Identification of source areas of polycyclic aromatic hydrocarbons in Ulsan, South Korea, using hybrid receptor models and the conditional bivariate probability function†
Received
30th July 2021
, Accepted 14th December 2021
First published on 17th December 2021
Abstract
This study identifies the emission source areas for the atmospheric polycyclic aromatic hydrocarbons (PAHs) detected in Ulsan, South Korea. To achieve this, in addition to a conditional bivariate probability function (CBPF), two hybrid receptor models – the three-dimensional potential source contribution function (3D-PSCF) model and the 3D concentration weighted function (3D-CWT) model – were used, both of which adopt trajectory segments within the mixing layer. Notably, the fraction-weighted trajectory (FWT), a combination of PAH gas/particle partitioning with a hybrid receptor model, was introduced for the first time in this study to support the identification of emission source areas using other approaches (i.e., 3D-PSCF, 3D-CWT, and CBPF). Consequently, it was found that gaseous PAHs in Ulsan mostly originated from local emission sources (i.e., transportation and industrial emissions) throughout the year, whereas particulate PAHs were likely to originate from emission sources in China (e.g., Shandong, Hebei, and Liaoning) during spring and winter via long-range transport. However, in summer and fall, the influence of local emissions on particulate PAHs appeared to be stronger. The FWT was able to distinguish between local and distant sources more effectively, especially in summer and fall, i.e., the periods when local sources increased their contribution. This study thus increases the understanding of the long-range transport of PAHs in Northeast Asia, and the novel FWT approach exhibits the potential to be employed in the source area identification of various semi-volatile organic chemicals.
Environmental significance
This study identifies the emission source areas for the atmospheric polycyclic aromatic hydrocarbons (PAHs) detected in Ulsan, South Korea using a conditional bivariate probability function and hybrid receptor models which adopt trajectory segments within the mixing layer. Notably, the fraction-weighted trajectory (FWT), a combination of PAH gas/particle partitioning with a hybrid receptor model, was introduced for the first time in this study to support the identification of emission source areas using the other approaches. Consequently, gaseous and particulate PAHs in Ulsan mostly originated from local emission sources and long-range transport from China, respectively. The FWT distinguished between local and distant sources more effectively, therefore, this approach can be employed in the source area identification of various semi-volatile organic chemicals.
|
1. Introduction
Polycyclic aromatic hydrocarbons (PAHs) are a group of organic chemicals containing at least two aromatic rings. In the atmosphere, PAHs can be found in both gaseous and particulate forms depending on their molecular weight and the meteorological conditions (e.g., ambient temperature and relative humidity). Because the physiochemical properties of gaseous and particulate PAHs (e.g., mobility, half-life, and volatility) differ, the two phases exhibit distinctive characteristics and behavior in the atmosphere. In particular, particulate PAHs tend to have longer atmospheric half-lives1 and can be removed from the air during transport through dry and wet deposition.2 Dry deposition acts on particulate PAHs via gravity, Brownian diffusion, and compaction.3 Thus, it primarily affects coarse particle-bound PAHs. In contrast, wet deposition removes gaseous PAHs and fine/ultrafine particle-bound PAHs when precipitation (i.e., rainfall or snowfall) occurs.4
PAHs originate from pyrogenic (i.e., combustion processes) and petrogenic (e.g., heavy oil) sources. To identify the emission sources of atmospheric PAHs, hybrid receptor models that associate backward air trajectories with PAH concentrations at the receptor site can be used.5 Several techniques have been developed based on this approach, including residence time analysis (RTA),6 the potential source contribution function (PSCF), and the concentration weighted function (CWT).7 Because air parcels can traverse several areas prior to arriving at a receptor site, these techniques have been adopted to investigate the transboundary transport of air pollutants at a non-local scale, i.e., over several hundreds to thousands of kilometers.8 In addition to hybrid receptor models, the conditional probability function (CPF) has also been used to determine the direction of emission sources by associating pollutant concentrations with the wind direction9 and is thus mostly employed to identify source areas at a local scale.10 More recently, the CPF was modified by combining it with the wind speeds at receptor sites to produce the conditional bivariate probability function (CBPF). Based on this, both the location and type of emission source (i.e., ground common, stack, and distant sources) can be determined.11
South Korea is located in Northeast Asia, which is one of the most PAH-polluted areas in the world.12 Because it is located downwind of the Asian monsoon system,13 the contribution of upwind sources to air pollution in South Korea has been widely investigated. For instance, the PSCF and a multivariate receptor model have been employed to demonstrate that northern China (e.g., Beijing, Hebei, and Tianjin), eastern China (e.g., Shanghai), and/or North Korea are contributors to an increase in particulate matter and particulate PAHs in the air of Seoul, South Korea, especially in winter.14–17 In addition, a PSCF that considers the vertical dispersion of air pollutants has been introduced to verify that the burning of biomass in northern China (e.g., Beijing, Tianjin, and Hebei), northeastern China (e.g., Liaoning), and/or North Korea can contribute to particulate PAH levels in Seoul.17,18 The concentrations of PAHs emitted from Chinese mega-cities (e.g., Beijing, Harbin, and Shanghai) have declined due to the Clean Air Action enacted by the Chinese government.19,20 Consequently, the long-range transport of PAHs emitted from China decreased in Seoul.21
Recently, three-dimensional (3D) hybrid receptor models that consider the vertical dispersion of pollutants (i.e., within the mixing layer or under a pre-defined height) have been reported to more effectively identify the source areas of pollutants.18,22,23 This is because trajectories with an elevation above the mixing height are likely to trap pollutants transported from other source areas because the pollutants are well mixed within the mixing layer after their emission. Thus, the mixing height along the backward trajectories and vertical dispersion of pollutants need to be considered for source identification using hybrid receptor models.
This study aims to identify regional and local source areas of gaseous and particulate PAHs in Ulsan, South Korea, using 3D hybrid receptor models (i.e., 3D-PSCF and 3D-CWT) and CBPF analysis. According to our recent study using the conventional PSCF, PAHs in Ulsan would also be contributed by emission sources in China in winter and spring.24 Therefore, conventional and 3D hybrid receptor models in these seasons are also compared in this study to more clearly understand the influence of vertical dispersion on source area identification for PAHs. Notably, a combination of PAH gas/particle partitioning and the CWT hybrid receptor model is developed for the first time in this study to confirm the PAH source areas identified by the 3D-PSCF and 3D-CWT models.
2. Materials and methods
2.1. Study area and PAH data
In this study, we used PAH data from our previous study.25 Gaseous and particulate PAHs were measured in Ulsan, the largest industrial city in South Korea. The industrial area of Ulsan, comprising petrochemical, non-ferrous, shipbuilding, and automobile complexes, is located in the eastern region of the city (Fig. 1). Under the influence of the Asian monsoon system, the prevailing wind direction in summer/fall and winter/spring in Ulsan is southeasterly and northwesterly, respectively. During winter (January–February) and spring (March–May), northwesterly winds arrive in Ulsan from several upwind areas (e.g., China, North Korea, and western regions of South Korea), promoting the transboundary transport of air pollutants to this city.
