DOI:
10.1039/D4RA01735H
(Paper)
RSC Adv., 2024,
14, 20290-20299
Differentiation of different dibenzothiophene (DBT) desulfurizing bacteria via surface-enhanced Raman spectroscopy (SERS)†
Received
6th March 2024
, Accepted 1st May 2024
First published on 26th June 2024
Abstract
Fossil fuels are considered vital natural energy resources on the Earth, and sulfur is a natural component present in them. The combustion of fossil fuels releases a large amount of sulfur in the form of SOx in the atmosphere. SOx is the major cause of environmental problems, mainly air pollution. The demand for fuels with ultra-low sulfur is growing rapidly. In this aspect, microorganisms are proven extremely effective in removing sulfur through a process known as biodesulfurization. A major part of sulfur in fossil fuels (coal and oil) is present in thiophenic structures such as dibenzothiophene (DBT) and substituted DBTs. In this study, the identification and characterization of DBT desulfurizing bacteria (Chryseobacterium sp. IS, Gordonia sp. 4N, Mycolicibacterium sp. J2, and Rhodococcus sp. J16) based on their specific biochemical constituents were conducted using surface-enhanced Raman spectroscopy (SERS). By differentiating DBT desulfurizing bacteria, researchers can gain insights into their unique characteristics, thus leading to improved biodesulfurization strategies. SERS was used to differentiate all these species based on their biochemical differences and different SERS vibrational bands, thus emerging as a potential technique. Moreover, multivariate data analysis techniques such as principal component analysis (PCA) and partial least squares discriminant analysis (PLS-DA) were employed to differentiate these DBT desulfurizing bacteria on the basis of their characteristic SERS spectral signals. For all these isolates, the accuracy, sensitivity, and specificity are above 90%, and an AUC (area under the curve) value of close to 1 was achieved for all PLS-DA models.
1. Introduction
Fossil fuels are essential natural energy resources on Earth. Owing to the combustion of these fossil fuels, SO2 is released in large amounts into the atmosphere, and it is the major cause of environmental problems on Earth as it leads to acid rain and air pollution.1 Therefore, the demand for fuels with ultra-low sulfur content is growing.2 Sulfur may be present in organic and inorganic forms,3 and most of the sulfur (70%) in fossil fuels (coal and oil) is present in thiophenic structures such as DBT and substituted DBTs.4,5 Physical and chemical methods such as hydrodesulfurization (HDS) can remove a significant quantity of inorganic sulfur and a small amount of sulfur that is bonded to organic materials from fuels.2 Through HDS, cyclic sulfides known as thiolanes and aliphatic acyclic sulfides known as thioethers can be easily removed. However, this method fails to remove sulfur from heterocyclic organic compounds such as thiophene, benzothiophene (BT), and dibenzothiophene (DBT).6,7 Another drawback of HDS is that it is a catalytic process and requires high operating pressure and temperature conditions for the conversion of organic sulfur to H2S gas.4 Therefore, there is a need to use another extremely efficient alternative biocatalytic desulfurization method known as biodesulfurization (BDS) for the removal of recalcitrant sulfur. This technique is noninvasive and can be operated at low temperatures and pressures.8 Most importantly, it is very specific in removing sulfur from refractory sulfur-based organic compounds. BDS is the process that uses different types of microorganisms for metabolizing sulfur compounds. Therefore, by selecting the appropriate organic sulfur metabolizing strain, biodesulfurization of fossil fuels can easily be performed.9 Microorganisms that degrade DBT can easily remove sulfur from coal.10 Some bacterial strains, like Gordonia sp., Rhodococcus sp., R. erythropolis H-2, Corynebacterium sp., Mycobacterium sp. G3 etc., have been reported for the process of biodesulfurization.1,11 In this process, the breakdown of the C–S bond that is present in the thiophenic compounds like DBT occurs by following the 4S pathway. DBT is the recalcitrant organo-sulfur compound that is abundantly present in fossil fuels. Microorganisms fulfill their need for nutrients from DBT by consuming sulfur, while the remaining structure is not disturbed. In this way, a decrease in the sulfur emission from the fossil fuels occurs.12,13 So, it is a matter of interest to identify and characterize the desulfurizing bacteria. Different spectroscopic techniques, like MS14 FTIR,15 and NMR,16 have been used for the characterization and identification of bacteria. Notably, these methods have some drawbacks, like time-consuming sample preparation, unsuitability for an aqueous environment and laboriousness. These drawbacks can be overcome by the use of another spectroscopic technique called Raman spectroscopy. This technique has no such disadvantages, and it can be used for both quantitative and qualitative analysis of the sample on the basis of their spectra. It is a promising technique and when combined with a multivariate data analysis technique (like PCA and PLS-DA), it becomes valuable for different analyses. This method is non-destructive and involves minimum or no sample preparation.17 Raman peaks can be used to determine the chemical components of bacteria, including their DNA and RNA, lipids, proteins, pigments, and carbohydrates. Although the spectrum profiles of bacteria are fairly similar, distinct species of bacteria exhibit variances in peak intensities, which indicates their diverse biochemistry.18 Raman spectroscopy can fingerprint the entire organism, making it a quick method for identifying and characterizing bacteria. However, the weak Raman signal presents a barrier.19
SERS has been used to overcome this issue because it enhances the Raman signals by chemical and electromagnetic mechanism. The electromagnetic mechanism is the most studied so far. This mechanism occurs by the magnification of light after the excitation of localized surface plasmon resonance, which results in enhancement of Raman signals. Thus, SERS is a promising technique that gives structural information, and molecular fingerprints can be obtained from it even if the sample is in small quantity.20
Because of their high surface plasmon properties, noble metal nanoparticles exhibit strong SERS enhancement factors. For the SERS spectral investigation of diverse microorganisms, silver nanoparticles (Ag-NPs) as a SERS substrate are used because there are more active sites in Ag NPs, the resonance of the surface plasmon is amplified, and can lead to a stronger SERS enhancement than in gold NPs.19
Different bacteria have varying abilities to desulfurize DBT. In the present work, the DBT desulfurization activity of bacteria is determined by Gibb's assay after their growth in mineral glucose (MG) media. Characterization of these DBT desulfurizing bacteria by SERS has been done based on the specific biochemical constituents present in them, and discrimination is successfully carried out by using data analysis techniques like PCA and PL-SDA. Through differentiation of DBT desulfurizing bacteria, researchers can learn more about their distinct traits and develop more effective biodesulfurization strategies.
