DOI:
10.1039/D4RA01801J
(Paper)
RSC Adv., 2024,
14, 22434-22448
Naturally based pyrazoline derivatives as aminopeptidase N, VEGFR2 and MMP9 inhibitors: design, synthesis and molecular modeling†
Received
8th March 2024
, Accepted 6th July 2024
First published on 15th July 2024
Abstract
Aminopeptidase N (APN) is regarded as an attractive target for cancer treatment due to its overexpression in various types of malignancies and its close association with cancer angiogenesis, metastasis and invasion. Herein the authors describe the design, synthesis and biological evaluation of some naturally based pyrazoline derivatives. Among these compounds, the diphenylpyrazole carbothioamide 8 showed significant activity and selectivity index (SI = 4.7) on breast (MCF-7) human cancer cell line and was capable of inhibiting APN with pIC50 value of 4.8, comparable to the reference standard. Further evaluation of derivative 8 against VEGFR2 and MMP9 as biomarkers for angiogenesis and invasion showed that the selected compound had an inhibitory activity on both proteins with pIC50 values of 6.7 and 6.4, respectively. Additionally, the migration ability of cells following treatment with the diphenylpyrazole derivative decreased to record a percentage wound closure of 57.77 for compound 8 versus 97.03 for the control. The promising derivative arrested cell growth at the G1 phase inducing early and late apoptosis. Finally, docking and ADMET in silico studies were performed.
1. Introduction
Aminopeptidases are recruited for appropriate prokaryotic and eukaryotic cells functioning, yet they might be associated with many diseases as cancer, malaria and diabetes. Aminopeptidase N (APN), is a zinc-dependent exopeptidase which is involved in metastasis of many types of tumors such as breast cancer.1,2
Metastasis is a complex process which implicates angiogenesis, invasion and cell migration and is accountable for most tumor related deaths. APN metabolizes the extracellular matrix to promote the metastasis of cancer cells. In addition, APN can induce angiogenesis and is detected on angiogenic blood vessels surface as compared to normal ones. Moreover, an intimate relationship between up-regulated APN and poor prognosis of cancer is described in the literature. Altogether, these findings may introduce APN as a potential target for management of cancer, Fig. 1.3–7
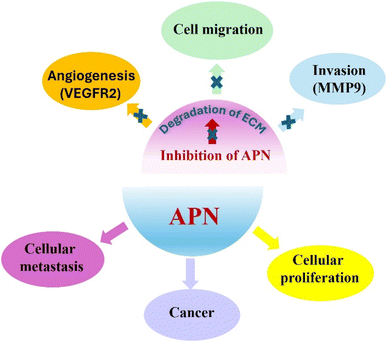 |
| Fig. 1 APN as a potential target for management of cancer. | |
Concerning its structure, APN is composed of 967 amino acids; it retains three hydrophobic pockets that bind the substrates and surrounds one catalytic zinc ion. The SAR of potent APN inhibitors (APNIs) showed the existence of zinc binding groups (ZBGs) and hydrophobic groups that interact with one or more of the three hydrophobic pockets of APN. A variety of naturally occurring compounds and synthetic small molecules are considered as APN inhibitors, an example of the natural APNIs are bestatin, amastatin and probestin. As for the synthetic APNIs, literature reported a number of structurally diverse compounds, among which some incorporated pyrazoline and thiadiazole rings in their structures, Fig. 2 and 3. Such heterocyclic scaffolds having the nitrogen and/or sulfur atoms are involved in coordination of the zinc ion, and their rigidness is usually favored for formation of the desired hydrophobic interactions.8,9
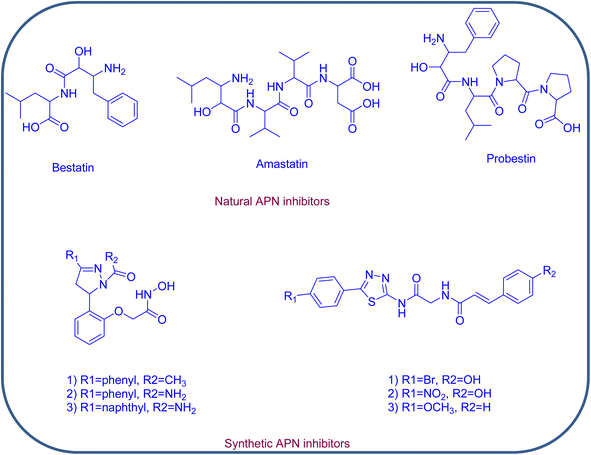 |
| Fig. 2 Natural and synthetic APN inhibitors. | |
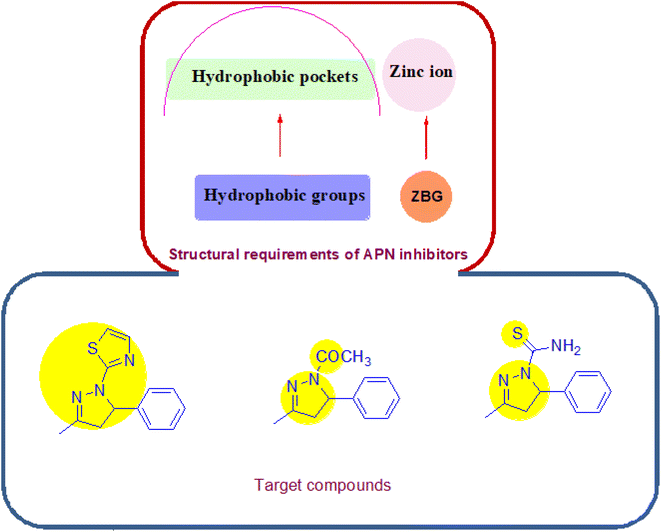 |
| Fig. 3 Design strategy of APN inhibitors. | |
Based on the aforementioned information and continuing our research on natural bioactive compounds,10–17 the authors developed a new series of pyrazoline based APN inhibitors, starting from the naturally occurring benzylidene acetone compound, to examine their inhibition effect on angiogenesis, invasion and migration by inhibiting vascular endothelial growth factor receptor-2 (VEGFR2), matrix metalloproteinase-9 (MMP9) and wound healing ability. The newly synthesized derivatives exhibited a combination of three electronegative atoms: (i) the aromatic nitrogens of the pyrazoline ring, (ii) the free sulfur atom (carbothioamide moiety) or that included in the heteroaromatic system of the thiazole ring and (iii) the carbonyl moiety in some of the synthesized compounds, Fig. 3. The electronegative atoms form essential system for the tight binding with the target protein. The promising derivative was subjected to cell cycle analysis, apoptosis assay, molecular modeling and ADMET study.
2. Results and discussion
2.1. Chemistry
The target compounds were prepared according to the procedures outlined in schemes 1 and 2. The pyrazoline derivatives 1–4 were synthesized by the cycloaddition reaction of the starting benzylidene acetone with hydrazine derivatives thus, heterocyclization of benzylidene acetone with hydrazine hydrate in glacial acetic acid afforded the corresponding N-acetylpyrazoline 1. While the corresponding N-phenylpyrazoline compounds 2–4 were obtained by condensation of benzylidene acetone with phenyl hydrazine compounds, namely, phenylhydrazine hydrochloride, 2-chlorophenyl hydrazine hydrochloride and/or 2,4,6- trichlorophenyl hydrazine hydrochloride in ethyl alcohol. The target pyrazoline carbothioamides 5–9 were furnished upon refluxing benzylidene acetone and different thiosemicarbazides, namely: thiosemicarbazide, 4-methylthiosemicarbazide, 4-ethylthiosemicarbazide, 4-phenylthiosemicarbazide and/or 4-benzylthiosemicarbazide, Scheme 1. The potential of carbothioamide 5 as an intriguing critical intermediate in the synthesis of new thiazoline and thiazolidinone derivatives was investigated. S-Alkylation was the first step in these reactions, followed by dehydration or the loss of an alcohol molecule. The relevant thiazoline derivatives 10–13 were obtained by treating carbothioamide 5 with the appropriate phenacyl bromide compounds; phenacyl bromide, 4-fluorophenacyl bromide, 4-methoxyphenacyl bromide and/or 2,4-dichlorophenacyl bromide in refluxing ethanol in the presence of anhydrous sodium acetate. Furthermore, the reaction of carbothioamide 5 with ethylbromoacetate and/or ethyl 2-bromopropionate in the presence of anhydrous sodium acetate resulted in the corresponding thiazolidinone 14 and 5-methylthiazolidinone 15 derivatives, respectively. The substituted benzylidene thiazolidinone derivatives 16–19 were obtained via one pot three component reaction of carbothioamide 5, chloroacetic acid and the appropriate aromatic aldehydes: namely, 4-fluorobenzaldehyde, 4-methoxybenzaldehyde, 2,4-dichlorobenzaldehyde and/or 3,4-dimethoxybenzaldehyde in acetic acid and catalytic amounts of acetic anhydride and anhydrous sodium acetate, Scheme 2. The structures of the newly synthesized compounds were confirmed by means of analytical and spectral methods (Experimental part).
