DOI:
10.1039/C4MB00265B
(Paper)
Mol. BioSyst., 2015,
11, 239-251
Systems analysis reveals down-regulation of a network of pro-survival miRNAs drives the apoptotic response in dilated cardiomyopathy†
Received
29th April 2014
, Accepted 18th October 2014
First published on 20th October 2014
Abstract
Apoptosis is a hallmark of multiple etiologies of heart failure, including dilated cardiomyopathy. Since microRNAs are master regulators of cardiac development and key effectors of intracellular signaling, they represent novel candidates for understanding the mechanisms driving the increased dysfunction and loss of cardiomyocytes during cardiovascular disease progression. To determine the role of cardiac miRNAs in the apoptotic response, we used microarray technology to monitor miRNA levels in a validated murine phospholambam mutant model of dilated cardiomyopathy. 24 miRNAs were found to be differentially expressed, most of which have not been previously linked to dilated cardiomyopathy. We showed that individual silencing of 7 out of 8 significantly down-regulated miRNAs (mir-1, -29c, -30c, -30d, -149, -486, -499) led to a strong apoptotic phenotype in cell culture, suggesting they repress pro-apoptotic factors. To identify putative miRNA targets most likely relevant to cell death, we computationally integrated transcriptomic, proteomic and functional annotation data. We showed the dependency of prioritized target abundance on miRNA expression using RNA interference and quantitative mass spectrometry. We concluded that down regulation of key pro-survival miRNAs causes up-regulation of apoptotic signaling effectors that contribute to cardiac cell loss, potentially leading to system decompensation and heart failure.
Introduction
Diverse forms of cardiovascular disease (CVD) lead to heart failure (HF), a severe disorder characterized by an inability of the heart to pump sufficient blood throughout the body1 and a major cause of mortality.2 Of variable aetiology, dilated cardiomyopathy (DCM) is a particularly serious variant of CVD. Clinically, DCM patients exhibit low cardiac output, circulatory congestion, systolic and/or diastolic dysfunction, and pathological remodeling of the heart leading to HF. Dysregulated intracellular stress responses associated with perturbed signaling cascades and elevated cell death have been implicated in DCM progression and poor patient outcomes.3 Genetic mapping of DCM/HF has revealed mutations in contractile proteins such as phospholamban4 (PLN), which is associated with impaired Ca2+ cycling by the cardiac sarco(endo)plasmic reticulum. Elevated apoptosis of cardiomyocytes has also been observed in DCM affected hearts,5 suggesting that cell loss likewise contributes to cardiac dysfunction. Yet despite recent progress, perturbations to the regulatory mechanisms driving cardiac cell death in mid- to late-stage DCM as a result of mutations in PLN or other factors that result in decreased cardiac contractility, decompensation, and ultimately overt HF, remain unclear.
MicroRNAs (miRNAs, or miRs) are a class of small non-coding regulatory RNAs that act as master regulators of gene expression by binding to target transcripts. They are known to play important roles both in normal cardiac development and heart pathology, such as hypertrophy and HF.6–10 Compared to transcription factors, the levels of mature miRNAs are more closely correlated to regulatory activity,11 making it relatively easier to predict their biological effects by RNA expression measurements. MiRNAs repress protein expression post-transcriptionally by destabilizing target gene transcripts and by inhibiting translation.12 MiRNAs bind to short seed sequences in the 3′ untranslated region (UTR) of target genes. Given their small size, miRNA–target recognition sites are difficult to accurately predict often resulting in multiple miRNAs associated with an individual target. However, integrating multiple lines of evidence can help to accurately filter these predictions.13 MiRNAs are excellent candidates for high-throughput profiling studies to identify novel CVD/HF-relevant effectors that can then be studied further to determine previously overlooked functional roles in cardiac pathogenesis. Moreover, little is known about the role of miRNA and their targets in cardiac cell death in DCM and/or HF.
To map the role of miRNAs and their putative targets in the late-stage pathological changes associated with DCM, we performed comprehensive surveys of messenger RNA transcript and protein expression levels in a validated transgenic PLN mutant (dominant-negative PLN-R9C) mouse model to identify changes associated with early stage CVD preceding HF as compared with normal control littermates. Previously, we reported a time-course quantification of over 6000 cardiac proteins as a function of disease progression using shotgun mass spectrometry.3 We identified both well-established biomarkers of HF (e.g. b-type natriuretic peptide (BNP), atrial natriuretic factor (ANF), and angiotensin-converting enzyme (ACE))1,14 and novel candidates, which we subsequently validated by RT-PCR and western-blotting, several of which are currently undergoing clinical assessment as potential diagnostic indicators of human heart failure. Pathway enrichment analysis revealed significant disease perturbation to apoptotic signaling. Notably, we observed dysregulation of key apoptotic regulators in DCM, including the pro-apoptotic factors p38 (MAPK14) and JNK (MAPK8),15 which are components of conserved MAP kinase signaling pathways. Up-regulation of p38 in rat myocytes is known to induce heart dilation,16 whereas down-regulation of the p38 or JNK catalytic subunits by aortic banding-induced stress leads to cardiac hypertrophy and eventually HF in transgenic mouse models.17,18 Consistent with this, a significant increase in apoptosis was detected in PLN-R9C DCM cardiac tissue, with clear activation of caspases 3, 9 and 12 evident at the earliest stages of cardiac dysfunction without overt pathology (albeit levels were strongest once pathological, biochemical, and functional remodeling was evident).3
We hypothesized that elevated levels of these and other stress-activated pro-apoptotic factors results in the loss of cardiomyocytes observed in DCM underlying the progression to HF, consistent with the view that modulation of death effector pathways may be a clinically relevant target.19 Indeed, administration of the beta blocker propranolol, commonly used clinically to treat HF,20 reduced MAPK-dependent pathway activation and improved DCM mice survival curves and restored both p38 and JNK to near wild-type levels,21 strengthening the association of apoptosis to DCM progression. However, it remained unclear if cardiomyocyte apoptosis was tempered or induced by misregulation of miRNAs and their targets during DCM.
In the present study, we apply a systems biology approach to identify and prioritize miRNAs linked to cardiac cell death in DCM, and using targeted mass spectrometry in the context of miRNA perturbation experiments in cell culture demonstrate support for relevant targets. Strikingly, we found that stress resistance is regulated by targets of miRNAs that are significantly down-regulated in early-stage DCM, including a key member of the Wnt signaling cell survival pathway that is inhibited by mir-499.
Results
Identifying differentially expressed miRNAs in DCM
We measured miRNA levels during DCM progression in our PLN-R9C transgenic model, compared with isogenic control littermates, using two independent microarray-based platform technologies (see Methods, Table S1, ESI†). Even at an early (pre-symptomatic) stage of disease (i.e. 8 weeks), which shows only very slight phenotypic perturbations in cardiac function assessed by cardiac echocardiography,3 widespread differential miRNA abundance was readily detected. The miRNA expression results from the two platforms were highly correlated (R2 ∼ 0.7) (Fig. 1), enabling confident identification of 139 differentially expressed miRNAs.
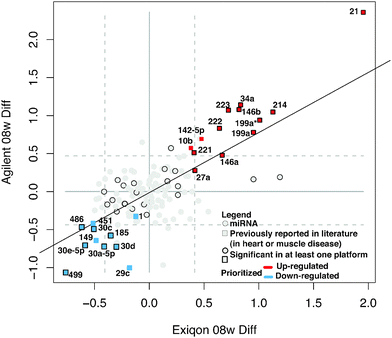 |
| Fig. 1 Differentially expressed miRNAs in early-stage DCM. Scatterplot shows the results of global miRNA profiling using two independent platforms (Exiqon and Agilent) of cardiac ventricle tissue (8 weeks post-partum) in PLN-R9C transgenic DCM model mice vs. wild-type littermates. Points in the graph represent log2 differential expression of each miRNA in the two platforms. Dashed grey lines indicate top/bottom 10% of the data. A subset of miRNAs is highlighted in red or blue if they were reproducibly up- or down-regulated in DCM in both platforms, respectively. Squares indicate the miRNA was previously shown to be associated with heart disease or muscular disorders. Dark borders indicate the differential expression was statistically significant in at least one of the platforms. | |
Application of stringent prioritization criteria based on the magnitude (fold change), statistical significance and consistency of change recorded using the two platforms (see Methods) revealed an initial candidate set of 24 early-stage disease-associated miRNAs (Fig. 1), of which nine were strongly (miR-21, 214, 34a, 199a*, 199a, 146a, 146b, 223, 222) and four moderately (mir-142-5p, 221, 27a, 10b) up-regulated in DCM, whereas five were strongly (miR-499, 30e-5p, 30a-5p, 30c, 486) and six moderately down-regulated in the disease state (miR-1, -149, 451, 30d, 185, 29c). Notably, the mature forms of all of these miRNAs were perfectly conserved from mouse to human, implying critical functions, with potential clinical relevance in the human system.