All isolates are identified by the 16S rRNA sequence under accession no. Chryseobacterium sp. IS (OM866180), Gordonia sp. 4N (KP771870), Mycolicibacterium sp. J2 (OM866178), and Rhodococcus sp. J16 (OM866179) present in the NCBI database (Table 1).
Table 1 Details of four different types of DBT desulfurizing bacterial strains
Sample name |
Bacterial strains |
Accession no. (16S rRNA) |
C1 |
Chryseobacterium sp. IS |
(OM866180) |
C2 |
Chryseobacterium sp. IS |
(OM866180) |
C3 |
Chryseobacterium sp. IS |
(OM866180) |
G1 |
Gordonia sp. 4N |
(KP771870) |
G2 |
Gordonia sp. 4N |
(KP771870) |
G3 |
Gordonia sp. 4N |
(KP771870) |
M1 |
Mycolicibacterium sp. J2 |
(OM866178) |
M2 |
Mycolicibacterium sp. J2 |
(OM866178) |
M3 |
Mycolicibacterium sp. J2 |
(OM866178) |
R1 |
Rhodococcus sp. J16 |
(OM866179) |
R2 |
Rhodococcus sp. J16 |
(OM866179) |
R3 |
Rhodococcus sp. J16 |
(OM866179) |
2. Materials and methods
2.1. Growth media
Four previously isolated DBT desulfurizing bacterial isolates including Mycolicibacterium sp. J2 and Rhodococcus sp. J16 (isolated from oil-contaminated soil samples), Gordonia sp. 4N (isolated from soil near a coal heap), and Chryseobacterium sp. IS (isolated from sludge sample), are used in this study. The bacterial isolates are cultured in triplicate in sulfur-free MG medium. DBT serves as the sole sulfur source for bacterial growth. The MG medium (sulfur-free) is made up of 5.0 g glucose, 0.2 g MgCl2·6H2O, 2.0 g of KH2PO4 2, 4.0 g K2HPO4, 1.0 g NH4Cl, 1.0 ml vitamin mixture, and metal solution (10.0 ml) in distilled water (1000 ml) (pH 7.0).12
2.2. Bacterial culture
In Erlenmeyer flasks (250 ml), MG medium (100 ml) with a pH adjustment of 1 M NaOH is taken. Then, using an automated pipette, DBT solution (50 mM stock solution dissolved in ethanol) is added in each flask until the final concentration is 0.2 mM. Each flask is inoculated with a freshly prepared MG medium containing grown bacterial culture at an inoculum size of 5% (v/v). The flasks are incubated for 72 hours at 30 °C and 180 rpm in a gyratory shaker. Samples are collected, and the Gibb's assay is used to determine the bio desulfurization of DBT into 2-hydroxybiphenyl (2-HBP).12
2.3. Gibb's assay for the detection of 2-HBP
A calorimetric method called the Gibb's assay is used to evaluate the bacterial cultures for their DBT desulfurization activity. This method is used to quantify and detect 2-HBP in the culture medium in the presence of Gibb's reagent. For this purpose, in an Eppendorf tube, 1.0 ml (aliquot removed from each culture broth) is centrifuged for 5 minutes at 12
000 rpm. Then, 150 μl of each cell-free supernatant is taken to fresh tubes, and the pH is adjusted to 8 by adding 30 μl of NaHCO3 (1 M) to it. A 20 μl volume of Gibb's reagent (formulated by mixing 100 mg of 2,6-dichloroquinone-4-chloroimide in 100 ml of ethanol) is added to the tubes. The formation of 2-HBP, the end-product of DBT desulfurization, is indicated by the appearance of a blue colour due to the formation of a complex between the Gibb's reagent and the hydroxyl group (aromatic) of 2-HBP within half an hour, as presented in Fig. 1. These Gibb's positive isolates are employed in the next studies.6
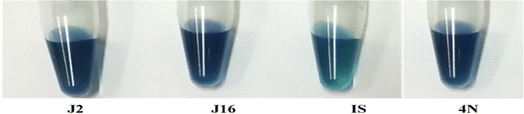 |
| Fig. 1 Blue color representing the conversion of DBT to 2-HBP. | |
2.4. Harvesting of the DBT grown cultures
After 72 hours of incubation as described earlier, the whole culture is harvested and the culture broth are kept at 4 °C until further use. Centrifugation is done at 10
000 rpm for 10 minutes, and the pellets are isolated and stored at 4 °C until further SERS analysis is conducted.
2.5. Synthesis of Ag-NPs
To synthesize Ag-NPs, in the present study, the chemical reduction method is used. It includes silver nitrate (AgNO3) as a precursor and tri-sodium citrate (Na3C6H5O7), which acts both as a capping ligand and a reducing agent.21 Briefly, in the deionized water, AgNO3 solution (0.0085 g/300 ml) is heated at 100 °C, and 10 ml of 1% Na3C6H5O7 is added to it. The solution is heated on a hot plate with continuous stirring by using a magnetic stirrer for 90 minutes. Ag-NPs of spherical shape and gray color with an intermediate size of 53 nm are obtained, which are used as a substrate for acquiring SERS spectra from the bacterial pellets. A field emission electron microscope (FEM) (JEOL, JSM-7500F), which operates in both modes as SEM and TEM, is used for the characterization of the synthesized Ag-NPs. This has already been reported in our previous work, and is also shown in supplementary material Fig. S1(a)–(c).†22 Ag-NPs have received significant attention because of their physical and chemical attributes. The textured surface of Ag-NPs exhibits the stronger Raman enhancement effect.