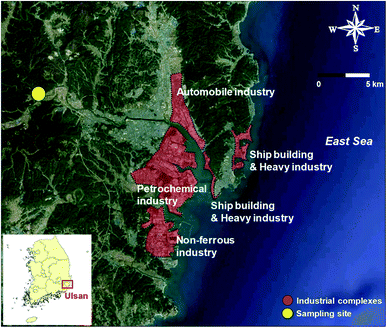 |
| Fig. 1 Location of the sampling site and industrial area in Ulsan, South Korea. | |
The target compounds are the US EPA priority PAHs, including fluorene (Flu), phenanthrene (Phe), anthracene (Ant), fluoranthene (Flt), pyrene (Pyr), benzo[a]anthracene (BaA), chrysene (Chr), benzo[b]fluoranthene (BbF), benzo[k]fluoranthene (BkF), benzo[a]pyrene (BaP), dibenzo[ah]anthracene (DahA), indeno[123-cd]pyrene (Ind), and benzo[ghi]perylene (BghiP). The particulate PAHs exhibit longer atmospheric lifetimes than the gaseous PAHs and have been primarily considered for long-range transport.18,26 Therefore, only the particulate and total (particulate + gaseous) concentrations of the ∑13 PAHs were used to identify regional emission source of PAHs through 3D hybrid receptor models. The gaseous and particulate samples were collected in duplicate using high-volume air samplers (Sibata, HV-700F, Japan) from June 2013 to May 2014. Sampling was conducted twice a week in February and April, when Asian dust events are common, and once a week in the other months. However, the samples collected in November and December 2013 were lost. The sampling campaign, therefore, covered 48 days and was divided into four seasons: summer (June–August 2013), fall (September–October 2013), winter (January–February 2014), and spring (March–May 2014). The sampling site was located at the Ulsan National Institute of Science and Technology (UNIST) in Ulsan, South Korea (35°34′18.32′′N, 129°11′20.46′′E). More details on the sampling method, chemical analysis, and QA/QC in our previous study25 are provided in Text S1 in the ESI.†
2.2. Backward air trajectories
The backward air trajectories for the 48 sampling days were simulated using the Hybrid Single-Particle Lagrangian Integrated Trajectory (HYSPLIT 4) model adopting Global Data Assimilation System (GDAS1) meteorological data. To evaluate the PAH transport from outside of South Korea to the receptor site in Ulsan, the total run time for the backward air trajectories was set at 120 hours (5 days) to fully observe the transport of air pollutants from Inner Mongolia to South Korea.27 The trajectories were obtained hourly for 24 hours from 11:00 a.m. local time for each sampling day. Data on the atmospheric mixing height and key meteorological parameters (i.e., rainfall level, air pressure, and solar radiation) along the transport path of the air parcels were also obtained using the HYSPLIT 4 model and GDAS1 data. The starting height of the trajectories was set at 10% to 90% of the mixing height at the sampling site to increase the resolution for the hybrid receptor models. A total of 10
368 backward trajectories (48 sampling days × 9 starting heights × 24 hours per day) were used for one sampling year. The number of trajectories for each season was 3456, 2595, 2376, and 1944 for spring, summer, fall, and winter, respectively. The uncertainty of the mixing height along the trajectory path was also considered by removing the trajectory segments exceeding 50% of the mixing layer. Endpoint selection was conducted using SAS 9.4 (SAS Institute Inc., USA).
2.3. Hybrid receptor models and the vertical dispersion of air pollutants
2.3.1. Three-dimensional PSCF (3D-PSCF) model.
The conventional PSCF model considers the entire backward air trajectory to identify source areas.28 However, the 3D-PSCF model reflects the vertical dispersion of air pollutants by only using trajectory segments within the mixing layer. The 3D-PSCF model was calculated following eqn (1): |  | (1) |
where 3D-PSCF is the potential source contribution function reflecting the vertical dispersion of air pollutants, and mij is the number of endpoints falling within cell (i, j) when the PAH concentrations are higher than the threshold value. Because there is no fixed threshold for PAH concentrations, the 50th, 75th, and 90th percentiles of the ∑13 PAH concentration were employed as thresholds for the 3D-PSCF model, with the most appropriate threshold then selected for further calculation. nij is the number of times the trajectories, as processed following Section 2.2, passed through cell (i, j). Because a low nij can lead to high PSCF output, a weighting function was multiplied by the 3D-PSCF to reduce this uncertainty. Two widely used weighting functions taken from previous research, W1
26,29–31 and W2,32,33 were assessed to determine their influence on the results. The most appropriate weighting function for the PAH dataset was then used for further calculation. The weighting functions W1 and W2 are described as follows: | 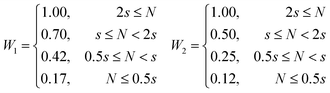 | (2) |
where s is the average number of trajectory endpoints per cell. The s values calculated for each sampling season are shown in Table S1 in the ESI.†N represents the total number of trajectory endpoints in each grid cell.
2.3.2. Three-dimensional CWT (3D-CWT) model.
The PSCF model is a relatively straightforward approach for locating the source areas of air pollutants; however, it cannot clearly distinguish between areas with strong, moderate, and weak emissions.34 In addition, source areas associated with concentrations slightly lower than the threshold can be underestimated by the PSCF model. Therefore, a 3D-CWT model that reflects the vertical dispersion of the pollutants by considering trajectory segments within the mixing layer was also used to confirm the source areas for the target PAHs. The 3D-CWT model was estimated based on eqn (3): | 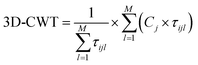 | (3) |
where M is the total number of backward trajectories, τijl is the number of endpoints in cell (i, j) for trajectory l, which has its transport route within the mixing layer, and Cj denotes the PAH concentrations at the sampling site. To prevent uncertainty arising from the low value of τijl, the weighting functions were also applied to the 3D-CWT model, as described in Section 2.3.1.
The conventional PSCF/CWT and 3D-PSCF/3D-CWT models were also compared to evaluate the influence of vertical dispersion on the identification of PAH source areas. The conventional PSCF/CWT and 3D-PSCF/3D-CWT models were calculated for 0.5° × 0.5° grid cells over a domain encompassing 110°–140°E and 25°–50°N (Fig. S1 in the ESI†) using TrajStat.35 The same threshold and weighting functions were used for the conventional PSCF/CWT and 3D-PSCF/3D-CWT models. In general, areas exhibiting high PSCF or CWT values can be regarded as source areas for the emission of pollutants.7 ArcGIS 10.5 (ESRI, USA) was employed to plot PSCF and CWT maps.
To consider the uncertainty of the 3D-hybrid receptor models, the bootstrap technique was applied.36–38 In particular, the number of trajectory endpoints per cell (i, j) was assumed to follow a normal distribution and then re-sampled repeatedly 500 times. The 3D-PSCF/3D-CWT models were re-calculated for their mean values and 95% confidence levels.
2.3.3. PSCF and CWT anomalies.
To understand any differences between the conventional and 3D hybrid receptor models, PSCF and CWT anomalies were calculated by subtracting the output of the 3D-PSCF/3D-CWT models from that of the conventional PSCF/CWT models. Anomalous values close to zero suggest a relatively small difference between the two approaches. Additionally, areas with positive anomalous values suggest that they have been overestimated as emission source areas and vice versa. PSCF and CWT anomalies were calculated using ArcGIS 10.5 (ESRI, USA).
