DOI:
10.1039/D4LC00073K
(Paper)
Lab Chip, 2024,
24, 2039-2048
Lab on skin: real-time metabolite monitoring with polyphenol film based subdermal wearable patches†
Received
24th January 2024
, Accepted 22nd February 2024
First published on 22nd February 2024
Abstract
The advent of digital technologies has spurred the development of wearable sensing devices marking a significant shift in obtaining real-time physiological information. The principal objective is to transition from blood-centric monitoring to minimally invasive modalities, which will enable movement from specialised settings to more accessible environments such as the practices of general practitioners or even home settings. While subcutaneously implanted continuous monitoring devices have demonstrated this transition, detection of analytes from sample matrices like skin interstitial fluid (ISF), is a frontier that offers attractive minimally invasive routes for detection of biomarkers. This manuscript presents a comprehensive overview of our work in subdermal wearable biosensing patches for the simultaneous monitoring of glucose and lactate from ISF in ambulatory conditions. The performance of the subdermal wearable glucose monitoring patch was evaluated over a duration of three days, which is the longest reported duration reported till date. The subdermal wearable lactate sensing patch was worn for the duration of the exercise. Our findings highlight a critical observation that biofouling effects become apparent after a 24 h period. The data presented in this manuscript extends on the knowledge in the areas of continuous metabolite monitoring by introducing multifunctional polyphenol polymer films that can be used for both glucose and lactate monitoring with appropriate modifications. This study underscores the potential of subdermal wearable patches as versatile tools for real-time metabolite monitoring, positioning them as valuable assets in the evolution of personalised healthcare in diverse settings.
1.0 Introduction
The landscape of wearable sensing devices represents a transformative paradigm in the personalised healthcare domain, driven by the profound impact of digital technologies.1–3 Also referred to as “lab under the skin”, these devices embody miniature laboratories engineered to furnish real-time insights into the physiology of the human body.4
Different theories pertaining to the presence of analytes in the skin introduce further challenges to this progressing field. Fig. 1A shows the microanatomy of the skin showing the different layers. The two-compartment theory, posits that metabolites within the skin interstitial fluid (ISF) undergo equilibration with the sub vascular plexus, thereby influencing the local composition of analytes.5–7
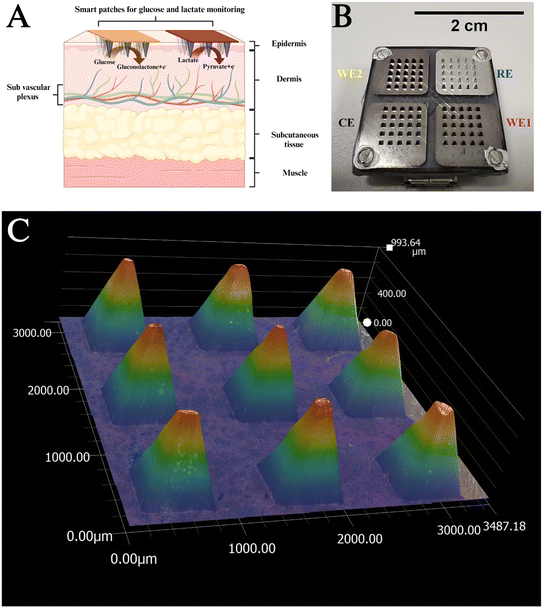 |
| Fig. 1 (A) Showing microanatomy of skin (created with BioRender, license number PY26AQ25FL). (B) Photograph of a patch used for physiological monitoring. (C) Images obtained using a Keyence high resolution microscope showing the morphology of the patch (each MN is 1 mm in height, 0.6 mmm base, the pitch distance between the tips is 0.6 mm). | |
In delineating the categories of wearable devices observed over the last decade, epidermal wearable sensors stand out for their utilisation of sweat as the primary sample matrix.8,9 These devices are characterised by their simplicity and adopt conformal designs that encase the skin, often incorporating integrated microfluidic systems to facilitate single or multianalyte sensing.10–12 However, epidermal wearable sensors pose challenges such as complexities in sample transport, sample contamination, and dynamic nature of the matrix.13 In contrast, subdermal wearable sensing devices employ microneedle (MN) technology (Fig. 1A), which feature out-of-plane structures for the penetration of the skin's stratum corneum.14,15 These devices leverage either hollow MN to extract ISF for subsequent analysis or, alternatively, functionalise solid MN surfaces (Fig. 1B) into electrochemical devices for monitoring metabolites such as glucose,16–18 lactate,19,20 and ketones.21,22 However, hollow MNs involve challenges like extracting sufficient volume of ISF for sensing, sample handling, and necessity to adapt the sensing surface to the dynamic nature of the sample matrix. In contrast, solid MNs are simpler to fabricate and perform sensing in the subdermal space, which ensures continuous monitoring of biomarkers without the involvement of challenges like sample handling and contamination.23 Over the last few years, attempts have been made to modify the terminology to exclude “needles” as it has been identified that a larger number of people suffer from needle phobia.24 Hence, MN array (MNA) based biosensing devices (Fig. 1) are referred to as “subdermal wearable sensors” or “smart patches”.