2.6. Acquisition of SERS spectra
Four bacterial strains, including Chryseobacterium sp. IS, Gordonia sp. 4N, Mycolicibacterium sp. J2, and Rhodococcus sp. J16, are employed in this work to identify their characteristic SERS spectral features. A Raman spectrometer (OPTOSKY (ATR 8300)) is used to acquire the SERS spectral features of cell pellets of each bacterial strain in triplicate. For this, a diode laser (785 nm) is employed as a source for excitation. For the SERS spectral acquisition of samples, 30 μl of bacterial pellet, suspended in an equal volume of saline solution of each bacterial strain, is mixed with 30 μl of Ag-NPs and the mixture is incubated for 30 minutes. Both sample and Ag-NPs are mixed in equal proportion in the Eppendorf, and this protocol (1
:
1) is taken from the literature.23 After 30 minutes, 50 μl from this mixture is placed on the aluminum (Al) slide by using a micropipette. 15 SERS spectra of each of the 12 samples (4 strains in triplicate) are recorded by moving the stage to different positions on the sample to eliminate the chances of bias in the results. For this purpose, 50 mW laser power, 40× lens, and 5 seconds integration time are used. The spectra of the aluminum slide and the pure NPs are also recorded by following the same protocol for the purpose of background removal.
2.7. Preprocessing of the SERS spectra of bacterial samples
The SERS spectral data of strains are preprocessed in the range between 400 and 1800 cm−1. Pre-processing steps, including vector normalization, smoothing, substrate removal, and baseline correction, are done by using MATLAB 7.2. Savitzky–Golay is an algorithm that is employed for vector normalization and smoothening purposes for the normalization of spectra. Polynomial and rubber band methods are utilized for baseline correction. Using MATLAB codes, substrate removal (background) is done by subtracting the spectra of “Ag-NPs on the Al slide” from the SERS spectra of the bacterial pellets.
2.8. SERS spectral data analysis
The SERS spectral features of the bacterial strains are analyzed by comparing their mean spectra to differentiate the different characteristic SERS peaks of each sample, and interpretation is done by comparing the SERS features with the literature assigned in Table 2. For further differentiation of the spectra of the four different bacteria, multivariate data analysis techniques like PCA and PLS-DA are used. PCA is employed to check the differentiation and variability of the SERS spectral data sets of desulfurizing bacterial pellets, and also the reason for differentiation in the form of PCA loadings, which help to identify the characteristic SERS peaks. The loading plot shows the reason for classification as a function of wavenumber. Using PCA scatter plots, the SERS spectra of each sample are grouped into clusters. PCA works by maintaining the variability, while decreasing the dimensionality in the sets of data. A supervised chemometric method, PLS-DA is used to successfully differentiate the SERS spectral data sets. It combines the elements of both PCA and linear discriminant analysis (LDA). In the MATLAB software 7.2, the PLS Toolbox is used to analyze the preprocessed SERS spectral data of different bacteria. This method is designed to capture the data's systematic change, while reducing the influence of random noise. PLS-DA proves to be a versatile method that may be used for predictions of spectral data, model descriptions, and dividing data into multiple sets in order to categorize various bacterial species. PLS-DA findings can be seen using score plots and loading plots. It plays a crucial role in both quantitative and qualitative analysis. These visualizations can help in the interpretation of the SERS data by clearly illustrating the distinction between classes and the connections between the variables.
Table 2 Peak assignments of the SERS spectral features of desulfurizing bacterial strains
SERS peaks |
Assignments |
Components |
References |
533 |
C–O–C (glycosidic ring) deformation |
Carbohydrates |
24 |
564 |
(C–S–S–C) vibration |
Proteins |
21 |
635 |
C–S stretching |
Proteins |
25 |
653 |
Guanine ring breathing |
DNA |
18 and 26 |
697 |
C–S stretching |
Proteins |
25 and 27 |
725 |
Adenine ring stretching |
DNA/RNA |
18 |
746 |
Ring breathing in tyrosine |
Amino acids |
28 |
813 |
C–O–P–O–C–RNA binding |
Proteins |
18 |
868 |
C–O–C stretching (ribose) |
Carbohydrates |
29 |
885 |
(C–C) stretching |
Carbohydrates |
30 |
957 |
CH out-of-plane wagging |
Carotenoids |
31 |
986 |
C–C stretching |
Proteins (peptidoglycan) |
18 |
1004 |
Phenylalanine symmetric ring breathing mode |
Proteins |
18, 32 and 33 |
1071 |
C–N stretching |
Proteins |
24 |
1097 |
C–C, C–O, –C–OH deformation |
Carbohydrates |
24 |
1129 |
NAD (co-enzyme) |
Protein |
34 |
1206 |
C–C6H5 stretching, (phenylalanine) |
Proteins |
24 and 35 |
1222 |
Amide III |
Proteins |
25 |
1242 |
N–H bending (β-sheet amide III) |
DNA/RNA |
18 and 36 |
1307 |
CH2 twisting |
Lipids |
24 |
1323 |
C–NH2 stretching of amide II |
Proteins |
37 |
1371 |
(COO−) in guanine |
DNA |
21 |
1404 |
N(CO2−) stretch α-amino acids |
Proteins |
38 |
1452 |
(CH2) bending |
Lipids |
32 |
1590 |
(C C) olefinic stretching |
Lipids |
25 and 37 |
1692 |
C O stretching |
Lipids |
39 |
1707 |
Amide I α-helix |
Proteins |
29 |
3. Results and discussion
3.1. SERS mean spectra
Fig. 2 shows the SERS mean spectra of Chryseobacterium sp. IS, Gordonia sp. 4N, Mycolicibacterium sp. J2 and Rhodococcus sp. J16, which clearly differentiates the bacterial cell pellets of different species based on their chemical constituents molecules, such as carbohydrates, proteins, lipids, genetic constituents (DNA/RNA), and pigments. The solid lines in the SERS spectra indicate the differentiating features in various types of bacteria. Meanwhile, dashed lines are used to indicate the bands that are present in all types of bacteria, but differ only in intensity.