Of these, seven (miR-1, -199a, -21, -214, -30, -34a, -499) are known to modulate apoptosis in cardiomyocytes,8,22–27 while several others (i.e. mir-146a,28 -34a,29,30 -221,31–35 and -27a36) had previously been implicated in cell death in other biological contexts, such as cancer. We independently tested the relative change in transcript abundance of 10 of these candidates (mir-1, mir-30a, mir-499, mir-34a, mir-30e, mir-486, mir-451, mir-222, mir-223, mir-21) using quantitative qRT-PCR assays (Fig. 2). This confirmed up-regulation of mir-21, -222, -223 and down-regulation of miR-1, -30a, -30e, -451, -486, -499. We also noted that mir-21, -214, -199a*, -199a, -27a, -10b, and -29c are similarly up-regulated and down-regulated, respectively, in cardiac hypertrophy.37 Additionally, mir-21, -214, -199a, -221, -499, -30e-5p, -30a-5p, -149, -29c are up-regulated and down-regulated in the border zone associated with myocardial infarction.38
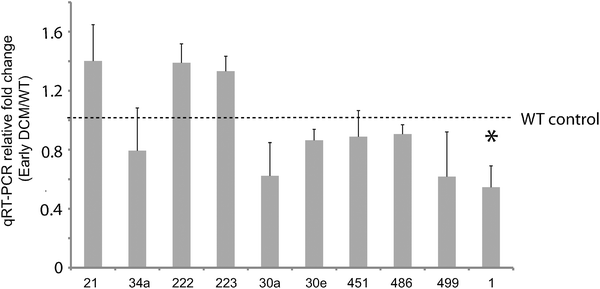 |
| Fig. 2 Validation of miRNA expression in early stage DCM via qRT-PCR. Ten miRNAs were independently validated for differential expression in early DCM relative to control by qRT-PCR. All miRNA microarray expression results were verified except for mir-34a, i.e. the direction of differential expression was consistent with what we expected. Only one miRNA(mir-1) demonstrated significant differential expression, indicated with a star. | |
Prediction and prioritization of apoptotic miRNAs using an integrative computational approach
Few experimentally confirmed targets were known for most of the differential miRNAs seen in DCM, but several had been predicted computationally based on matches of the miRNA seed region to complementary sequences in the 3′ UTR of mouse mRNA transcripts.39,40 As target prediction consistency and accuracy are typically low,41,42 we developed a novel integrative computational approach to predict and prioritize miRNAs and their targets that are involved in regulating apoptosis in DCM (Fig. 3). We computationally filtered the list of prioritized miRNAs and their targets using biological pathway information (targets linked to apoptosis and cell death) and global transcript and protein expression data we previously collected from mouse heart3,43 (agreement between miRNA, proteomic and transcriptomic profiles) for the PLN-R9C transgenic model.
 |
| Fig. 3 Apoptotic miRNA–target prioritization scheme. Pipeline for selecting differential miRNA and putative protein targets linked to apoptosis for follow up analysis. Starting with 139 cardiac miRNAs, measured by two expression array platforms, and their associated targets, as defined by TargetScan (version 5.2), we narrowed down our analysis to only those candidates with annotations pertaining to apoptosis that had supporting evidence in expression profiling datasets. | |
Putative mouse miRNA targets were collected from the TargetScan database (version 5.2), resulting in 38
937 miRNA–target pairs (consisting of 153 miRNA families and 7719 unique protein targets). Considering only the set of 24 differentially expressed miRNA candidates resulted in a reduction to 5542 pairs (consisting of 3249 unique proteins). Our integrative analysis identifying expected anti-correlated patterns between miRNA vs. protein and transcript expression levels followed by filtering to include targets in apoptosis, cell death and related pathways (see Table 1 and Methods) resulted in a final set of 302 putative miRNA–target pairs, encompassing 161 unique cardiac proteins, and 17 high-confidence differentially expressed miRNAs predicted to regulate cell death (Table 2).
Table 1 Scoring criteria used to quantify miRNA–protein target likelihood. We calculated a cumulative score for each miRNA–protein pair based on the listed rules. Higher agreement between multiple data sources supporting a regulatory relationship was reflected in a higher cumulative score for a given miRNA–target pair
Score |
Rule |
+1 |
Transcriptomics and proteomics agree (early DCM) |
+1 |
Proteomics and mir expression agree (early DCM) |
+1 |
Transcriptomics mir expression agree (early DCM) |
+1 |
Transcriptomics and proteomics agree (mid DCM) |
+1 |
Proteomics and mir expression agree (mid DCM) |
+1 |
Transcriptomics and mir expression agree (mid DCM) |
|
Penalties |
|
−1 |
Proteomics and mir expression disagree (early DCM) |
−1 |
Transcriptomics and mir expression disagree (early DCM) |
−1 |
Proteomics and mir expression disagree (mid DCM) |
−1 |
Transcriptomics and mir expression disagree (mid DCM) |
Table 2 Relation of miRNAs differentially expressed in early-stage DCM to other CVD studies and apoptosis. This chart summarizes previously published findings for our set of differential miRNAs. Highlighted in bold are miRNAs related to apoptosis that were selected for further study. miRNA abundance (red = elevated expression; blue = reduced expression; purple = mixed behavior) in independent profiling studies of tissue from: mouse PLN-R9C model of DCM (this study), focused small-scale study of late-stage human dilated cardiomyopathy (DCM),60 cardiac hypertrophy (CH),37 myocardial infarction (MI),38 muscle disorders (MD).88 Ap-C: miRNA has predicted targets relating to apoptosis; Ap-L: published links to apoptosis, dark gray if heart specific, light gray if reported for other cell/tissue types, with primary reference provided with a PubMed identifier (PMID) or an R indicated if reported in one of three reviews8,22,23
Although all of the targets included in this analysis were annotated to apoptotic terms and pathways through our prioritization scheme, less than a fifth (58) were previously linked to cell death in heart (based on PubMed searches for each target gene name and “apoptosis” and “heart”). Also, the majority (79, or 80%) of the 99 top ranked targets from our prioritization scheme (pairs with positive scores, as described in Methods) were associated with the set of down-regulated miRNAs (Fig. 4B). This supports the notion that the apoptotic cardiac events seen in heart in DCM preceding HF21 results, at least in part, from the selective down-regulation of anti-apoptotic miRNAs and the resulting up-regulation of important pro-apoptotic factors, some of which were likely identified in this integrative analysis.
Validation of apoptotic miRNAs
To validate the significance of the 17 differential miRNAs in apoptotic outcomes, we performed phenotypic assessments using an established Human embryonic kidney (HEK293) cell-based assay (see Methods) to examine the consequences of antagomiR-based miRNA silencing on cell viability in the absence or presence of oxidative stress (i.e. H2O2 challenge), a proxy for decompensation seen in DCM.44,45 During apoptosis, the phospholipid phosphatidylserine (PS) is translocated to the outer surface of the plasma membrane before nuclear DNA fragmentation occurs. Hence, we used annexin V, a small (∼35 kDa) Ca2+ dependent phospholipid-binding protein with high affinity for PS, conjugated to fluorochrome FITC, as an apoptosis probe in flow cytometric analysis of the treated cells. Measurement of FITC annexin V staining by FACS identified the fraction of apoptotic cells with exposed PS.
Strikingly, antagomiRs targeting most of the 17 miRNAs substantially impacted cell viability in HEK293 cells, showing a mix of pro- and anti-apoptotic effects upon knockdown as compared to control transfections with scrambled RNA (Fig. 4A); eight antagomiRs targeting miR-1, -10b, -29c, -30c, -30d, -149, -486, and -499 showed a significant decrease in viability in stressed cells, consistent with a potentially pro-survival role (i.e. anti-apoptotic targets). An opposite result was observed with miRNAs up-regulated in DCM, where antagomiRs targeting miR-199a, -21, -214, -34a resulted in a significant increase in viability of stressed cells, pointing to a potential apoptotic role. Intriguingly, antagomiRs targeting seven of eight miRNAs were also significantly down-regulated in early-stage DCM and appeared among this pro-survival subset, suggesting that the apoptotic response seen in late stage disease and replicated in cell culture is potentially a result of up-regulation of pro-apoptotic mRNA targets that impair the viability and stress resistance of cardiac cells. The one remaining antagomiR (-10b) with a significant decrease in viability in stressed cells was up-regulated in DCM instead of down-regulated as expected. It is possible that mir-10b can cause decreased viability but doesn't in our in vivo setting in the presence of other differential miRNAs.