Biofouling poses a significant challenge for wearable devices operating in the in situ environment (ISF), where constant exposure to biological fluids and tissues increases the risk of organic material accumulation on device surfaces.25 This accumulation can lead to various adverse effects, including sensor performance degradation, biocompatibility concerns, infection risks, and aesthetic issues. Surface modification techniques such as anti-fouling coatings or textures can help minimize the adhesion of biological substances, while careful material selection can enhance resistance to biofouling.26,27 The use of patches that operate in the subdermal compartment come into direct contact with biological fluids during skin penetration during prolonged wear. This prolonged wear and presence of microbes on the skin surface cause a buildup of biological substances on the patch surfaces. To prevent such a build-up, antibiofouling coating are applied to the surface of MNAs. These biofouling coatings are often composed on hydrophilic polymers like polyphenols, which prevent the adhesion of biological substances in addition to preserving the functionality of these surfaces.28
Polyphenols can be incorporated into coatings through various methods, such as chemical modification, electropolymerisation or blending with other polymers. These coatings create a surface that resists the attachment of proteins, cells, and bacteria, thereby reducing biofouling.29 The mechanism behind the anti-biofouling activity of polyphenols involves their ability to interact with biological molecules, forming a protective barrier that inhibits adhesion. Moreover, polyphenols have been shown to exhibit biocompatibility with human tissues, making them suitable for applications involving prolonged contact with the body.30 By leveraging the natural properties of polyphenols, we aim to develop biofouling-resistant coatings that not only enhance the performance and longevity of wearable devices but also minimize the risk of adverse reactions or infections in users.
Electropolymerisation is a ‘one step electrochemical approach’, which involves the immobilization of a bioreceptor like enzymes inside a polymer matrix.31 This method facilitates conformal immobilisation of enzymes on 3D electrodes possessing a complex architecture.32 In this manuscript, the electropolymerisation of phenol has been utilized for enzyme immobilization because of advantages like superior diffusion dynamics, which results in decreased response time of the sensor.33
The natural presence of polyphenols in plant-derived foods endows them with inherent biocompatibility. Furthermore, polyphenols are recognized for their potential antioxidant and anti-inflammatory properties. Leveraging these advantageous traits, polyphenols emerge as promising biocompatible agents.34 Incorporating polyphenols into sensor technology is anticipated to mitigate inflammatory responses and inhibit the attachment of biofouling proteins onto sensor surfaces. Consequently, this preservation of biosensor functionality leads to enhanced sensor stability and performance.
This manuscript presents our preliminary findings on real-time monitoring through the deployment of subdermal sensors comprised of stable conformallt electropolymerised polyphenol films, as immobilsation matrices for glucose oxidase (GOx) and lactate oxidase (LOx), which are specifically engineered for monitoring of glucose and lactate levels over longer periods (>3 days) in ambulatory conditions. These preliminary studies are done in preparation of longer term (>3 days) human studies. These patches were worn by a participant and were used for the in vivo monitoring of glucose and lactate. The performance of these patches was found to be comparable with commercially available continuous glucose monitoring device (CGM; Free Style Libre 3) and capillary blood glucose levels, indicating the ability of these patches in capturing real-time physiological events. This manuscript employs the same electrochemical polymerisation of phenol for the immobilization of GOx and LOx and hence has the potential for in vivo detection of these analytes using the subdermal wearable patch.
2.0 Experimental section
2.1 Materials
Glucose oxidase (GOx), lactate oxidase (LOx), calcium chloride, glucose, HEPES buffer, potassium chloride, magnesium sulphate, sodium chloride, sodium dihydrogen phosphate, sucrose, bovine serum albumin, phenol, and phosphate buffer saline (PBS) tablets were purchased from Sigma Aldrich. Electrical wires and electrical tape were obtained from RS Components. Insulating varnish and Free Style Libre 3 CGM was purchased from Amazon. Platinum screen printed electrodes (Pt SPE) were procured from Metrohm. Deionised (DI) water having a resistivity of 18.2 MΩ was used for all experiments.
Artificial ISF was prepared by mixing 2.5 mM calcium chloride, 5.5 mM glucose, 10 mM HEPES buffer, 3.5 mM KCl, 0.7 mM magnesium sulphate, 123 mM sodium chloride, 1.5 mM sodium dihydrogen phosphate, 7.4 mM sucrose, 20 mg mL−1 bovine serum albumin in DI water.
2.2 Subdermal patch fabrication
Poly(carbonate) patches were fabricated at the University of Glasgow using injection molding. Each array in the patch is 20 mm wide, consists of square-based pyramids 1 mm in height and 0.5 × 0.5 mm at the base. The base of each pyramid is 0.5 mm away from its neighbouring structure. The calculated active surface area of each pyramid is therefore 1.03 mm2. Each electrode (working (WE1 and WE2), counter (CE), and reference (RE)) has 25 (5 × 5) pyramids, and therefore, the total active surface area of each electrode is calculated at 25.75 mm2. In vivo, the true area in contact with the ISF is lower and less defined as the needles do not fully penetrate to their bases.35 This has been established in an earlier study through OCT studies done in humans using the MN with similar design.18,20
The poly(carbonate) arrays were metallised at Torr Scientific (Bexhill, UK). Four separate areas of the patch were first sputter-coated with an initial seed layer of titanium (110 nm). Following this, three sections (forming the WE and CE) were coated with 150 nm of Pt by e-beam evaporation, and the final section (forming the RE) was coated with 150 nm of Ag by e-beam evaporation.
For in vitro trials, the patches were assembled on a printed circuit board (PCB), as seen in Fig. 1B. The space between the polycarbonate MNA structure and the PCB was insulated and made watertight using a parafilm piece to avoid sweat and other external fluids flowing onto the PCB circuit and potentially causing a short circuit. Insulating varnish was applied along the edges to ensure the 200 μL of solution employed for in vitro testing was limited within the boundaries of the active area of the MNA.