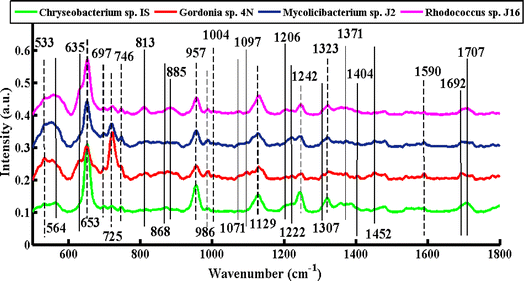 |
| Fig. 2 SERS mean spectra of Chryseobacterium sp. IS (green), Gordonia sp. 4N (red), Mycolicibacterium sp. J2 (blue), and Rhodococcus sp. J16 (magenta). | |
The SERS signals observed in the SERS spectra of pellets from all four types of bacteria include features at 533, 653, 697, 725, 746, 957, 986, 1004, 1129, 1242, 1323, and 1590 cm−1.
A SERS peak at 533 cm−1 (C–O–C glycosidic ring deformation) with the same intensity is observed in all of the spectra, which can be associated with carbohydrates. SERS bands of almost the same intensity at 697 cm−1 (C–S stretching) and 1004 cm−1 (phenylalanine ring breathing) are seen in all spectra, and can be assigned to proteins. A high-intensity DNA band at 653 cm−1 (guanine ring breathing) is observed with high intensity in the spectrum of Chryseobacterium sp. IS (Gram-negative), Mycolicibacterium sp. J2, and Rhodococcus sp. J16, as compared to Gordonia sp. 4N (Gram-positive), where it is less intense. This more pronounced peak of Chryseobacterium sp. IS than the other three Gram-positive strains might be explained by a higher percentage of GC present in the DNA of Gram-negative bacteria.18,40 The most prominent band at 725 cm−1 (adenine ring stretching) is present with greater intensity in Gordonia sp. 4N, Mycolicibacterium sp. J2, and Rhodococcus sp. J16 (Gram-positive) than Chryseobacterium sp. IS (Gram-negative). This is probably due to the fact that the peptidoglycan layer in the Gram-positive bacteria is thicker (10–20 layers) than the peptidoglycan layer in the Gram-negative bacteria by about 1 to 3 layers.41 The SERS feature at 746 cm−1 (tyrosine ring breathing in amino acid) is noticed with less intensity in Gordonia sp. 4N than in the other three. The SERS feature at 957 cm−1 (CH out-of-plane wagging) might be due to carotenoids, which impart different colors to microorganisms with respect to their chemical structure.31 In the present study, all four strains have this biochemical component (carotenoid).42–45 This peak has a high intensity in Chryseobacterium sp. IS, Mycolicibacterium sp. J2 and Rhodococcus sp. J16, but it has low intensity in Gordonia sp. 4N. The SERS band at 986 cm−1 (C–C stretching) is because of peptidoglycan. It is observed to have reduced intensity in Chryseobacterium sp. IS, but has a somewhat increased intensity in the other three Gram-positive species. The SERS signal at 1129 cm−1 (NAD co-enzyme) used during the process of biodesulfurization is present with less intensity in Gordonia sp. 4N and Mycolicibacterium sp. J2, but has a greater intensity in Chryseobacterium sp. IS and Rhodococcus sp. J16. The SERS band at 1242 cm−1 that corresponds to N–H bending (beta sheet amide III) is due to proteins, and has greater intensity in Chryseobacterium sp. IS than the other three bacterial strains. The SERS characteristic feature at 1323 cm−1 (C–NH2 stretching of amide II) has increased intensity in Chryseobacterium sp. IS and Rhodococcus sp. J16, but lower in Gordonia sp. 4N and Mycolicibacterium sp. J2. The SERS band at 1590 cm−1 (C
C olefinic stretching of lipids) has almost the same intensity in all of the bacterial cell pellet samples.
The SERS bands that show dissimilarities in the spectra of various types of bacteria include 564, 635, 813, 868, 885, 1071, 1097, 1206, 1222, 1307, 1371, 1404, 1452, 1692, and 1707 cm−1.
The SERS differentiating feature at 564 cm−1 (C–S–S–C vibration in proteins) is present only in Chryseobacterium sp. IS. For proper functioning and stability of proteins in bacteria, these disulfide bonds are considered important.21 A protein band at 635 cm−1 (C–S stretch) is observed only in Gordonia sp. 4N and Rhodococcus sp. J16. This may be due to the fact that proteins involved in desulfurization processes may exhibit increased band intensity. The protein band at 813 cm−1 (C–O–P–O–C RNA binding tyrosine) may be due to the production of tyramine by the presence of tyrosine decarboxylase.18 The production of tyramine by certain Gram-positive bacteria has been previously reported.46 A more pronounced peak at this position is present in Rhodococcus sp. J16, while it is absent in Mycolicibacterium sp. J2 and Gordonia sp. 4N. In the literature, the presence of tyramine has also been reported in Rhodococcus sp. J16,47 although no clear evidence was found for its presence in Gram-negative species.48 The signal at 813 cm−1 is absent in Chryseobacterium sp. IS. The SERS signature band at 868 cm−1 (C–O–C stretching ribose), and 885 cm−1 (C–C stretching) can be assigned to carbohydrates, which is present only in Chryseobacterium sp. IS and Rhodococcus sp. J16, respectively. This may due to the fact that some proteins involved in desulfurization may be glycosylated, meaning they have a carbohydrate molecule attached to them. Glycoproteins are involved in enhancing the activity of enzymes involved in the desulfurization process.49 The protein bands found at 1071 cm−1 (C–N stretching) and 1206 cm−1 (C–C6H5 stretching, phenylalanine) are also observed only in Rhodococcus sp. J16, which may be due to the protein responsible for the desulfurizing ability. The SERS feature at 1097 cm−1 (C–C, C–O, –C–OH deformation) may be due to carbohydrates, which is only observed in Gordonia sp. 4N. The SERS signal at 1222 cm−1 (amide III) is only observed in Gordonia sp. 4N and Mycolicibacterium sp. J2. The band at 1307 cm−1 (CH2 twist) may be due to lipids present only in Gordonia sp. 4N. Lipids also modulate the activity of enzymes involved in the desulfurization in Gordonia sp.50 The SERS band at 1371 cm−1 (COO− in guanine DNA) is noticed only in Gordonia sp. 4N and Mycolicibacterium sp. J2, and may be due to the specific genetic trait responsible for desulfurization in these strains. The lipid bands at 1404 cm−1 ((CO2−) stretching α-amino acids), 1452 cm−1 (CH2 bending vibration lipids) and 1692 cm−1 (C
O stretching of fatty acids) are only present in Gordonia sp. 4N. The signal at 1707 cm−1 may be due to the amide I α-helix, and is only observed in Chryseobacterium sp. IS and Rhodococcus sp. J16 bacteria.
