DOI:
10.1039/D3LC01012K
(Critical Review)
Lab Chip, 2024,
24, 1307-1326
High-throughput microfluidic systems accelerated by artificial intelligence for biomedical applications
Received
25th November 2023
, Accepted 10th January 2024
First published on 12th January 2024
Abstract
High-throughput microfluidic systems are widely used in biomedical fields for tasks like disease detection, drug testing, and material discovery. Despite the great advances in automation and throughput, the large amounts of data generated by the high-throughput microfluidic systems generally outpace the abilities of manual analysis. Recently, the convergence of microfluidic systems and artificial intelligence (AI) has been promising in solving the issue by significantly accelerating the process of data analysis as well as improving the capability of intelligent decision. This review offers a comprehensive introduction on AI methods and outlines the current advances of high-throughput microfluidic systems accelerated by AI, covering biomedical detection, drug screening, and automated system control and design. Furthermore, the challenges and opportunities in this field are critically discussed as well.
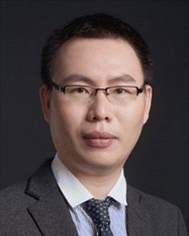 Jianhua Zhou | Jianhua Zhou is a full professor of Biomedical Engineering at Sun Yat-sen University, China. He received his Ph.D. from the Hong Kong University of Science and Technology in 2011. He was an American Fulbright visiting PhD student at Washington University in St. Louis in 2009. After a postdoc at Tohoku University, Japan, he was appointed associate professor of Engineering at Sun Yat-sen University and became a full professor in 2019. He was a visiting scholar at Harvard Medical School, US. His current research focuses on microfluidics, biosensors, rapid diagnosis, high-throughput technologies and precision medicine. |
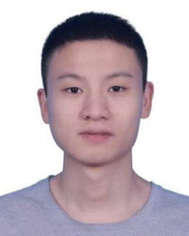 Jianpei Dong | Jianpei Dong received his Bachelor's degree in Mechanical Engineering from Zhengzhou University in China in 2020. He is currently pursuing a doctoral degree at the School of Biomedical Engineering, Sun Yat-sen University. His current research focuses on high-throughput technologies and 3D bioprinting. |
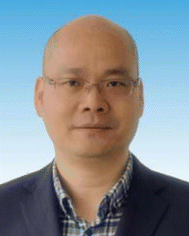 Hongwei Hou | Hongwei Hou is currently a principal investigator at Beijing Life Science Academy, China. He received his Ph.D. in 2005 from the University of Science and Technology of China. His current research focuses on high-throughput technologies in chemical biology. |
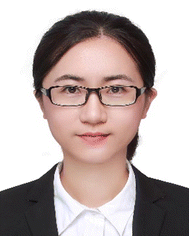 Lu Huang | Lu Huang received her B.Sc. from Tsinghua University in 2014 and obtained her Ph.D. from the Hong Kong University of Science and Technology in 2018. After conducting postdoctoral research at the University of Hong Kong, she is now an assistant professor at the School of Biomedical Engineering, Sun Yat-sen University, China. Her current research focuses on microfluidic technologies, single-cell analysis, high-throughput technologies and organ-on-chips. |
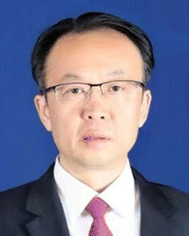 Jinghong Li | Jinghong Li is currently an Academician of Chinese Academy of Sciences and a Cheung Kong Professor at the Department of Chemistry, Tsinghua University, China. He is the Director of Academic Committee of Department of Chemistry, the Head of Analysis Center, Tsinghua University. His current research interests include electroanalytical chemistry and bioanalysis, nanoanalysis and biosensing, synthetic biology and chemical biology. |
1 Introduction
Microfluidics focuses on the manipulation and control of small amounts of fluids, typically in the microliter to picoliter range, within microscale channels or devices.1–3 In microfluidics, researchers use microfabrication techniques to create miniaturized devices known as “lab-on-a-chip” or “microfluidic chips”. These chips can perform specific fluidic tasks, such as mixing, separation, reaction, and analysis. The advantages of microfluidics lie in its ability to provide precise fluid control, reduced reagent consumption, and increased experimental throughput,4–7 making it ideal for biomedical experiments like biomolecular detection,8,9 cellular manipulation and analysis,10–12 drug screening,13,14 and biomaterials systhesis.15–17 And its high throughput enables the generation of large and detailed datasets. For instance, for a microfluidic platform with 1000 parallel cell culture units, automatic time-lapse fluorescence microscopy imaging will generate over 1 TB of image data (1 bright-field and 3 fluorescence images per unit, 72 time points, 5 MB per image).18 Analyzing such big data would be labor intensive, time consuming and error prone if not impossible, which seriously hampers the improvement of system throughput. Besides, some important hidden patterns and unexpected trends in data might be hard to discover by manual analysis. Apart from the challenges in data processing, many current platforms, such as organs-on-chips (OoCs), require persistent human inspection and manual adjustment to ensure the normal function, which greatly hinders the scalability of microfluidic systems. Therefore, while high-throughput microfluidics has proven to be an effective tool in biomedical research, it also presents challenges (particularly in large-scale data analysis and system automation) that must be addressed to fully utilize its potential.
As a data-driven technology, AI (first proposed by John McCarthy in 1956)19,20 aims to build smart machines to perform tasks that typically require human intelligence.20,21 The early development of AI encountered bottlenecks and could barely solve any practical problems due to the limited memory and processing speed of computers. In the 1980s, the emergence of expert systems brought an opportunity for the practical use of AI.22 However, they were heavily dependent on human-crafted rules and lacked the ability to adapt to new situations effectively. Then, the rise of machine learning23 revolutionized AI research by extracting meaningful signals from input data and learning patterns automatically. Until the 2010s, the advent of the big data era and the utilization of fast graphics processing units (GPUs) have enabled AI to flourish; simultaneously, deep learning (a subfield of machine learning) has attracted wide attention because of its superior learning ability.24
The emerging AI approaches are reshaping the way for data processing and analysis, especially they have been ideal means to detect, quantify, screen, or even predict the massive data from microfluidics.25–27 For example, AI algorithms trained on extensive medical images have significantly aided in the detection and diagnosis of diseases.28,29 AI-driven data analysis has assisted researchers with rapid result processing in the biomedicine field, bypassing time-consuming theoretical calculations or expensive and labor-intensive trials.30,31 With considerably enhanced computational power and growing accessible databases, AI has now transitioned from theoretical computer science studies to more practical applications.32,33 Integrating AI with microfluidics unlocks new possibilities for both experimental and analytical efficiencies in biomedical research, further unleashing the vast potential of microfluidic systems.34–36 In this review, we provide a comprehensive introduction on AI methods and seek to summarize recent exciting advances in AI-accelerated microfluidic systems for biomedical applications (Fig. 1). Finally, we discuss the challenges and prospects in this rapidly evolving field.
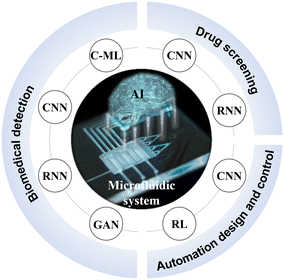 |
| Fig. 1 Overview of the high-throughput microfluidic systems accelerated by AI for biomedical applications. In biomedical detection, common AI algorithmic models include classical machine learning algorithms (C-ML, e.g., support vector machine (SVM), random forest (RF), K-means), convolutional neural networks (CNNs), recurrent neural networks (RNNs), and generative adversarial networks (GANs). For drug screening, the commonly used AI algorithmic models are CNNs and RNNs. In automated system control and design, reinforcement learning (RL) and CNNs are commonly applied AI algorithmic models. | |
2 Fundamentals of AI
The development of AI traces its roots back to the 1950s when scientists began exploring how to simulate human intelligent behavior in machines.19,20 Initially, AI research focused on rule-based reasoning and the development of expert systems.22 However, progress in AI was hampered by limited data, algorithms, and computer processing power. Fortunately, advancements in the neural network models, the GPU-based computation, and the well-developed frameworks like TensorFlow37 have revolutionized the field of AI.38 This has led to an explosive growth of AI technology, particularly in machine learning and deep learning. Machine learning enables computers to learn from data and improve performance, while deep learning achieves higher-level pattern recognition and abstraction capabilities. Thus, AI has found broad applications in domains such as computer vision,39 speech recognition,40 natural language processing,41 and more.42
2.1 Foundational AI techniques
AI techniques are widely employed to tackle five types of problems: regression, classification, clustering, dimensionality reduction, and reinforcement learning (Table 1). The regression problem involves predicting continuous value outcomes based on the feature parameters present in the data samples.43 For instance, in cancer research, regression models can predict the probability of cancer cell invasion based on variables such as tumor size, genetic markers, and patient characteristics. The classification problem focuses on categorizing data into predefined classes or groups based on their features.44,45 Its algorithms learn from labeled data to identify patterns and make predictions about the class of new, unseen instances. They play a crucial role in automating decision-making processes (e.g., cell sorting). In contrast, clustering algorithms group similar data points together based on their intrinsic properties.46 Clustering does not rely on predefined classes but discovers hidden structures or clusters within the data, for example, clustering gene expression data in genomics, aiding in discovering gene expression patterns and identifying gene sets associated with specific diseases. Dimensionality reduction algorithms are employed to handle datasets with a high number of variables or features. Such datasets often suffer from the curse of dimensionality, leading to increased computational complexity and data interpretation challenges.47 Dimensionality reduction helps overcome these challenges by reducing the number of variables while retaining the crucial information (e.g., principal component analysis (PCA)48,49 or t-distributed stochastic neighbor embedding (t-SNE)50). And reinforcement learning focuses on training an agent to make sequential decisions in an environment to maximize a reward signal.51 The agent learns through trial and error, contemplating how to act based on the environment to achieve maximum benefit.
Table 1 Classic AI models and their applications
Target problems |
Representative algorithms |
Main applications |
Regression problems43 |
Linear regression58 |
Probability prediction, function fitting, parameter correlation analysis, image generation, object detection, etc. |
Polynomial regression59 |
Neural network60–62 |
Classification problems44,45 |
Logistic regression63 |
Classification of known categories, image recognition, object detection, target dynamic tracking, text analysis, etc. |
Support vector machine (SVM)64 |
Random forest (RF)65 |
Decision tree (DT)66 |
Naïve bayes67 |
k-Nearest neighbor (kNN)68 |
Neural network60–62 |
Clustering problems46,69 |
K-Means70 |
Unknown category clustering, potential association discovery, phenomenon analysis, image segmentation, etc. |
Density peak clustering (DPC)71 |
Gaussian mixture model (GMM)72,73 |
Density-based spatial clustering of applications with noise (DBSCAN)74 |
Neural network60–62 |
Dimensionality reduction problems47 |
Principal component analysis (PCA)48,49 |
Feature extraction and selection, data visualization, noise reduction, etc. |
t-distributed stochastic neighbor embedding (t-SNE)50 |
Linear discriminant analysis (LDA)75 |
Reinforcement learning problems51 |
Q-learning76 |
Intelligent recommendation, path planning, adaptive control, etc. |
Deep Q-network (DQN)51 |
In practical applications, selecting suitable AI models and applying them flexibly is crucial for solving problems (Table 1). Moreover, multimodal data analysis may offer more comprehensive solutions to challenges.52,53 For example, several multimodal data processing algorithms have already been applied in the biomedical field like the correlation-based method canonical correlation analysis (CCA),54 the matrix factorization-based method multi-omics factor analysis v2 (MOFA+),55,56 and a multi-view autoencoder.57
2.2 The position of machine learning and deep learning in AI
The tremendous success of AI stems from machine learning and deep learning.77 Herein, we provide a further comprehension about these two cutting-edge technologies.