2.4. Application of gas/particle partitioning for the identification of source areas
Particulate PAHs can be more persistent than gaseous PAHs because of their longer half-lives and lower mobility.1 Therefore, particulate PAHs are expected to contribute more to transboundary transport via near-surface winds in Northeast Asia.13,14,26,39 Atmospheric PAHs are found in both gaseous and particulate forms, and these phases can change during transport. In particular, gaseous PAHs can undergo nucleation, condensation, and coagulation to form particle-bound PAHs,40 while PAHs bound to aerosols can desorb and become gaseous ones.2 Thus, a combination of backward air trajectories and the gas/particle partitioning of PAHs is expected to more effectively determine emission source areas than the PSCF and CWT models, which adopt particulate or total (particulate + gaseous) concentrations of ∑13 PAHs. This new approach is referred to in this paper as the fraction-weighted trajectory (FWT) and is calculated following the 3D-CWT model, but using the particulate mass fraction of PAHs, a ratio of the particulate to total (gas + particle) concentrations of ∑13 PAHs. In general, areas with higher FWT values make a greater contribution to an increase in particulate PAH levels at a receptor site. Based on this approach, the FWT can also be applied for other semi-volatile organic compounds (e.g., polychlorinated biphenyls, polychlorinated naphthalenes, and halogenated PAHs) existing in both gaseous and particulate phases.
2.5. Trajectory cluster analysis
Trajectories simulated using HYSPLIT 4 were clustered into several groups based on their similar directions and lengths7 using TrajStat.35 In general, the distance between two trajectories was calculated based on the Euclidean distance following eqn (4): |  | (4) |
where d12 is the Euclidean distance between two backward trajectories, and X1, X2 and Y1, Y2 are the latitudes and longitudes of these trajectories, respectively. The number of clusters was then identified using the eyeball method35 and selected based on the variation in the total spatial variance (TSV) computed among the trajectory endpoints. A dramatic change in the TSV indicates that a suitable number of clusters has been found.35
2.6. Conditional bivariate probability function (CBPF) and polar concentration
Hybrid receptor models such as the PSCF and CWT typically determine source areas on a non-local scale (i.e., via transboundary transport)8 because the air parcels pass over several areas before arriving at the receptor site. To focus on the identification of source areas on a local scale, the conditional bivariate probability function (CBPF) was used. The CBPF provides information on the direction of source areas and source types based on the wind. For example, a high CBPF probability at low and high wind speeds suggests common ground-level and stack sources, respectively.11 Low wind speeds generally disperse atmospheric pollutants over a small area (i.e., on a local scale), whereas high wind speeds increase the dispersion of pollutants and contribute to their transport to distant areas. Therefore, low and high wind speeds associated with high CBPF probabilities can also indicate local and non-local emission sources, respectively.41,42
The CBPF was calculated based on eqn (5):
| 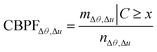 | (5) |
where Δ
θ and Δ
u are the wind sector (a range of wind directions) and wind speed, respectively.
C is the concentration of ∑
13 PAHs, which is higher than threshold
x within wind sector Δ
θ and for wind speed Δ
u.
mΔθ,Δu is the number of samples in wind sector Δ
θ and wind speed Δ
u with concentrations higher than threshold
x.
nΔθ,Δu denotes the total number of samples for the corresponding wind sector and wind speed interval. The threshold used for CBPF calculations was the same as that used for the 3D-PSCF model, as described in Section 2.3.1. Data for the wind speed and wind direction in the study area were obtained from the Korean Meteorological Administration (https://web.kma.go.kr). Details about the meteorological stations can be found in our previous study.
25 Additionally, polar concentration plots, showing the PAH concentrations associated with wind speed and direction, were considered to more understand the wind effects on the variation in PAH concentrations. The R program, downloaded from https://www.r-project.org, and the openair package
43 were employed to calculate and plot the CBPF and polar concentrations.
3. Results and discussion
Inputs for the 3D-PSCF/3D-CWT models and CBPF are concentrations of ∑13 PAHs, concentration threshold, backward trajectories, and weighting functions. Of these variables, seasonal mean concentrations of gaseous, particulate, and total (gaseous + particulate) PAHs are illustrated in Fig. S2.† Additionally, seasonal fractions of PAHs by ring number associated with wind speed and wind direction are shown in Fig. S3.† Discussion on the concentrations and fractions of PAHs can be seen in Text S2.†
To determine the optimal weighting function and concentration threshold for subsequent analysis, the 3D-PSCF and 3D-CWT models were first run with two weighting functions (i.e., W1 and W2) and three thresholds (i.e., the 50th, 75th, and 90th percentiles of ∑13 PAHs). Based on the results of source areas suggested by each threshold and weighting function, the 75th percentile and weighting function W1 were selected for further modeling. More details on this comparison are provided in Text S3.† The 3D-PSCF and 3D-CWT models identified relatively consistent source areas for particulate and total PAHs, possibly because the PSCF and CWT models employ a similar approach based on air trajectories and PAH concentrations at the receptor site to determine source areas.
3.1. Comparison of conventional and 3D hybrid receptor models
PSCF and CWT anomalies, which reflect the differences between the conventional and 3D-hybrid receptor models, are illustrated in Fig. 2. Areas with highly positive anomalous values are likely to have been overestimated as emission source areas by the conventional PSCF/CWT. The PAHs emitted in these areas could not have substantially affected the receptor site because the air parcels passing across these areas were higher than the mixing layer. The PSCF and CWT anomalies for summer and fall were not considered because air pollution in South Korea in these seasons was mainly affected by local emission sources.14,24
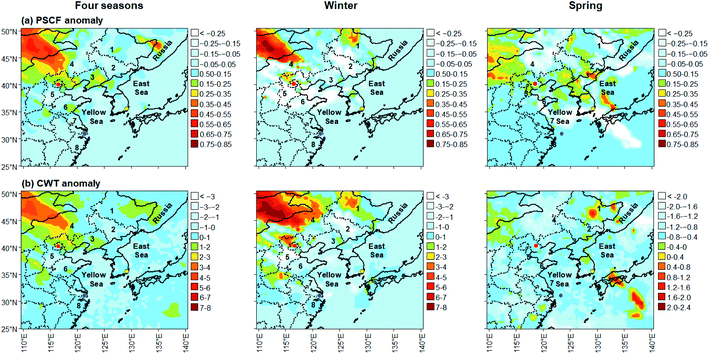 |
| Fig. 2 PSCF and CWT anomalies at the 95% upper confidence levels for ∑13 particulate PAHs. The concentrations of ∑13 PAHs for the four seasons were used. The names of the Chinese provinces and cities marked by numbers and colored circles are listed in Fig. S1.† | |
The PSCF and CWT anomalies were higher in Mongolia and Inner Mongolia (Fig. 2), indicating that emissions from these areas may not considerably contribute to PAH pollution in Ulsan. The pressure of the air parcels in Inner Mongolia and Mongolia was low (i.e., <800 hPa) (Fig. S5†), which would lead to the upward movement of air masses (Fig. S6†) (i.e., to 4000 m height). There are a number of meteorological explanations for this upward movement of the air parcels, including the expansion of cold surges from Mongolia and Siberia44,45 associated with the low-pressure system (<800 hPa)46 in northern and northeastern China (e.g., Inner Mongolia, Jilin, and Liaoning) in winter (e.g., January–February) and the warm conveyor belt in spring (e.g., March–May).47,48 In addition, wind in the higher atmospheric layer (e.g., at 4000 m height) in Northeast Asia moves rapidly toward the east (Fig. S7†), thus tending to transport PAHs to the Pacific Ocean,45 meaning that they have an insignificant effect on downwind areas in Northeast Asia, including the Korean Peninsula. For this reason, the 3D hybrid receptor models can more accurately predict emission source areas than can the conventional models.