3.2. Principal component analysis (PCA)
Fig. 3 shows the PCA scatter plot of the SERS spectral data of all desulfurizing bacterial pellets, including Chryseobacterium sp. IS (green), Gordonia sp. 4N (red), Mycolicibacterium sp. J2 (blue), and Rhodococcus sp. J16 (magenta). The Gram-positive species, including Gordonia sp. 4N and Mycolicibacterium sp. J2, are represented by the red and blue dots, respectively, which are clustered on the negative side of the first principal component (PC-1). The SERS spectra of the Gram-positive specie Rhodococcus J16 and Gram-negative specie Chryseobacterium IS are shown by magenta and green dots, respectively, in the PC-1 of the scatter plot clustered on the positive side. In the PCA scatter plot, each dot represents one spectrum, while each cluster belongs to a specific SERS data set of one bacterial sample. It can be observed in the PCA scatter plot that there is a clear differentiation in the SERS spectra of the different bacterial species, and it shows the potential of the SERS technique to discriminate the species based on the changes in their biochemical components. The PCA scatter plot represents the differentiation of PC-1 with 67.96% data variability.
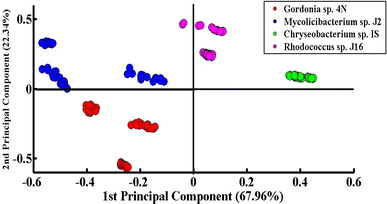 |
| Fig. 3 PCA scatter plot of the SERS spectra of four different desulfurizing bacterial strains. | |
3.2.1. Pairwise PCA analysis. The potential of SERS to differentiate cell pellets of different bacteria based on their different biochemical components is further explored by analyzing their SERS spectral data sets by using pairwise PCA analysis, in which one group of spectra is compared with the other.Fig. 4(a) indicates the PCA comparison between the SERS spectra of Chryseobacterium sp. IS (Gram-negative) and Gordonia sp. 4N (Gram-positive). In the scatter plot, the spectra of Chryseobacterium sp. IS are represented by green dots and those of Gordonia sp. 4N are represented by red dots. PCA depicts a clear differentiation in the spectral data sets of two bacterial pellets comprising Chryseobacterium sp. IS and Gordonia sp. 4N by clustering them separately on the negative and positive sides of PC-1, respectively. The variance obtained by PC-1 and PC-2 is 76.04% and 19.20%, respectively.
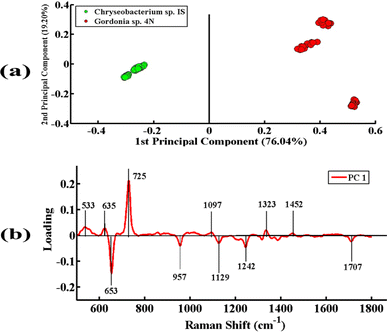 |
| Fig. 4 (a) PCA scatter plot of SERS spectra of Chryseobacterium sp. IS (green) and Gordonia sp. 4N (red). (b) PCA loadings of SERS spectral comparison between Chryseobacterium sp. IS, and Gordonia sp. 4N. | |
Fig. 4(b) illustrates the PCA loadings of the SERS spectral data sets of cell pellets from these two desulfurizing bacterial species. A scatter plot shows the clustering of spectra of different species at different positions in the PC-1 and the biochemical components responsible for this differentiation are indicated by PCA loadings (PC-1 loadings).
The positive loadings including 533 cm−1 (C–O–C glycosidic ring deformation), 635 cm−1 (C–S stretching), 725 cm−1 (adenine ring stretching), 1097 cm−1 (C–C, C–O, –C–OH deformation), 1323 cm−1 (C–NH2 stretching of amide II), and 1452 cm−1 (CH2 bending vibration) are associated with Gordonia sp. 4N. The negative loadings include 653 cm−1 (guanine ring breathing), 957 cm−1 (CH out-of-plane wagging), 1129 cm−1 (NAD co-enzyme), 1242 cm−1 (N–H bending), and 1707 cm−1 (amide I α-helix), which are associated with Chryseobacterium sp. IS. These PCA loadings have the same SERS features of differentiation, as shown in the mean plot presented in Fig. 2; hence, confirming the findings related to the biochemical differences between the two bacterial strains.
Fig. 5(a) presents the PCA pairwise comparison between the SERS spectra of Chryseobacterium sp. IS (Gram-negative) and Mycolicibacterium sp. J2 (Gram-positive) bacteria. In the PCA scatter plot, the spectra of Chryseobacterium sp. IS and Mycolicibacterium sp. J2 are represented by green dots and blue dots, respectively. It depicts a clear differentiation between the SERS spectra of both bacterial pellets by clustering them separately on the positive and negative sides of PC-1. The spectra of Chryseobacterium sp. IS are clustered on the negative side of PC-1, while those of the other strain are clustered on the positive side of PC-1. The variance obtained by PC-1 and PC-2 is 85.74% and 11.63%, respectively.
