DOI:
10.1039/D5RA00488H
(Paper)
RSC Adv., 2025,
15, 6994-7003
Discovery of novel theophylline derivatives bearing tetrazole scaffold for the treatment of Alzheimer's disease†
Received
20th January 2025
, Accepted 23rd February 2025
First published on 4th March 2025
Abstract
Alzheimer's disease (AD) is associated with AChE and BACE1 enzymes. Designing inhibitors for preventing these enzymes can be benefit for AD treatment. In this context, theophylline derivatives were generated to prevent the biological activity of AChE and BACE1. In particular, the potential inhibitory of these compounds was rapidly and accurately estimated via knowledge-methods. The in vitro tests were then performed to validate the artificial intelligent approach. Among these, compound 12 exhibited the most potent AChE inhibition with an IC50 of 15.68 μM, while showing limited activity against BACE1. In addition, six compounds were indicated that are able to inhibit AChE, however, the theophylline derivatives play poor performance over the BACE1 target. Atomistic simulations were finally applied to clarify the ligand-binding mechanism to the biological target. The outcomes disclose that theophylline derivatives rigidly form van der Waals interactions to AChE via π-stacking and SC contacts. Overall, the theophylline derivatives may offer a potential scaffold for novel anti-AD agents.
Introduction
Alzheimer's disease (AD) is a multifaceted neurodegenerative disorder, commonly associated with progressive cognitive decline (impairment), memory loss and sudden behavioral shifts. Research shows that individuals diagnosed with Alzheimer's dementia at age 65 or older typically live for an average of four to eight years, although some may survive up to 20 years.1 As of today, an estimated 6.9 million Americans aged 65 and older are living with Alzheimer's dementia, a figure that is projected to rise significantly to 13.8 million by 2060, barring the medical advancements to prevent or cure the disease. In 2020 and 2021, amid the COVID-19 pandemic, Alzheimer's disease remained a leading cause of death, ranking seventh in the United States.1 Despite extensive research into AD-type dementia, current treatments offer only temporary and limited effects.2
Several hypotheses have been proposed for the underlying cause of Alzheimer's disease, focusing on different pathological mechanisms. Some key hypotheses including amyloid-beta, cholinergic, and metal ion hypotheses.3,4 Despite ongoing research, only a limited number of drugs for Alzheimer's have been approved by the FDA and EMA. These includes: galantamine, rivastigmine, donezepil, lecanemab, donanemab, aducanumab.5,6 Most available treatments focus on the cholinergic hypothesis, which highlights the role of acetylcholine (ACh) in cognitive function. Thus, targeting of AChE emerges as a highly promising therapeutics strategy for the treatment of AD. The binding site of AChE is divided into three distinct regions, including the catalytic active site (CAS), the binding gorge, and the peripheral anionic site (PAS). An effective AChE inhibitor must either bind to the CAS or PAS region, or possess a linker of suitable length and structure that bridges these two sites, allowing interaction with both, especially have two aromatic residues.7 In addition, it is well-established that AD is associated with the self-assembly of Aβ peptides, a cornerstone of the amyloid cascade hypothesis. In the amyloidogenic pathway, the amyloid precursor protein (APP), a transmembrane protein, undergoes proteolytic cleavage by β- and γ-secretases, resulting in the generation of Aβ peptides.8 Therefore, targeting β-secretase (BACE-1) inhibition presents a promising therapeutic strategy for preventing AD.
Theophylline, a methylxanthine drug, has been associated with a reduced risk of neurodegenerative diseases, including AD.9 The ability of this compound to cross the blood–brain barrier positions them as attractive scaffolds for the development of neuroprotective agents. Theophylline has been widely studied to develop AChE inhibitory compounds because its derivatives are capable of inhibiting AChE to the micromolar range (Fig. 1A),9 and also reduce Aβ aggregation.10 In addition, tetrazole is heterocyclic contain four nitrogen atoms, and tetrazole-bearing compounds have shown potential in inhibiting AChE with IC50 values in the micromolar range (Fig. 1B).11
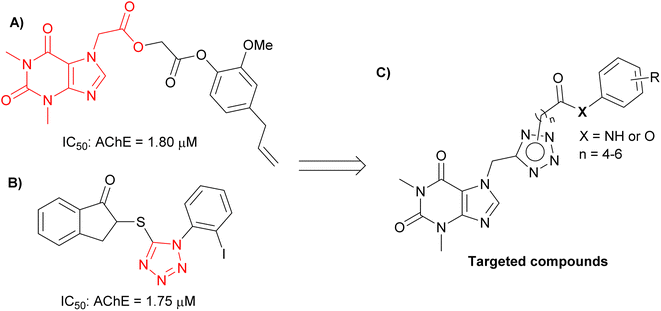 |
| Fig. 1 (A) Structure of theophylline compound as an AChE inhibitor; (B) structure of tetrazol compound as an AChE inhibitor; (C) design targeted compounds. | |
In the present study, we employed a theophylline-tetrazole scaffold as a core aromatic residue, linked with various phenyl derivatives by alkylamide or alkylester linkages, to design, synthesize, and evaluate novel compounds (Fig. 1C) for their inhibitory activity against AChE and BACE-1. The objective was to expand the repertoire of potential AChE and BACE-1 inhibitors. The ligand-binding affinity of these compounds was subsequently characterized through both computational and experimental approaches. Furthermore, their drug-likeness properties were assessed to identify privileged scaffolds for the development of innovative anti-AD agents.
Materials & methods
Chemistry
Reagents sourced from domestic and international suppliers (Merck, Sigma-Aldrich) were employed without further purification. Solvents were procured from Merck, Sigma-Aldrich, Fisher, and suppliers in South Korea and China. The progression of reactions was monitored via thin layer chromatography (TLC) using Merck Kieselgel 60F254 plates, visualized under UV light at 254 nm.
Procedure for synthesis of compound 2. A solution of theophylline (1) (5 mmol, 900.8 mg) in acetone (10 ml) was added K2CO3 (827.7 mg, 6 mmol) and KI (16.6 mg, 0.1 mmol). After stirring for further 15 min, 0.36 ml of chloroacetonitrile (6 mmol) was dropped slowly into the mixture. The reaction mixtures were again stirred at 60 °C for 4 h. After completion of the reaction, the resulting mixtures were evaporated under reduced pressure to give the residues, which were extracted with dichloromethane (DCM) (5 × 30 ml) and 30 ml of water. The organic layer was combined, dried over Na2SO4 and purified by chromatography on a silica gel column (EA/n-hexane = 1/1, v/v) to give an off-white solid 2.