Machine learning, a subset of AI, focuses on developing algorithms and techniques that enable computer systems to learn and improve from data.23 It involves supervised learning, unsupervised learning, semi-supervised learning, and reinforcement learning (Table 2).23,78 Supervised learning is one of the most widely applied methods in machine learning.79,80 It receives labeled training data, where the relationship between the features and labels is known. By learning from the samples, a mapping function is constructed from the input to the output. Supervised learning is suitable for classification and regression problems, such as image classification and metric prediction. Conversely, unsupervised learning is a method of learning and pattern discovery without labeled data. Common tasks include clustering (grouping data into different categories) and dimensionality reduction (reducing the dimensionality of the data). This method contributes to a deeper understanding of the intrinsic structure and features of data, offering benefits like minimizing manual data preparation and uncovering hidden patterns. However, challenges arise from result unpredictability and the difficulty of measuring accuracy without predefined answers during training.81,82
Table 2 Four types of machine learning (categorized by the learning approach)
Learning types |
Brief description |
Supervised learning79,80 |
• The algorithm receives labeled training data where the relationship between features and labels is known. The purpose is to learn from the samples and construct a mapping function from inputs to outputs |
• Supervised learning is applicable to classification and regression problems |
Unsupervised learning81,82 |
• The algorithm receives unlabeled training data where the relationship between features and labels is unknown. The purpose is to discover hidden patterns, structures, and relationships within the data |
• Unsupervised learning is applicable to clustering and dimensionality reduction problems |
Semi-supervised learning83 |
• The algorithm receives a small amount of labeled training data and a large amount of unlabeled data for learning. The purpose is to enhance the performance and generalization capability of the algorithm by combining labeled and unlabeled data |
• Semi-supervised learning is applicable for problems where obtaining labeled data is difficult or expensive |
Reinforcement learning51,85 |
• The algorithm learns how to make decisions by observing feedback from the environment. The purpose is to maximize cumulative rewards through interactions with the environment |
• Reinforcement learning is applicable to reinforcement learning problems |
Semi-supervised learning bridges the gap between supervised learning and unsupervised learning. In semi-supervised learning, algorithms utilize a small amount of labeled training data along with a large amount of unlabeled data for learning.83 This is particularly useful for problems where labeled data are difficult to obtain or expensive. In addition, reinforcement learning is a learning paradigm where an agent learns how to make decisions by observing feedback from the environment.51 In reinforcement learning, an agent takes actions based on the current state and receives rewards or penalties as feedback. The goal is to maximize cumulative rewards through interaction with the environment. It is commonly used in building autonomous decision-making systems, such as robot control and system optimization.84,85 Overall, these learning approaches provide diverse methods to tackle various machine learning problems.
Deep learning, based on deep neural networks (DNNs),24 is at the forefront of machine learning. Neural networks (Fig. 2 and 3) are the core architecture of deep learning, where a structured nonlinear function y = f(x) is learned to map input x onto output y. It includes well-known network types like CNNs, RNNs, and GANs (Table 3).62,86,87 CNNs are extensively employed in image processing tasks. They are specifically designed to extract features from images using convolutional layers and pooling layers, resulting in efficient classification and recognition. Thus, CNNs have strong connections to the field of microfluidics. In 1998, the pioneers of deep learning, Lecun et al., proposed CNNs to successfully realize handwritten digit recognition and established the basic architecture of CNNs, including convolutional layers, pooling layers, and fully connected layers.88 However, due to limitations in datasets and computing power, as well as the continued advantages of shallow machine learning methods such as SVM and RF,89,90 CNNs did not initially receive widespread application and adoption. It was not until the ImageNet Large-Scale Visual Recognition Challenge in 2012 that AlexNet introduced deep architectures and GPU acceleration, significantly improving the accuracy of image classification and speeding up model training and inference.91 Subsequently, a wave of new models emerged, with VGGNet and GoogleNet standing out as notable examples in 2014.92,93 However, as the network depth increased, new challenges arose such as learning stagnation (caused by gradient vanishing) and training degradation (caused by overfitting). In 2015, ResNet successfully addressed the issue of training degradation by introducing residual connections, enabling direct information propagation across layers and becoming a crucial foundation for subsequent network designs.94 In 2016, DenseNet tackled the challenges of gradient vanishing and feature reuse by introducing dense connections.95 Another notable advancement in CNNs is MobileNet, proposed by Google in 2017, specifically designed for mobile and embedded devices.96 These advancements have driven the progress of CNNs, offering valuable insights and methodologies for deep learning. Moreover, they have paved the way for the development of classic object detection algorithms like Faster R-CNN,97 Mask R-CNN,98 and You Only Look Once (YOLO),99 as well as semantic segmentation algorithms such as U-Net,100 and DeepLab.101
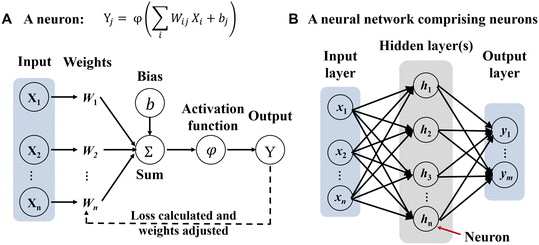 |
| Fig. 2 Neural network basics. A) Schematic of a discrete computing nonlinear element (neuron). A neural network is a type of deep learning architecture where a structured nonlinear function Y = f(X) is learned to map input X onto output Y. Specifically, the function is described as , where Xi is the input data and Yi is the output data. Wij is the connection weight from each neural node in the preceding layer to every neural node in the current layer. Wij is the most important training target in the neural network. bj is the bias of the neural node, accelerating the fitting of neural networks. The activation function φ nonlinearizes node data, allowing the neural network to approximate any nonlinear functions.110 B) Schematic of a neural network with one output layer and one hidden layer. The key of neural networks lies in the multiple layers of algebraic operations about function f.24,111 The term “depth” in deep learning refers to the number of hidden layers, i.e., the depth of a neural network. Traditional shallow neural networks typically have one to two hidden layers, while DNNs have more hidden layers, making the network deeper. This deep structure allows DNNs to learn more complex and abstract feature representations, thereby improving their performance in various tasks. | |
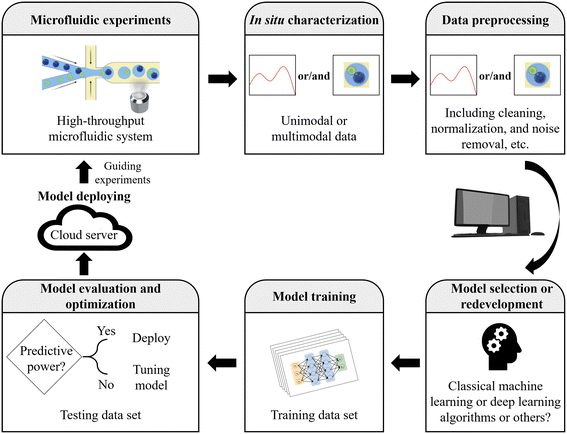 |
| Fig. 3 Workflow for applying AI. Microfluidics with AI fosters a powerful synergy, driving the development of efficient and scalable AI solutions in various microfluidic applications. | |
Table 3 Classic deep learning models (mainly in the microfluidic field)
Representative deep neural networks24,62,86 |
Brief description |
Convolutional neural networks 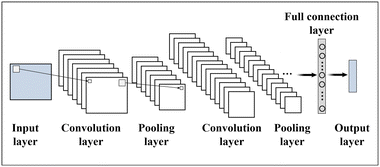 |
• CNNs are a type of deep neural network primarily used for image processing |
• Their main characteristic is the utilization of convolutional layers and pooling layers to extract features from images, enabling efficient classification and recognition. It automatically extracts local features and combine them in subsequent layers to achieve a global understanding of the entire image |
Recurrent neural networks 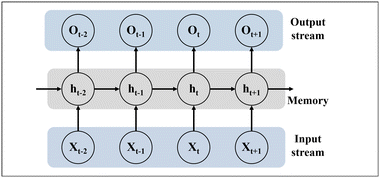 |
• RNNs are a type of deep neural network primarily used for sequence data processing |
• Their main characteristic is the presence of recurrent connections. In RNNs, each step has a hidden state that receives the previous time step's hidden state (ht−1) and the current time step's input (Xt), generating the output (Ot) and hidden state for the current time step (ht) |
Generative adversarial networks 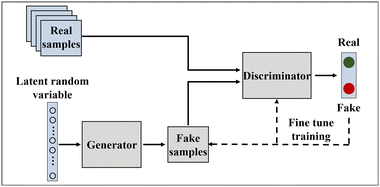 |
• GANs are a type of deep neural network primarily used for generating new samples of data |
• They consist of two components: the generator and the discriminator. The generator takes in a random noise vector and outputs a new sample of data. The discriminator takes in a sample of data and determines if it is real or fake. They are trained in an alternating fashion, and eventually, the generator generates new samples of data that resemble real samples |
RNNs have significantly contributed to sequence-based tasks like natural language processing and speech recognition, utilizing recurrent connections in their architecture.102 One special type of RNNs is known as long short-term memory (LSTM), which excels at capturing patterns and trends in sequences more effectively due to the unique feature of memory and selective forgetting.103 And as one of the most representative generative models, GANs mainly succeed in generating realistic and high-quality synthetic data, such as images.104 They function within a game-like framework, where a generator network and a discriminator network compete.104 Additionally, several other popular generative models in deep learning like variational autoencoders (VAEs)105 and diffusion models106 have also made strides in data generation. Another noteworthy deep learning algorithm is the transformer. It efficiently captures long-range dependencies in sequence data by introducing the attention mechanism,107 and it has demonstrated strong versatility across various domains, from natural language processing (e.g., generative pre-trained transformer,108 GPT) to computer vision (e.g., vision transformer,109 ViT).
However, the neural network is not omnipotent, especially for the “simple” tasks.77,112 While neural networks boast strong learning capabilities, they are susceptible to overfitting in the case of certain “simple” tasks.23 Meanwhile, deep learning models typically necessitate larger datasets to leverage their advantages, given their millions of parameters requiring sufficient data for effective training.24 On the other hand, models from traditional machine learning algorithms often provide interpretable feature importance, enabling a clear understanding of each feature's contribution to predictions. Conversely, deep learning models are often viewed as “black boxes” due to their numerous parameters and intricate structures, posing challenges for intuitive interpretation. This lack of interpretability may limit the suitability of deep learning, particularly in applications where a high degree of model interpretability is essential.
2.3 Working steps for applying AI
The workflow for applying AI encompasses several essential steps, providing a general guideline for AI in microfluidics (Fig. 3).34,113,114 Initially, it involves clearly defining the problem to be addressed through microfluidic experiments and AI techniques, while understanding project objectives, constraints, and desired outcomes. Relevant and representative data are then collected, ensuring their quality, diversity, and proper labeling or annotation if necessary. Herein, the data can be unimodal or multimodal. Next, the collected data undergo preprocessing, including cleaning, normalization, handling missing values, and noise removal. The data are transformed into a suitable format for training the AI model.
The appropriate model or algorithm is then selected or redeveloped, considering factors such as data nature, problem complexity, available resources, and performance requirements. With the model determined, the process of training comes into play, iteratively adjusting its internal parameters through optimization techniques to minimize error or loss. The performance of the trained AI model is evaluated using a variety of metrics to assess its generalization and prediction accuracy. This evaluation involves employing techniques such as data splitting or cross-validation, which help validate the model's performance on unseen data and ensure its reliability in real-world scenarios. Model optimization follows, involving fine-tuning through adjustments in hyperparameters, architecture modifications, or regularization techniques. The model is continuously iterated and refined to enhance its performance. Once optimized, the AI model is deployed in the desired environment or integrated into the target application or system, ensuring that the deployment infrastructure can handle computational requirements and input/output efficiently. It is important to note that each step in this workflow may require additional subtasks and considerations tailored to the specific project and domain at hand.
2.4 The synergy of high-throughput microfluidics and AI
Advancements in high-throughput microfluidics such as lab-on-a-chip for high-throughput single-cell analyses115 and high-throughput DNA and RNA sequencing116,117 have greatly enriched our data acquisition capabilities. However, handling and analyzing such extensive datasets through manual means would pose considerable challenges. The emerging AI approaches are reshaping the way for data processing and analysis, especially they have been ideal means to detect, quantify, screen, or even predict the massive data from microfluidics.25–27
On the other hand, the development of AI relies on the availability of data. The abundance of data could serve as a valuable resource for training and optimizing AI models. The synergy of high-throughput microfluidics and AI holds immense potential (e.g., AI-assisted high-throughput single-cell analysis)18 in advancing our understanding of biomedical systems and driving innovative applications in various domains.25,118
3 How can high-throughput microfluidic systems benefit from AI?
Microfluidic systems play a pivotal role as an indispensable platform for the construction and deployment of AI in a large-scale, high-throughput, automated, and cost-effective manner. In the upcoming sections, we would explore the multifaceted applications and the synergistic potential of AI-accelerated microfluidic systems.