2.3 Biosensor fabrication
2.3.1. Functionalisation of SPE and MNs.
The Pt SPEs were modified with GOx and LOx to monitor glucose and lactate, respectively, by drop coating 70 μL of a mixture of an electropolymerisation solution of phenol and enzyme in PBS. The functionalisation solutions comprised of 10 mg mL−1 of GOx or LOx dissolved in 50 mM phenol solution. The electropolymerised films are expected to be 50 nm with the enzymes entrapped in them. The electropolymerisation was performed by employing multiple cycles of chronoamperometry (CA). Each cycle involved applying 0 V for 20 s and then applying a voltage of 0.9 V for 900 s against the integrated Ag/AgCl RE.
Before functionalisation of the subdermal patches, they were cleaned with 70% ethanol and dried with a stream of compressed air. The Ag electrode after modification with sodium hypochlorite to Ag/AgCl (25 μL drop cast onto the Ag electrode for 30 s and then washed with deionised water) would act as the RE. Functionalisation on two designated Pt WE of each array, was carried out using the same electropolymerisation as that used for the SPEs. A third Pt electrode would act as the CE. The electropolymerisation was carried out employing the same protocol as the SPEs. On completion of the electropolymerisation, equilibration was done employing CA at 0.7 V for 60 min. This step helps in ensuring that any unreacted phenol is removed.
2.4 Dose response curves
The dose response curves for glucose were carried out using Pt SPEs and were obtained by introducing 70 μL concentrations of glucose ranging from 0.5 mM to 30 mM onto the PT SPEs. The reported current for each concentration of glucose was measured in triplicates and the final value for dose response curve taken as an average value of the currents observed at the 30th second after each addition (with current measured every 0.1 second). The dose response curves were fitted with Michaelis Menten fittings to calculate the apparent Km values.
2.5 Glucose, lactate and biofouling monitoring in vivo
Human studies were performed in triplicate on a healthy participant, in preparation for a larger human pilot study. The objectives were to investigate the ability of the sensor to monitor events related to food intake and activity and observe any biofouling effects of wearing it over longer period. A healthy volunteer wore freshly prepared glucose and lactate sensors on the left and right hands, respectively. Elasticated bandages were employed to hold the devices in place while they were worn. The devices were left in situ for over 3 days and the performance of these devices was evaluated using capillary blood glucose using a Biosen C-Line Glucose and lactate analyser as the primary standard and a subcutaneously implanted commercially available continuous glucose monitoring sensor (Abbott Freestyle Libre 3) as a secondary standard. All these methods were carried out when the participant was involved in daily activities including working in the office space and walking to different locations in the workplace. The post prandial peaks produced by these devices was compared to derive information regarding biofouling occurring in the skin compartment. For the continuous monitoring lactate sensors, the performance of the wearable subdermal devices was compared against capillary blood lactate measurements, which were carried out using a Biosen C-Line Glucose and lactate analyser. The lactate measurements were done continuously over a period of 1 h. The lactate tests were carried out while the participant was engaged in a low intensity exercise on a H/P/Cosmos Quasar tread mill. The tread mill was set at a speed of 5.2 km h−1 and an elevation of 1% for a period of 1 h. The pulmonary gas exchange was measured throughout the lactate measurement period using a breath-by-breath Jaeger Vyntus CPX (Hoechberg, Germany) gas analyser. The ambient temperature in both these cases was in the range of 16–20 °C.
A major limitation with continuous lactate monitoring is the lack of a secondary standard such as a commercially available continuous monitoring device that we could employ in the case of glucose.
2.6 Evaluation of clinical accuracy of subdermal wearable glucose sensors using Clarke error grid analysis
The subdermal wearable patches measured current over time (CA) and were used as an indicator of events related to food intake and physical exercise. We expected to see a time lag between the event captured by the subdermal wearable sensor and that seen with capillary blood.36 The current output from the subdermal wearable sensors were calibrated against capillary blood glucose values. The calibration was performed by matching the highest value of current and the highest value of capillary blood glucose after removing the lag. The assessment of the subdermal wearable sensing device values in comparison to capillary blood reference blood glucose values and was conducted through Clarke error grid (CEG) analysis, employing the MatLab routine for CEG analysis.37–39 The concurrent representation of the current values derived from CA measurements was juxtaposed with capillary blood or the commercially available CGM readings. Instances where sensors displayed a complete loss of function during post-clinical study tests resulted in the exclusion of corresponding data from the analysis. Similarly, data from studies experiencing technical issues such as subdermal wearable sensor warm up time and potentiostat issues, were omitted from the analysis. The lactate measurements were also carried out followed by comparing obtained lactate values with values obtained from blood.
2.7 Calculation of the mean absolute relative difference (MARD) for subdermal wearable glucose sensors
The mean absolute relative difference (MARD) for the subdermal wearable sensors was characterised as the average percentage disparity between the microneedle sensor's glucose estimate and the reference capillary blood glucose.
It was calculated as follows:
|
MARD = ∑(YCGMi − YRBGi)/(YRBGi) × 100/n | (1) |
where
YRBGi is the
ith reference capillary glucose value,
YCGMi is the corresponding subdermal wearable sensor measured value at identical time and
n is the total number of reference measurements.