General procedure for synthesis of compounds 3–6. A mixture of compound 2 (1 mmol) in dimethylsulfoxide (DMSO) (5 ml) was added NaN3 (97.5 mg, 1.5 mmol) and ZnCl2 (13.6 mg, 0.1 mmol). The resulting mixtures were stirred at 120 °C for 2 h, and checked by thin layer chromatography (TLC) with mobile phase as ethyl acetate (EA)/n-hexan (3/1, v/v), then halogenide ester derivatives (1.2 mmol) was added into the mixture. The reaction mixtures were again stirred at 90 °C for 12 h. After completion of the reaction, the resulting mixtures were cooled, and 40 ml solution of saturated Na2CO3 was added slowly, after which the solution was filtered through Celite and extracted with EA (30 ml), washed with water (3 × 50 ml), The organic layers were combined, and dried over Na2SO4, and purified by chromatography on a silica gel column (EA/n-hexane = 3/1, v/v) to give 3–6 as an off-white solid.
General procedure for synthesis of compounds 7–10. A solution of compounds 3–6 (1 equiv.) in methanol (10 ml) was added NaOH (2 equiv.), which was dissolved in 1 ml of water. The reaction mixtures were stirred at room temperature for 2 h and checked by TLC with mobile phase as EA/n-hexan (1/1, v/v). After completion of the reaction, the resulting mixtures were adjusted to pH = 2–3 by concentrated hydrochloric acid solution, evaporated under reduced pressure, and dried to give 7–10 as off-white solid.
General procedure for synthesis of compounds 11–27. A mixture of carboxylic acids 7–10 (1 equiv.) dissolved in DMF (0.5 ml), EDC.HCl (1.2 equiv.), HOBt (1.2 equiv.), DMAP (1.2 equiv.) and phenol/aniline derivatives (1.2 equiv.) in DCM (10 ml) was stirred at room temperature for 12 h. The reaction mixture was evaporated under reduced pressure and extracted with EA (30 ml), washed with water (3 × 50 ml), and dried over Na2SO4, evaporated under reduced pressure, and purified by chromatography on a silica gel column (EA/n-hexane = 3
:
1) to give 11–27 as off-white solid.
Acetylcholinesterases inhibition assay
A SPL 96-well plate (Korea), 1000 μL and 200 μL tips (Germany), a 1000 μL and 200 μL micropipette (Nichiryo, Japan), AChE enzyme (Sigma-Aldrich, USA), acetylthiocholine iodide (ATCI, Sigma-Aldrich, USA), 5,5-dithiobis(2-nitrobenzoic acid) (DTNB, TCI, Japan), and galantamine hydrobromide (TCI, Japan) were employed to evaluate the AChE inhibitory activity of the synthesized compounds using the modified Ellman's method.12,13 AChE inhibition was assessed on a Varioskan Lux 96-well plate reader. Each compound was tested at five concentrations (256 μM to 16 μM) in triplicate. The percentage inhibition was calculated using the standard formula.
% inhibition = [1 − (test sample − blank)/(control − blank)] × 100% |
In which: blank: ATCI, DNTB in pH 8.0 buffer solution control: ATCI, DTNB and enzyme in pH 8.0 buffer test sample: test substance (or galantamine hydrobromide control), ATCI, DTNB and enzyme in pH 8.0 buffer solution. The IC50 values were determined using TableCurve 2Dv4 software.
Beta secretasae 1 inhibition activity
BACE1 inhibitory activity of synthesized compounds (11–27) was examined using the BACE1 Inhibitor Screening Kit (Beyotime Biotech, Shanghai, China) according to the manufacturer's instructions. Briefly, BACE1 was incubated with various concentrations of compounds for 5 min at 37 °C in a black 96-well plate, followed by adding a specific fluorescence resonance energy transfer (FRET) substrate into the reaction mixture. The cleavage of FRET substrate was monitored through measurement of fluorescent intensity at 325/393 nm every 3 min for 30 min using a multimode microplate reader (VarioskanLux, ThermoScientific). The inhibitory percentage of BACE1 activity was calculated as following: I(%) = (ΔFIcontrol − ΔFIcompound) × 100/ΔFIcontrol, in which ΔFI denotes the change of fluorescent intensity per min. The inhibitory activity of compounds was initially screened at a fixed concentration of 200 μM.
Machine learning calculation
Machine learning (ML) models were employed to fast and accurate predict the ligand-binding free energy to AChE and BACE1 targets, which were developed previously.14,15 Two models use the XGBoost algorithm that adopted high Pearson correlation coefficient, R = 0.8 ± 0.03 and R = 0.77 ± 0.02, with the respective experiments, respectively.
Molecular docking simulation
AutoDock Vina16 (Vina) using altered empirical parameters17 (mVina) were used to rapidly evaluate the ligand-binding affinity and pose to AChE enzyme. In particular, the AChE structure was downloaded from the Protein Data Bank with identify of 4EY7.18 The Open Babel package19 was employed to generate the structure of theophylline derivatives. The AutoDockTools20 was first applied to parameterize the protein and ligand. The docking grid size was selected as 24 × 24 × 24 Å, which center of grid was chosen as the center of mass of the native inhibitors. The docking exhaustiveness was chosen as 8 due to the previous test.17 The ligand-binding pose was selected according to the lowest binding energy.
MD simulation
The interaction of the AChE + inhibitors and free inhibitors with solution can be evaluated by using atomistic simulation via GROMACS version 2019.21 Due to previous benchmarks,22,23 the Amber99SB-iLDN force field24 was used to model the AChE enzyme and the neutralized ions. In combination with the Amber99SB-iLDN force field, the TIP3P water model25 was utilized to topologize the water molecule. Besides, the general Amber force field (GAFF)26 was utilized to parameterize the ligands. In particular, the chemical information of ligands was obtained by ACPYPE27 and AmberTools18 (ref. 28) approaches from the outcome of quantum calculations using B3LYP at 6-31G(d,p) level of theory.
The AChE + ligand system was put into a dodecahedron periodic boundary conditions (dPBC) box with a size of ca. 940 nm3 that comprised of ca. 92
000 atoms totally. The free inhibitor was put into the dPBC box with a size ca. 60 nm3 consisting of 6000 atoms totally. Initially, the steepest descent method was energetically minimized. The minimized conformation was then relaxed during NVT and NPT simulations. It should be noted that all atoms of the complex was positionally restrained during NVT simulations via a small harmonic force. The non-bonded interaction would be counted within a range of 9.0 Å. In particular, the van der Waals and electrostatics interactions were treated via the cut-off scheme and particle mesh Ewald method.
