DOI:
10.1039/C8AN01572D
(Critical Review)
Analyst, 2019,
144, 114-129
Molecular methods in electrochemical microRNA detection
Received
14th August 2018
, Accepted 22nd October 2018
First published on 26th October 2018
Abstract
High-throughput profiling/sensing of nucleic acids has recently emerged as a highly promising strategy for the early diagnosis and improved prognosis of a broad range of pathologies, most notably cancer. Among the potential biomarker candidates, microRNAs (miRNAs), a class of non-coding RNAs of 19–25 nucleotides in length, are of particular interest due to their role in the post-transcriptional regulation of gene expression. Developing miRNA sensing technologies that are quantitative, ultrasensitive and highly specific has proven very challenging because of their small size, low natural abundance and the high degree of sequence similarity among family members. When compared to optical based methods, electrochemical sensors offer many advantages in terms of sensitivity and scalability. This non-comprehensive review aims to break-down and highlight some of the most promising strategies for electrochemical sensing of microRNA biomarkers.
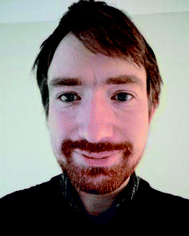 Philip Gillespie | Philip Gillespie MPharm is a PhD student in the Department of Bioengineering at Imperial College London. Prior to this, he received his MPharm Degree at the University of East Anglia in 2015 and became a professionally qualified pharmacist in 2016. His research interests are in nucleic acid structure, biosensing, and simulation. |
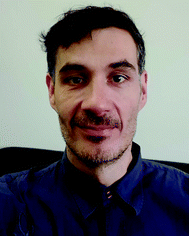 Sylvain Ladame | Dr Sylvain Ladame (FRSC) received his PhD in Chemistry of Biomolecules from the University of Toulouse (France) in 2001 before moving to Cambridge (U.K.) to work for five years as a post-doctoral researcher in the group of Sir Prof. Shankar Balasubramanian. In 2006, he started his independent research career as a junior group leader with the Institute of Science and Supramolecular Engineering (ISIS, Strasbourg, France) before being appointed lecturer at Imperial College London in 2010 where he is currently employed as a senior lecturer in biosensor development within the Department of Bioengineering. SL has worked in the field of nucleic acids for over fifteen years and now specialises in the engineering and validation of novel non-invasive and low-cost diagnostic tests based on the detection of circulating, cell-free nucleic acids (DNA and RNA) in liquid biopsies. Applications range from early diagnosis and improved prognosis of prostate cancer to early prediction of pre-term birth. SL has published over 50 papers and has two patents to his name. |
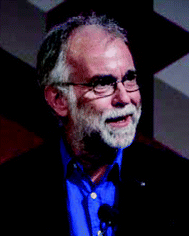 Danny O'Hare | Dr Danny O'Hare FRSC graduated in chemistry from Imperial College. He then undertook doctoral work on the development of electrochemical sensors for biomedical applications in the Physiological Flow Studies Unit at Imperial. After postdoctoral work he took his first academic position in the School of Pharmacy, University of Brighton as senior lecturer in analytical sciences. He took up his current appointment in the Department of Bioengineering, Imperial College in 2001 where he is currently reader in sensor research. His research interests are in electrochemical sensors in biomedicine, microfabrication, diagnostics and environmental analysis. |
Introduction
MicroRNAs (miRNAs) are short non-coding, single-stranded sequences of RNA, approximately 19–25 nucleotides long, with length and sequence variation.1 They are found intra and extra cellularly in plants, animals and humans. Specific alterations in their expression profiles have been correlated with many diseases, including cancer, fitting the IUPAC definition of biomarkers.2–11 Cost-effective technologies for blood-based, sensitive and specific detection of miRNA biomarkers could potentially transform the way cancer is diagnosed and treated, assuming they are compatible with widespread public screening.12 In diagnostics, short times to results (TTR) arising from point of care testing (POCT) have been found to improve patient outcomes.13 Comparable molecular techniques can be used in both optical and electrochemical detection, often leading to similar limits of detection and dynamic ranges.14,15
The clear majority of clinically used POCT methods (most notably blood glucose monitoring) are based on electrochemical detection. This is mainly due to enhanced scalability of readout devices in comparison to optical-based detection methods. This review therefore focuses on electrochemical techniques associated with microRNA detection.
Electrochemical methods encompass a broad variety of measurements that can be made on a system. A detailed explanation of each is beyond the scope of this review and there are many good primers on them.16–19 The IUPAC definition of electrochemical biosensors identifies four signal transduction types: amperometric, potentiometric, impedimetric, and ion charge/field effect.20 Amperometric sensors involve applying an electrical potential (or a potential/time wave) to a working electrode while measuring the resulting current or current–voltage characteristics. Subsets of these methods include: chronoamperometry (CA), cyclic voltammetry (CV), linear sweep voltammetry, differential pulse voltammetry (DPV) and square wave voltammetry (SWV). Such sensors comprise the bulk of the literature reports. Potentiometric sensors measure the equilibrium potential difference between two electrodes in solution, usually with no current flowing, and typically relate electrochemical cell potential to the potential drop across a membrane which interacts selectively with the target analyte. Few microRNA biosensors use this technique.21,22 Impedance-based techniques measure the complex impedance resistance of the electrode–electrolyte solution interface. Double-layer capacitance, charge transfer resistance and diffusional impedance are extracted by fitting the data to model circuits. Changes to these fitting parameters can be correlated with molecular recognition events. Many papers have used such techniques for signal transduction; and this can be used for label free detection.23–31 Field effect transistors (ion selective field effect transistors, ISFETs or CHEMFETs) measure the dependence of the source–drain current. Transduction arises from the change in charge density on the gate as its chemistry interacts with the target.
Herein, we present an overview of strategies in electrochemical biosensor design applied to microRNA detection. After a brief introduction on the biogenesis of microRNAs and their endogenous forms, the bulk of this review focuses on the molecular approaches capable of quantitatively transducing sequence-specific microRNA binding events into electrochemical signals. The methods discussed are by no means mutually exclusive, and a key to achieving high sensitivity, large dynamic range and high specificity appears to be combining techniques intelligently.
Biological background of microRNA
In animal cells, miRNAs are synthesised in the nucleus of cells via transcription.1,32 As shown in Fig. 1, this forms a pri-miRNA hairpin structure, which is cleaved by the enzyme Drosha to form pre-miRNA. This pre-miRNA exists as a hairpin, with a 3′ overhang. This 3′ overhang targets the pre-miRNA for export from the nucleus by Exp5 protein. In the cytoplasm, the pre-miRNA loop is cleaved by enzyme drosha to leave a double-stranded duplex. The active strand becomes complexed to Ago proteins and the inactive strand dissociates and is degraded. The mature microRNA becomes available to bind to cytoplasmic messenger RNA (mRNA) and inhibit their translation. Altered expression of miRNAs has been frequently reported in cancer and has shown great promise as tissue-based markers for cancer classification. Approximately 3% of human genes encode for miRNAs and about 30% of human protein-coding genes may be regulated by these molecules. It is now well established that miRNAs play an important role in various cellular processes such as cell differentiation, cell growth, and cell death. Tumour-derived miRNAs in serum or plasma are emerging as novel blood-based fingerprints for the detection of human cancers, especially at an early stage via non-invasive or minimally invasive diagnostic tools. These miRNAs can potentially be detected and quantified not only in frozen tissues but also in serum, plasma, urine, saliva and other body fluids. Interestingly, miRNAs present in human serum proved extremely stable even in harsh conditions of pH (from 1 to 13) and temperature (from freezing to boiling conditions) and significantly more resistant to RNase activity than tissue or cellular miRNAs. To summarise, the correlation between miRNA expression profiles and specific types of cancers or pathogenic conditions, their remarkable stability and their relatively easy accessibility in patient samples in a non-invasive manner could make them ideal cancer biomarkers with enormous potential.
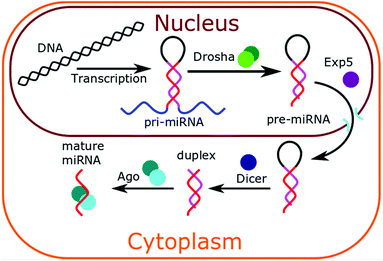 |
| Fig. 1 Schematic representation of microRNA biogenesis in animal cells. | |
Key challenges to microRNA detection
MicroRNA sensing faces many technical challenges before considering full implementation in a clinical or laboratory setting. MiRNAs have only been recently discovered and consequently many unknowns remain, most notably about their localisation and endogenous concentration. The current lack of standardised protocols for extracting, processing and quantifying miRNAs has resulted in major inconsistencies in the recent literature.
First and foremost challenge is the low and unknown level of microRNA to detect.33 How abundant and under which form(s) circulating miRNAs exist in blood are two critical questions for which we are yet to find answers. Currently most changes to specific microRNA levels are reported as up- or down-regulation between healthy and disease states. There is little to no mention of absolute concentrations in biological samples. This is partly due to the lack of suitable analytical tools and of standardised protocols to detect miRNAs, making it extremely difficult to draw quantitative comparisons between studies carried out under slightly different conditions. This may also be due to the intrinsic properties of these miRNAs that can be either free in solution, trapped into exosomes or complexed with one or more proteins. For instance, some studies have reported high concentration of miRNAs in exosomes, while others have shown an average of less than one miRNA molecule per exosome. As a result, to date, it is impossible to confidently answer the question of how sensitive a technology needs to be to detect endogenous miRNAs. The limit of detection and dynamic range of the miRNA sensor will therefore be highly dependent on the nature of the biofluid being tested (e.g. whole blood, serum or plasma) and on the way this fluid is processed prior to analysis.
Normalisation is also a key issue when considering miRNA as quantitative biomarkers. Like for many other biological molecules, the absolute level of expression of certain miRNAs is likely to be influenced by variables that include circadian rhythm, physical activity and diet, thus highlighting the need for strict control of the pre-analytical phase.
Another commonly reported challenge is the miRNAs’ short length and high sequence homology between family members. Sequence specificity is therefore important, and most papers will report whether their biosensor can distinguish between miRNAs that differ by a single base mismatch. Studies have shown not all mismatches are equal, and the position of mismatches has a stronger destabilising effect in the middle of sequences in comparison to the ends.34,35 RNA
:
DNA and DNA
:
DNA mismatches are not necessarily equivalent, and therefore mismatch detection assays should be performed with variations on the target miRNA, and not with more stable DNA.36 Synthetic oligonucleotide analogues such as Peptide Nucleic Acid (PNA), locked nucleic acid (LNA), or phosphorothioate oligonucleotide (PTO) represent a valuable alternative to standard DNA or RNA.37 This is because of their ability to (i) hybridise more tightly to complementary sequences and (ii) be more responsive to point mutations.