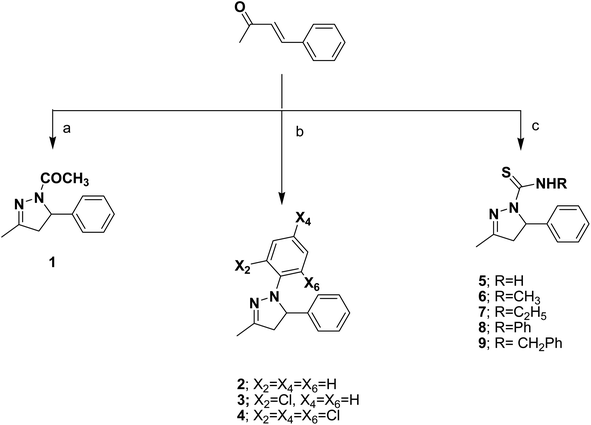 |
| Scheme 1 (a) NH2NH2·H2O, AcOH, reflux; (b) ArNHNH2·HCl, ethanol, reflux; (c) RNHCSNHNH2, ethanol, HCl, reflux. | |
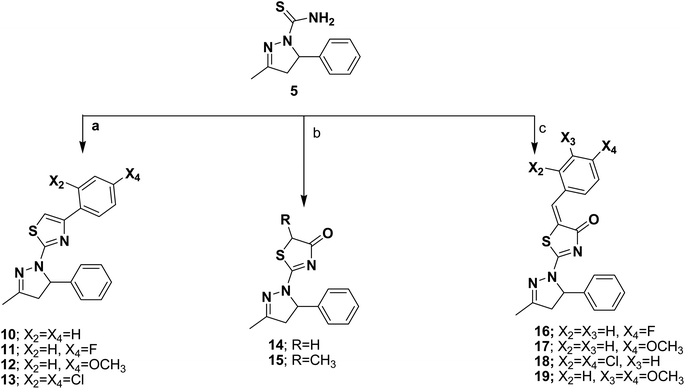 |
| Scheme 2 (a) ArCOCH2Br, ethanol, anhydrous CH3COONa, reflux; (b) BrCH2COOC2H5/CH3CHBrCOOC2H5, ethanol, anhydrous CH3COONa, reflux; (c) ClCH2COOH, ArCHO, AcOH, (CH3CO)2O, anhydrous CH3COONa, reflux. | |
2.2. Biology
2.2.1. In vitro antiproliferative activity. The synthesized compounds were examined for their in vitro activity against MCF-7 human cancer cell line and human healthy cell line (BJ-1) using the LDH assay. In case of MCF-7 cell line, all compounds showed remarkable cytotoxic activity relative to the reference drug (IC50 = 5.8–8.8 μM). The acetyl pyrazoline, compound 1 and pyrazoline carbothioamides compounds 5, 6, 7 and 8 showed the most potent effect among all the synthesized series (IC50 = 6.2, 5.8, 6.4, 5.8 and 6.2 μM, respectively). Within the thiazole-pyrazoline hybrids, it was observed that the halo substituted derivatives 11, 13, 16 and 18 were the most potent (IC50 = 6.9, 6.7, 6.6 and 6.9 μM, respectively). The most active compounds 1, 5, 6, 7 and 8 were assessed against non-tumor fibroblast-derived cell line (BJ-1). Compound 8 showed an IC50 value of 29.1 μM, exhibiting a selectivity index equals 4.7, Table 1.
Table 1 The antiproliferative IC50 of the nineteen compounds against cancer and normal cell lines according to the LDH assay
Compound code |
MCF-7 IC50 (μM) |
BJ-1 IC50 (μM) |
1 |
6.2 ± 0.2 |
18.6 ± 1.2 |
2 |
7.5 ± 0.3 |
— |
3 |
8.8 ± 0.5 |
— |
4 |
8.1 ± 0.5 |
— |
5 |
5.8 ± 0.2 |
18.2 ± 1.2 |
6 |
6.4 ± 0.3 |
18.2 ± 1.5 |
7 |
5.8 ± 0.2 |
18.7 ± 1.5 |
8 |
6.2 ± 0.2 |
29.1 ± 2.3 |
9 |
7.0 ± 0.3 |
— |
10 |
7.6 ± 0.3 |
— |
11 |
6.9 ± 0.2 |
— |
12 |
7.1 ± 0.2 |
— |
13 |
6.7 ± 0.2 |
— |
14 |
8.3 ± 0.3 |
— |
15 |
8.6 ± 0.3 |
— |
16 |
6.6 ± 0.2 |
— |
17 |
7.0 ± 0.3 |
— |
18 |
6.9 ± 0.2 |
— |
19 |
7.5 ± 0.2 |
— |
Doxorubicin |
4.9 ± 0.3 |
12.9 ± 1.3 |
2.2.2. APN inhibitory activity assay. With respect to malignant tumor growth, APN is a vital target for anticancer therapy because it is associated with different cancer hallmarks such as cell proliferation, angiogenesis, metastasis and invasion. The authors evaluated the APN inhibitory activity of the promising derivative 8 and the results demonstrated that the compound inhibited APN at pIC50 value of 4.8 compared to the reference GC42788 (pIC50 = 4.6).
2.2.3. Anti-angiogenesis assay. VEGFR-2 is regarded as the main target for antitumor vasculogenesis and angiogenesis. VEGF which is secreted by tumor cells binds to and activates its receptor VEGFR2, promoting vascular growth and supplying the hypoxic areas of tumor tissues with oxygen and nutrition. VEGF-activated VEGFR2 mediates proteins phosphorylation in the downstream signaling pathways, which subsequently promote tumor proliferation, survival, and angiogenesis.18 Therefore, in our study VEGFR2 was used as a biomarker for angiogenesis. When compared to sorafenib (pIC50 = 7.4) as the reference drug, derivative 8 inhibited VEGFR2 at pIC50 value of 6.7.
2.2.4. Anti-invasion assay. Tumor metastasis is closely related to the extracellular environment, MMP9 plays vital roles in cleaving extracellular matrix (ECM) and many plasma surface proteins and also degradation of basement membrane which are essential steps for supporting tumor invasion. Many studies have explored the value of MMP9 as a metastasis biomarker in breast cancer.19 In our study, the promising carbothioamide compound 8 was capable of inhibiting MMP9 with pIC50 value of 6.4 in comparison to pIC50 value of 6.7 for the reference quercetin.
2.2.5. Anti-migration assay. For many years, the wound healing assay has been one of the standard techniques to study cell migration and invasion by which tumor cells expand into nearby environments.20,21 The authors used the wound-healing assay on MCF-7 cells to detect the effect of compound 8 on cell migration inhibition in breast cancer cells. The results showed that, the percentage closure of the wound gap was 57.77% after treatment with the promising derivative 8 compared to 97.03% closure for the control cells. The migration ability of the cells was calculated as the ratio of the open area at 24 and 48 hours compared to the open area at zero hours. Data are expressed as % of wound closure.
2.2.6. Cell cycle inhibition. Using flow cytometry, cell cycle inhibition was used to assess tumor cells growth arrest after treatment of MCF7 cell line with compound 8, Fig. 4. The promising derivative showed significant change in G0/G1-phase compared to control cells, a marked increase in the cell population of G0/G1 stage from 65.02% to 72.11% and comparable alteration in the S phase from 24.79% to 23.02% were observed, demonstrating cell cycle arrest at G1 phase, and indicating the anti-proliferative effect of derivative 8 against breast cancer cells.
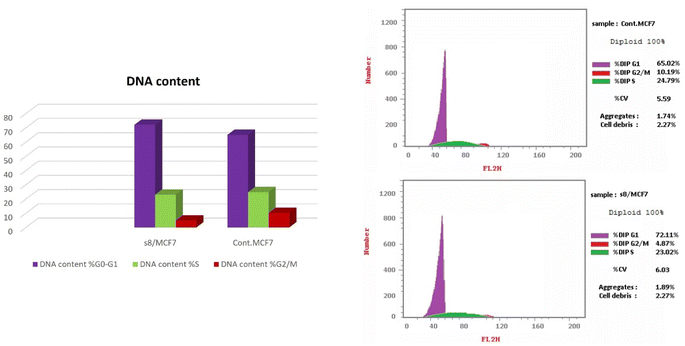 |
| Fig. 4 Cell cycle analysis of MCF-7 after incubation with compound 8 for 48 h. | |
2.2.7. Cell apoptosis. To specify the exact mechanism of apoptosis induced by compound 8, Annexin-V/FITC staining coupled with flow cytometry was used to evaluate treated MCF-7 breast cancer cells, as outlined in Fig. 5. Compound 8 caused early apoptosis of 11.52% and late apoptosis of 17.11%, compared to the control MCF-7 cells with 0.37% and 0.15% early and late apoptosis, respectively, with total MCF-7 cell death of 32.91% compared to 1.95% for the control cells.