Free energy perturbation simulation
The free energy perturbation method29,30 was executed to obtain the binding free energy between inhibitors and AChE. In particular, the inhibitor was turned from full-interaction to non-interaction two times, in solvated complex and in free ligand in solution, via the coupling parameter λ. During which, each simulation is length of 3.0 ns. More details was described in the previous work.31
Analysis tools
A webapp server of ChemAxon, chemicalize,32 was carried out to predict the inhibitor protonation states. The principal component analysis (PCA)33 was performed to calculate first and second principal components, which were used to construct the free energy landscape (FEL) of non-hydrogen atoms of the AChE active site and ligands. The representative conformation of the AChE + ligand system was found via the clustering method,34,35 which was integrated in a tools of GROMACS “gmx cluster”. The interaction diagrams of AChE and BACE1 to ligand were prepared by using the Maestro free version.36
Physicochemical properties and drug-likeness predictions
Some physicochemical properties and drug-likeness predictions of synthesized compounds were predicted by using the SwissADME platform.37 A potential oral drug should observe the criteria of Lipinski's rule of five38 and Veber's rule.39 The PreADMET web-server40 was employed to assess the metrics corresponding to the capability of the most potential compound to be able across blood–brain barrier (BBB) and human intestinal absorption (HIA) as well as the toxicity of this compound.
Results and discussion
ML calculations
Developing possible inhibitors to obstruct the biological action of AChE remains a significant focus,41–43 particularly as this enzyme is the most effective target for treating Alzheimer's disease to far.44–48 Recently, ML models are usually generated to accurately and rapidly evaluate the ligand-binding affinity.49 As mentioned above, theophylline derivatives (see Fig. 1C) were generated in order to prevent the activity of AChE and BACE1 enzymes.15,50
The designed compounds based on theophylline molecule were fast and accurately assessed the ligand-binding affinity, ΔGML, using the trained ML models of AChE and BACE1 targets. The outcomes were shown in Table S1 of the ESI.† The predicted ligand-binding affinity to AChE and BACE1 range from −10.56 to −8.80 and −10.07 to −9.05 kcal mol−1, respectively. Moreover, for AChE target, ML model using the XGBoost method adopted a value of RMSE = 1.36 ± 0.10 kcal mol−1 and correlation coefficient of R = 0.81 ± 0.03.50 Furthermore, the corresponding metrics of the BACE1 target are of RMSE = 1.02 + 0.05 kcal mol−1 and R = 0.77 ± 0.02. The model was also based on the XGBoost method.15 It may thus be argued that all of designed compounds are able to inhibit the biological activity of AChE and BACE1 enzymes.
Chemistry
The synthesis of theophylline bearing tetrazol scaffold derivatives was illustrated in Scheme 1. Briefly, theophylline was alkylated by chloroacetonitrile using potassium carbonate and potassium iodide (catalyst) in acetone to form 2. Ester 3–6 prepared through click reaction using sodium azide and ZnCl2 in N,N-dimethylformamide (DMF), then alkylation with halogenide-ester derivatives. The structure of isomers were confirmed by 2D-NMR before carrying out hydrolysis by sodium hydroxide in methanol to form acid 7–10. Next, the amide or ester derivatives 11–27 were obtained through EDC/HOBt coupling with aniline or phenol derivatives.
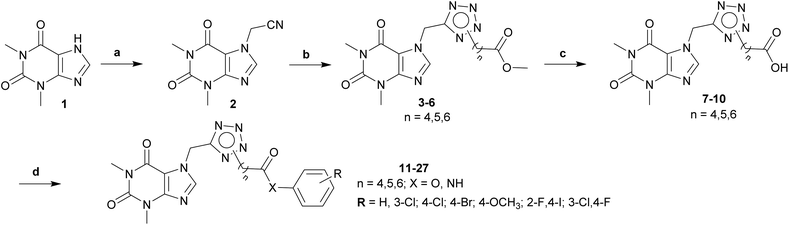 |
| Scheme 1 General procedure for synthesis final compounds 11–27. Reagents and conditions: (a) ClCH2CN, K2CO3, KI, acetone, 60 °C, 4 h; (b) (i) NaN3, ZnCl2, DMSO, 120 °C, 2 h; (ii) halogenide-ester derivatives, DMSO, 90 °C, 12 h; (c) (i) NaOH, MeOH, rt, 2 h; (ii) HCl to pH = 2–3; (d) aniline/phenol derivatives, EDC·HCl, DMAP, HOBt, DCM, DMF, rt to 50 °C, 12 h. | |
AChE and BACE1 inhibition activity
Based on the ML results (Table S1†), seventeen theophylline derivatives were selected, synthesized, and evaluated AChE and BACE-1 inhibition activities (Table 1). Most of the synthesized compounds showed moderate to low activity on AChE with the IC50 value below 200 μM compared with a IC50 value of 2.50 μM of positive control, galatamine hydrobromide. In particular, compound 12 with 4-carbon linker and substituent groups 2-F–4-I on the phenyl ring was the most potent with a IC50 value of 15.68 μM. The substitution at 4 positions on the phenyl ring was seen better for the activity on all cases of the linker. Specially, compounds bearing a 4-bromo substituent (15, 22, 25) demonstrated better AChE inhibitory activity relative to those substituted with 3-Cl, 4-Cl, or 4-OCH3 groups. These findings suggest that the 4-bromo substituent may be particularly well-suited for interactions with the AChE active site. For the 4-carbon linker, compound 11 with non-substituted group on the phenyl ring displayed an IC50 value of 110.31 μM. And the IC50 value decreased about seven folds when 2-F–4-I groups were on the phenyl ring (compound 12, IC50 = 15.68 μM). In the case of 5-carbon liker, the activity of compound 14 was improved from 153.43 μM to 84.07–114.98 μM (compounds 15–17) when the 3-Cl was replaced by other substituents at 4 positions. Similarly, with the 6-carbon linker the values changed from 220.62 μM (compound 20) to 63.51–126.08 μM (compound 21–23), respectively. Subsequently, when N2 isomers were changed to N1 isomers (compound 25–27) their activities did not improve so much (IC50 = 55.35–143.49 μM). Similarly, changing amide to ester (compounds 13, 18, 19, 24) did not lead to the more potent compounds. For beta-secretase 1 (BACE1) inhibition activity, all synthesized compounds did not show significant inhibition of the enzyme at the concentration of 200 μM. None of them could inhibit up to 50% of the enzyme activity at the testing concentration. The results suggested that these compounds were highly selective for AChE (compared to BACE1).