The ideal microRNA biosensor
The ideal microRNA sensor is dependent on the perceived use. A clear distinction should be made between point of care and laboratory use. Developing a (bio)sensor for point-of-care testing demands its use to be by somebody who is minimally trained, impatient and unable to pre-process samples.13,38 A laboratory biosensor will be used by a trained professional able to perform sample preparation steps and able to wait for a result. It appears obvious that the laboratory setting would be the “low hanging fruit” of sensing. However, there are already many powerful laboratory methods for detecting microRNAs. Quantitative reverse transcriptase PCR (RT-qPCR) is the gold standard here, however microarrays, northern blotting and next generation sequencing are also powerful methods. PCR-based technologies appear to be ubiquitous in biological labs, which in combination with their versatility for detecting sequences of different lengths, begs the question “why change?” Any new sensing technology for laboratory use must therefore pose significant (economic and/or practical) advantages for the end user. For instance, both amplification steps in RT-qPCR come with associated risks of contamination and error and sensing probes are costly and often suffer from high and variable background noise. The short sequence length and high sequence homology of miRNA compounds some of these issues, requiring careful and intelligent PCR probe design to circumvent.39,40
For point of care use, ideal miRNA sensors will provide a quantitative readout in near real-time with high accuracy, will require no sample preparation, and most importantly, will be fit for a diagnostic purpose. The validation of prototypes and devices with clinical samples is therefore a critical step towards having a microRNA biosensor which is translatable into a technology usable in the field. A low number of steps, long shelf life and ease of storage are also very important here.
Molecular recognition
An electrochemical sensor transduces a molecular recognition event into a measurable electrical signal. The readout can only happen with these two key components combined.18 Molecular recognition of microRNA is most commonly achieved through complementary Watson–Crick base-pairing using either DNA oligonucleotide or oligonucleotide analogue probes. Most biosensors mentioned in this review are amperometric. They therefore rely on the oxidation or reduction of a molecule to generate a readout signal. As will be discussed in the sections below, this molecule is often part of, or attached to the microRNA target. However, this is not the only option. Often the microRNA acts indirectly within the biosensor, creating the signal from another separate molecule.
Labelling with a redox probe
A simple and straightforward approach to introduce the source of the electrochemical signal is via a so-called labelled signal probe. This idea lends itself to a sandwich sensor type design (Fig. 2), where immobilised capture probes are used alongside reporter probes containing the redox active molecule. This technique has not been used in recent years to detect microRNAs, possibly due to the low concentrations of analyte present and the short length of the miRNA preventing simultaneous hybridisation of both capture and signal probes to the same miRNA strand. However, when combined with amplification strategies, these techniques have proven to be fruitful.14,41,42
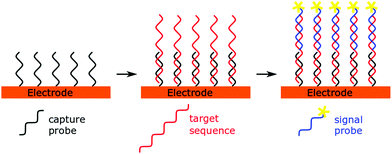 |
| Fig. 2 Schematic representation of a sandwich sensor. | |
Direct labelling of microRNA
Direct labelling of microRNA is another option that has been exploited. Bartosik et al. modified all RNAs in a sample by introduction of Osmium IV 2′2-bipyridine ligands at their 3′ end, followed by selectively capturing the target sequence onto an electrode using a complementary capture probe.43 Gao et al. also directly modified RNA bases with ruthenium complexes, which offers the advantage of creating many electroactive species per microRNA molecule.44 This again used an immobilised DNA capture probe to hold the microRNA at the electrode surface. Both these techniques used direct labelling of the entire sample, followed by selective immobilisation of the only microRNA sequence of interest at the electrode surface. The major drawback of direct labelling is the additional sample preparation needed to create a signal.
Guanine oxidation
An alternative to labelling the microRNA target with redox probes is to use the oxidation of its guanine residues as a signal.45–47 DNA capture probes can be immobilised onto an electrode, using electrochemically inactive inosine residues to replace guanine, but still enable complementary base pairing. This technique has been rarely used, can only be applied to miRNA sequences naturally containing guanine residues, but has advantages in that it minimises sample pre-processing steps. Once hybridised to the capture probes, guanine residues from the target miRNA can undergo an up-to four electron oxidation which can lead to multiple products.48,49 Guanine oxidation is considered chemically irreversible, with no corresponding reduction peaks appearing on a voltammogram. For this reason, any biosensor using guanine oxidation as a detection method, will rely on a single measurement, and cannot average out multiple voltammetric scans. Guanine oxidation also gives an upper limit of the potential window that most microRNA biosensors must use.
Electroactive intercalators
Intercalators are molecules that bind to double-stranded oligonucleotides by inserting themselves in between base-pairs. They form stable, non-covalent pi-stacking interactions, and hence typically have large aromatic ring structures. Although they have most traditionally been used to stain and optically detect DNA, there exist several electrochemically active DNA intercalators, including oracet blue, methylene blue and the structurally similar toluidine blue, which have been used as redox reporters in microRNA sensing.50–54 Typically, hybridisation of the target microRNA to a complementary capture probe immobilised at the electrode surface creates a DNA–RNA heteroduplex that is recognised by the electroactive intercalator which can then be oxidised or reduced.
An inverse take on this idea was adopted by Hou et al.55 Complementary binding was used to keep methylene blue away from the electrode, instead of concentrating it at the electrode. The complementary DNA was not immobilised, but rather kept in solution and the bound methylene blue resulted in a much lower diffusion current to the electrode. This effect was increased by a hybridisation chain reaction which resulted in long double-stranded and G-quadruplex sequences forming because of the microRNA being present.
Backbone binding molecules
Ruthenium(II) hexamine is a redox active molecule that has been shown to bind DNA and RNA through complexing to their phosphate backbone.41,56 Improved electrochemical signal has been shown for ruthenium(II) hexamine on a DNA modified electrode compared to unmodified, which has been attributed to a facilitated diffusion mechanism, distinct from intercalator behaviour. Little work has been done to exploit this behaviour for sensing. Islam et al. employed ruthenium(III) hexamine backbone binding as part of a larger sensing strategy with multiple chemical amplification steps.57 Ruthenium(III) was reduced to ruthenium(II) as it complexed microRNA at gold-loaded superparamagnetic iron oxide nanocubes. This system was coupled with ferricyanide which is reduced by the ruthenium(II). This leads to amplification of the signal as it cycles between the ruthenium(II) and ruthenium(III) oxidation states and continuous reduction of the reagent ferricyanide. A similar redox reporting strategy was employed by Su et al.24 Two partial complementary sequences ensure coupling of Au-nanoparticle (NPs) decorated MoS2 to a glassy carbon electrode. This recognition event is transduced by impedance spectroscopy of ferri/ferrocyanide and differential pulse voltammetry of intercalated ruthenium(III) hexamine.
Enzyme modification
Enzyme based biosensors are perhaps the oldest and most well studied type of biosensors. There are numerous books and reviews discussing them in depth.58–61 From the perspective of microRNA detection, enzymes are employed to catalytically generate and amplify the electrochemical signal. The easiest way to do this is via a sandwich sensor involving an immobilised capture probe and a secondary probe conjugated to an enzyme, either directly or indirectly, via a biotin–streptavidin interaction which occurs after the molecular recognition event.62–73 Enzymes typically used are the same found in ELISAs and include horseradish peroxidase (HRP), alkaline phosphatase, and glucose oxidase.74–76 Such enzymes are well studied, cheap and exhibit predictable behaviour. Depending on the nature of the enzyme, detection can originate from the product of the catalysis or the regeneration of the enzyme. Each microRNA can now be responsible for the generation of many signal molecules which depends on the catalytic turnover of the enzyme and the concentration of bound analyte.
A straightforward improvement to this can be via increasing the number of enzymes bound per microRNA molecule. As will be discussed later in this review, this can be achieved via various DNA constructs and nanoparticles. Commercially available polymers of horseradish peroxidase (polyHRP) can be conjugated instead of using a single enzyme.62 Alternative conjugation techniques can also be used. Ma et al. used a polymerase-based pre-amplification step which replaced the microRNA signal with a DNA signal, to detect microRNAs in breast cancer cells.77 The cytosine residues used had further been functionalised with biotin which, in a subsequent step, became conjugated with a streptavidin bound alkaline phosphatase and generated the signal. A similar technique was employed by Zhou et al. to detect microRNAs in rice seedlings, using poly-U polymerase and biotinylated UTP.78 Both these techniques gave similar results in terms of limit of detection (9 and 7 fM, respectively) and were declared fit-for-purpose in each application.
An alternative technique used has been to assemble enzymes into a larger structure for detection. Enzyme spheres, as reported by Wu et al., are nanoporous spheres of HRP coated in porous Pd.79 These spheres showed high activity toward H2O2 electrochemistry thus obviating the need for additional redox reporters. The spheres were then conjugated to amine-terminated DNA probes complementary to the miRNA target. A complex detection strategy used Pb2+-induced cleavage of the ternary Y structure and rolling circle amplification techniques to further increase the sensitivity of the sensor, reaching a limit of detection of 0.2 fM.
DNAzymes
DNAzymes (or Deoxyribozymes) are single-stranded DNA aptamers that exhibit a catalytic activity.80–84 Their sequences are discovered from SELEX type processes, through successive cycles of selection and enrichment cycles.85,86 They can exhibit a broad range of catalytic activities that could theoretically be exploited for microRNA sensing. The most commonly exploited DNAzyme for microRNA detection is a heme-based G-quadruplex peroxidase87,88 which reduces hydrogen peroxide into water in a similar way to HRP.
A common approach is to use DNAzymes directly for their catalytic ability.27,89,90 The DNAzymes can built into the signal probes without need for conjugation chemistries. When compared to traditional peptide-based enzymes, DNAzymes offer the advantage of greater thermal and functional stability. Other biosensors have taken advantage of the ability to change DNA conformation to create a switch in their design.91–94 The general principle is quite simple. The DNA is blocked by a complementary sequence from folding into its G-quadruplex functional structure. The presence of the microRNA causes a strand displacement which enables the DNAzyme to fold into its active conformation. The heme groups necessary for function are also added to the solution and so the DNAzyme can function only when the microRNA is present. This is a typical example of a strand displacement reaction being used for microRNA detection, which will be discussed in more general terms later in this review.
Blocking electrode and polymer deposition
An alternative to having the miRNA binding event creating a detectable signal, is for this interaction to change the nature of the detector (Fig. 3).23–29,88,95–100 Two similar but distinct methods can be identified here: (i) directly blocking the electrode with microRNA, and (ii) using microRNA binding to guide deposition of an insulating polymer. Directly blocking the electrode relies on miRNA binding leading to a large change in electron transfer kinetics. Hence, the lowest limit of detection in this technique have been achieved by Labib et al. by enabling complementary binding to bring the protein streptavidin to the surface, through interactions with biotin.96 However, Yammouri et al. recently demonstrated that complementary binding alone was able to induce a detectable impedance signal, but only with comparatively high concentrations in the nano- to micro-molar range.26 Magnetic beads have also been used to amplify the signal, or pre-concentrate selected microRNAs at the electrode surface.23,99,100 Nucleases are also commonly used in these detection methods to amplify and invert the signal.23,25,97 Such enzymatic methods will be discussed in more detail later in this review.