3.1 AI-accelerated high-throughput microfluidic systems in biomedical detection
Biomedical detection provides vital information on cell biology, human physiology or pathology by measuring a wide range of targets, including proteins, nucleic acids, exosomes, cells, etc. In this field, microfluidics technology offers a powerful tool for constructing high-throughput experimental platforms, while AI, through a data-driven framework, further aids in achieving accurate and efficient target detection and outcome classification, and contributes to disease diagnosis and the development of intelligent detection devices.
3.1.1 AI-aided target detection.
In microfluidics, traditional target detection relies on manual identification and positioning using tools such as microscopes, which is subjective and limits the efficiency and reliability of detection. In contrast, AI-aided target detection offers the potential for swift, accurate, and consistent detection within the vast imaging data generated by microfluidic devices.119–121 This not only improves the efficiency of detection but also provides researchers with more valuable analysis and insights.
For example, Karl Gardner et al. applied YOLO (a CNN architecture) to develop an automated droplet and cell detector. Their system comprised an object detection model for identifying individual droplets within the ensemble, along with another object detection model designed to detect cells inside the droplets (Fig. 4A).122 The model achieved an impressive precision rate of 98%. Yellen et al. introduced a high-throughput live-cell biology platform that integrated the Mask R-CNN model. By utilizing ladder microfluidic networks, they created precise cell traps and employed Mask R-CNN for instance segmentation, allowing for the extraction and analysis of cellular phenotypic properties in a high-throughput manner (Fig. 4B).123 Finally, this platform quantified the responses of single cell-derived acute myeloid leukemia clones to targeted therapy, identifying rare resistance and morphological phenotypes at frequencies down to 0.05%.
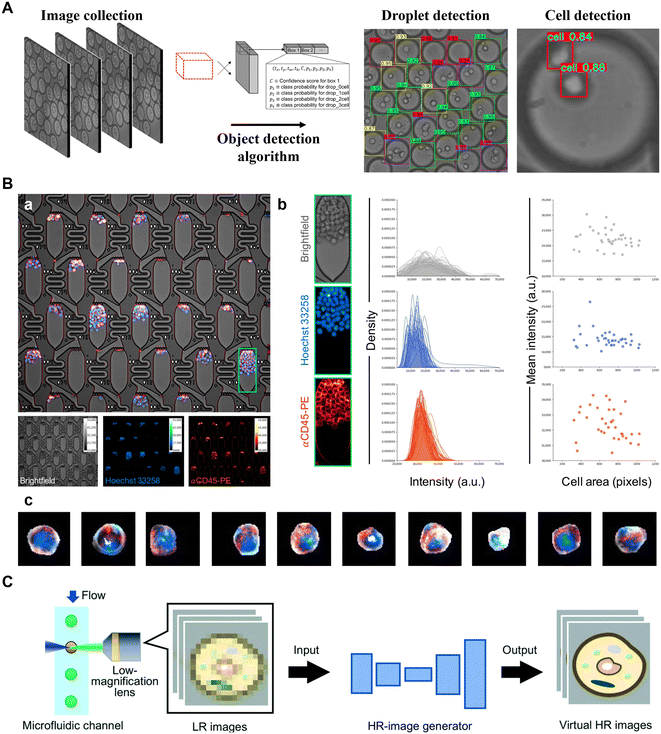 |
| Fig. 4 Applications of AI to microfluidic systems in target detection. A) Deep learning detector for high precision monitoring of cell encapsulation statistics in microfluidic droplets. Reproduced from ref. 122 with permission from The Royal Society of Chemistry.122 B) High-throughput single-cell segmentation. (a) Multichannel live-cell imaging. (b) Signal quantification of cells within a single culture apartment. (c) Example multichannel images demonstrating diversity of individual cells segmented using Mask R-CNN. Reproduced from ref. 123 with permission from the American Association for the Advancement of Science.123 C) High-resolution processing of sample images. Reproduced from ref. 126 with permission from The Royal Society of Chemistry.126 | |
Another notable aspect of AI-aided target detection is to recognize and monitor targets in real time. For instance, M. White et al. have employed CNN models to dynamically analyze real-time images, selectively extracting carrier cell-laden hydrogel microcapsules within chip channels. This innovative application allows for label-free detection of microcapsules, achieving an efficiency of approximately 100%.124 Moreover, Aspert et al. employed a CNN + LSTM architecture to monitor candidate regions and perform automatic cell division tracking and survival analysis from image sequences in a single-cell capture chip.125
In addition, enhancing data quality plays a crucial role in improving the capability of target detection, particularly in cases where the resolution of the detection objects is low. AI-aided target detection has proven to be effective in addressing these challenges. Researchers have developed techniques such as high-resolution image generation (a GAN architecture) to synthesize “virtual” high-resolution images from low-resolution cell images (Fig. 4C).126 The resulting images resembled the high-resolution images acquired by a high-magnification lens. This advancement enhances throughput without compromising sensitivity and spatial resolution. Moreover, Göröcs et al. also explored the potential of DNN-based image reconstruction and phase recovery techniques, enabling them to effectively reconstruct diffraction images of microscopic objects within microfluidic channels.127 This advancement enabled the development of a field-portable, intelligent imaging flow cytometer capable of performing label-free analysis of diverse phytoplankton and zooplankton compositions in water, with an impressive throughput of up to 100 mL h−1. In general, the unique capabilities of AI in object recognition, localization, segmentation, and image enhancement make it highly promising for microfluidic target detection. It holds great potential for achieving higher efficiency and accuracy in biomedical detection while minimizing the need for human intervention.
3.1.2 AI-aided outcome classification.
High-throughput microfluidic systems can rapidly generate a large volume of experimental results from numerous similar experiments. Analyzing, categorizing, and differentially processing these results have become essential for microfluidic data analysis and mining. The remarkable classification and clustering abilities of AI will be highly beneficial for this point.128,129
Firstly, proper classification can effectively distinguish different states of the tested substances, encompassing small molecules,130 proteins,131,132 nucleic acids,133 and cells.134,135 For instance, Ren et al. proposed a molecular identification platform based on the wavelength-multiplexed hook nanoantenna array (Fig. 5A).136 They effectively employed classical machine learning techniques, specifically SVM in combination with PCA, to classify a diverse collection of spectral data. This dataset included various analytes such as mixed alcohols (methanol, ethanol, and isopropanol), achieving a remarkable 100% accuracy in the classification process. Similarly, John-Herpin et al. designed a highly sensitive multiresonant plasmonic metasurface in a microfluidic device, which covers the major absorption bands of biomolecules.137 They utilized a DNN to extract signals from a large collection of spectrotemporal data, enabling precise differentiation of all coexisting biomolecules (proteins, nucleic acids, carbohydrates, and lipids). Another exemplary study by Wang et al. demonstrated a high-throughput microfluidic platform capable of analyzing multiple secreted biomarkers in individual live cells, employing machine learning for accurate tumor cell classification (Fig. 5B).138 Specifically, the researchers aligned a single-cell culture chip with a capture antibody array, facilitating the capture of secreted biomarkers from the cells. Using the K-means strategy, they analyzed secretion data from thousands of individual tumor cells, successfully achieving a tumor cell classification accuracy of 95.0%. Furthermore, to gain deeper insights, they employed the t-SNE dimensionality reduction algorithm to further analyze the grouping results, unveiling the distinctive secretion characteristics of subgroups. Recently, popular transformer algorithms have proven effective for less distinctive classifications. Cao et al. achieved a 98% accuracy rate by training a vision transformer model to analyze similar colors in multiplex digital PCR datasets using a single fluorescent channel.133
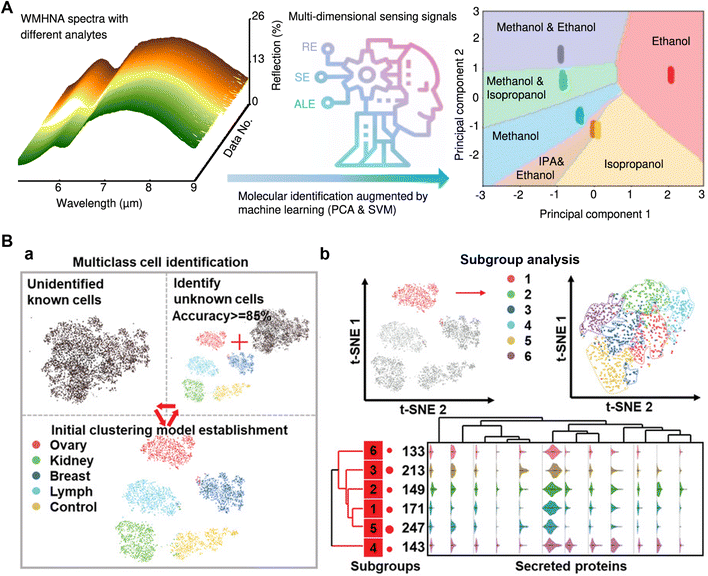 |
| Fig. 5 Applications of AI to microfluidic systems in outcome classification. A) Small molecular intelligent classification. Reproduced from ref. 136 with permission from Springer Nature.136 B) A high-throughput single-cell multi-index secreted biomarker profiling platform, combined with machine learning, to achieve accurate tumor cell classification. (a) Multiple tumor cell clustering and classification processes. (b) Subgroup analysis of tumor cells. Reproduced from ref. 138 with permission from Wiley.138 | |
Powerful target classification techniques also offer the ideal solution for efficient sorting of large-scale unlabeled samples.139–142 For example, Nitta et al. developed a CNN-assisted image-activated cell sorting technology, which enabled real-time intelligent cell searching and sorting at an unprecedented rate of about 100 cells per second.143 Compared with traditional flow cytometry, AI-assisted imaging flow cytometry has reached a comparable level of sorting speed and accuracy. This demonstrates the remarkable analytical ability of AI in outcome classification. By boosting the imaging performance and computational power, the research group further improved this technique to provide a 20-fold improvement in performance with a high throughput of ∼2000 events per second and a high sensitivity of ∼50 molecules of equivalent soluble fluorophores.144 The droplet microfluidics also benefits from the powerful classification capabilities of AI.145,146 For instance, Anagnostidis et al. demonstrated the effectiveness of combining CNNs with microfluidics for classifying real-time images of cell-laden hydrogel droplets based on the presence and quantity of micro-objects.147
Indeed, classic machine learning classification methods generally have lower computational complexity and are frequently employed in conjunction with dimensionality reduction techniques. They are particularly well-suited for scenarios where the features are clearly defined and the number of classification types is limited. On the other hand, neural network-based models can handle higher complexity and larger-scale tasks. The efficient classification of microfluidic results using AI helps accelerate result analysis, improve classification accuracy, and enhance the capability for comprehensive analysis of multiple targets.
3.1.3 AI-aided target detection and outcome classification: advancing the diagnosis of disease and the development of an intelligent detection device.
In disease antigen detection, microfluidic chips can be utilized to capture and analyze various biomolecules in bodily fluid samples, such as proteins,148–150 nucleic acids,151 and extracellular vesicles.152,153 AI technology assists in interpreting the biological information data generated by microfluidic chips, enabling fast and accurate identification and classification of specific disease biomarkers or pathological cells,154–156 providing crucial evidence for early disease diagnosis and treatment.