Table 1 In vitro AChE and BACE1 inhibition activites of synthesized compounds (11–27)
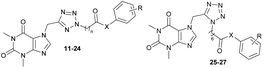
|
Cpd |
n |
X |
R |
ICAChE50 (μM) |
% Inhibition of BACE1 at 200 μM |
11 |
4 |
NH |
H |
110.31 ± 17.53 |
−0.32 ± 2.05 |
12 |
4 |
NH |
2-F, 4-I |
15.68 ± 0.06 |
18.18 ± 3.9 |
13 |
4 |
O |
4-OCH3 |
303.33 ± 34.06 |
1.40 ± 0.03 |
14 |
5 |
NH |
3-Cl |
153.43 ± 45.01 |
−2.01 ± 0.83 |
15 |
5 |
NH |
4-Br |
84.07 ± 20.19 |
8.94 ± 1.45 |
16 |
5 |
NH |
3-Cl, 4-F |
114.98 ± 10.56 |
−1.54 ± 1.19 |
17 |
5 |
NH |
2-F, 4-I |
104.69 ± 12.78 |
18.71 ± 2.26 |
18 |
5 |
O |
H |
94.36 ± 8.27 |
0.07 ± 3.57 |
19 |
5 |
O |
4-OCH3 |
266.98 ± 30.37 |
−1.23 ± 0.35 |
20 |
6 |
NH |
3-Cl |
220.62 ± 37.73 |
10.72 ± 0.54 |
21 |
6 |
NH |
4-Cl |
126.08 ± 22.41 |
12.17 ± 0.47 |
22 |
6 |
NH |
4-Br |
63.51 ± 2.48 |
1.56 ± 3.43 |
23 |
6 |
NH |
3-Cl, 4-F |
109.94 ± 17.17 |
12.57 ± 0.45 |
24 |
6 |
O |
4-OCH3 |
105.77 ± 25.73 |
0.74 ± 0.17 |
25 |
6 |
NH |
4-Br |
70.30 ± 0.10 |
−0.48 ± 0.76 |
26 |
6 |
NH |
3-Cl, 4-F |
55.35 ± 18.56 |
8.89 ± 0.49 |
27 |
6 |
NH |
2-F, 4-I |
143.49 ± 23.07 |
7.69 ± 0.86 |
Galantamine hydrobromide |
|
|
|
2.50 ± 0.21 |
|
Verubecestat |
|
|
|
|
IC50 = 49.21 ± 7.20 (nM) |
Molecular docking simulations
Due to experimental results, physics-based methods including molecular docking and MD simulations were employed to clarify the physical insights into the ligand-binding process.51 In this context, the mVina package51 was suggested that form a successful-docking rate and correlation coefficient of 90 ± 10% and 0.86 ± 0.09, respectively.51 The obtained docking energy was described in Table 2 implying that the docking ligand affinity overestimates the ML calculations. It is in good agreement with the previous work that the mVina often adopts an overestimated outcome compared with experimental and ML results.17,52 Interestingly, six compounds almost form SC contacts to AChE-residues. Besides, the π-stacking interaction between six compounds to Tpr86, Tpr286, and Tyr341 (cf. Table S2 in ESI†). There is no HB contact between two molecules was found. The outcomes imply that vdW interaction may plays an important role in the ligand binding process.
Table 2 The in silico binding energy of compounds 12, 15, 18, 22, 25, and 26 to AChEa
Cpd |
n |
X |
R |
ΔGAChEmVina |
ΔGAChEcou |
ΔGAChEvdW |
ΔGAChEFEP |
The unit of energy is of kcal mol−1. |
12 |
4 |
NH |
2-F, 4-I |
−14.1 |
0.14 |
−13.79 |
−13.65 ± 0.33 |
15 |
5 |
NH |
4-Br |
−14.6 |
−0.15 |
−17.76 |
−17.91 ± 1.03 |
18 |
5 |
O |
H |
−13.5 |
−1.78 |
−13.33 |
−15.11 ± 0.87 |
22 |
6 |
NH |
4-Br |
−15.2 |
−2.24 |
−15.39 |
−17.63 ± 1.30 |
25 |
6 |
NH |
4-Br |
−14.3 |
−5.17 |
−19.78 |
−24.95 ± 2.54 |
26 |
6 |
NH |
3-Cl, 4-F |
−14.7 |
−1.84 |
−10.39 |
−12.23 ± 4.44 |
Atomistic simulations
To rapidly gain the outcomes, molecular docking simulations often apply several computational restraints such as using united-atoms, rigid receptors, etc.20,53 Therefore, MD simulations were utilized to validate the molecular docking results.50,54 The ligand-binding mechanisms can be obtained.55,56 In this work, the AChE + ligand complexes were thus mimicked in solution starting from docking conformation. Each MD simulation was 200 ns and repeated two times. During conventional MD simulations, the AChE + ligand complexes archive the equilibrium domains after ca. 75 ns (cf. Tables S3 and S4 of the ESI†).
Over equilibrium snapshots, analyzed tools were employed to discover the physical insights into the ligand-binding mechanism of theophylline derivatives to AChE enzyme.57–59 The popular conformations of the complexes were obtained by using a combination of FEL and clustering methods.34,35 In particular, the PCA approach33 was employed to calculate the first and second principal components, denoted as CV1 and CV2. The metrics were then used as two reaction coordinates to construct the FEL. The obtained FEL was then provided and shown in Fig. 2. The minima of the AChE – ligand complexes corresponding to the most popular conformations was denoted as a, a′, b, c, c′, d, d′, e and f, respectively. In details, the corresponding coordinates (CV1, CV2) of the minima is (−2.71, 0.31), (2.59, 0.42), (−1.48, −0.15), (−1.18, −1.02), (2.04, −0.76), (−2.88, −1.60), (2.40, 0.00), (−1.04, −1.04), and (1.26, −0.20), respectively. The population of the minima at the zero free energy is of 4.6, 1.9, 2.9, 1.8, 9.2, 3.5, 5.9, 2.1, and 10.8%, respectively.
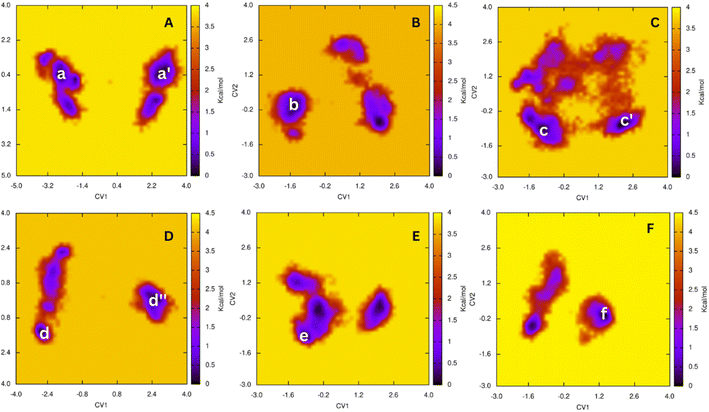 |
| Fig. 2 FELs of six complexes AChE + compounds over equilibrium conformations via PCA approach during interval 100–200 ns of MD simulations. In particular, (A–F) patterns are FEL of compounds 12, 15, 18, 22, 25, and 26, respectively. | |
The representative conformations of the AChE – ligand complexes were then searched using the clustering method.34,35 In particular, the typical structure was archived via 0.3 nm non-hydrogen atom RMSD estimation. The two-dimension ligand-binding pose was thus analyzed and described in Fig. 3. In particular, theophylline derivatives adopt rigid π-stacking contacts to residues tryptophan and tyrosine such as Trp86, Tyr124, Trp286, Tyr337, and Tyr341. Besides, HB contacts between ligands and AChE were mostly measured between residues tyrosine including Tyr124 and ligands.
