DOI:
10.1039/D2SD00230B
(Tutorial Review)
Sens. Diagn., 2023,
2, 559-581
Developing an electrochemical sensor for the in vivo measurements of dopamine
Received
23rd December 2022
, Accepted 2nd March 2023
First published on 22nd March 2023
Abstract
Measurements inside the human body are complicated. Here, we provide a short introduction of the main requirements for the successful in vivo determination of dopamine concentrations, together with a discussion of how the type of the measurements, short-term vs. long-term and dynamic vs. basal measurements, affects what is expected from the sensor material. Next, we provide a step-by-step guide for characterising electrode performance and performing in vivo measurements with practical tips. Finally, we discuss the typical approaches for overcoming the challenges set by the environment.
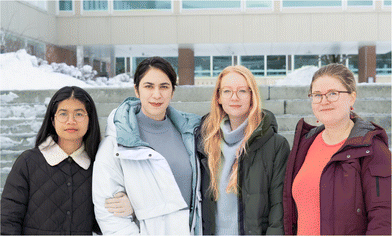 Naela Delmo, Bahar Mostafiz, Johanna Suni and Emilia Peltola | The Materials in Health Technology research group started at the University of Turku in October 2021. Emilia Peltola is an Associate Professor in Materials Engineering, focusing on understanding the interface of materials in biomedical applications and developing sensor technologies for health care. Naela Delmo, Bahar Mostafiz, and Johanna Suni are doctoral candidates in the research group. Naela Delmo completed her joint MSc-MSc (Tech) degree in the field of Analytical Chemistry from the University of Tartu and Åbo Akademi University in 2022, specialising in electrochemistry and sensors. Bahar Mostafiz has also specialised in electrochemical sensor fabrication in her analytical chemistry master studies at Al-Zahra University in 2020. Johanna Suni completed her MSc in the field of Animal Physiology and Genetics from the University of Turku in 2022. |
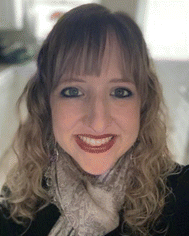 Ashley Ross | Ashley Ross is an Associate Professor of Chemistry at the University of Cincinnati. Her research interests include investigations into the electrode–analyte interface, development of new methods to measure neurochemicals in the brain and immune system, and the development of microengineered platforms to probe neurochemical signaling along the gut–brain axis. Ashley has received the Alfred P. Sloan Fellowship and was recently named a “Top 40 under 40” in the Analytical Scientist. She currently serves on the Advisory Board for the Analyst and RSC Chemical Reviews, and is on the Board of Directors for the Society of Electroanalytical Chemistry. |
1. Requirements for successful detection in vivo
Since its discovery as a neurotransmitter in the 1950s,1 neuroscientists have tried different means of accurate and convenient determination of dopamine (DA), other neurotransmitters, and their metabolites. DA is a neurotransmitter that plays essential roles in motor control, motivation, arousal, reinforcement, and reward systems. Abnormal DA transmission has been connected with several neurological and psychiatric disorders, e.g., Parkinson's disease, schizophrenia, and Huntington's disease.2 The accurate measurement of DA would provide a better understanding of these diseases and a tool to follow up the output of the treatments. Electrochemistry offers a cheap, in vivo-compatible option for the real-time detection of DA.
As a catecholamine, DA (3,4-dihydroxyphenethylamine) is an electroactive compound that can be directly detected by electrochemical methods as they undergo redox reactions at the surface of an electrode. DA oxidises in a 2e− 2H+ transfer process to form dopamine quinone (DAQ), as shown in Fig. 1. In its neutral form, DAQ undergoes intramolecular cyclisation via 1,4-Michael addition forming the easily oxidisable leucodopaminechrome (LDAC). This is followed by another 2e− oxidation reaction forming dopaminechrome (DAC). The overall reaction pathway follows the widely used ECE model (electron transfer – chemical reaction – electron transfer).3 A different mechanism, where DAQ oxidises LDAC to form DAC, is referred to as the ECC model.4 DAC is a reactive molecule that easily rearranges into 5,6-dihydroxyindole and further oxidised into 5,6-indolequinone. These last two DA oxidation products were found to have a high tendency to polymerise.5
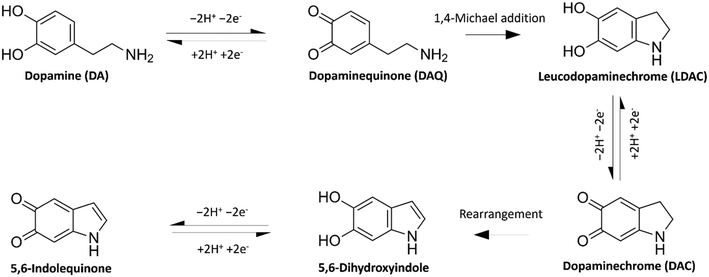 |
| Fig. 1 Oxidation pathway of dopamine. | |
The main requirements for the successful in vivo determination of DA concentrations include sensitivity, selectivity, temporal resolution, spatial resolution, and stability. However, the precise goal of the measurement defines the relevance of the requirements. For example, for the dynamic measurement of DA concentrations, the temporal resolution is essential, but the selectivity towards downstream metabolites, which concentrations can be considered stable compared to DA concentrations, becomes less critical. For short measurements, stability and biofouling are still issues to be addressed, but the implant integration into the host tissue does not have time to occur. On the other hand, for the measurement of basal concentrations of DA and long-term measurements, the temporal resolution is less critical, but the selectivity is of utmost importance.
1.1 Selectivity and sensitivity
International Union of Pure and Applied Chemistry (IUPAC) defines selectivity as the degree to which a compound interferes with analyte detection, while sensitivity is a numerical expression of how the signal changes with analyte concentration.6 In developing a sensor for the in vivo detection of DA, one must estimate the basal DA concentration in the extracellular brain fluid, know what other electroactive components are present, and understand how these compounds interfere with the detection of DA.1 In practice, it is desirable to have a linear signal response in the physiological range of DA concentrations.
During the 1970s, the group of Adams introduced the possibility of detecting neurotransmitters in vivo using electroanalytical techniques such as voltammetry.7–9 However, this promising and inspiring discovery did not come without challenges. The brain is a complex and heterogeneous structure, to begin with. At the initial stages of utilising voltammetry in neurophysiology, the detection limit was high, typically in the micromolar range.7 Sensitivity was a considerable concern due to the small and fluctuating concentration of DA in the extracellular brain fluid. Another significant issue is selectivity due to the abundance of electroactive species that oxidise within the set potential window. Selectivity is not achieved when the oxidation potentials are too close to each other, producing poorly resolved peaks or a single peak corresponding to multiple species.7,9
This was primarily addressed using microelectrodes and taking advantage of the aforementioned brain heterogeneity. In certain brain regions, such as the striatum, DA concentrations can be adequately high and even within the detection range of electroanalytical techniques.9 The striatum is composed of the caudate nucleus and another brain structure called the nucleus accumbens.10 One promising approach is to implant the electrodes as close as possible to these brain regions with minimal interfering compounds and a high estimated concentration of the target DA analyte.11 Almost all the succeeding in vivo measurements were performed in these structures. The cerebrospinal fluid (CSF) that carries different molecules from the brain, such as neurotransmitters, is also a typical sample for in vivo measurements. Changes in their respective concentrations were related to neuronal activity.7
Other analytical techniques that offer good sensitivity and selectivity helped establish concentrations of DA and its common interferences and are good reference points for developing electrochemical sensors. When sampling is strategically done, DA can be quantified using different analytical techniques, such as radioenzymatic assays.12,13 The need for radioactive labelling was eliminated by developing procedures for liquid chromatography with electrochemical detection (LCED), which allowed the simultaneous detection of multiple analytes. Small volumes of extracellular brain fluid were obtained for such analyses by the perfusion “push–pull” method.13–16 This was later modified into the microdialysis perfusion method, which allowed a further improvement in sample clean-up leading to better selectivity.17 Initial in vivo experiments using microdialysis coupled with LCED emphasised the excellent sensitivity and selectivity contributed by the good chromatographic resolution prior to electrochemical detection.14,18 With this, extracellular DA concentrations of 20–30 nM (ref. 19) and 10–15 nM,20 were detected in the rat striatum. In the caudate nucleus, as low as 30 nM (ref. 11) extracellular DA concentrations were recorded, while 4 nM (ref. 21) and 10 nM (ref. 22) were detected at the nucleus accumbens of rats.
Unfortunately, the dimensions of the dialysis probes are usually in the millimetre range, which makes it quite challenging to access smaller brain regions.11 Moreover, the perfusion rates are typically slow to ensure equilibrium inside and outside the probe, leading to a good approximation of DA in the extracellular fluid. The sampling time generally is in the minute scale.11,20 More recently, a one-minute temporal resolution for DA monitoring was reported.23 Still, better temporal resolution is desired to complement neural stimulation experiments, considering the release pattern of DA is in the millisecond time scale (see Table 1). Temporal and spatial resolutions are another critical factor to consider in DA detection, which will be discussed in more detail in the following sections.
Table 1 Physiological concentrations and release pattern of dopamine and its common interferents
Compound |
Charge at physiological pH |
Physiological concentration/μM |
Approximate oxidation potential in vivo (using a carbon paste electrode vs. Ag/AgCl)/V (ref. 9 and 46) |
Release pattern |
Dopamine |
+ |
0.004–0.030 |
+0.2 |
Neurotransmitter, rapid (ms) release |
Serotonin |
+ |
<0.1 |
+0.8 |
Neurotransmitter, rapid (ms) release |
Ascorbic acid |
— |
100–500 |
+0.2 |
Nutrient not synthesised by primates, slow |
Uric acid |
— |
17–30 |
+0.3 |
Purine metabolite, slow |
3,4-Dihydroxyphenylacetic acid |
— |
0.5–30 |
+0.2 |
Dopamine metabolite, slow |
Homovanillic acid |
— |
0.6–26.6 |
+0.5 |
Catecholamine metabolite, slow |
1.1.1 Interferents.
When placed in a biological medium for in vivo determination, the sensory surface is exposed to various biomolecules and interfering agents. Proteins, ions, and mimicking compounds are some examples. At physiological pH, the amino group of DA is protonated, giving the molecule a positive charge (see Fig. 2a). Aside from DA detection, LCED was also used in listing possible interfering compounds in the extracellular brain fluid and establishing their concentrations. Ascorbic acid (AA), uric acid (UA), 3,4-dihydroxyphenylacetic acid (DOPAC), and homovanillic acid (HVA) were some of the electroactive components identified in the extracellular brain fluid, and the measured concentrations, typically 2–3 orders of magnitude larger than DA parallel those determined by voltammetry.7,11,14,17 Based on the present co-existing molecules, in the case of sensors intended for DA detection in the brain, AA and UA can tamper with the electroanalytical signal more than the rest due to similarities with DA in terms of 3D structure and electrochemical behaviour. Furthermore, the oxidation potentials of AA and UA often overlap with DA, and because their concentration is considerably higher than DA, the effect on the electrical current can be problematic. This issue is not always addressed; low AA and UA concentrations are used in selectivity studies. Fig. 3 gives an example of selective DA detection in the presence of UA and AA at their physiological concentrations.
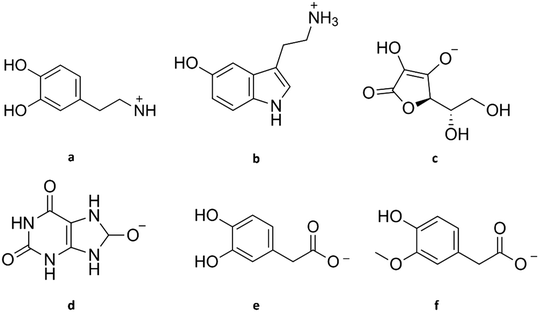 |
| Fig. 2 Structure of dopamine and its common interferents at physiological pH. (a) Dopamine cation, (b) serotonin cation, (c) ascorbate, (d) urate, (e) 3,4-dihydroxyphenylacetate, (f) homovanillate. | |
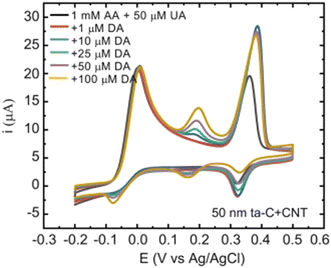 |
| Fig. 3 Cyclic voltammograms showing the selectivity of the 50 nm tetrahedral amorphous carbon/carbon nanotube electrodes. Scan rate 50 mV s−1. Reproduced from ref. 24 with permission from Elsevier, copyright 2018. | |
Most of these interferents (AA, UA, DOPAC, and HVA) are metabolites that are not rapidly released. Thus, the electrode's selectivity towards these compounds is not critical in fast-scan cyclic voltammetry (FSCV), which focuses on dynamic changes of DA. However, when one considers the basal concentrations or long-term measurements, the selectivity of all interferents becomes critical. This section discusses how the interfering compounds affect DA detection, and Table 1 provides a summary of their electrochemical properties, physiological concentrations, and release pattern.
Serotonin, or 5-hydroxytryptamine (5-HT), is a tryptophan-derived neurotransmitter that can be found in almost all regions of the brain. Like DA, its primary amine nitrogen accepts a proton, making it cationic at physiological pH (see Fig. 2b) and giving it hydrophilicity. Moreover, DA and serotonin have similar regulatory proteins, metabolism, and temporal and spatial scales, e.g., sub-second release and uptake.25
The two electroactive neurotransmitters also have similar oxidation potentials (see Table 1), and the oxidation products of serotonin quickly polymerise and coat the electrode surface,26 making selective DA detection challenging. However, the reduction potential of serotonin is about 200 mV more positive than DA, so their reduction peaks can be discriminated using FSCV, which allows monitoring of DA and serotonin concentrations in the rat striatum after stimulation.27 However, the use of FSCV for serotonin measurements was only established years after DA, and its concentration is estimated to be typically less than 100 nM, even after stimulation.25
Ascorbic acid (AA) is a water-soluble vitamin that functions as an antioxidant, serves as a cofactor in several enzymatic reactions, and participates in some synthetic pathways in higher primates.28 It exists as the anion ascorbate (see Fig. 2c) and is a powerful reducing agent at physiological pH.29 Because of its high concentration and similar oxidation potential to DA, AA is believed to be one of the most significant interferences in DA detection in vivo. Due to the high physiological concentration, AA occupies many of the sensors' active sites.30 Moreover, AA can indirectly contribute to the measured current in DA determinations by reducing DAQ back to DA.31 This regeneration step is typically not considered in published papers.
Extracellular AA concentration was estimated by voltammetry to be around 100–500 μM, depending on the location.29 Using carbon fibre microelectrodes (CFME) and FSCV, 200–400 μM AA was estimated in rat extracellular fluid and 500 μM in CSF.32 Succeeding experiments showed good agreement with these values.33–36 However, it was proven very recently that it is possible to perform DA measurements in vitro without considering AA as an interference. The rapid decay of AA in a cell culture medium was investigated, with an estimated half-life of about two hours.37
Uric acid (UA) is the end product of purine catabolism in higher primates due to the lack of the enzyme uricase.38 It is a heterocyclic organic compound and a weak acid that exists mainly as urate (see Fig. 2d) at physiological pH.39 Multiple studies have estimated the basal concentration of UA in the brain with a rough average of 20 μM using chemiluminescence assay,34 enzymatic method,40 and high-performance liquid chromatography (HPLC) with UV-detection.41,42
3,4-Dihydroxyphenylacetic acid (DOPAC) is a major product of DA metabolism from the reaction catalysed by monoamine oxidase.43 Like AA, DOPAC is a weak acid, and it can be found as a negatively charged species (3,4-dihydroxyphenylacetate, see Fig. 2e) at physiological pH.11 Using differential pulse voltammetry (DPV) and electrochemically pre-treated CFME implanted in the striatum of rats, a DOPAC concentration of 22 μM was detected.43 Like other metabolites in the brain, its concentration is affected by the brain region where the measurement was done. By performing microdialysis and HPLC, 0.5, 3, and 7 μM DOPAC was estimated in the frontal cortex, striatum, and nucleus accumbens of rats, respectively.44 Succeeding experiments produced agreeable results.20,21 Due to its high concentration and similar oxidation potential, it is challenging to distinguish DA from DOPAC without a powerful separation technique such as HPLC. In earlier studies, DOPAC synthesis was prevented by introducing a monoamine oxidase-inhibiting drug such as pargyline.45
Homovanillic acid (HVA) is another metabolite of DA, a weak acid that is negatively charged (homovanillate) at physiological pH (see Fig. 2f). In earlier measurements where electrodes were placed in a fluid cavity instead of being directly implanted in the brain tissue, spatial separation and time delay led to the detection of HVA instead of DA.