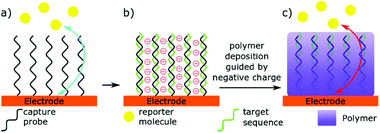 |
| Fig. 3 Principle of an impedance-based polymer film biosensor. (a) In absence of microRNA target, the electrode can easily detect reporter molecules. (b) Complementary binding brings a negative charge to the surface. (c) Polymer deposition inhibits charge transfer. | |
Selectively depositing polymers on electrodes for microRNA detection has been explored with coulometric and impedance-based biosensors.26,95 In a typical example, miRNA hybridisation to a complementary probe immobilised at the surface of an electrode creates a negatively charged surface that is subsequently used to guide the deposition of a positively charged, electrically insulating polymer. The net result of this process is a decrease in the efficacy of the electrode, measured by an increase in electrochemical impedance. A key to sensitivity in this technique is to use a charge-neutral capture probe. For this reason, Phosphorodiamidate Morpholino Oligomer (PMO) and Peptide Nucleic Acid (PNA) probes are typically preferred to standard, negatively charged, oligonucleotides.
Peng and Gao initially used a variation of this technique, by pre-tagging a microRNA with Ruthenium oxide nanoparticles.29 The nanoparticles were then used to catalyse the polymerisation and deposition of a benzidine onto the electrodes. This avoided the need to catalyse the polymerisation separately and avoided the need for charge-neutral capture probes. A downside of this technique was the multi-step preparation of microRNA samples, using extraction kits, followed by a three-step process to attach the nanoparticles. This did, however, act as a good proof-of-concept for these types of microRNA sensors. Ding et al. used a similar concept, although using DNA enzymes to catalyse polyaniline deposition.88 This was used in combination with HCR amplification, and will be discussed later in this review. Optimisation of the preparation steps, ideally into a one-pot system would be ideal for taking these ideas forward.
Nanoparticles
The use of nanoparticles and nanomaterials in biosensing in general has been extensively reviewed elsewhere, and theoretical models of such systems with regards to electrochemistry have already been thoroughly mathematically described.101–104 Nanoparticles are multifunctional and for electrochemical sensing applications, we have separated them into five categories based on their mode of action: (i) increasing the electrode surface area, (ii) acting directly as a redox couple (iii) acting indirectly through catalysis (iv) acting indirectly by attaching to multiple redox couples or enzymes, and (v) acting to concentrate samples.
The effect of increasing the surface area of an electrode is reasonably straightforward. The exact nature of the surface is dependent on the size and distribution of nanoparticles across it. The change in surface geometry will change the diffusion and bulk transport properties to it, therefore altering the signal. This change may be unimportant when dealing with immobilised species. It is however worth noting that surface area scale differently according to the physico-chemical process: diffusion scales linearly as (2Dt)1/2 where D is the diffusion coefficient (typically 10−10 m2 s−1 for small aqueous species) which implies that asperities (roughness) smaller than 1–10 μm will not affect diffusion limited currents in typical electrochemistry set-ups. In the short time domain however, the presence of the nanoparticles can lead to thin film behaviour and further complicate analysis. The length scale for electrostatic phenomena is the Debye length, which for physiological ionic strength is many orders of magnitude below the diffusional length scale, typically 0.5 nm. Techniques exploiting this are commonly used.
Through direct action as redox active species, nanoparticles or nanoclusters can generate a higher signal than their unimolecular counterparts by having more redox active atoms and delivering a higher effective concentration to the electrode surface. Silver nanoparticle (or nanostructure) reduction or oxidation has been used as a signal transducer.105 Nanoparticles can be synthesised onto DNA probes, however Yang et al. used a DNA-templated nanoparticle synthesis as part of their detection mechanism.106 Other nanoparticles can be used for a similar effect. Wang et al. precipitated copper nanoclusters onto their sensor then re-solubilised the copper to be redetected by differential pulse stripping voltammetry.107
The innate catalytic activity of certain nanoparticles is not to be ignored. Silver and platinum nanoparticles have been used for their ability to catalyse hydrogen peroxide reduction,107,108 similar to the Pd nanospheres with HRP scheme employed by Wu et al., vide supra.79 By allowing complementary binding to bring these to an electrode, this reduction can be quantified. Other catalytic activities have also been studied.109,110 Yu et al. used cobalt iron oxide (CoFe2O4) nanoparticles functionalised onto a DNA probe, in a sandwich type sensor, to catalyse the reduction of terbium. The re-oxidation could then be used to indicate microRNA concentration. Wang et al. used copper metal organic frameworks modified onto gold nanoparticles to catalyse glucose oxidation.110 The catalysis idea does not always have to give direct product detection. As discussed earlier, Peng and Gao flipped the idea by using ruthenium oxide nanoparticles to catalyse the polymerisation of 3,3′ dimethoxy benzidine as a blocking film above their electrode.29 This required pre-tagging their microRNA with ruthenium oxide labelled with a complementary short sequence and using a capture probe to selectively keep it at the electrode surface. The target miRNA effectively templates the deposition of an insulating film when the monomer solution and oxidant are added. The progress of the reaction and thus the degree of templating was followed using impedance spectroscopy in a solution of Ru(II)(NH3)3+. Interestingly, charge transfer resistance for this outer sphere probe was affected. Incubation times were lengthy at 60 min, but good LODs of ∼3 fM for their target miRNA were achieved.
Nanoparticles can also be used to bring complementary binding and transducer elements together. A single nanoparticle can host complementary binding probe, as well as redox active reporters. Ferrocene capped gold nanoparticles conjugated with streptavidin have been used and will assemble onto a biotinylated probe.111,112 Similarly, other redox active molecules, including (but not limited to) dopamine, thionine, and ferrocene, have been immobilised onto nanoparticles for the purposes of creating a large signal from a single microRNA binding event.111–117
Magnetic micro- or nanoparticles can be used to pre-concentrate microRNA from the samples.23,43,57,99,100,111,118,119 Strictly speaking, most magnetic beads used in device have micrometer scale, and so do not fit the IUPAC definition of nanoparticles (dimensions 1–100 nm).120 Some devices however, use nano-scale magnetic beads for the same purposes.109,121 Magnetic beads are particles that can be influenced by a magnetic field.122–124 These tend to be ferromagnetic iron oxide, often coated in polystyrene. The magnetic beads are added to a biological sample, given time for the microRNA to hybridise, and then removed with a magnet. Then, typically one of two options are taken: heating the sample to remove the microRNA from the capture sequence, or using a magnetic electrode, to concentrate the magnetic beads at the surface. The former option is simply just a purification technique, while the latter is somewhat more creative and has the benefit of being isothermal. Alternatively, Ma et al. made use of a strand displacing polymerase to remove the microRNA from the magnetic beads.77 This was just part of a more complicated mechanism, which resulted in modification of the magnetic beads with alkaline phosphatase, which then generated an electrochemical signal through its action on 2-phospho-L-ascorbic acid. In general, magnetic beads offer an easy, effective option for sample preparation and miRNA separation. If developing a biosensor for a laboratory setting, these methods are clearly effective. Further automation of this technique would be required for developing it into point of care devices, and successfully doing so could create very powerful biosensors.
Other nano-structures
Further to nanoparticles, other nano-structures have been exploited in the formation of biosensors. Nano-structure is a very broad term, and rightfully applies to most of the content of this review. This section therefore focuses on molecular methods that would otherwise go without description.
DNA tetrahedra
DNA tetrahedra are self-assembling DNA nanostructures. They have been widely used in biosensing for their ability to give surface control.62,64,66,90,125–128 They are assembled from four sequences of 55 nucleotides in one step.129 Each of the four sequences creates the edges to one of the four faces, through complementary binding to the three other structures. The range of potential biological applications for these structures has been well reviewed.130 The most common design for DNA tetrahedral based biosensors was suggested by Mitchell et al.131,132 They are typically immobilised face down onto a gold electrode via three thiol groups, with the upward facing vertex carrying the probe of interest.132 This controls the spread of this probe across the surface, causing an ordered and well defined spacing of 4 nm. Each probe is well anchored with three Au–thiol bonds, conveying approximately 5000 times as much stability as a single bond.131 The tetrahedra can also help prevent electrode fouling through blocking non-specific protein absorption. Through this, DNA tetrahedra based biosensors are less likely to fail than those with less surface control. Further development using this, or other surface controlling methods, are likely to result in more clinically successful biosensors.
Mesoporous silica
Several recent studies have been using mesoporous silica as a novel method to detect miRNA (Fig. 4).21,22,133,134 The concept involves entrapping an electroactive reporter molecule within these nanoparticles and blocking their exit with single stranded capture probes. The capture probes are typically made of DNA and bind to the mesoporous silica due to a positive zeta potential on the nanoparticles. Upon complementary binding with target miRNA, the capture probes dissociate from the silica, causing the entrapped signal molecule to escape the silica. This allows a potentially massive signal amplification, and the choice of an ideal molecule to detect. Gai et al. entrapped ferricyanide particles, initially for a ratiometric sensor, comparing the DPV signal to Ruthenium hexamine in solution.133 This work was later expanded upon, to create a similar potentiometric sensor, comparing the ease of ferricyanide reduction at a cathode, to an glucose oxidase modified anode.21 Similar work was carried out by Deng et al., by entrapping glucose within the mesoporous silica, and detecting it using a portable personal glucometer.22 This work creates a great proof of concept for a portable microRNA biosensor. However, glucose as a signalling molecule will likely be unviable in patient samples, due to the high levels already present in blood, and false positives coming from diabetic patients.
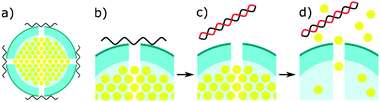 |
| Fig. 4 Mechanism of miRNA detection using mesoporous silica. (a) Mesoporous silica is loaded with electroactive signalling molecules. (b) The pores of the silica are capped with miRNA capture probes. (c) Complementary binding leads to dissociation from the pores. (d) The electroactive molecules escape the silica to be detected on an electrode. | |
Other useful techniques
Often, sensing strategies are used with a myriad of other useful techniques, designed to increase sensitivity, amplify the signal, or aid in separation from the biological matrix. These techniques create additional complexity in the biosensor design, and often work in close synergy with the detection scheme. It becomes difficult to assess the relative merits of a technique, as they are rarely used in isolation. The following section discusses some of the more common techniques used.