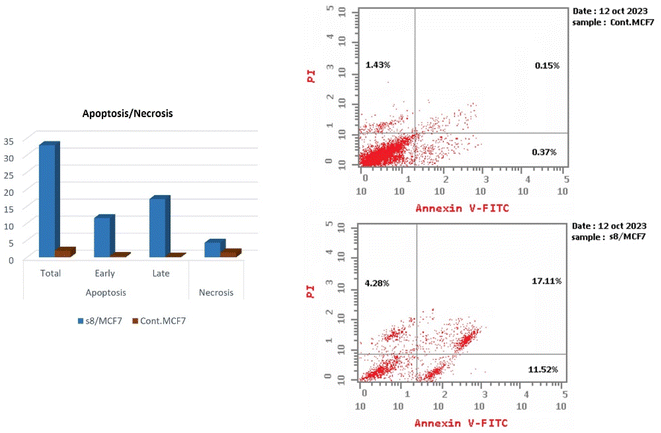 |
| Fig. 5 Induction of apoptosis by compound 8. Cells were exposed to compound 8 for 48 hours and analyzed by annexin V/PI staining. | |
2.3. Molecular modeling studies
2.3.1. Molecular docking. In order to examine the possible ligand-structure/enzyme-binding relationships, compound 8 was docked into the active site of aminopeptidase N. Molecular docking of the selected compound was accomplished using Autodock vina. Docking setup was first validated by self-docking of bestatin as the co-crystallized ligand in the vicinity of the binding site of the target enzyme, which showed a docking score (S) of −9.4 kcal mol−1 and root mean square deviation (RMSD) of 0.70 Å. Molecular docking study showed that compound 8 attained promising ΔG values of −8.1, kcal mol−1 against APN, as shown in Table 4. The 2D representations of the docked poses are illustrated in Fig. 8.
Table 4 Docking results of compound 8 on the target protein
Compound |
Protein |
S (kcal mol−1) |
Amino acid involved |
Interacting group/fragment |
Type of interaction |
8 |
Aminopeptidase-N |
−8.1 |
Ala262 |
NH |
H-bond |
Zn |
Sulfur |
Metal-acceptor |
Tyr381 |
Sulfur |
Cation-pi |
His301 |
Sulfur |
Cation-pi |
Met263 |
Phenyl |
Cation-pi |
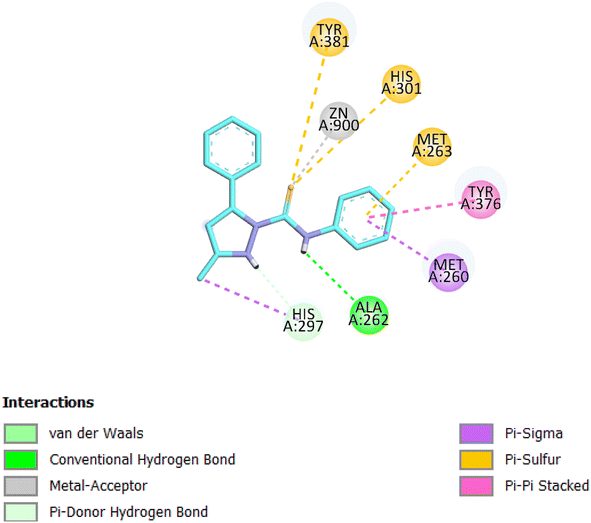 |
| Fig. 8 2D interactions of compound 8 within APN active site. | |
The selected derivative interacted with the target protein revealing the structural requirements for activity of potent APN inhibitors, the presence of the heterocyclic scaffold, having the sulfur atom involved in coordination of the zinc ion, is usually favored for formation of the desired interactions. The sulfur atom representing the zinc binding group interacted with the Zn ion, Tyr381 and His301 amino acids of the enzyme, while the NH group formed H-bond interaction with Ala262, also the phenyl ring interacted with Met263 amino acid.
2.3.2. Molecular dynamic study. Upon completion of compound 8 docking, evaluation of the stability of the complex structure was necessary on the APN receptor. A 100 ns MD simulation was conducted to analyze the longevity of the optimal pose for the 8-APN complex at room temperature. Throughout the simulation, the temperature, pressure, and potential energy of the intricate system displayed indications of convergence. The findings suggest that the ligand remained bound to the active site of the receptor pocket for the entire duration of the simulation, as depicted in Fig. 9 showcasing the gyration radius, SASA, average distance at the mass center, and computed RMSD. Additionally, the presence or absence of compound 8 during the simulation period showed higher stabilization for certain amino acids in the RMSF of the APN protein, as illustrated in Fig. 10.
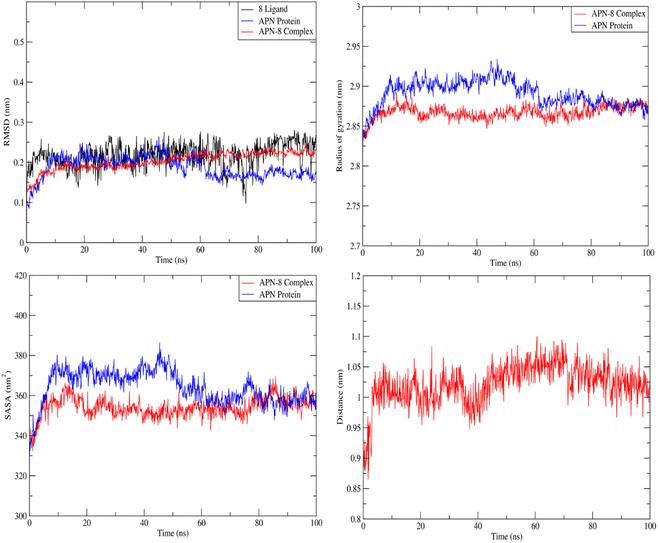 |
| Fig. 9 Plot of RMSD, gyration radius, SASA, protein–ligand distance. | |
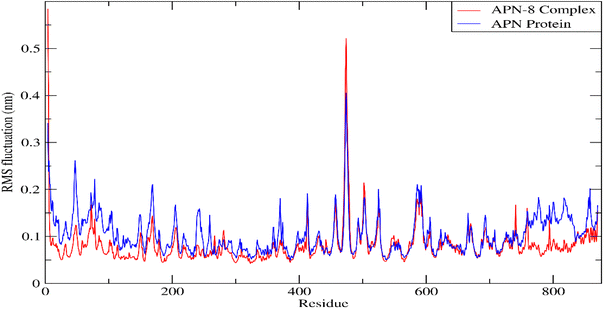 |
| Fig. 10 Plot of RMSF for APN protein and APN-8 complex. | |
Forces binding compound 8 with surrounding amino acids of APN active site revealed that Ala262 and Tyr381 are among the residues that mostly interacted with derivative 8 during the simulation study, as Fig. 11 illustrates, supporting the docking results. In addition, distance between the sulfur atom of compound 8 and zinc atom of APN protein was in range of 1.2–2.5 Å corresponding to sulfur metal bond. Finally, binding free energy values (MMPBSA), which was used to gauge the sustainability of the complexes produced, included all those forces, Table 5.
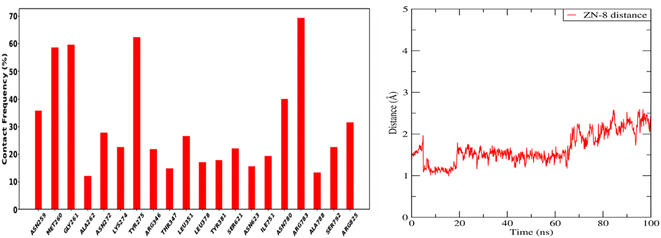 |
| Fig. 11 Frequently contacting amino acids with compound 8 and the distance with Zn atom. | |
Table 5 Free binding energies (in kJ mol−1) between 8 and APN protein
Binding energy |
Pim-1 |
Electrostatic energy |
−19.762 ± 3.341 |
Polar solvation energy |
47.094 ± 2.425 |
SASA energy |
−12.445 ± 1.806 |
van der Waal energy |
−110.247 ± 25.232 |
ΔG |
−95.519 ± 3.099 |
2.4. In silico ADMET prediction study
The authors used the SwissADME online application to reveal important information about different properties and parameters of the promising compound 8. The diphenylpyrazole carbothioamide derivative complied with Lipinski's rule predicting its suitability as a lead compound for oral administration, Table 6.
Table 6 Expected physicochemical features of compound 8
Compd |
Molecular weight |
TPSA (Å2)a |
#Rotatable bonds |
#H-bond acceptors |
#H-bond donors |
M log Pb |
Lipinski's violations |
Topological polar surface area. Calculated lipophilicity (M log P o/w). |
8 |
295.40 |
59.72 |
4 |
1 |
1 |
3.22 |
0 |
The bioavailability radar chart showed that the screened derivative 8 is situated in the pink colored zone which is the suitable area regarding its lipophilicity, size, polarity, solubility, and flexibility, Fig. 12, but not its saturation. This provides anticipation for its oral bioavailability.