3.2. Local emission sources of PAHs
3.2.1. Gaseous PAHs.
In summer and fall, the CBPF plots exhibited high probabilities for high concentrations at relatively low wind speeds (<3 m s−1) (Fig. 3a), indicating that gaseous species may primarily derive from local emissions. The dispersion of PAHs under these wind speeds is likely to cover a relatively small area (e.g., a few kilometers). In addition, the prevailing wind direction in Ulsan during summer and fall is southeasterly and northeasterly, respectively, moving from the eastern areas of the city and passing over the industrial and urban areas prior to reaching the sampling site (Fig. 1). The petrochemical and non-ferrous industrial complexes located in the eastern region of Ulsan are important emission sources for PAHs due to coal/coke combustion and the use of heavy oil.25,49 Thus, gaseous PAHs produced by this industrial area could clearly affect the sampling site. The higher concentrations of gaseous PAHs associated with southeasterly and northeasterly winds in summer and fall, respectively (Fig. 3b and S8†), could support this suggestion.
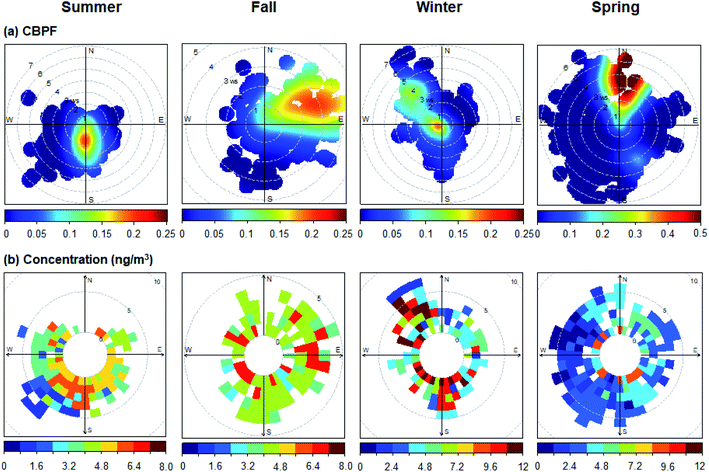 |
| Fig. 3 Plots for (a) CBPF and (b) polar concentrations of gaseous PAHs in summer, fall, winter, and spring. The concentrations of ∑13 PAHs were used as input data. | |
CBPF analysis for gaseous PAHs in winter suggested a source to the northwest of the sampling site associated with medium wind speeds (3–4 m s−1) (Fig. 3a). The gaseous PAHs transported from this direction would combine with PAHs emitted from other areas. Additionally, the CBPF plot for the gas phase in winter also identified a common ground source (e.g., vehicle emissions) (Fig. 3a) associated with low wind speeds (<1 m s−1). Because the CBPF probability of this source (0.25) was higher than that of the distant source (0.10–0.15), the influence of local sources on gaseous PAHs may be more important in Ulsan during winter. In particular, the levels of 3-ring PAHs increased at low wind speeds (Fig. S8a†), indicating that the ground level sources could have a stronger influence on light compounds in the gaseous phase.
The CBPF plot for spring showed a high probability for high gaseous concentrations to the north of the sampling site associated with low to high wind speeds (2–6 m s−1) (Fig. 3a). Low wind speeds generally disperse air pollutants on a local scale, while high wind speeds increase the pollutant dispersion and contribute to their transport to distant areas. Thus, the gaseous PAHs in spring could derive from both common ground-level (e.g., vehicle exhaust) and distant (e.g., long- or middle-range transport) sources located to the north of the sampling site. The photochemical reactions of gaseous PAHs with free oxidants (e.g., OH, O3, and NO3),2 which tend to increase in spring,50 could promote the degradation of gaseous PAHs in the atmosphere. Thus, the ground emission sources (e.g., vehicle emission) could more contribute to an increase in gaseous concentrations during spring. This interpretation is supported by the higher concentrations of gaseous PAHs (Fig. 3b) and 3- to 4-ring species (Fig. S8†) associated with the low wind speeds (<1 m s−1). Additionally, the CBPF and polar plots for winter and spring exhibited high probabilities and concentrations at relatively different directions (Fig. 3) because concentrations higher than 75th percentile values were used for the CBPF plot, while the whole PAH dataset was used for the polar plot.
3.2.2. Particulate PAHs.
In summer and fall, local emissions are more likely to affect particulate PAH levels in Ulsan because the CBPF plots for these seasons exhibited high probabilities at relatively low (<3 m s−1) wind speeds (Fig. 4a). Low wind speeds reduce dispersion and prolong the local residence of air pollutants. The primary local emission source in summer and fall is likely to be the industrial area in eastern Ulsan. Industrial activity, especially petrochemical and non-ferrous production, would be a primary contributor to PAHs in Ulsan during summer and fall due to the monsoon system and its prevailing southeasterly winds. The higher concentrations of particulate PAHs, especially 5- and 6-ring species, were also associated with southeasterly winds in summer and fall (Fig. 4b, S9c and d†). Moreover, the CBPF plots for fall showed high probabilities for high particulate concentrations to the southwest of the receptor site associated with relatively low wind speeds (<3 m s−1). Particulate PAHs transported from this direction might be contributed by emission sources located in the proximity of Ulsan, such as several industrial complexes and commercial areas on the south coast, as well as open burning following harvesting.51
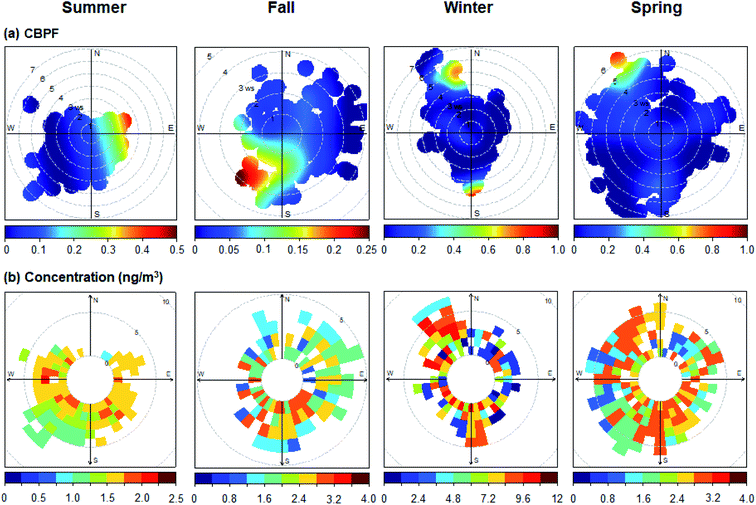 |
| Fig. 4 Plots for (a) CBPF and (b) polar concentration of particulate PAHs in summer, fall, winter, and spring. The concentrations of ∑13 PAHs were used as input data. | |
In winter, two sources located to the south and northwest of the sampling site associated with high wind speeds (5–6 m s−1) were subsequently identified (Fig. 4a). The southern source is assumed to be stack emissions11 from the industrial complexes located in the east and southeast of Ulsan. The prevailing wind direction in Ulsan during winter is northwesterly, leading to the transport of PAHs from the industrial area to the East Sea (Fig. 1). However, the low mixing height and increased fossil fuel burning in winter enhanced PAH concentrations at a local scale (Fig. S2†). Moreover, the influence of the land-sea breeze cannot be ignored. Additionally, markers of heavy oil combustion, including 4- to 6-ring PAHs (e.g., Flt, Pyr, BaP, Ind, and BghiP),52 also exhibited high concentrations associated with southeasterly winds (Fig. S9†), carrying the particulate PAHs from the industrial and residential areas of Ulsan. The other source in the northwest could be a distant source with contributions from non-local and regional emissions (e.g., long-range transport from outside Korea and/or transport from other areas in South Korea) because the concentrations of particulate PAHs with long lifetimes (i.e., 4- to 6-ring species) increased at high wind speeds (4–6 m s−1) blowing from the northwest (Fig. 4b and S9†). A detailed discussion on the long-range transport of particulate PAHs is presented in Section 3.3.