Glucose levels in healthy individuals in steady state condition range from 4–6 mM. Postprandial peaks range up to 9 mM in health participants. Lactate levels in healthy individuals at rest are approximately 1–2 mM in blood and ISF.40,41 The lactate sensors employed for intense anaerobic exercise where the levels may rise to 15 mM or higher; require the biosensor designed ideally for a linear range from 1 to 20 mM.42,43 The protocols approved for this study involved light exercises so we employed lactate sensors comprising of polyphenol films as reported earlier.20
3.0 Results and discussion
3.1 Surface characterisation of SPE and MN electrodes
Functionalised SPEs were characterised using scanning electron microscopy (Zeiss EVO LS25 SEM). SEM images of the bare (Fig. S1A†) and GOx/LOx functionalised SPEs are presented in Fig. S1B and C.† There are variations in surface morphology between the bare and functionalised surfaces. The highly porous Pt surface exhibits fibrous components dispersed across the Pt substrate. The GOx and LOx surfaces exhibit elongated fibrous structures with varying lengths and diameters. The electropolymerized GOx reveals a distinctive surface characterized by a homogeneous and conformal coating and the fibrous structures associated with the LOx modified electrode displays a sharper three-dimensional morphology. Fig. 1B shows the photograph of a patch having dimensions of 2 cm× 2 cm (scale bar of 2 cm top right). Fig. 1C and S2A and B† shows optical microscopy images of the patches, which reveal pyramidal morphology with approximately ∼1000 μm and base dimensions of 500 μm (length and width). The spacing between the pyramids in the patch is approximately 600 μm, which makes these patches suited for continuous monitoring applications. Additionally, the dimensions of tips of these patches being in the range of 40–60 μm also ensures efficient and painless penetration of the stratus corneum.44
3.2 Sensor response in vitro
In vitro studies were carried out for glucose sensing on Pt SPEs modified with GOx immobilized on polyphenol films. The sensors showed good response when tested for different concentrations of glucose from 0 mM to 30 mM. The baseline current (I0) was found to be 0.0635 μA and this current was subtracted from concentration dependent current (Imax) to obtain the absolute current values (Fig. 2A). A dose response curve was also plotted, and it was found that the sensor followed Michaelis–Menten equation as was expected due to the response originating from enzymatic oxidation of GOx. The apparent Km values obtained for this sensor was 6.84 ± 0.50243 mM, which was in good agreement with earlier reports.18 This indicates the capability of the sensor to effectively measure glucose concentrations up to approximately 14 mM. Furthermore, Bantle et al. reported a 10% lower glucose concentration in interstitial fluid (ISF) compared to blood glucose levels.45 This observation underscores the suitability of the polyphenol immobilized enzymes for glucose concentration monitoring in ISF. Due to the promising results obtained on Pt SPEs, these modifications were extended to the patches, and they were employed for in vivo studies. Before being employed for in vivo studies, we also verified the performance of the modified patches for glucose detection (Fig. S3†). It was observed that these patches were able to detect glucose in similar ranges as that of the modified Pt SPEs, which was in corroboration with existing reports.20
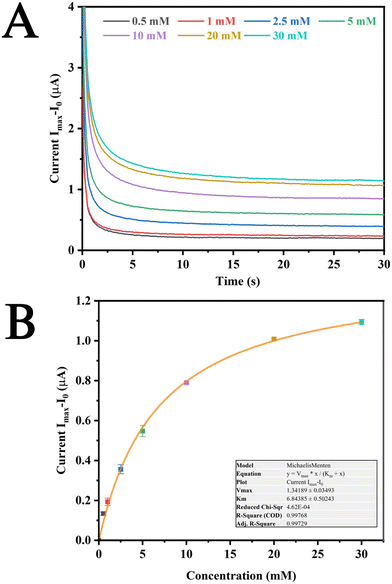 |
| Fig. 2
In vitro studies: (A) chronoamperometric data obtained at a potential of 0.7 V for GOx modified Pt SPEs showing increase in baseline corrected currents and (B) dose response curves obtained for Pt SPEs from 0.5 mM to 30 mM additions of glucose (in 0.1 M PBS pH 7.4). | |
The influence of biofouling on the Pt SPEs were also investigated by artificial ISF containing bovine serum albumin (Fig. S4†). It could be observed that the Pt SPEs were able to sense concentrations ranging from 0 to 30 mM. However, due to the buildup of insulating proteins on the surface of the SPEs, the current responses were observed to be lowest on the 3rd day (—) in comparison to a fresh electrode (—) despite which these sensors were able to measure glucose concentrations successfully.
3.3 Sensor response in vivo
For in vivo studies, a smart patch functionalised with either GOx or LOx, a PCB for electrical contact with the patch, and a connecting wire as shown in Fig. 3A were used. These components were connected to a potentiostat controlled using software on the tab (PS Touch Ver 5.9) (Fig. 3B). In vivo, the patches may not fully be in contact with the ISF as the entire structure does not penetrate the stratum corneum, which reduces the active surface area of the electrodes to the tips of the electrodes. It is important in this case that the surface area in contact with the ISF remains constant. This means that the patch must be held securely using an elasticated strap in the case of in vivo studies (Fig. 3C). Post in vivo studies, the patches were removed, and the participant's skin exhibited marks corresponding to the patch being pressed on the skin. However, these marks were found to resolve within a duration of 24 h, which implied that these patches did not cause permanent scarring of the skin surface (Fig. 3D–F). This was in agreement with an earlier study conducted on participants for the monitoring of antibiotic drugs.46 This implied that the subdermal sensing devices were very well tolerated during the entire duration of the study. The study protocol was reviewed and given favourable opinion by the MOD Research Ethics Committee (MODREC). The study was sponsored by Swansea University and conducted at the ASTEM and Engineering Laboratories based at Bay Campus.