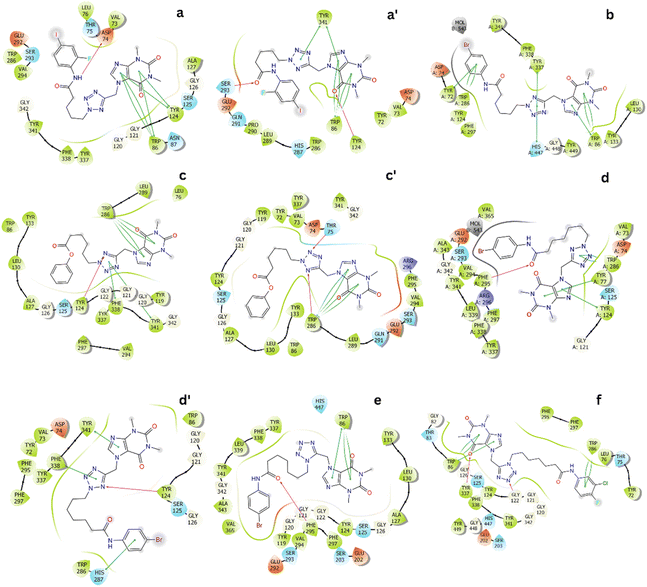 |
| Fig. 3 Two-dimension ligand-binding poses of AChE – theophylline derivatives matching to the minima a, a′, b, c, c′, d, d′, e and f. The diagram were generated via the Maestro free version.36 | |
In addition, ligands form SC contacts to more than 20 residues of the AChE. The obtained results are consistent with perturbation simulations, as mentioned below, in which indicated that ligands form rigid vdW interactions to AChE.
Perturbation calculation
The perturbation simulations were performed to calculate the binding free energy between AChE and theophylline derivatives. The last structures of the complexes were used as initial conformation of the simulations. Besides, the last structures of free ligand in solution were also used as starting shape for λ-alteration simulations. The ligand-binding free energy of theophylline derivatives to AChE was acquired and designated in Table 2. The outcomes, range from −12.23 ± 4.44 to −24.95 ± 2.54 kcal mol−1, are in good agreement with experiments that six compounds named 12, 15, 18, 22, 25, and 26 can be play as potential inhibitors for preventing the biological activity of AChE. Moreover, the vdW interaction free energy adopts in the range from −10.39 to −19.78 kcal mol−1 that rules the ligand-binding free energy since the electrostatic interaction free energy diffuses in the range from 0.14 to −5.17 kcal mol−1. The observation confirm the finding above when ligands mostly form SC and π-stacking contacts to AChE instead of HB one.
Physicochemical properties and drug-likeness predictions
The synthesized compounds were predicted drug-likeness based on Linpinski's rule of five and Veber's rule through the physicochemical properties: the log
P, molecular weight, number of rotatable bonds, number of hydrogen bonding acceptor/donors, and topological polar surface area (Table 3). None of them, except compound 24, violated more than one property indicating they can use the oral route with good bioavailability. Furthermore, the BBB and HIA values observed for compound 12 were comparable to those of galantamine (cf. Table S5 in ESI†). These data indicate that compound 12 may exhibit favorable BBB permeability and absorption from the human intestine, suggesting its potential suitability as a drug candidate.
Table 3 The ADME prediction of the AChE inhibitor compounds 11–27a
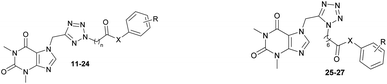
|
Cpd |
n |
X |
R |
Formula |
log P |
MW |
#Rot |
#H-a |
#H-d |
TPSA (Å2) |
*TPSA: Topological Polar Surface Area; MW: Molecular Weight; #H-a: Hydrogen Bonding Acceptor; #H-d: Hydrogen Bonding Donor; #Rot: Number of Rotatable Bonds; **RO5: Lipinski's rule of five. |
11 |
4 |
NH |
H |
C20H23N9O3 |
0.95 |
437.46 |
9 |
7 |
1 |
134.52 |
12 |
4 |
NH |
2-F, 4-I |
C20F21FIN9O3 |
2.06 |
581.34 |
9 |
8 |
1 |
134.52 |
13 |
4 |
O |
4-OCH3 |
C21H24N8O5 |
1.42 |
468.47 |
10 |
9 |
0 |
140.95 |
14 |
5 |
NH |
3-Cl |
C21H24ClN9O3 |
1.92 |
485.93 |
10 |
7 |
1 |
134.52 |
15 |
5 |
NH |
4-Br |
C21H24BrN9O3 |
2.00 |
530.38 |
10 |
7 |
1 |
134.52 |
16 |
5 |
NH |
3-Cl, 4-F |
C21H23ClFN9O3 |
2.22 |
503.92 |
10 |
8 |
1 |
134.52 |
17 |
5 |
NH |
2-F, 4-I |
C21H23FIN9O3 |
2.39 |
595.37 |
10 |
8 |
1 |
134.52 |
18 |
5 |
O |
H |
C21H24N8O4 |
1.72 |
452.47 |
10 |
8 |
0 |
131.72 |
19 |
5 |
O |
4-OCH3 |
C22H26N8O5 |
1.75 |
482.49 |
11 |
9 |
0 |
140.95 |
20 |
6 |
NH |
3-Cl |
C22H26ClN9O3 |
2.15 |
499.95 |
11 |
7 |
1 |
134.52 |
21 |
6 |
NH |
4-Cl |
C22H26ClN9O3 |
2.38 |
499.95 |
11 |
7 |
1 |
134.52 |
22 |
6 |
NH |
4-Br |
C22H26BrN9O3 |
2.22 |
544.40 |
11 |
7 |
1 |
134.52 |
23 |
6 |
NH |
3-Cl, 4-F |
C22H25ClFN9O3 |
2.40 |
517.94 |
11 |
8 |
1 |
134.52 |
24 |
6 |
O |
4-OCH3 |
C23H28N8O5 |
2.14 |
496.52 |
12 |
9 |
0 |
140.95 |
25 |
6 |
NH |
4-Br |
C22H26BrN9O3 |
2.18 |
544.40 |
11 |
7 |
1 |
134.52 |
26 |
6 |
NH |
3-Cl, 4-F |
C22H25ClFN9O3 |
2.38 |
517.94 |
11 |
8 |
1 |
134.52 |
27 |
6 |
NH |
2-F, 4-I |
C22H25FIN9O3 |
2.53 |
609.40 |
11 |
8 |
1 |
134.52 |
RO5** |
|
|
|
|
≤5 |
≤500 |
|
≤10 |
≤5 |
|
Veber |
|
|
|
|
|
|
≤10 |
Total H-bond ≤12 |
≤140 |
Conclusions
Seventeen theophylline derivatives were designed, evaluated anti-AD through their in vitro AChE and BACE1 inhibitor ability. In which, compound 12 demonstrated the best activity on AChE with the IC50 of 15.68 μM but poor activity on BACE1. In addition, six compounds showed significant AChE inhibition with IC50 value less than 100 μM, while their activity against BACE1 was limited. Atomistic simulations revealed that theophylline derivatives interact strongly with AChE, primarily through van der Waals forces such as π-stacking and sulfur–carbon (SC) contacts. All of seventeen compounds passed the Linpinski's rule of five and Veber's rule for the orally drugs. These findings suggest that inhibiting AChE with theophylline derivatives may offer a potential therapeutic strategy for AD.