1.2 Spatial resolution
Spatial resolution is critical for obtaining information about neuronal communication. For the measurement of cellular dynamics, a spatial resolution in the scale of 10–100 s μm is required. A resolution of 10–100 μm enables the measurement of neuronal communication between individual cells, while a resolution of 100–500 μm allows communication between bundles of cells to be recorded.47 To measure synaptic release events, it is necessary to consider vesicles with a size of ca. 50 nm, with distances between synapses (site of release and uptake) in the single-digit nanometre range.47,48
1.3 Temporal resolution
The goal of the measurement defines the relevance of the temporal resolution of the sensor. It is of utmost importance for recording dynamic changes in DA levels but not so relevant in basal DA levels. To put requirements for the temporal resolution in context, we will briefly describe the operation of dopaminergic neurons. Dopaminergic neurons are a group of neurons in the midbrain responsible for synthesising and releasing DA in mammals. Predominantly, they are localised in 3 major groups, the retrobulbar field (RRF-A8), the substantia nigra pars compacta (SNc-A9), and the ventral tegmental area (VTA-A10).49,50 The latter two have been the subject of most in vivo DA studies. DA releases initiated from mentioned areas are sent to the dorsal and ventral striatum and other brain sub-regions. Then two types of signalling are observed, phasic and tonic.51 These release patterns have distinct waveforms showing slow rates for tonic firing (2–10 Hz) and rapid, phasic bursts (10–30 Hz), which periodically interrupt the tonic releases.52,53 The ever-varying concertation in the multitudes of brain regions across individuals makes it challenging to pinpoint a specific release and intake speed. Therefore, a range of 0.2 to 10 Hz firing pace can be reported in the midbrain neurons as the regular release rates.54 Axonal DA release is remarkably rapid, occurring within sub-seconds, and the exocytosis is directed to spaces opposite postsynaptic receptors. When released into the synaptic cleft, most DA molecules are in taken within less than a millisecond due to fast diffusion.55–57
1.4 Stability
The electrode properties can already be affected by storage. For example, in FSCV measurements, a freshly trimmed electrode is known to improve analyte adsorption and sensitivity. Therefore, in-depth surface characterisation should be compared on freshly made vs. stored electrodes when developing novel electrode materials. Grave concerns for the electrode's stability are the fouling and host response, which will be discussed in greater detail below.
1.4.1 Electrochemical fouling.
Electrochemical fouling means the formation of an insulating film on the electrode as a sequence of the reaction used for the detection. This electrochemical fouling is typical for DA as its oxidation products are very reactive. Under a proper environment (pH >7.5, DA concentrations higher than 2 mg mL−1), polydopamine formation occurs spontaneously.58 The polydopamine formation results in signal attenuation and compromises the quality of the measurements, as the thick layer may prevent or hinder electron transfer. In addition, the electrochemical oxidation of DA is an inner-sphere reaction involving surface adsorption, which the fouled layer may block.
Liu et al. proposed several mechanisms for the formation of polydopamine, but the precise molecular mechanism is still under scientific debate. DA undergoes a complex redox process, generating a series of intermediates during the polymerisation and reaction processes. Consequently, many functional groups, including planar indole units, amino groups, carboxylic acid groups, catechol or quinone functions, and indolic/catecholic π-systems, are integrated into polydopamine.59 As a result, polydopamine has a robust adhesion capability to virtually all surfaces.58
When comparing the electrooxidation of DA on the unmodified surfaces of five different classes of carbon electrodes (glassy carbon, oxygen-terminated polycrystalline boron-doped diamond (BDD), edge plane pyrolytic graphite, basal plane pyrolytic graphite, and the basal surface of highly oriented pyrolytic graphite), the polycrystalline BDD was the least sensitive material to DA surface fouling. Polycrystalline BDD also showed sluggish DA reaction kinetics, while highly oriented pyrolytic graphite, which gave the best voltammetric signal to DA, was very susceptible to blocking by dopaminergic products.60 Heterogeneous surface chemical or geographical modification can offer the best approach to reducing the risk of fouling while maintaining strong voltammetric signals. For example, heterogeneous amendment of carbon electrodes with carboxyl-amine functionalities protects against the formation of an insulating polydopamine layer while retaining the ability to detect DA.61 The benefits of the heterogeneous termination are proposed to be due to the electrostatic repulsion between amino-functionalities and DA. Another study suggests that a carbon nanotube (CNT) fibre microelectrode is less prone to DA fouling than a typical CFME due to a decreased binding affinity of the insulating film to the CNT fibre surface.62
1.4.2 Biofouling.
Another type of fouling is biofouling, which is the undesirable accumulation of proteins and other biological molecules on the sensor surface. Typically, the adsorption of different molecules on the electrode surface interferes with the DA adsorption process, decreasing the sensitivity of the electrode in orders of magnitude and significantly increasing the ΔEp. Interestingly, biofouling affects inner and outer sphere redox probes differently.63 To preserve sufficient functionality of the electrode in a biological environment, surfaces that promote the spreading of the proteins, resulting in completely blocking the access of the probe to the inner sphere, should be avoided.
The combined effect of biological and electrochemical fouling is critical for the evaluation of the functionality of a sensor.64
1.4.3 Scar formation.
The main reason for the sensor failure in vivo is considered an electrode fouling which can induce uncontrolled host response.65 Host response causes a foreign body reaction (FBR), a response to any materials implanted in the body.66 Primarily, FBR is caused by host cell recognition of the biomaterial surface. The problem with host response and biosensors is glial scar formation around the sensor and chronic inflammation in the target tissue, which disrupts the interface between the implant and the target tissue.67 The capsule around the sensor leads to reduced analyte diffusion and perfusion to implanted sensors, severely affecting the analytical characteristics of a sensor.
Nervous tissue consists of neurons and glial cells. Glial cells are supporting cells protecting neurons in the central nervous system (CNS) and the peripheral nervous system. In the CNS, glial cells include microglia, astrocytes, oligodendrocytes and ependymal cells. Due to their capsulation formation, microglia and astrocytes are the most studied glial cells in response to brain implants.67 Elsewhere in the body, the study of scar formation is focused on fibroblasts. However, fibroblasts are restricted only to vascular and meningeal niches in the brain tissue, so their participation in glial scarring is partly unclear.
Acute phase reaction begins immediately after sensor implantation due to tissue damage and the rush of inflammatory cells to the area.67,68 Different adhesion proteins, many of which have arrived from blood, become non-specifically adsorbed to the sensor's surface, followed by the recruitment of inflammatory cells.68,69 The first arriving inflammatory cells are neutrophils. Together with chemical signals resulting from blood clotting and mast cell activation, neutrophils attract monocytes into the area. Neutrophils are soon beginning to be replaced by monocytes.68 In target tissue, monocytes differentiate into macrophages, in brain microglial cells, which become the dominant cell type around the biomaterial.70,71
Up to this stage, the inflammatory response to device implantation is like any injury. However, as microglia populate the implantation site, this initial acute inflammatory response develops into FBR.68 Microglial cells recognise the implant as foreign through the adsorbed protein layer and try to degrade the foreign material. Activated microglial cells near the sensor start to extend processes toward the implant surface, adhering through integrins.68,72 However, microglial cells cannot phagocytose the entire sensor due to its large size, so the degradation fails, and they start to cover and form a thin cellular sheath encapsulating the sensor. Later astrocytes are also activated, and approximately 2–3 weeks after implantation, they begin to create a cellular sheath around microglia.72,73 Little by little, glial cells form a physical barrier, a scar-like capsule, around the sensor surface with lamellipodia to isolate it from the rest of the body.67,70,73 This cellular sheath encapsulation is called the chronic phase of FBR (Fig. 4).
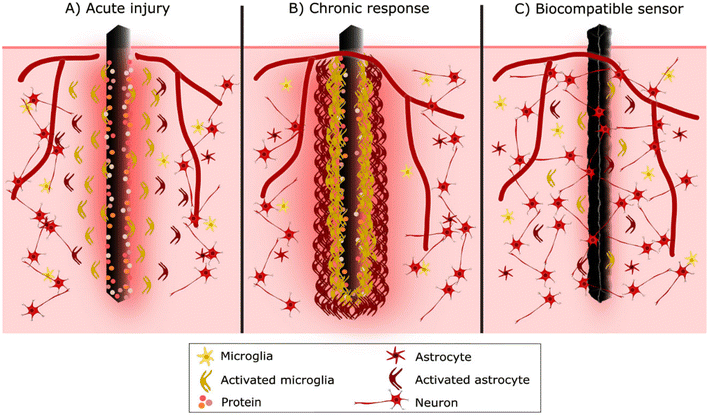 |
| Fig. 4 A visualisation of a foreign body reaction and glial scar formation in response to neural sensor implantation. A) Acute phase starts immediately after sensor implantation by protein absorption and cellular activation. B) In the chronic phase, microglial cells and astrocytes form a scar-like capsule to isolate the sensor from the rest of the body. C) The purpose of a biocompatible sensor is to reduce tissue trauma, foreign body reactions and glial scar formation while increasing neural cell adhesion and growth. | |
2. Basic principle of electrochemical measurement
CV is a fast, versatile and relatively simple method for investigating electrochemical reactions and electrode materials. In CV, the working electrode's potential is scanned linearly between an initial and switching potential. The direction of the linear scan is reversed at the switching potential, and this potential range is then scanned for several cycles. The scan rate (υ) indicates the speed at which the potential changes. The oxidation and reduction of the desired electroactive species (i.e., DA) should lie within the measurement window. The forward or anodic scan causes the electroactive species to oxidise, while the reverse or cathodic scan causes it to reduce back to the initial state. Both reactions are observed as current peaks in the cyclic voltammograms since electrochemical reactions involve the transfer of electrons.
The fast temporal resolution of CV makes it the most frequently used technique for investigating rapid neurotransmission events. However, other methods, such as DPV, offer higher selectivity.46 DPV and square-wave voltammetry (SWV) are forms of linear sweep voltammetry. DPV involves a series of regular voltage pulses superimposed on the potential linear sweep or stairsteps, while SWV combines a square wave and staircase potential applied to a stationary electrode. For the in vivo measurements, FSCV, which is CV with a very high scan rate (up to 1 × 106 V s−1), is commonly applied.
There is an excellent practical beginner's guide to CV74 and a more specialised guide for in vivo FSCV.75
3. Characterising electrode performance
The process of fabrication and evaluation of an electrochemical sensor requires multiple assessments. One of the earlier steps is overseeing how is the initial electrode performance and how the modifications and measurement techniques influence the electrochemical response. It is strongly recommended that in the case of in vivo sensors, biocompatibility and electrode response in various biological mediums be investigated as well. The upcoming passages will provide a comprehensive view of the mentioned matters.
3.1 Electrochemical characterisation in saline
The measurements are typically started in buffer solutions when developing a new electrode material. Electrochemical properties such as pseudocapacitance, potential window and open circuit potential can be defined in various buffers, with pH varying from acidic to alkali. In addition, the heterogeneous electron transfer kinetics are often determined for outer-sphere redox probes, such as hexaammineruthenium(III) chloride and iridium chloride.
The solvent window of an electrolyte is defined as the potential range between the oxygen evolution reaction and hydrogen evolution reaction, which occur at the cathodic and anodic ends, respectively. The analytical potential window is defined using a self-chosen threshold current value and is, by definition, narrower than the solvent window. The purpose is to determine the window where the analyte signal can be precisely measured.
Pseudocapacitance is a faradaic property that arises on electrode surfaces during electrochemical reactions. Several electrochemical processes contribute to pseudocapacitance, including adsorption, intercalation and surface redox reactions. The amount of pseudocapacitance depends on the surface area, material and structure of the electrodes, and it helps compare complex surface structures. The numerical value of the pseudocapacitance can be determined from the difference between anodic and cathodic current densities (defined from the measured current dividing it by the geometric area of the electrode) at different scan rates, and the equation Δj = 2 × C × v, where j is the current density, C the pseudocapacitance and v the scan rate.
Peak potential separation (ΔEp) and the ratio of the oxidation to the reduction peak current (Ip,a/Ip,c) are often defined to characterise the CV. In addition, these values help define the reaction type, whether it is reversible, quasi-reversible or irreversible. In the first stage of testing, the DA measurements are typically done in phosphate-buffered saline (PBS, pH 7.4) to mimic the pH in the body. Typical values to be defined are the limit of detection (LOD) and sensitivity of the sensor. One can determine the LOD as the lowest amount of the analyte that may be detected to produce a response significantly different from that of a blank. However, the LOD is more commonly calculated using the equation LOD = 3.3 × σ/S (where σ is the standard deviation of the blank CVs and S the sensitivity). For the definition of sensitivity, a concentration series of DA is measured. A calibration curve is defined from the oxidation current versus concentration plot. The slope in the linear part of the calibration divided by the electrode area gives the sensitivity of the electrode. It is desirable to have a linear response in the physiologically relevant concentration range.
As DA is an inner sphere redox probe, and the adsorption and electron-transfer kinetics are strongly sensitive to the structure and composition. Many surfaces show self-catalysis of DA oxidation, in which adsorbed DA catalyses the oxidation of solution-phase DA, likely facilitated by H-bonding interactions.76 Nonetheless, a pristine (albeit oxidized) electrode surface, such as pre-treated glassy carbon, can show rapid kinetics without any observable adsorption.77 Interestingly, nanostructured surfaces appeared to increase the time required for DA to adsorb on available surface sites.78 Importantly, both surface nanostructures and chemistry are important for adsorption and charge transfer kinetics.77 Therefore, a comprehensive characterization after surface modification should be a standard protocol. Unfortunately, the effect of surface treatment on different surface characteristics is often neglected. For example, when the surface is structured, only surface morphology changes and not chemistry is measured. Similarly, only surface chemistry is characterized after chemical treatment, and changes in structure are not considered.
Whether the reaction kinetics is defined by diffusion or adsorption can be evaluated from the logarithmic plot of oxidation current versus scan rate. Fig. 5 provides an example of the measurement of the DA concentration series and the definition of the reaction kinetics. The slope for a diffusion-controlled process should be 0.5, and for an adsorption-controlled process, it should be 1. In addition, a computational approach to the DA–material system can provide more detailed insight into the interactions. Density functional theory is a computational quantum mechanical modelling method used to investigate the electronic structure of many-body systems. For example, in recent years, several publications have utilised density functional theory for studying the DA–carbonaceous material system.61,79–81 However, it should be noted that calculations carried out on graphene or diamond only cannot be directly generalised for all carbonaceous surfaces.