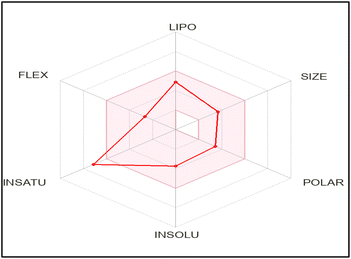 |
| Fig. 12 The promising derivative 8 bioavailability radar chart. The pink area indicates the optimum values for each oral bioavailability factor and the red lines represent the predicted value for the screened compound. | |
The boiled-egg chart showed that derivative 8 was positioned in the yellow area, to indicate high GIT absorption and a probability (P < 1) of BBB penetration, Fig. 13. On the other hand, the potential derivative 8 showed no PAINS alerts recording 0.55 bioavailability value and was categorized as non-carcinogenic substance.
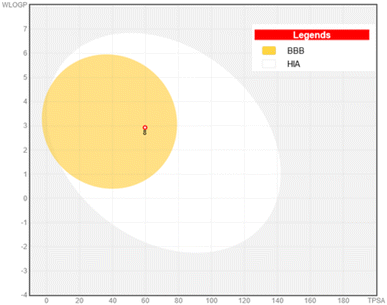 |
| Fig. 13 Boiled egg model for compound 8. | |
3. Conclusion
A new series of APN inhibitors with naturally based pyrazoline scaffold was synthesized. The new compounds showed promising cytotoxicity against MCF-7 cell line. In the assays against APN, VEGFR2 and MMP9 and the wound healing assay, compound 8 exhibited promising in vitro anti-APN, anti-angiogenesis, anti-metastasis, and anti-invasion activities. Moreover, the promising derivative was capable of arresting cell growth at G1 phase and inducing early and late apoptosis. The docking study showed that the selected compound attained promising results against the target protein.
4. Materials and methods
4.1. Chemistry
All melting points were measured using Electrothermal IA 9000 apparatus and they were uncorrected. NMR spectra were assessed by Bruker 400 MHz spectrometer using TMS as the internal standard. The reactions were followed by TLC (silica gel, aluminum sheets 60 F254, Merck) using Benzene-ethylacetate (7
:
3 v/v) as eluent. The purity of the synthesized derivatives was determined by elemental analysis and was found to be more than 95%. Compounds 1,23 2,24 525 and 1426 were previously prepared.
4.1.1. Synthesis of 1-((un)substituted phenyl)-4,5-dihydro-3-methyl-5-phenyl-1H-pyrazole 2–4. To a solution of benzylideneacetone (0.36 gm, 2.5 mmol) in ethyl alcohol (20 mL), appropriate phenyl hydrazine hydrochloride derivatives were added, namely: phenyl hydrazine hydrochloride, 2-chlorophenyl hydrazine hydrochloride, and/or 2,4,6-trichlorophenylhydrazine hydrochloride (2.5 mmol) and the mixture was refluxed for 6–8 h. The reaction mixture was cooled, the formed precipitate was filtered, dried, and crystallized from the ethanol to give the title compounds.
4.1.1.1. 1-(2-Chlorophenyl)-4,5-dihydro-3-methyl-5-phenyl-1H-pyrazole 3. Yellow crystals, mp = 77–8 °C, yield (87%). Anal. Calcd. for C16H15ClN2 (270.76): C, 70.98; H, 5.58; N, 10.35. Found: C, 71.08; H, 5.66; N, 10.43. 1HNMR (DMSO-d6, δ ppm): 2.07 (s, 3H, CH3), 2.81–2.87 (dd, 1H, pyrazoline, J = 5.60, 17.32 Hz), 3.39–3.47 (dd, 1H, pyrazoline, J = 10.92, 17.36 Hz), 5.52–5.56 (dd, 1H, pyrazoline, J = 5.72, 10.88 Hz), 6.83–7.30 (m, 9H, Ar–H). 13C NMR (DMSO-d6, δ ppm): 16.10, 46.77, 65.64, 122.70, 123.94, 124.42, 126.98, 127.55, 127.86, 128.78, 130.40, 141.53, 143.88, 152.25.
4.1.1.2. 1-(2,4,6-Trichlorophenyl)-4,5-dihydro-3-methyl-5-phenyl-1H-pyrazole 4. Buff crystals, mp = 161–2 °C, yield (89%). Anal. Calcd. for C16H13Cl3N2 (339.65): C, 56.58; H, 3.86; N, 8.25. Found: C, 56.68; H, 3.95; N, 8.33. 1HNMR (DMSO-d6, δ ppm): 2.01 (s, 3H, CH3), 2.94–3.01 (dd, 1H, pyrazoline, J = 7.32, 17.44 Hz), 3.33–3.40 (dd, 1H, pyrazoline, J = 11.28, 17.44 Hz), 5.22–5.27 (dd, 1H, pyrazoline, J = 7.88, 10.58 Hz), 7.23–7.30 (m, 5H, Ar–H), 7.52 (s, 2H, Ar–H). 13C NMR (DMSO-d6, δ ppm): 15.80, 46.23, 67.36, 128.22, 128.26, 128.62, 129.41, 131.60, 135.49, 139.26, 140.75, 150.46.
4.1.2. Synthesis of carbothioamide derivatives 5–9. A mixture of benzylideneacetone (0.36 gm, 2.5 mmol) and thiosemicarbazide derivatives, namely: thiosemicarbazide, 4-methylthiosemicarbazide, 4-ethylthiosemicarbazide, 4-phenylthiosemicarbazide and/or 4-benzylthiosemicarbazide (2.5 mmol) in ethanol (20 mL) in the presence of a catalytic amount of conc. HCl, was refluxed for 7–10 h. The reaction mixture was cooled, the formed precipitate was filtered, dried, and crystallized from the ethanol to give the target compounds.
4.1.2.1. 4,5-Dihydro-N,3-dimethyl-5-phenylpyrazole-1-carbothioamide 6. Yellow crystals, mp = 171–2 °C, yield (85%). Anal. Calcd. for C12H15N3S (233.33): C, 61.77; H, 6.48; N, 18.01; S, 13.74. Found: C, 61.84; H, 6.55; N, 18.09; S, 13.84. 1HNMR (DMSO-d6, δ ppm): 2.04 (s, 3H, CH3), 2.57–2.63 (dd, 1H, pyrazoline, J = 3.56, 18.32 Hz), 2.90 (s, 3H, NCH3), 3.49–3.57 (dd, 1H, pyrazoline, J = 11.44, 18.24 Hz), 5.73–5.77 (dd, 1H, pyrazoline, J = 3.64, 11.44 Hz), 7.23–7.69 (m, 5H, Ar–H), 8.13 (s, 1H, NH, D2O exchangeable). 13C NMR (DMSO-d6, δ ppm): 16.24, 31.69, 46.45, 63.11, 125.80, 127.18, 128.86, 143.87, 157.89, 176.49.
4.1.2.2. N-Ethyl-4,5-dihydro-3-methyl-5-phenylpyrazole-1-carbothioamide 7. Yellow crystals, mp = 170–1 °C, yield (67%). Anal. Calcd. for C13H17N3S (247.36): C, 63.12; H, 6.93; N, 16.99; S, 12.96. Found: C, 63.21; H, 6.99; N, 17.08; S, 13.04. 1HNMR (DMSO-d6, δ ppm): 1.08–1.12 (t, 3H, CH3, 7.2 Hz), 2.23 (s, 3H, CH3), 3.31–3.37 (dd, 1H, pyrazoline, J = 8.32, 18.24 Hz), 3.43 (br m, 2H, CH2), 3.49–3.56 (dd, 1H, pyrazoline, J = 9.44, 18.24 Hz), 5.08–5.13 (dd, 1H, pyrazoline, J = 8.96, 11.52 Hz), 7.42–7.49 (m, 5H, Ar–H), 8.78 (s, 1H, NH, D2O exchangeable). 13C NMR (DMSO-d6, δ ppm): 11.16, 16.41, 34.56, 44.91, 60.44, 127.01, 128.59, 129.31, 129.68, 146.59, 148.72, 179.06.
4.1.2.3. 4,5-Dihydro-3-methyl-N,5-diphenylpyrazole-1-carbothioamide 8. Yellow crystals, mp = 155–60 °C, yield (87%). Anal. Calcd. for C17H17N3S (295.4): C, 69.12; H, 5.80; N, 14.22; S, 10.85. Found: C, 69.20; H, 5.87; N, 14.29; S, 10.93. 1HNMR (DMSO-d6, δ ppm): 2.11 (s, 3H, CH3), 2.67–2.72 (dd, 1H, pyrazoline, J = 3.40, 18.44 Hz), 3.58–3.66 (dd, 1H, pyrazoline, J = 11.28, 18.32 Hz), 5.87–5.90 (dd, 1H, pyrazoline, J = 3.56, 11.32 Hz), 7.09–7.57 (m, 10H, Ar–H), 9.83 (s, 1H, NH, D2O exchangeable). 13C NMR (DMSO-d6, δ ppm): 16.36, 46.60, 63.21, 124.95, 125.20, 125.87, 127.36, 128.40, 128.98, 140.05, 143.32, 159.46, 173.84.