Signal amplification through polymerases
Polymerases are well known enzymes which catalyse the formation of DNA strands and were made famous by polymerase chain reaction which revolutionised molecular biology.135 These useful enzymes are often implemented into microRNA biosensors as a signal amplifier. Many biosensors use microRNA as a primer for DNA polymerase, which alongside a nuclease or strand displacement causes a large amount of a signalling sequence to be formed.54,92,94,106,118,127 Rolling circle amplification (RCA) is a particular example of this, which uses a circular DNA (or RNA) as a template molecule.136 The polymerase processes around the template molecule multiple times, creating tandem repeats of complementary sequences (Fig. 5). Unlike traditional PCR-based methods, RCA is isothermal and one-step. The length of the amplification is dependent on the number of free nucleotides present for the polymerase to use. In microRNA sensing, RCA is most commonly used in combination with other detection methods as a signal amplifier.79,109,127,137 Typically, the miRNA allows an initial primer to bind for the amplification to occur. The tandem repeats resulting from the amplification allow multiple signalling molecules to bind. The value of RCA arises from this ability to isothermally amplify a signal.
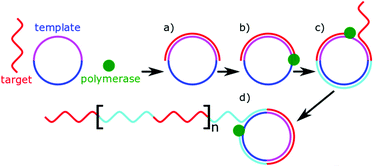 |
| Fig. 5 General principle of rolling circle amplification (RCA). (a) Target complementary binds to circular template. (b) The polymerase uses target sequence as a primer. (c) The polymerase displaces the target as it processes around the template. (d) The result is tandem repeats of the target sequence with a designed secondary sequence. | |
Template-independent polymerases have also been used to amplify microRNA signals.78,99 As the name suggests, template-independent polymerases, can catalyse the addition of nucleotides to DNA or RNA without a template sequence to copy. Typically adding string of a single nucleotide. As described earlier, Zhou et al. made use of poly(U)polymerase in combination with biotinylated uracil residues for detection.78 Koo et al. alternatively used poly(A)polymerase to allow the pre-concentrated target microRNA to adsorb to an electrode, hindering electron transfer.99 In both cases, the polymerases are acting as signal amplifiers enabling transduction. The isothermal amplification of polymerase techniques is powerful; however, it comes at a need for additional reagents within the sample, chiefly nucleotide triphosphates.
Use of nucleases
Specific nucleases have been used in miRNA sensors, as part of signal transduction or amplification. Duplex Specific Nucleases (DSN) are enzymes that can specifically recognise duplex DNA or DNA
:
RNA hybrids.138 Only the DNA component of the duplex is then hydrolysed, allowing RNA to dissociate from the enzyme. This unique specificity allows these enzymes to be used in microRNA biosensors, acting as an isothermal signal amplifier. DSN has lent itself readily to impedance-based biosensors.23,25 An electrode is functionalised with a DNA capture probe. When the complementary microRNA binds, the DSN will selectively cleave the DNA, allowing a redox reporter to get closer to the electrode, thus reducing the resistance to electron transfer (Fig. 6). An alternative mechanism has been used to remove reporter molecules which are tagged onto the immobilised DNA capture sequence.139 The action of this enzyme leaves a 3′ hydroxyl at the electrode surface, which can also be exploited for further chemical or enzymatic reactions.54 A novel use of DSN was proposed by Castaneda et al.140 using platinum nanoparticles functionalised with DNA capture probes. MiRNA binding allowed DSN to remove the DNA from the nanoparticles, which could then impact an ultramicroelectrode, and catalyse hydrazine reduction.
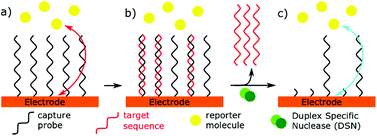 |
| Fig. 6 The use of duplex specific nuclease in biosensing. (a) Electron transfer is hindered by a surface concentration of capture probes. (b) Complementary binding of the target sequence allows duplex specific nuclease to selectively remove capture probes. (c) Electron transfer is easier as a result. | |
Strand displacement reactions
Strand displacement reactions (SDR) and Toehold Mediated Strand Displacement Reactions (TMSDR) are a growing area in nanotechnology.48,141,142 They have already been used to create logic gates, catalysis, and nanomachines. The principle is summarised in Fig. 7. Using DNA sequences containing overhangs, a new initiator strand which has more complementary bases to one of the bound strands can displace another. The exact combination of effects can be carefully tweaked, and advanced mechanisms can be set up from this principle. One strand displacement can lead to another strand displacement, allowing a recycling of the initiator strand. A typical use of this mechanism in biosensing would be to have the microRNA as an initiator and to detect one of the displaced strands.
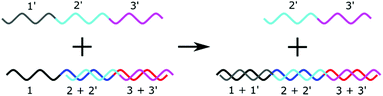 |
| Fig. 7 Principle of a strand displacement reaction. In the presence of a “toehold” sequence, a longer complementary sequence will displace a shorter sequence. | |
A novel and complicated take on this mechanism was used by Zhang et al.14 Here a strand displacement reaction, caused by microRNA-binding released a “DNA Walker” sequence. This DNA walker then bound to a complementary DNA hairpin on the electrode surface, causing a stem loop to open. It was then displaced by another hairpin structure which was functionalised with ferrocene. This recycled the walker for further binding. The electrochemical oxidation of the ferrocene was then detected by DPV. The whole system was considered regeneratable through heating. Stem-loops are hairpin shaped DNA secondary structures commonly used in DNA sensing, and their opening is an intramolecular case of a strand displacement reaction. They have a large single-stranded loop of DNA, generally with a short self-complementary stem. Oligonucleotides complementary to the loop and one of the stem sequences can therefore cause the loop to open upon binding. The stem-loop opening has been traditionally used in molecular beacon technology, where each end of the stem loop is functionalised, such that a quenched fluorescence is enabled upon binding to oligonucleotides. This creates a highly sequence specific sensing. The applications in electrochemistry can be quite different. In all examples found, the stem loops are immobilised onto an electrode. The similar DNA architecture can be exploited in different ways that we categorised into functionalised stem loops and non-functionalised stem loops.
Most commonly, stem loops are functionalised with biotin. When closed, the biotin is held close to the electrode and inaccessible to binding with streptavidin. Binding with complementary sequences, causes the hairpin to open and streptavidin to bind to the now accessible biotin. Signal transduction is then achieved through whatever streptavidin is bound to. Stem loops can also be functionalised with electroactive species. However, this is not enough to give signal transduction. Yang et al. used this idea with a nuclease: complementary binding caused stem loop opening, causing the electroactive moiety to be removed from the electrode. They multiplexed this by using ferrocene and methylene blue on separate stem loops with different sequences.139 Another strategy was used by Liu et al. where the ferrocene bound stem loop was held away from the electrode by using rigid DNA tetrahedral nanostructures. Complementary binding enabled stem loop opening, allowing enough flexibility for the ferrocene to reach the electrode, thus generating the detectable signal.125
Non-functionalised probes typically allow signal transduction through a secondary sequence binding.73 The opened stem-loop leaves either part of the microRNA or part of itself single stranded, allowing complementary binding of this second sequence. These secondary sequences can be functionalised to generate a signal in many ways as previously described. The stem loop therefore offers greater freedom in sequence design than a single-stranded probe. This freedom of design was exploited by Meng et al.93 Upon complementary binding and stem loop opening, there was a leftover single stranded section of the probe. This was designed to form a G-quadruplex structure, in the presence of potassium, and the addition of a hemin group allowed this quadruplex to become a DNAzyme, which in turn enabled oxidation of hydrogen peroxide.
Hybridisation chain reaction (HCR) is a popular case of strand displacement reaction used to amplify a signal.88,110,137,143–146 A single-stranded initiator sequence is placed amongst two hairpin sequences. The initiator (microRNA signal) causes the opening of the first hairpin, which opens the second, which in turn opens another copy of the first. This causes a cascade reaction, and enzyme-free amplification of the DNA. This can be converted into an electrochemical signal. The simplest way to do so is to modify the hairpins. This has been achieved with biotin, allowing a streptavidin bound enzyme to signal.64 Alternatively, the use of nanoparticles or other attached molecules is also possible.55,106,107 Hairpins can also be designed to leave single-stranded sections after HCR. New auxiliary probes, conjugated to signalling molecules can then be added to bind to these single-stranded sections.114,115 A novel take on HCR, has recently been described by Zhang et al. that includes regeneration of the miRNA.147 Techniques like this enable high levels of isothermal amplification, creating very sensitive systems.
SDR can create isothermal amplification and novel sensing mechanisms. There is a lot of potential for advanced logic gates and data processing within these systems and it is highly likely that new variations on these methods will provide fascinating next generation biosensors.
Junction probes
Novel Y-shaped junction probes have been used in a lot of miRNA sensing strategies.79,119,148 These structures were initially described in 2008 by Nakayama et al. as a method of isothermally amplifying sample signals149 through use of an oligonucleotide 3-way junction involving two detection probes instead of one. In this strategy, the detection probes cannot complementary bind to each other at room temperature. The signal probe can partially complementary bind to each of the probes, enabling the Y-shaped structure to form. This concept boasts high sequence specificity and mismatch detection because the formed structure is the result of three partially stable interactions. A proof of concept biosensor using this strategy was developed in 2009 to detect DNA.150
Conclusions
Nucleic acid sensing is on the frontier of diagnostic medicine, with many diseases being linked to altered expression of specific miRNA biomarkers. This creates a clinical need to develop effective microRNA biosensors for point of care testing. With modern day technology, many novel electrochemical biosensors are being developed, often exploiting unique and exciting molecular mechanisms (Table 1). Such mechanisms are not mutually exclusive and can work synergistically to improve the detection of microRNA. Most articles report on technologies incorporating a host of different ideas, and as such it can be challenging to identify the merits of any one component.