For instance, Gao et al. proposed a machine learning-assisted microfluidic nanoplasmonic digital immunoassay to monitor the cytokine storm in COVID-19 patients (Fig. 6A).157 The researchers utilized antigen capture arrays to capture multiple cytokines and employed CNNs to efficiently count the specific imaging signals. By establishing the correlation between the imaging signal count and cytokine concentration, reliable diagnostic evidence can be provided for COVID-19. Additionally, Manak et al. cultured cells derived from solid prostate tumor tissue or breast tumor tissue on a microfluidic chip and employed machine vision algorithms to directly quantify the dynamic phenotypic behavior of individual live tumor cells.158 Herein, the RF algorithm, incorporating approximately 300 individual and aggregate biomarker inputs, generates cell-level and patient-level tissue scores. These scores differentiate cell populations and provide risk stratification and prognostic assessment for cancer patients with low-grade and intermediate-grade diseases and tumor behavior. Similar approaches translate to the diagnosis of other types of disorders. Ellett et al. conducted a study where they measured the spontaneous motility of neutrophils from a drop of diluted blood. They correlated the neutrophil movement in a complex microfluidic network with the severity of sepsis using supervised learning and employed SVM to differentiate septic and non-septic patients.159 Besides, Singh et al. conducted label-free detection of circulating tumor cells in blood using microfluidics, machine learning, and holographic microscopy techniques. The platform screened cells flowing through microchannels and generated a numerical fingerprint for each cell based on their size and intensity characteristics. It could detect as few as 10 cancer cells per milliliter of blood with an error rate of only 0.001%.160 Similarly, Jiang et al. demonstrated the diagnosis of thrombotic diseases through label-free morphological detection of clustered platelets in blood. By imaging individual cells using optical stretchable microfluidic time-stretch microscopy, a machine learning algorithm accurately distinguished clustered platelets, individual platelets, and white blood cells with a precision of 96.6%.161
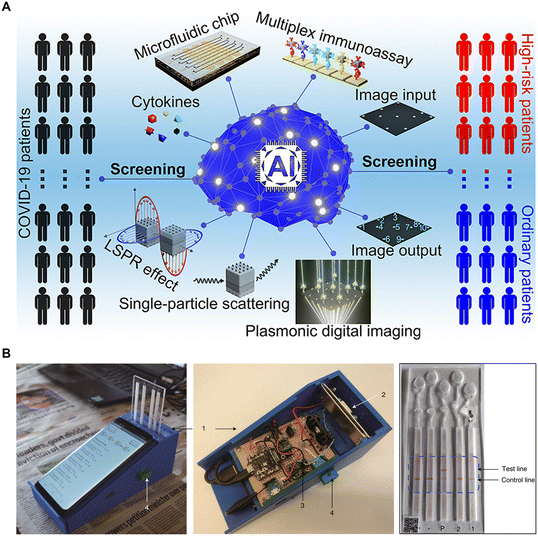 |
| Fig. 6 AI-aided target detection and outcome classification: advancing the diagnosis of disease and the development of the intelligent detection device. A) Machine learning-assisted microfluidic nanoplasmonic digital immunoassay for cytokine storm profiling in COVID-19 patients. Reproduced from ref. 157 with permission from American Chemical Society.157 B) A smartphone-based microfluidic platform for multiplexed DNA diagnosis of malaria. Reproduced from ref. 163 with permission from Springer Nature.163 | |
Moreover, AI-based inference holds significant advantages in the field of intelligent detection device development. For instance, Potluri et al. developed an affordable, smartphone-based device for detecting ovulation.162 This innovative device utilized a microfluidic chip to process saliva and generate distinct patterns. The patterns were then analyzed using a MobileNet model, a type of CNN, through a dedicated app on the smartphone. This analysis enables the determination of a woman's ovulation capacity with an accuracy rate exceeding 99%. Similarly, Guo et al. reported a smartphone-based nucleic acid testing platform for multiplexed DNA diagnosis of malaria (Fig. 6B).163 Deep learning algorithms were integrated into a mobile application to automatically classify images of paper-based microfluidic diagnostic tests and provide local decision support. In addition, Braz et al. demonstrated the use of machine learning and an electronic tongue to differentiate saliva samples from oral cancer patients and healthy individuals, thus aiding in the diagnosis of oral cancer.164 Similar strategies can also be employed in the development of smart devices for detecting samples such as sweat130,165 and urine.166
3.2 AI-accelerated high-throughput microfluidic systems in drug screening
Traditional drug screening typically involves testing a large number of compounds, which is time-consuming, labor-intensive, and reliant on empirical knowledge.167,168 However, by integrating microfluidic technology and AI, the screening process from drug design, synthesis to testing can be transformed, which is of significant importance in accelerating drug development.168–171 For example, Grisoni et al. pioneered the strategy of combining deep learning-based molecular design with microfluidic automated synthesis (Fig. 7A).172 The authors used a generative model for molecular design and microfluidic platforms for on-chip chemical synthesis. Implementing this strategy, they successfully synthesized 28 molecules, 12 of which were potent liver X receptor (LXR) agonists, substantially reducing time and cost in the preclinical drug discovery process.
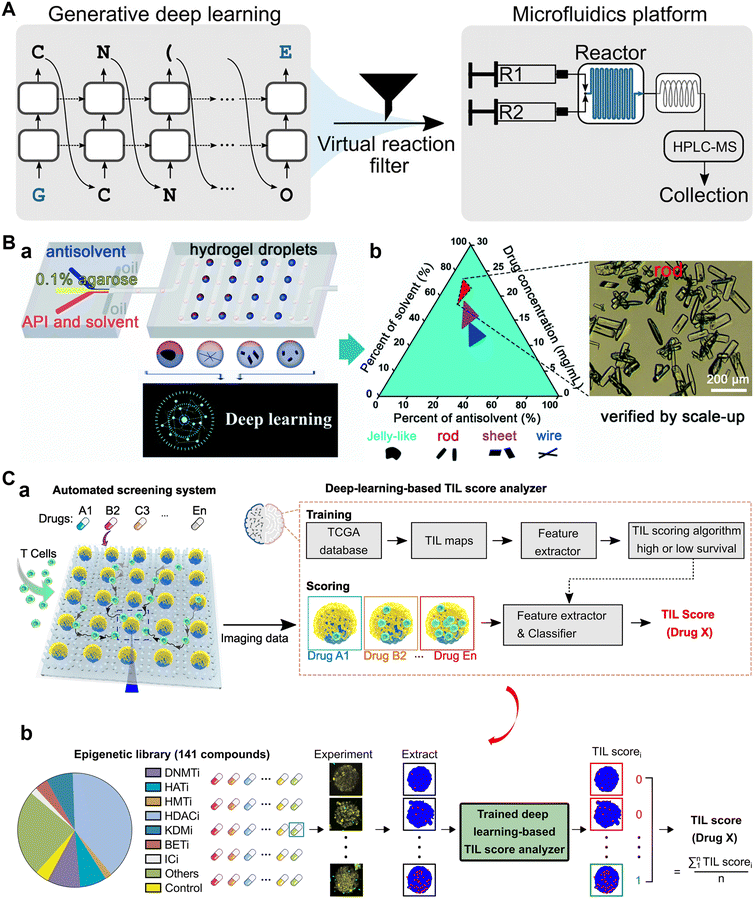 |
| Fig. 7 Applications of AI to microfluidic systems in drug screening. A) Combining generative AI and on-chip synthesis for de novo drug screening. Reproduced from ref. 172 with permission from American Association for the Advancement of Science.172 B) A high-throughput system combining microfluidic hydrogel droplets with deep learning (a) for screening the antisolvent-crystallization conditions of active pharmaceutical ingredients (b). Reproduced from ref. 173 with permission from The Royal Society of Chemistry.173 C) Microfluidics guided by deep learning for cancer immunotherapy compounds screening. (a) The automated screening platform for cancer immunotherapy screening. (b) Scoring based on deep learning and clinical data. Reproduced from ref. 183 with permission from National Academy of Sciences.183 | |
Besides, the screening of the synthesis conditions of a known drug also benefits from the combination of AI and microfluidic systems. Su et al. proposed a high-throughput system that combined microfluidic hydrogel droplets with deep learning for screening the antisolvent-crystallization conditions of active pharmaceutical ingredients (APIs) (Fig. 7B). Faster R-CNN was applied to analyze and classify the morphologies of the indomethacin crystals to find out the optimal antisolvent-crystallization conditions.173 Similarly, Huang et al. developed a deep learning-aided programmable microliter-droplet system and used it for the high-throughput screening of time-resolved protein crystallization. The time-resolved crystallization of target proteins under different crystallization conditions was revealed, which was promising to guide the scale-up production of the pharmaceutical protein crystals.174
In preclinical trials, cells, OoCs, or model animals often serve as drug testing platforms.175–178 By introducing AI into these platforms, efficient drug screening and evaluation can be achieved.179–182 For instance, Ao et al. integrated deep learning with chips to continuously track the dynamics of both T cell infiltration behavior and cytotoxicity (Fig. 7C).183 The AI model extracted T-cell tumor invasion characteristics under drug treatment, and scored tumor-infiltrating lymphocyte invasion characteristics according to clinicopathological data and patient survival data. The score is directly related to drug efficacy, thus achieving the purpose of screening immunotherapy compounds for treating solid tumors. As another example, Lin et al. described an in vivo drug screening strategy that combined large-scale brain activity maps (BAMs) of zebrafish (immobilized on chips) with machine learning. They scanned neural cell activity in thousands of zebrafishes treated with different clinical central nervous system drugs and extracted BAMs to build a reference library. These drugs were classified into 10 clusters using the machine learning algorithm. Next, they compared and analyzed new BAMs under the treatment of several new drugs with the reference library, and used machine learning to predict potential clinical therapeutic efficacy of those drugs. Among the 121 novel compounds tested, 30 were predicted to have antiepileptic properties.184 In short, microfluidics provided high-throughput experimentation platforms for the drug testing, with AI closing the loop with a data-driven framework for evaluation and screening.
3.3 AI-accelerated high-throughput microfluidic systems with automated design and control
The design and control of high-throughput microfluidic systems with the aid of AI allow for rapid optimization of device performance, increase automation levels, and accelerate the commercialization of such systems.185–187 In 2017, Stoecklein et al. demonstrated one of the first uses of deep learning in microfluidic design automation, to sculpt flow using passive pillars in inertial fluid flow (1 < Re < 100).188 The authors showed how precise flow profiles can be generated using clever pillar distributions along the channel, and answered the question “what kind of geometry is required to produce an ideal microfluidic shape?”. Another representative study is the design automation of flow-focusing droplet generators by Ali Lashkaripour et al. (Fig. 8A).189 They gathered a comprehensive dataset of experimental droplet data from flow-focusing junctions with diverse geometries and flow conditions. Utilizing this dataset, they trained a multi-layer feed-forward neural network to accurately predict the performance of droplet formation. Additionally, they explored reverse predictive models to estimate the output parameters, such as the geometry and flow conditions, based on the given input parameters like droplet size and generation frequency. This allowed them to convert user-specified performance requirements into the desired geometry and flow rate, effectively completing the design process of microfluidic devices.
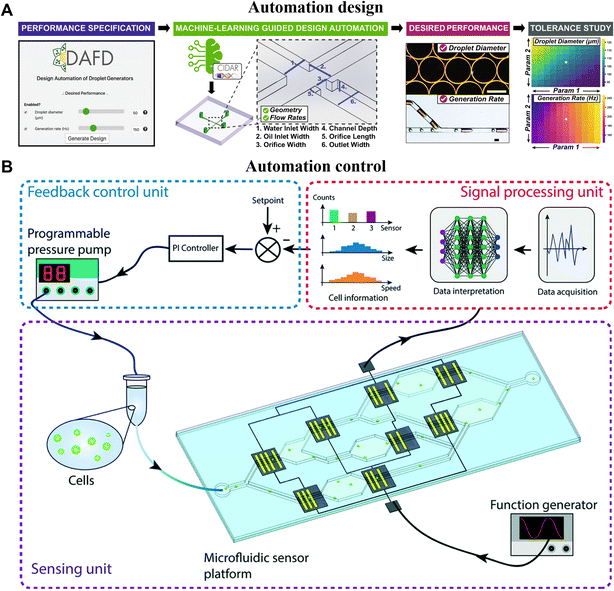 |
| Fig. 8 AI-accelerated high-throughput microfluidic systems with intelligent design and control. A) Machine-learning-empowered design automation for the control of microfluidic droplet generation. Reproduced from ref. 189 with permission from Springer Nature.189 B) Closed-loop feedback control of microfluidic cell manipulation via deep-learning integrated sensor networks. Reproduced from ref. 195 with permission from The Royal Society of Chemistry.195 | |
On the other hand, human-based control and adjustment are often time-consuming, labor-intensive, and prone to low accuracy as well as user bias.185 By incorporating advanced control algorithms, microfluidic systems can be guided for smooth operation, ensuring timely feedback in case of anomalies.190,191 This approach would pave the way for robust and reproducible long-term experiments, reducing human intervention and mitigating the impact of potential biases.192–194 For example, Wang et al. employed a hybrid approach, combining deep learning with a traditional proportional-integral (PI) feedback controller, to establish a closed-loop control system for regulating cell flow rates in microchannels. By leveraging CNNs, they achieved real-time interpretation of cell data and generated actionable control signals. This enabled the PI feedback controller to promptly adjust the driving pressure of the sample, ensuring a consistent flow velocity of cells within the microdevice (Fig. 8B).195 Another notable example is the work by Dressler et al., who utilized two reinforcement learning methods, namely DQN and model-free episodic controllers (MFECs), to automate the control of dynamic flow rates in precision pumps, addressing two control problems in microfluidics.196 Firstly, they successfully achieved precise positioning of the interface between two miscible fluids under laminar flow conditions. Secondly, they dynamically controlled the size of water-in-oil droplets in segmented flow. As mentioned earlier, reinforcement learning is a learning paradigm where an agent learns to make decisions by observing feedback from the environment. Thus, it is a versatile algorithm with wide applicability for optimizing control in microfluidic systems. For instance, the benefits of reinforcement learning were also made use of by Abe et al., to realize intelligent peristaltic pumps for flow control.197 They effectively control the micro-valves of the peristaltic pump, enabling manipulation of flow conditions such as flow switching and micro-mixing within microchannels. In summary, AI-assisted automation design and control facilitate the autonomous operation of microfluidic systems, minimizing the need for human intervention and enabling rapid adaptation to diverse experimental requirements.