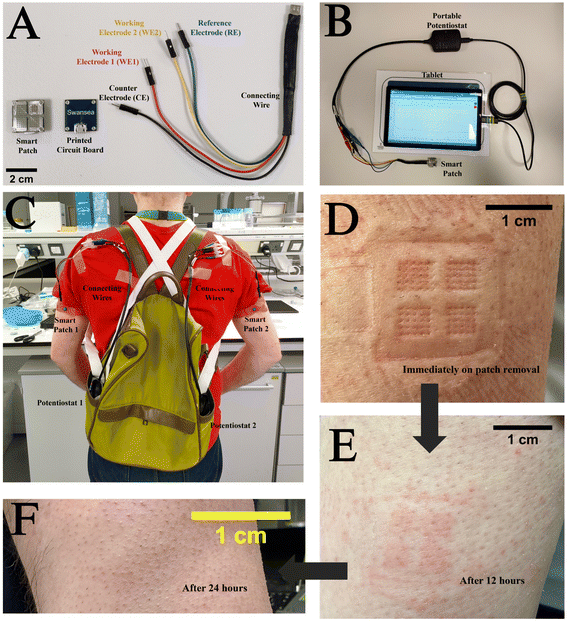 |
| Fig. 3 Setup for in vivo studies: (A) shows a metallised patch, a PCB on which it is assembled and wired with mini-USB connector. (B) Shows a commercially available portable potentiostat and a tablet to observe patch response. (C) Participant wearing a MN on the forearms for physiological monitoring. Marks on the skin (D) immediately after removal of the patches, (E) 12 hours are patch removal, and (F) 24 hours after patch removal. | |
Fig. 4A shows the results of in vivo studies performed employing the subdermal wearable sensing device; the sensor response (data point obtained every 1 s) is plotted as a blue line (—) incorporating a second order Savitzky–Golay filter.19 A current peak coincidence with CGM (—) and capillary glucose values (●) was observed at 14:00, which was comparable to reports by Sharma et al., Ribet et al., and Liu et al. who performed these studies in ambulatory conditions, stationary human forearm and diabetic mice.16,47,48 (Fig. 4A). It was reported by Basu et al. that a delay of <10 min between CGM and vascular space would be crucial for the development of CGM devices.49 This observation underscores the potential of the sensor for commercial application, given its alignment with established benchmarks and critical performance criteria.
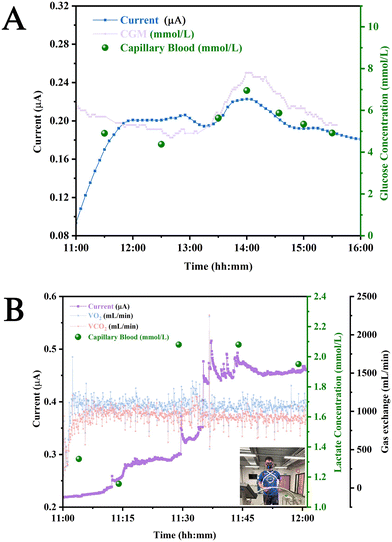 |
| Fig. 4
In vivo studies: (A) current produced in vivo by the GOx patch pre (—) and post lag (—) correction in comparison to glucose concentrations reported from CGM device (—) and capillary blood (●) for different times of the day. (B) Current produced by the LOx patch (—) plotted with VCO2 (—) and VO2 (—) during physical exercise (replace Fig. 4A with something that does not exaggerate the lag). | |
The monitoring of lactate was also carried out using patches functionalised with LOx. These patches were worn by the volunteer before the initiation of a physical exercise (inset: Fig. 4B). It can be observed that the currents produced by the patch (—) increase during the course of the physical exercise due to glycolysis, which produces lactate.50 This peak can be observed to onset in the time interval between 11:30 and 11:45. It can also be seen that there is a slight variation in the levels of VO2 (—) and VCO2 (from 11:30 to 11:35) (—), which could be attributed to the threshold that occurs when CO2 production (VCO2) and ventilation increase. This increase occurs due to gas exchange as a result of metabolic acidosis occurring from lactate accumulation.51
Biofouling is a process, which is caused by the adhesion of noxious films on the surface of a devices.52 Since the glucose monitoring smart patches were worn by the participant continuously for 3 days, a decrease in magnitude of post prandial peaks was observed, which could be attributed to the adhesion of albumin and fibrinogen on the active areas of these patches.53Fig. 5A shows the data produced by the patches in the prandial period. The decrease in the currents obtained in response to the consumption of the same food (quantity and quality) measured over three subsequent days were observed (D1, D2, and D3). A bar chart has been plotted between the postprandial peak currents and the day number (Fig. 5B).
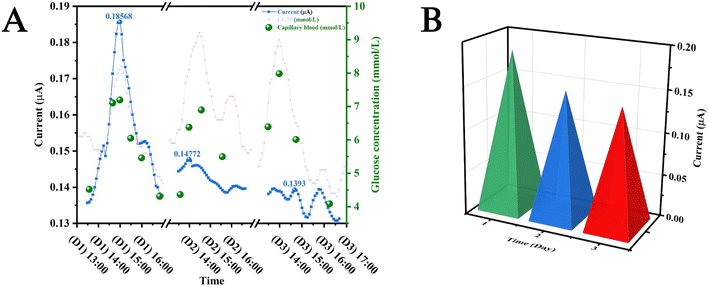 |
| Fig. 5
In vivo biofouling studies: (A) data obtained by the MNAs in prandial period for day 1, 2, and 3 (D1, D2, and D3) showing a decrease in current values. (B) Bar charts of the post prandial peaks plotted as a function of number of days. | |
The current generated by patches is directly impacted by the accumulation of biofouling on their surfaces. Consequently, this accumulation significantly influences the postprandial peaks observed in the generated current. The current was observed to be 0.18 μA on the first day, whereas it was observed to reduce to approximately 0.14 μA on the second day. This decreasing trend was found to stabilize on the third day where the current reduced to approximately 0.13 μA. However, more detailed studies are needed to quantify this decrease and study detailed mechanisms behind this decrease.