Table 1 Summary of detection types
Detection method |
LOD (M) |
Dynamic range |
Biological sample |
Techniques used |
Ref. |
Backbone binding
|
DPV |
1 × 10−16 |
100 aM to 100 pM |
Serum |
Stem loop opening |
151
|
DNA concatemers |
Coulometric |
1 × 10−16 |
100 aM to 1 nM |
RNA extracts |
Magnetic beads |
57
|
Nanoparticles |
DPV |
7.8 × 10−16 |
10 fM to 1 nM |
Spiked serum |
Nanostructure formation |
24
|
Two detection methods |
|
Blocking electrode surface
|
SWV |
2 × 10−18 |
10 aM to 1 fM |
Serum |
Four-way junction |
96
|
SWV |
5 × 10−18 |
10 aM to 1 μM |
Serum |
Four-way junction |
152
|
Enzyme |
EIS |
6 × 10−17 |
0.5 fM to 40 fM |
Serum |
Magnetic beads |
23
|
Nuclease |
DPV |
1.7 × 10−16 |
0.5 to 100 fM |
RNA extract |
Nuclease |
97
|
EIS |
4.5 × 10−16 |
10 fM to 1 nM |
Spiked serum |
Nanostructure formation |
24
|
Two methods of detection |
EIS |
1 × 10−15 |
2 fM to 2 pM |
RNA extracts and serum |
Nuclease |
25
|
SWV |
8 × 10−15 |
1 fM to 1 nM |
— |
Antibody based removal |
98
|
DPV |
1 × 10−14 |
5 fM to 5 pM |
RNA extracts |
Magnetic beads |
99
|
Poly(A)polymerase |
Coulometric |
2 × 10−14 |
10 fM to 10 nM |
Urine |
— |
95
|
EIS |
7 × 10−13 |
1 pM to 100 pM |
RNA extracts |
— |
31
|
DPV |
1 × 10−12 |
1 pM to 10 nM |
RNA extracted from exosomes |
Magnetic beads |
100
|
EIS |
1 × 10−11 |
80 nM to 150 μM |
Serum |
— |
26
|
|
DNA enzyme
|
CA |
5 × 10−19 |
1 aM to 100 pM |
None |
Polymerase and endonuclease cascade reaction |
92
|
DPV |
1.2 × 10−15 |
10 fM to 1 nM |
Cell lysates |
Strand displacement reaction |
91
|
Junction probes |
DPV |
2.0 × 10−15 |
1 nM to 10 fM |
Spiked serum |
Nanostructure formation |
90
|
Nanoparticles |
DNA tetrahedra |
DPV |
5.4 × 10−15 |
20 fM to 50 pM |
RNA extracts |
Polymerase |
94
|
DPV |
6 × 10−15 |
0.01 to 500 pM |
RNA extract |
Nanoparticles |
93
|
Stem loop opening |
Nanostructure formation |
SWV |
5.2 × 10−12 |
0.01 nM to 1 μM |
Spiked serum |
Strand displacement reaction |
89
|
|
Enzyme
|
CA |
2.2 × 10−19 |
1 aM to 10 fM |
Spiked saliva |
Magnetic beads |
119
|
Junction probes |
CA |
5 × 10−18 |
“Approximately 5 orders of magnitude” |
RNA extracts |
Junction probes |
153
|
CA |
1 × 10−17 |
10 aM to 1 pM |
None |
DNA tetrahedra |
64
|
Hybridisation chain reaction |
CA |
1.4 × 10−16 |
1 fM to 100 pM |
Spiked serum |
Nanoparticles |
63
|
DPV |
1.6 × 10−16 |
0.1 fM to 0.1 nM |
Diluted serum |
Nanoparticles |
73
|
Stem loop opening |
Strand displacement reaction |
DPV |
2 × 10−16 |
3 fM to 1 nM |
None |
Nanoparticles |
79
|
Stem loop opening |
Junction probes |
Rolling circle amplification |
CA |
2 × 10−16 |
0.5 fM to 1 pM |
Serum |
Stem loop opening |
68
|
DPV |
3.5 × 10−16 |
1 fM to 10 nM |
Spiked serum |
Stem loop opening |
110
|
Strand displacement reaction |
Nanoparticles |
CV |
1 × 10−15 |
“6 orders of magnitude” |
None |
DNA tetrahedra |
66
|
Stem loop opening |
DPV |
1.7 × 10−15 |
10 fM to 1000 fM |
RNA extract from rice seedlings |
Poly(U) polymerase |
78
|
CA |
3 × 10−15 |
10 fM to 5 pM |
None |
Nanoparticles |
154
|
Labelling microRNA |
DPV |
3.6 × 10−15 |
10 fM to 0.1 nM |
Cell lysates |
Nanoparticles |
67
|
Stem loop opening |
CA |
4 × 10−15 |
8 fM to 10 pM |
RNA extracts |
Stem loop opening |
76
|
CA |
6 × 10−15 |
0.01 pM to 7 pM |
RNA extracts |
Stem loop opening |
71
|
Nanoparticles |
Nanostructure formation |
DPV |
9 × 10−15 |
10 fM to 10 nM |
RNA extracts |
Polymerase recycling |
155
|
Stem loop opening |
Magnetic beads |
CA |
1 × 10−14 |
20 fM to 10 pM |
RNA extracts |
Nuclease |
69
|
CA |
1 × 10−14 |
10 fM to 1 nM |
Serum |
DNA tetrahedra |
62
|
DPV |
2.5 × 10−13 |
0.5 pM to 12.5 nM |
RNA extract |
Strand displacement |
137
|
Rolling circle amplification |
EIS |
4 × 10−13 |
1.7 pM to 900 pM |
Diluted, spiked serum |
Pre-labelled miRNA |
156
|
Liposomes |
CA |
6 × 10−13 |
0.6 pM to 20 nM |
RNA extract, serum |
Strand displacement reaction |
147
|
DPV |
6 × 10−13 |
1 pM to 25 nM |
Diluted RNA extracts |
Strand displacement reaction |
157
|
CA |
1 × 10−12 |
1 pM to 1 nM |
RNA extracts |
— |
65
|
Pulse voltammetry |
2 × 10−12 |
2 pM to 200 nM |
RNA extracts |
— |
158
|
CA |
7 × 10−12 |
7 pM to 2.5 nM |
RNA extracts |
Magnetic beads |
74
|
CA |
1.1 × 10−11 |
100 pM to 100 nM |
10% serum |
Stem loop opening |
159
|
Secondary structure change |
CA |
4 × 10−11 |
0.14 to 100nM |
RNA extracts |
Magnetic beads |
75
|
DPV |
1 × 10−7 |
— |
RNA extracts |
Attaching RNA to electrode |
70
|
DPV |
1.6 × 10−7 |
— |
RNA extracts |
RNA specific protein |
160
|
CV integrating peaks |
— |
— |
Serum |
Stem loop opening |
161
|
Four-way junction |
DPV |
6 × 10−17 |
0.1 fM to 100 pM |
Serum |
Nanoparticles |
72
|
|
Inorganic catalyst labelled
|
CA |
2 × 10−13 |
0.5 pM to 400 pM |
RNA extracts |
Pre-labelled microRNA |
44
|
|
Guanine oxidation
|
DPV |
1 × 10−12 |
1 pM to 1 nM |
None |
Carbon nanotubes |
46
|
1 nM to 10 nM |
(Two dynamic ranges reported) |
DPV |
5 × 10−9 |
— |
None |
— |
45
|
DPV |
7 × 10−7 |
1.4 μM to 5.6 μM |
None |
— |
47
|
|
Intercalators
|
DPV |
1.5 × 10−17 |
50 aM to 50 pM |
Spiked plasma |
Nuclease |
54
|
Polymerase |
DPV |
3.3 × 10−17 |
100 aM to 100 nM |
Serum |
Nanoparticles |
53
|
DPV |
6 × 10−16 |
2 fM to 8 pM |
Plasma |
Nanoparticles |
51
|
DPV |
8.4 × 10−14 |
0.1 to 500 pM |
— |
Carbon nanotubes |
52
|
DPV |
5 × 10−13 |
10 fM to 1 nM and 1 nM to 100 nM |
None |
Carbon nanotubes |
50
|
DPV |
1 × 10−12 |
1 pM to 800 pM |
None |
Hybridisation chain reaction |
55
|
|
Mesoporous silica
|
Potentiometric |
2.7 × 10−18 |
10 aM to 1 pM |
Serum |
Nanoparticles |
21
|
DPV |
3.8 × 10−17 |
0.05 fM to 100 fM |
Tumour cells |
— |
134
|
Potentiometric |
1.9 × 10−11 |
50 pM to 5 nM |
Cell lysates |
— |
22
|
DPV |
3.3 × 10−17 |
0.1 fM to 1500 fM |
Cell lysates |
— |
133
|
|
Nanoparticles as a direct redox couple
|
DPV |
6.7 × 10−14 |
10 nM to 100 pM |
None |
Stem loop opening |
108
|
LSV |
2 × 10−17 |
0.1 to 50 fM |
Serum |
Stem loop opening |
162
|
Labelled miRNA |
LSV |
5 × 10−17 |
1 fM to 10 pM |
RNA extracts and serum samples |
DNA tetrahedra |
127
|
Rolling circle amplification |
LSV |
4 × 10−16 |
1 fM to 1 nM |
Spiked serum |
DNA tetrahedra |
126
|
Stem loop opening |
Polymerase |
DPV |
6.4 × 10−16 |
1 fM to 0.1 nM |
Spiked serum |
Polymerase |
106
|
Hybridisation chain reaction |
DPV stripping voltammetry |
1 × 10−17 |
0.1 fM to 10 pM |
Spiked blood |
Strand displacement reaction |
107
|
Nuclease |
Hybridisation chain reaction |
SWV |
1.2 × 10−14 |
50 fM to 30 pM |
Spiked serum |
Magnetic beads |
118
|
Polymerase |
|
Nanofoam acting as a redox couple
|
CV |
2 × 10−16 |
0.2 fM to 1 nM |
Plasma with and without dilution and spiking |
— |
105
|
|
Nanoparticles labelled with redox couple
|
SWV |
7.6 × 10−19 |
1 aM to 10 pM |
Serum |
Nanoparticles |
113
|
DPV |
1.1 × 10−17 |
0.1 fM to 100 pM |
Spiked serum |
Stem loop opening |
115
|
Nuclease |
Hybridisation chain reaction |
CV |
1.4 × 10−16 |
5 fM to 100 fM |
Serum |
Magnetic beads |
111
|
Stem loop opening |
Nanoparticles |
DPV |
4.4 × 10−16 and 4.6 × 10−16 |
1 fM to 1 nM |
Cell lysates |
Stem loop opening |
115
|
Hybridisation chain reaction |
|
SWV |
8 × 10−15 |
10−15 to 10−10 M |
Diluted serum |
— |
116
|
CV |
1 × 10−14 |
10 fM to 2 pM |
Serum |
Pre-labelled microRNA |
112
|
DPV |
4.5 × 10−14 |
0.1 to 10 pM |
None |
Pre-labelled microRNA |
117
|
|
Nanoparticle (catalytic)
|
SWV |
3 × 10−16 |
1 fM to 2 nM |
RNA extracts |
Magnetic beads |
109
|
Rolling circle amplification |
DPV |
1.92 × 10−15 |
0.5 fM to 5 pM |
Cell lysates |
Stem loop opening |
107
|
CA |
8 × 10−14 |
0.3 pM to 200 pM |
RNA extracts |
— |
163
|
CV |
1.87 × 10−12 |
5.6 pM to 560 nM |
Serum |
— |
164
|
CA frequency analysis |
1 × 10−10 |
0.1 nM to 10 nM |
None |
Nuclease |
140
|
|
Nanoparticle (other)
|
CV |
1.6 × 10−14 |
0.05 to 0.9 pM |
RNA extracts |
— |
165
|
|
Polymer deposition
|
EIS |
5 × 10−16 |
1 fM to 5 pM |
RNA extracts |
DNA enzyme |
27
|
DPV |
5 × 10−16 |
1 fM to 100 pM |
Serum |
Stem loop opening |
88
|
Hybridisation chain reaction |
EIS |
2 × 10−15 |
5 fM to 2 pM |
RNA extracts, and serum |
— |
28
|
EIS |
3 × 10−15 |
6 fM to 2 pM |
RNA extracts |
pre-labelled microRNA |
29
|
EIS and DPV |
1.7 × 10−10 |
— |
RNA extracts |
— |
30
|
|
Redox labelled
|
SWV |
1 × 10−17 |
10 aM to 1 nM |
50% diluted blood |
Magnetic nanoparticles |
121
|
Conformational changes |
SWV |
3 × 10−17 |
100 aM to 1 nM |
Spiked serum |
Nuclease |
148
|
Junction probes |
DPV |
6.7 × 10−17 |
0.1 to 100 fM |
RNA extract from exosomes |
Magnetic beads |
14
|
Strand displacement reaction |
Stem loop opening |
CV |
5 × 10−16 |
1 fM to 50 fM |
— |
DNA flexibility |
166
|
SWV |
1.4 × 10−15 |
5 fM to 500 pM |
Cell lysates |
Strand displacement reaction |
167
|
SWV |
4.2 × 10−15 |
5 fM to 50 pM |
Cell lysates |
Steam loop opening |
139
|
3 × 10−15 |
Nuclease |
|
(Two sequences detected) |
SWV |
3 × 10−14 |
100 fM to 2 nM |
Tumour cells |
Strand displacement reaction |
42
|
DPV |
1 × 10−13 |
0.1 pM to 10 nM |
None |
Hybridisation chain reaction |
168
|
Dendrimer formation |
DPV |
1 × 10−11 |
100 pM to 1 μM |
Spiked cell lysis |
DNA tetrahedra |
125
|
Nanoparticles |
Stem loop opening |
DPV |
1 × 10−8 |
10 nM to 200 nM |
RNA extracts |
Magnetic beads |
43
|
Unsurprisingly, with such new detection chemistries, the number of reports on clinical or other real-world samples remains modest. Evaluation under realistic diagnostic conditions will yield valuable information of the development of these technologies.