4 Conclusions and perspectives
In conclusion, we proposed an overview of the AI-accelerated high-throughput microfluidic systems and their applications in biomedical fields. Herein, AI has enhanced the accuracy and efficiency of microfluidic systems for biomedical detection, advancing disease diagnosis and intelligent detection devices. Moreover, AI-driven high-throughput microfluidic systems support the drug screening process, covering drug design, synthesis, and testing. Additionally, AI-assisted automation demonstrates the potential of AI in optimizing workflow and personnel resource utilization.
Despite the proven advantages of AI-for-microfluidics in this review, several potential challenges and pitfalls still exist. Firstly, the widespread adoption of AI in microfluidics requires increased technical expertise for users. The complexity of deep learning and machine learning prompts researchers in microfluidics to learn new skills and tools. Until now, there is also no definite and precise instruction for deciding which type of algorithm is the most appropriate one for a specific application. Secondly, the “black box” nature of AI, especially deep learning models, makes their decision-making process challenging to interpret. Understanding the principles behind AI predictions is crucial for researchers to trust and use AI. The lack of interpretability may lead to resistance or skepticism in critical areas, such as the medical field where explicit explanations for disease diagnosis or treatment decisions are essential.118,198 Additionally, AI-for-microfluidics faces the challenge of meeting the “quality and quantity” requirements for training data, which translates into the need for stability and robustness in data acquisition from high-throughput microfluidic platforms. Also, AI-based analysis suffers from several other issues like time-consuming training, hyper-parameter tuning, and dependence on labels in learning.
In the highlighted research fields of this review, CNNs are widely used because they take a set of images as input data. And transformer models, with their revolutionary attention mechanism for capturing intricate relationships in complex data, may find increased applications in target detection and outcome classification. Also, generative models (e.g., GANs,104 diffusion models106) are presenting more prospects in aiding microfluidic research by generating synthetic data, such as the generative design of potential drugs.199 In addition, reinforcement learning and semi-supervised learning will further unlock the potential of AI in microfluidics. Presently, many existing platforms rely on supervised learning algorithms, which typically demand training on large amounts of manually labeled data. Embracing unlabeled or minimally labeled reinforcement learning and semi-supervised learning can aid in addressing the challenges. Furthermore, since each modality of data has inherent limitations, learning and reasoning based on multimodal data can contribute to addressing complex problems within microfluidic systems.200
Certainly, the synergistic combination of high-throughput microfluidic systems and AI has created an emerging yet rapidly growing field. Although some additional technical skills may be required, standardized AI development frameworks (e.g., Scikit-learn,201 TensorFlow,202 PyTorch203), along with accessible AI application libraries (e.g., Github, https://github.com/),204 have provided extensive technical support for the use of AI. Herein, we have also provided a summary of algorithms applicable to different task demands in microfluidics (Fig. 1, Tables 1 and 2), providing valuable references for the use of AI in microfluidics. However, these insights are insufficient. In the future, more people need to learn about programming like they learn a new language. Additionally, we encourage the establishment of accessible databases in the microfluidics field (e.g., collections of various biomedical images). This will directly alleviate the challenges associated with data collection and preprocessing for training models. Moreover, the sharing of similar algorithmic architectures may reduce technical barriers for the use of AI in microfluidics, for example, training high-performance generic image classifiers for a wide range of medical image classification tasks. In this way, AI could indeed change the underpinning paradigm of microfluidics' use, where massively parallelized efforts could be made feasible to solve problems completely impossible with AI-absent efforts. We foresee a sustained upward trend in the utilization of AI within microfluidic systems.
Author contributions
J. Z.: conceptualization, investigation, methodology, writing – review & editing, funding acquisition. J. D.: investigation, analysis, methodology, summary, writing – original draft, review & editing. H. H.: investigation, summary, writing – original draft. L. H.: investigation, analysis, writing – review & editing, resources, funding acquisition. J. L.: conceptualization, methodology, supervision, writing – review & editing, resources, funding acquisition. J. Z. and J. D. contributed equally to this work.
Conflicts of interest
There are no conflicts of interest to declare.
Acknowledgements
This work was financially supported by the National Key Research and Development Program of China (No. 2021YFA1200104), the New Cornerstone Science Foundation, the National Natural Science Foundation of China (No. 22004135; 22174167; 22027807; 22034004), the Guangdong Basic and Applied Basic Research (Grant No. 2023A1515010647; 2021A1515110388), the Shenzhen Science and Technology Program (Grant No. RCBS20210706092409020, JCYJ20220818102014028, GXWD20220817153451002), the Strategic Priority Research Program of Chinese Academy of Sciences (No. XDB36000000), the Tsinghua-Vanke Special Fund for Public Health and Health Discipline Development (No. 2022Z82WKJ003), and the Shenzhen Medical Research Fund (Grant No. A2303049).
References
- G. M. Whitesides, Nature, 2006, 442, 368–373 CrossRef CAS PubMed.
- D. R. Reyes, D. Iossifidis, P. A. Auroux and A. Manz, Anal. Chem., 2002, 74, 2623–2636 CrossRef CAS PubMed.
- J. K. Nunes and H. A. Stone, Chem. Rev., 2022, 122, 6919–6920 CrossRef CAS PubMed.
- S. Y. Teh, R. Lin, L. H. Hung and A. P. Lee, Lab Chip, 2008, 8, 198–220 RSC.
- L. Y. Yeo, H. C. Chang, P. P. Chan and J. R. Friend, Small, 2011, 7, 12–48 CrossRef CAS PubMed.
- P. N. Nge, C. I. Rogers and A. T. Woolley, Chem. Rev., 2013, 113, 2550–2583 CrossRef CAS PubMed.
- Y. Yang, E. Noviana, M. P. Nguyen, B. J. Geiss, D. S. Dandy and C. S. Henry, Anal. Chem., 2017, 89, 71–91 CrossRef CAS PubMed.
- C. Zhang and D. Xing, Chem. Rev., 2010, 110, 4910–4947 CrossRef CAS PubMed.
- A. M. Nightingale, C. L. Leong, R. A. Burnish, S. U. Hassan, Y. Zhang, G. F. Clough, M. G. Boutelle, D. Voegeli and X. Niu, Nat. Commun., 2019, 10, 2741 CrossRef PubMed.
- F. Lan, B. Demaree, N. Ahmed and A. R. Abate, Nat. Biotechnol., 2017, 35, 640–646 CrossRef CAS PubMed.
- O. Caen, H. Lu, P. Nizard and V. Taly, Trends Biotechnol., 2017, 35, 713–727 CrossRef CAS PubMed.
- S. Sart, G. Ronteix, S. Jain, G. Amselem and C. N. Baroud, Chem. Rev., 2022, 122, 7061–7096 CrossRef CAS PubMed.
- P. S. Dittrich and A. Manz, Nat. Rev. Drug Discovery, 2006, 5, 210–218 CrossRef CAS PubMed.
- Y. X. Liu, L. Y. Sun, H. Zhang, L. R. Shang and Y. J. Zhao, Chem. Rev., 2021, 121, 7468–7529 CrossRef CAS PubMed.
- K. S. Elvira, I. S. X. Casadevall, R. C. Wootton and A. J. Demello, Nat. Chem., 2013, 5, 905–915 CrossRef CAS PubMed.
- P. P. Zhou, J. X. He, L. Huang, Z. M. Yu, Z. N. Su, X. T. Shi and J. H. Zhou, Nanomaterials, 2020, 10, 2514 CrossRef CAS PubMed.
- A. A. Volk, R. W. Epps and M. Abolhasani, Adv. Mater., 2021, 33, 2004495 CrossRef CAS PubMed.
- L. Huang, Z. Liu, J. He, J. Li, Z. Wang, J. Zhou and Y. Chen, Cell Rep. Phys. Sci., 2023, 4, 101276 CrossRef CAS.
-
P. Norvig and S. J. Russell, Artificial intelligence: a modern approach, Prentice Hall, Englewood Cliffs, N.J., 1995 Search PubMed.
- M. Haenlein and A. Kaplan, Calif. Manag. Rev., 2019, 61, 5–14 CrossRef.
- Y. N. Harari, Nature, 2017, 550, 324–327 CrossRef CAS PubMed.
- R. O. Duda and E. H. Shortliffe, Science, 1983, 220, 261–268 CrossRef CAS PubMed.
- M. I. Jordan and T. M. Mitchell, Science, 2015, 349, 255–260 CrossRef CAS PubMed.
- Y. Lecun, Y. Bengio and G. Hinton, Nature, 2015, 521, 436–444 CrossRef CAS.
- J. Riordon, D. Sovilj, S. Sanner, D. Sinton and E. Young, Trends Biotechnol., 2019, 37, 310–324 CrossRef CAS PubMed.
- N. Pouyanfar, S. Z. Harofte, M. Soltani, S. Siavashy, E. Asadian, F. Ghorbani-Bidkorbeh, R. Kecili and C. M. Hussain, Trends Environ. Anal. Chem., 2022, 34, e00160 CrossRef CAS.
- J. T. Li, J. Chen, H. Bai, H. W. Wang, S. P. Hao, Y. Ding, B. Peng, J. Zhang, L. Li and W. Huang, Research, 2022, 2, 20 Search PubMed.
- G. Litjens, T. Kooi, B. E. Bejnordi, A. A. A. Setio, F. Ciompi, M. Ghafoorian, J. A. W. M. van der Laak, B. van Ginneken and C. I. Sanchez, Med. Image Anal., 2017, 42, 29 CrossRef PubMed.
- H. S. Zare, M. Soltani, S. Siavashy and K. Raahemifar, Small, 2022, 18, e2203169 CrossRef PubMed.
- E. A. Galan, H. R. Zhao, X. K. Wang, Q. H. Dai, W. Huck and S. H. Ma, Matter, 2020, 3, 1893–1922 CrossRef.
- L. Liu, M. Bi, Y. Wang, J. Liu, X. Jiang, Z. Xu and X. Zhang, Nanoscale, 2021, 13, 19352–19366 RSC.
- S. M. Mckinney, M. Sieniek, V. Godbole, J. Godwin, N. Antropova, H. Ashrafian, T. Back, M. Chesus, G. S. Corrado, A. Darzi, M. Etemadi, F. Garcia-Vicente, F. J. Gilbert, M. Halling-Brown, D. Hassabis, S. Jansen, A. Karthikesalingam, C. J. Kelly, D. King, J. R. Ledsam, D. Melnick, H. Mostofi, L. Peng, J. J. Reicher, B. Romera-Paredes, R. Sidebottom, M. Suleyman, D. Tse, K. C. Young, J. De Fauw and S. Shetty, Nature, 2020, 586, E19 CrossRef CAS PubMed.
- D. V. Ouyang, B. He, A. Ghorbani, N. Yuan, J. Ebinger, C. P. Langlotz, P. A. Heidenreich, R. A. Harrington, D. Liang, E. A. Ashley and J. Zou, Nature, 2020, 580, 252 CrossRef CAS PubMed.
- A. Isozaki, J. Harmon, Y. Zhou, S. Li, Y. Nakagawa, M. Hayashi, H. Mikami, C. Lei and K. Goda, Lab Chip, 2020, 20, 3074–3090 RSC.
- J. Zheng, T. Cole, Y. Zhang, J. Kim and S. Y. Tang, Biosens. Bioelectron., 2021, 194, 113666 CrossRef CAS.
- X. Y. Chen and H. L. Lv, NPG Asia Mater., 2022, 14, 69 CrossRef.
- L. Rampasek and A. Goldenberg, Cell Syst., 2016, 2, 12–14 CrossRef CAS PubMed.
- H. El-Sayed, S. Sankar, M. Prasad, D. Puthal, A. Gupta, M. Mohanty and C. Lin, IEEE Access, 2018, 6, 12 Search PubMed.
- A. Voulodimos, N. Doulamis, A. Doulamis and E. Protopapadakis, Comput. Intel. Neurosc., 2018, 2018, 7068349 Search PubMed.