Data availability
The data that support the findings of this study are available from the corresponding author, upon reasonable request.
Author contributions
P.-T. Tran conceived and planned the experiments. N. V. Hung, L. Q. Tien, V. N. H. Linh, H. Tran, H.-V. Hai, and T. T. T. Hien carried out the synthetic experiments. T. K. Nguyen, D.-V. Pham, and T. X. Nguyen carried out the bio-assay experiments. S. T. Ngo, T. H. Nguyen, and Q. M. Thai planned and carried out the simulations. P.-T. Tran, N. V. Hung, L. Q. Tien, V. N. H. Linh, and H. Tran contributed to sample preparation. P.-T. Tran, N. V. Hung, H.-V. Hai, Q. M. Thai and S. T. Ngo contributed to the interpretation of the results. P.-T. Tran took the lead in writing the manuscript. All authors provided critical feedback and helped shape the research, analysis and manuscript.
Conflicts of interest
There are no conflicts to declare.
Acknowledgements
This research was funded by Vingroup Innovation Foundation (VINIF) under project code VINIF.2022.DA00061.
References
- Alzheimer's Association Report: 2024 Alzheimer's disease facts and figures, Alzheimers Dement., 2024, vol. 20, 5, pp. 3708–3821 Search PubMed.
- E. Passeri, K. Elkhoury, M. Morsink, K. Broersen, M. Linder, A. Tamayol, C. Malaplate, F. T. Yen and E. Arab-Tehrany, Alzheimer's disease: treatment strategies and their limitations, Int. J. Mol. Sci., 2022, 23(22), 13954 CrossRef CAS PubMed.
- L.-L. Chen, Y.-G. Fan, L.-X. Zhao, Q. Zhang and Z.-Y. Wang, The metal ion hypothesis of Alzheimer's disease and the anti-neuroinflammatory effect of metal chelators, Bioorg. Chem., 2023, 131, 106301 CrossRef CAS PubMed.
- M. Agarwal, M. R. Alam, M. K. Haider, M. Z. Malik and D. K. Kim, Alzheimer’s Disease: An Overview of Major Hypotheses and Therapeutic Options in Nanotechnology, Nanomaterials, 2020, 11(1), 59 CrossRef PubMed.
- M. B. Colovic, D. Z. Krstic, T. D. Lazarevic-Pasti, A. M. Bondzic and V. M. Vasic, Acetylcholinesterase inhibitors: pharmacology and toxicology, Curr. Neuropharmacol., 2013, 11(3), 315–335 CrossRef CAS PubMed.
- I. Terao and W. Kodama, Comparative efficacy, tolerability and acceptability of donanemab, lecanemab, aducanumab and lithium on cognitive function in mild cognitive impairment and Alzheimer's disease: a systematic review and network meta-analysis, Ageing Res. Rev., 2024, 102203 CrossRef CAS PubMed.
- S. Ghafary, R. Ghobadian, M. Mahdavi, H. Nadri, A. Moradi, T. Akbarzadeh, Z. Najafi, M. Sharifzadeh, N. Edraki, F. H. Moghadam and M. Amini, Design, synthesis, and evaluation of novel cinnamic acid-tryptamine hybrid for inhibition of acetylcholinesterase and butyrylcholinesterase, Daru, J. Pharm. Sci., 2020, 28(2), 463–477 CrossRef PubMed.
- J. A. Hardy and G. A. Higgins, Alzheimer's disease: the amyloid cascade hypothesis, Science, 1992, 256(5054), 184–185 Search PubMed.
- A. A. Elgazar, R. A. El-Domany, W. M. Eldehna and F. A. Badria, Theophylline-based hybrids as acetylcholinesterase inhibitors endowed with anti-inflammatory activity: synthesis, bioevaluation, in silico and preliminary kinetic studies, RSC Adv., 2023, 13(36), 25616–25634 RSC.
- D. Janitschke, C. Nelke, A. A. Lauer, L. Regner, J. Winkler, A. Thiel, H. S. Grimm, T. Hartmann and M. O. W. Grimm, Effect of Caffeine and Other Methylxanthines on Aβ-Homeostasis in SH-SY5Y Cells, Biomolecules, 2019, 9(11), 689 CrossRef CAS PubMed.
- A. Dişli, M. Gümüş, K. Önal, N. Sarı and F. Arslan, New multifunctional agents and their inhibitory effects on the acetyl cholinesterase enzyme, J. Chem. Chem. Eng., 2018, 37(1), 21–34 Search PubMed.
- G. L. Ellman, K. D. Courtney, V. Andres Jr and R. M. Featherstone, A new and rapid colorimetric determination of acetylcholinesterase activity, Biochem. Pharmacol., 1961, 7(2), 88–95 CrossRef CAS PubMed.
- T. H. N. L. Bui, B. L. T, V. H. Nguyen, K. N. Tiep, T. H. Dang, H. H. Do and P.-T. Tran, Structures and Acetylcholinesterase Inhibition Abilities of some Derivatives Bearing (pyridin-2-yl)tetrazole Scaffold, VNU J. Sci.: Nat. Sci. Technol., 2024, 40(2), 1–9 Search PubMed.
- Q. M. Thai, T. H. Nguyen, G. B. Lenon, H. T. T. Phung, J.-T. Horng, P.-T. Tran and S. T. Ngo, Estimating AChE inhibitors from MCE database by machine learning and atomistic calculations, J. Mol. Graphics Modell., 2025, 134, 108906 CrossRef CAS PubMed.
- D. T. M. Dung, T. D. Quang, Q. M. Thai, P.-T. Tran, T. H. Nguyen and S. T. Ngo, Characterizing Potential BACE1 Inhibitors from ChEMBL Database using Knowlege- and Physics-Based Approaches, unpublished work, 2024.
- O. Trott and A. J. Olson, Improving the Speed and Accuracy of Docking with a
New Scoring Function, Efficient Optimization, and Multithreading, J. Comput. Chem., 2010, 31, 455–461 Search PubMed.
- T. N. H. Pham, T. H. Nguyen, N. M. Tam, T. Y. Vu, N. T. Pham, N. T. Huy, B. K. Mai, N. T. Tung, M. Q. Pham, V. V. Vu and S. T. Ngo, Improving Ligand-Ranking of AutoDock Vina by Changing the Empirical Parameters, J. Comput. Chem., 2021, 43(3), 160–169 CrossRef PubMed.