Conflicts of interest
There are no conflicts to declare.
Acknowledgements
PG acknowledges support from a BBSRC PhD scholarship, SL and DOH acknowledge support from a CRUK grant (C49996/A26141). We also thank Dr Robert Channon for proofreading this paper.
References
- L. He and G. J. Hannon, Nat. Rev. Genet., 2004, 5, 522–531 CrossRef CAS PubMed
.
- J. Wang, J. Chen and S. Sen, J. Cell Physiol., 2016, 231, 25–30 CrossRef CAS PubMed
.
- M. Weiland, X. H. Gao, L. Zhou and Q. S. Mi, RNA Biol., 2012, 9, 850–859 CrossRef CAS PubMed
.
- Y. S. Lee and A. Dutta, Annu. Rev. Phytopathol., 2009, 4, 199–227 CrossRef CAS PubMed
.
- S. Mi, J. Zhang, W. Zhang and R. S. Huang, Microrna, 2013, 2, 63–71 CrossRef PubMed
.
- R. E. Drury, D. O'Connor and A. J. Pollard, Front. Immunol., 2017, 8, 1182 CrossRef PubMed
.
- G. Iacomino and A. Siani, Genes Nutr., 2017, 12, 23 CrossRef PubMed
.
- L. Maegdefessel, J. Intern. Med., 2014, 276, 633–644 CrossRef CAS PubMed
.
- O. Issler and A. Chen, Nat. Rev. Neurosci., 2015, 16, 201–212 CrossRef CAS PubMed
.
- D. Sayed and M. Abdellatif, Physiol. Rev., 2011, 91, 827–887 CrossRef CAS PubMed
.
- M. Nordberg, J. Duffus and D. M. Templeton, Journal, 2004, 76, 1033 CAS
.
- V. Prasad, J. Lenzer and D. H. Newman, Br. Med. J., 2016, 352, h6080 CrossRef PubMed
.
- C. P. Price, Br. Med. J., 2001, 322, 1285–1288 CrossRef CAS PubMed
.
- J. Zhang, L. L. Wang, M. F. Hou, Y. K. Xia, W. H. He, A. Yan, Y. P. Weng, L. P. Zeng and J. H. Chen, Biosens. Bioelectron., 2017, 102, 33–40 CrossRef PubMed
.
- R. Wang, L. Wang, X. Xu and W. Jiang, Sens. Actuators, B, 2018, 268, 287–292 CrossRef CAS
.
- J. S. Daniels and N. Pourmand, Electroanalysis, 2007, 19, 1239–1257 CrossRef CAS PubMed
.
- A. Chen and B. Shah, Anal. Methods, 2013, 5, 2158–2173 RSC
.
- D. Grieshaber, R. MacKenzie, J. Vörös and E. Reimhult, Sensors, 2008, 8, 1400–1458 CrossRef CAS PubMed
.
- C. Batchelor-McAuley, E. Katelhon, E. O. Barnes, R. G. Compton, E. Laborda and A. Molina, ChemistryOpen, 2015, 4, 224–260 CrossRef CAS PubMed
.
- D. R. Thevenot, K. Tóth, R. A. Durst and G. S. Wilson, Journal, 1999, 71, 2333 CAS
.
- P. Gai, C. Gu, T. Hou and F. Li, ACS Appl. Mater. Interfaces, 2018, 10, 9325–9331 CrossRef CAS PubMed
.
- K. Deng, Y. Zhang and X. Tong, Anal. Bioanal. Chem., 2018, 410, 1863–1871 CrossRef CAS PubMed
.
- J. Zhang, D. Z. Wu, S. X. Cai, M. Chen, Y. K. Xia, F. Wu and J. H. Chen, Biosens. Bioelectron., 2016, 75, 452–457 CrossRef CAS PubMed
.
- S. Su, W. Cao, W. Liu, Z. Lu, D. Zhu, J. Chao, L. Weng, L. Wang, C. Fan and L. Wang, Biosens. Bioelectron., 2017, 94, 552–559 CrossRef CAS PubMed
.
- Y. Ren, H. Deng, W. Shen and Z. Gao, Anal. Chem., 2013, 85, 4784–4789 CrossRef CAS PubMed
.
- G. Yammouri, J. Mandli, H. Mohammadi and A. Amine, J. Electroanal. Chem., 2017, 806, 75–81 CrossRef CAS
.
- H. Deng, W. Shen, Y. Ren and Z. Gao, Biosens. Bioelectron., 2014, 60, 195–200 CrossRef CAS PubMed
.
- Z. Gao, H. Deng, W. Shen and Y. Ren, Anal. Chem., 2013, 85, 1624–1630 CrossRef CAS PubMed
.
- Y. Peng and Z. Gao, Anal. Chem., 2011, 83, 820–827 CrossRef CAS PubMed
.
- M. Kaplan, T. Kilic, G. Guler, J. Mandli, A. Amine and M. Ozsoz, Biosens. Bioelectron., 2017, 92, 770–778 CrossRef CAS PubMed
.
- D. Voccia, M. Sosnowska, F. Bettazzi, G. Roscigno, E. Fratini, V. De Franciscis, G. Condorelli, R. Chitta, F. D'Souza, W. Kutner and I. Palchetti, Biosens. Bioelectron., 2017, 87, 1012–1019 CrossRef CAS PubMed
.
- J. L. Mott and A. M. Mohr, Semin. Liver Dis., 2015, 35, 3–11 CrossRef PubMed
.
- K. A. Cissell, S. Shrestha and S. K. Deo, Anal. Chem., 2007, 79, 4754–4761 CrossRef CAS
.
- G. Rossetti, P. D. Dans, I. Gomez-Pinto, I. Ivani, C. Gonzalez and M. Orozco, Nucleic Acids Res., 2015, 43, 4309–4321 CrossRef CAS PubMed
.
- X. Y. Piao, L. C. Sun, T. B. Zhang, Y. L. Gan and Y. F. Guan, Acta Biochim. Pol., 2008, 55, 713–720 CAS
.
- T. Naiser, O. Ehler, J. Kayser, T. Mai, W. Michel and A. Ott, BMC Biotechnol., 2008, 8, 48 CrossRef PubMed
.
- A. Bala and Ł. Górski, Anal. Methods, 2016, 8, 236–244 RSC
.
- C. P. Price, Br. Med. J., 2001, 322, 1285–1288 CrossRef CAS PubMed
.
- R. Deng, K. Zhang and J. Li, Acc. Chem. Res., 2017, 50, 1059–1068 CrossRef CAS PubMed
.
- Y. Cheng, L. Dong, J. Zhang, Y. Zhao and Z. Li, Analyst, 2018, 143, 1758–1774 RSC
.
- A. B. Steel, T. M. Herne and M. J. Tarlov, Anal. Chem., 1998, 70, 4670–4677 CrossRef CAS PubMed
.
- J. Yao, Z. Zhang, Z. Deng, Y. Wang and Y. Guo, Analyst, 2017, 142, 4116–4123 RSC
.
- M. Bartosik, R. Hrstka, E. Palecek and B. Vojtesek, Anal. Chim. Acta, 2014, 813, 35–40 CrossRef CAS PubMed
.
- Z. Gao and Y. H. Yu, Biosens. Bioelectron., 2007, 22, 933–940 CrossRef CAS PubMed
.
- E. A. Lusi, M. Passamano, P. Guarascio, A. Scarpa and L. Schiavo, Anal. Chem., 2009, 81, 2819–2822 CrossRef CAS PubMed
.
- F. Li, J. Peng, J. Wang, H. Tang, L. Tan, Q. Xie and S. Yao, Biosens. Bioelectron., 2014, 54, 158–164 CrossRef CAS PubMed
.
- E. Eksin and A. Erdem, J. Electroanal. Chem., 2018, 810, 232–238 CrossRef CAS
.
- Y.-X. Chen, K.-J. Huang and K.-X. Niu, Biosens. Bioelectron., 2018, 99, 612–624 CrossRef CAS PubMed
.
- Q. Li, C. Batchelor-McAuley and R. G. Compton, J. Phys. Chem. B, 2010, 114, 7423–7428 CrossRef CAS PubMed
.
- F. Li, J. Peng, Q. Zheng, X. Guo, H. Tang and S. Yao, Anal. Chem., 2015, 87, 4806–4813 CrossRef CAS PubMed
.
- M. Azimzadeh, M. Rahaie, N. Nasirizadeh, K. Ashtari and H. Naderi-Manesh, Biosens. Bioelectron., 2016, 77, 99–106 CrossRef CAS PubMed
.
- H. A. Rafiee-Pour, M. Behpour and M. Keshavarz, Biosens. Bioelectron., 2016, 77, 202–207 CrossRef CAS PubMed
.