4.1.2.4. N-Benzyl-4,5-dihydro-3-methyl-5-phenylpyrazole-1-carbothioamide 9. Yellow crystals, mp = 135–6 °C, yield (83%). Anal. Calcd. for C18H19N3S (309.43): C, 69.87; H, 6.19; N, 13.58; S, 10.36. Found: C, 69.96; H, 6.27; N, 13.62; S, 10.44. 1HNMR (DMSO-d6, δ ppm): 2.05 (s, 3H, CH3), 2.61–2.67 (dd, 1H, pyrazoline), 3.51–3.58 (dd, 1H, pyrazoline), 4.71–4.73 (d, 2H, CH2), 5.76–5.80 (dd, 1H, pyrazoline), 7.09–7.32 (m, 10H, Ar–H), 8.97 (brs, 1H, NH, D2O exchangeable). 13C NMR (DMSO-d6, δ ppm): 16.28, 46.78, 47.35, 63.27, 125.75, 127.19, 127.74, 128.08, 128.44, 140.13, 143.72, 158.52, 175.94.
4.1.3. Synthesis of 1-(4-(un) substituted phenylthiazol-2-yl)-4,5-dihydro-3-methyl-5-phenyl-1H-pyrazole 10–13. A mixture of compound 5 (0.54gm, 2.5 mmol) in absolute ethanol (20 mL) and the appropriate phenacylbromides, namely: phenacyl bromide, 4-fluorophenacylbromide, 4-methoxyphenacyl bromide and/or 2,4-dichlorophenacyl bromide (2.5 mmol), in the presence of anhydrous sodium acetate (0.2 gm, 2.5 mmol), was refluxed for 8–10 h. Upon cooling, the obtained product was filtered off, washed with water and recrystallized from ethanol to obtain the desired compounds.
4.1.3.1. 4,5-Dihydro-3-methyl-5-phenyl-1-(4-phenylthiazol-2-yl)-1H-pyrazole 10. Yellow crystals, mp = 130–1 °C, yield (88%). Anal. Calcd. for C19H17N3S (319.42): C, 71.44; H, 5.36; N, 13.15; S, 10.04. Found: C, 71.51; H, 5.45; N, 13.22; S, 10.14. 1HNMR (DMSO-d6, δ ppm): 2.09 (s, 3H, CH3), 2.88–2.94 (dd, 1H, pyrazoline, J = 6.80, 18.00 Hz), 3.59–3.67 (dd, 1H, pyrazoline, J = 11.76, 18.20 Hz), 5.41–5.46 (dd, 1H, pyrazoline, J = 6.96, 11.68 Hz), 7.23–7.69 (m, 11H, Ar–H and CH-thiazole). 13C NMR (DMSO-d6, δ ppm): 15.84, 47.66, 64.31, 104.17, 125.91, 127.12, 127.79, 127.89, 128.80, 135.02, 142.67, 150.73, 155.82, 165.59.
4.1.3.2. 1-(4-(4-Fluorophenyl)thiazol-2-yl)-4,5-dihydro-3-methyl-5-phenyl-1H-pyrazole 11. Yellow crystals, mp = 139–40 °C, yield (91%). Anal. Calcd. for C19H16FN3S (337.41): C, 67.63; H, 4.78; N, 12.45; S, 9.50. Found: C, 67.71; H, 4.84; N, 12.52; S, 9.61. 1HNMR (DMSO-d6, δ ppm): 2.08 (s, 3H, CH3), 2.87–2.94 (dd, 1H, pyrazoline, J = 6.84, 18.16 Hz), 3.59–3.67 (dd, 1H, pyrazoline, J = 11.64, 18.08 Hz), 5.41–5.46 (dd, 1H, pyrazoline, J = 6.92, 11.72 Hz), 7.14–7.73 (m, 10H, Ar–H and CH-thiazole). 13C NMR (DMSO-d6, δ ppm): 15.82, 47.67, 64.26, 103.89, 115.65, 115.87, 127.08, 127.83, 128.92, 131.56, 142.56, 149.59, 156.02, 160.77, 165.65.
4.1.3.3. 4,5-Dihydro-1-(4-(4-methoxyphenyl)thiazol-2-yl)-3-methyl-5-phenyl-1H-pyrazole 12. Brownish yellow crystals, mp = 177–8 °C, yield (90%). Anal. Calcd. for C20H19N3OS (349.45): C, 68.74; H, 5.48; N, 12.02; S, 9.18. Found: C, 68.82; H, 5.55; N, 12.11; S, 9.28. 1HNMR (DMSO-d6, δ ppm): 2.07 (s, 3H, CH3), 2.87–2.93 (dd, 1H, pyrazoline, J = 6.92, 18.16 Hz), 3.58–3.65 (dd, 1H, pyrazoline, J = 11.68, 18.20 Hz), 3.75 (s, 3H, OCH3), 5.40–5.44 (dd, 1H, pyrazoline, J = 7.04, 11.68 Hz), 6.88–7.62 (m, 10H, Ar–H and CH-thiazole). 13C NMR (DMSO-d6, δ ppm): 15.81, 47.62, 55.53, 64.35, 102.00, 114.27, 127.12, 127.26, 127.77, 127.94, 128.88, 142.89,156.59, 155.65, 159.15, 165.56.
4.1.3.4. 1-(4-(2,4-Dichlorophenyl)thiazol-2-yl)-4,5-dihydro-3-methyl-5-phenyl-1H-pyrazole 13. Gray crystals, mp = 147–8 °C, yield (87%). Anal. Calcd. for C19H15Cl2N3S (388.31): C, 58.77; H, 3.89; N, 10.82; S, 8.26. Found: C, 58.85; H, 3.96; N, 10.92; S, 8.35. 1HNMR (DMSO-d6, δ ppm): 2.08 (s, 3H, CH3), 2.88–2.94 (dd, 1H, pyrazoline, J = 6.72, 18.16 Hz), 3.59–3.66 (dd, 1H, pyrazoline, J = 11.72, 18.24 Hz), 5.41–5.46 (dd, 1H, pyrazoline, J = 6.80, 11.68 Hz), 7.26–7.71 (m, 9H, Ar–H and CH-thiazole). 13C NMR (DMSO-d6, δ ppm): 15.83, 47.65, 64.22, 104.95, 127.09, 127.58, 127.83, 128.92, 132.29, 133.85, 142.53, 149.48, 156.04, 165.66.
4.1.4. Synthesis of 2-(4,5-dihydro-3-methyl-5-phenylpyrazol-1-yl)-5-un(substituted) thiazol-4(5H)-one 14 and 15. A mixture of compound 5 (0.54gm, 2.5 mmol) in absolute ethanol (20 mL) and the appropriate α haloketones, namely: ethylbromoacetate and/or ethyl 2-bromopropionate (2.5 mmol), in the presence of anhydrous sodium acetate (0.2 gm, 2.5 mmol), was refluxed for 8–10 h. The reaction mixture was cooled and poured onto ice/water. The formed precipitate was filtered, washed with water and dried to give the corresponding thiazolidinone derivatives.
4.1.4.1. 2-(4,5-Dihydro-3-methyl-5-phenylpyrazol-1-yl)-5-methylthiazol-4(5H)-one 15. Yellow crystals, mp = 122–3 °C, yield (88%). Anal. Calcd. for C14H15N3OS (273.35): C, 61.51; H, 5.53; N, 15.37; S, 11.73. Found: C, 61.60; H, 5.63; N, 15.44; S, 11.82. 1HNMR (DMSO-d6, δ ppm): 1.41–1.46 (dd, 3H, CH3-thiazolidinone, J = 7.40, 10.56 Hz), 2.13 (s, 3H, CH3), 2.87–2.91 (dd, 1H, pyrazoline), 3.68–3.75 (dd, 1H, pyrazoline, J = 11.16, 17.8 Hz), 4.11–4.12 (q, 1H, CH-thiazolidinone, J = 2.4 Hz), 5.60 (br, 1H, pyrazoline), 7.17–7.37 (m, 5H, Ar–H). 13C NMR (DMSO-d6, δ ppm): 16.10, 19.21, 47.77, 49.17, 63.44, 126.02, 126.10, 128.19, 129.31, 140.98, 164.58, 175.55, 190.40.
4.1.5. Synthesis of 5-(substituted benzylidene)-2-(4,5-dihydro-3-methyl-5-phenylpyrazol-1-yl)thiazol-4(5H)-one 16–19. To a solution of compound 5 (0.54 gm, 2.5 mmol) in glacial acetic acid (20 mL), chloroacetic acid (0.23 gm, 2.5 mmol) and the appropriate aromatic aldehydes, namely: 4-fluorobenzaldehyde, 4-methoxybenzaldehyde, 2,4-dichlorobenzaldehyde and/or 3,4-dimethoxybenzaldehyde (2.5 mmol) were added, in the presence of anhydrous sodium acetate (0.2 gm, 2.5 mmol) and a catalytic amount of acetic anhydride. The reaction mixture was refluxed for 7–10 h, after completion of the reaction, it was cooled and poured onto ice/water. The formed precipitate was filtered, washed with water and dried to afford the target compounds.