CBPF analysis for particulate PAHs in spring identified an emission source to the northwest associated with high wind speeds (5–6 m s−1) (Fig. 4a). Because high wind speeds more readily disperse air pollutants, this source is assumed to be a non-local or distant source,53 such as long-range transport from outside South Korea and/or transport from other areas in South Korea that the air parcels pass over before reaching the receptor site. The non-local emission sources of particulate PAHs are more discussed in the next section.
3.3. Long-range transport of particulate PAHs
3.3.1. 3D-PSCF and 3D-CWT models.
As discussed in Section 3.2, the particulate PAHs in Ulsan might be contributed by those emitted from non-local sources, especially in spring and winter. In this section, therefore, the hybrid receptor models (i.e., 3D-PSCF and 3D-CWT) were only applied for the particulate PAH data to identify their emission source areas on a non-local scale. In winter, both the 3D-PSCF and 3D-CWT models identified Liaoning, Hebei, and Shandong as potential source areas for particulate PAHs in Ulsan (Fig. 5a). An increase in PAH emission (Fig. S11†) combined with higher aerosol levels in these provinces during winter, especially in February (Fig. S10†), could lead to higher levels of particulate PAHs via coagulation and/or condensation.54 Particulate PAHs, especially 4- to 6-ring species, are known to sorb to the organic matter in fine and coarse particles with an aerodynamic diameter smaller than 2.5 μm and 10 μm, respectively.55 These particles can be removed from the air by dry and wet deposition (i.e., in-cloud and below-cloud deposition).56 However, the absence of rainfall when air parcels pass over China in winter (Fig. S13d†) could prolong the residence of particulate PAHs in the atmosphere and enhance the long-range transport of particulate PAHs emitted from northern and eastern China. Moreover, PAHs partitioned to fine particles through physicochemical processes (e.g., coagulation and condensation) could exhibit low volatility and high viscosity,57,58 which protect the sorbed PAHs from degradation and promote the long-range transport of particle-bound PAHs to downwind areas.
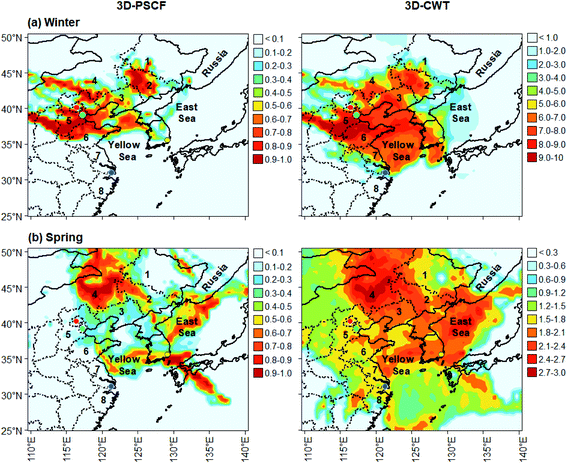 |
| Fig. 5 Bootstrapped 3D-PSCF and 3D-CWT results for particulate PAHs at the 95% upper confidence levels in (a) winter and (b) spring. The concentrations of ∑13 PAHs were used as input data. | |
In spring, it is possible that particulate PAHs from eastern China (e.g., Shandong and Jiangsu), northern China (e.g., Hebei), and northeastern China (e.g., Jilin and Liaoning) are transported to Ulsan (Fig. 5b). This observation was emphasized more strongly by the 3D-CWT model, which determines source areas based on the residence time of air trajectories and can distinguish the intensity (i.e., strong, moderate, or weak) of the emission sources.7 In addition, the movement of particulate PAHs from China appears to be stronger for northern China (e.g., Beijing, Tianjin, and Hebei), and this may be associated with the higher concentration of aerosols (Fig. S10†) as well as the larger emissions of particulate PAHs (Fig. S11†). Particulate PAHs could then be transported to downwind areas (e.g., Ulsan) by westerly winds. Moreover, air parcels coming from the north of China were dominant (30% and 25%) (Fig. S12d†), meaning that particulate PAHs from these areas may make a higher contribution to the levels found at the receptor site. Previous studies12,59 have also reported that eastern and northern China (35°–40°N, including Shandong, Beijing, Tianjin, and Hebei) contributes more to particulate PAH levels in South Korea than does northeastern China (>40°N, including Liaoning, Jilin, and Heilongjiang).
3.3.2. Fraction-weighted trajectory.
The outputs of hybrid receptor models (i.e., PSCF and CWT models) can be affected by several variables. For instance, the simulation of backward trajectories with longer travel distance, concentration threshold, and equal weighting function along the trajectory paths may cause a misinterpretation of emission source areas.60–62 In addition, chemical reactions, gas/particle partitioning, and emission inventory, affecting pollutant concentrations at the receptor site, are not considered in the PSCF and CWT models.
To compensate for the shortcomings of the 3D-PSCF and 3D-CWT models, the FWT, a combination of hybrid receptor and gas/particle partitioning models, was employed (Fig. 6). Generally, areas with higher FWT values (i.e., close to 1.0) make a greater contribution to an increase in particulate PAHs at a receptor site. For spring and winter, the FWT confirmed relatively similar source areas to the result of the 3D-PSCF and 3D-CWT models. That is, eastern China (e.g., Shandong), northern China (e.g., Hebei and Tianjin), and northeastern China (e.g., Liaoning) could be major contributors to an increase in particulate PAHs in Ulsan (Fig. 6c and d). In addition, for spring, the FWT values were high in southeastern Japan (0.7–0.8) but lower in Ulsan (0.4–0.5), suggesting that PAHs originated from Japan might not contribute to an increase in particulate PAHs in Ulsan.
