DOI:
10.1039/D3SC00517H
(Edge Article)
Chem. Sci., 2023,
14, 7381-7392
Local solvation structures govern the mixing thermodynamics of glycerol–water solutions†
Received
30th January 2023
, Accepted 12th June 2023
First published on 16th June 2023
Abstract
Glycerol is a major cryoprotective agent and is widely used to promote protein stabilization. By a combined experimental and theoretical study, we show that global thermodynamic mixing properties of glycerol and water are dictated by local solvation motifs. We identify three hydration water populations, i.e., bulk water, bound water (water hydrogen bonded to the hydrophilic groups of glycerol) and cavity wrap water (water hydrating the hydrophobic moieties). Here, we show that for glycerol experimental observables in the THz regime allow quantification of the abundance of bound water and its partial contribution to the mixing thermodynamics. Specifically, we uncover a 1
:
1 connection between the population of bound waters and the mixing enthalpy, which is further corroborated by the simulation results. Therefore, the changes in global thermodynamic quantity – mixing enthalpy – are rationalized at the molecular level in terms of changes in the local hydrophilic hydration population as a function of glycerol mole fraction in the full miscibility range. This offers opportunities to rationally design polyol water, as well as other aqueous mixtures to optimize technological applications by tuning mixing enthalpy and entropy based on spectroscopic screening.
Introduction
Glycerol (1,2,3-propanetriol), a flexible polyol containing three hydroxyl (OH) groups, is a ubiquitously used co-solvent. For instance, it is a major cryoprotective agent (CPA),1–4 and widely used to promote protein stabilization through preferential solvation.5–7 In cryobiology, the roles of water molecules are strongly modulated by the specific interactions with the CPA and can be classified into three categories: water released from the cell during freezing, water participating in intracellular ice formation and growth, and water bound to CPAs (e.g., glycerol molecules) and cytoplasmic proteins through hydrogen bonds (HBs) or electrostatic interactions that may hinder ice formation.8 The delicate balance of these water populations is dictated by the nature of the CPA and its mixing ratio with water. Molecular dynamics (MD) simulation studies can provide a molecular understanding of the underlying complex interplay of inter-molecular forces.9 Among these three water populations, the one bound to CPAs and proteins is the least understood, owing to the complexity of resolving the molecular details of the CPA–water interactions.9–11 Nonetheless, these interactions are key in determining the amount of water and CPA molecules in the protein first hydration shell and the balance between the three water populations in the mixture. These ultimately modulate the ability of water–CPA mixtures to preserve biological functionality by cryoprotection.12,13 The amount of water and CPA in the protein solvation shells was recently investigated by Chéron et al., who proposed that it is dictated by entropic effects related to volume exclusion and protein surface accessibility (i.e., to the size of the CPA) and by enthalpic effects due to H-Bonding.6 Weng et al. further proposed that the population of H-bonded water to various CPA polyols have a minor temperature dependence, but strongly vary with the nature of the polyol and its mole fraction.9 These studies suggest that water–CPA HB interactions, which are enthalpically favored, regulate the balance between released and bound water populations, hence impacting the different cryoprotection behavior of glycerol compared to other polyols, such as ethylene glycol.8,14,15
Despite intense interest and many compelling studies on the subject matter, the understanding of the role of water and CPA in cryobiology is to date mostly based on mean field thermodynamic and kinetic theories,9,16,17 and the glycerol–water mixture composition is generally adjusted heuristically to tune macroscopic properties such as viscosity. However, macroscopic and solvation properties cannot be predicted or extrapolated from CPA composition, yet. The structural, thermodynamical and solvation properties of even the simple CPA, i.e., glycerol–water mixtures are subtly interconnected and strongly depend on the mixing ratio.4,6,18–22 Understanding these interconnections at the molecular level is a hitherto unmet challenge for both theory and experiments that require reconciling local and macroscopic properties, and it is the missing link to intentionally optimize the fraction of glycerol in solvent mixtures used in technological applications, including cryopreservation. Moreover, owing to its simple molecular structure and complete miscibility, glycerol–water mixtures have great value as a model system to study the structural heterogeneity in binary mixtures and its connection to their thermodynamic properties. Compared to a more hydrophobic model system, such as alcohol–water mixtures, the higher number of OH moieties in the glycerol molecule (3 vs. 1) offers an excellent case to investigate the impact of hydrophilic interactions on mixing thermodynamics. In glycerol–water mixtures, hydrophilic interactions are expected to outweigh contributions from hydrophobic solvation.23 However, so far a quantitative correlation between local hydration water motifs and mixing enthalpy is missing.