4.1.5.1. 5-(4-Fluorobenzylidene)-2-(4,5-dihydro-3-methyl-5-phenylpyrazol-1-yl)thiazol-4(5H)-one 16. Yellow crystals, mp = 200–1 °C, yield (92%). Anal. Calcd. for C20H16FN3OS (365.42): C, 65.74; H, 4.41; N, 11.50; S, 8.77. Found: C, 65.82; H, 4.50; N, 11.59; S, 8.88. 1HNMR (DMSO-d6, δ ppm): 2.19 (s, 3H, CH3), 2.97–3.03 (dd, 1H, pyrazoline, J = 4.04, 18.64 Hz), 3.76–3.84 (dd, 1H, pyrazoline, J = 11.00, 18.76 Hz), 5.72–5.75 (dd, 1H, pyrazoline, J = 4.08, 11.04 Hz), 7.23–7.73 (m, 10H, Ar–H and CH-benzylidene). 13C NMR (DMSO-d6, δ ppm): 16.17, 47.99, 63.61, 116.72, 116.94, 126.21, 128.36, 129.37, 129.81, 130.99, 131.02, 132.43, 132.53, 132.88, 132.97, 140.58, 164.24, 165.89, 170.00, 179.59.
4.1.5.2. 5-(4-Methoxybenzylidene)-2-(4,5-dihydro-3-methyl-5-phenylpyrazol-1-yl)thiazol-4(5H)-one 17. Yellow crystals, mp = 219–20 °C, yield (91%). Anal. Calcd. for C21H19N3O2S (377.46): C, 66.82; H, 5.07; N, 11.13; S, 8.49. Found: C, 66.89; H, 5.16; N, 11.22; S, 8.57. 1HNMR (DMSO-d6, δ ppm): 2.18 (s, 3H, CH3), 2.95–3.01 (dd, 1H, pyrazoline, J = 4.00, 18.60 Hz), 3.75–3.83 (dd, 1H, pyrazoline, J = 11.08, 18.60 Hz), 3.83 (s, 3H, OCH3), 5.70–5.74 (dd, 1H, pyrazoline, J = 4.08, 11.08 Hz), 7.09–7.81 (m, 10H, Ar–H and CH-benzylidene). 13C NMR (DMSO-d6, δ ppm): 16.14, 47.94, 55.88, 63.52, 115.27, 125.62, 126.17, 126.74, 128.34, 129.37, 130.98, 132.02, 140.67, 160.99, 165.45, 170.07, 179.95.
4.1.5.3. 5-(2,4-Dichlorobenzylidene)-2-(4,5-dihydro-3-methyl-5-phenylpyrazol-1-yl)thiazol-4(5H)-one 18. Yellow crystals, mp = 202–3 °C, yield (93%). Anal. Calcd. for C20H15Cl2N3OS (416.32): C, 57.70; H, 3.63; N, 10.09; S, 7.70. Found: C, 57.79; H, 3.69; N, 10.16; S, 7.81. 1HNMR (DMSO-d6, δ ppm): 2.19 (s, 3H, CH3), 2.99–3.05 (dd, 1H, pyrazoline, J = 4.00, 18.72 Hz), 3.77–3.85 (dd, 1H, pyrazoline, J = 10.96, 18.48 Hz), 5.73–5.77 (dd, 1H, pyrazoline, J = 4.00, 10.96 Hz), 7.22–7.82 (m, 9H, Ar–H and CH-benzylidene). 13C NMR (DMSO-d6, δ ppm): 16.18, 48.04, 63.74, 124.68, 126.22, 128.41, 128.66, 128.80, 129.39, 130.22, 130.35, 130.45, 131.47, 132.73, 135.24, 135.55, 140.39, 166.54, 169.75, 178.94.
4.1.5.4. 5-(3,4-Dimethoxybenzylidene)-2-(4,5-dihydro-3-methyl-5-phenylpyrazol-1-yl)thiazol-4(5H)-one 19. Yellow crystals, mp = 229–30 °C, yield (90%). Anal. Calcd. for C22H21N3O3S (407.49): C, 64.85; H, 5.19; N, 10.31; S, 7.87. Found: C, 64.94; H, 5.26; N, 10.39; S, 7.95. 1HNMR (DMSO-d6, δ ppm): 2.19 (s, 3H, CH3), 2.95–3.01 (dd, 1H, pyrazoline, J = 4.00, 18.64 Hz), 3.74–3.82 (dd, 1H, pyrazoline), 3.82 (s, 3H, OCH3), 3.83 (s, 3H, OCH3), 5.70–5.74 (dd, 1H, pyrazoline, J = 4.00, 11.04 Hz), 7.16–7.58 (m, 9H, Ar–H and CH-benzylidene). 13C NMR (DMSO-d6, δ ppm): 16.20, 47.94, 56.06, 56.13, 63.54, 112.58, 113.80, 123.31, 124.17, 125.82, 126.19, 127.04, 128.31, 129.36, 131.39, 132.61, 140.74, 149.40, 150.80, 165.41, 169.98, 179.80.
4.2. Biology
4.2.1 In vitro antiproliferative activity. Roswell Park Memorial Institute (RPMI) 1640 medium was purchased from Sigma Chem. Co. (St. Louis, MO, USA). Fetal bovine serum (FBS) and fetal calf serum (FCS) were purchased from Gibco, UK. Dimethyl sulfoxide (DMSO) and methanol were of HPLC grade and all other reagents and chemicals were of analytical reagent grade. MCF-7 (human breast adenocarcinoma), and the normal human skin fibroblast (BJ-1) cell lines were purchased from the American Type Culture Collection (Rockville, MD, USA) and maintained in RPMI-1640 medium which was supplemented with 10% heat-inactivated FBS, 100 U ml−1 penicillin and 100 U ml−1 streptomycin. The cells were grown at 37 °C in a humidified atmosphere of 5% CO2. All experiments were conducted thrice in triplicate (n = 3). All the values were represented as means ± SD.To determine the effect of each synthesized compound on membrane permeability in MCF-7 cancer cell line as well as BJ-1 normal cell line, a lactate dehydrogenase (LDH) release assay was used.27–30 The cells were seeded in 24-well culture plates at a density of 2 × 105 cells per well in 500 μL volume and allowed to grow for 18 h before treatment. After treatment with a series of different concentrations of each compound or Doxorubicin® (positive control), the plates were incubated for 48 h. Then, the supernatant (40 μL) was transferred to a new 96 well to determine LDH release and 6% Triton X-100 (40 μL) was added to the original plate for determination of total LDH. An aliquot of 0.1 M potassium phosphate buffer (100 μL, pH 7.5) containing 4.6 mM pyruvic acid was mixed to the supernatant using repeated pipetting. Then, 0.1 M potassium phosphate buffer (100 μL, pH 7.5) containing 0.4 mg mL−1 reduced β-NADH was added to the wells. The kinetic changes were read for 1 min using ELISA microplate reader in absorbance at wavelength 340 nm. This procedure was repeated with 40 μL of the total cell lysate to determine total LDH. The percentage of LDH release was determined by dividing the LDH released into the media by the total LDH following cell lysis in the same well.
4.2.2 In vitro APN inhibition assay. The assay for aminopeptidase N (APN) was determined using ab273292 Aminopeptidase N (APN/CD13) Activity Assay Kit, according to the instructions of the manufacturer.
4.2.3 In vitro VEGFR2 inhibition assay. The VEGFR2 assay was carried out using ab213476 – Human VEGF Receptor 2 SimpleStep ELISA Kit according to the manufacturer's instructions.
4.2.4 In vitro MMP9 inhibition assay. The matrix metalloproteinase 9 (MMP9) assay was done according to the manufacturer using ab100610 Human MMP9 ELISA Kit.