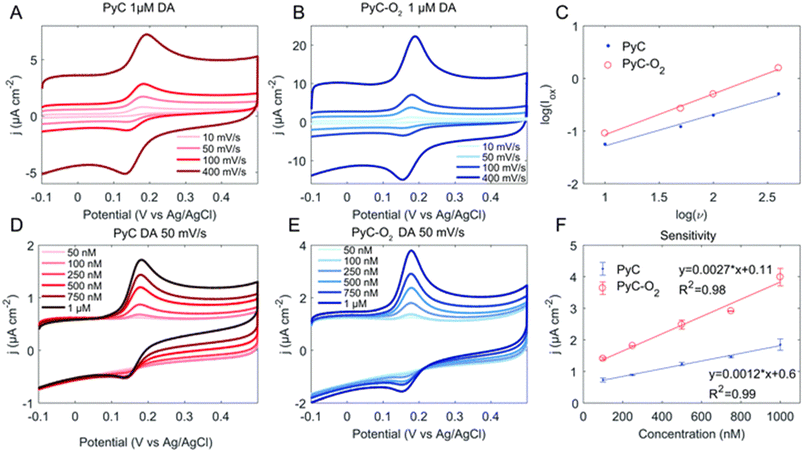 |
| Fig. 5 Cyclic voltammogram of the pyrolytic carbon (PyC) (A and D) and PyC–O2 (B and E) electrodes in DA with varying scan rates (A and B) and concentrations (D and E). Panel (C) shows the linear dependence of peak oxidation current and scan rate and (F) the sensitivity of the electrodes. Reprinted from ref. 82 with permission from the Royal Society of Chemistry. | |
For the measurement of selectivity, DA is often measured with AA and UA. In the typical experiment, the concentration of two of the three is kept constant, while one is varied. As a result, it is desirable to obtain a sufficient peak-to-peak separation, which is critical for selectivity. In the selectivity measurements, it is essential to perform the measurements at the physiological concentrations of each compound (Table 1), so that the selectivity measurements have relevance to the application. It is to be noted that the interferents, like AA and UA, have orders of magnitude higher concentrations than DA.
The electrochemical fouling of DA can be measured to evaluate the DA–material interaction further. For example, the change in the rate of electron transfer kinetics at the fouled electrode surface can be determined by scanning electrochemical microscopy approach curves.61 In addition, scanning electrochemical microscopy imaging allowed the determination of different morphologies, such as continuous layers or islands, of insulating material.
3.2 Measurements in a biological environment
None of the above-mentioned measurements considers the biological environment. Literature is more focused on ways to prevent biofouling, and no standardized methods for the measurements in a biological environment exist. Considering that this paper aims to provide information regarding in vivo determination of a neurotransmitter, the biological medium we should focus on is a cerebrospinal fluid which has quite a different composition than blood or plasma. However, limited studies use this medium, and those studies mostly strategize on developing antifouling techniques rather than tuning biofouling. Nevertheless, in the upcoming passage, some approaches incorporated into minimalizing biofouling effects in the electro-determination of dopamine in biological environments are discussed.
For example, pre-incubation of the electrodes in well-defined protein solutions before the above-described experiments can indicate how biofouling affects the redox kinetics of DA. Most utilised proteins for such purposes include bovine serum albumin, fibronectin and fibrinogen, often as single solutions. Albumin is the most abundant protein in the blood and cerebrospinal fluid, while fibronectin and fibrinogen adsorption affects cell adhesion to biomaterials. Fibrinogen also plays a critical role in blood clotting. Their size differences are also interesting from the biofouling point: albumin is small (67 kDa) and forms 77 contacts per molecule, while fibrinogen is large (340 kDa) and forms 703 contacts per molecule.83
Single-protein solutions can give some first insight into biofouling. However, the examination of competitive protein adsorption shows that conclusions drawn from studies on the adsorption of individual proteins cannot be extrapolated to forecast the composition of the protein adsorption layer in the case of multi-protein adsorption.84 In addition to single- or binary protein solutions, pre-incubation in plasma, full serum, or blood can be used to estimate biofouling. However, these pre-incubation approaches might not provide a good understanding of electrode performance in a biological environment. For example, pre-incubation approaches do not consider the possible impact of the applied potential on the proteins on the surface. An electric potential can directly influence surface interactions.85
Another technique is to take advantage of deproteination methods. Deproteination eliminates protein structures by a chemical reagent such as protease enzymes, methanol, or a strong acid/base compound.86–89 More specifically, the reagent acts in the biological medium and does not impact other factors, such as pH or the integrity of other present molecules, the closer the final solution to a real sample. These methods, if carried out in the lab, are usually followed by centrifuging to remove the undesirable portion of the final product, but there is a possibility to purchase pre-deproteinized plasma commercially.90 An issue with this process is it is not deemed suitable for in vivo studies, based on the consequences of entering biological altering agents. An alternative solution for estimating the sensor's performance in a biological environment, even though the measurements should be done in an undiluted biological medium, such as blood, is performing measurements in 10–1000× timed diluted media (most commonly by buffer solutions) with spiked concentrations of the analyte (e.g.,91–93). This common practice decreases protein concentration levels significantly and limits the probability of active site occupation by interfering species. Still, one must point out that this procedure severely mutates the physiological characteristics of the solution and might not produce applicable results in terms of in vivo measurements.
3.3 Approaches to investigate the biocompatibility of a brain sensor
Biocompatibility means the ability of materials to locally trigger and guide normal wound healing, reconstruction and tissue integration.94 By this definition, present materials in biosensors are only biotolerable. Today, the goal is to develop innovative materials with superior biocompatibility. The first step can be the assessment of possible toxicity, followed by more detailed in vitro-, ex vivo- and in vivo – techniques. ISO 10993-5:2009 describes test methods to assess the in vitro cytotoxicity of medical devices. Many in vitro-, ex vivo- and in vivo – techniques and experimental models are used for studying the tissue response of different neural implant materials.95
3.3.1 Cell toxicity tests.
Various cytotoxicity assays are the most common methods to study material toxicity to neural cells.96–98 Assays measure cell reactions, cellular or metabolic changes, viability and proliferation in cell cultures. Standard cytotoxicity tests are colourimetric MTT/XTT/MTS/WST-1 assays based on different tetrazolium salts measuring mitochondrial activity. Other used assays are based on various molecules, like lactate dehydrogenase, which detect damaged or lysed cells or oxidative stress. Dye exclusion viability tests, such as trypan blue and neutral red, based on cellular contents to leak out. Pro-inflammatory cytokines or protein signals of the inflammatory response are detected using enzyme-linked immunosorbent assays (ELISA). All potential interferences must be considered when choosing a valid assay to avoid false results.99 A good example is CNTs attached to the MTT-formazan crystals that were formed after the reduction of MTT and were not soluble in the solvents. As a result, reduced cell viability was observed in the MTT test.100 In addition to assays, with flow cytometry, gel electrophoresis and DNA microarray studies, it is possible to determine DNA damage in the cells. Changes in cell and nuclear morphology can also be observed microscopically. Finally, cell proliferation is studied with different agar overlay methods.
3.3.2
In vitro models.
In vitro, 2D cultures are the standard and straightforward model to investigate the responses of new electrode materials or coatings biocompatibility and cell interactions. Fibroblasts are one of the most common cell types to study material biocompatibility due to fibrotic scarring in response to tissue injury. Of course, glial cell lines are an important target due to their central role in brain tissue and glial scarring. Commonly used cell lines include the L929 mouse fibroblast cell line, NIH/3T3 mouse embryonic fibroblast cell line, C6 rat astrocytoma cell line,101 PC-12 rat pheochromocytoma cell line,102–104 and BV-2 mouse microglia cell line.105
Immortalised cell experimental conditions are controlled, and cells are simple to culture, genotypically stable and can be grown long times. However, immortalised cell lines display evident phenotypic and physiological differences from the cell type of origin. Primary cell lines are more reliable as they do not have a tumor origin or were not manipulated, and therefore, they more closely recapitulate the neural cells' characteristics in vivo.95 However, their use has ethical and economic limitations because primary cells are isolated from animal models. Recent advances in neuronal cell derivation from pluripotent stem cells can provide valuable models in the future.106,107
In the study of glial scars, 3D matrices provide applicable models before in vivo studies.108–110 3D cell cultures enable mixed cultures with different matrix components and bioactive factors by mimicking CNS tissue. However, many challenges are associated with this technology, and optimisations are still required to ensure controlled culture conditions as close as possible to the in vivo environment.
3.3.3
Ex vivo models.
Organotypic cultures are tissue explants from animals or obtained from human biopsies, most often brain slices from rodents, and cultured in vitro.95,111 The advantage of organotypic cultures is the long-term assessment of the host response reactions and biocompatibility of the electrode materials. However, in addition to ethical and economic challenges, the problem is tissue and neuron injury caused by slice preparation.
3.3.4
In vivo models.
Animal models, most often mice and rats, help finding answers to biological questions concerning the role of different cell types in FBR.95 The standard method to assess the tissue response is to implant model sensors into test animals and sacrifice the animals at various time points. Sensors are removed following tissue fixation, and the excised tissue surrounding the implant is sectioned and stained to evaluate cell types and locations. The first tissue dyeing technique was hematoxylin and eosin to determine the location of different cell types around the implant.70 To gain more specificity of cell types, immunostaining for cell-type-specific proteins is a widely used dyeing technique to analyse cell density in brain tissue around the implant.112,113
Bio-imaging allows the imaging of living animals with high resolution.95 Two-photon laser scanning microscopy has been used to investigate the nervous tissue response to new electrode coatings114 and glial cell characterisation.115 Optical coherence tomography and X-ray micro computed tomography are an alternative to histological staining to quantify and characterise the tissue around electrode implantation.116,117
3.4 Fast scan cyclic voltammetry measurements in vivo
Over the last few decades, FSCV has become a predominant method for making in vivo measurements of neurotransmitters like DA in the brain.51,118 FSCV is an electroanalytical technique which involves applying voltage sweeps to an electrode at rapid scan rates to oxidise or reduce electroactive neurochemicals. The typical electrical waveform used for DA detection involves holding at −0.4 V, ramping to 1.3 V and back at a rate of 400 V s−1, and a frequency of 10 Hz.119 This waveform enables 100 ms temporal resolution, resulting in the ability to monitor subsecond fluctuations of electroactive neurochemicals in the brain. Due to the rapid scan rates, large non-faradaic charging currents are generated; however, background subtraction is used in FSCV to remove this capacitive current. Because of this, all cyclic voltammograms presented from FSCV experiments are background subtracted. To do this, specialised software built for FSCV analysis is typically used. Background subtraction presents both an advantage and disadvantage of the technique. FSCV is only capable of measuring rapidly changing electroactive neurochemicals resulting in less interfering analytes during in vivo detection. Conversely, this provides a relatively narrow analyte window for detection. Despite this disadvantage, recent advancements in FSCV capabilities have combined the concept of rapid anodic stripping voltammetry to aid in quantification of basal levels of neurotransmitters.120–122 Additionally, the stability of the background current is vital for accurate removal and ultimately accurate quantification in vivo. FSCV suffers tremendously from background drift, especially in biological samples.123 Over the last several years, advances have been made to combat the background drift problem including novel waveforms for drift removal and using high pass filtering.123,124 Practically though, many researchers cycle the electrode for at least 30 minutes prior to the experiment at extended waveforms scanning to at least 1.3 or 1.4 V and at varying frequencies (10–60 Hz) to improve equilibration and robustness of the electrode for in vivo sampling.
The potential limits and scan rate of the waveform are strategically chosen to maximise analyte adsorption to the electrode surface,119,125 avoid detrimental chemical fouling,126–128 and to improve selectivity between chemically similar neurotransmitters.129–131 Over the last several years, these advancements in waveform development have expanded FSCV detection to many neurochemicals beyond DA, including serotonin,26,132 adenosine,133 guanosine,134 hydrogen peroxide,135 neuropeptides,130 among others.126,136,137 The most common electrode material used in FSCV is the CFME. Carbon-fibre is most often made from wet-spinning polyacrylonitrile (PAN) or melt-spinning pitch. Carbon-fibres are excellent electrode materials for in vivo detection due to their small size (typically 5–10 μm), resulting in less tissue damage compared to typical microdialysis-based sampling methods,138 background current stability, low limits of detection, and their relatively bio-inert surface. Despite these advantages, carbon-fibre is susceptible to electropolymerisation fouling and biofouling, presenting a significant challenge in chronic in vivo measurements. Recently, researchers have expanded beyond amorphous carbon-fibre, to more highly structured carbon-based materials including CNTs,139 carbon nanospikes,140 and graphene-based microfibres.141 These new carbon-based materials have provided an improvement in fouling-resistant surfaces to advance long-term in vivo recording.
Neurotransmitter detection with FSCV is often done through either evoking neurotransmitter release by electrical stimulation119,122 or with optogenetically-controlled stimulation,142 or measuring spontaneous (unevoked) transient neurochemical release.135,143 These approaches provide a powerful method to either evoke an endogenous response or to measure transient neuromodulatory signalling; however, implementing these strategies well in vivo can be challenging. A practical tip for improving the robustness of in vivo detection is to use freshly made cylindrical CFME, cut to at least 150 μm in length, as opposed to electrodes that are shorter and fabricated days prior. It is unclear exactly why a freshly cut electrode improves the likelihood of in vivo detection. We speculate that it could be due to the oxide functionality present on a freshly trimmed electrode which is known to improve analyte adsorption and sensitivity; however, more in-depth surface characterisation should be compared on freshly trimmed vs. days-old electrodes to detangle this observation. Additionally, some researchers have discovered that eliminating the typical epoxy-sealing step for CFME fabrication drastically improved their chances of getting a signal in vivo. To do this properly, one must be sure that the seal between the glass capillary and the carbon-fibre is tight to eliminate the chance of having a “leaky” electrode. Eliminating the epoxy-sealing step may reduce the likelihood of the active sites on the carbon surface from being covered with epoxy, leading to limited surface area available for electrochemical detection.
Overall, FSCV in vivo detection has significantly advanced our understanding of neurotransmission in the brain during behavior, addiction, various diseases and brain injuries. Despite its many capabilities, widespread adaptation of the technique has been limited to a handful of researchers worldwide. FSCV is a very niche field, requiring specialised training in its use and implementation. Additionally, most CFMEs are made in-house, requiring training from an experienced FSCV scientist to make robust electrodes for in vivo. Improvements in the translatability of the technique are certainly necessary in the future to expand its use to a wider array of researchers. Many of the tips to get started with in vivo FSCV detection involve paying careful attention to the electrode. To summarise and expand on some of the tips presented in this tutorial review for getting started in FSCV in vivo detection: (A) make freshly cut, cylindrical CFME on the day of the experiment, (B) adjust the parameters on the capillary puller to improve the seal between the glass capillary and the carbon-fibre so that you can eliminate the epoxy sealing step, (C) cycle your electrode for at least 30 minutes at an extended waveform in vivo prior to measurement to improve electrode calibration and surface reactivity, and (D) use a software like HDCV144 so that you can monitor the background current in real-time-this enables you to visualise the drift and either change out the electrode if appropriate or wait for the background current to stabilise.
4. Current state and approaches to overcome the challenges
4.1 Selectivity on different electrode materials
There are several solutions for improving the selectivity of DA sensors. Besides material-based approaches, which are discussed below, an attractive approach, due to its availability and simplicity, is cycle rate and potential adjustment, i.e., holding the potential at −0.4 V preconcentrates the positively charged DA ion and high scan rates let the DA oxidation that has a faster electron transfer rate than AA take place more efficiently, also using alternative electroanalytical methods such as DPV and SWV instead of CV adds to sensitivity and selectivity.145
Table 2 summarises the LODs achieved using selected electrode materials. However, it is essential to note that multiple factors must be considered for successful in vivo DA detection.
Table 2 Comparison of LODs achieved using selected electrode materials
Electrode material |
Electrochemical technique |
LOD/nM |
Ref. |
Tyrosinase/ZnO-rGO/ITO |
DPV |
0.009 |
182
|
Fe/N-GR/GCE |
DPV |
0.027 |
185
|
Nano-Au/PPyox/GCE |
DPV |
0.15 |
170
|
PEDOT:Nafion/CFMEs |
FSCV |
4–5 |
158
|
CFME with electrochemical pretreatment |
DPNV |
5 |
45
|
CNH/CFMEs, and subsequent oxidative etching |
FSCV |
6 |
160
|
CNT on Nb wire |
FSCV |
11 |
179
|
Interfacing aptamers with CFMEs |
FSCV |
13 |
163
|
CNTyarn-discs microelectrode |
FSCV |
13.4 |
157
|
MWCNTs/ta-C |
CV |
42–85 |
181
|
ND/ta-C |
CV |
50 |
30
|
Tyrosinase/NiO/ITO/PET |
CV |
1038 |
184
|
Tyrosinase-SWCNT-Ppy |
Amperometry |
5000 |
183
|
4.1.1 Carbon-based electrodes.
Carbon-based microelectrodes have been used in the early voltammetric detection of DA and other neurotransmitters due to their biocompatibility, enabling implantation and in vivo measurements as close to the synaptic region as possible.11,146,147 Multiple studies involving the treatment and modification of the electrode materials were performed to address the sensitivity and selectivity problems and improve detection capability.