- D. O'Shaughnessy, Proc. IEEE, 2003, 91, 34 CrossRef.
- J. Hirschberg and C. D. Manning, Science, 2015, 349, 6 CrossRef PubMed.
- Y. K. Dwivedi, L. Hughes, E. Ismagilova, G. Aarts, C. Coombs, T. Crick, Y. Duan, R. Dwivedi, J. Edwards, A. Eirug, V. Galanos, P. V. Ilavarasan, M. Janssen, P. Jones, A. K. Kar, H. Kizgin, B. Kronemann, B. Lal, B. Lucini, R. Medaglia, K. Le Meunier-Fitzhugh, L. C. Le Meunier-Fitzhugh, S. Misra, E. Mogaji, S. K. Sharma, J. B. Singh, V. Raghavan, R. Raman, N. P. Rana, S. Samothrakis, J. Spencer, K. Tamilmani, A. Tubadji, P. Walton and M. D. Williams, Int. J. Inf. Sci. Manag., 2021, 57, 47 Search PubMed.
- F. Stulp and O. Sigaud, Neural Netw., 2015, 69, 20 CrossRef PubMed.
- L. Chen, S. Li, Q. Bai, J. Yang, S. Jiang and Y. Miao, Remote Sens., 2021, 13, 51 Search PubMed.
- S. B. Kotsiantis, I. D. Zaharakis and P. E. Pintelas, Artif. Intell. Rev., 2006, 26, 32 CrossRef.
- R. Xu and D. Wunsch, IEEE Trans. Neural Netw., 2005, 16, 34 CrossRef PubMed.
- F. Anowar, S. Sadaoui and B. Selim, Comput. Sci. Rev., 2021, 40, 13 Search PubMed.
- H. Hotelling, J. Educ. Psychol., 1933, 6, 417–441 Search PubMed.
- S. Wold, K. Esbensen and P. Geladi, Chemom. Intell. Lab. Syst., 1987, 2, 37–52 CrossRef CAS.
- L. Van der Maaten and G. Hinton, J. Mach. Learn. Res., 2008, 9, 2579–2605 Search PubMed.
- V. Mnih, K. Kavukcuoglu, D. Silver, A. A. Rusu, J. Veness, M. G. Bellemare, A. Graves, M. Riedmiller, A. K. Fidjeland, G. Ostrovski, S. Petersen, C. Beattie, A. Sadik, I. Antonoglou, H. King, D. Kumaran, D. Wierstra, S. Legg and D. Hassabis, Nature, 2015, 518, 529–533 CrossRef CAS PubMed.
- F. Bao, Y. Deng, S. Wan, S. Q. Shen, B. Wang, Q. Dai, S. J. Altschuler and L. F. Wu, Nat. Biotechnol., 2022, 40, 1295 CrossRef CAS PubMed.
- J. Park, J. Kim, T. Lewy, C. M. Rice, O. Elemento, A. F. Rendeiro and C. E. Mason, Genome Biol., 2022, 23, 256 CrossRef CAS PubMed.
- D. R. Hardoon, S. Szedmak and J. Shawe-Taylor, Neural Comput., 2004, 16, 2639–2664 CrossRef PubMed.
- R. Argelaguet, B. Velten, D. Arnol, S. Dietrich, T. Zenz, J. C. Marioni, F. Buettner, W. Huber and O. Stegle, Mol. Syst. Biol., 2018, 14, e8124 CrossRef PubMed.
- R. Argelaguet, D. Arnol, D. Bredikhin, Y. Deloro, B. Velten, J. C. Marioni and O. Stegle, Genome Biol., 2020, 21, 111 CrossRef PubMed.
- T. Ma and A. Zhang, BMC Genomics, 2019, 20, 1–11 CrossRef PubMed.
- D. Maulud and A. M. Abdulazeez, J. Appl. Sci. Technol. Trends, 2020, 1, 140–147 CrossRef.
- E. Ostertagová, Procedia Eng., 2012, 48, 500–506 CrossRef.
- C. M. Bishop, Rev. Sci. Instrum., 1994, 65, 1803–1832 CrossRef.
- A. D. Dongare, R. R. Kharde and A. D. Kachare, Int. J. Eng. Innov. Technol., 2012, 1, 189–194 Search PubMed.
- J. Schmidhuber, Neural Netw., 2015, 61, 33 CrossRef PubMed.
- J. C. Stoltzfus, Acad. Emerg. Med., 2011, 18, 1099–1104 CrossRef PubMed.
- W. S. Noble, Nat. Biotechnol., 2006, 12, 1565–1567 CrossRef PubMed.
- L. Breiman, Mach. Learn., 2001, 5–32 CrossRef.
- B. Charbuty and A. Abdulazeez, J. Appl. Sci. Technol. Trends, 2021, 2, 20–28 CrossRef.
- N. Friedman, D. Geiger and M. Goldszmidt, Mach. Learn., 1997, 131–163 CrossRef.
- Z. Zhang, Ann. Transl. Med., 2016, 4, 218 CrossRef PubMed.
- C. Fraley and A. E. Raftery, Comput J., 1998, 8, 578–588 CrossRef.
- D. Steinley, Br. J. Math. Stat. Psychol., 2006, 59, 1–34 CrossRef PubMed.
- A. Rodriguez and A. Laio, Science, 2014, 344, 1492–1496 CrossRef CAS PubMed.
- Y. Zhang, M. Li, S. Wang, S. Dai, L. Luo, E. Zhu, H. Xu, X. Zhu, C. Yao and H. Zhou, ACM Trans. Multimed. Comput. Commun. Appl., 2021, 17, 1–14 Search PubMed.
- X. Lin, X. Yang and Y. Li, J. Phys.: Conf. Ser., 2019, 3, 32012 CrossRef.
-
M. Ester, H. Kriegel, J. Sander and X. Xu, KDD-96 Proceedings, 1996, vol. 34, pp. 226–231 Search PubMed.
- A. Sharma and K. K. Paliwal, Int. J. Mach. Learn. Cybern., 2015, 6, 443–454 CrossRef.
- C. J. Watkins and P. Dayan, Mach. Learn., 1992, 279–292 Search PubMed.
- C. Janiesch, P. Zschech and K. Heinrich, Electron. Mark., 2021, 31, 11 CrossRef.
- Y. Zhang and Q. Yang, IEEE Trans. Knowl. Data Eng., 2022, 34, 24 Search PubMed.
- Z. Zhou, Natl. Sci. Rev., 2018, 5, 44–53 CrossRef.
- V. Havlicek, A. D. Corcoles, K. Temme, A. W. Harrow, A. Kandala, J. M. Chow and J. M. Gambetta, Nature, 2019, 567, 209–212 CrossRef CAS PubMed.
- H. B. Barlow, Neural Comput., 1989, 3, 295–311 CrossRef.
- A. Glielmo, B. E. Husic, A. Rodriguez, C. Clementi, F. Noe and A. Laio, Chem. Rev., 2021, 121, 37 CrossRef PubMed.
- J. E. Van Engelen and H. H. Hoos, Mach. Learn., 2020, 109, 68 CrossRef.
- J. Kober, J. A. Bagnell and J. Peters, Int. J. Rob. Res., 2013, 32, 37 CrossRef.
- B. Kiumarsi, K. G. Vamvoudakis, H. Modares and F. L. Lewis, IEEE Trans. Neural Netw. Learn. Syst., 2018, 29, 21 Search PubMed.
- A. Shrestha and A. Mahmood, IEEE Access, 2019, 7, 26 Search PubMed.
- C. Bhatt, I. Kumar, V. Vijayakumar, K. U. Singh and A. Kumar, Multimed. Syst., 2021, 27, 599–613 CrossRef.
- Y. Lecun, L. Bottou, Y. Bengio and P. Haffner, Proc. IEEE, 1998, 86, 2278–2324 CrossRef.
- C. Chang and C. Lin, ACM Trans. Intell. Syst. Technol., 2011, 2, 27 Search PubMed.
- G. Biau and E. Scornet, Test, 2016, 25, 31 Search PubMed.
- A. Krizhevsky, I. Sutskever and G. E. Hinton, Commun. ACM, 2017, 60, 7 CrossRef.
-
S. Karen and Z. Andrew, Computer Science, arXiv, 2014, preprint, arXiv:1409.1556, DOI:10.48550/arXiv.1409.1556.
-
C. Szegedy, W. Liu, Y. Jia, P. Sermanet, S. Reed, D. Anguelov, D. Erhan, V. Vanhoucke and A. Rabinovich, IEEE Conference on Computer Vision and Pattern Recognition (CVPR), 2015, p. 9.
-
K. He, X. Zhang, S. Ren and J. Sun, IEEE Conference on Computer Vision and Pattern Recognition (CVPR), 2016, p. 9.
-
G. Huang, Z. Liu, L. van der Maaten and K. Q. Weinberger, IEEE Conference on Computer Vision and Pattern Recognition, 2017, p. 9.
-
A. Howard, M. Sandler, B. Chen, W. Wang, L. C. Chen, M. Tan, G. Chu, V. Vasudevan, Y. Zhu, R. Pang, H. Adam and Q. Le, IEEE International Conference on Computer Vision (ICCV), 2019, pp. 1314–1324.
- S. Ren, K. He, R. Girshick and J. Sun, Adv. Neural Inf. Process. Syst., 2015, 28, 9 Search PubMed.
-
K. He, G. Gkioxari, P. Dollar and R. Girshick, IEEE International Conference on Computer Vision (ICCV), 2017, p. 9.
-
J. Redmon, S. Divvala, R. Girshick and A. Farhadi, IEEE Conference on Computer Vision and Pattern Recognition (CVPR), 2016, p. 10.
-
O. Ronneberger, P. Fischer and T. Brox, Medical Image Computing and Computer-Assisted Intervention (MICCAI), 2015, vol. 9351, p. 8.
-
S. Zheng, J. Lu, H. Zhao, X. Zhu, Z. Luo, Y. Wang, Y. Fu, J. Feng, T. Xiang, P. H. S. Torr and L. Zhang, IEEE Conference on Computer Vision and Pattern Recognition (CVPR), 2021, p. 10.
- A. Onan, J. King Saud Univ. - Comput. Inf. Sci., 2022, 34, 20 Search PubMed.
- S. Hochreiter and J. Schmidhuber, Neural Comput., 1997, 9, 1735–1780 CrossRef CAS PubMed.
- I. J. Goodfellow, J. Pouget-Abadie, M. Mirza, B. Xu, D. Warde-Farley, S. Ozair, A. Courville and Y. Bengio, Adv. Neural Inf. Process. Syst., 2014, 27, 9 Search PubMed.
- D. P. Kingma and M. Welling, Found. Trends Mach. Learn., 2019, 4, 307–392 CrossRef.
- L. Yang, Z. Zhang, Y. Song, S. Hong, R. Xu, Y. Zhao, W. Zhang, B. Cui and M. Yang, ACM Comput. Surv., 2023, 56, 1–39 CrossRef.
- A. Vaswani, N. Shazeer, N. Parmar, J. Uszkoreit, L. Jones, A. N. Gomez, A. Kaiser and I. Polosukhin, Adv. Neural Inf. Process. Syst., 2017, 30, 5998–6008 Search PubMed.
-
A. Radford, K. Narasimhan, T. Salimans and I. Sutskever, Improving Language Understanding by Generative Pre-Training, 2018.
-
A. Dosovitskiy, L. Beyer, A. Kolesnikov, D. Weissenborn, X. Zhai, T. Unterthiner, M. Dehghani, M. Minderer, G. Heigold, S. Gelly, J. Uszkoreit and N. Houlsby, arXiv, 2020, preprint, arXiv:2010.11929, DOI:10.48550/arXiv.2010.11929.
- S. R. Dubey, S. K. Singh and B. B. Chaudhuri, Neurocomputing, 2022, 503, 92–108 CrossRef.
- A. Krogh, Nat. Biotechnol., 2008, 26, 195–197 CrossRef CAS PubMed.
- Y. Zhang and C. Ling, npj Comput. Mater., 2018, 4, 25 CrossRef.
- H. Lv and X. Chen, Nanoscale, 2022, 14, 6688–6708 RSC.
- J. M. Stokes, K. Yang, K. Swanson, W. Jin, A. Cubillos-Ruiz, N. M. Donghia, C. R. MacNair, S. French, L. A. Carfrae, Z. Bloom-Ackermann, V. M. Tran, A. Chiappino-Pepe, A. H. Badran, I. W. Andrews, E. J. Chory, G. M. Church, E. D. Brown, T. S. Jaakkola, R. Barzilay and J. J. Collins, Cell, 2020, 181, 9 CrossRef PubMed.