Fig. 6 shows the Clark error grid (CEG) plotted for the performance of the subdermal patches versus CGM devices (Fig. 6A) and capillary blood (Fig. 6B). For the former, 350 data points were taken into consideration whereas for the latter, 43 data points were compared. In both cases all the data points fell in zone A and B. The fewer points in CEG comparing the patches to capillary blood are due to low frequency of capillary blood sampling (every half hour to record postprandial events and every hour during the rest of the study). Approximately 65% data points could be categorised in zone A and 35% data points fell into zone B for Fig. 6A. For Fig. 6B 74.4% were found to be in zone A and 25.6% data points were found to be in zone B. This distribution implied that the patches were in good agreement with both CGM and capillary blood values and hence are clinically acceptable. The MARD value obtained from comparing subdermal devices versus commercially available CGM was 16.6%, whereas when compared to capillary blood was 13.8% (Fig. 6B). Interestingly, the MARD value obtained from comparing the commercially available CGM versus capillary blood was 15.4%. This was investigated by analysing the agreement between CGM glucose measurements and capillary blood glucose measurements. It was observed that 100% of the data points in this case could be categorised in sectors A and B, which indicated that this comparison was clinically acceptable (Fig. S5†).
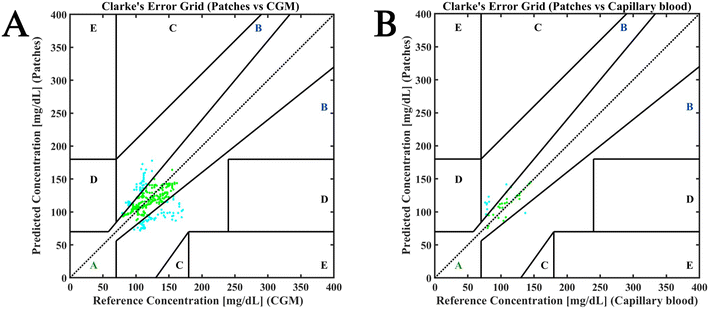 |
| Fig. 6 CEG plots: (A) shows the predicted concentration from the patches in comparison to reference concentrations reported by the CGM device. (B) Shows the predicted concentration from the patches in comparison to reference concentrations from capillary blood measurements. | |
The gold standard for blood glucose measurement is venous blood. The comparisons made in this manuscript suggest that the performance of our subdermal wearable sensors is comparable to that of self-monitoring of blood glucose measurements on capillary blood and the commercially available CGMs. In the case of the subdermal wearable lactate sensors, the exercise events were captured by the sensor. However, due to low number of capillary blood measurements, we could not perform a Bland–Altman plot.
A source of variability between the sensors observed during the trials was due to initial application and mechanical stability of the device on the skin. The subdermal wearable sensor must be reliable and provide continuous measurements and be secured using an elasticated strap for effective measurements to be obtained. Product development of an applicator for the subdermal wearable sensing device should take this into account when considering the method of skin attachment, to standardise the dermal penetration of the patch. Additionally, other modes of enzyme immobilization like hydrogels may be used for producing biofouling resistant enzyme films, which could lead to better performance of the devices in vivo. This pilot study was conducted as a prelude to a large-scale human study involving smart patches and the larger study will aim to monitor different analytes on the same patch.
4.0 Conclusions and future perspective
A glucose and lactate-sensing subdermal wearable patch prototype has been fabricated and shown to be functional in human volunteers at a level comparable to a commercially available method. Electropolymerised films were employed for functionalising the subdermal wearable biosensors for entrapping the enzymes. It was found that these devices were able to monitor glucose and lactate levels in humans showing peaks corresponding to meal intake and initiation of physical exercise. Additionally, the data points produced by the glucose monitoring devices were positioned in sectors A and B of a CEG, which implied their good agreement with CGM and capillary blood values. The peaks produced by the glucose monitoring patches were found to decrease in magnitude while reporting post prandial peaks even after being left on the skin continuously for 3 days. This decrease could be attributed to biofouling in devices inserted into the skin compartment. Variability between batches of devices is a concern but will be improved with the process of fabrication being fully automated and quality management systems being implemented. The device in its current form requires a PCB and performed well. The report highlights the novelty of subjecting the devices to a 72-hour wear period, surpassing previous literature. During this extended duration, glucose measurements were consistently recorded, showing correlation with blood glucose levels. This correlation is attributed to the anti-biofouling properties of the polyphenol film used for enzyme immobilization. Additionally, performance of the smart patch was evaluated against blood glucose concentrations, leveraging the established correlation between blood and interstitial fluid (ISF) glucose levels for potential effectiveness.
Miniaturization of the electronics and the incorporation of a battery and Bluetooth (or other wireless) connectivity components will result in devices that are easier to use. The hardware enabling such approaches is already available commercially and could be integrated to be used for smart patch-based sensing. Through easy-to-apply and easy-to-interpret continuous monitoring of glucose and lactate levels, people will be empowered to monitor their well-being and physical training activity and personalise their dietary habits and training regimes. These subdermal patches can also be employed for military use for monitoring the holistic health and well-being of soldiers in combat conditions.
In clinical scenarios, such as surgery, where metabolite levels are already under observation, a cost-effective, minimally invasive, and continuous monitoring device presents substantial benefits over laboratory testing. Minimising the need for blood sampling also decreases associated risks and is especially valuable when treating babies and children due to the discomfort associated with constant drawing of blood. A convenient and accurate subdermal wearable patch provides excellent benefits in these scenarios.