For instance, the electrochemical treatment of CFME by applying a triangular wave potential was observed to improve selectivity, sensitivity, and reproducibility, allowing the correct identification of oxidation peaks which was not possible in the untreated electrode material.43 Following the same pretreatment for CFME implanted in rat striatum, a DA concentration of 15–25 nM was recorded, and a detection limit of 5 nM was achieved through differential normal pulse voltammetry (DNPV).45 Using carbon-fibre microelectrodes and amperometric measurements with a detection limit of less than 20 μM, a basal DA concentration of 30 μM was predicted, consistent with values obtained using microdialysis.148
As a surface phenomenon, the sensitivity and selectivity of electrochemical detection are typically enhanced by modification of the electrode surface. The chemical modification involves the introduction of charged groups onto the electrode material to shift the oxidation potential of the interfering compounds. At physiological pH, primary neurotransmitters such as DA are protonated, while most interfering compounds are negatively charged (see Table 1), so cation-exchangers such as carboxylic and sulfonic groups are desirable. One of the first examples of this is the incorporation of stearic acid into graphite paste electrodes enabled the selective in vivo monitoring of DA in the presence of AA and DOPAC.149 Still, on graphite electrodes, dip-coating with Nafion, a perfluorosulfonated polymer, was found to strongly reject DOPAC with minimal response to AA.150
Through extrapolation, a value of as low as 6 nM was estimated for the unstimulated synaptic striatal DA CFME dip coated with Nafion and FSCV.151 Other surface modification techniques on CFME, such as overoxidation, resulted in improved surface adsorption and led to a 9-fold increase in sensitivity, but at the expense of electrode response time and selectivity.152 Chemical pretreatment of CFME by covalent attachment of 4-sulfobenzene after electroreduction increased the adsorption of DA at the electrode surface without affecting the response time using FSCV, improving the sensitivity and selectivity only to a certain extent because it remains permeable to negatively charged compounds.153
Carbon nanomaterials are widely used in electrochemistry and sensor research because of their brilliant electrocatalytic properties for the selective detection of DA, even in the presence of mentioned interfering species. A review by Yang and Venton contains detailed information on using carbon nanomaterials as electrochemical sensors for the direct detection of DA, highlighting its advantages, such as cheaper cost and the possibility for batch fabrication.154 Immobilisation of single-walled CNT (SWCNT) onto CFMEs performed by dip-coating produced larger measured currents and S/N ratios due to increased surface area, but the results had poor reproducibility because of the inconsistencies in the orientation and density of CNTs during deposition.27 A study on the effect of different surface functionalities of SWCNTs on dip-coated CFMEs showed that carboxylic acid functionalisation improved the sensitivity of the electrodes even after implantation, but reproducibility is still to be improved.155 More control was achieved through the chemical self-assembly of carboxylic acid functionalised SWCNTs, producing aligned CNT forests that maximised accessible electroactive surface and increased signal-to-noise ratio by 1.8 times compared to dip-coating.156 Using multi-walled CNT yarns disk (CNTy-D) microelectrodes to measure DA in live brain tissues, a LOD of 13.4 nM was achieved.157
CFMEs coated with poly(3,4-ethylenedioxythiophene) (PEDOT):Nafion composite polymer resulted in increased sensitivity compared to bare electrodes and LOD of 4–5 nM DA, depending on the density of the monomer 3,4-ethylenedioxythiophene (EDOT). When implanted in the nucleus accumbens of rats, 5–200 nM DA transients were recorded. In a span of 30 minutes, uncoated electrodes exhibited a 60% and 33% reduction in sensitivity when implanted in the prefrontal cortex and nucleus accumbens of rats, respectively, while the modified CFMEs lost only 9% after 6 hours.158 In another study, PEDOT and graphene oxide were electrochemically copolymerised on CFMEs and implanted into the dorsal striatum of rats, achieving about an 880% increase in DA sensitivity and a 50% decrease in LOD compared to bare electrodes using the optimal PEDOT/GO polymer deposition time.159 Deposition of carbon nanohorns (CNH) to CFMEs and subsequent oxidative etching resulted not only in the increase of surface oxide groups for DA adsorption but also in the electrostatic repulsion of negatively charged interferences such as AA, obtaining a LOD of about 6 nM.160
Supporting experiments using conducting polymers and/or carbon-based nanomaterials showed that the enhanced electrode performance is due to the increase in surface area, charge (capacitance), or both. Thicker deposited material often leads to higher sensitivities, but at the expense of response time due to slower adsorption and electron transfer kinetics.158,159
Another study performed in 2017 demonstrated that by employing CNT yarn 3D printed electrodes, the final sensor can present distinguishable peaks of UA, AA, and DA by three folds near physiological concentrations.161 In 2018, a carbon black and CNT co-doped polyimide (PI) mixture was used to modify a sensory unit. The information published in this paper showed the sensor could distinguish between DA, AA and UA oxidation peaks in CV and DPV.162 The concentrations were close to biological mediums, and the effect of a steady increase in one species was studied. In a 2022 paper, interfacing aptamers with CFME posed an excellent solution for minimising the impact of interferences by showing a 5-fold higher sensitivity toward DA than AA.163 In the same year, a fast-scanning potential-gated organic electrochemical transistor (OECT) was introduced that its measurements were validated in living rats' brains, showing an acceptable selectivity for DA (50 μM) against AA (200 μM), UA (50 μM), and DOPAC (50 μM).164
4.1.2 Metal-based electrodes.
Metals have desirable properties as electrode materials, such as high electrical conductivity and electrocatalytic activity, high specific surface area in the case of metal nanoparticles, and ease of fabrication into microarrays.46,165–167 Noble metals such as gold and platinum are common electrode materials, but not particularly in the direct in vivo detection of electroactive neurotransmitters such as DA. Compared to carbon materials, metals easily undergo electrooxidation resulting in a smaller potential window for measurements.46 Moreover, it has less biocompatibility and is more prone to fouling.75,168 For instance, one common problem for Pt electrodes is the pH-dependent surface oxidation and chemisorption of many species, while thiol adsorption is typical for Au.168
One of the first successful in vivo neurotransmitter experiments using metal electrodes was performed by Lane and Hubbard in 1976 with an iodine-modified platinum surface that could distinguish DA from AA using DPV.168 However, surface fouling is a problem, resulting in high background currents and irreproducible results, making it unsuitable for quantitative analyses.
In the succeeding years, several studies using metal nanostructures and techniques such as lithographic fabrication enabled more control over the electrode surface, improving sensitivity and selectivity compared to thin metal films.169 In 2007, a nano-Au/PPyox composite-coated glassy carbon electrode (GCE) sensor was developed to detect DA and serotonin simultaneously. It has been reported that utilising the mentioned modification resulted in a LOD of 0.15 nM for DA in the presence of overlapping peaks of 1000-fold AA.170 Another choice is sensor modification with metallic NPs such as gold, silver, palladium and platinum.171,172 Metallic electrodes can also be easily assembled into microarrays for simultaneous and/or improved detection of DA in the presence of interfering compounds.165,167,173–176
However, insufficient selectivity and sensitivity are still a challenge in detecting DA in vivo.166,177 Because of this and the persistent problem of fouling, noble metals were typically used in combination with other materials like conducting polymers,178 self-assembled monolayers,176 and carbon nanomaterials173,179 to investigate its effect on selectivity and sensitivity, but most of the measurements were made in vitro.
4.1.3 Integrated structures.
A study in 2013 demonstrated after a baseline correction, the presence of an array of vertically aligned carbon nanofibres (CNFs) grown by plasma-enhanced chemical vapour deposition with Ni as a catalyst on the sensor surface can distinguish the individual compounds in a ternary mixture of AA, DA, and serotonin, with AA close to its biological concentration.180 Later on, metals such as Nb, Ta, Mo, W, Pd, and Ti were tested as substrates for the growth of aligned CNTs. These metals have higher conductivity than CFMEs but do not have any electrochemical reactivity to DA. Metal wires were used instead of planar substrates to be more suitable for implantation. Among the metals, CNT-Nb exhibited the best LOD of 11 nM for DA.179 More studies were conducted on the effect of different metals on DA detection. For example, carbon nanostructures grown from Ni and Al + Co + Fe hybrid were reported to satisfy the required sensitivity and selectivity for DA detection.177
Another interesting material is tetrahedral amorphous carbon (ta-C) with an expansive water potential window, and it has been reported to improve the sensitivity of DA detection. Integrating nanodiamonds (NDs) with ta-C films deposited on Ti-coated Si-substrates lowered the LOD to a physiologically relevant value of 50 nM.30 Furthermore, unmodified and multi-walled CNT (MWCNT)-modified ta-C films deposited on Si wafers were found to have LODs in the range of 42–85 nM DA. The unmodified ta-C films have higher sensitivity and a broader linear range, but the deposition of MWCNTs provided the selectivity needed for the simultaneous detection of DA, AA, and UA at physiologically meaningful concentrations.181
4.1.4 Biorecognition elements.
Immobilising biorecognition elements on the electrode surface is another way to improve the sensors' detection capabilities. Tyrosinase is an enzyme that catalyses the oxidation of DA into DAQ, leading to higher oxidation currents.182 In general, the addition of the enzyme led to good selectivity in the presence of interfering compounds, while sensitivity, LOD, and linear range are typically in the nM–μM range. A LOD of 5 μM for DA by amperometry using tyrosinase-SWCNT-polypyrrole (PPy) composite electrodes,183 but when tyrosinase was adsorbed on NiO nanoparticles deposited on indium tin oxide (ITO), a lower LOD of 1.038 μM was obtained.184 There were a few more studies employing tyrosinase, and there is no doubt that it improves analyte recognition in the presence of interferences, but the LODs remain in the micromolar range due to the enzyme's slow electron transfer capabilities.182 Therefore, more recent studies employed materials with excellent electron transfer capabilities, such as graphene oxide, obtaining LODs in the picomolar range. For instance, a LOD of about 9 pM was achieved using ITO electrodes deposited with tyrosinase on ZnO-reduced graphene oxide (rGO) nanocomposite films.182 This is the lowest LOD value obtained for DA so far. Very recently, a non-enzymatic electrochemical biosensor composed of Fe-sites dispersed in N-doped graphene (Fe/N-GR/GCE) achieved a LOD of 27 pM due to the abundance of exposed active sites.185
4.2 Stability of the sensors
When investigating the sensor's performances, one key aspect is the sensor's ability to stay stable during extended periods of measurement, especially in studies centred around in vivo implants. Unfortunately, due to many issues such as sensor surface degradation, over-concentration of sensor active sites, the reaction of neighbouring cells and tissues to a foreign agent, uncontrolled release and everchanging concentration of DA, and sub-reactions taking place nearby the sensor, the duration of in vivo DA measurements is limited compared to other conventional sensors and mediums. Therefore, increasing the determination timeline remains an obstacle.
A notable matter on electrochemical DA determination is that a considerable portion of the studies revolves around detecting DA only in buffer solutions, pre-treated biological mediums such as urine and blood or on cell cultures and tissues in vitro. This leads to result maladjustments to in vivo applications. However, those sensors are utilised for DA detection, which cannot be overlooked.
Starting with the broadest group, electrochemical sensors developed and optimised in buffer solution, and pre-treated biological fluids demonstrate significant outcomes. Their detection limit, durability, selectivity, and linear range outperform other categories,186 but how replicable are these results in in vivo measurements? Even though recently, more and more studies are concentrating on experimenting in the simulated cell environment, such as solutions containing glucose and salt and exposing the electrode in the solution for extended periods, due to cautiously selected solution environments, there are limited, if any, interferences present. For example, the target molecule is often spiked, and blood/urine proteins are eliminated with various procedures. In most cases, the sensor's exposure to the solution is short, and its surface is regularly cleaned and polished physically and chemically. Therefore, faced with reports of 2 weeks,187 30 days,188,189 60 days,190 or 68 days191 of sensor stability, it is necessary to pay attention to the determination environment.
Moving from mentioned types of detection to in vivo implants, several studies have been successful in implanting a sensor for relatively long periods. However, it is worth noting that based on the subject specie, the sensor's size, width, geometry, and design changes drastically. Since the nervous system and brain structure vary, head movements significantly impact the implant's stability and durability. In vivo studies have been performed on several species: insects, rats, and primates.192 The detection technique can differ as well. Some in vivo measurements are done momentarily by drilling several holes in the skull and inserting the electrodes, like in the case of micrometre-sized tungsten wires deposited with BDD,193 but the method in this paper's scope is the long-term implants placed on the brain tissue. The most prominent studies revolving implanting a stable and durable brain starts with Adams,7 utilising a carbon-based micro-electrode to detect DA levels in the brain of a live open-skulled rat. Still, the question is, can long measurements be reliably performed? In 2007 Seymour et al. worked on durable implants based on perylene that lasted about four weeks in the brain without significant changes in response.194 Between 2014 to 2016, some notable studies focusing on live DA detection in non-human primates were published that were able to operate and stay stable in a couple of hours, therefore not suitable for long-term observation.195–197 In 2017, an extensive study on the stability of silicon probes in brain tissues was done to investigate the scarring effect in live mice. The probes were demonstrated to show stability for up to 8–10 weeks.198 In the same year, a paper was published by Schwerdt et al. on implanting sensors in primates, reporting over 100 days of sensor stability199 and the following year, a staggering timeframe of 398 days of stability in rodents was written by the same group.200 A 2021 study by Schwerdt's group on rodents and non-human primates' brains showed months of implant functionality.201 Subsequently, it is safe to infer that as new materials and techniques are introduced, the chances for long durable implantation are rising.