- L. Tian, K. Qian, J. Qi, Q. Liu, C. Yao, W. Song and Y. Wang, Biosens. Bioelectron., 2018, 99, 564–570 CrossRef CAS PubMed
.
- J. Guo, C. Yuan, Q. Yan, Q. Duan, X. Li and G. Yi, Biosens. Bioelectron., 2018, 105, 103–108 CrossRef CAS PubMed
.
- T. Hou, W. Li, X. Liu and F. Li, Anal. Chem., 2015, 87, 11368–11374 CrossRef CAS PubMed
.
- A. A. Gorodetsky, M. C. Buzzeo and J. K. Barton, Bioconjugate Chem., 2008, 19, 2285–2296 CrossRef CAS PubMed
.
- M. N. Islam, M. K. Masud, N. T. Nguyen, V. Gopalan, H. R. Alamri, Z. A. Alothman, M. S. A. Hossain, Y. Yamauchi, A. K. Lam and M. J. A. Shiddiky, Biosens. Bioelectron., 2017, 101, 275–281 CrossRef PubMed
.
- C. R. Ispas, G. Crivat and S. Andreescu, Anal. Lett., 2012, 45, 168–186 CrossRef CAS
.
- L. El Harrad, I. Bourais, H. Mohammadi and A. Amine, Sensors, 2018, 18, 164 CrossRef PubMed
.
- J. Wang, Chem. Rev., 2008, 108, 814–825 CrossRef CAS PubMed
.
- I. Palchetti, Mini-Rev. Med. Chem., 2016, 16, 1125–1133 CrossRef CAS PubMed
.
- D. Zeng, Z. Wang, Z. Meng, P. Wang, L. San, W. Wang, A. Aldalbahi, L. Li, J. Shen and X. Mi, ACS Appl. Mater. Interfaces, 2017, 9, 24118–24125 CrossRef CAS PubMed
.
- T. Hu, L. Zhang, W. Wen, X. Zhang and S. Wang, Biosens. Bioelectron., 2016, 77, 451–456 CrossRef CAS PubMed
.
- Z. Ge, M. Lin, P. Wang, H. Pei, J. Yan, J. Shi, Q. Huang, D. He, C. Fan and X. Zuo, Anal. Chem., 2014, 86, 2124–2130 CrossRef CAS PubMed
.
- S. Hofmann, Y. Huang, P. Paulicka, A. Kappel, H. A. Katus, A. Keller, B. Meder, C. F. Stahler and W. Gumbrecht, Anal. Chem., 2015, 87, 12104–12111 CrossRef CAS PubMed
.
- M. Lin, Y. Wen, L. Li, H. Pei, G. Liu, H. Song, X. Zuo, C. Fan and Q. Huang, Anal. Chem., 2014, 86, 2285–2288 CrossRef CAS PubMed
.
- F. F. Cheng, J. J. Zhang, T. T. He, J. J. Shi, E. S. Abdel-Halim and J. J. Zhu, Analyst, 2014, 139, 3860–3865 RSC
.
- N. Xia, Y. Zhang, X. Wei, Y. Huang and L. Liu, Anal. Chim. Acta, 2015, 878, 95–101 CrossRef CAS PubMed
.
- Z. Gao and Y. Peng, Biosens. Bioelectron., 2011, 26, 3768–3773 CrossRef CAS PubMed
.
- T. Kilic, S. N. Topkaya, D. Ozkan Ariksoysal, M. Ozsoz, P. Ballar, Y. Erac and O. Gozen, Biosens. Bioelectron., 2012, 38, 195–201 CrossRef CAS PubMed
.
- H. Yin, Y. Zhou, C. Chen, L. Zhu and S. Ai, Analyst, 2012, 137, 1389–1395 RSC
.
- Y. X. Chen, W. J. Zhang, K. J. Huang, M. Zheng and Y. C. Mao, Analyst, 2017, 142, 4843–4851 RSC
.
- Y.-X. Chen, X. Wu and K.-J. Huang, Sens. Actuators, B, 2018, 270, 179–186 CrossRef CAS
.
- F. Bettazzi, E. Hamid-Asl, C. L. Esposito, C. Quintavalle, N. Formisano, S. Laschi, S. Catuogno, M. Iaboni, G. Marrazza, M. Mascini, L. Cerchia, V. De Franciscis, G. Condorelli and I. Palchetti, Anal. Bioanal. Chem., 2013, 405, 1025–1034 CrossRef CAS PubMed
.
- S. Campuzano, R. M. Torrente-Rodriguez, E. Lopez-Hernandez, F. Conzuelo, R. Granados, J. M. Sanchez-Puelles and J. M. Pingarron, Angew. Chem., Int. Ed., 2014, 53, 6168–6171 CrossRef CAS PubMed
.
- Z. Gao, Analyst, 2012, 137, 1674–1679 RSC
.
- W. Ma, B. Situ, W. Lv, B. Li, X. Yin, P. Vadgama, L. Zheng and W. Wang, Biosens. Bioelectron., 2016, 80, 344–351 CrossRef CAS PubMed
.
- Y. Zhou, H. Yin, J. Li, B. Li, X. Li, S. Ai and X. Zhang, Biosens. Bioelectron., 2016, 79, 79–85 CrossRef CAS PubMed
.
- Y. Wu, K. Sheng, Y. Liu, Q. Yu and B. Ye, Anal. Chim. Acta, 2016, 948, 1–8 CrossRef CAS PubMed
.
- S. K. Silverman, Trends Biochem. Sci., 2016, 41, 595–609 CrossRef CAS PubMed
.
- M. Liu, D. Chang and Y. Li, Acc. Chem. Res., 2017, 50, 2273–2283 CrossRef CAS PubMed
.
- I. Willner, B. Shlyahovsky, M. Zayats and B. Willner, Chem. Soc. Rev., 2008, 37, 1153–1165 RSC
.
- J. Kosman and B. Juskowiak, Anal. Chim. Acta, 2011, 707, 7–17 CrossRef CAS PubMed
.
- M. Mahdiannasser and Z. Karami, Biosens. Bioelectron., 2018, 107, 123–144 CrossRef CAS PubMed
.
- R. Stoltenburg, C. Reinemann and B. Strehlitz, Biomol. Eng., 2007, 24, 381–403 CrossRef CAS PubMed
.
- A. D. Ellington and J. W. Szostak, Nature, 1990, 346, 818 CrossRef CAS PubMed
.
- X. Cheng, X. Liu, T. Bing, Z. Cao and D. Shangguan, Biochemistry, 2009, 48, 7817–7823 CrossRef CAS PubMed
.
- L. Ding, L. Zhang, H. Yang, H. Liu, S. Ge and J. Yu, Sens. Actuators, B, 2018, 268, 210–216 CrossRef CAS
.
- D. Kong, S. Bi, Z. Wang, J. Xia and F. Zhang, Anal. Chem., 2016, 88, 10667–10674 CrossRef CAS PubMed
.
- L. Liu, C. Song, Z. Zhang, J. Yang, L. Zhou, X. Zhang and G. Xie, Biosens. Bioelectron., 2015, 70, 351–357 CrossRef CAS PubMed
.
- K. Shi, B. Dou, J. Yang, R. Yuan and Y. Xiang, Anal. Chim. Acta, 2016, 916, 1–7 CrossRef CAS PubMed
.
- F. F. Cheng, N. Jiang, X. Li, L. Zhang, L. Hu, X. Chen, L. P. Jiang, E. S. Abdel-Halim and J. J. Zhu, Biosens. Bioelectron., 2016, 85, 891–896 CrossRef CAS PubMed
.
- X. Meng, Y. Zhou, Q. Liang, X. Qu, Q. Yang, H. Yin and S. Ai, Analyst, 2013, 138, 3409–3415 RSC
.
- Y. Yu, Z. Chen, L. Shi, F. Yang, J. Pan, B. Zhang and D. Sun, Anal. Chem., 2014, 86, 8200–8205 CrossRef CAS PubMed
.
- D. A. Smith, L. J. Newbury, G. Drago, T. Bowen and J. E. Redman, Sens. Actuators, B, 2017, 253, 335–341 CrossRef CAS PubMed
.
- M. Labib, S. M. Ghobadloo, N. Khan, D. M. Kolpashchikov and M. V. Berezovski, Anal. Chem., 2013, 85, 9422–9427 CrossRef CAS PubMed
.
- M. Wang, Z. Fu, B. Li, Y. Zhou, H. Yin and S. Ai, Anal. Chem., 2014, 86, 5606–5610 CrossRef CAS PubMed
.
- H. V. Tran, B. Piro, S. Reisberg, H. T. Duc and M. C. Pham, Anal. Chem., 2013, 85, 8469–8474 CrossRef CAS PubMed
.
- K. M. Koo, L. G. Carrascosa, M. J. Shiddiky and M. Trau, Anal. Chem., 2016, 88, 2000–2005 CrossRef CAS PubMed
.
- K. Boriachek, M. Umer, M. N. Islam, V. Gopalan, A. K. Lam, N. T. Nguyen and M. J. A. Shiddiky, Analyst, 2018, 143, 1662–1669 RSC
.
- M. Holzinger, A. Le Goff and S. Cosnier, Front. Chem., 2014, 2, 63 Search PubMed
.
- S. E. Kleijn, S. C. Lai, M. T. Koper and P. R. Unwin, Angew. Chem., Int. Ed., 2014, 53, 3558–3586 CrossRef CAS PubMed
.
- M. Pumera, S. Sánchez, I. Ichinose and J. Tang, Sens. Actuators, B, 2007, 123, 1195–1205 CrossRef CAS
.
- N. Xia and L. Zhang, Materials, 2014, 7, 5366–5384 CrossRef PubMed
.
- T. Kangkamano, A. Numnuam, W. Limbut, P. Kanatharana, T. Vilaivan and P. Thavarungkul, Biosens. Bioelectron., 2018, 102, 217–225 CrossRef CAS PubMed
.
- C. Yang, K. Shi, B. Dou, Y. Xiang, Y. Chai and R. Yuan, ACS Appl. Mater. Interfaces, 2015, 7, 1188–1193 CrossRef CAS PubMed
.
- Y. Wang, X. Zhang, L. Zhao, T. Bao, W. Wen, X. Zhang and S. Wang, Biosens. Bioelectron., 2017, 98, 386–391 CrossRef CAS PubMed
.
- H. Dong, S. Jin, H. Ju, K. Hao, L. P. Xu, H. Lu and X. Zhang, Anal. Chem., 2012, 84, 8670–8674 CrossRef CAS PubMed
.
- N. Yu, Z. Wang, C. Wang, J. Han and H. Bu, Anal. Chim. Acta, 2017, 962, 24–31 CrossRef CAS PubMed
.
- H. Wang, Y. Jian, Q. Kong, H. Liu, F. Lan, L. Liang, S. Ge and J. Yu, Sens. Actuators, B, 2018, 257, 561–569 CrossRef CAS
.