Given its importance in applications and in fundamental solvation science, the structure and dynamics of glycerol aqueous solutions have been extensively investigated with various experimental tools, such as dielectric spectroscopy,20,24–28 nuclear magnetic resonance (NMR),29 X-ray photoelectron spectroscopy,24 infrared (IR) spectroscopy,4,24,30,31 as well as with computer simulations techniques.1,32,33 These studies provided valuable insight on the molecular structural changes that occur with changing glycerol content. For instance, Feldman et al. reported that water–water interactions overwhelm the water–glycerol and glycerol–glycerol interactions in glycerol–water mixtures with less than 55% glycerol content.27,28 In another study, Ahmed et al. further showed that the HB-network of water adopts three distinct states with increasing glycerol concentration: one in which bulk-like water dominates and solvates glycerol molecules at high water content, a second one containing confined water populations and a third state dominated by bulk glycerol at high glycerol content.24 In light of these results, it is still unknown whether and how such structural transformations of a solvent mixture dictate the mixing enthalpy of glycerol and water, and by extension the thermodynamics of hydrophobic solutes and their interactions in mixtures. Answering such fundamental questions is the basis for tailoring glycerol–water interactions to optimize cryoprotective and solvation properties, e.g., regulating the balance between released and bound water populations in cryoprotection and the composition of the solvation shells of biomolecules. However, doing this requires going beyond average thermodynamic quantities, as measured by standard calorimetry techniques, and to dissect the overall changes in entropy and enthalpy into partial contribution which can be 1
:
1 correlated to local hydration contributions. While theoretical approaches show promising results in this direction,34 what was missing yet were experimental observables that allow to quantify these local quantities and therefore provide a check for theory.
Terahertz (THz) absorption spectroscopy has the potential to address this challenge, since it directly probes the collective motions of water and that of the solvation shells. In previous studies, we introduced difference THz spectroscopy to characterize hydration water networks,24,35–39 where the THz spectrum of the solute and its hydration shell is obtained by subtracting the volume-scaled absorption spectrum of bulk water from the absorption spectrum of the solution of interest. By calculating the difference THz spectra, we identified spectroscopic features that directly probe the distinct structural motifs of HB-network around hydrophilic and hydrophobic moieties.35 In a more general approach we will show that these spectroscopic observables can be correlated with partial hydrophilic and hydrophobic contributions to solvation entropy and enthalpy. While being developed on a large dataset of hydrated alcohols, and thereby focusing mostly on the entropic part; this concept is more general and can also be applied to water hydrating other solutes and biomolecules.40–43 Here, we exploit such concept in a combined experimental–theoretical approach to reveal that solvation thermodynamics of glycerol–water mixtures can be traced back to subtle changes occurring at the molecular level specifically for the HB motifs around the hydrophilic moieties in the glycerol–water network. Accompanying classical MD simulations were used to characterize the associated structural motifs and to independently quantify the impact of changes in the water structure on the global mixing enthalpy in water–glycerol solutions. Our study maps a direct connection between mixing enthalpy and the local hydration motifs associated with water molecules bound to glycerol hydrophilic groups across the whole miscibility range of glycerol–water mixtures.