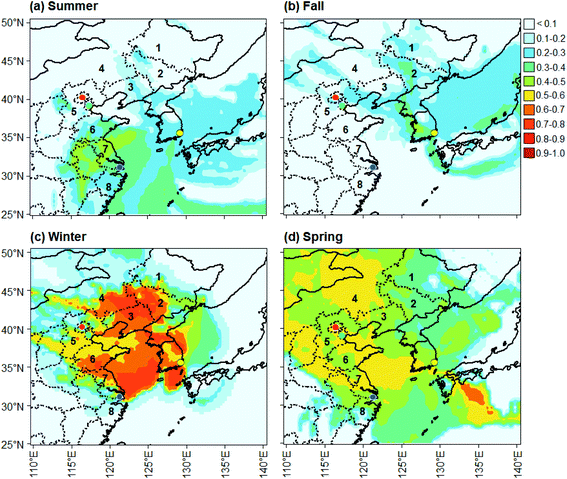 |
| Fig. 6 Bootstrapped FWT results for PAHs at the 95% upper confidence levels in (a) summer, (b) fall, (c) winter, and (d) spring. | |
In summer, the FWT values were higher for eastern China (e.g., Shanghai, Jiangsu, and Henan) but lower for the Korean Peninsula (Fig. 6a). This suggests that the concentration of particulate PAHs may fall during their transport from emission sources in eastern China to the receptor site in Ulsan. The higher rainfall (Fig. S13a†) and solar radiation in summer, corresponding to wet deposition and photochemical degradation, could explain this observation. In addition, the FWT confirmed that particulate PAHs emitted from western Korea are likely to be transported to the sampling site in fall (Fig. 6b). However, the FWT results in summer and fall (<0.5) were appreciably lower than the maximal value (1.0), suggesting that non-local sources have less influence on particle-bound PAHs during these seasons. These results are also in line with those confirmed by the CBPF in Section 3.2, indicating that local emissions contributed more to particulate PAH levels in Ulsan during summer and fall. In fact, the long-range transport of air pollutants from upwind (e.g., China and North Korea) to downwind areas (e.g., South Korea and Japan) in Northeast Asia has also been reported to weaken in summer and fall (e.g., June–October).12,45,63
4. Conclusion
This study suggests that the mixing height and vertical dispersion of air pollutants should be considered when using hybrid receptor models (e.g., the PSCF and CWT models) to identify emission source areas. In particular, the 3D-PSCF and 3D-CWT models, which reflect the vertical dispersion of PAHs, employed in the present study are able to more accurately identify source areas than conventional PSCF and CWT models. For example, the 3D models predict that Inner Mongolia and Mongolia are unlikely to be major source areas for PAHs in Ulsan because the PAHs emitted in these areas will tend to disperse into the upper-tropospheric layer and have much lower contributions to those PAHs measured in Ulsan.
It is found that particulate PAHs in Ulsan are likely to be affected by long-range transport from China, while gaseous PAHs are primarily emitted from the local emissions (e.g., industrial and vehicle emissions). In particular, local emission sources are the primary contributors to gaseous and particulate PAHs in summer and fall. However, in spring and winter, particulate PAHs originating from eastern, northern, and northeastern China can be transported to the receptor site in Ulsan.
The present study also employed the FWT for the first time to verify the emission source areas identified by the 3D-PSCF and 3D-CWT models. The FWT was able to confirm the contribution of regional sources to the increase in particulate PAHs at the receptor site, especially in summer and fall when PAHs degraded more rapidly during transport. However, this approach should be studied further to understand its influence on the identification of source areas.
Conflicts of interest
There are no conflicts to declare.
Acknowledgements
This study was supported by the National Research Foundation of Korea (NRF) grant funded by the Korean Government (MEST) (2020R1A2B5B01002669, 2020R1A6A1A03040570) and the Korea Rural Development Administration (PJ014297). This work was also supported by the Korea Institute of Energy Technology Evaluation and Planning (KETEP) through “Human Resources Program in Energy Technology” (No. 20164030201010/No. 20184030202250).
References
-
D. Mackay, W. Y. Shui, K.-C. Ma and S. C. Lee, Handbook of physical-chemical properties and environmental fate for organic chemicals. Volume I: Introduction and hydrocarbons, Taylor & Francis, 2006 Search PubMed.
- I. J. Keyte, R. M. Harrison and G. Lammel, Chemical reactivity and long-range transport potential of polycyclic aromatic hydrocarbons – A review, Chem. Soc. Rev., 2013, 42, 9333–9391 RSC.
- M. Shiraiwa, Y. Li, A. P. Tsimpidi, V. A. Karydis, T. Berkemeier, S. N. Pandis, J. Lelieveld, T. Koop and U. Pöschl, Global distribution of particle phase state in atmospheric secondary organic aerosols, Nat. Commun., 2017, 8, 15002 CrossRef.
- P. Luo, H.-G. Ni, L.-J. Bao, S.-M. Li and E. Y. Zeng, Size distribution of airborne particle-bound polybrominated diphenyl ethers and its implications for dry and wet deposition, Environ. Sci. Technol., 2014, 48, 13793–13799 CrossRef CAS.
- P. G. Simmonds, S. Seuring, G. Nickless and R. G. Derwent, Segregation and interpretation of ozone and carbon monoxide measurements by air mass origin at the TOR station Mace Head, Ireland from 1987 to 1995, J. Atmos. Chem., 1997, 28, 45–59 CrossRef CAS.
- L. L. Ashbaugh, A statistical trajectory technique for determining air pollution source regions, J. Air Pollut. Control Assoc., 1983, 33, 1096–1098 CrossRef.
- Z. L. Fleming, P. S. Monks and A. J. Manning, Review: Untangling the influence of air-mass history in interpreting observed atmospheric composition, Atmos. Res., 2012, 104, 1–39 CrossRef.
- A. V. Polissar, P. K. Hopke and J. M. Harris, Source regions for atmospheric aerosol measured at Barrow, Alaska, Environ. Sci. Technol., 2001, 35, 4214–4226 CrossRef CAS.
- W. Wimolwattanapun, P. K. Hopke and P. Pongkiatkul, Source apportionment and potential source locations of PM2.5 and PM2.5–10 at residential sites in metropolitan Bangkok, Atmos. Pollut. Res., 2011, 2, 172–181 CrossRef CAS.
- E. Kim and P. K. Hopke, Comparison between conditional probability function and nonparametric regression for fine particle source directions, Atmos. Res., 2004, 38, 4667–4673 CrossRef CAS.
- I. Uria-Tellaetxe and D. C. Carslaw, Conditional bivariate probability function for source identification, Environ. Model. Software, 2014, 59, 1–9 CrossRef.
- Y. Inomata, M. Kajino, K. Sato, J. Kurokawa, N. Tang, T. Ohara, K. Hayakawa and H. Ueda, Source–receptor relationship analysis of the atmospheric deposition of PAHs subject to long-range transport in Northeast Asia, Environ. Sci. Technol., 2017, 51, 7972–7981 CrossRef CAS.
- S. Tamamura, T. Sato, Y. Ota, X. Wang, N. Tang and K. Hayakawa, Long-range transport of polycyclic aromatic hydrocarbons (PAHs) from the eastern Asian continent to Kanazawa, Japan with Asian dust, Atmos. Res., 2007, 41, 2580–2593 CrossRef CAS.