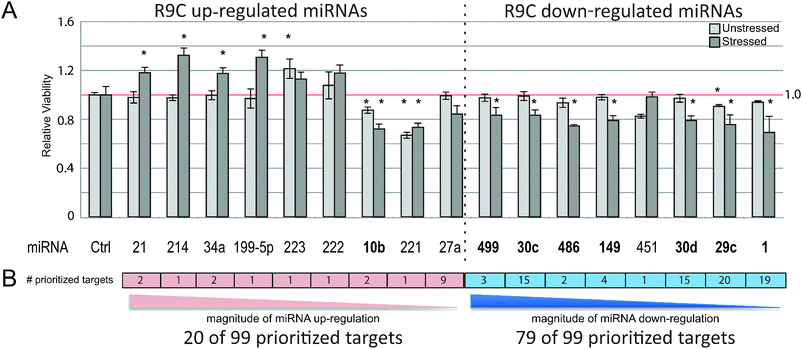 |
| Fig. 4 Impact of miRNAs on cell viability in HEK293 cells. (A) The bar graph shows cell viability in HEK293 cells under unstressed and stressed conditions after transfection with an antagomiR blocking the indicated miRNA (Ctrl refers to a control scrambled sequence); asterisks indicate significant difference in cell number, while the bold font indicates reduced viability after cell stress. miRNAs are divided into two sets, up- and down-regulated, separated by a dotted line. miRNAs in each set are sorted by decreasing differential expression as determined by our expression profiles. (B) Chart indicates the number of putative apoptotic targets found by our integrative prioritization scheme associated with that particular miRNA. Boxes highlighted in red are associated with miRNAs up-regulated in DCM, while those highlighted in blue are down-regulated in DCM. Total prioritized targets for both up and down regulated miRNAs are indicated. | |
To evaluate our findings in an experimental system closer to DCM, we validated that the eight miRNAs that showed decreased viability in HEK293 cells (mir-10b, -499, -30c, -486, -149, -30d, -29c, and -1) specifically affect cell viability in neonatal cardiomyocytes. Fig. 5 shows that of the eight tested, four (mir-499, -30c, -29c, and -1) showed a significant reduction in cell viability during a H2O2 stress, similar to the exposure in HEK293 cells. Importantly, we next determined that all eight miRNAs tested showed significant increase in both apoptosis and necrosis in H2O2-stressed cardiomyocytes by annexin V+ and PI+ staining (Fig. 6).
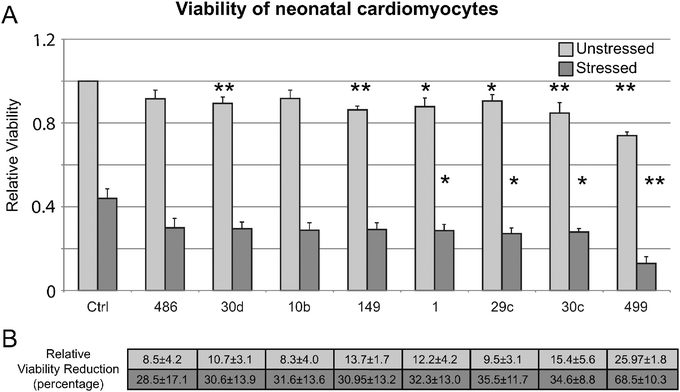 |
| Fig. 5 Impact of miRNA on cell viability in neonatal cardiomyocytes. (A) Cell viability in neonatal cardiomyocytes for the set of miRNAs found to have reduced viability in 1 mM H2O2 stressed HEK293 cells. Asterisks indicate significant difference in viability in antagomiR treated cardiomyocytes and control. Six out of the eight miRNAs tested in unstressed cells had significant differences between antagomiR treated cells and control. Four miRNAs had significant differences between antagomiR treated cells and controls under stressed conditions. (B) The average relative percent reduction in viability for each miRNA in both unstressed and stressed samples is indicated. | |
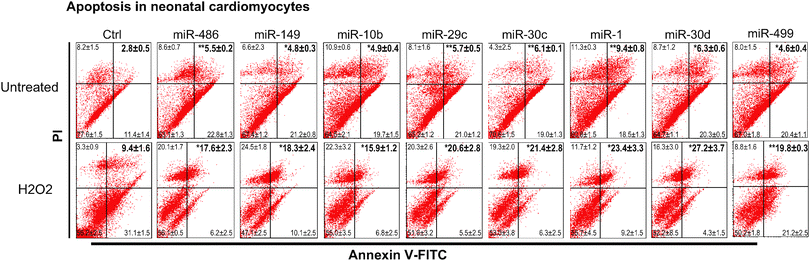 |
| Fig. 6 Impact of miRNAs on apoptosis in neonatal cardiomyocytes. Dual staining with fluorescent annexin V FITC and propidium iodide (PI) FACS analysis showing increased necrosis (PI+) and apoptosis (annexin V+/PI+) in cardiomyocytes with inhibition of targeted miRNAs and following 60 μM H2O2 for 24 hours. | |
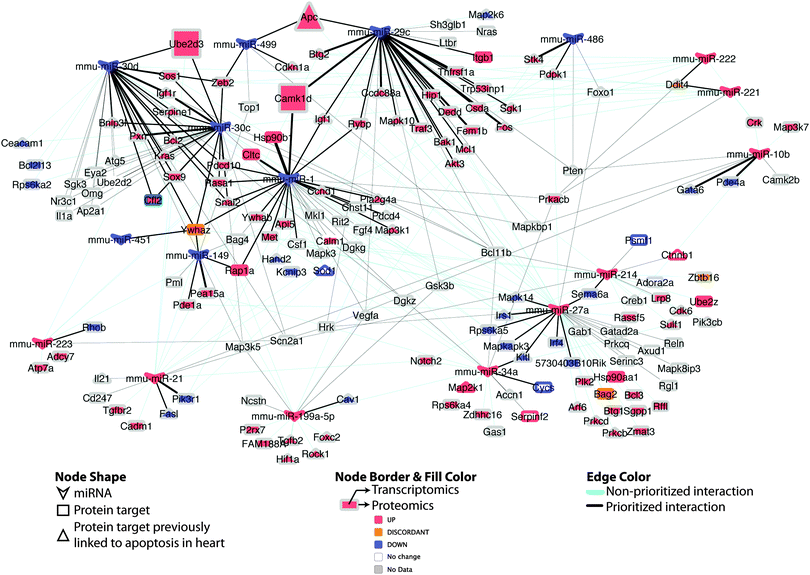 |
| Fig. 7 Differential miRNA–target network linked to apoptosis in DCM. Regulatory network of putative miRNA–target pairs implicated in cardiac cell death. Edges connect annotated target proteins to their putatively regulating miRNA. Node coloring indicates proteomic and transcriptomic expression at early-stage DCM, edge coloring indicates prioritized vs. non-prioritized miRNA–target associations, while edge thickness is proportional to the strength (integrative score, from Table S3, ESI†) of the available evidence supporting the regulatory interaction. Larger nodes (Apc; Ube2d3; Camk1d) indicate experimentally supported targets. | |
Intriguingly, when the set of apoptosis associated miRNA–target pairs is viewed as a network (Fig. 7), the potential for crosstalk and reinforcement between the set of miRNAs down-regulated in DCM becomes evident, with the majority of their targets being multiply and redundantly controlled. Hence, it appears likely that a robust apoptotic response is triggered in DCM upon perturbation of a group of miRNA–target pairs that work in tandem to influence cell viability.
Validation of differential miRNA targets
To verify our prioritized candidate apoptosis miRNA–target pairs, we quantified putative target protein abundance using Selected Reaction Monitoring (SRM) mass spectrometry (see Methods) following miRNA interference relative to untreated control cells. We artificially silenced a given endogenous miRNA in cell culture after transfection with individual antagomiRs, chemically engineered oligonucleotides that selectively block mRNA target recognition by a particular miRNA.