This concept, THz calorimetry, is as well quantitative as predictive, since the same scaling between spectroscopic and thermodynamic quantities can be used now for other solutes in the future. These results establish a direct link between macroscopic/thermodynamic and microscopic/structural changes on a molecular level occurring as a function of the glycerol
:
water mixing ratio, paving the way to a rational tuning of solvation properties of these ubiquitously used binary solvents.
Principles of THz-calorimetry
Local water structures close to the hydrophilic and hydrophobic moieties of a solute provide two distinct fingerprints in difference FIR/THz spectra that are well distinguished from that of bulk water. These two populations are illustrated in Fig. 1 for the case of hydrated glycerol. On the one hand, water molecules around hydrophobic (CH1–4) groups are not H-bonded to the solute and form a water–water HB-network wrapped around the solute. These wrap water around hydrophobic solutes, create a two-dimensional network of HBs that surrounds the cavity formed by the solute in the liquid (denoted HB-wrap).37,44 Their characteristic THz-signature is found in the 100–150 cm−1 spectral region of HB-stretching modes. The slightly weaker water–water HBs formed within the wrap as compared to bulk water, cause a red-shift of the intermolecular stretch.43 The HB-wrap coexist with relatively weak interactions, such as secondary HBs that wrap water can form with the methyl groups. However, it is the collective stretching of the HBs composing the HB-wrap that characterize the spectroscopic response of the cavity-wrap population in the THz spectra.37 The more the water network is perturbed to accommodate the solute, the more the wrap fingerprint contributes to the difference FIR/THz spectrum. Therefore, the wrap population measures how good/bad a solute molecule fits in the water network. It is expected to disappear for solutes that do not perturb the water network (e.g. urea).45 On the other hand, “bound” water molecules H-bonded to the hydrophilic (OH) moieties have their imprint in the 350–600 cm−1 frequency range of water's librational mode. They originate from steric constraints in water rotational motions induced by the proximity to and direct H-bonding with the solutes. This causes a reduction in the amplitude of soft librational modes (around 350 cm−1) and an increase in that of hard librations (at 600 cm−1), resulting in a characteristic intensity increase in the >400 cm−1 frequency range in the difference spectra.37 Since the bound spectral feature extends outside of the frequency range probed in our FIR/THz spectra (50–600 cm−1), the maximum amplitude cannot be determined. However, the slope obtained by linearly fitting the intensity increase in the >400 cm−1 frequency is correlated to the bound water population.37 The correlation between the bound water population and the slope is further demonstrated in the ESI† by means of theoretical THz spectroscopy from ab initio simulations. Therefore, we will use the slope as a measure of the strength and number of attractive interactions that stabilize hydration of a solute.37
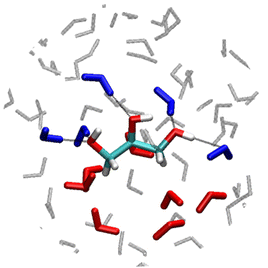 |
| Fig. 1 Schematic representation of the hydration shell of a glycerol molecule with bound (blue colored) and wrap (red colored) water molecules. The non-shell (bulk-like) water molecules are indicated with gray color. | |
THz-calorimetry relates these spectroscopic signatures to thermodynamic quantities, through direct correlation of the measured wrap and bound amplitudes with ΔS and ΔH. The main concepts of THz-calorimetry are summarized in the equations below.
| ΔGmixing = ΔGmixingwrap + ΔGmixingbound = (ΔHmixingwrap + ΔHmixingbound) − T(ΔSmixingwrap + ΔSmixingbound) | (1) |
with
| ΔHmixingwrap = Δ wrapδεwraphydration | (2) |
| ΔSmixingwrap = Δ wrapδεwraphydration | (3) |
| ΔHmixingbound = Δ boundδεboundhydration/Δν | (4) |
| ΔSmixingbound = Δ boundδεboundhydration/Δν | (5) |
where the solvation free energy Δ
Gmixing is exactly expressed as a sum of wrap (hydrophobic – cavity formation term) and bound (hydrophilic – solute insertion, attractive interactions term) contributions.