- B. M. Kim, S.-B. Lee, J. Y. Kim, S. Kim, J. Seo, G.-N. Bae and J. Y. Lee, A multivariate receptor modeling study of air-borne particulate PAHs: Regional contributions in a roadside environment, Chemosphere, 2016, 144, 1270–1279 CrossRef CAS.
- U. Jeong, J. Kim, H. Lee, J. Jung, Y. J. Kim, C. H. Song and J.-H. Koo, Estimation of the contributions of long range transported aerosol in East Asia to carbonaceous aerosol and PM concentrations in Seoul, Korea using highly time resolved measurements: A PSCF model approach, J. Environ. Monit., 2011, 13, 1905–1918 RSC.
- U. Jeong, J. Kim, H. Lee and Y. G. Lee, Assessing the effect of long-range pollutant transportation on air quality in Seoul using the conditional potential source contribution function method, Atmos. Res., 2017, 150, 33–44 CrossRef CAS.
- K. Miura, K. Shimada, T. Sugiyama, K. Sato, A. Takami, C. K. Chan, I. S. Kim, Y. P. Kim, N.-H. Lin and S. Hatakeyama, Seasonal and annual changes in PAH concentrations in a remote site in the Pacific Ocean, Sci. Rep., 2019, 9, 12591 CrossRef.
- I. S. Kim, D. Wee, Y. P. Kim and J. Y. Lee, Development and application of three-dimensional potential source contribution function (3D-PSCF), Environ. Sci. Pollut. Res., 2016, 23, 16946–16954 CrossRef CAS.
- F.-J. Zhu, W.-L. Ma, P.-T. Hu, Z.-F. Zhang and Y.-F. Li, Temporal trends of atmospheric PAHs: Implications for the influence of the clean air action, J. Cleaner Prod., 2021, 296, 126494 CrossRef CAS.
- K. Hayakawa, N. Tang, E. Nagato, A. Toriba, J.-M. Lin, L. Zhao, Z. Zhou, W. Qing, X. Yang, V. Mishukov, A. Neroda and H.-Y. Chung, Long-term trends in urban atmospheric polycyclic aromatic hydrocarbons and nitropolycyclic aromatic hydrocarbons: China, Russia, and Korea from 1999 to 2014, Int. J. Environ. Res. Public Health, 2020, 17, 431 CrossRef CAS.
- S. Han, J. Y. Lee, J. Heo and Y. P. Kim, Temporal trend of the major contributors for the particulate polycyclic aromatic hydrocarbons (PAHs) in Seoul, Aerosol Air Qual. Res., 2019, 19, 318–330 CrossRef CAS.
- A. Stojić and S. Stanišić Stojić, The innovative concept of three-dimensional hybrid receptor modeling, Atmos. Res., 2017, 164, 216–223 CrossRef.
- I. S. Kim, J. Y. Lee, D. Wee and Y. P. Kim, Estimation of the contribution of biomass fuel burning activities in North Korea to the air quality in Seoul, South Korea: Application of the 3D-PSCF method, Atmos. Res., 2019, 230, 104628 CrossRef CAS.
- Q. T. Vuong, P. Q. Thang, T. N. T. Nguyen, T. Ohura and S.-D. Choi, Seasonal variation and gas/particle partitioning of atmospheric halogenated polycyclic aromatic hydrocarbons and the effects of meteorological conditions in Ulsan, South Korea, Environ. Pollut., 2020, 263, 114592 CrossRef CAS.
- T. N. T. Nguyen, K.-S. Jung, J. M. Son, H.-O. Kwon and S.-D. Choi, Seasonal variation, phase distribution, and source identification of atmospheric polycyclic aromatic hydrocarbons at a semi-rural site in Ulsan, South Korea, Environ. Pollut., 2018, 236, 529–539 CrossRef.
- Q. Wang, M. Liu, Y. Yu and Y. Li, Characterization and source apportionment of PM2.5-bound polycyclic aromatic hydrocarbons from Shanghai city, China, Environ. Pollut., 2016, 218, 118–128 CrossRef CAS.
- J. Kim, S.-C. Yoon, A. Jefferson and S.-W. Kim, Aerosol hygroscopic properties during Asian dust, pollution, and biomass burning episodes at Gosan, Korea in April 2001, Atmos. Res., 2006, 40, 1550–1560 CrossRef CAS.
- P. K. Hopke, N. Gao and M.-D. Cheng, Combining chemical and meteorological data to infer source areas of airborne pollutants, Chemom. Intell. Lab. Syst., 1993, 19, 187–199 CrossRef CAS.
- Y.-J. Han, T. M. Holsen and P. K. Hopke, Estimation of source locations of total gaseous mercury measured in New York State using trajectory-based models, Atmos. Res., 2007, 41, 6033–6047 CrossRef CAS.
- M. Zhao, Z. Huang, T. Qiao, Y. Zhang, G. Xiu and J. Yu, Chemical characterization, the transport pathways and potential sources of PM2.5 in Shanghai: Seasonal variations, Atmos. Res., 2015, 158–159, 66–78 CrossRef CAS.
- Z. Zong, X. Wang, C. Tian, Y. Chen, S. Fu, L. Qu, L. Ji, J. Li and G. Zhang, PMF and PSCF based source apportionment of PM2.5 at a regional background site in North China, Atmos. Res., 2018, 203, 207–215 CrossRef CAS.
- I. Hwang and P. K. Hopke, Estimation of source apportionment and potential source locations of PM2.5 at a west coastal IMPROVE site, Atmos. Res., 2007, 41, 506–518 CrossRef CAS.
- S.-O. Lai, T. M. Holsen, P. K. Hopke and P. Liu, Wet deposition of mercury at a New York state rural site: Concentrations, fluxes, and source areas, Atmos. Res., 2007, 41, 4337–4348 CrossRef CAS.
- Y.-K. Hsu, T. M. Holsen and P. K. Hopke, Locating and quantifying PCB sources in Chicago: Receptor modeling and field sampling, Environ. Sci. Technol., 2003, 37, 681–690 CrossRef CAS.
- Y. Q. Wang, X. Y. Zhang and R. R. Draxler, TrajStat: GIS-based software that uses various trajectory statistical analysis methods to identify potential sources from long-term air pollution measurement data, Environ. Model. Software, 2009, 24, 938–939 CrossRef.
- A. Lupu and W. Maenhaut, Application and comparison of two statistical trajectory techniques for identification of source regions of atmospheric aerosol species, Atmos. Res., 2002, 36, 5607–5618 CrossRef CAS.
- P. K. Hopke, C. L. Li, W. Ciszek and S. Landsberger, The use of bootstrapping to estimate conditional probability fields for source locations of airborne pollutants, Chemom. Intell. Lab. Syst., 1995, 30, 69–79 CrossRef CAS.
- S. Squizzato and M. Masiol, Application of meteorology-based methods to determine local and external contributions to particulate matter pollution: A case study in Venice (Italy), Atmos. Res., 2015, 119, 69–81 CrossRef CAS.
- Y. Zhang, J. Chen, H. Yang, R. Li and Q. Yu, Seasonal variation and potential source regions of PM2.5-bound PAHs in the megacity Beijing, China: Impact of regional transport, Environ. Pollut., 2017, 231, 329–338 CrossRef CAS.