Of the nine putative apoptotic signaling targets detectable at endogenous levels by SRM (Table S2, ESI†), three matched the expected expression changes as a result of knockdown of the cognate upstream miRNA regulator (i.e. up-regulation after antagomiR treatment as compared to control as determined by t-test, assuming equal variance at a 95% confidence level; Table 3). Targets with supporting data include mir-29c (down-regulated in DCM) regulation of CAMK1D, a calcium/calmodulin-dependent protein kinase linked to apoptosis in murine erthroleukemia,46 mir-499 regulation of the tumor suppressor APC (adenomatous polyposis coli), a Wnt pathway antagonist with multiple connections to apoptosis,47,48 and mir-30d regulation of the ubiquitin-conjugating enzyme UBE2D3, which targets NRDP1 that in turn promotes degradation of the inhibitor of apoptosis, BRUCE, a novel pathway for triggering cell death.49 While each of these target proteins was previously reported to be involved in apoptosis, they have generally not been connected to heart disease, although APC negatively regulates canonical Wnt signaling during early embryonic heart development.50
Table 3 Independent validation of apoptotic miRNA–targets. Reported peptide levels for the indicated set of miRNA targets quantified by mass spectrometry. Italicised rows indicate that the expected anti-correlated protein vs. miRNA level is observed, following antagomiR treatment relative to control
miR |
R9C 8w differential |
Target protein |
Gene name |
Peptide |
p-value |
log2 ratio (miR antagomiR/control) |
* No synthetic peptide for confirmation. ** No statistical significance. *** Two peptides showing good agreement for slight differential expression in target although statistical significance was not reached. |
mmu-mir-221 |
Up-moderate |
sp|Q9D3F7|DDIT4_MOUSE |
Ddit4* |
LAYSEPCGLRGALLDVCVEQG |
0.003 |
−2.556393349 |
mmu-mir-149 |
Down-moderate |
sp|P63101|1433Z_MOUSE |
Ywhaz |
TAFDEAIAELDTLSEESYK |
0.025 |
−1.689659879 |
SVTEQGAELSNEER |
0.026 |
−1.556393349 |
mmu-mir-30c |
Down |
sp|P45591|COF2_MOUSE |
Cfl2 |
QILVGDIGDTVEDPYTSFVK |
0.043 |
−1.120294234 |
mmu-mir-30c |
Down |
sp|P63101|1433Z_MOUSE |
Ywhaz |
TAFDEAIAELDTLSEESYK |
0.048 |
−1.152003093 |
mmu-mir-451 |
Down-moderate |
p|P63101|1433Z_MOUSE |
Ywhaz*** |
AFDEAIAELDTLSEESYK |
0.12 |
−0.736965594 |
SVTEQGAELSNEER |
0.26 |
−0.59946207 |
mmu-mir-223 |
Up |
sp|P62746|RHOB_MOUSE |
Rhob** |
EVFETATR |
0.19 |
−1.689659879 |
mmu-mir-29c
|
Down-moderate
|
sp|Q8BW96|KCC1D_MOUSE
|
Camk1d
|
NIHESVSAQIR
|
9.5185 × 10−05
|
1.678071905
|
mmu-mir-499
|
Down
|
sp|Q61315|APC_MOUSE
|
Apc* |
QASSDSDSILSLK
|
0.02
|
1.117695043
|
mmu-mir-30d
|
Down-moderate
|
sp|P61079|UB2D3_MOUSE
|
Ube2d3*** |
SQWSPALTISK
|
0.21
|
0.678071905
|
VLLSICSLLCDPNPDDPLVPEIA
|
0.38
|
0.925999419
|
Discussion
Although CVD progression can result from necrosis, autophagy, and/or mitophagy, increasing lines of evidence from both in vitro and in vivo studies, including this work, strongly implicate cardiomyocyte loss through apoptosis as an important determinant in the development of HF after injury due to chronic cardiac stress stemming from impaired contraction, hypertension, ischemia or myocardial infarction.19,51–53 We recently identified a set of pathways, including actin processing, metabolism, various signaling pathways and apoptosis, to be dysregulated in DCM.21 Apoptosis was among the top pathways and also offered an easily measurable phenotype for targeted experiments and therefore was our first choice for directed analysis. To elucidate the mechanistic basis for this, we applied a prioritization approach, integrating multiple complementary data types – namely, global RNA and protein expression profiles with miRNA target predictions and pathway annotations, to assess if and how miRNAs control key aspects of CVD progression.
Seven apoptosis associated miRNAs, which we verified as potentially pro-survival in a stress response cell viability assay, were down-regulated in our mouse model of DCM. Four miRNAs were further shown to affect viability in neonatal cardiomyocytes. These seven apoptosis associated miRNAs are also predicted to regulate 80% of the prioritized apoptotic target set, with overlapping target sets creating a large interconnected cluster of down-regulated miRNAs and their associated targets (Fig. 7). We hypothesized that stress activated, miRNA-controlled pro-apoptotic signaling results in the loss of cardiomyocytes previously observed in DCM,54 and that modulation of these death effector pathways may be a clinically relevant target to mitigate the course of HF to improve patient outcomes.
Over 1500 miRNAs have been identified in the human genome,55 some of which (e.g. miR-1, -133, and -499) are preferentially expressed in normal heart and are known to play a key role in normal cardiac tissue specification and electrophysiology.56 Additionally, considerable effort has recently been invested in uncovering miRNAs causally associated with maladaptive heart remodeling and decompensation under different pathological settings (for reviews, see ref. 8, 10, 57 and 58).
Several miRNAs have been linked to the pathobiological changes leading to HF.6–8,37,38,59,60 For example, miR-133 has been shown to be a potent inhibitor of apoptosis and fibrosis in cardiac hypertrophy (CH),61 mis-expression of miR-208 underlies CH and fibrosis seen from transthoracic aortic banding in mice62 while over-expression of miR-195 in mouse myocardium is sufficient to induce both CH and HF.37 Therapeutic inhibition of miR-34 has also recently been shown to attenuate pathological cardiac remodeling and improve heart function in pressure-overload models of HF.63
Notable pathway targets of miRNAs include pro-hypertrophic signaling components.64 For example, down-regulation of miR-29, which is often up-regulated in CVDs accompanied by fibrosis such as myocardial infarction, promotes the expression of extracellular matrix components in cardiac fibroblasts, whereas its over-expression induces the opposite effect.38 Collectively, available data emphasize the strong causal links between miRNAs, signaling and heart disease progression, and suggest that additional critical regulatory connections remain to be discovered.
MiRNAs regulate translation by binding to complementary sequences in mRNA, usually in the 3′ UTR, thereby targeting transcripts for degradation through an RNA interference mechanism, or preventing initiation without affecting mRNA levels.65–67 As the latter mechanism does not depend on perfect sequence complementarity, a miRNA can potentially target dozens to hundreds of targets to affect entire signaling regulatory networks. However, knowledge and prediction of miRNA targets remains imperfect.68–70 Indeed, of the many thousands of predicted targets (reviewed in ref. 71–73), few relevant to cardiac signaling have been experimentally verified to date. Simply using the overlapping subset of targets generated between all available prediction algorithms is overly conservative, leaving only a handful of potential candidates for any given miRNA. Hence, we reasoned that experimental verification of prioritized miRNA–target pairs is essential to elucidate potentially clinically-relevant connections. By focusing our enrichment analysis on apoptosis, a process previously observed to become activated in DCM and other CVDs,74 we were able to predict a high quality set of miRNAs and targets associated with cardiac cell survival.
Apoptosis is a complex cellular response to signals that occur normally during heart development. In mature cardiomyocytes, activation of the apoptotic program in response to stress may be controlled by miRNAs modulating the abundance of multiple distinct targets in these cell death-inducing cascades. For example, we demonstrated here that, in the presence of an antagomiR blocking mir-499, expression of APC was significantly increased (more than 2-fold), presumably causing a depletion in β-catenin.50 Alterations in β-catenin-dependent Wnt signaling have been demonstrated under certain cardiac conditions, such as hypoxic conditions during differentiation of mouse pluripotent stem cells to beating cardiomyocytes, hypoxia activated Wnt signaling and impaired cardiomyocyte differentiation.75 Furthermore, Wnt has been shown to enhance apoptosis in cardiomyocytes76 suggesting a plausible mechanism for therapeutic intervention. We caution that while our protein quantification results largely supported our top ranked candidate miRNA–target pairs, the data do not strictly prove a direct miRNA–target relationship, which would require, for example, disrupting the predicted binding site(s) in the putative target mRNA. Nevertheless, the effects we observed are both consistent and most readily explained by a direct impact on mRNA stability or translation.
Increasing knowledge of cardiac pathways and physiologically relevant miRNA–target relationships should lead to substantial improvements in functional inference. Public resources such as TarBase currently report more than 65
000 experimentally verified miRNA–mRNA interactions,77 providing a potentially richer mechanistic view of targets relevant to cardiac disease progression. Hence, expanding the integrative prioritization approach described here should lead to a better understanding, and more informed clinical management, of other signaling-related processes perturbed in DCM that impact patient outcomes.
Additionally we can focus on other differential pathways and processes including the larger set of signaling pathways found to be differential in DCM. Prioritizing miRNA targets also found in kinase pathways followed by high throughput phospho-proteomic analysis of antagomiR transfected cardiomyocytes can help uncover potential key players in DCM.
Experimental methods
Measuring miRNA expression patterns in DCM
We used two different microarray platforms, manufactured by Exiqon and Agilent, to avoid platform-specific technical biases, such as probe cross-hybridization, to identify and quantify miRNA expression levels at early DCM time points. Total RNA was collected from ventricle tissue from a transgenic model expressing a mutant form of PLN, which results in DCM and healthy littermates (strain FVB/N) as a control.3
For the Exiqon platform four biological replicates of each DCM and wildtype (control) were used but only three of the control set passed RNA quality tests. The samples were labelled using the miRCURY™ Hy3™/Hy5™ power labeling kit and hybridized on the miRCURY™ LNA Array (v.9.2). Resulting signals, similar to two-dye microarray design, consisted of intensities from the sample relative to their corresponding references. Background correction was performed using the global locally weighted scatterplot smoothing regression (lowess) algorithm.78 Probes with not available (NA) values were discarded resulting in a set of 234 miRNAs. These were normalized using central value normalization. miRNAs were scored for differential expression between DCM and control using a student t-test (with Benjamini–Hochberg false discovery rate correction) with 36 miRNAs having corrected p-values < 0.05.