Research ethics approval
Research ethics approval for the human pilot studies was obtained from the Ministry of Defence Research Ethics committee (MODREC), Application No: 2257/MODREC/23.
Conflicts of interest
The authors declare no conflict of interest.
Acknowledgements
G. V., G. L., and S. O. contributed equally to this work. This is a summary of independent research partly funded by DASA/DSTL grant on “Simultaneous monitoring of glucose and lactate using wearable sensors in ambulatory conditions (DSTL 0000010329)”. The views expressed are those of the author(s) and not necessarily those of DASA/DSTL. G. V. would like to acknowledge M2A for her DEng studentship. Contributions by Professor Nikolaaj Gadegaard, Glasgow University, Professor Tony Cass, Dr David Freeman and Mr James McLeod, Imperial College London, towards device fabrication and bioinstrumentation is greatly appreciated. We also acknowledge Dr Benjamin Bryant, Keyence for his help with high resolution images of the microneedle and screen-printed electrodes. We acknowledge Dr. Mark Coleman for his help with obtaining SEM images.
References
- H. C. Ates, P. Q. Nguyen, L. Gonzalez-Macia, E. Morales-Narváez, F. Güder, J. J. Collins and C. Dincer, Nat. Rev. Mater., 2022, 7, 887–907 CrossRef PubMed.
- J. Tu, R. M. Torrente-Rodríguez, M. Wang and W. Gao, Adv. Funct. Mater., 2020, 30, 1906713 CrossRef CAS.
- C. Xu, S. A. Solomon and W. Gao, Nat. Mach. Intell., 2023, 5, 1344–1355 CrossRef PubMed.
- H. Teymourian, F. Tehrani, K. Mahato and J. Wang, Adv. Healthcare Mater., 2021, 10, 2002255 CrossRef CAS PubMed.
- K. D. McCarley and A. L. Bunge, J. Pharm. Sci., 2000, 89, 1212–1235 CrossRef CAS PubMed.
- E. Cengiz and W. V. Tamborlane, Diabetes Technol. Ther., 2009, 11, S-11–S-16 CrossRef PubMed.
- M. Friedel, I. A. P. Thompson, G. Kasting, R. Polsky, D. Cunningham, H. T. Soh and J. Heikenfeld, Nat. Biomed. Eng., 2023, 7, 1541–1555 CrossRef PubMed.
- J. R. Sempionatto, J. A. Lasalde-Ramírez, K. Mahato, J. Wang and W. Gao, Nat. Rev. Chem., 2022, 6, 899–915 CrossRef PubMed.
- T. Saha, R. Del Caño, E. De la Paz, S. S. Sandhu and J. Wang, Small, 2023, 19, 2206064 CrossRef CAS PubMed.
- L. Lyzwinski, M. Elgendi, A. V. Shokurov, T. J. Cuthbert, C. Ahmadizadeh and C. Menon, Comms. Eng., 2023, 2, 48 CrossRef.
- J. Min, J. Tu, C. Xu, H. Lukas, S. Shin, Y. Yang, S. A. Solomon, D. Mukasa and W. Gao, Chem. Rev., 2023, 123, 5049–5138 CrossRef CAS PubMed.
- M. Ankitha, A. M. Arjun, N. Shabana and P. A. Rasheed, Biomedical Materials & Devices, 2023, 1, 339–350 Search PubMed.
- R. Ghaffari, J. A. Rogers and T. R. Ray, Sens. Actuators, B, 2021, 332, 129447 CrossRef CAS PubMed.
- H. Sun, Y. Zheng, G. Shi, H. Haick and M. Zhang, Small, 2023, 2207539 CrossRef CAS PubMed.
- O. Howells, N. Rajendran, S. McIntyre, S. Amini-Asl, P. Henri, Y. Liu, O. Guy, A. E. G. Cass, M. C. Morris and S. Sharma, ChemBioChem, 2019, 20, 2198–2202 CrossRef CAS PubMed.
- S. Sharma, A. El-Laboudi, M. Reddy, N. Jugnee, S. Sivasubramaniyam, M. El Sharkawy, P. Georgiou, D. Johnston, N. Oliver and A. E. G. Cass, Anal. Methods, 2018, 10, 2088–2095 RSC.
- M. Parrilla, U. Detamornrat, J. Domínguez-Robles, R. F. Donnelly and K. De Wael, Talanta, 2022, 249, 123695 CrossRef CAS PubMed.
- S. Sharma, Z. Huang, M. Rogers, M. Boutelle and A. E. G. Cass, Anal. Bioanal. Chem., 2016, 408, 8427–8435 CrossRef CAS PubMed.
- D. M. E. Freeman, D. K. Ming, R. Wilson, P. L. Herzog, C. Schulz, A. K. G. Felice, Y.-C. Chen, D. O'Hare, A. H. Holmes and A. E. G. Cass, ACS Sens., 2023, 8, 1639–1647 CrossRef CAS PubMed.
- S. Sharma, A. Saeed, C. Johnson, N. Gadegaard and A. E. G. Cass, Sens. Bio-Sens. Res., 2017, 13, 104–108 CrossRef PubMed.
- R. Del Caño, T. Saha, C. Moonla, E. De la Paz and J. Wang, TrAC, Trends Anal. Chem., 2023, 159, 116938 CrossRef.
- J. Wang, Z. Lu, R. Cai, H. Zheng, J. Yu, Y. Zhang and Z. Gu, Lab Chip, 2023, 23, 869–887 RSC.
- N. Tariq, M. W. Ashraf and S. Tayyaba, J. Pharm. Innov., 2022, 17, 1464–1483 CrossRef.