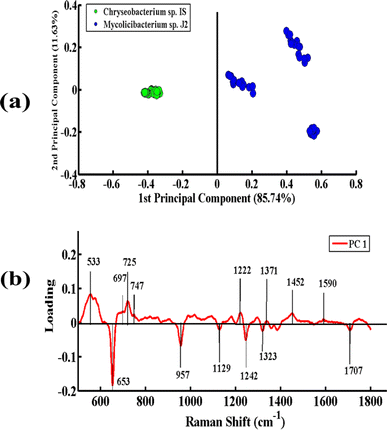 |
| Fig. 5 (a) PCA scatter plot of the SERS spectra of Chryseobacterium sp. IS (green), Mycolicibacterium sp. J2 (blue). (b) PCA loadings of the SERS spectra between Chryseobacterium sp. IS and Mycolicibacterium sp. J2. | |
Fig. 5(b) presents the PCA loadings of the SERS spectra of these two bacterial species. The positive loadings, including 533 cm−1 (C–O–C glycosidic ring deformation), 697 cm−1 (C–S stretching), 725 cm−1 (adenine ring stretching), 746 cm−1 (tyrosine ring breathing in amino acid), 1222 cm−1 (amide III), 1371 cm−1 (COO− in guanine DNA), 1452 cm−1 (CH2 bending vibration lipids), and 1590 cm−1 (C
C olefinic stretching of lipids), are associated with Mycolicibacterium sp. J2. The negative loadings, including 653 cm−1 (guanine ring breathing), 957 cm−1 (CH out-of-plane wagging), 1129 cm−1 (NAD co-enzyme), 1242 cm−1 (N–H bending (beta sheet amide III)), and 1323 cm−1 (C–NH2 stretching of amide II), are linked to Chryseobacterium sp. IS. These PCA loadings have the same SERS features, as observed in the mean SERS plot (Fig. 2), and thus can be utilized as biomarkers for SERS differentiation of the bacterial strains.
Fig. 6(a) represents the PCA pairwise comparison between the SERS spectra of Chryseobacterium sp. IS (Gram-negative) and Rhodococcus sp. J16 (Gram-positive). In the PCA scatter plot, the spectra of Chryseobacterium sp. IS are represented by green dots and those of Rhodococcus sp. J16 as magenta dots. PCA depicts a clear discrimination between the spectra of the two bacterial pellets by clustering them separately on the positive and negative sides of PC-1. The spectra of Chryseobacterium sp. IS are clustered on PC-1 (negative side), while those of Rhodococcus sp. J16 strain are clustered on PC-1 (positive side). The variance obtained by PC-1 and PC-2 is 86.72% and 9.15%, respectively.
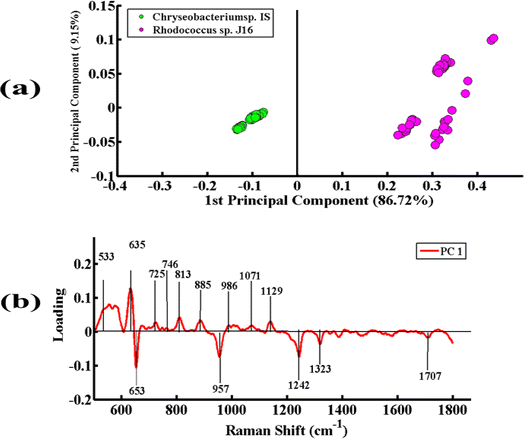 |
| Fig. 6 (a) PCA scatter plot of the SERS spectra of Chryseobacterium sp. IS (green) and Rhodococcus sp. J16 (magenta). (b) PCA loadings of the SERS spectra of Chryseobacterium sp. IS and Rhodococcus sp. J16. | |
Fig. 6(b) presents the PCA loadings of the spectra of these two bacterial species. The positive loadings, including 533 cm−1 (C–O–C glycosidic ring deformation), 635 cm−1 (C–S stretching), 725 cm−1 (adenine ring stretching), 746 cm−1 (tyrosine ring breathing in amino acid), 813 cm−1 (C–O–P–O–C–RNA binding tyrosine), 885 cm−1 (C–C stretching), 986 cm−1 (C–C stretching), 1071 cm−1 (C–N stretching), and 1129 cm−1 (NAD co-enzyme), are associated with Rhodococcus sp. J16 (shows on the positive side (PC-1)). The negative loadings, including 653 cm−1 (guanine ring breathing), 957 cm−1 (CH out-of-plane wagging), 1242 cm−1 (N–H bending), 1323 cm−1 (C–NH2 stretching of amide II), and 1707 cm−1 (amide I α-helix), are associated with Chryseobacterium sp. IS. It is observed that all of these features match with the SERS peaks observed in the SERS mean plot (Fig. 2), therefore confirming these findings.
3.3. Discrimination of the bacterial SERS spectra using PLS-DA
PLS-DA is an excellent discrimination model, employed in this study for the differentiation of the SERS spectra of the desulfurizing bacterial pellets. It is a supervised statistical model that gives quantitative classification. PLS-DA has the benefit that the model development and differentiation of different groups of spectra do not require information related to the sample constitution. To prevent data bias, the SERS spectral data from four different desulfurizing bacterial pellets were independently split into two groups comprising 45% of the calibration data and 55% of the validation data.
The scatter score plot of the PLS-DA model of the SERS spectra acquired from four different bacterial pellets is shown in Fig. 7. The spectra of all four strains are shown by separate clusters. Green dots present on the positive side are related to the SERS spectra of Chryseobacterium sp., blue dots on the positive side are related to Mycolicibacterium sp., red dots on the negative side are due to Gordonia sp., and the magenta-colored dots on the positive side are related to the SERS spectra of Rhodococcus sp.
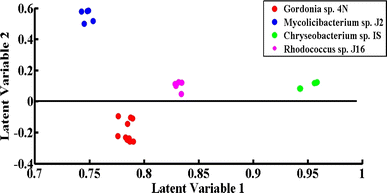 |
| Fig. 7 PLS-DA scatter score plot of the SERS spectra of Chryseobacterium sp. IS (green), Gordonia sp. 4N (red), Mycolicibacterium sp. J2 (blue) and Rhodococcus sp. J16 (magenta). | |
Fig. 8 represents the PLS-DA score plot of the SERS spectral data sets acquired from the cell pellets of four different desulfurizing bacterial strains, showing good differentiation of these spectra with an AUC value of 0.78 obtained from the ROC curve. A ROC value near 1 indicates the maximum validation and accuracy. In the present case, the value of 0.78 demonstrates the excellent performance of the model. The PLS-DA model of four desulfurizing bacterial strains shows the precision of 95%, specificity of 96%, sensitivity of 95.8%, AUC 0.78, and accuracy of 97.09%.