For the Agilent platform, three DCM and two control samples were run in duplicate with technical replicates run on different plates to generate six DCM and four control samples. Signals were extracted, and thresholded using GeneSpring. Signals were further log transformed and data was normalized using quantile normalization resulting in a set of 534 miRNAs to be assessed. miRNAs were scored for differential expression between DCM and control using a student t-test (with Benjamini–Hochberg false discovery rate correction) with 12 miRNAs having corrected p-values < 0.05.
All miRNA expression data has been deposited in NCBI's Gene Expression Omnibus79 and are accessible through GEO Series accession number GSE59961 (http://www.ncbi.nlm.nih.gov/geo/query/acc.cgi?acc=GSE59961).
The miRNAs were categorized into “strongly” and “moderately” differentially regulated, considering the magnitude (fold change), statistical significance and consistency of change recorded using the two platforms (miRNAs showing discordant expression between the two platforms were discarded). Only miRNAs common to both platforms were retained for subsequent analysis resulting in a set of 139 miRNAs. Each miRNA and its associated p-value, differential statistic and rank according to both Exiqon and Agilent platforms was calculated. The overall rank of each miRNA was determined by its sum of ranks from both platforms (Table S1, ESI†).
To prioritize the miRNAs, all miRNAs that showed consistent absolute differential expression >0.45 (which corresponds to the top 10% of the data) was included. Additional miRNAs that did not pass the above criteria were included if it had previously been reported in the literature to be associated with heart disease, muscular disease or fibrosis(as outlined in Table 2). This filtering resulted in a set of 24 candidate miRNAs.
Validation of miRNA expression via qRT-PCR
Total RNA including miRNAs was isolated from 8-week mouse hearts with Trizol/Chloroform method.3 0.5 μg RNA templates were used for converting into cDNA with ArrayScript reverse transcription kit from Ambion. These samples were diluted to a final concentration of 10 μg ml−1, and 20 ng samples were used for the quantitative RT-PCR mix for each reaction in 96-well plates with the Quantitect SYBR green RT-PCR kit from Qiagen. The ABIprism 7000 Sequence Detection System from Applied Systems was used. The amplification was as follows: 95 °C for 15 min initial activation, 94 °C for 15 s, 50–60 °C for 30 s, 72 °C for 30 s, 40 cycles. We examined differential expression by qRT-PCR for 10 of our significantly differential miRNAs (six down-regulated and four up-regulated). miRNA primers were obtained from Ambion and consisted of mir-1, mir-30a, mir-499, mir-30e, mir-486, mir-451 from our down regulated set and mir-222, mir-223, mir-21, and mir-34a from our up-regulated miRNAs. The 2−ΔΔCT method for miRNA expression quantification was applied as described80 using the RPL34 (ribosomal protein L34) RNA as a reference for normalization.
Prioritization of apoptotic miRNA and their regulatory targets
We collected an extensive set of biological pathway annotations from diverse curated sources including the Gene Ontology,81 KEGG,82 BioCarta and Reactome83 databases. A subset of pathways was retained for enrichment analysis based on relevance to cell death (annotated with the terms ‘apoptosis’ or ‘death’). Additional pathways were added manually if they had more than 50% overlap in gene membership with a selected apoptotic pathway. This resulted in a final set of 153 pathways associated with cell death (Table S2, ESI†).
For each miRNA, only those predicted targets (extracted from the TargetScan 5.284 database) that map to the annotated apoptotic or cell death functions and pathways were kept. Each miRNA–target pair was scored based on the experimentally recorded pattern of differential abundance and the overall qualitative (up vs. down) agreement between its protein and transcript expression levels based on our previously published R9C expression profiling study3 using a set of rules (Table 1). Concordance of protein and transcript expression to the changes in miRNA levels observed during disease progression was also assessed (e.g. up-regulated miRNAs with a corresponding down-regulation in associated target abundance received higher scores, and vice versa). Putative targets with differential expression in protein and transcript data and which correlated predictably with a targeting miRNA received the highest rank. Targets that exhibited behaviour contrary to the existing miRNA mechanism of action paradigm, i.e. miRNA and target protein expression both go up or down coordinately, were penalized. Only prioritized miRNAs, as described above, which had positively scored (score > 0) apoptotic targets were considered for further analysis.
miRNA–target interaction network creation
The apoptotic miRNA–target pair network of 176 nodes (with 17 miRNAs and 159 protein targets) and 302 edges was visualized with Cytoscape version 3.0.2. Two node types were defined (miRNA or protein target) and visualized as arrowheads or rectangles, respectively. Protein targets previously published in articles relating to heart and apoptosis are visualized as triangles. Protein target nodes were annotated with early-stage and mid stage transcriptomics and proteomics expression using the node and node border coloring, respectively. Edges represent a miRNA–target regulatory interaction, where the thickness of the edge correlates to our calculated prioritization score (ranging from −3 to 3). Non-prioritized edges, scores < 0 are colored in cyan.
AntagomiR transfection
HEK293 cells, grown under standard culture conditions, were transfected with anti-miRNA chemical inhibitors (antagomiRs; [short interfering oligonucleotides that bind to and block the action of miRNAs44,45]). Cells were plated 24 hours prior to transfection at a concentration ensuring a monolayer cell density of approximately 80% confluence prior to transfection. For each reaction, 3 μL miRNA (GenePharma, Shanghai) was diluted in 100 μL pre-warmed serum free DMEM with high glucose. 9 μL LipoD293 (SignaGen) was also diluted in 100 μL pre-warmed serum free DMEM with high glucose. Diluted LipoD293 reagent was added all at once to the diluted miRNA solution and mixed by pipetting up and down. Solutions were left at RT for 20 minutes. 200 μl lipo/miRNA mix was added drop-wise to a single well of a six well plate and mixed by gentle rocking back and forth. Cells were left at 37 °C/5% CO2. After 24 hours, transfection media was changed for fresh media (DMEM, 10% FBS, 0.1% antibiotics) and cells were left another 24 hours prior to processing. After 48 hours, media was removed and 1 mL PBS added to each well. Cells were scraped and transferred to 1.5 mL Eppendorf tubes. From this 1 mL, 250 μL was transferred to a fresh tube, spun down for 30 seconds at 14
000 × g and supernatant discarded. Pellet was stored at −80 °C for later use. Remaining 750 μL was spun down at 14
000 × g for 30 seconds and supernatant discarded. Pellet was resuspended in 500 μL ice cold lysis buffer (0.25 M sucrose, 50 mM Tris-Cl pH 7.6, 1 mM MgCl2, 1 mM DTT, 1× Complete protease inhibitor (Roche)) and passed through a 26 gauge needle five times. Lysates were spun at 700 × g for five minutes at 4 °C and the supernatant transferred to a fresh Eppendorf tube and then stored at −80 °C. All reactions were performed in triplicate.
Phenotypic screening of disease-associated miRNAs in HEK293 cells
After 24 hours, half of the antagomiR transfected cells was subsequently treated for 24 hours with an oxidizing agent (1 mM H2O2) in serum free media to stress the cells and emphasize an apoptotic response, while the other half were exposed to media alone. We then performed a colorimetric-based cell viability assay as described.85,86 The cells were incubated for four hours with the dye (5% w/v) WST-8 (2-(2-methoxy-4-nitrophenyl)-3-(4-nitrophenyl)-5-(2,4-disulfophenyl)-2H-tetrazolium; Dojindo Molecular Technologies), at which point absorbance was read at 450 nm. Stressed and unstressed results were normalized relative to the negative control group (transfected with a control scrambled sequence). Viability of each miRNA was calculated using a student t-test comparing the control to the antagomiR-transfected cells for the stressed and unstressed condition.
Phenotypic screening of disease-associated miRNAs in neonatal cardiomyocytes
Mouse neonatal cardiomyocytes (MNCs) were isolated according to our previously published procedures.86 Briefly, each heart was isolated and cut into small pieces, washed and then incubated with 1 mg ml−1 collagenase type II (Worthington Biochemicals) and 0.5 mg ml−1 pancreatin (Sigma) for 2 h, deactivated with 1 ml of 10% FBS, 10% Horse serum DMEM/F12 media and then cells were isolated and pre-plated for 2 h to remove the cardiac fibroblasts. Ventricular cells were plated, in 96 well plates (4 × 104 per well) allowed to recover and cultured for one day. One day-old cells were used for antagomiR transfection experiment using lipofectamine RNAiMAX Reagent (Life Technologies). After 24 h the cells were cultured in defined serum free maintenance media: medium (DMEM/F12) with additions of insulin (1 μg ml−1), ascorbic acid (25 μg ml−1) and thyroxine (1 nm).
3-(4,5-Dimethylthiazol-2-yl)-2,5-diphenyltetrazolium bromide MTT assays were carried out for detection of cell viability using the MTT Assay (Sigma) according to the manufacturer's instructions. After 72 hours antagomiR transfected MNCs were treated in 96-well plates with/without 1 mM H2O2 for four hours or a low dose of 60 μM H2O2 for 24 h86 at 37 °C, followed by 0.5 mg ml−1 of MTT was added to each well and the plate was placed in the incubator (37 °C) for a period of four hours. Untransformed MTT was removed and formazan crystals were dissolved in dimethyl sulfoxide (150 μl per well). Formazan absorbance was measured at 570 nm. Viability of each miRNA was calculated using a student t-test comparing the control to the antagomiR transfected cells for the stressed and unstressed condition.