- S.-Y. Jeong, J.-H. Park, Y.-S. Lee, Y.-S. Kim, J.-Y. Park and S.-Y. Kim, Pharmaceutics, 2020, 12 Search PubMed.
- N. Wisniewski and M. Reichert, Colloids Surf., B, 2000, 18, 197–219 CrossRef CAS PubMed.
- V. Ruiz-Valdepeñas Montiel, J. R. Sempionatto, B. Esteban-Fernández de Ávila, A. Whitworth, S. Campuzano, J. M. Pingarrón and J. Wang, J. Am. Chem. Soc., 2018, 140, 14050–14053 CrossRef PubMed.
- M. J. Russo, M. Han, P. E. Desroches, C. S. Manasa, J. Dennaoui, A. F. Quigley, R. M. I. Kapsa, S. E. Moulton, R. M. Guijt, G. W. Greene and S. M. Silva, ACS Sens., 2021, 6, 1482–1507 CrossRef CAS PubMed.
- P.-H. Lin and B.-R. Li, Analyst, 2020, 145, 1110–1120 RSC.
- R. Zhang, M. He, D. Gao, Y. Liu, M. Wu, Z. Jiao, Y. Su and Z. Jiang, J. Membr. Sci., 2018, 566, 258–267 CrossRef CAS.
- J. Hwan Shin, C.-H. Choi, S. Wang and J. Pil Park, Appl. Surf. Sci., 2024, 652, 159302 CrossRef CAS.
- F. Shen, S. Arshi, E. Magner, J. Ulstrup and X. Xiao, Synth. Met., 2022, 291, 117205 CrossRef CAS.
- X. Chen, N. Matsumoto, Y. Hu and G. S. Wilson, Anal. Chem., 2002, 74, 368–372 CrossRef CAS PubMed.
- G. Ziyatdinova, E. Guss and E. Yakupova, Sensors, 2021, 21(24), 8385 CrossRef CAS PubMed.
- J. Han, J. Hong, H. Lee, S. Choi, K. Shin, M. Gu and S.-H. Kim, Eur. Polym. J., 2023, 197, 112354 CrossRef CAS.
- S. A. Coulman, J. C. Birchall, A. Alex, M. Pearton, B. Hofer, C. O'Mahony, W. Drexler and B. Považay, Pharm. Res., 2011, 28, 66–81 CrossRef CAS PubMed.
- D. P. Zaharieva, K. Turksoy, S. M. McGaugh, R. Pooni, T. Vienneau, T. Ly and M. C. Riddell, Diabetes Technol. Ther., 2019, 21, 313–321 CrossRef CAS PubMed.
- W. L. Clarke, D. Cox, L. A. Gonder-Frederick, W. Carter and S. L. Pohl, Diabetes Care, 1987, 10, 622–628 CrossRef CAS PubMed.
- D. Stöckl, K. Dewitte, C. Fierens and L. M. Thienpont, Diabetes Care, 2000, 23, 1711–1712 CrossRef PubMed.
- B. W. Bequette, J. Diabetes Sci. Technol., 2010, 4, 404–418 CrossRef PubMed.
- X. Liu and J. Liu, View, 2021, 2, 20200102 CrossRef.
- R. Kano and K. Sato, J. Funct. Morphol. Kinesiol., 2021, 6(4), 95 CrossRef PubMed.
- M. L. Goodwin, J. E. Harris, A. Hernández and L. B. Gladden, J. Diabetes Sci. Technol., 2007, 1, 558–569 CrossRef PubMed.
- V. Vucetic, M. Mozek and M. Rakovac, J. Strength Cond. Res., 2015, 29, 1057–1063 CrossRef PubMed.
- R. F. Donnelly, M. J. Garland, D. I. J. Morrow, K. Migalska, T. R. R. Singh, R. Majithiya and A. D. Woolfson, J. Controlled Release, 2010, 147, 333–341 CrossRef CAS PubMed.
- J. P. Bantle and W. Thomas, J. Lab. Clin. Med., 1997, 130, 436–441 CrossRef CAS PubMed.
- T. M. Rawson, S. A. N. Gowers, D. M. E. Freeman, R. C. Wilson, S. Sharma, M. Gilchrist, A. MacGowan, A. Lovering, M. Bayliss, M. Kyriakides, P. Georgiou, A. E. G. Cass, D. O'Hare and A. H. Holmes, Lancet Digital Health, 2019, 1, e335–e343 CrossRef PubMed.
- F. Ribet, G. Stemme and N. Roxhed, Biomed. Microdevices, 2018, 20, 101 CrossRef PubMed.
- Y. Liu, Q. Yu, X. Luo, L. Yang and Y. Cui, Microsyst. Nanoeng., 2021, 7, 75 CrossRef PubMed.
- A. Basu, S. Dube, M. Slama, I. Errazuriz, J. C. Amezcua, Y. C. Kudva, T. Peyser, R. E. Carter, C. Cobelli and R. Basu, Diabetes, 2013, 62, 4083–4087 CrossRef CAS PubMed.
- S. P. Cairns, Sports Med., 2006, 36, 279–291 CrossRef PubMed.
- W. Stringier, K. Wasserman and R. Casaburi, Eur. J. Appl. Physiol. Occup. Physiol., 1995, 72, 25–31 CrossRef PubMed.
- J. L. Harding and M. M. Reynolds, Trends Biotechnol., 2014, 32, 140–146 CrossRef CAS PubMed.
- N. Arroyo-Currás, P. Dauphin-Ducharme, K. Scida and J. L. Chávez, Anal. Methods, 2020, 12, 1288–1310 RSC.
|
This journal is © The Royal Society of Chemistry 2024 |
Click here to see how this site uses Cookies. View our privacy policy here.