4.3 Temporal resolution of the sensors
One of the critical factors in the fabrication of electrochemical sensors for the determination of neurotransmitters, especially DA, is their capability of rapid detection and result production. Thus far, sampling and response time are not fixed across the proposed sensors. The possibility of collecting results at nearly the same time as DA release paves the way for more real-time studies of brain functions. Several aspects affect the overall time efficiency of the electrochemical sensor. Some of these factors are 1) electroanalytical method (FSCV, DPV, and SWV), 2. electroanalytical variables (scan rate, potential limits, and waveform frequency), 3) materials and geometry of sensory unit, and 4) determination medium (saline, biological fluids, and in vivo environment). Most often, the waveform for DA ranges from −0.4 to +1.3 V.202 Studies have shown that by increasing the scan rate to >400 V s−1, the timescale for DA detection can be reduced to milliseconds.202,203 To prove this point, several studies have been conducted showing that only with tampering with the scan rate119,204 and changing the frequency of the waveform, overall changes in the timeframe for DA detection are visible. However, based on the electrode material, scan parameters might affect the sensitivity insignificantly or greatly.121,205 Another factor that can affect the sensor's speed tremendously is the incorporation of various modifications that would enhance the electron transfer rates, generating higher current in shorter periods. In 2018 such modifications of glassy carbon electrodes with Fe2O3 and graphene nanosheets increased the electron transfer rate.206 In the same year, two other studies experimenting with different modifications, i.e., poly(3,4-ethylenedioxythiophene), a conductive polymer207 or MWCNT embedded with CeO2, demonstrated that these modifications prove effective in increasing the electron transfer rate and subsequently, faster detections.208 Like the previous passage, it is worth mentioning that many sensors developed for DA detection have only been tested in solution and in vitro mediums. Therefore, the timescales provided in these papers can rarely match the actual performance in vivo. For example, in a study conducted in 2010,209 even though the sensor was developed with the intention of in vivo determination, only the response time of measurements in solutions are reported (<8 s to ∼20 s). In 2016, a biosensor based on surface-functionalised nanostructured nickel oxide platforms had a response time of 45 seconds.184 A sensor based on gold nanoparticles anchored nitrogen-doped graphene was introduced in 2016 for simultaneous detection of glucose and DA, showing an 8-second response time.210 In 2018, a flexible electrochemical transducer platform based on PEDOT-titania-poly(dimethylsiloxane) was developed to detect various neurotransmitters simultaneously. They reported a 15 s response time and speculated the platform could be modified to perform decently in vitro and in vivo.172
When considering the requirements for the temporal resolution, one needs to consider the three major forms of DA presence in the body: 1) transmissions, which occur in milliseconds, 2) ramps lasting possibly for a number of seconds, and 3) oscillations that might last up to a few hours.57 Because neurotransmission is a notably fast procedure, the need for an instrument which can operate between the release time and the compound termination is dire.202 The sensing unit must be able to perform consecutive measurements in ultrashort spans of time in the same area and map the changes in the analyte concentration. This variable is defined as temporal resolution. Most DA sensors intended for in vivo applications are carbon-based electrodes utilising the FSCV method. FSCV with tremendously high scan rates (≥400 V s−1, even stretching to 1200 V s−1), ultrafast cycle durations (about 100 ms), and frequencies ranging from 10 to 110 Hz, provides the opportunity for obtaining sets of data with high temporal resolution. For comparison, DPV can provide 10 m resolution while FSCV has sub-second intervals. In this method, DA kinetics is studied according to the changes in various scans over a fixed time.145 A prominent feature of FSCV is the possibility for multiplex studies that pave the way to investigate other present compounds, be it other neurotransmitters or interferences. Because in FSCV measurements, the time periods between oxidation and reduction phases are drastically small, it results in longer electrode lifetimes and more linear responses.203 It is notable that the most accurate results are obtained when DA is at its peak concentration.119,165,211 In the existing literature, diverse amplitudes of temporal resolution are reported. From 45-second resolution184 in a solution environment to single-digit milliseconds in vivo measurements. A problem raised in the study survey is the lack of scientific clarity of reported temporal resolutions. Many electrochemical DA detection in vivo and/or in vitro papers claim to develop methods or sensors that would increase the efficiency of temporal resolution but fail to affirm them using figures, tables, and statistically approved numbers. What they provide is, at best, a repetition of this sentence “This method demonstrates higher than average temporal resolution”. This issue creates uncertainty and vagueness because they cannot be tested nor confirmed and confuses the researchers who go through the previous studies to fix their shortcomings. However, this being said, here are some recent studies that did include comparable results regarding temporal resolution. A one-minute temporal resolution was reported by Gu et al. for in vivo detection of DA with coupling online capillary LC and microelectrodes.23 A study in 2019 utilised the SWV method for DA detection using a poly(3,4-ethylenedioxythiophene) based nanocomposite on functionalised CNT (PEDOT/CNT)-coated CMFE and reported a 15 s temporal resolution.212 In the same year, a 0.01 s temporal resolution was reported for a DA microelectrode array system modified with platinum nanoparticles and reduced graphene oxide nanocomposites utilising FSCV.213
4.4 Spatial resolution of the sensors
The design and sensor's size can drastically impact the overall outcome of sensing efficiency. Even though the structure must offer robustness and rigidness, at the same time, it must cause minimal damage when implanted in the brain as well.75,192 Considering DA's various local concentrations,214–216 it is essential to pay attention to affinity and dynamic range as well as sensor scaffoldings and kinetics.171 At the same time, it has to be as small as possible to penetrate the Synaptic spaces and not deteriorate due to its micro-scaled size.192 The ability of the sensor to detect the concentration variance between individual cells or bundles of cells in specific requires 10–100 and 100–500 μm spatial resolution. In contrast, in synaptic gaps, nanometric spatial resolution is needed.217 Spatial resolution can be defined in several ways regarding electrochemical sensors. From one perspective, it can be defined as the smallest area the sensor can detect the concentration without confusing it with the adjacent spaces, or it can be defined as a specific area that can be scanned in fixed time intervals. This leads sensor fabrication into introducing microelectrodes and arrays. Recent sensors mostly utilising carbon-based materials such as pyrolytic carbon fibres, have introduced micro-electrodes with small diameters in orders of 30, 10, and 8 μm.51,216,218,219 Their most conventional forms are either cylindrical carbon fibres encapsulated in glass capillaries for acute recordings (hours to days) or fibres encapsulated in fused-silica tubing for chronic recordings (weeks to months).75 The sensor's diameter can indeed correlate to better spatial resolutions, but it is not the cause for it. Compared to other in vivo techniques, electrochemical sensors' spatial resolution offers an acceptable spatial resolution, ranking better than micro-dialysis but falling behind when tested next to optical sensors.192,220,221 However, there is a contradictory opinion in some studies that optical sensors bridge the spatial resolution accuracy between micro-dialysis and electrochemical sensors. This claim is not as highly supported as the last, but it might point out that the results are heavily based on instrumentation and implanting techniques. In vivo electrochemical sensors provide <10 μm spatial resolution so far. Like the temporal resolutions a similar issue is seen in the studies focused on temporal resolution, however much more pronounced. Aside from a considerable percentage of papers that do not dedicate a part of their sensor development procedure to spatial resolution, many articles claim to have improved the spatial resolution but fail to provide numerical comparisons or present data in comparative tables. Once again, this matter makes it significantly hard to validate these scientific reports and poses a problem for building upon the results.
Even though much research has been conducted on detecting sub-second phasing DA, determining the resting DA concentration is still challenging. It is heavily dependent on the determination technique. The most common way is micro-dialysis.222,223 Utilising this method has led to several studies on the matter, reporting the basal concentration between 2.5–15 nM. However, when FSCV is the primary technique, because of the electrochemical nature of measurements and interferences present in the environment, as well as the fact that FSCV is a differential method and the background subtraction is inevitable in the final results, it is almost impossible to detect resting DA levels with it.222,224 A solution to this problem is to induce pharmacological manipulations, which up to this point have offered results indicating DA ionic liquid basal presence of more than 2.6 μM. Other electrochemical methods have been studied for this purpose showing promising results. For instance, fast scan-controlled adsorption voltammetry estimated 90 ± 9 nM DA in mice, and convolution-based FSCV did measure basal DA concentration in rats around 41 nM, also with differential normal pulse voltammetry, the basal DA level was detected to be 26 ± 8 nM in the pargyline-pretreated rat striatum.212 Therefore, it can be concluded that advances in electrochemical methods and utilising more novel sensor structures make it feasible to quantify the DA basal concentration more accurately.
4.5 Approaches to avoid glial scarring
Currently, the interface limits the use of recording sensors, as the glial scar reduces the effective transfer of signal. Prevention of scar-like capsule formation would increase the lifetime and effectiveness of biosensors. When improving better biosensors, the idea is to get the sensor integrated into the tissue as well as possible and perform with an appropriate host response. Ideally, brain sensor materials should promote the growth of neurons and the formation of synapses while decreasing the viability of glial cells. Furthermore, materials should reduce the footprint and enhance surface porosity to mitigate immune responses and, at the same, have good electrical properties.67 Potential strategies have been explored to develop biocompatible neural sensors: low-fouling materials, biomimetic coatings, soft and flexible sensor materials or coatings, porous materials, bio-inert materials, anti-inflammatory compounds and topography properties of the sensor (see Table 3).68,73,225
Table 3 Summary of sensor material properties to avoid glial scar formation
Sensor properties |
Methods and materials |
How glial scarring is prevented |
Ref. |
Chemical |
Anti-inflammatory compounds |
Anti-inflammatory compounds reduce inflammatory reactions and that way, prevent glial scarring Surface coatings reduce adhesion of glial cells Adhesion molecules, bioactive molecules and functional groups increase the adhesion of neurons |
67, 73, 228, 231–235 |
Adhesion proteins |
Bioactive molecules |
Biological coatings |
Non-biological coatings |
Surface functionalities |
Physical |
Small electrode size |
Small and rounded electrodes avoid tissue injury, and that way FBR and glial scarring Nanostructured surfaces render neuron adhesion and neural networks and prevent biofouling |
68, 72, 73, 94, 236–242 |
Rounded shape |
Small cross-sectional area nanostructured surface topography |
Mechanical |
Softness and flexibility mimicking brain tissue stiffness |
Materials with the same stiffness as the brain tissue avoid tissue injury and increase sensor integration to the tissue |
67, 73, 225, 227, 243, 244 |
Electrical |
Metals and metal oxides |
Sensor material should have good biocompatibility with neural tissue and good electrical properties to avoid host response and improve signal transduction and long-term electrical stability |
166, 171, 177, 226, 228, 245 |
Conductive polymers |
Organic semiconductors |
Carbon-based materials |
The biocompatibility and performance of the sensor in the neural interface depend on the material's physical, chemical, mechanical and electrical properties. Before most antifouling strategies for electrode integration into neural tissue have been improved with a protective layer or barrier. However, these strategies reduce the sensitivity and temporal resolution of a sensor and the effectiveness of a stimulating device. Today the focus on neural sensor materials is on different nanomaterials due to their good biocompatibility.220 Common materials are metals, semiconductors, conducting polymers and carbon-based materials, which are widely used to modify electrodes to detect DA directly.166,171,226–229 In addition, the sensor material cell adhesion has a pivotal role in the formation of glial scars in brain tissue.67,230
4.5.1 Physical properties.
Sensor implantation cause always pressure changes and traumatic injury in the target tissue. FBR is related to that injury, as inflammation attracts immune cells to the area, which can join the reaction against the implant. Hence, the effective strategy to avoid glial scarring is to design sensors that minimise the trauma.246 In addition, sensor movement in tissue can cause injury even after implantation. Reducing tissue injury, and that way, glial scar formation can be affected by electrode size and shape,72,194,247 cross-sectional area,242 and surface topography.248 Smaller electrode size and cross-sectional area and rounded electrode tip shape reduce the impact of tissue displacement and injury.72 In neural sensors, feature sizes below 10 μm have been observed to minimise gliosis and preserve neuronal density.240
Sensor surface nanotopography affects protein adsorption, vascularity, cell adhesion, morphology and activation in neural tissue.95,249 Nanostructure modifications and nanoporous structures on electrode surfaces have been noticed to reduce glial scar encapsulation while maintaining neural cell adhesion.105,237,250 In CNS, neurons form functional networks where their efficiency depends on network topology. Cells attach to the environment with cell adhesion molecules. With textured, porous and rough surfaces, it is possible to increase neural cell adhesion and growth, which improves sensor integration into neural tissue.251 Potential nanomaterials for topographical surface modifications are metals, such as gold237,250 and carbon materials.252 Hybrid carbon nanomaterials are promising materials for the electrical detection of DA. By tuning the nanofibres on surface geometry, it may be possible to control host response in neural tissue.238,239
4.5.2 Chemical properties.
There are plenty of chemical modifications in the neural interface that can modulate the inflammatory response.67,73,232 For example, anti-inflammatory compounds,253 adhesion proteins,249 biological and non-biological coatings,234 like peptides,254 hydrogels,233 and polymers.231 Anti-inflammatory compounds reduce inflammation reactions which prevent FBR and glial scarring. Cell adhesion is based on transmembrane proteins' surface functionalities between the electrode and neural tissue, affecting protein interaction. Adhesion proteins and other biological compounds at the sensor surface can potentially enhance tissue integration and improve biocompatibility affecting the cell interactions like neural cell adhesion. The idea of biocompatible coatings is to functionalise the electrode surface with a buffer layer to reduce the adhesion of glial cells at the implant surface.235 For the detection of neurotransmitters, challenges with coatings are increased background electrode capacitance and impedance and acting as a diffusion barrier. Today, the purpose is regulating the FBR just with the electrode material. Functional groups affect surface functionalities and cell interaction.155,255 Oxygen groups, for example, on graphene, render the surface hydrophilic and improve cell adhesion and viability.256 Hydrogen or mixed carboxyl/amino functionalities also potentially improve tissue integration and prevent glial scar formation.238
4.5.3 Mechanical properties.
Brain tissue is one of the softest tissues in the body; the stiffness is around 1 kPa. Earlier, the most used materials for neural sensors were rigid materials, such as silicon, whose stiffness can be even 150 GPa. However, that mechanical mismatch causes tissue trauma during implantation and continuous injury and chronic inflammation of brain tissue, which activates astrocytes and microglia.230 Rigid materials also upregulate pro-inflammatory cytokines in neural tissue.73 The purpose of designing next-generation devices are soft and flexible materials that could mimic the stiffness of the brain tissue and reduce mechanical mismatch.67,227,243,244 Conductive polymers are potential sensor materials due to the mechanical properties matched with biological tissues. Rigid materials can also be coated with elastic coatings, such as hydrogels.234,257
4.5.4 Electrical properties.
Good electrical properties are essential for better biocompatibility in neural applications for successful and long-time signal transduction at the electrode-tissue interface.232 Metals, metal oxides and conductive polymers are widely used sensor materials to decrease impedance in electrodes. Metals and metal oxides have been the most used electrode material for the past 50 years.166,226,227 Common metals for neural sensor electrode materials are gold (Au) and platinum (Pt). Organic semiconductors, such as silicon, and conducting polymers, such as PEDOT, have both good electrical and mechanical properties in neural sensors.166,226–228,231,258 Carbon-based materials have shown remarkable biocompatibility with excellent electric properties, which makes them essential for neural interface applications.166,226–228,259,260 Mainly used carbon-based materials are graphene,245,261 carbon nanostructures,252,260 such as CNTs and carbon nanofibres (CNFs), glassy carbon and diamond. Usually, different nanomaterials and nanoparticles are modified and joined together to obtain better electrical, electrochemical and biological properties. Ideally, the impact of each component should be examined separately. A step-by-step fabrication, i.e., starting from a simple electrode platform where the complexity is increased gradually and the material is characterised carefully after each step, would allow the correlation of the material properties with the device's functionality.
5. Conclusions
Electrochemical measurements are a powerful tool for defining DA concentrations in vivo. However, long-term in vivo measurements remain challenging, underpinning the need to develop enhanced electrode materials. Through this tutorial, we have provided researchers with an approachable and comprehensive guide to the requirements of the biological environment: sensitivity, selectivity, temporal resolution, spatial resolution, and stability. Detection of the physiological concentrations of DA (0.004–0.030 μM) is challenged by the presence of abundant interferents with overlapping oxidation potential. Extremely high temporal and spatial resolutions are desirable for recording neurotransmitter release into the synaptic cleft. Stability in the biological environment, controlling biofouling and host response, remains perhaps the most challenging problem to be solved for facilitating long-term in vivo measurements. When considering the requirements, it is critical to consider the purpose of the measurement, as short-term vs. long-term and dynamic vs. basal measurements affect what is expected from the sensor material.
We continue with a step-by-step guide for characterising electrode performance and performing in vivo measurements, starting from basic electrochemical characterization in saline, moving on to methodologies for mimicking the biofouling environment and approaches to measure the biocompatibility of a brain sensor, and ending with fast-scan measurements in vivo. Basic knowledge of the properties of the electrode, such as pseudocapacitance, potential window and open circuit potential in various buffers, is often beneficial for understanding the behaviour in more complex systems. To allow the comparison of the performance in other approaches, the LOD and sensitivity of the sensor are typically defined. However, the calculated LOD value might not reflect well what concentrations the electrode is able to measure. Defining the linear response range gives better insight into this. To measure selectivity, paying attention to the actual physiological concentrations of both DA and the interferents is crucial. To better understand the DA–material system, one approach is to define whether the reaction kinetics is defined by diffusion or adsorption. Recently, computational approaches have been used to give more detailed insight into the interactions at the DA–material system, but one should be careful when correlating the results calculated on ideal surfaces (e.g., graphene) to experimental results performed on non-ideal surfaces (e.g., glassy carbon). No standardized methods for the measurements in a biological environment exist, and while many of the simplified approaches can give some insight into understanding the biofouling mechanism, they might not give sufficient insight into electrodes' true performance in vivo.