Levels of apoptosis and necrosis were measured by annexin V and propidium iodide (PI) staining and fluorescence-activated cell sorting (FACS) analysis87 at St Michaels Hospital Flow Facility (Toronto).
SRM-based quantification of putative apoptotic targets of miRNAs
Multiplexed Selected Reaction Monitoring (SRM) assays were performed using a nanoflow pump (Proxeon nanoLC system) coupled to a Vantage triple quadrupole mass spectrometer (ThermoFisher Scientific, San Jose, CA). For each target, targeted assays for three to five unique peptides were devised using the Pinpoint software (version 1.1) according to the following criteria: (i) length less than 30 amino acids whenever possible (ii) avoiding C and M amino acid residues whenever possible (iii) avoiding K and R residues in close proximity and (iv) peptides observed in Peptide Atlas and GPM databases. SRM transitions and collision energy were predicted in silico using Pinpoint software and the four most intense transitions were then selected experimentally using synthetic peptides, where available, except for short peptides where only three usable transitions could be obtained. The list of all peptides and transitions used are summarized in Table S4 (ESI†).
Following harvest, total protein was extracted from antagomiR transfected HEK293 cells. The amount of protein was normalized across each sample based on Bradford assay measurements, and 50 μg total protein was subject to enzymatic digestion. Higher starting amounts of protein (up to 1 mg) were also tested during preliminary experiments, but did not improve detection of low/not expressed targets.
Equivalent aliquots of the samples were prepared prior to SRM as follows: after thawing on ice, samples were precipitated using trichloroacetic acid (10% v/v), incubated overnight at 4 °C, and then centrifuged at 17
000g at 4 °C. The supernatant was removed carefully, and the precipitate washed twice with ice-cold 300 μL acetone. After decanting, the proteins were resolubilized in 50 mM ammonium bicarbonate (pH 8.0) containing 1 mM calcium chloride, treated with 5 mM DTT for 30 min on ice at RT, followed by carboxymethylation using 15 mM iodoacetamide for 30 min in the dark. Finally, the samples were digested using 1 μg sequencing grade trypsin (Roche Applied Science) for 18 hours. The samples were dried using a Speedvac, and resolubilized in 1% formic acid and 5% acetonitrile. A QC peptide standard mix (ThermoFisher, 0.5 μL) was spiked into all samples as an internal control prior to injection to monitor retention time reproducibility and signal intensity throughout the runs. If any signal drift was observed for a particular sample, the response was corrected based on the response of these QC peptides. A mixture of synthetic peptide standards for retention time and ion ratio confirmation was also run for each batch of miRNA related samples.
Chromatographic separation was performed using a 75 μm inner diameter fused silica column packed with 10 cm of Luna 3 μm C18(2) 100 Å reversed phase particles (Phenomenex). Flow rate was set to 300 nl min−1 for a 80 min gradient: 8 min hold at 95% buffer A (98% water, 2% acetonitrile, 0.1% formic acid), linear gradient to 30% buffer B (100% acetonitrile with 0.1% formic acid) in 50 min, 10 min linear gradient to 80% B, followed by 12 min wash with 80% B. The MS instrument was operated using unit resolution, with a collision gas pressure of 1.5 mTorr, a nanosource electrospray voltage of 2600 V, and a capillary temperature of 250 °C.
Scheduled SRMs were used to increase the number of transitions monitored per run. For miRNAs for which a large number of putative target proteins were monitored, the analysis was split into two or three separate methods to ensure a minimum dwell time of 0.015 s per transition. Data were acquired using Xcalibur 2.1 and processed using Pinpoint Version 1.1 or 1.3. Targets were deemed reliably detected if the observed transitions matched both the retention time and isotope ion ratios observed for synthetic reference peptide standards.
Conclusion
We have combined computation and experimentation to identify cardiac miRNAs and their targets that are differentially expressed in a validated mouse model of DCM, providing insights into the mechanistic basis for cardiac decompensation to HF. Given that these miRNAs, their cell death associated targets, and apoptotic signaling pathways in general are highly conserved, follow up studies should reveal if these same regulatory connections underlie human pathology.
Author contribution
NB performed cell viability, antagomiR transfections, QT PCR and generated all samples for SRM validation. DM analyzed miRNA expression and prioritized candidates. DV performed the SRM measurement and analysis. DW performed antagomiR transfection and cell viability in neonatal cardiomyocytes. RI prioritized protein–miRNA pairs for validation and wrote the paper with AE with input from all other authors. AOG, GB, and AE supervised the project.
Conflict of interest
The authors declare that they have no conflict of interest.
Acknowledgements
This work was supported by the Heart and Stroke Foundation of Ontario (grant numbers T-7284, T-6281, and NS-6636 to AE, GB and AOG), the U.S. National Institutes of Health National Center for Research Resources (grant number P41 GM103504 to GDB); and the Ontario Research Fund – Global Leadership Round in Genomics and Life Sciences (GL2-01012) to AOG and AE. AOG is a Canada Research Chair in Cardiovascular Proteomics and Molecular Therapeutics, AE is the Ontario Chair in Biomarkers.
References
- T. Thom, N. Haase, W. Rosamond, V. J. Howard, J. Rumsfeld, T. Manolio, Z. J. Zheng, K. Flegal, C. O'Donnell, S. Kittner, D. Lloyd-Jones, D. C. Goff, Jr., Y. Hong, R. Adams, G. Friday, K. Furie, P. Gorelick, B. Kissela, J. Marler, J. Meigs, V. Roger, S. Sidney, P. Sorlie, J. Steinberger, S. Wasserthiel-Smoller, M. Wilson and P. Wolf, Circulation, 2006, 113, e85–151 CrossRef PubMed.
- A. S. Go, D. Mozaffarian, V. L. Roger, E. J. Benjamin, J. D. Berry, W. B. Borden, D. M. Bravata, S. Dai, E. S. Ford, C. S. Fox, S. Franco, H. J. Fullerton, C. Gillespie, S. M. Hailpern, J. A. Heit, V. J. Howard, M. D. Huffman, B. M. Kissela, S. J. Kittner, D. T. Lackland, J. H. Lichtman, L. D. Lisabeth, D. Magid, G. M. Marcus, A. Marelli, D. B. Matchar, D. K. McGuire, E. R. Mohler, C. S. Moy, M. E. Mussolino, G. Nichol, N. P. Paynter, P. J. Schreiner, P. D. Sorlie, J. Stein, T. N. Turan, S. S. Virani, N. D. Wong, D. Woo and M. B. Turner, Circulation, 2013, 127, e6–e245 CrossRef PubMed.
- A. O. Gramolini, T. Kislinger, R. Alikhani-Koopaei, V. Fong, N. J. Thompson, R. Isserlin, P. Sharma, G. Y. Oudit, M. G. Trivieri, A. Fagan, A. Kannan, D. G. Higgins, H. Huedig, G. Hess, S. Arab, J. G. Seidman, C. E. Seidman, B. Frey, M. Perry, P. H. Backx, P. P. Liu, D. H. MacLennan and A. Emili, Mol. Cell. Proteomics, 2008, 7, 519–533 CAS.
- H. Morita, J. Seidman and C. E. Seidman, J. Clin. Invest., 2005, 115, 518–526 CrossRef CAS PubMed.
- G. Olivetti, R. Abbi, F. Quaini, J. Kajstura, W. Cheng, J. A. Nitahara, E. Quaini, C. Di Loreto, C. A. Beltrami, S. Krajewski, J. C. Reed and P. Anversa, N. Engl. J. Med., 1997, 336, 1131–1141 CrossRef CAS PubMed.
- Y. Cheng, R. Ji, J. Yue, J. Yang, X. Liu, H. Chen, D. B. Dean and C. Zhang, Am. J. Pathol., 2007, 170, 1831–1840 CrossRef CAS PubMed.
- S. Ikeda and W. T. Pu, Curr. Drug Targets, 2010, 11, 913–925 CrossRef CAS PubMed.
- M. V. Latronico and G. Condorelli, Nat. Rev. Cardiol., 2009, 6, 419–429 Search PubMed.
- D. L. Mann, N. Engl. J. Med., 2007, 356, 2644–2645 CrossRef CAS PubMed.
- T. Thum, D. Catalucci and J. Bauersachs, Cardiovasc. Res., 2008, 79, 562–570 CrossRef CAS PubMed.
- H. Guo, N. T. Ingolia, J. S. Weissman and D. P. Bartel, Nature, 2010, 466, 835–840 CrossRef CAS PubMed.
- T. Du and P. D. Zamore, Development, 2005, 132, 4645–4652 CrossRef CAS PubMed.