- Z. Lu, H. Tang, D. Wu, Y. Xia, M. Wu, X. Yi, H. Li and J. Wang, Biosens. Bioelectron., 2016, 86, 502–507 CrossRef CAS PubMed
.
- J. Wang, X. Yi, H. Tang, H. Han, M. Wu and F. Zhou, Anal. Chem., 2012, 84, 6400–6406 CrossRef CAS PubMed
.
- F. F. Cheng, T. T. He, H. T. Miao, J. J. Shi, L. P. Jiang and J. J. Zhu, ACS Appl. Mater. Interfaces, 2015, 7, 2979–2985 CrossRef CAS PubMed
.
- Y.-H. Yuan, B.-Z. Chi, S.-H. Wen, R.-P. Liang, Z.-M. Li and J.-D. Qiu, Biosens. Bioelectron., 2017, 97, 325–331 CrossRef CAS PubMed
.
- Y. H. Yuan, Y. D. Wu, B. Z. Chi, S. H. Wen, R. P. Liang and J. D. Qiu, Biosens. Bioelectron., 2017, 97, 325–331 CrossRef CAS PubMed
.
- H. V. Tran, B. Piro, S. Reisberg, L. D. Tran, H. T. Duc and M. C. Pham, Biosens. Bioelectron., 2013, 49, 164–169 CrossRef CAS PubMed
.
- N. Xia, L. Zhang, G. Wang, Q. Feng and L. Liu, Biosens. Bioelectron., 2013, 47, 461–466 CrossRef CAS PubMed
.
- W. Zhu, X. Su, X. Gao, Z. Dai and X. Zou, Biosens. Bioelectron., 2014, 53, 414–419 CrossRef CAS PubMed
.
- Z. Wang, J. Zhang, Y. Guo, X. Wu, W. Yang, L. Xu, J. Chen and F. Fu, Biosens. Bioelectron., 2013, 45, 108–113 CrossRef CAS PubMed
.
- M. Vert, Y. Doi, K.-H. Hellwich, M. Hess, P. Hodge, P. Kubisa, M. Rinaudo and F. Schué, Pure Appl. Chem., 2012, 84, 377–410 CAS
.
- R. Tavallaie, J. McCarroll, M. Le Grand, N. Ariotti, W. Schuhmann, E. Bakker, R. D. Tilley, D. B. Hibbert, M. Kavallaris and J. J. Gooding, Nat. Nanotechnol., 2018 DOI:10.1038/s41565-018-0232-x
.
- J. Gómez-Pastora, X. Xue, I. H. Karampelas, E. Bringas, E. P. Furlani and I. Ortiz, Sep. Purif. Technol., 2017, 172, 16–31 CrossRef
.
- E. Paleček and M. Fojta, Talanta, 2007, 74, 276–290 CrossRef PubMed
.
- P. Yáñez-Sedeño, S. Campuzano and J. M. Pingarrón, Sensors, 2016, 16, 1585 CrossRef PubMed
.
- S. Liu, W. Su, Z. Li and X. Ding, Biosens. Bioelectron., 2015, 71, 57–61 CrossRef CAS PubMed
.
- P. Miao, B. Wang, X. Chen, X. Li and Y. Tang, ACS Appl. Mater. Interfaces, 2015, 7, 6238–6243 CrossRef CAS PubMed
.
- P. Miao, B. Wang, F. Meng, J. Yin and Y. Tang, Bioconjugate Chem., 2015, 26, 602–607 CrossRef CAS PubMed
.
- H. Pei, X. Zuo, D. Pan, J. Shi, Q. Huang and C. Fan, NPG Asia Mater., 2013, 5, e51–e51 CrossRef CAS
.
- R. P. Goodman, R. M. Berry and A. J. Turberfield, Chem. Commun., 2004, 1372–1373, 10.1039/B402293A
.
- N. Xie, S. Liu, X. Yang, X. He, J. Huang and K. Wang, Analyst, 2017, 142, 3322–3332 RSC
.
- N. Mitchell, R. Schlapak, M. Kastner, D. Armitage, W. Chrzanowski, J. Riener, P. Hinterdorfer, A. Ebner and S. Howorka, Angew. Chem., Int. Ed., 2009, 48, 525–527 CrossRef CAS PubMed
.
- H. Pei, N. Lu, Y. Wen, S. Song, Y. Liu, H. Yan and C. Fan, Adv. Mater., 2010, 22, 4754–4758 CrossRef CAS PubMed
.
- P. Gai, C. Gu, H. Li, X. Sun and F. Li, Anal. Chem., 2017, 89, 12293–12298 CrossRef CAS PubMed
.
- C. Gu, P. Gai, X. Liu, J. Liu and F. Li, Sens. Actuators, B, 2018, 270, 1–8 CrossRef CAS
.
- L. Garibyan and N. Avashia, J. Invest. Dermatol., 2013, 133, 1–4 CrossRef PubMed
.
- M. M. Ali, F. Li, Z. Zhang, K. Zhang, D. K. Kang, J. A. Ankrum, X. C. Le and W. Zhao, Chem. Soc. Rev., 2014, 43, 3324–3341 RSC
.
- Q. Li, F. Zeng, N. Lyu and J. Liang, Analyst, 2018, 143, 2304–2309 RSC
.
- X. Qiu, H. Zhang, H. Yu, T. Jiang and Y. Luo, Trends Biotechnol., 2015, 33, 180–188 CrossRef CAS PubMed
.
- C. Yang, B. Dou, K. Shi, Y. Chai, Y. Xiang and R. Yuan, Anal. Chem., 2014, 86, 11913–11918 CrossRef CAS PubMed
.
- A. D. Castaneda, N. J. Brenes, A. Kondajji and R. M. Crooks, J. Am. Chem. Soc., 2017, 139, 7657–7664 CrossRef CAS PubMed
.
- T. Fu, Y. Lyu, H. Liu, R. Peng, X. Zhang, M. Ye and W. Tan, Trends Biochem. Sci., 2018, 43, 547–560 CrossRef CAS PubMed
.
- S. G. Harroun, C. Prévost-Tremblay, D. Lauzon, A. Desrosiers, X. Wang, L. Pedro and A. Vallée-Bélisle, Nanoscale, 2018, 10, 4607–4641 RSC
.
- R. M. Dirks and N. A. Pierce, Proc. Natl. Acad. Sci. U. S. A., 2004, 101, 15275 CrossRef CAS PubMed
.
- D. Evanko, Nat. Methods, 2004, 1, 186 CrossRef CAS
.
- S. Bi, S. Yue and S. Zhang, Chem. Soc. Rev., 2017, 46, 4281–4298 RSC
.
- L. Tian, J. Qi, O. Oderinde, C. Yao, W. Song and Y. Wang, Biosens. Bioelectron., 2018, 110, 110–117 CrossRef CAS PubMed
.
- H. Zhang, Q. Wang, X. Yang, K. Wang, Q. Li, Z. Li, L. Gao, W. Nie and Y. Zheng, Analyst, 2017, 142, 389–396 RSC
.
- P. Miao, B. Wang, Z. Yu, J. Zhao and Y. Tang, Biosens. Bioelectron., 2015, 63, 365–370 CrossRef CAS PubMed
.
- S. Nakayama, L. Yan and H. O. Sintim, J. Am. Chem. Soc., 2008, 130, 12560–12561 CrossRef CAS PubMed
.
- J. Zhang, J. H. Chen, R. C. Chen, G. N. Chen and F. F. Fu, Biosens. Bioelectron., 2009, 25, 815–819 CrossRef CAS PubMed
.
- C. Y. Hong, X. Chen, T. Liu, J. Li, H. H. Yang, J. H. Chen and G. N. Chen, Biosens. Bioelectron., 2013, 50, 132–136 CrossRef CAS PubMed
.
- M. Labib, N. Khan, S. M. Ghobadloo, J. Cheng, J. P. Pezacki and M. V. Berezovski, J. Am. Chem. Soc., 2013, 135, 3027–3038 CrossRef CAS PubMed
.
- C. Li, D. Wu, X. Hu, Y. Xiang, Y. Shu and G. Li, Anal. Chem., 2016, 88, 7583–7590 CrossRef CAS PubMed
.
- L. Liu, N. Xia, H. Liu, X. Kang, X. Liu, C. Xue and X. He, Biosens. Bioelectron., 2014, 53, 399–405 CrossRef CAS PubMed
.
- C. Ma, M. Zhang, S. Chen, C. Liang and C. Shi, Analyst, 2016, 141, 2883–2886 RSC
.
- D. Voccia, F. Bettazzi, E. Fratini, D. Berti and I. Palchetti, Anal. Bioanal. Chem., 2016, 408, 7271–7281 CrossRef CAS PubMed
.
- Y. Zhang, Y. Yan, W. Chen, W. Cheng, S. Li, X. Ding, D. Li, H. Wang, H. Ju and S. Ding, Biosens. Bioelectron., 2015, 68, 343–349 CrossRef CAS PubMed
.
- C. Pohlmann and M. Sprinzi, Anal. Chem., 2010, 82, 4434–4440 CrossRef PubMed
.
- Z. Cai, Y. Song, Y. Wu, Z. Zhu, C. J. Yang and X. Chen, Biosens. Bioelectron., 2013, 41, 783–788 CrossRef CAS PubMed
.
- T. Kilic, S. Nur Topkaya and M. Ozsoz, Biosens. Bioelectron., 2013, 48, 165–171 CrossRef CAS PubMed
.
- M. Labib, N. Khan and M. V. Berezovski, Anal. Chem., 2015, 87, 1395–1403 CrossRef CAS PubMed
.
- L. Liu, Y. Chang, N. Xia, P. Peng, L. Zhang, M. Jiang, J. Zhang and L. Liu, Biosens. Bioelectron., 2017, 94, 235–242 CrossRef CAS PubMed
.
- Z. Gao and Z. Yang, Anal. Chem., 2006, 78, 1470–1477 CrossRef CAS PubMed
.
- X. Wu, Y. Chai, R. Yuan, H. Su and J. Han, Analyst, 2013, 138, 1060–1066 RSC
.
- Y. Li, R. Tian, X. Zheng and R. Huang, Anal. Chim. Acta, 2016, 934, 59–65 CrossRef CAS PubMed
.
- M. Mohammadniaei, T. Lee, J. Yoon, D. Lee and J. W. Choi, Biosens. Bioelectron., 2017, 98, 292–298 CrossRef CAS PubMed
.
- K. Shi, B. Dou, C. Yang, Y. Chai, R. Yuan and Y. Xiang, Anal. Chem., 2015, 87, 8578–8583 CrossRef CAS PubMed
.
- F. Xuan, T. W. Fan and I. M. Hsing, ACS Nano, 2015, 9, 5027–5033 CrossRef CAS PubMed
.
|
This journal is © The Royal Society of Chemistry 2019 |