4.2.5 Wound healing assay. A scratch (wound) healing assay was performed following our suggested treatment. MCF7 was seeded in 6-well plates. When cell confluency reached 90%, the cells were scraped in a straight line with a sterile 100 μl pipette tip to create a wound in the middle of each well. Cells were washed twice with PBS to remove the remaining debris, and fresh serum-free media was added along with our synthetic compound. Images of the wound gap for each treated well were captured using an inverted microscope Axio Imager Z2 (Carl-Zeiss-Promenade, Jena, Germany) at a magnification of 10×, at time points 0, 24, and 48 h following the pipette tip scratching and treatment. This experiment was carried out in triplicate. The distance between the wound edges “scratch width” was measured using the Image-Pro Plus 5.1 software.31
4.2.6 Cell cycle analysis and apoptosis assay. Cell cycle analysis and apoptosis detection were carried out by flow cytometry.32 MCF-7 cells were seeded at 8 × 104 cells per mL. Cells were plated and treated with helichrysetin for 24, 48, and 72 hours. The cells were harvested, washed with PBS, resuspended in 1× Annexin V binding buffer, and stained with annexin V and PI for 15 min at room temperature in the dark.To clarify the cytotoxic effect of the newly synthesized compound, the cell cycle progression was examined against MCF-7 cells. Cell pellets were fixed with 70% ethanol on ice for 15 min and collected again. The collected pellets were incubated with propidium iodide (PI) staining solution (50 μg mL−1 PI, 0.1 mg mL−1 RNaseA, 0.05% Triton X-100) at room temperature for 1 h and analyzed by Gallios flow cytometer (Beckman Coulter, Brea, CA, USA) according to the manufacturer's instructions. Cell cycle analysis was determined using a FACS Calibur flow cytometer (BD Biosciences, USA).
Whereas, apoptosis detection was performed by FITC Annexin-V/PI commercial kit (Becton Dickenson, Franklin Lakes, NJ, USA) following the manufacturer protocol. The samples were analyzed by fluorescence-activated cell sorting (FACS) with a Gallios flow cytometer (Beckman Coulter, Brea, CA, USA) within 1 h after staining. Data were analyzed using Kaluza v1.2 (Beckman Coulter).
4.2.7 Statistical analysis. All experiments were conducted in triplicate (n = 3). All the values were represented as mean ± SD. Significant differences between the means of parameters as well as IC50s were determined by probit analysis using SPSS software program (SPSS Inc., Chicago, IL).
4.3. 2D QSAR study
The 19 target pyrazoline derivatives were split into training and test sets of 16 and 3 compounds, respectively, while maintaining the IC50 value distribution of the original dataset in both sets. Molecular mechanical descriptors available in MOE QSAR module (313 descriptors) were initially computed for the training set compounds. The resulted “descriptors-target property” matrix was passed to RapidMiner Studio 9.3.001 for QSAR model construction.33 Redundant descriptors “low variance descriptors” that do not have an added value to the model were first filtered out leaving 299 descriptors for model construction. Variable selection was then performed utilizing forward selection (FS) provided in RapidMiner.34 MLR was used as the machine learning algorithm for the construction of a linear model which is known for its interpretability, transparency and simplicity.
RMSECV was employed for the assessment of the model quality. FS protocol depends on the inclusion of an initial descriptor in the regression which gives the best one-descriptor model quality (minimum RMSECV). Further descriptors are progressively included to the regression and only the descriptor yields the maximum increase in model quality (minimum RMSECV) is included to the chosen descriptor set at a time. The training process is ended when further descriptor inclusion does not enhance the model quality (does not decrease RMSECV anymore). FS generated a three-descriptor model (eqn (1)) with a significantly high quality as shown in its statistical evaluation parameters and its predictions.
|
IC50 = −0.0012 × E − 0.0295 × log p(o/w) + 0.0583 × Mr + 5.0596
| (1) |
4.4. Molecular modeling studies
4.4.1 Molecular docking. Autodock Vina software was used to perform the docking protocol,35,36 with a grid box of 253 Å3 centered on co-crystalized ligand in the active site of Aminopeptidase N which was retrieved from the protein data bank (PDB ID: 2DQM37) using Exhaustiveness of 16. The compound was created using Marvin sketch. Autodock tools were utilized to create the required pdbqt files since Vina Autodock requires the receptor and ligands to be in pdbqt format. The selected compound was docked into the predetermined active site for the targeted proteins. The screening of the molecules was completed using PyRx 0.8.38 Validation was achieved by redocking of co-crystalized ligand and calculating RMSD using DockRMSD server.39 Finally, the free Biovia Discovery Studio 2021 visualizer was used to demonstrate the docking poses.
4.4.2 Molecular dynamic simulation. The input files for MD calculations were generated using CHARMM-GUI solution builder using CHARMM force field parameters for APN.40 The topologies of the selected ligands were generated using CHARMM General Forcefield through CgenFF server.41 The CHARMM-GUI solution builder includes five steps. In the first step, the coordinates of protein–ligand complex are read by the tool. The second step involves solvation of the protein–ligand complex as well as determining the shape and size of the system. Na+ and Cl− ions are added in this step to neutralize the system. Periodic Boundary Conditions (PBC) are set in the third step which are used for approximation of a large system by using a unit cell which is then replicated in all directions. The simulation takes place only for the atoms that are present inside the PBC box. Complexes have been neutralized by adding 32 Na+ ions and have 113 Å, 113 Å and 113 Å dimensions for the PBC box in x, y and z, respectively. Bad contacts are removed in this step by running short minimization. The fourth and fifth step involve equilibration of the system and production. Equilibration is done in two phases: NVT ensemble and NPT ensemble to ensure that the system has achieved the desired temperature and pressure. The input files for equilibration and production are then downloaded and the desired changes were made which include number of steps of MD run, frequency of saving of trajectories and calculation of energy, etc. Non-bonded interactions were treated with a 12 Å cutoff distance and the neighbor searching list was buffered with the Verlet cutoff-scheme and the long-range electrostatic interactions were treated with the particle mesh Ewald (PME) method. Prior to production simulation, energy minimization of the system was carried out by using steepest descent algorithm (5000 steps). The complex was then equilibrated for stabilizing its temperature and pressure by subjecting it to NVT and NPT ensemble and simulating for 125 ps at 300.15 K temperature using 400 kJ mol−1 nm−2 and 40 kJ mol−1 nm−2 positional restraints on the backbone and side chains, respectively. Finally, the complex is subjected to production simulation run for 100 ns in NPT ensemble at 300.15 K and 1 bar. To maintain the temperature Nose–Hoover thermostat was used and similarly for maintaining the pressure Parrinello-Rahman barostat was used. The LINCS algorithm was used for constraining H-bonds using the inputs provided by CHARMM-GUI. The V-rescale thermostat at 300 K with a coupling constant of 1 ps was used. The trajectories were stored every 2 ps. Simulations of 100 ns in NPT assembly were performed for the production stage. GROMACS 2020.2 software was used to carry out 100 ns MD simulation for the complexes.42 GROMACS utilities were used for the analysis of the MD simulations trajectories. The root mean square deviation (RMSD) of atom position for selected ligands and protein were calculated by fitting protein backbone atom with the gmx_rms subprogram. Similarly, root mean square fluctuations (RMSF) based on the protein C-alpha atoms were calculated using gmx_rmsf. Radius of gyration of all protein atoms was calculated with the gmx_gyrate and number of hydrogen bonds was calculated (in-side the protein–ligand interface) with the gmx_hbond. The utility gmx_distance was used to calculate the center of mass distance between the protein and the ligand during the simulation. The VMD molecular graphics program was used for trajectory visualization,43 detailed hydrogen bonding information and contact frequency analysis. MM/PBSA (Molecular Mechanics/Poisson−Boltzmann Surface Area) calculations were done using g_mmpbsa, a GROMACS tool used to calculate an estimated binding affinity.44 In general terms, the binding free energy of the protein with ligand in solvent can be expressed as:
ΔGBinding = ΔGComplex − (ΔGProtein − ΔGLignad) |
where, ΔGComplexis the total free energy of the protein–ligand complex, and ΔGProtein and ΔGLignad are total free energies of the isolated protein and ligand in solvent, respectively. g_mmpbsa can also be used to estimate the energy contribution per residue to the binding energy. To decompose the binding energy, at first ΔEMM, ΔGpolar and ΔGnon−polar were separately calculated for each residue and were then summed up to obtain the contribution of each residue to the binding energy. Considering that g_mmpbsa only read the files of some specific GROMACS versions, the binary run input file (.tpr) required for MM-PBSA calculation through the g_mmpbsa was regenerated by GROMACS 5.1.4. The molecular structure file (.gro), topology file (.top) and MD-parameter file (.mdp) were necessary to generate the binary run input file, and they all came from the MD process.
4.5. In silico ADMET prediction study
Using the freely accessible web application SwissADME, the promising derivative 8 was investigated for its expected physicochemical and pharmacokinetic properties. Furthermore, the admetSAR 1.0 internet server (http://lmmd.ecust.edu.cn/admetsar1) was utilized to compute their toxicity.
Data availability
The data supporting this article have been included as part of the ESI.†
Conflicts of interest
The authors declared that there are no actual or potential conflicts of interest and have approved the article.
Acknowledgements
This research article was funded by NRC, Cairo, Egypt (2022–2024), ethical approval number: (13010137-2) through project no. 13010137 entitled “Synthesis and computational studies of new naturally derived anticancer heterocyclic compounds”.
References
- A. Mucha, M. Drag, J. P. Dalton and P. Kafarski, Biochimie, 2010, 92, 1509–1529 CrossRef CAS.