- K. Matula, F. Rivello and W. T. S. Huck, Adv. Biosyst., 2020, 4, e1900188 CrossRef PubMed.
- E. Z. Macosko, A. Basu, R. Satija, J. Nemesh, K. Shekhar, M. Goldman, I. Tirosh, A. R. Bialas, N. Kamitaki, E. M. Martersteck, J. J. Trombetta, D. A. Weitz, J. R. Sanes, A. K. Shalek, A. Regev and S. A. McCarroll, Cell, 2015, 161, 1202–1214 CrossRef CAS PubMed.
- A. M. Klein, L. Mazutis, I. Akartuna, N. Tallapragada, A. Veres, V. Li, L. Peshkin, D. A. Weitz and M. W. Kirschner, Cell, 2015, 161, 1187–1201 CrossRef CAS PubMed.
- J. G. Greener, S. M. Kandathil, L. Moffat and D. T. Jones, Nat. Rev. Mol. Cell Biol., 2022, 23, 40–55 CrossRef CAS PubMed.
- Y. Song, J. Zhao, T. Cai, A. Stephens, S. H. Su, E. Sandford, C. Flora, B. H. Singer, M. Ghosh, S. W. Choi, M. Tewari and K. Kurabayashi, Biosens. Bioelectron., 2021, 180, 113088 CrossRef CAS PubMed.
- J. Lamanna, E. Y. Scott, H. S. Edwards, M. D. Chamberlain, M. Dryden, J. Peng, B. Mair, A. Lee, C. Chan, A. A. Sklavounos, A. Heffernan, F. Abbas, C. Lam, M. E. Olson, J. Moffat and A. R. Wheeler, Nat. Commun., 2020, 11, 5632 CrossRef CAS PubMed.
- C. A. Patino, P. Mukherjee, E. J. Berns, E. H. Moully, L. Stan, M. Mrksich and H. D. Espinosa, ACS Nano, 2022, 16, 7937–7946 CrossRef CAS PubMed.
- K. Gardner, M. M. Uddin, L. Tran, T. Pham, S. Vanapalli and W. Li, Lab Chip, 2022, 22, 4067–4080 RSC.
- B. B. Yellen, J. S. Zawistowski, E. A. Czech, C. I. Sanford, E. D. Sorelle, M. A. Luftig, Z. G. Forbes, K. C. Wood and J. Hammerbacher, Sci. Adv., 2021, 7, f9840 CrossRef PubMed.
- A. M. White, Y. Zhang, J. G. Shamul, J. Xu, E. A. Kwizera, B. Jiang and X. He, Small, 2021, 17, e2100491 CrossRef PubMed.
- T. Aspert, D. Hentsch and G. Charvin, eLife, 2022, 11, e79519 CrossRef CAS PubMed.
- K. Huang, H. Matsumura, Y. Zhao, M. Herbig, D. Yuan, Y. Mineharu, J. Harmon, J. Findinier, M. Yamagishi, S. Ohnuki, N. Nitta, A. R. Grossman, Y. Ohya, H. Mikami, A. Isozaki and K. Goda, Lab Chip, 2022, 22, 876–889 RSC.
- Z. Göröcs, M. Tamamitsu, V. Bianco, P. Wolf, S. Roy, K. Shindo, K. Yanny, Y. C. Wu, H. C. Koydemir, Y. Rivenson and A. Ozcan, Light: Sci. Appl., 2018, 7, 66 CrossRef PubMed.
- S. Kim, M. H. Lee, T. Wiwasuku, A. S. Day, S. Youngme, D. S. Hwang and J. Y. Yoon, Biosens. Bioelectron., 2021, 188, 113335 CrossRef CAS PubMed.
- G. Graham, N. Csicsery, E. Stasiowski, G. Thouvenin, W. H. Mather, M. Ferry, S. Cookson and J. Hasty, Proc. Natl. Acad. Sci. U. S. A., 2020, 117, 3301–3306 CrossRef CAS PubMed.
- E. Yuzer, V. Dogan, V. Kilic and M. Sen, Sens. Actuators, B, 2022, 371, 132489 CrossRef CAS.
- Y. Zhang, M. A. Wright, K. L. Saar, P. Challa, A. S. Morgunov, Q. Peter, S. Devenish, C. M. Dobson and T. Knowles, Lab Chip, 2021, 21, 2922–2931 RSC.
- H. Sheng, L. Chen, Y. Zhao, X. Long, Q. Chen, C. Wu, B. Li, Y. Fei, L. Mi and J. Ma, Talanta, 2023, 266, 124895 CrossRef PubMed.
- C. Y. Cao, M. L. You, H. Y. Tong, Z. R. Xue, C. Liu, W. H. He, P. Peng, C. Y. Yao, A. Li, X. Y. Xu and F. Xu, Lab Chip, 2022, 22, 3837–3847 RSC.
- C. Honrado, A. Salahi, S. J. Adair, J. H. Moore, T. W. Bauer and N. S. Swami, Lab Chip, 2022, 22, 3708–3720 RSC.
- R. Zenhausern, A. S. Day, B. Safavinia, S. Han, P. E. Rudy, Y. W. Won and J. Y. Yoon, Biosens. Bioelectron., 2022, 200, 113916 CrossRef CAS PubMed.
- Z. H. Ren, Z. X. Zhang, J. X. Wei, B. W. Dong and C. K. Lee, Nat. Commun., 2022, 13, 3859 CrossRef CAS PubMed.
- A. John-Herpin, D. Kavungal, L. von Mucke and H. Altug, Adv. Mater., 2021, 33, e2006054 CrossRef PubMed.
- C. Wang, C. Wang, Y. Wu, J. Gao, Y. Han, Y. Chu, L. Qiang, J. Qiu, Y. Gao, Y. Wang, F. Song, Y. Wang, X. Shao, Y. Zhang and L. Han, Adv. Healthcare Mater., 2022, 11, e2102800 CrossRef PubMed.
- Y. Suzuki, K. Kobayashi, Y. Wakisaka, D. Deng, S. Tanaka, C. J. Huang, C. Lei, C. W. Sun, H. Q. Liu, Y. Fujiwaki, S. Lee, A. Isozaki, Y. Kasai, T. Hayakawa, S. Sakuma, F. Arai, K. Koizumi, H. Tezuka, M. Inaba, K. Hiraki, T. Ito, M. Hase, S. Matsusaka, K. Shiba, K. Suga, M. Nishikawa, M. Jona, Y. Yatomi, Y. Yalikun, Y. Tanaka, T. Sugimura, N. Nitta, K. Goda and Y. Ozeki, Proc. Natl. Acad. Sci. U. S. A., 2019, 116, 15842–15848 CrossRef CAS PubMed.
- K. Lee, S. E. Kim, J. Doh, K. Kim and W. K. Chung, Lab Chip, 2021, 21, 1798–1810 RSC.
- Y. X. Feng, Z. Cheng, H. C. Chai, W. H. He, L. Huang and W. H. Wang, Lab Chip, 2022, 22, 240–249 RSC.
- A. Hirotsu, H. Kikuchi, H. Yamada, Y. Ozaki, R. Haneda, S. Kawata, T. Murakami, T. Matsumoto, Y. Hiramatsu, K. Kamiya, D. Yamashita, Y. Fujimori, Y. Ueda, S. Okazaki, M. Kitagawa, H. Konno and H. Takeuchi, Lab Chip, 2022, 22, 3464–3474 RSC.
- N. Nitta, T. Sugimura, A. Isozaki, H. Mikami, K. Hiraki, S. Sakuma, T. Iino, F. Arai, T. Endo, Y. Fujiwaki, H. Fukuzawa, M. Hase, T. Hayakawa, K. Hiramatsu, Y. Hoshino, M. Inaba, T. Ito, H. Karakawa, Y. Kasai, K. Koizumi, S. Lee, C. Lei, M. Li, T. Maeno, S. Matsusaka, D. Murakami, A. Nakagawa, Y. Oguchi, M. Oikawa, T. Ota, K. Shiba, H. Shintaku, Y. Shirasaki, K. Suga, Y. Suzuki, N. Suzuki, Y. Tanaka, H. Tezuka, C. Toyokawa, Y. Yalikun, M. Yamada, M. Yamagishi, T. Yamano, A. Yasumoto, Y. Yatomi, M. Yazawa, D. Di Carlo, Y. Hosokawa, S. Uemura, Y. Ozeki and K. Goda, Cell, 2018, 175, 266–276 CrossRef CAS PubMed.
- A. Isozaki, H. Mikami, H. Tezuka, H. Matsumura, K. R. Huang, M. Akamine, K. Hiramatsu, T. Iino, T. Ito, H. Karakawa, Y. Kasai, Y. Li, Y. Nakagawa, S. Ohnuki, T. Ota, Y. Qian, S. Sakuma, T. Sekiya, Y. Shirasaki, N. Suzuki, E. Tayyabi, T. Wakamiya, M. Z. Xu, M. Yamagishi, H. C. Yan, Q. Yu, S. Yan, D. Yuan, W. Zhang, Y. Q. Zhao, F. Arai, R. E. Campbell, C. Danelon, D. Di Carlo, K. Hiraki, Y. Hoshino, Y. Hosokawa, M. Inaba, A. Nakagawa, Y. Ohya, M. Oikawa, S. Uemura, Y. Ozeki, T. Sugimura, N. Nitta and K. Goda, Lab Chip, 2020, 20, 2263–2273 RSC.
- L. Howell, V. Anagnostidis and F. Gielen, Adv. Mater. Technol., 2022, 7, 2101053 CrossRef CAS.
- S. Zhang, X. Liang, X. Huang, K. Wang and T. Qiu, Chem. Eng. Sci., 2022, 247 CAS.
- V. Anagnostidis, B. Sherlock, J. Metz, P. Mair, F. Hollfelder and F. Gielen, Lab Chip, 2020, 20, 889–900 RSC.
- C. J. Potter, Y. M. Hu, Z. Xiong, J. Wang and E. Mcleod, Lab Chip, 2022, 22, 3744–3754 RSC.
- L. L. Cong, J. Q. Wang, X. L. Li, Y. Tian, S. Z. Xu, C. Y. Liang, W. Q. Xu, W. G. Wang and S. P. Xu, Anal. Chem., 2022, 94, 10375–10383 CrossRef CAS PubMed.
- B. K. Ashley, J. Y. Sui, M. Javanmard and U. Hassan, Lab Chip, 2022, 22, 3055–3066 RSC.
- A. Shokr, L. Pacheco, P. Thirumalaraju, M. K. Kanakasabapathy, J. Gandhi, D. Kartik, F. Silva, E. Erdogmus, H. Kandula, S. Luo, X. G. Yu, R. T. Chung, J. Z. Li, D. R. Kuritzkes and H. Shafiee, ACS Nano, 2021, 15, 665–673 CrossRef CAS PubMed.
- J. Ko, N. Bhagwat, S. S. Yee, N. Ortiz, A. Sahmoud, T. Black, N. M. Aiello, L. Mckenzie, M. O'Hara, C. Redlinger, J. Romeo, E. L. Carpenter, B. Z. Stanger and D. Issadore, ACS Nano, 2017, 11, 11182–11193 CrossRef CAS PubMed.
- C. Nicoliche, R. de Oliveira, S. G. Da, L. F. Ferreira, I. L. Rodrigues, R. C. Faria, A. Fazzio, E. Carrilho, L. G. de Pontes, G. R. Schleder and R. S. Lima, ACS Sens., 2020, 5, 1864–1871 CrossRef CAS PubMed.
- J. Schutt, B. D. Sandoval, E. Avitabile, M. E. Oliveros, G. Milyukov, J. Colditz, L. G. Delogu, M. Rauner, A. Feldmann, S. Koristka, J. M. Middeke, K. Sockel, J. Fassbender, M. Bachmann, M. Bornhauser, G. Cuniberti and L. Baraban, Nano Lett., 2020, 20, 6572–6581 CrossRef PubMed.
- D. Z. Tang, M. Chen, Y. Han, N. Xiang and Z. H. Ni, Sens. Actuators, B, 2021, 336, 129719 CrossRef CAS.
- C. R. Oliver, M. A. Altemus, T. M. Westerhof, H. Cheriyan, X. Cheng, M. Dziubinski, Z. F. Wu, J. Yates, A. Morikawa, J. Heth, M. G. Castro, B. M. Leung, S. Takayama and S. D. Merajver, Lab Chip, 2019, 19, 1162–1173 RSC.