We end the tutorial review by providing an overview of the current approaches to solving the challenges. By integrating the theoretical background with practical experimental details and highlighting experimental pitfalls, this text reflects current best practices in developing new electrode material for the successful in vivo determination of DA. Our final recommendation is that when developing a novel sensor material, a step-by-step approach for electrode fabrication and characterisation is critical to understand the correlation of the material properties with the device's functionality. Only this approach would allow optimization of the material for the specific purpose.
Conflicts of interest
There are no conflicts of interest to declare.
Acknowledgements
This research has been supported by the Academy of Finland (grants #352421, #352422, #347021).
References
- A. Carlsson, M. Lindqvist and T. Magnusson, Nature, 1957, 180, 1200–1200 CrossRef CAS PubMed.
- J.-M. Beaulieu and R. R. Gainetdinov, Pharmacol. Rev., 2011, 63, 182–217 CrossRef CAS PubMed.
- J. Vatrál, R. Boča and W. Linert, Monatsh. Chem., 2015, 146, 1799–1805 CrossRef.
- M. D. Hawley, S. V. Tatawawadi, S. Piekarski and R. N. Adams, J. Am. Chem. Soc., 1967, 89, 447–450 CrossRef CAS PubMed.
- M. Bisaglia, S. Mammi and L. Bubacco, J. Biol. Chem., 2007, 282, 15597–15605 CrossRef CAS PubMed.
-
The IUPAC Compendium of Chemical Terminology: The Gold Book, International Union of Pure and Applied Chemistry (IUPAC), ed. V. Gold, Research Triangle Park, NC, 4th edn, 2019 Search PubMed.
- R. N. Adams, Anal. Chem., 1976, 48, 1126A–1138A CrossRef CAS PubMed.
-
J. B. Justice, in Voltammetry in the Neurosciences: Principles, Methods, and Applications, The Springer Science+Business Media New York, 1987, pp. 3–52 Search PubMed.
- P. T. Kissinger, J. B. Hart and R. N. Adams, Brain Res., 1973, 55, 209–213 CrossRef CAS PubMed.
- R.-J. Ni, Z.-H. Huang, Y.-M. Shu, Y. Wang, T. Li and J.-N. Zhou, Neurosci. Bull., 2018, 34, 405–418 CrossRef PubMed.
- R. M. Wightman, L. J. May, L. J. May and A. C. Michael, Anal. Chem., 1988, 60, 769–779 CrossRef PubMed.
- G. J. Kant and J. L. Meyerhoff, Life Sci., 1978, 23, 2111–2117 CrossRef CAS PubMed.
- S. R. Philips, A. M. Robson and A. A. Boulton, J. Neurochem., 1982, 38, 1106–1110 CrossRef CAS PubMed.
- C. C. Loullis, J. N. Hingtgen, P. A. Shea and M. H. Aprison, Pharmacol., Biochem. Behav., 1980, 12, 959–963 CrossRef CAS PubMed.
- R. D. Myers and L. Gurley-Orkin, Brain Res. Bull., 1985, 14, 477–483 CrossRef CAS PubMed.
- R. D. Myers and P. J. Knott, Ann. N. Y. Acad. Sci., 1986, 473, 1–560 CrossRef.
- T. Zetterström, T. Sharp, C. A. Marsden and U. Ungerstedt, J. Neurochem., 1983, 41, 1769–1773 CrossRef PubMed.
- I. N. Mefford, J. Neurosci. Methods, 1981, 3, 207–224 CrossRef CAS PubMed.
- W. H. Church, J. B. Justice and L. D. Byrd, Eur. J. Pharmacol., 1987, 139, 345–348 CrossRef CAS PubMed.
- A. D. Smith, R. J. Olson and J. B. Justice, J. Neurosci. Methods, 1992, 44, 33–41 CrossRef CAS PubMed.
- L. H. Parsons and J. B. Justice, J. Neurochem., 1992, 58, 212–218 CrossRef CAS PubMed.
- H. J. Yim and R. A. Gonzales, Alcohol, 2000, 22, 107–115 CrossRef CAS PubMed.
- H. Gu, E. L. Varner, S. R. Groskreutz, A. C. Michael and S. G. Weber, Anal. Chem., 2015, 87, 6088–6094 CrossRef CAS PubMed.
- T. Palomäki, E. Peltola, S. Sainio, N. Wester, O. Pitkänen, K. Kordas, J. Koskinen and T. Laurila, Biosens. Bioelectron., 2019, 123, 281–284 CrossRef PubMed.
- P. Hashemi, E. C. Dankoski, R. Lama, K. M. Wood, P. Takmakov and R. M. Wightman, Proc. Natl. Acad. Sci. U. S. A., 2012, 109, 11510–11515 CrossRef CAS PubMed.
- P. Hashemi, E. C. Dankoski, J. Petrovic, R. B. Keithley and R. M. Wightman, Anal. Chem., 2009, 81, 9462–9471 CrossRef CAS PubMed.
- B. E. K. Swamy and B. J. Venton, Analyst, 2007, 132, 876 RSC.
- S. H. Heruye, T. J. Warren, J. A. Kostansek IV, S. B. Draves, S. A. Matthews, P. J. West, K. A. Simeone and T. A. Simeone, Nutrients, 2022, 14, 613 CrossRef CAS PubMed.
- R. A. Grünewald, Brain Res. Rev., 1993, 18, 123–133 CrossRef PubMed.
- E. Peltola, N. Wester, K. B. Holt, L.-S. Johansson, J. Koskinen, V. Myllymäki and T. Laurila, Biosens. Bioelectron., 2017, 88, 273–282 CrossRef CAS PubMed.
- A. W. Sternson, R. McCreery, B. Feinberg and R. N. Adams, J. Electroanal. Chem. Interfacial Electrochem., 1973, 46, 313–321 CrossRef CAS.
- J. A. Stamford, Z. L. Kruk and J. Millar, Brain Res., 1984, 299, 289–295 CrossRef CAS PubMed.
- M. Miele and M. Fillenz, J. Neurosci. Methods, 1996, 70, 15–19 CrossRef CAS PubMed.
- K. Lönnrot, T. MetsÄ-KetelÄ, G. Molnár, J.-P. Ahonen, M. Latvala, J. Peltola, T. PietilÄ and H. Alho, Free Radical Biol. Med., 1996, 21, 211–217 CrossRef PubMed.
- L. Xiang, P. Yu, J. Hao, M. Zhang, L. Zhu, L. Dai and L. Mao, Anal. Chem., 2014, 86, 3909–3914 CrossRef CAS PubMed.
- F. Cao, L. Zhang and Y. Tian, J. Electroanal. Chem., 2016, 781, 278–283 CrossRef CAS.
- S. Rantataro, L. Ferrer Pascual and T. Laurila, Sci. Rep., 2022, 12, 20225 CrossRef CAS PubMed.
- R. D. O'Neill and J. P. Lowry, Behav. Brain Res., 1995, 71, 33–49 CrossRef PubMed.
- A. So and B. Thorens, J. Clin. Invest., 2010, 120, 1791–1799 CrossRef CAS PubMed.
- F. M. Rosenbloom, JAMA, 1967, 202, 175 CrossRef CAS PubMed.
- H. Reiber, M. Ruff and M. Uhr, Clin. Chim. Acta, 1993, 217, 163–173 CrossRef CAS PubMed.
- J. F. Stover, K. Lowitzsch and O. S. Kempski, Neurosci. Lett., 1997, 238, 25–28 CrossRef CAS PubMed.
- F. Gonon, M. Buda, M. Buda, R. Cespuglio, R. Cespuglio, M. Jouvet and J.-F. Pujol, Nature, 1980, 286, 902–904 CrossRef CAS PubMed.
- E. D. Abercrombie, K. A. Keefe, D. S. DiFrischia and M. J. Zigmond, J. Neurochem., 1989, 52, 1655–1658 CrossRef CAS PubMed.
- F. G. Gonon, F. Navarre and M. J. Buda, Anal. Chem., 1984, 56, 573–575 CrossRef CAS PubMed.
- D. L. Robinson, A. Hermans, A. Hermans, A. T. Seipel and R. M. Wightman, Chem. Rev., 2008, 108, 2554–2584 CrossRef CAS PubMed.
- G. S. Wilson and R. Gifford, Biosens. Bioelectron., 2005, 20, 2388–2403 CrossRef CAS PubMed.
- L. Pellerin and P. J. Magistretti, Neuroscientist, 2004, 10, 53–62 CrossRef CAS PubMed.
- N. Prakash and W. Wurst, Cell. Mol. Life Sci., 2006, 63, 187–206 CrossRef CAS PubMed.
- A. G. Yocky and D. P. Covey, Pharmacol., Biochem. Behav., 2021, 200, 173078 CrossRef CAS PubMed.
- D. L. Robinson, B. J. Venton, M.
L. A. V. Heien and R. M. Wightman, Clin. Chem., 2003, 49, 1763–1773 CrossRef CAS PubMed.
- A. Grace and B. Bunney, J. Neurosci., 1984, 4, 2866–2876 CrossRef CAS PubMed.
- M. A. Ungless and A. A. Grace, Trends Neurosci., 2012, 35, 422–430 CrossRef CAS PubMed.
- C. Liu and P. S. Kaeser, Curr. Opin. Neurobiol., 2019, 57, 46–53 CrossRef CAS PubMed.
- S. J. Cragg and M. E. Rice, Trends Neurosci., 2004, 27, 270–277 CrossRef CAS PubMed.
- F. Gonon, J. Neurosci., 1997, 17, 5972–5978 CrossRef CAS PubMed.
- C. Liu, P. Goel and P. S. Kaeser, Nat. Rev. Neurosci., 2021, 22, 345–358 CrossRef CAS PubMed.
- Y. Liu, K. Ai and L. Lu, Chem. Rev., 2014, 114, 5057–5115 CrossRef CAS PubMed.
- S. Hong, Y. S. Na, S. Choi, I. T. Song, W. Y. Kim and H. Lee, Adv. Funct. Mater., 2012, 22, 4711–4717 CrossRef CAS.
- A. N. Patel, S. Tan, T. S. Miller, J. V. Macpherson and P. R. Unwin, Anal. Chem., 2013, 85, 11755–11764 CrossRef CAS PubMed.
- E. Peltola, S. Sainio, K. B. Holt, T. Palomäki, J. Koskinen and T. Laurila, Anal. Chem., 2018, 90, 1408–1416 CrossRef CAS PubMed.
- W. Harreither, R. Trouillon, P. Poulin, W. Neri, A. G. Ewing and G. Safina, Anal. Chem., 2013, 85, 7447–7453 CrossRef CAS PubMed.
- E. Peltola, A. Aarva, S. Sainio, J. J. Heikkinen, N. Wester, V. Jokinen, J. Koskinen and T. Laurila, Phys. Chem. Chem. Phys., 2020, 22, 16630–16640 RSC.
- A. Kousar, E. Peltola and T. Laurila, ACS Omega, 2021, 6, 26391–26403 CrossRef CAS PubMed.
- N. Wisniewski and M. Reichert, Colloids Surf., B, 2000, 18, 197–219 CrossRef CAS PubMed.
- C. A. Homsy, J. Biomed. Mater. Res., 1970, 4, 341–356 CrossRef CAS PubMed.
- J. W. Salatino, K. A. Ludwig, T. D. Y. Kozai and E. K. Purcell, Nat. Biomed. Eng., 2017, 1, 862–877 CrossRef CAS PubMed.
- A. Carnicer-Lombarte, S.-T. Chen, G. G. Malliaras and D. G. Barone, Front. Bioeng. Biotechnol., 2021, 9, 622524 CrossRef PubMed.
- S. M. Wellman and T. D. Y. Kozai, ACS Chem. Neurosci., 2017, 8, 2578–2582 CrossRef CAS PubMed.
- V. S. Polikov, P. A. Tresco and W. M. Reichert, J. Neurosci. Methods, 2005, 148, 1–18 CrossRef PubMed.
- J. M. Anderson, A. Rodriguez and D. T. Chang, Semin. Immunol., 2008, 20, 86–100 CrossRef CAS PubMed.
- T. D. Y. Kozai, N. B. Langhals, P. R. Patel, X. Deng, H. Zhang, K. L. Smith, J. Lahann, N. A. Kotov and D. R. Kipke, Nat. Mater., 2012, 11, 1065–1073 CrossRef CAS PubMed.
- T. D. Y. Kozai, A. S. Jaquins-Gerstl, A. L. Vazquez, A. C. Michael and X. T. Cui, ACS Chem. Neurosci., 2014, 6, 48–67 CrossRef PubMed.
- N. Elgrishi, K. J. Rountree, B. D. McCarthy, E. S. Rountree, T. T. Eisenhart and J. L. Dempsey, J. Chem. Educ., 2018, 95, 197–206 CrossRef CAS.
- N. T. Rodeberg, S. G. Sandberg, J. A. Johnson, P. E. M. Phillips and R. M. Wightman, ACS Chem. Neurosci., 2017, 8, 221–234 CrossRef CAS PubMed.
- S. H. DuVall and R. L. McCreery, J. Am. Chem. Soc., 2000, 122, 6759–6764 CrossRef CAS.
- J. A. Behan, F. Grajkowski, D. R. Jayasundara, L. Vilella-Arribas, M. García-Melchor and P. E. Colavita, Electrochim. Acta, 2019, 304, 221–230 CrossRef CAS.
- D. L. Swinya, D. Martín-Yerga, M. Walker and P. R. Unwin, J. Phys. Chem. C, 2022, 126, 13399–13408 CrossRef CAS PubMed.
- E. Laviron, J. Electroanal. Chem. Interfacial Electrochem., 1984, 164, 213–227 CrossRef CAS.
- M. R. Deakin, P. M. Kovach, K. J. Stutts and R. M. Wightman, Anal. Chem., 1986, 58, 1474–1480 CrossRef CAS PubMed.
- M. R. Deakin and R. M. Wightman, J. Electroanal. Chem. Interfacial Electrochem., 1986, 206, 167–177 CrossRef CAS.
- E. Peltola, J. J. Heikkinen, K. Sovanto, S. Sainio, A. Aarva, S. Franssila, V. Jokinen and T. Laurila, J. Mater. Chem. B, 2017, 45, 9033–9044 RSC.
-
K. C. Dee, D. A. Puleo and R. Bizios, An Introduction To Tissue-Biomaterial Interactions: Tissue-Biomaterial, John Wiley & Sons, Inc., New York, USA, 2002 Search PubMed.
- G. Rudolph, T. Virtanen, M. Ferrando, C. Güell, F. Lipnizki and M. Kallioinen, J. Membr. Sci., 2019, 588, 117221 CrossRef CAS.
- P. A. Fritz, B. Bera, J. van den Berg, I. Visser, J. M. Kleijn, R. M. Boom and C. G. P. H. Schroën, Langmuir, 2021, 37, 6549–6555 CrossRef CAS PubMed.
- Y. Zhang, S. Qi, Z. Liu, Y. Shi, W. Yue and C. Yi, Mater. Sci. Eng., C, 2016, 61, 207–213 CrossRef CAS PubMed.
- D. Patrascu, I. David, V. David, C. Mihailciuc, I. Stamatin, J. Ciurea, L. Nagy, G. Nagy and A. A. Ciucu, Sens. Actuators, B, 2011, 156, 731–736 CrossRef CAS.
- H. Bahadori, M. R. Majidi and E. Alipour, Microchem. J., 2021, 161, 105724 CrossRef CAS.
- S. Harold Díaz, H. Walter Torres, G. Fernando and E. Larmat, J. Phys.: Conf. Ser., 2018, 1119, 012014 CrossRef.
- L. Kiss, V. David, I. G. David, P. Lazăr, C. Mihailciuc, I. Stamatin, A. Ciobanu, C. D. Ştefănescu, L. Nagy, G. Nagy and A. A. Ciucu, Talanta, 2016, 160, 489–498 CrossRef CAS PubMed.
- M. Raj, P. Gupta, R. N. Goyal and Y.-B. Shim, Sens. Actuators, B, 2017, 239, 993–1002 CrossRef CAS.
- R. N. Goyal, V. K. Gupta, N. Bachheti and R. A. Sharma, Electroanalysis, 2008, 20, 757–764 CrossRef CAS.