- J. Cheung, M. J. Rudolph, F. Burshteyn, M. S. Cassidy, E. N. Gary, J. Love, M. C. Franklin and J. J. Height, Structures of Human Acetylcholinesterase in Complex with Pharmacologically Important Ligands, J. Med. Chem., 2012, 55(22), 10282–10286 CrossRef CAS PubMed.
- Open Babel, http://www.openbabel.org.
- G. M. Morris, R. Huey, W. Lindstrom, M. F. Sanner, R. K. Belew, D. S. Goodsell and A. J. Olson, AutoDock4 and AutoDockTools4: Automated Docking with Selective Receptor Flexibility, J. Comput. Chem., 2009, 30(16), 2785–2791 CrossRef CAS PubMed.
- M. J. Abraham, T. Murtola, R. Schulz, S. Páll, J. C. Smith, B. Hess and E. Lindahl, GROMACS: High Performance Molecular Simulations through Multi-Level Parallelism from Laptops to Supercomputers, SoftwareX, 2015, 1–2, 19–25 CrossRef.
- H. Zhang, C. Yin, Y. Jiang and D. van der Spoel, Force Field Benchmark of Amino Acids: I. Hydration and Diffusion in Different Water Models, J. Chem. Inf. Model., 2018, 58(5), 1037–1052 Search PubMed.
- H. Zhang, Y. Jiang, Z. Cui and C. Yin, Force Field Benchmark of Amino Acids. 2. Partition Coefficients between Water and Organic Solvents, J. Chem. Inf. Model., 2018, 58(8), 1669–1681 CrossRef CAS PubMed.
- A. E. Aliev, M. Kulke, H. S. Khaneja, V. Chudasama, T. D. Sheppard and R. M. Lanigan, Motional Timescale Predictions by Molecular Dynamics Simulations: Case Study using Proline and Hydroxyproline Sidechain Dynamics, Proteins: Struct., Funct., Bioinf., 2014, 82(2), 195–215 Search PubMed.
- W. L. Jorgensen, J. Chandrasekhar, J. D. Madura, R. W. Impey and M. L. Klein, Comparison of Simple Potential Functions for Simulating Liquid Water, J. Chem. Phys., 1983, 79(2), 926–935 Search PubMed.
- J. Wang, R. M. Wolf, J. W. Caldwell, P. A. Kollman and D. A. Case, Development and Testing of a General Amber Force Field, J. Comput. Chem., 2004, 25(9), 1157–1174 CrossRef CAS PubMed.
- A. W. Sousa da Silva and W. F. Vranken, ACPYPE - AnteChamber PYthon Parser interfacE, BMC Res. Notes, 2012, 5(1), 1–8 Search PubMed.
- D. A. Case, I. Y. Ben-Shalom, S. R. Brozell, D. S. Cerutti, T. E. Cheatham, I. V. W. D. Cruzeiro, T. A. Darden, R. E. Duke, D. Ghoreishi, M. K. Gilson, H. Gohlke, A. W. Goetz, D. Greene, R. Harris, N. Homeyer, Y. Huang, S. Izadi, A. Kovalenko, T. Kurtzman, T. S. Lee, S. LeGrand, P. Li, C. Lin, J. Liu, T. Luchko, R. Luo, D. J. Mermelstein, K. M. Merz, Y. Miao, G. Monard, C. Nguyen, H. Nguyen, I. Omelyan, A. Onufriev, F. Pan, R. Qi, D. R. Roe, A. Roitberg, C. Sagui, S. Schott-Verdugo, J. Shen, C. L. Simmerling, J. Smith, R. SalomonFerrer, J. Swails, R. C. Walker, J. Wang, H. Wei, R. M. Wolf, X. Wu, L. Xiao, D. M. York and P. A. Kollman, AMBER 2018, University of California, San Francisco, 2018 Search PubMed.
- R. W. Zwanzig, High-Temperature Equation of State by a Perturbation Method. I. Nonpolar Gases, J. Chem. Phys., 1954, 22(8), 1420–1426 CrossRef CAS.
- D. Hamelberg and J. A. McCammon, Standard Free Energy of Releasing a Localized Water Molecule from the Binding Pockets of Proteins: Double-Decoupling Method, J. Am. Chem. Soc., 2004, 126(24), 7683–7689 CrossRef CAS PubMed.
- S. T. Ngo, Q. M. Thai, T. H. Nguyen, N. N. Tuan, T. N. H. Pham, H. T. T. Phung and D. T. Quang, Alchemical approach performance in calculating the ligand-binding free energy, RSC Adv., 2024, 14(21), 14875–14885 RSC.
- Chemicalize was Used for Prediction of Chemical Properties, https://chemicalize.com/welcome.
- A. Amadei, A. B. M. Linssen and H. J. C. Berendsen, Essential dynamics of proteins, Proteins Struct. Funct. Genet., 1993, 17(4), 412–425 CrossRef CAS PubMed.
- X. Daura, K. Gademann, B. Jaun, D. Seebach, W. F. van Gunsteren and A. Mark, Peptide folding: When simulation meets experiment, Angew. Chem., Int. Ed., 1999, 38, 236–240 Search PubMed.
- E. Papaleo, P. Mereghetti, P. Fantucci, R. Grandori and L. De Gioia, Free-Energy Landscape, Principal Component Analysis, and Structural Clustering to Identify Representative Conformations from Molecular Dynamics Simulations: The Myoglobin Case, J. Mol. Graph. Model., 2009, 27(8), 889–899 CrossRef CAS PubMed.
- Schrödinger LLC, P. Schrödinger Release 2020-4: Maestro; August, 2020 Search PubMed.
- A. Daina, O. Michielin and V. Zoete, SwissADME: a free web tool to evaluate pharmacokinetics, drug-likeness and medicinal chemistry friendliness of small molecules, Sci. Rep., 2017, 7(1), 42717 CrossRef PubMed.
- C. A. Lipinski, F. Lombardo, B. W. Dominy and P. J. Feeney, Experimental and computational approaches to estimate solubility and permeability in drug discovery and development settings, Adv. Drug Deliv. Rev., 2012, 64, 4–17 CrossRef.
- D. F. Veber, S. R. Johnson, H.-Y. Cheng, B. R. Smith, K. W. Ward and K. D. Kopple, Molecular properties that influence the oral bioavailability of drug candidates, J. Med. Chem., 2002, 45(12), 2615–2623 CrossRef CAS PubMed.
- S. K. Lee, I. H. Lee, H. J. Kim, G. S. Chang, J. E. Chung and K. T. No, The PreADME approach: Web-based program for rapid prediction of physico-chemical, drug absorption and drug-like properties, EuroQSAR 2002 Designing Drugs and Crop Protectants: Processes, Problems and Solutions, Blackwell Publishing, Maldenh, MA, 2003, pp, 418–420 Search PubMed.