- Z. Gao, Y. Song, T. Y. Hsiao, J. He, C. Wang, J. Shen, A. Maclachlan, S. Dai, B. H. Singer, K. Kurabayashi and P. Chen, ACS Nano, 2021, 15, 18023–18036 CrossRef CAS PubMed.
- M. S. Manak, J. S. Varsanik, B. J. Hogan, M. J. Whitfield, W. R. Su, N. Joshi, N. Steinke, A. Min, D. Berger, R. J. Saphirstein, G. Dixit, T. Meyyappan, H. M. Chu, K. B. Knopf, D. M. Albala, G. R. Sant and A. C. Chander, Nat. Biomed. Eng., 2018, 2, 761–772 CrossRef CAS PubMed.
- F. Ellett, J. Jorgensen, A. L. Marand, Y. M. Liu, M. M. Martinez, V. Sein, K. L. Butler, J. Lee and D. Irimia, Nat. Biomed. Eng., 2018, 2, 207–214 CrossRef CAS PubMed.
- D. K. Singh, C. C. Ahrens, W. Li and S. A. Vanapalli, Lab Chip, 2017, 17, 2920–2932 RSC.
- Y. Y. Jiang, C. Lei, A. Yasumoto, H. Kobayashi, Y. Aisaka, T. Ito, B. S. Guo, N. Nitta, N. Kutsuna, Y. Ozeki, A. Nakagawa, Y. Yatomi and K. Goda, Lab Chip, 2017, 17, 2426–2434 RSC.
- V. Potluri, P. S. Kathiresan, H. Kandula, P. Thirumalaraju, M. K. Kanakasabapathy, S. Pavan, D. Yarravarapu, A. Soundararajan, K. Baskar, R. Gupta, N. Gudipati, J. C. Petrozza and H. Shafiee, Lab Chip, 2019, 19, 59–67 RSC.
- X. Guo, M. A. Khalid, I. Domingos, A. L. Michala, M. Adriko, C. Rowel, D. Ajambo, A. Garrett, S. Kar, X. X. Yan, J. Reboud, E. M. Tukahebwa and J. M. Cooper, Nat. Electron., 2021, 4, 615–624 CrossRef CAS.
- D. C. Braz, M. P. Neto, F. M. Shimizu, A. C. Sa, R. S. Lima, A. L. Gobbi, M. E. Melendez, L. Arantes, A. L. Carvalho, F. V. Paulovich and O. N. Oliveira, Talanta, 2022, 243, 123327 CrossRef CAS PubMed.
- L. B. Baker, M. S. Seib, K. A. Barnes, S. D. Brown, M. A. King, P. De Chavez, S. K. Qu, J. Archer, A. S. Wolfe, J. R. Stofan, J. M. Carter, D. E. Wright, J. Wallace, D. S. Yang, S. Liu, J. Anderson, T. Fort, W. H. Li, J. A. Wright, S. P. Lee, J. B. Model, J. A. Rogers, A. J. Aranyosi and R. Ghaffari, Adv. Mater. Technol., 2022, 7, 2200249 CrossRef.
- H. Chen, S. Kim, J. M. Hardie, P. Thirumalaraju, S. Gharpure, S. Rostamian, S. Udayakumar, Q. Lei, G. Cho, M. K. Kanakasabapathy and H. Shafiee, Lab Chip, 2022, 22, 4531–4540 RSC.
- K. L. Fetah, B. J. Dipardo, E. M. Kongadzem, J. S. Tomlinson, A. Elzagheid, M. Elmusrati, A. Khademhosseini and N. Ashammakhi, Small, 2019, 15, e1901985 CrossRef PubMed.
- S. Ekins, A. C. Puhl, K. M. Zorn, T. R. Lane, D. P. Russo, J. J. Klein, A. J. Hickey and A. M. Clark, Nat. Mater., 2019, 18, 435–441 CrossRef CAS PubMed.
- B. Desai, K. Dixon, E. Farrant, Q. Feng, K. R. Gibson, W. P. van Hoorn, J. Mills, T. Morgan, D. M. Parry, M. K. Ramjee, C. N. Selway, G. J. Tarver, G. Whitlock and A. G. Wright, J. Med. Chem., 2013, 56, 3033–3047 CrossRef CAS PubMed.
- G. Schneider, Nat. Rev. Drug Discovery, 2018, 17, 97–113 CrossRef CAS PubMed.
- D. Yang, Z. Yu, M. Zheng, W. Yang, Z. Liu, J. Zhou and L. Huang, Lab Chip, 2023, 23, 3961–3977 RSC.
- F. Grisoni, B. Huisman, A. L. Button, M. Moret, K. Atz, D. Merk and G. Schneider, Sci. Adv., 2021, 7, eabg3338 CrossRef CAS PubMed.
- Z. Su, J. He, P. Zhou, L. Huang and J. Zhou, Lab Chip, 2020, 20, 1907–1916 RSC.
- L. Huang, D. Y. Yang, Z. M. Yu, J. X. He, Y. Chen and J. H. Zhou, Chem. Eng. J., 2022, 450, 138267 CrossRef CAS.
- A. Astashkina, B. Mann and D. W. Grainger, Pharmacol. Ther., 2012, 134, 82–106 CrossRef CAS PubMed.
- S. N. Bhatia and D. E. Ingber, Nat. Biotechnol., 2014, 32, 760–772 CrossRef CAS PubMed.
- L. Broutier, G. Mastrogiovanni, M. M. Verstegen, H. E. Francies, L. M. Gavarro, C. R. Bradshaw, G. E. Allen, R. Arnes-Benito, O. Sidorova, M. P. Gaspersz, N. Georgakopoulos, B. K. Koo, S. Dietmann, S. E. Davies, R. K. Praseedom, R. Lieshout, J. Ijzermans, S. J. Wigmore, K. Saeb-Parsy, M. J. Garnett, L. J. van der Laan and M. Huch, Nat. Med., 2017, 23, 1424–1435 CrossRef CAS PubMed.
- X. D. Lin, S. Q. Wang, X. D. Yu, Z. G. Liu, F. Wang, W. T. Li, S. H. Cheng, Q. Y. Dai and P. Shi, Lab Chip, 2015, 15, 680–689 RSC.
- K. Paek, S. Kim, S. Tak, M. K. Kim, J. Park, S. Chung, T. H. Park and J. A. Kim, Bioeng. Transl. Med., 2023, 8, e10313 CrossRef CAS PubMed.
- Z. Zhang, L. Chen, Y. Wang, T. Zhang, Y. C. Chen and E. Yoon, Anal. Chem., 2019, 91, 14093–14100 CrossRef CAS PubMed.
- L. H. Chong, T. Ching, H. J. Farm, G. Grenci, K. H. Chiam and Y. C. Toh, Lab Chip, 2022, 22, 1890–1904 RSC.
- L. Xin, W. Xiao, L. P. Che, J. J. Liu, L. Miccio, V. Bianco, P. Memmolo, P. Ferraro, X. P. Li and F. Pan, ACS Omega, 2021, 6, 31046–31057 CrossRef CAS PubMed.
- Z. Ao, H. Cai, Z. Wu, L. Hu, A. Nunez, Z. Zhou, H. Liu, M. Bondesson, X. Lu, X. Lu, M. Dao and F. Guo, Proc. Natl. Acad. Sci. U. S. A., 2022, 119, e2080398177 Search PubMed.
- X. D. Lin, X. Duan, C. Jacobs, J. Ullmann, C. Y. Chan, S. Y. Chen, S. H. Cheng, W. N. Zhao, A. Poduri, X. Wang, S. J. Haggarty and P. Shi, Nat. Commun., 2018, 9, 5142 CrossRef PubMed.
- D. Mcintyre, A. Lashkaripour, P. Fordyce and D. Densmore, Lab Chip, 2022, 22, 2925–2937 RSC.
- E. P. Garcia, T. Duriez, J. M. Cabaleiro and G. Artana, Lab Chip, 2022, 22, 4860–4870 RSC.
- A. E. Siemenn, E. Shaulsky, M. Beveridge, T. Buonassisi, S. M. Hashmi and I. Drori, ACS Appl. Mater. Interfaces, 2022, 14, 4668–4679 CrossRef CAS PubMed.
- D. Stoecklein, K. G. Lore, M. Davies, S. Sarkar and B. Ganapathysubramanian, Sci. Rep., 2017, 7, 46368 CrossRef CAS PubMed.
- A. Lashkaripour, C. Rodriguez, N. Mehdipour, R. Mardian, D. Mcintyre, L. Ortiz, J. Campbell and D. Densmore, Nat. Commun., 2021, 12, 25 CrossRef CAS PubMed.
- J. Selberg, M. Jafari, J. Mathews, M. P. Jia, P. Pansodtee, H. Dechiraju, C. X. Wu, S. Cordero, A. Flora, N. Yonas, S. Jannetty, M. Diberardinis, M. Teodorescu, M. Levin, M. Gomez and M. Rolandi, Adv. Intell. Syst., 2020, 2, 2000140 CrossRef.
- N. H. Bhuiyan, J. H. Hong, M. J. Uddin and J. S. Shim, Anal. Chem., 2022, 94, 3872–3880 CrossRef CAS PubMed.
- J. Wang, N. Zhang, J. Chen, G. Su, H. Yao, T. Y. Ho and L. Sun, Lab Chip, 2021, 21, 296–309 RSC.
- B. Talebjedi, M. Heydari, E. Taatizadeh, N. Tasnim, I. Li and M. Hoorfar, Front. Bioeng. Biotechnol., 2022, 10, 878398 CrossRef PubMed.
- J. Su, X. Chen, Y. Zhu and G. Hu, Lab Chip, 2021, 21, 2544–2556 RSC.
- N. Wang, R. Liu, N. Asmare, C. H. Chu, O. Civelekoglu and A. F. Sarioglu, Lab Chip, 2021, 21, 1916–1928 RSC.
- O. J. Dressler, P. D. Howes, J. Choo and A. J. Demello, ACS Omega, 2018, 3, 10084–10091 CrossRef CAS PubMed.
- T. Abe, S. Oh-Hara and Y. Ukita, Biomicrofluidics, 2021, 15, 34101 CrossRef PubMed.
- A. Adadi and M. Berrada, IEEE Access, 2018, 6, 52138–52160 Search PubMed.
- B. Sanchez-Lengeling and A. Aspuru-Guzik, Science, 2018, 361, 360–365 CrossRef CAS PubMed.
- S. Zhu, T. Yu, T. Xu, H. Chen, S. Dustdar, S. Gigan, D. Gunduz, E. Hossain, Y. Jin, F. Lin, B. Liu, Z. Wan, J. Zhang, Z. Zhao, W. Zhu, Z. Chen, T. S. Durrani, H. Wang, J. Wu, T. Zhang and Y. Pan, Intelligent Computing, 2023, 2, 0006 CrossRef.
- F. Pedregosa, G. Varoquaux, A. Gramfort, V. Michel, B. Thirion, O. Grisel, M. Blondel, P. Prettenhofer, R. Weiss, V. Dubourg, J. Vanderplas, A. Passos, D. Cournapeau, M. Brucher, M. Perrot and E. Duchesnay, J. Mach. Learn. Res., 2011, 2825–2830 Search PubMed.
-
M. Abadi, P. Barham, J. Chen, Z. Chen, A. Davis, J. Dean, M. Devin, S. Ghemawat, G. Irving, M. Isard, M. Kudlur, J. Levenberg, R. Monga, S. Moore, D. G. Murray, B. Steiner, P. Tucker, V. Vasudevan, P. Warden, M. Wicke, Y. Yu and X. Zheng, USENIX Association, arXiv, 2016, preprint, arXiv:1605.08695, DOI:10.48550/arXiv.1605.08695.
-
A. Paszke, S. Gross, F. Massa, A. Lerer, J. Bradbury, G. Chanan, T. Killeen, Z. Lin, N. Gimelshein, L. Antiga, A. Desmaison, A. Köpf, E. Yang, Z. DeVito, M. Raison, A. Tejani, S. Chilamkurthy, B. Steiner, F. Lu, J. Bai and S. Chintala, arXiv, 2019, preprint, arXiv:1912.01703, DOI:10.48550/arXiv.1912.01703.
- Github, https://github.com/.
Footnote |
† Jianhua Zhou and Jianpei Dong contributed equally to this work. |
|
This journal is © The Royal Society of Chemistry 2024 |
Click here to see how this site uses Cookies. View our privacy policy here.