- J. C. Huang, Q. D. Morris and B. J. Frey, J. Comput. Biol., 2007, 14, 550–563 CrossRef CAS PubMed.
- C. S. Lam, J. C. Burnett, Jr., L. Costello-Boerrigter, R. J. Rodeheffer and M. M. Redfield, J. Am. Coll. Cardiol., 2007, 49, 1193–1202 CrossRef CAS PubMed.
- C. P. Baines and J. D. Molkentin, J. Mol. Cell. Cardiol., 2005, 38, 47–62 CrossRef CAS PubMed.
- P. Liao, D. Georgakopoulos, A. Kovacs, M. Zheng, D. Lerner, H. Pu, J. Saffitz, K. Chien, R. P. Xiao, D. A. Kass and Y. Wang, Proc. Natl. Acad. Sci. U. S. A., 2001, 98, 12283–12288 CrossRef CAS PubMed.
- J. C. Braz, O. F. Bueno, Q. Liang, B. J. Wilkins, Y. S. Dai, S. Parsons, J. Braunwart, B. J. Glascock, R. Klevitsky, T. F. Kimball, T. E. Hewett and J. D. Molkentin, J. Clin. Invest., 2003, 111, 1475–1486 CrossRef CAS PubMed.
- Q. Liang, O. F. Bueno, B. J. Wilkins, C. Y. Kuan, Y. Xia and J. D. Molkentin, EMBO J., 2003, 22, 5079–5089 CrossRef CAS PubMed.
- G. Kung, K. Konstantinidis and R. N. Kitsis, Circ. Res., 2011, 108, 1017–1036 CrossRef CAS PubMed.
- M. Klapholz, Mayo Clin. Proc., 2009, 84, 718–729 CrossRef CAS PubMed.
- R. Isserlin, D. Merico, R. Alikhani-Koupaei, A. Gramolini, G. D. Bader and A. Emili, Proteomics, 2010, 10, 1316–1327 CrossRef CAS PubMed.
- Z. P. Huang, R. L. Neppl and D. Z. Wang, J. Cardiovasc. Transl. Res., 2010, 3, 212–218 CrossRef PubMed.
- P. Li, J. Cardiovasc. Transl. Res., 2010, 3, 219–224 CrossRef PubMed.
- R. A. Boon, K. Iekushi, S. Lechner, T. Seeger, A. Fischer, S. Heydt, D. Kaluza, K. Treguer, G. Carmona, A. Bonauer, A. J. Horrevoets, N. Didier, Z. Girmatsion, P. Biliczki, J. R. Ehrlich, H. A. Katus, O. J. Muller, M. Potente, A. M. Zeiher, H. Hermeking and S. Dimmeler, Nature, 2013, 495, 107–110 CrossRef CAS PubMed.
- J. X. Wang, J. Q. Jiao, Q. Li, B. Long, K. Wang, J. P. Liu, Y. R. Li and P. F. Li, Nat. Med., 2011, 17, 71–78 CrossRef CAS PubMed.
- G. Lv, S. Shao, H. Dong, X. Bian, X. Yang and S. Dong, J. Cell. Biochem., 2014, 115, 93–101 CrossRef CAS PubMed.
- S. Rane, M. He, D. Sayed, H. Vashistha, A. Malhotra, J. Sadoshima, D. E. Vatner, S. F. Vatner and M. Abdellatif, Circ. Res., 2009, 104, 879–886 CrossRef CAS PubMed.
- G. Chen, I. A. Umelo, S. Lv, E. Teugels, K. Fostier, P. Kronenberger, A. Dewaele, J. Sadones, C. Geers and J. De Greve, PLoS One, 2013, 8, e60317 CAS.
- C. Welch, Y. Chen and R. L. Stallings, Oncogene, 2007, 26, 5017–5022 CrossRef CAS PubMed.
- M. Yamakuchi, M. Ferlito and C. J. Lowenstein, Proc. Natl. Acad. Sci. U. S. A., 2008, 105, 13421–13426 CrossRef CAS PubMed.
- A. M. Cheng, M. W. Byrom, J. Shelton and L. P. Ford, Nucleic Acids Res., 2005, 33, 1290–1297 CrossRef CAS PubMed.
- R. Dai, J. Li, Y. Liu, D. Yan, S. Chen, C. Duan, X. Liu, T. He and H. Li, Biol. Chem., 2010, 391, 791–801 CrossRef CAS PubMed.
- M. Garofalo, G. Di Leva, G. Romano, G. Nuovo, S. S. Suh, A. Ngankeu, C. Taccioli, F. Pichiorri, H. Alder, P. Secchiero, P. Gasparini, A. Gonelli, S. Costinean, M. Acunzo, G. Condorelli and C. M. Croce, Cancer Cell, 2009, 16, 498–509 CrossRef CAS PubMed.
- Q. Lu, C. Lu, G. P. Zhou, W. Zhang, H. Xiao and X. R. Wang, Urol. Oncol., 2010, 28, 635–641 CrossRef CAS PubMed.
- M. T. van Jaarsveld, J. Helleman, E. M. Berns and E. A. Wiemer, Int. J. Biochem. Cell Biol., 2010, 42, 1282–1290 CrossRef CAS PubMed.
- R. Chhabra, Y. K. Adlakha, M. Hariharan, V. Scaria and N. Saini, PLoS One, 2009, 4, e5848 Search PubMed.
- E. van Rooij, L. B. Sutherland, N. Liu, A. H. Williams, J. McAnally, R. D. Gerard, J. A. Richardson and E. N. Olson, Proc. Natl. Acad. Sci. U. S. A., 2006, 103, 18255–18260 CrossRef CAS PubMed.
- E. van Rooij, L. B. Sutherland, J. E. Thatcher, J. M. DiMaio, R. H. Naseem, W. S. Marshall, J. A. Hill and E. N. Olson, Proc. Natl. Acad. Sci. U. S. A., 2008, 105, 13027–13032 CrossRef CAS PubMed.
- P. Maziere and A. J. Enright, Drug Discovery Today, 2007, 12, 452–458 CrossRef CAS PubMed.
- N. D. Mendes, A. T. Freitas and M. F. Sagot, Nucleic Acids Res., 2009, 37, 2419–2433 CrossRef CAS PubMed.
- P. Alexiou, M. Maragkakis, G. L. Papadopoulos, M. Reczko and A. G. Hatzigeorgiou, Bioinformatics, 2009, 25, 3049–3055 CrossRef CAS PubMed.
- T. M. Witkos, E. Koscianska and W. J. Krzyzosiak, Curr. Mol. Med., 2011, 11, 93–109 CrossRef CAS PubMed.
- A. O. Gramolini, T. Kislinger, P. Liu, D. H. MacLennan and A. Emili, Methods Mol. Biol., 2007, 357, 15–31 CAS.
- J. Krutzfeldt, S. Kuwajima, R. Braich, K. G. Rajeev, J. Pena, T. Tuschl, M. Manoharan and M. Stoffel, Nucleic Acids Res., 2007, 35, 2885–2892 CrossRef CAS PubMed.
- J. Krutzfeldt, N. Rajewsky, R. Braich, K. G. Rajeev, T. Tuschl, M. Manoharan and M. Stoffel, Nature, 2005, 438, 685–689 CrossRef PubMed.
- T. Yamada, M. Suzuki, H. Satoh, F. Kihara-Negishi, H. Nakano and T. Oikawa, Exp. Cell Res., 2004, 294, 39–50 CrossRef CAS PubMed.
- J. Qian, K. Steigerwald, K. A. Combs, M. C. Barton and J. Groden, Oncogene, 2007, 26, 4872–4876 CrossRef CAS PubMed.
- K. Steigerwald, G. K. Behbehani, K. A. Combs, M. C. Barton and J. Groden, Mol. Cancer Res., 2005, 3, 78–89 CrossRef CAS PubMed.
- X. B. Qiu, S. L. Markant, J. Yuan and A. L. Goldberg, EMBO J., 2004, 23, 800–810 CrossRef CAS PubMed.
- M. Rezvani and C. C. Liew, J. Biol. Chem., 2000, 275, 18470–18475 CrossRef CAS PubMed.
- V. P. van Empel, A. T. Bertrand, L. Hofstra, H. J. Crijns, P. A. Doevendans and L. J. De Windt, Cardiovasc. Res., 2005, 67, 21–29 CrossRef CAS PubMed.
- K. Konstantinidis, R. S. Whelan and R. N. Kitsis, Arterioscler., Thromb., Vasc. Biol., 2012, 32, 1552–1562 CrossRef CAS PubMed.
- D. A. Kubli and A. B. Gustafsson, Circ. Res., 2012, 111, 1208–1221 CrossRef CAS PubMed.
- J. Narula, P. Pandey, E. Arbustini, N. Haider, N. Narula, F. D. Kolodgie, B. Dal Bello, M. J. Semigran, A. Bielsa-Masdeu, G. W. Dec, S. Israels, M. Ballester, R. Virmani, S. Saxena and S. Kharbanda, Proc. Natl. Acad. Sci. U. S. A., 1999, 96, 8144–8149 CrossRef CAS.