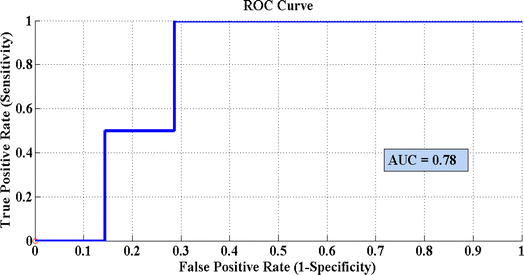 |
| Fig. 8 Receiver operating curve (ROC) of the PLS-DA model for differentiation of the SERS spectral data sets of desulfurizing bacterial pellets of Chryseobacterium sp. IS, Gordonia sp. 4N, Mycolicibacterium sp. J2 and Rhodococcus sp. J16. | |
4. Conclusions
The results demonstrated that SERS can differentiate and identify differences in the SERS spectral features of cell pellets of four different desulfurizing bacterial strains based on their biochemical composition. After their growth in MG media, and confirmation of the desulfurization activity of the bacteria by Gibb's assay, the SERS spectral features associated with these pellets were used to differentiate the four desulfurizing bacteria, including Chryseobacterium sp. IS, Gordonia sp. 4N, Mycolicibacterium sp. J2, and Rhodococcus sp. J16. Statistically, significant variations in the peak positions and intensity were found to be associated with the proteins, lipids, nucleic acids, and peptidoglycan (biochemical components). Moreover, to further clarify the differences, multivariate analytical techniques like PCA and PLS-DA were used. Pairwise PCA analysis of Gram-negative species with Gram-positive ones was used to further support the results. PLS-DA was also an excellent technique to discriminate and identify the SERS spectral data of cell pellets of desulfurizing bacterial strains with a sensitivity of 95.8%, specificity of 96%, AUC value of 0.78, precision of 95%, and accuracy of 97.09%.
Conflicts of interest
The authors have no known competing financial interests or personal relationships that could have appeared to influence the work reported in this paper.
Acknowledgements
This research work was supported by Higher Education Commission, Pakistan through a research Grant (NRPU-11570) awarded to Dr Nasrin Akhtar. The authors are thankful to the Researchers Supporting Project number (RSPD2024R1035), King Saud University, Riyadh, Saudi Arabia.
References
- T. Ohshiro, Y. Ishii, T. Matsubara, K. Ueda, Y. Izumi, K. Kino and K. Kirimura, J. Biosci. Bioeng., 2005, 100, 266–273 CrossRef CAS PubMed.
- N. Akhtar, K. Akhtar and M. A. Ghauri, Curr. Microbiol., 2018, 75, 597–603 CrossRef CAS PubMed.
- W. H. Calkins, Fuel, 1994, 73, 475–484 CrossRef CAS.
- E. Karimi, F. Yazdian, B. Rasekh, C. Jeffryes, H. Rashedi, A. A. Sepahi, S. Shahmoradi, M. Omidi, M. Azizi and M. E. Bidhendi, Fuel, 2018, 216, 787–795 CrossRef CAS.
- R. Javadli and A. De Klerk, Appl. Petrochem. Res., 2012, 1, 3–19 CrossRef CAS.
- N. Akhtar, M. A. Ghauri, M. A. Anwar and K. Akhtar, FEMS Microbiol. Lett., 2009, 301, 95–102 CrossRef CAS PubMed.
- M. R. Gray, A. R. Ayasse, E. W. Chan and M. Veljkovic, Energy Fuels, 1995, 9, 500–506 CrossRef CAS.
- G. Couch, London: Coal Research Report, 1993 Search PubMed.
- M. Soleimani, A. Bassi and A. Margaritis, Biotechnol. Adv., 2007, 25, 570–596 CrossRef CAS PubMed.
- T. Ö. Bozdemir, T. Durusoy, E. Erincin and Y. Yürüm, Fuel, 1996, 75, 1596–1600 CrossRef.
- K. Kodama, K. Umehara, K. Shimizu, S. Nakatani, Y. Minoda and K. Yamada, Agric. Biol. Chem., 1973, 37, 45–50 CrossRef CAS.
- N. Akhtar, M. A. Ghauri and K. Akhtar, Arch. Microbiol., 2016, 198, 509–519 CrossRef CAS.
- M. Kalita, M. Chutia, D. K. Jha and G. Subrahmanyam, Curr. Microbiol., 2022, 79, 82 CrossRef CAS PubMed.
- L. Favre, A. Ortalo-Magné, S. Greff, T. Perez, O. P. Thomas, J.-C. Martin and G. Culioli, J. Proteome Res., 2017, 16, 1962–1975 CrossRef CAS PubMed.
- O. Preisner, J. A. Lopes, R. Guiomar, J. Machado and J. C. Menezes, Anal. Bioanal. Chem., 2007, 387, 1739–1748 CrossRef CAS PubMed.
- T. L. Palama, I. Canard, G. J. Rautureau, C. Mirande, S. Chatellier and B. Elena-Herrmann, Analyst, 2016, 141, 4558–4561 RSC.
- W. Premasiri, D. Moir, M. Klempner, N. Krieger, G. Jones and L. Ziegler, J. Phys. Chem. B, 2005, 109, 312–320 CrossRef CAS PubMed.
- F. S. de Siqueira e Oliveira, A. M. da Silva, M. T. T. Pacheco, H. E. Giana and L. Silveira, Lasers Med. Sci., 2021, 36, 289–302 CrossRef PubMed.
- M. Shakeel, M. I. Majeed, H. Nawaz, N. Rashid, A. Ali, A. Haque, M. U. Akbar, M. Tahir, S. Munir and Z. Ali, Photodiagn. Photodyn. Ther., 2022, 40, 103145 CrossRef CAS PubMed.
- S. Efrima and L. Zeiri, J. Raman Spectrosc., 2009, 40, 277–288 CrossRef CAS.
- M. Kashif, M. I. Majeed, H. Nawaz, N. Rashid, M. Abubakar, S. Ahmad, S. Ali, H. Hyat, S. Bashir and F. Batool, Spectrochim.