37 If the ratio between the spectroscopic observables and the calorimetric quantities is solute independent then both contributions can be directly quantified from the measured amplitude (for wrap) or slope (for bound) in the difference THz spectra. The related spectroscopic observables are
δεwrap/boundhydration and
δεboundhydration/Δ
ν, respectively, where
ε is the molar extinction coefficient as defined in
eqn (7) (see below). Δ
wrap/bound and Δ
wrap/bound are constant scaling factors (independent of the solute) that define the linear correlation between
δεwrap/boundhydration and hydration entropy and enthalpy.
37,44,46 Such linear correlation constants are fitted to reproduce the standard calorimetry data of the studied system, as previously introduced for a large set of alcohol solutes. This THz-calorimetry approach has been applied to solvated alcohol chains and was shown to provide total solvation entropy in excellent agreement with standard calorimetry as well as partial hydrophobic and hydrophilic contributions in agreement with MD simulations results.
37,44,46 For small solutes, such as alcohols or alkanes, Δ
bound < 0, Δ
bound ∼ 0, Δ
wrap < 0, Δ
wrap < 0,
i.e. the hydrophobic wrap population contributes mostly as an entropic cost of hydration, which is balanced by an enthalpic gain from the hydrophilic bound population. In previous works on alcohol solutes, we were able to quantitative interpret mixing entropy in terms of changes in the cavity-wrap population and the Δ
wrap scaling factor for the cavity wrap population was quantified to be 7.6 J cm dm
−3 K
−1. In this work, we focus exclusively on the bound water spectroscopic population and its quantitative correlation with mixing enthalpy. We show here for the first time that we can quantify and interpret at the molecular level the changes in mixing enthalpy of glycerol–water mixtures directly from the experimentally measured THz signature of the bound water population.
Results and discussion
Fig. 2a shows room temperature (20 °C) spectra of absorption coefficients (αsolution(ν)) of glycerol–water mixtures in the 30–600 cm−1 FIR/THz spectral range as a function of glycerol mole fraction (Xgly). From αsolution(ν), we calculate the frequency dependent molar extinction coefficient εsolution(ν) (following eqn (7)) as shown in Fig. 2b. As discussed in Section 1 of the ESI,† the estimated error due to signal-to-noise is on the order of 1% of the obtained εsolution(ν) spectra. The extinction coefficient of pure glycerol and pure water at three different temperatures (5 °C, 20 °C, and 40 °C) are shown in Fig. S2 of the ESI,† for comparison. Spectra in Fig. 2 include contributions from glycerol, bulk and hydration water, including glycerol modes at ∼140, 310, 415, 490, 560 cm−1, bulk water modes, as well as the specific bound and wrap hydration water fingerprints we are interested in.47
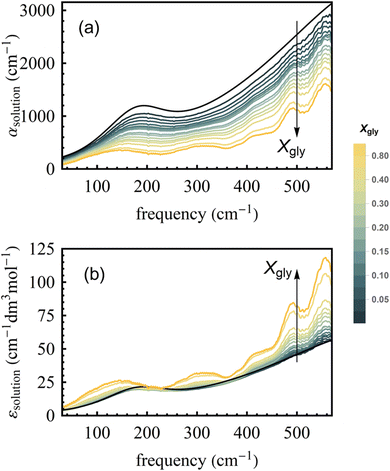 |
| Fig. 2 The frequency dependent (a) absorption coefficient and (b) average molar extinction coefficient of glycerol–water binary mixtures with various mole fraction of glycerol (Xgly). Arrows indicate increasing Xgly. | |
To disentangle the hydration water fingerprint, we calculate the effective molar extinction coefficient (εhydration(ν)), by subtracting the mole fraction-scaled extinction spectrum of bulk water (εwater) and of pure bulk glycerol (εglycerol) from the extinction spectrum of each mixture (see eqn (8)). As per definition εhydration gives exactly zero for the ideal case where the mixture is the sum of the two individual bulk components. Therefore, εhydration spectra shown in Fig. 3a report on the direct interactions between water and glycerol (and associated structural perturbations, if any).