- A. Soleh, P. Kanatharana, P. Thavarungkul and W. Limbut, Microchem. J., 2020, 153, 104379 CrossRef CAS.
- B. D. Ratner, Regener. Biomater., 2016, 3, 107–110 CrossRef CAS PubMed.
- M. Gulino, D. Kim, S. Pané, S. D. Santos and A. P. Pêgo, Front. Neurosci., 2019, 13, 689 CrossRef PubMed.
- N. Lewinski, V. Colvin and R. Drezek, Small, 2008, 4, 26–49 CrossRef CAS PubMed.
- C. Liao, Y. Li and S. C. Tjong, Int. J. Mol. Sci., 2018, 19, 3564 CrossRef PubMed.
- R. Madannejad, N. Shoaie, F. Jahanpeyma, M. H. Darvishi, M. Azimzadeh and H. Javadi, Chem.-Biol. Interact., 2019, 307, 206–222 CrossRef CAS PubMed.
- B. Kong, J. H. Seog, L. M. Graham and S. B. Lee, Nanomedicine, 2011, 6, 929–941 CrossRef CAS PubMed.
- J. M. Wörle-Knirsch, K. Pulskamp and H. F. Krug, Nano Lett., 2006, 6, 1261–1268 CrossRef PubMed.
- N. Isoaho, E. Peltola, S. Sainio, J. Koskinen and T. Laurila, RSC Adv., 2018, 8, 35802–35812 RSC.
- A. Klymov, C. T. Rodrigues Neves, J. te Riet, M. J. H. Agterberg, E. A. M. Mylanus, A. F. M. Snik, J. A. Jansen and X. F. Walboomers, Hear. Res., 2015, 320, 11–17 CrossRef CAS PubMed.
- M. Li, H. Zhou, T. Li, C. Li, Z. Xia and Y. Y. Duan, Neural Regener. Res., 2015, 10, 2048–2053 CrossRef CAS PubMed.
- J. Wandiyanto, D. Linklater, P. Perera, A. Orlowska, V. Truong, H. Thissen, S. Ghanaati, V. Baulin, R. Crawford, S. Juodkazis and E. Ivanova, Materials, 2018, 11, 605 CrossRef PubMed.
- Z. Bérces, J. Pomothy, Á. C. Horváth, T. Kőhidi, É. Benyei, Z. Fekete, E. Madarász and A. Pongrácz, J. Neural Eng., 2018, 15, 056030 CrossRef PubMed.
- L. Song, K. Wang, Y. Li and Y. Yang, Colloids Surf., B, 2016, 148, 49–58 CrossRef CAS PubMed.
- W. Chen, S. Han, W. Qian, S. Weng, H. Yang, Y. Sun, L. G. Villa-Diaz, P. H. Krebsbach and J. Fu, Nanoscale, 2018, 10, 3556–3565 RSC.
- I. Smith, M. Haag, C. Ugbode, D. Tams, M. Rattray, S. Przyborski, A. Bithell and B. J. Whalley, Neurosci. Lett., 2015, 609, 198–202 CrossRef CAS PubMed.
- D. N. Rocha, J. P. Ferraz-Nogueira, C. C. Barrias, J. B. Relvas and A. P. Pêgo, Front. Cell. Neurosci., 2015, 9, 377 Search PubMed.
- K. C. Spencer, J. C. Sy, R. Falcón-Banchs and M. J. Cima, Lab Chip, 2017, 17, 795–804 RSC.
- C. Humpel, Neuroscience, 2015, 305, 86–98 CrossRef CAS PubMed.
- J. Yang, A. C. Charif, J. E. Puskas, H. Phillips, K. J. Shanahan, J. Garsed, A. Fleischman, K. Goldman, M. T. Luebbers, S. M. Dombrowski and M. G. Luciano, J. Mech. Behav. Biomed. Mater., 2015, 45, 83–89 CrossRef CAS PubMed.
- B. D. Winslow, M. B. Christensen, W.-K. Yang, F. Solzbacher and P. A. Tresco, Biomaterials, 2010, 31, 9163–9172 CrossRef CAS PubMed.
- J. R. Eles, A. L. Vazquez, N. R. Snyder, C. Lagenaur, M. C. Murphy, T. D. Y. Kozai and X. T. Cui, Biomaterials, 2017, 113, 279–292 CrossRef CAS PubMed.
- S. M. Wellman and T. D. Y. Kozai, Biomaterials, 2018, 164, 121–133 CrossRef CAS PubMed.
- D. Hammer, D.-H. Kim, A. Lozzi, A. Boretsky, M. Ye and C. Welle, SPIE Newsroom, 2016 DOI:10.1117/2.1201601.006321.
- J. Masís, D. Mankus, S. B. E. Wolff, G. Guitchounts, M. Joesch and D. D. Cox, Sci. Rep., 2018, 8, 5184 CrossRef PubMed.
- P. Puthongkham and B. J. Venton, Analyst, 2020, 145, 1087–1102 RSC.
- B. J. Venton and Q. Cao, Analyst, 2020, 145, 1158–1168 RSC.
- S. Mena, M. Visentin, C. E. Witt, L. E. Honan, N. Robins and P. Hashemi, ACS Meas. Sci. Au, 2022, 2, 241–250 CrossRef CAS PubMed.
- C. W. Atcherley, N. D. Laude, K. L. Parent and M. L. Heien, Langmuir, 2013, 29, 14885–14892 CrossRef CAS PubMed.
- A. Abdalla, C. W. Atcherley, P. Pathirathna, S. Samaranayake, B. Qiang, E. Peña, S. L. Morgan, M. L. Heien and P. Hashemi, Anal. Chem., 2017, 89, 9703–9711 CrossRef CAS PubMed.
- C. J. Meunier, G. S. McCarty and L. A. Sombers, Anal. Chem., 2019, 91, 7319–7327 CrossRef CAS PubMed.
- P. Puthongkham, J. Rocha, J. R. Borgus, M. Ganesana, Y. Wang, Y. Chang, A. Gahlmann and B. J. Venton, Anal. Chem., 2020, 92, 10485–10494 CrossRef CAS PubMed.
- B. D. Bath, D. J. Michael, B. J. Trafton, J. D. Joseph, P. L. Runnels and R. M. Wightman, Anal. Chem., 2000, 72, 5994–6002 CrossRef CAS PubMed.
- A. L. Hensley, A. R. Colley and A. E. Ross, Anal. Chem., 2018, 90, 8642–8650 CrossRef CAS PubMed.
- S. E. Cooper and B. J. Venton, Anal. Bioanal. Chem., 2009, 394, 329–336 CrossRef CAS PubMed.
- B. P. Jackson, S. M. Dietz and R. M. Wightman, Anal. Chem., 1995, 67, 1115–1120 CrossRef CAS PubMed.
- M. T. Cryan and A. E. Ross, Anal. Chem., 2019, 91, 5987–5993 CrossRef CAS PubMed.
- S. E. Calhoun, C. J. Meunier, C. A. Lee, G. S. McCarty and L. A. Sombers, ACS Chem. Neurosci., 2019, 10, 2022–2032 CrossRef CAS PubMed.
- K. M. Glanowska, B. J. Venton and S. M. Moenter, J. Neurosci., 2012, 32, 14664–14669 CrossRef CAS PubMed.
- M. Hersey, M. Reneaux, S. N. Berger, S. Mena, A. M. Buchanan, Y. Ou, N. Tavakoli, L. P. Reagan, C. Clopath and P. Hashemi, J. Neuroinflammation, 2022, 19, 167 CrossRef CAS PubMed.
- J. R. Borgus, P. Puthongkham and B. J. Venton, J. Neurochem., 2020, 153, 216–229 CrossRef CAS PubMed.
- M. T. Cryan and A. E. Ross, Analyst, 2019, 144, 249–257 RSC.
- L. R. Wilson, C. A. Lee, C. F. Mason, S. Khodjaniyazova, K. B. Flores, D. C. Muddiman and L. A. Sombers, ACS Meas. Sci. Au, 2022, 2, 120–131 CrossRef CAS PubMed.
- P. Puthongkham, S. T. Lee and B. J. Venton, Anal. Chem., 2019, 91, 8366–8373 CrossRef CAS PubMed.
- A. N. Perry, M. T. Cryan and A. E. Ross, Anal. Bioanal. Chem., 2021, 413, 6727–6735 CrossRef CAS PubMed.
- A. Jaquins-Gerstl and A. C. Michael, J. Neurosci. Methods, 2009, 183, 127–135 CrossRef PubMed.
- Z. Shao, P. Puthongkham, K. Hu, R. Jia, M. V. Mirkin and B. J. Venton, Electrochim. Acta, 2020, 361, 137032 CrossRef CAS PubMed.
- Q. Cao, D. K. Hensley, N. V. Lavrik and B. J. Venton, Carbon, 2019, 155, 250–257 CrossRef CAS PubMed.
- Y. Li, R. Jarosova, M. E. Weese-Myers and A. E. Ross, Anal. Chem., 2022, 94, 4803–4812 CrossRef CAS PubMed.
- N. Xiao, E. Privman and B. J. Venton, ACS Chem. Neurosci., 2014, 5, 666–673 CrossRef CAS PubMed.
- M. D. Nguyen, S. T. Lee, A. E. Ross, M. Ryals, V. I. Choudhry and B. J. Venton, PLoS One, 2014, 9, e87165 CrossRef PubMed.
- E. S. Bucher, K. Brooks, M. D. Verber, R. B. Keithley, C. Owesson-White, S. Carroll, P. Takmakov, C. J. McKinney and R. M. Wightman, Anal. Chem., 2013, 85, 10344–10353 CrossRef CAS PubMed.
- S. Banerjee, S. McCracken, M. F. Hossain and G. Slaughter, Biosensors, 2020, 10, 101 CrossRef CAS PubMed.
- R. D. Armstrong and G. Horvai, Electrochim. Acta, 1990, 35, 1–7 CrossRef CAS.
- J. L. Ponchon, R. Cespuglio, F. Gonon, M. Jouvet and J. F. Pujol, Anal. Chem., 1979, 51, 1483–1486 CrossRef CAS PubMed.
- B. J. Venton, H. Zhang, P. A. Garris, P. E. M. Phillips, D. Sulzer and R. M. Wightman, J. Neurochem., 2003, 87, 1284–1295 CrossRef CAS PubMed.
- C. D. Blaha and R. F. Lane, Brain Res. Bull., 1983, 10, 861–864 CrossRef CAS PubMed.
- G. A. Gerhardt, A. F. Oke, G. Nagy, B. Moghaddam and R. N. Adams, Brain Res., 1984, 290, 390–395 CrossRef CAS PubMed.
- K. T. Kawagoe, P. A. Garris, D. J. Wiedemann and R. M. Wightman, Neuroscience, 1992, 51, 55–64 CrossRef CAS PubMed.
- M. L. A. V. Heien, P. E. M. Phillips, G. D. Stuber, A. T. Seipel and R. M. Wightman, Analyst, 2003, 128, 1413 RSC.
- A. Hermans, A. T. Seipel, C. E. Miller and R. M. Wightman, Langmuir, 2006, 22, 1964–1969 CrossRef CAS PubMed.
- C. Yang and B. J. Venton, Nanocarbons Electroanal., 2017, 55–83 CAS.
- C. B. Jacobs, T. L. Vickrey and B. J. Venton, Analyst, 2011, 136, 3557 RSC.
- N. Xiao and B. J. Venton, Anal. Chem., 2012, 84, 7816–7822 CrossRef CAS PubMed.
- A. C. Schmidt, X. Wang, Y. Zhu and L. A. Sombers, ACS Nano, 2013, 7, 7864–7873 CrossRef CAS PubMed.
- R. F. Vreeland, C. W. Atcherley, W. S. Russell, J. Y. Xie, D. Lu, N. D. Laude, F. Porreca and M. L. Heien, Anal. Chem., 2015, 87, 2600–2607 CrossRef CAS PubMed.
- I. M. Taylor, E. M. Robbins, K. A. Catt, P. A. Cody, C. L. Happe and X. T. Cui, Biosens. Bioelectron., 2017, 89, 400–410 CrossRef CAS PubMed.
- P. Puthongkham, C. Yang and B. J. Venton, Electroanalysis, 2018, 30, 1073–1081 CrossRef CAS PubMed.
-
C. Yang and B. J. Venton, in 2017 IEEE International Symposium on Medical Measurements and Applications (MeMeA), IEEE, Rochester, MN, USA, 2017, pp. 100–105 Search PubMed.
- Y. Wang, T. Yang, Y. Hasebe, Z. Zhang and D. Tao, Materials, 2018, 11, 1691 CrossRef PubMed.
- X. Li, Y. Jin, F. Zhu, R. Liu, Y. Jiang, Y. Jiang and L. Mao, Angew. Chem., Int. Ed., 2022, 61, e202208121 CAS.
- W. Li, J. Jin, T. Xiong, P. Yu and L. Mao, Angew. Chem., Int. Ed., 2022, 61, e202204134 CAS.
- D.-S. Kim, E.-S. Kang, S. Baek, S.-S. Choo, Y.-H. Chung, D. Lee, J. Min and T.-H. Kim, Sci. Rep., 2018, 8, 14049 CrossRef PubMed.
- S. Lakard, S. Lakard, I.-A. Pavel, I.-A. Pavel and B. Lakard, Biosensors, 2021, 11, 179 CrossRef CAS PubMed.
- M. K. Zachek, A. Hermans, R. M. Wightman and G. S. McCarty, J. Electroanal. Chem., 2008, 614, 113–120 CrossRef CAS PubMed.
- R. F. Lane and A. T. Hubbard, Anal. Chem., 1976, 48, 1287–1293 CrossRef CAS PubMed.
- Y. Zhao, S.-H. Li, J. Chu, Y.-P. Chen, W.-W. Li, H.-Q. Yu, G. Liu, Y.-C. Tian and Y. Xiong, Biosens. Bioelectron., 2012, 35, 115–122 CrossRef CAS PubMed.
- J. Li and X. Lin, Sens. Actuators, B, 2007, 124, 486–493 CrossRef CAS.
- K. Jackowska and P. Krysiński, Anal. Bioanal. Chem., 2013, 405, 3753–3771 CrossRef CAS PubMed.
- A. Aashish, N. K. Sadanandhan, K. P. Ganesan, U. N. Saraswathy Hareesh, S. Muthusamy and S. J. Devaki, ACS Omega, 2018, 3, 3489–3500 CrossRef CAS PubMed.
- J. Huang, Y. Liu, H. Hou and T. You, Biosens. Bioelectron., 2008, 24, 632–637 CrossRef CAS PubMed.
- M. D. Johnson, R. K. Franklin, M. D. Gibson, R. B. Brown and D. R. Kipke, J. Neurosci. Methods, 2008, 174, 62–70 CrossRef CAS PubMed.
- S. Palanisamy, Mater. Res. Express, 2014, 1, 045020 CrossRef CAS.
- T.-C. Tsai, C.-X. Guo, H.-Z. Han, Y.-T. Li, Y.-Z. Huang, C.-M. Li and J.-J. J. Chen, Analyst, 2012, 137, 2813 RSC.
- S. Sainio, E. Leppänen, E. Mynttinen, T. Palomäki, N. Wester, J. Etula, N. Isoaho, E. Peltola, J. Koehne, M. Meyyappan, J. Koskinen and T. Laurila, Mol. Neurobiol., 2020, 57, 179–190 CrossRef CAS PubMed.
- C.-H. Chen and S.-C. Luo, ACS Appl. Mater. Interfaces, 2015, 7, 21931–21938 CrossRef CAS PubMed.
- C. Yang, C. B. Jacobs, M. D. Nguyen, M. Ganesana, A. G. Zestos, I. N. Ivanov, A. A. Puretzky, C. M. Rouleau, D. B. Geohegan and B. J. Venton, Anal. Chem., 2016, 88, 645–652 CrossRef CAS PubMed.
