Chamsaz, A. Safavi and J. Fadaee, Anal. Chim. Acta, 2007, 603, 140–146 CAS.
- Y. Ni, Y. Wang and S. Kokot, Spectrochim. Acta, Part A, 2008, 70, 1049–1059 CrossRef.
-
Y. Ni, Science Press: Peking., 2004, 134–142.
- M. A. El-Sayed, Acc. Chem. Res., 2001, 34, 257–264 CrossRef CAS.
- A. C. Templeton, W. P. Wuelfing and R. W. Murray, Acc. Chem. Res., 2000, 33, 27–36 CrossRef CAS.
- Y. Sun and Y. Xia, Analyst, 2003, 128, 686–691 RSC.
- A. D. McFarland and R. P. Van Duyne, Nano Lett., 2003, 3, 1057–1062 CrossRef CAS.
- T. R. Jensen, M. Duval Malinsky, C. L. Haynes and R. P. Van Duyne, J. Phys. Chem. B, 2000, 104, 10549–10556 CrossRef CAS.
- K. L. Kelly, E. Coronado, L. Zhao and G. C. Schatz, J. Phys. Chem. B, 2003, 107, 668–677 CrossRef CAS.
- C. L. Haynes, A. D. McFarland, L. Zhao, R. P. Van Duyne, G. C. Schatz, L. Gunnarsson, J. Prikulis, B. Kasemo and M. Kãll, J. Phys. Chem. B, 2003, 107, 7337–7342 CrossRef CAS.
- S. Y. Lin, S. W. Liu, C. M. Lin and C. H. Chen, Anal. Chem., 2002, 74, 330–335 CrossRef CAS.
- S. O. Obare, R. E. Hollowell and C. J. Murphy, Langmuir, 2002, 18, 10407–10410 CrossRef CAS.
- S. Watanabe, H. Seguchi, K. Yoshida, K. Kifune, T. Tadaki and H. Shiozaki, Tetrahedron Lett., 2005, 46, 8827–8829 CrossRef CAS.
- J. Liu and Y. Lu, J. Fluoresc., 2004, 14, 343–354 CrossRef CAS.
- M. Reza Hormozi Nezhad, M. Alimohammadi, J. Tashkhourian and S. Mehdi Razavian, Spectrochim. Acta, Part A, 2008, 71, 199–203 CrossRef.
- R. Baron, M. Zayats and I. Willner, Anal. Chem., 2005, 77, 1566–1571 CrossRef CAS.
- F. X. Zhang, L. Han, L. B. Israel, J. G. Daras, M. M. Maye, N. K. Ly and C. J. Zhong, Analyst, 2002, 127, 462–465 RSC.
-
C. S. Thaxton, C. A. Mirkin, in: C. M. Niemeyer, C. A. Mirkin (ed.), Nanobiotechnology, Wiley-VCH, Weinheim, 2004, 288 Search PubMed.
- J. Ling, Y. Sang and C. Zhi Huang, J. Pharm. Biomed. Anal., 2008, 47, 860–864 CrossRef CAS.
- C. Yan Kang, D. Li Xi, Y. Yuan Chen and Zh. Liang Jiang, Talanta, 2008, 74, 867–870 CrossRef CAS.
- P. J. Gemperline, J. R. Long and G. Gerogriou, Anal. Chem., 1991, 63, 2313–2323 CrossRef CAS.
-
J. S. Bradley, in “Clusters and Colloids” (G. Schmid, Ed.), p. 469. VCH, Weinheim, 1994 Search PubMed.
- H. Bönnemann and K. S. Nagabhushana, Encycl. Nanosci. Nanotechnol., 1, 2004, 779–784 Search PubMed.
- H. Wang, X. Qiao, J. Chena, X. Wang and S. Ding, Mater. Chem. Phys., 2005, 94, 449–459 CrossRef CAS.
- M. Blanco, J. Coello, H. Iturriaga, S. Maspoch and M. Redon, Analyst, 1996, 121, 395 RSC.
|
This journal is © The Royal Society of Chemistry 2010 |