- R. Pasqualini, E. Koivunen, R. Kain, J. Lahdenranta, M. Sakamoto, A. Stryhn, R. A. Ashmun, L. H. Shapiro, W. Arap and E. Ruoslahti, Cancer Res., 2000, 60, 722–727 CAS.
- D. Wirtz, K. Konstantopoulos and P. C. Searson, Nat. Rev. Cancer, 2011, 11, 512–522 CrossRef CAS.
- I. Saiki, H. Fujii, J. Yoneda, F. Abe, M. Nakajima, T. Tsuruo and I. Azuma, Int. J. Cancer, 1993, 54, 137–143 CrossRef CAS PubMed.
- J. Yoneda, I. Saiki, H. Fujii, F. Abe, Y. Kojima and I. Azuma, Clin. Exp. Metastasis, 1992, 10, 49–59 CrossRef CAS PubMed.
- S. V Bhagwat, J. Lahdenranta, R. Giordano, W. Arap, R. Pasqualini and L. H. Shapiro, Blood, 2001, 97, 652–659 CrossRef.
- M. Wickström, R. Larsson, P. Nygren and J. Gullbo, Cancer Sci., 2011, 102, 501–508 CrossRef PubMed.
- J. Cao, C. Zhao, H. Dong, Q. Xu and Y. Zhang, RSC Adv., 2021, 11, 21426–21432 RSC.
- J. Cao, J. Zang, X. Kong, C. Zhao, T. Chen, Y. Ran, H. Dong, W. Xu and Y. Zhang, Bioorg. Med. Chem., 2019, 27, 978–990 CrossRef CAS.
- R. Z. Batran, S. M. El-Daly, W. A. El-Kashak and E. Y. Ahmed, Chem. Biol. Drug Des., 2022, 99, 470–482 CrossRef CAS PubMed.
- R. Z. Batran, W. A. El-Kashak, S. M. El-Daly and E. Y. Ahmed, ChemistrySelect, 2021, 6, 11012–11021 CrossRef CAS.
- E. Y. Ahmed, N. A. Abdel Latif, M. F. El-Mansy, W. S. Elserwy and O. M. Abdelhafez, Bioorg. Med. Chem., 2020, 28, 115328 CrossRef CAS PubMed.
- E. Y. Ahmed, O. M. Abdelhafez, D. Zaafar, A. M. Serry, Y. H. Ahmed, R. F. A. El-Telbany, Z. Y. Abd Elmageed and H. I. Ali, Arch. Pharm., 2022, 355, 2100327 CrossRef CAS.
- E. Y. Ahmed, N. A. Abdel Latif, T. Nasr, H. M. Awad and O. M. Abdelhafez, Chem. Biol. Drug Des., 2022, 99, 609–619 CrossRef CAS PubMed.
- R. Z. Batran, D. H. Dawood, S. A. El-Seginy, M. M. Ali, T. J. Maher, K. S. Gugnani and A. N. Rondon-Ortiz, Arch. Pharm., 2017, 350, e1700064 CrossRef PubMed.
- R. Z. Batran, E. Y. Ahmed, H. M. Awad, K. A. Ali and N. A. Abdel Latif, RSC Adv., 2023, 13, 29070–29085 RSC.
- R. Z. Batran, E. Y. Ahmed, E. S. Nossier, H. M. Awad and N. A. Abdel Latif, J. Mol. Struct., 2024, 1305, 137790 CrossRef CAS.
- X. Wang, A. M. Bove, G. Simone and B. Ma, Front. Cell Dev. Biol., 2020, 8, 599281 CrossRef.
- H. Huang, Sensors, 2018, 18, 3249 CrossRef.
- D. Hanahan and R. A. Weinberg, Cell, 2011, 144, 646–674 CrossRef CAS.
- J.-B. Guy, S. Espenel, A. Vallard, P. Battiston-Montagne, A.-S. Wozny, D. Ardail, G. Alphonse, C. Rancoule, C. Rodriguez-Lafrasse and N. Magne, J. Vis. Exp., 2017, 129, e56337 Search PubMed.
- M. Shahlaei, Chem. Rev., 2013, 113, 8093–8103 CrossRef CAS.
- M. Linden, S. Hofmann, A. Herman, N. Ehler, R. M. Bär and S. R. Waldvogel, Angew. Chem. Int. Ed., 2023, 62, e202214820 CrossRef CAS.
- J. Gaba, S. Sharma, G. Arora and P. Sharma, Asian J. Chem., 2016, 28, 2031–2037 CrossRef CAS.
- N. A. Danilkina, L. E. Mikhaylov and B. A. Ivin, Chem. Heterocycl. Compd., 2011, 47, 886–900 CrossRef CAS.
- D. Havrylyuk, B. Zimenkovsky, O. Karpenko, P. Grellier and R. Lesyk, Eur. J. Med. Chem., 2014, 85, 245–254 CrossRef CAS PubMed.
- H. A. Soliman, A. Y. Mubarak, A. El-Mekabaty, H. M. Awad and S. S. Elmorsy, Monatsh. Chem., 2016, 147, 809–816 CrossRef CAS.
- A. F. Kassem, I. F. Nassar, M. T. Abdel-Aal, H. M. Awad and W. A. El-Sayed, Chem. Pharm. Bull., 2019, 67, 888–895 CrossRef CAS PubMed.
- E. M. Flefel, W. I. El-Sofany, H. M. Awad and M. El-Shahat, Mini-Rev. Med. Chem., 2020, 20, 152–160 CrossRef CAS.
- A. A. H. A. Rahman, I. F. Nassar, A. K. F. Shaban, D. S. EL-Kady, H. M. Awad and W. A. El Sayed, Mini-Rev. Med. Chem., 2019, 19, 1093–1110 CrossRef.
- S. M. El-Daly, M. T. Abo-elfadl, J. Hussein and M. A. M. Abo-Zeid, Life Sci., 2023, 315, 121320 CrossRef CAS.
- S. Diab, T. Teo, M. Kumarasiri, P. Li, M. Yu, F. Lam, S. K. C. Basnet, M. J. Sykes, H. Albrecht, R. Milne and S. Wang, ChemMedChem, 2014, 9, 962–972 CrossRef CAS.
- I. Mierswa, M. Wurst, R. Klinkenberg, M. Scholz and T. Euler, in Proceedings of the 12th ACM SIGKDD International Conference on Knowledge Discovery and Data Mining, ACM, New York, NY, USA, 2006, pp. 935–940 Search PubMed.
- A. Agrwal, A. Verma, N. Chantola, S. Verma and V. Kasana, J. Environ. Sci. Health B, 2022, 57, 379–420 CrossRef CAS.
- J. Eberhardt, D. Santos-Martins, A. F. Tillack and S. Forli, J. Chem. Inf. Model., 2021, 61, 3891–3898 CrossRef CAS PubMed.
- O. Trott and A. J. Olson, J. Comput. Chem., 2010, 31, 455–461 CrossRef CAS PubMed.
- K. Ito, Y. Nakajima, Y. Onohara, M. Takeo, K. Nakashima, F. Matsubara, T. Ito and T. Yoshimoto, J. Biol. Chem., 2006, 281, 33664–33676 CrossRef CAS PubMed.
- S. Dallakyan and A. J. Olson, Methods Mol. Biol., 2015, 1263, 243–250 CrossRef CAS PubMed.
- E. W. Bell and Y. Zhang, J. Cheminf., 2019, 11, 40 Search PubMed.
- B. R. Brooks, C. L. Brooks, A. D. Mackerell, L. Nilsson, R. J. Petrella, B. Roux, Y. Won, G. Archontis, C. Bartels, S. Boresch, A. Caflisch, L. Caves, Q. Cui, A. R. Dinner, M. Feig, S. Fischer, J. Gao, M. Hodoscek, W. Im, K. Kuczera, T. Lazaridis, J. Ma, V. Ovchinnikov, E. Paci, R. W. Pastor, C. B. Post, J. Z. Pu, M. Schaefer, B. Tidor, R. M. Venable, H. L. Woodcock, X. Wu, W. Yang, D. M. York and M. Karplus, J. Comput. Chem., 2009, 30, 1545–1614 CrossRef CAS.
- K. Vanommeslaeghe, E. Hatcher, C. Acharya, S. Kundu, S. Zhong, J. Shim, E. Darian, O. Guvench, P. Lopes, I. Vorobyov and A. D. Mackerell, J. Comput. Chem., 2010, 31, 671–690 CrossRef CAS.
- M. J. Abraham, T. Murtola, R. Schulz, S. Páll, J. C. Smith, B. Hess and E. Lindahl, SoftwareX, 2015, 1–2, 19–25 CrossRef.
- W. Humphrey, A. Dalke and K. Schulten, J. Mol. Graph., 1996, 14(33–8), 27–28 Search PubMed.
- R. Kumari, R. Kumar and A. Lynn, J. Chem. Inf. Model., 2014, 54, 1951–1962 CrossRef CAS PubMed.
|
This journal is © The Royal Society of Chemistry 2024 |
Click here to see how this site uses Cookies. View our privacy policy here.