- E. Rand, A. Periyakaruppan, Z. Tanaka, D. A. Zhang, M. P. Marsh, R. J. Andrews, K. H. Lee, B. Chen, M. Meyyappan and J. E. Koehne, Biosens. Bioelectron., 2013, 42, 434–438 CrossRef CAS PubMed.
- T. Palomäki, Biosens. Bioelectron., 2018, 13 Search PubMed.
- S. Verma, P. Arya, A. Singh, J. Kaswan, A. Shukla, H. R. Kushwaha, S. Gupta and S. P. Singh, Biosens. Bioelectron., 2020, 165, 112347 CrossRef CAS PubMed.
- K. Min and Y. J. Yoo, Talanta, 2009, 80, 1007–1011 CrossRef CAS PubMed.
- A. Roychoudhury, S. Basu and S. K. Jha, Biosens. Bioelectron., 2016, 84, 72–81 CrossRef CAS PubMed.
- Z. Sun, S. Sun, X. Jiang, Y. Ai, W. Xu, L. Xie, H. Sun and Q. Liang, Biosens. Bioelectron., 2022, 211, 114367 CrossRef CAS PubMed.
- Z. Tavakolian-Ardakani, O. Hosu, C. Cristea, M. Mazloum-Ardakani and G. Marrazza, Sensors, 2019, 19, 2037 CrossRef CAS PubMed.
- L. Sun, H. Li, M. Li, C. Li, P. Li and B. Yang, J. Electroanal. Chem., 2016, 783, 167–175 CrossRef CAS.
- A. Ramachandran, S. Panda and S. Karunakaran Yesodha, Sens. Actuators, B, 2018, 256, 488–497 CrossRef CAS.
- G. Xu, Z. A. Jarjes, V. Desprez, P. A. Kilmartin and J. Travas-Sejdic, Biosens. Bioelectron., 2018, 107, 184–191 CrossRef CAS PubMed.
- R. Manikandan, P. N. Deepa and S. S. Narayanan, J. Solid State Electrochem., 2017, 21, 3567–3578 CrossRef CAS.
- M. Ates, A. S. Sarac, C. M. Turhan and N. E. Ayaz, Fibers Polym., 2009, 10, 46–52 CrossRef CAS.
- C. Tan, C. Tan, C. Tan, E. M. Robbins, B. Wu, B. Wu and X. T. Cui, Micromachines, 2021, 12, 208 CrossRef PubMed.
- A. Suzuki, T. A. Ivandini, K. Yoshimi, A. Fujishima, G. Oyama, T. Nakazato, N. Hattori, S. Kitazawa and Y. Einaga, Anal. Chem., 2007, 79, 8608–8615 CrossRef CAS PubMed.
- J. P. Seymour and D. R. Kipke, Biomaterials, 2007, 28, 3594–3607 CrossRef CAS PubMed.
- H.-K. Min, E. K. Ross, H. J. Jo, S. Cho, M. L. Settell, J. H. Jeong, P. S. Duffy, S.-Y. Chang, K. E. Bennet, C. D. Blaha and K. H. Lee, J. Neurosci., 2016, 36, 6022–6029 CrossRef CAS PubMed.
- E. W. Schluter, A. R. Mitz, J. F. Cheer and B. B. Averbeck, PLoS One, 2014, 9, e98692 CrossRef PubMed.
- K. Yoshimi, S. Kumada, A. Weitemier, T. Jo and M. Inoue, PLoS One, 2015, 10, e0130443 CrossRef PubMed.
- K. Mols, S. Musa, B. Nuttin, L. Lagae and V. Bonin, Sci. Rep., 2017, 7, 15642 CrossRef PubMed.
- H. N. Schwerdt, H. Shimazu, K. Amemori, S. Amemori, P. L. Tierney, D. J. Gibson, S. Hong, T. Yoshida, R. Langer, M. J. Cima and A. M. Graybiel, Proc. Natl. Acad. Sci. U. S. A., 2017, 114, 13260–13265 CrossRef CAS PubMed.
- H. N. Schwerdt, E. Zhang, M. J. Kim, T. Yoshida, L. Stanwicks, S. Amemori, H. E. Dagdeviren, R. Langer, M. J. Cima and A. M. Graybiel, Commun. Biol., 2018, 1, 144 CrossRef PubMed.
-
H. N. Schwerdt, D. J. Gibson, K. Amemori, L. L. Stanwicks, T. Yoshida, M. J. Cima and A. M. Graybiel, in Integrated Sensors for Biological and Neural Sensing, ed. H. Mohseni, SPIE, Online Only, United States, 2021, p. 20 Search PubMed.
- E. S. Bucher and R. M. Wightman, Rev. Anal. Chem., 2015, 8, 239–261 CrossRef CAS PubMed.
- J. Jeon, I. Hwang and T. D. Chung, Biomed. Eng. Lett., 2016, 6, 123–133 CrossRef.
- R. B. Keithley, P. Takmakov, E. S. Bucher, A. M. Belle, C. A. Owesson-White, J. Park and R. M. Wightman, Anal. Chem., 2011, 83, 3563–3571 CrossRef CAS PubMed.
- A. G. Zestos and B. J. Venton, J. Electrochem. Soc., 2018, 165, G3071–G3073 CrossRef CAS PubMed.
- T. Kokulnathan, A. Joseph Anthuvan, S.-M. Chen, V. Chinnuswamy and K. Kadirvelu, Inorg. Chem. Front., 2018, 5, 705–718 RSC.
- Z. Song, G. Sheng, Y. Cui, M. Li, Z. Song, C. Ding and X. Luo, Microchim. Acta, 2019, 186, 220 CrossRef PubMed.
- A. Üğe, D. Koyuncu Zeybek and B. Zeybek, J. Electroanal. Chem., 2018, 813, 134–142 CrossRef.
- J. Njagi, M. M. Chernov, M. M. Chernov, J. C. Leiter and S. Andreescu, Anal. Chem., 2010, 82, 989–996 CrossRef CAS PubMed.
- T. D. Thanh, J. Balamurugan, S. H. Lee, N. H. Kim and J. H. Lee, Biosens. Bioelectron., 2016, 81, 259–267 CrossRef CAS PubMed.
- T. Kishi, T. Fujie, H. Ohta and S. Takeoka, Frontiers in Sensors, 2021, 2, 725427 CrossRef.
- I. M. Taylor, N. A. Patel, N. C. Freedman, E. Castagnola, E. Castagnola, X. T. Cui and X. T. Cui, Anal. Chem., 2019, 91, 12917–12927 CrossRef CAS PubMed.
- G. Xiao, Y. Song, Y. Zhang, Y. Xing, H. Zhao, J. Xie, S. Xu, F. Gao, M. Wang, G. Xing and X. Cai, ACS Sens., 2019, 4, 1992–2000 CrossRef CAS PubMed.
- C. P. Ford, S. C. Gantz, P. E. M. Phillips and J. T. Williams, J. Neurosci., 2010, 30, 6975–6983 CrossRef CAS PubMed.
- J. B. Justice, J. Neurosci. Methods, 1993, 48, 263–276 CrossRef CAS PubMed.
- D. L. Robinson, M. L. A. V. Heien and R. M. Wightman, J. Neurosci., 2002, 22, 10477–10486 CrossRef CAS PubMed.
- A. G. Ewing, M. A. Dayton and R. M. Wightman, Anal. Chem., 1981, 53, 1842–1847 CrossRef CAS.
- K. T. Kawagoe, J. B. Zimmerman and R. M. Wightman, J. Neurosci. Methods, 1993, 48, 225–240 CrossRef CAS PubMed.
- J. A. Stamford, Brain Res. Rev., 1985, 10, 119–135 CrossRef CAS PubMed.
- F. B. Kamal Eddin and Y. Wing Fen, Sensors, 2020, 20, 1039 CrossRef PubMed.
- M. Lakshmanakumar, N. Nesakumar, A. J. Kulandaisamy and J. B. B. Rayappan, Measurement, 2021, 183, 109873 CrossRef.
- B. Si and E. Song, Chemosensors, 2018, 6, 1 CrossRef.
- J. Van Schoors, J. Viaene, Y. Van Wanseele, I. Smolders, B. Dejaegher, Y. Vander Heyden and A. Van Eeckhaut, J. Pharm. Biomed. Anal., 2016, 127, 136–146 CrossRef CAS PubMed.
- J. A. Johnson, N. T. Rodeberg and R. M. Wightman, Anal. Chem., 2018, 90, 7181–7189 CrossRef CAS PubMed.
- G. A. Woods, N. J. Rommelfanger and G. Hong, Matter, 2020, 3, 1087–1113 CrossRef PubMed.
- Y.-W. Cho, J.-H. Park, K.-H. Lee, T. Lee, Z. Luo and T.-H. Kim, Nano Convergence, 2020, 7, 40 CrossRef CAS PubMed.
- W. Yang, Y. Gong and W. Li, Front. Bioeng. Biotechnol., 2021, 8, 622923 CrossRef PubMed.
- M. Sajid, M. K. Nazal, M. Mansha, A. Alsharaa, S. M. S. Jillani and C. Basheer, TrAC, Trends Anal. Chem., 2016, 76, 15–29 CrossRef CAS.
- S. M. Won, E. Song, J. Zhao, J. Li, J. Rivnay and J. A. Rogers, Adv. Mater., 2018, 30, 1800534 CrossRef PubMed.
- P. Moshayedi, G. Ng, J. C. F. Kwok, G. S. H. Yeo, C. E. Bryant, J. W. Fawcett, K. Franze and J. Guck, Biomaterials, 2014, 35, 3919–3925 CrossRef CAS PubMed.
- R. Green and M. R. Abidian, Adv. Mater., 2015, 27, 7620–7637 CrossRef CAS PubMed.
- P. Fattahi, G. Yang, G. Kim and M. R. Abidian, Adv. Mater., 2014, 26, 1846–1885 CrossRef CAS PubMed.
- H. Yuk, B. Lu and X. Zhao, Chem. Soc. Rev., 2019, 48, 1642–1667 RSC.
- U. A. Aregueta-Robles, A. J. Woolley, L. A. Poole-Warren, N. H. Lovell and R. A. Green, Front. Neuroeng., 2014, 7, 15 CAS.
- E. Redolfi Riva and S. Micera, Bioelectron. Med., 2021, 7, 6 CrossRef PubMed.
- A. G. Harvey, E. W. Hill and A. Bayat, Expert Rev. Med. Devices, 2013, 10, 257–267 CrossRef CAS PubMed.
- C. A. R. Chapman, H. Chen, M. Stamou, J. Biener, M. M. Biener, P. J. Lein and E. Seker, ACS Appl. Mater. Interfaces, 2015, 7, 7093–7100 CrossRef CAS PubMed.
- E. Peltola, Front. Mater., 2019, 6, 202 CrossRef.
- S. Rantataro, I. Parkkinen, I. Pande, A. Domanskyi, M. Airavaara, E. Peltola and T. Laurila, Acta Biomater., 2022, 146, 235–247 CrossRef CAS PubMed.
- L. Gällentoft, L. M. E. Pettersson, N. Danielsen, J. Schouenborg, C. N. Prinz and C. E. Linsmeier, Biomaterials, 2015, 42, 172–183 CrossRef PubMed.
- E. Otte, A. Vlachos and M. Asplund, Cell Tissue Res., 2022, 387, 461–477 CrossRef PubMed.
-
J. L. Skousen, Sr., M. E. Merriam, O. Srivannavit, G. Perlin, K. D. Wise and P. A. Tresco, in Progress in Brain Research, Elsevier, 2011, vol. 194, pp. 167–180 Search PubMed.
- S. P. Lacour, G. Courtine and J. Guck, Nat. Rev. Mater., 2016, 1, 1–14 Search PubMed.
-
A. Carnicer Lombarte, D. G. Barone, I. Dimov, R. Hamilton, M. Prater, X. Zhao, A. Rutz, G. Malliaras, S. Lacour, C. Bryant, J. Fawcett and K. Franze, Mechanical matching of implant to host minimises foreign body reaction, 2019 Search PubMed.
- A. Pandikumar, G. T. S. How, T. P. See, F. S. Omar, S. Jayabal, K. Z. Kamali, N. Yusoff, A. Jamil, R. Ramaraj, S. A. John, H. N. Lim and N. M. Huang, RSC Adv., 2014, 4, 63296–63323 RSC.
- Y. Wang, S. Vaddiraju, B. Gu, F. Papadimitrakopoulos and D. J. Burgess, J. Diabetes Sci. Technol., 2015, 9, 966–977 CrossRef CAS PubMed.
- O. Veiseh, J. C. Doloff, M. Ma, A. J. Vegas, H. H. Tam, A. R. Bader, J. Li, E. Langan, J. Wyckoff, W. S. Loo, S. Jhunjhunwala, A. Chiu, S. Siebert, K. Tang, J. Hollister-Lock, S. Aresta-Dasilva, M. Bochenek, J. Mendoza-Elias, Y. Wang, M. Qi, D. M. Lavin, M. Chen, N. Dholakia, R. Thakrar, I. Lacík, G. C. Weir, J. Oberholzer, D. L. Greiner, R. Langer and D. G. Anderson, Nat. Mater., 2015, 14, 643–651 CrossRef CAS PubMed.
- R. Ding, N. C. Miller, K. M. Woeppel, X. T. Cui and T. D. B. Jacobs, Langmuir, 2022, 38, 7512–7521 CrossRef CAS PubMed.
- K. M. Woeppel and X. T. Cui, Adv. Healthcare Mater., 2021, 10, e2002150 CrossRef PubMed.
- C. A. R. Chapman, H. Chen, M. Stamou, P. J. Lein and E. Seker, Cell. Mol. Bioeng., 2016, 9, 433–442 CrossRef CAS PubMed.
- V. Onesto, L. Cancedda, M. L. Coluccio, M. Nanni, M. Pesce, N. Malara, M. Cesarelli, E. Di Fabrizio, F. Amato and F. Gentile, Sci. Rep., 2017, 7, 9841 CrossRef CAS PubMed.
- T. Laurila, A. Rautiainen, S. Sintonen, H. Jiang, E. Kaivosoja and J. Koskinen, Mater. Sci. Eng., C, 2014, 34, 446–454 CrossRef CAS PubMed.
- T. D. Y. Kozai, A. S. Jaquins-Gerstl, A. L. Vazquez, A. C. Michael and X. T. Cui, Biomaterials, 2016, 87, 157–169 CrossRef CAS PubMed.
- S. Sridar, M. A. Churchward, V. K. Mushahwar, K. G. Todd and A. L. Elias, Acta Biomater., 2017, 60, 154–166 CrossRef CAS PubMed.
- G. Joseph, R. P. Orme, T. Kyriacou, R. A. Fricker and P. Roach, ACS Omega, 2021, 6, 19901–19910 CrossRef CAS PubMed.
- R. Fabbri, E. Saracino, E. Treossi, R. Zamboni, V. Palermo and V. Benfenati, Nanoscale, 2021, 13, 4390–4407 RSC.
- Q. Liang, X. Xia, X. Sun, D. Yu, X. Huang, G. Han, S. M. Mugo, W. Chen and Q. Zhang, Adv. Sci., 2022, 9, 2201059 CrossRef CAS PubMed.
- M. Jorfi, J. L. Skousen, C. Weder and J. R. Capadona, J. Neural Eng., 2015, 12, 011001 CrossRef PubMed.
- T. Laurila, S. Sainio and M. A. Caro, Prog. Mater. Sci., 2017, 88, 499–594 CrossRef CAS.
- M. Devi, M. Vomero, E. Fuhrer, E. Castagnola, C. Gueli, S. Nimbalkar, M. Hirabayashi, S. Kassegne, T. Stieglitz and S. Sharma, J. Neural Eng., 2021, 18, 041007 CrossRef PubMed.
- Z. Hsine, R. Mlika, N. Jaffrezic-Renault and H. Korri-Youssoufi, Chemosensors, 2022, 10, 249 CrossRef CAS.
Footnote |
† These authors contributed equally to the manuscript. |
|
This journal is © The Royal Society of Chemistry 2023 |
Click here to see how this site uses Cookies. View our privacy policy here.