Acta, Part A, 2021, 261, 119989 CrossRef CAS.
- U. E. Habiba, A. Anwer, M. U. Hussain, M. I. Majeed, N. Alwadie, H. Nawaz, N. Akhtar, N. Rashid, S. Nadeem and M. Naz, Spectrochim. Acta, Part A, 2024, 313, 124126 CrossRef CAS PubMed.
- A. Ditta, M. I. Majeed, H. Nawaz, M. A. Iqbal, N. Rashid, M. Abubakar, F. Akhtar, A. Nawaz, W. Hameed and M. Iqbal, Photodiagn. Photodyn. Ther., 2022, 39, 102941 CrossRef CAS PubMed.
- M. L. Paret, S. K. Sharma, L. M. Green and A. M. Alvarez, Appl. Spectrosc., 2010, 64, 433–441 CrossRef CAS PubMed.
- M. Çulha, A. Adigüzel, M. M. Yazici, M. Kahraman, F. Slahin and M. Güllüce, Appl. Spectrosc., 2008, 62, 1226–1232 CrossRef PubMed.
- E. Koglin, J.-M. Séquaris and P. Valenta, SERS Detected Adsorption of Guanine on Small Particles of Colloidal Silver, in Surface Studies with Lasers: Springer Series in Chemical Physics, ed. F. R. Aussenegg, A. Leitner and M. E. Lippitsch, Springer, Berlin, Heidelberg, 1983, vol. 33, DOI:10.1007/978-3-642-82085-4_9.
- E. Podstawka, Y. Ozaki and L. M. Proniewicz, Appl. Spectrosc., 2004, 58, 1147–1156 CrossRef CAS PubMed.
- R. M. Jarvis, N. Law, I. T. Shadi, P. O'Brien, J. R. Lloyd and R. Goodacre, Anal. Chem., 2008, 80, 6741–6746 CrossRef CAS PubMed.
- S. Bashir, H. Nawaz, M. I. Majeed, M. Mohsin, S. Abdullah, S. Ali, N. Rashid, M. Kashif, F. Batool and M. Abubakar, Photodiagn. Photodyn. Ther., 2021, 34, 102280 CrossRef CAS PubMed.
- M. Saleem, H. Nawaz, M. I. Majeed, N. Rashid, F. Anjum, M. Tahir, R. Shahzad, A. Sehar, A. Sabir and N. Rafiq, Photodiagn. Photodyn. Ther., 2023, 41, 103278 CrossRef PubMed.
- A. S. Fernandes, S. M. Paixão, T. P. Silva, J. C. Roseiro and L. Alves, Bioprocess Biosyst. Eng., 2018, 41, 143–155 CrossRef CAS PubMed.
- K. C. Schuster, I. Reese, E. Urlaub, J. R. Gapes and B. Lendl, Anal. Chem., 2000, 72, 5529–5534 CrossRef CAS PubMed.
- C. Fan, Z. Hu, A. Mustapha and M. Lin, Appl. Microbiol. Biotechnol., 2011, 92, 1053–1061 CrossRef CAS PubMed.
- O. Siiman, R. Rivellini and R. Patel, Inorg. Chem., 1988, 27, 3940–3949 CrossRef CAS.
- L. J. Goeller and M. R. Riley, Appl. Spectrosc., 2007, 61, 679–685 CrossRef CAS PubMed.
- S. A. Strola, J.-C. Baritaux, E. Schultz, A. C. Simon, C. Allier, I. Espagnon, D. Jary and J.-M. Dinten, J. Biomed. Opt., 2014, 19, 111610 CrossRef PubMed.
- H. Félix-Rivera, R. González, G. D. M. Rodríguez, O. M. Primera-Pedrozo, C. Ríos-Velázquez and S. P. Hernández-Rivera, Int. J. Spectrosc., 2011, 2011, 989504 CrossRef.
- R. M. Jarvis, A. Brooker and R. Goodacre, Anal. Chem., 2004, 76, 5198–5202 CrossRef CAS PubMed.
- L. Lu and W. G. Qin, Biotechnol. Biotechnol. Equip., 2011, 25, 2528–2532 CrossRef CAS.
- A. Muto and S. Osawa, Proc. Natl. Acad. Sci. U. S. A., 1987, 84, 166–169 CrossRef CAS PubMed.
- A. Zhu, S. Ali, Y. Xu, Q. Ouyang, Z. Wang and Q. Chen, Spectrochim. Acta, Part A, 2022, 270, 120814 CrossRef CAS.
- J. Zhang, C. Gao, X.-M. Yu, H.-Y. Lun and Z.-J. Du, Front. Microbiol., 2020, 11, 251 CrossRef.
- T. P. Silva, L. Alves and S. M. Paixao, J. Environ. Manage., 2020, 270, 110825 CrossRef CAS.
- S. Takaichi, J.-i. Ishidsu, T. Seki and S. Fukada, Agric. Biol. Chem., 1990, 54, 1931–1937 CAS.
- R. G. Barletta and D. J. Steffen, Vet. Microbiol., 2022, 345–359 Search PubMed.
- M.-J. Kim and K.-S. Kim, LWT--Food Sci. Technol., 2014, 56, 406–413 CrossRef CAS.
- A. Orro, M. Cappelletti, P. D'Ursi, L. Milanesi, A. Di Canito, J. Zampolli, E. Collina, F. Decorosi, C. Viti and S. Fedi, PLoS One, 2015, 10, e0139467 CrossRef PubMed.
- A. Marcobal, B. De Las Rivas, J. M. Landete, L. Tabera and R. Muñoz, Crit. Rev. Food Sci. Nutr., 2012, 52, 448–467 CrossRef CAS PubMed.
- L. C. Mills, 2019.
- S. Parveen, N. Akhtar, T. E-kobon, R. Burchmore, A. I. Hussain and K. Akhtar, World J. Microbiol. Biotechnol., 2024, 40, 103 CrossRef CAS PubMed.
|
This journal is © The Royal Society of Chemistry 2024 |
Click here to see how this site uses Cookies. View our privacy policy here.