- Y. Zhou, Y. Fu, W. Yin, J. Li, W. Wang, F. Bai, S. Xu, Q. Gong, T. Peng, Y. Hong, D. Zhang, D. Zhang, Q. Liu, Y. Xu, H. E. Xu, H. Zhang, H. Jiang and H. Liu, Kinetics-Driven Drug Design Strategy for Next-Generation Acetylcholinesterase Inhibitors to Clinical Candidate, J. Med. Chem., 2021, 64(4), 1844–1855 Search PubMed.
- T.-S. Tran, T.-D. Tran, T.-H. Tran, T.-T. Mai, N.-L. Nguyen, K.-M. Thai and M.-T. Le, Synthesis, In Silico and In Vitro Evaluation of Some Flavone Derivatives for Acetylcholinesterase and BACE-1 Inhibitory Activity, Molecules, 2020, 25(18), 4064 Search PubMed.
- L. Wang, T. Sun, T. Zhen, W. Li, H. Yang, S. Wang, F. Feng, Y. Chen and H. Sun, Butyrylcholinesterase-Activated Near-Infrared Fluorogenic Probe for In Vivo Theranostics of Alzheimer's Disease, J.
Med. Chem., 2024, 67(8), 6793–6809 CrossRef CAS PubMed.
- P. Kasa, H. Papp, P. Kasa and I. Torok, Donepezil dose-dependently inhibits acetylcholinesterase activity in various areas and in the presynaptic cholinergic and the postsynaptic cholinoceptive enzyme-positive structures in the human and rat brain, Neuroscience, 2000, 101(1), 89–100 CrossRef CAS PubMed.
- N. Sudhapriya, A. Manikandan, M. R. Kumar and P. T. Perumal, Cu-mediated synthesis of differentially substituted diazepines as AChE inhibitors; validation through molecular docking and Lipinski's filter to develop novel anti-neurodegenerative drugs, Bioorg. Med. Chem. Lett., 2019, 29(11), 1308–1312 CrossRef CAS PubMed.
- W. Sippl, J.-M. Contreras, I. Parrot, Y. M. Rival and C. G. Wermuth, Structure-based 3D QSAR and design of novel acetylcholinesterase inhibitors, J. Comput. Aided Mol. Des., 2001, 15(5), 395–410 CrossRef CAS PubMed.
- Z. Ul-Haq, W. Khan, S. Kalsoom and F. L. Ansari, In silico modeling of the specific inhibitory potential of thiophene-2,3-dihydro-1,5-benzothiazepine against BChE in the formation of β-amyloid plaques associated with Alzheimer's disease, Theor. Biol. Med. Model., 2010, 7(1), 22 CrossRef PubMed.
- Z. Sang, K. Wang, J. Dong and L. Tang, Alzheimer's disease: updated multi-targets therapeutics are in clinical and in progress, Eur. J. Med. Chem., 2022, 238, 114464 CrossRef CAS PubMed.
- J. Vamathevan, D. Clark, P. Czodrowski, I. Dunham, E. Ferran, G. Lee, B. Li, A. Madabhushi, P. Shah, M. Spitzer and S. Zhao, Applications of Machine Learning in Drug Discovery and Development, Nat. Rev. Drug Discov., 2019, 18(6), 463–477 CrossRef CAS PubMed.
- Q. M. Thai, T. H. Nguyen, G. B. Lenon, H. T. Thu Phung, J.-T. Horng, P.-T. Tran and S. T. Ngo, Estimating AChE inhibitors from MCE database by machine learning and atomistic calculations, J. Mol. Graph. Model., 2025, 134, 108906 CrossRef CAS PubMed.
- T. H. Nguyen, P.-T. Tran, N. Q. A. Pham, V.-H. Hoang, D. M. Hiep and S. T. Ngo, Identifying Possible AChE Inhibitors from Drug-like Molecules via Machine Learning and Experimental Studies, ACS Omega, 2022, 7(24), 20673–20682 CrossRef CAS PubMed.
- Q. M. Thai, T. H. Nguyen, H. T. T. Phung, M. Q. Pham, N. K. T. Pham, J.-T. Horng and S. T. Ngo, MedChemExpress compounds prevent neuraminidase N1 via physics- and knowledge-based methods, RSC Adv., 2024, 14(27), 18950–18956 RSC.
- R. A. Friesner, J. L. Banks, R. B. Murphy, T. A. Halgren, J. J. Klicic, D. T. Mainz, M. P. Repasky, E. H. Knoll, M. Shelley, J. K. Perry, D. E. Shaw, P. Francis and P. S. Shenkin, Glide: A New Approach for Rapid, Accurate Docking and Scoring. 1. Method and Assessment of Docking Accuracy, J. Med. Chem., 2004, 47(7), 1739–1749 CrossRef CAS PubMed.
- H. N. Trung; N. Q. A. Pham; Q. Mai Thai; V. V. Vu; S. Tung Ngo; A. J.-T. Horng, Discovering Neuraminidase Inhibitors via Computational and Experimental Studies unpublished work 2024.
- S. Decherchi and A. Cavalli, Thermodynamics and Kinetics of Drug-Target Binding by Molecular Simulation, Chem. Rev., 2020, 12788–12833 CrossRef CAS PubMed.
- S. Genheden and U. Ryde, The MM/PBSA and MM/GBSA Methods to Estimate Ligand-Binding Affinities, Expet Opin. Drug Discov., 2015, 10(5), 449–461 CrossRef CAS PubMed.
- D. Rodriguez Camargo, K. Korshavn, A. Jussupow, K. Raltchev, D. Goricanec, M. Fleisch, R. Sarkar, K. Xue, M. Aichler, G. Mettenleiter, A. Walch, C. Camilloni, F. Hagn, B. Reif and A. Ramamoorthy, Stabilization and Structural Analysis of a
Membrane-Associated hIAPP Aggregation Intermediate, Elife, 2017, 6, e31226 Search PubMed.
- H.-J. Woo and B. Roux, Calculation of Absolute Protein–Ligand Binding Free Energy from Computer Simulations, Proc. Natl. Acad. Sci. U. S. A., 2005, 102(19), 6825–6830 Search PubMed.
- G. S. A. Wright, S. V. Antonyuk, N. M. Kershaw, R. W. Strange and S. Samar Hasnain, Ligand Binding and Aggregation of Pathogenic SOD1, Nat. Commun., 2013, 4(1), 1758 CrossRef PubMed.
Footnote |
† Electronic supplementary information (ESI) available: Experimental chemistry, biology and molecular simulation docking. Additional data of 1H NMR, 13C NMR & MS spectra of the compounds. See DOI: https://doi.org/10.1039/d5ra00488h |
|
This journal is © The Royal Society of Chemistry 2025 |
Click here to see how this site uses Cookies. View our privacy policy here.