- R. Zhang, G. Wang, S. Guo, M. L. Zamora, Q. Ying, Y. Lin, W. Wang, M. Hu and Y. Wang, Formation of urban fine particulate matter, Chem. Rev., 2015, 115, 3803–3855 CrossRef CAS.
- M. G. Perrone, S. Vratolis, E. Georgieva, S. Török, K. Šega, B. Veleva, J. Osán, I. Bešlić, Z. Kertész, D. Pernigotti, K. Eleftheriadis and C. A. Belis, Sources and geographic origin of particulate matter in urban areas of the Danube macro-region: The cases of Zagreb (Croatia), Budapest (Hungary) and Sofia (Bulgaria), Sci. Total Environ., 2018, 619–620, 1515–1529 CrossRef CAS.
- H. Zhou, P. K. Hopke, C. Zhou and T. M. Holsen, Ambient mercury source identification at a New York State urban site: Rochester, New York, Sci. Total Environ., 2019, 650, 1327–1337 CrossRef CAS.
- D. C. Carslaw and K. Ropkins, Openair – An R package for air quality data analysis, Environ. Model. Software, 2012, 27–28, 52–61 CrossRef.
- Q. Liang, L. Jaeglé and J. M. Wallace, Meteorological indices for Asian outflow and transpacific transport on daily to interannual timescales, J. Geophys. Res.: Atmos., 2005, 110, D18308 CrossRef.
- Y. Zhang, S. Tao, J. Ma and S. Simonich, Transpacific transport of benzo[a]pyrene emitted from Asia, Atmos. Chem. Phys., 2011, 11, 11993–12006 CrossRef CAS.
- H.-R. Oh, C.-H. Ho, J. Kim, D. Chen, S. Lee, Y.-S. Choi, L.-S. Chang and C.-K. Song, Long-range transport of air pollutants originating in China: A possible major cause of multi-day high-PM10 episodes during cold season in Seoul, Korea, Atmos. Res., 2015, 109, 23–30 CrossRef CAS.
- Y. Zhang, H. Shen, S. Tao and J. Ma, Modeling the atmospheric transport and outflow of polycyclic aromatic hydrocarbons emitted from China, Atmos. Res., 2011, 45, 2820–2827 CrossRef CAS.
- Q. Mu, M. Shiraiwa, M. Octaviani, N. Ma, A. Ding, H. Su, G. Lammel, U. Pöschl and Y. Cheng, Temperature effect on phase state and reactivity controls atmospheric multiphase chemistry and transport of PAHs, Sci. Adv., 2018, 4, eaap7314 CrossRef.
- S.-D. Choi, H.-O. Kwon, Y.-S. Lee, E.-J. Park and J.-Y. Oh, Improving the spatial resolution of atmospheric polycyclic aromatic hydrocarbons using passive air samplers in a multi-industrial city, J. Hazard. Mater., 2012, 241–242, 252–258 CrossRef CAS.
- K. Clarke, H.-O. Kwon and S.-D. Choi, Fast and reliable source identification of criteria air pollutants in an industrial city, Atmos. Res., 2014, 95, 239–248 CrossRef CAS.
- S. Y. Ryu, J. E. Kim, H. Zhuanshi, Y. J. Kim and G. U. Kang, Chemical composition of post-harvest biomass burning aerosols in Gwangju, Korea, J. Air Waste Manage. Assoc., 2004, 54, 1124–1137 CrossRef CAS.
- H.-H. Yang and C.-M. Chen, Emission inventory and sources of polycyclic aromatic hydrocarbons in the atmosphere at a suburban area in Taiwan, Chemosphere, 2004, 56, 879–887 CrossRef CAS.
- M. Masiol, S. Squizzato, M.-D. Cheng, D. Q. Rich and P. K. Hopke, Differential probability functions for investigating long-term changes in local and regional air pollution sources, Aerosol Air Qual. Res., 2019, 19, 724–736 CrossRef CAS.
- A. Indarto, A. Giordana, G. Ghigo, A. Maranzana and G. Tonachini, Polycyclic aromatic hydrocarbon formation mechanism in the “particle phase”: A theoretical study, Phys. Chem. Chem. Phys., 2010, 12, 9429–9440 RSC.
- S. S. Park, Y. J. Kim and C. H. Kang, Polycyclic aromatic hydrocarbons in bulk PM2.5 and size-segregated aerosol particle samples measured in an urban environment, Environ. Monit. Assess., 2007, 128, 231–240 CrossRef CAS.
- S. O. Baek, M. E. Goldstone, P. W. W. Kirk, J. N. Lester and R. Perry, Phase distribution and particle size dependency of polycyclic aromatic hydrocarbons in the urban atmosphere, Chemosphere, 1991, 22, 503–520 CrossRef CAS.
- M. Shrivastava, S. Lou, A. Zelenyuk, R. C. Easter, R. A. Corley, B. D. Thrall, P. J. Rasch, J. D. Fast, S. L. Massey Simonich, H. Shen and S. Tao, Global long-range transport and lung cancer risk from polycyclic aromatic hydrocarbons shielded by coatings of organic aerosol, Proc. Natl. Acad. Sci. U. S. A., 2017, 114, 1246–1251 CrossRef CAS.
- A. Zelenyuk, D. G. Imre, J. Wilson, D. M. Bell, K. J. Suski, M. Shrivastava, J. Beránek, M. L. Alexander, A. L. Kramer and S. L. Massey Simonich, The effect of gas-phase polycyclic aromatic hydrocarbons on the formation and properties of biogenic secondary organic aerosol particles, Faraday Discuss., 2017, 200, 143–164 RSC.
- Y. Inomata, M. Kajino, K. Sato, T. Ohara, J.-I. Kurokawa, H. Ueda, N. Tang, K. Hayakawa, T. Ohizumi and H. Akimoto, Emission and atmospheric transport of particulate PAHs in Northeast Asia, Environ. Sci. Technol., 2012, 46, 4941–4949 CrossRef CAS.
- I. Cheng, X. Xu and L. Zhang, Overview of receptor-based source apportionment studies for speciated atmospheric mercury, Atmos. Chem. Phys., 2015, 15, 7877–7895 CrossRef CAS.
- X. Fang, T. Saito, S. Park, S. Li, Y. Yokouchi and R. G. Prinn, Performance of back-trajectory statistical methods and inverse modeling method in locating emission sources, ACS Earth Space Chem., 2018, 2, 843–851 CrossRef CAS.
- U. Jeong, H. Lee, J. Kim, W. Kim, H. Hong and C. K. Song, Determination of the inter-annual and spatial characteristics of the contribution of long-range transport to SO2 levels in Seoul between 2001 and 2010 based on conditional potential source contribution function (CPSCF), Atmos. Res., 2013, 70, 307–317 CrossRef CAS.
- P. Bhardwaj, S. J. Ki, Y. H. Kim, J. H. Woo, C. K. Song, S. Y. Park and C. H. Song, Recent changes of trans-boundary air pollution over the Yellow Sea: Implications for future air quality in South Korea, Environ. Pollut., 2019, 247, 401–409 CrossRef CAS.
Footnote |
† Electronic supplementary information (ESI) available. See DOI: 10.1039/d1em00320h |
|
This journal is © The Royal Society of Chemistry 2022 |