- S. Griffiths-Jones, H. K. Saini, S. van Dongen and A. J. Enright, Nucleic Acids Res., 2008, 36, D154–D158 CrossRef CAS PubMed.
- G. W. Dorn, 2nd, Transl. Res., 2011, 157, 226–235 CrossRef PubMed.
- K. G. Barringhaus and P. D. Zamore, Circulation, 2009, 119, 2217–2224 CrossRef PubMed.
- E. van Rooij, W. S. Marshall and E. N. Olson, Circ. Res., 2008, 103, 919–928 CrossRef CAS PubMed.
- D. Sayed, C. Hong, I. Y. Chen, J. Lypowy and M. Abdellatif, Circ. Res., 2007, 100, 416–424 CrossRef CAS PubMed.
- T. Thum, C. Gross, J. Fiedler, T. Fischer, S. Kissler, M. Bussen, P. Galuppo, S. Just, W. Rottbauer, S. Frantz, M. Castoldi, J. Soutschek, V. Koteliansky, A. Rosenwald, M. A. Basson, J. D. Licht, J. T. Pena, S. H. Rouhanifard, M. U. Muckenthaler, T. Tuschl, G. R. Martin, J. Bauersachs and S. Engelhardt, Nature, 2008, 456, 980–984 CrossRef CAS PubMed.
- S. J. Matkovich, W. Wang, Y. Tu, W. H. Eschenbacher, L. E. Dorn, G. Condorelli, A. Diwan, J. M. Nerbonne and G. W. Dorn, 2nd, Circ. Res., 2010, 106, 166–175 CrossRef CAS PubMed.
- B. M. Palmer, Heart Failure Rev., 2005, 10, 187–197 CrossRef CAS PubMed.
- B. C. Bernardo, X. M. Gao, C. E. Winbanks, E. J. Boey, Y. K. Tham, H. Kiriazis, P. Gregorevic, S. Obad, S. Kauppinen, X. J. Du, R. C. Lin and J. R. McMullen, Proc. Natl. Acad. Sci. U. S. A., 2012, 109, 17615–17620 CrossRef CAS PubMed.
- E. van Rooij, L. B. Sutherland, X. Qi, J. A. Richardson, J. Hill and E. N. Olson, Science, 2007, 316, 575–579 CrossRef CAS PubMed.
- D. H. Chitwood and M. C. Timmermans, Nature, 2010, 467, 415–419 CrossRef CAS PubMed.
- L. He and G. J. Hannon, Nat. Rev. Genet., 2004, 5, 522–531 CrossRef CAS PubMed.
- M. Esteller, Nat. Rev. Genet., 2011, 12, 861–874 CrossRef CAS PubMed.
- J. Brennecke, A. Stark, R. B. Russell and S. M. Cohen, PLoS Biol., 2005, 3, e85 Search PubMed.
- A. Krek, D. Grun, M. N. Poy, R. Wolf, L. Rosenberg, E. J. Epstein, P. MacMenamin, I. da Piedade, K. C. Gunsalus, M. Stoffel and N. Rajewsky, Nat. Genet., 2005, 37, 495–500 CrossRef CAS PubMed.
- T. W. Lhakhang and M. A. Chaudhry, J. Appl. Genet., 2011, 53, 149–158 CrossRef PubMed.
- M. Thomas, J. Lieberman and A. Lal, Nat. Struct. Mol. Biol., 2010, 17, 1169–1174 CAS.
- N. H. Tan Gana, A. F. Victoriano and T. Okamoto, Genes Cells, 2012, 17, 11–27 CrossRef PubMed.
- W. Ritchie, J. E. Rasko and S. Flamant, Adv. Exp. Med. Biol., 2013, 774, 39–53 CrossRef CAS PubMed.
- M. M. Kockx and M. W. Knaapen, J. Pathol., 2000, 190, 267–280 CrossRef CAS PubMed.
- T. L. Medley, M. Furtado, N. T. Lam, R. Idrizi, D. Williams, P. J. Verma, M. Costa and D. M. Kaye, PLoS One, 2013, 8, e80280 CAS.
- H. Schumann, J. Holtz, H. R. Zerkowski and M. Hatzfeld, Cardiovasc. Res., 2000, 45, 720–728 CrossRef CAS PubMed.
- T. Vergoulis, I. S. Vlachos, P. Alexiou, G. Georgakilas, M. Maragkakis, M. Reczko, S. Gerangelos, N. Koziris, T. Dalamagas and A. G. Hatzigeorgiou, Nucleic Acids Res., 2012, 40, D222–D229 CrossRef CAS PubMed.
- W. S. Cleveland, J. Am. Stat. Assoc., 1979, 74, 829–836 CrossRef.
- R. Edgar, M. Domrachev and A. E. Lash, Nucleic Acids Res., 2002, 30, 207–210 CrossRef CAS PubMed.
- K. J. Livak and T. D. Schmittgen, Methods, 2001, 25, 402–408 CrossRef CAS PubMed.
- M. Ashburner, C. A. Ball, J. A. Blake, D. Botstein, H. Butler, J. M. Cherry, A. P. Davis, K. Dolinski, S. S. Dwight, J. T. Eppig, M. A. Harris, D. P. Hill, L. Issel-Tarver, A. Kasarskis, S. Lewis, J. C. Matese, J. E. Richardson, M. Ringwald, G. M. Rubin and G. Sherlock, Nat. Genet., 2000, 25, 25–29 CrossRef CAS PubMed.
- M. Kanehisa, S. Goto, M. Furumichi, M. Tanabe and M. Hirakawa, Nucleic Acids Res., 2010, 38, D355–D360 CrossRef CAS PubMed.
- D. Croft, G. O'Kelly, G. Wu, R. Haw, M. Gillespie, L. Matthews, M. Caudy, P. Garapati, G. Gopinath, B. Jassal, S. Jupe, I. Kataskaya, S. Mahajan, B. May, N. Ndegwa, E. Schmidt, V. Shamovsky, C. Yung, E. Birney, H. Hermjakob, P. D'Eustachio and L. Stein, Nucleic Acids Res., 2011, 39, D691–D697 CrossRef CAS PubMed.
- B. P. Lewis, C. B. Burge and D. P. Bartel, Cell, 2005, 120, 15–20 CrossRef CAS PubMed.
- N. Bousette, S. Chugh, V. Fong, R. Isserlin, K. H. Kim, A. Volchuk, P. H. Backx, P. Liu, T. Kislinger, D. H. MacLennan, A. Emili and A. O. Gramolini, Proc. Natl. Acad. Sci. U. S. A., 2010, 107, 18481–18486 CrossRef CAS PubMed.
- R. Chis, P. Sharma, N. Bousette, T. Miyake, A. Wilson, P. H. Backx and A. O. Gramolini, Am. J. Physiol.: Heart Circ. Physiol., 2012, 303, H967–H978 CrossRef CAS PubMed.
- A. M. Rieger, K. L. Nelson, J. D. Konowalchuk and D. R. Barreda, J. Visualized Exp., 2011, 50, e2597 Search PubMed.
- I. Eisenberg, A. Eran, I. Nishino, M. Moggio, C. Lamperti, A. A. Amato, H. G. Lidov, P. B. Kang, K. N. North, S. Mitrani-Rosenbaum, K. M. Flanigan, L. A. Neely, D. Whitney, A. H. Beggs, I. S. Kohane and L. M. Kunkel, Proc. Natl. Acad. Sci. U. S. A., 2007, 104, 17016–17021 CrossRef CAS PubMed.
Footnote |
† Electronic supplementary information (ESI) available: Fig. S1 FACS gating. (A) Graphs depict the gated events for FACS analysis for both untreated and H2O2 treated samples. Gates were set to include at least 10 000 cells and cut off the small cell debris. (B) Graphs represent the dot plot depiction of PI gate and annexin V gate in the gated cell without PI and annexin V staining. The percentages of each quadrant are shown as unstaining control. Table S1 miRNAs expression from Exiqon and Agilent. The set of miRNAs common to both platforms were retained for subsequent analysis resulting in a set of 139 miRNAs. Each miRNA is listed with its associated p-value, differential statistic according to Exiqon and Agilent platforms, and its overall rank calculated as the sum of its ranks in Exiqon and Agilent. Table S2 Apoptotic and related terms. The set of pathway annotations relating to apoptosis. Additional terms with a significant number of genes in common with apoptotic terms were also included. Table S3 miRNA–protein pairs. The set of 302 miRNA–target protein pairs used for the analysis. The set of 99 that were prioritized to be associated with apoptosis are indicated. Highlighted rows are miRNA–target protein pairs that were shown by SRM. Table S4 All peptides and transitions used in SRM Assay. Summary of all scheduled SRM transitions tested for each miRNA including parent to product ion transitions monitored, dwell time, and scheduling. See DOI: 10.1039/c4mb00265b |
|
This journal is © The Royal Society of Chemistry 2015 |