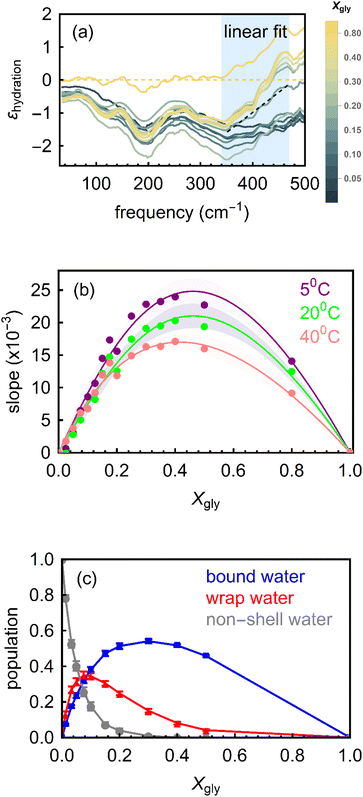 |
| Fig. 3 (a) Molar effective extinction coefficient (εhydration) at 20 °C as calculated by subtracting the bulk water and bulk glycerol spectra from the glycerol–water mixtures spectra (eqn (6)). The yellow dotted line represents pure water and pure glycerol. εhydration increases with frequency for all the solutions in the 350–450 cm−1 spectral region (gray shaded area). The dotted black line is the linear fit in that region for glycerol mole fraction; Xgly = 0.8, as an example. The slope obtained from such fit is then used for interpreting the spectral trends. (b) The slopes derived from experimental εhydration spectra are plotted for all Xgly at three different temperatures (5 °C, 20 °C and 40 °C, respectively). The lines are guided to the eye and the error bars are indicated by the shaded regions. (c) The populations of various types of water in glycerol–water mixtures as predicted by MD simulations. | |
Focusing first on the ∼100–250 cm−1 region that usually contains the signature of the cavity wrap population, we notice that this part of the spectra does not show any clear trend with Xgly. This can be explained by the overlap of the hydration water contribution with a low frequency solute mode in this frequency range, as is the case for the glycerol band at ∼140 cm−1, due to the generally low intensity of the wrap signature.37,44,46 Beyond the complexities raised by the overlap of wrap and glycerol modes, the absence of a well-defined wrap signature can also indicate minor perturbation of the water network wrapped around the solute (with respect to the bulk water reference state), i.e., glycerol molecules fit well within the water network25 (and vice versa at high Xgly). Since for the glycerol–water case it is difficult to quantitatively dissect wrap and glycerol contribution in the ∼100–250 cm−1 region, we will not further analyze the wrap population contribution, instead we focus on the higher frequency part of the THz spectrum.
In the ∼350–600 cm−1 region (i.e., the onset of the water libration band), Fig. 3a shows the frequency dependent εhydration for all the measured Xgly. The result is typical for bound water molecules interacting with polar solutes. Therefore, this feature is assigned to the formation of HBs between glycerol and bound water molecules. Here, instead of using the maximum amplitude of this band – which lies outside our accessible frequency range – we use the slope, i.e., the increase in amplitude in the range between 350 and 600 cm−1 as the spectroscopic observable. The slope (δεboundhydration/Δν) determined by linearly fitting the εhydration increase in the frequency range from 340 cm−1 to 475 cm−1 (blue shaded region in Fig. 3a, where 340 cm−1 is the turning point at which εhydration starts to rise in the experiments range) is assumed to be correlated to the population of bound water molecules in the glycerol–water mixtures.37,42
The experimentally determined slopes are shown in Fig. 3b as a function of Xgly, for three different temperatures. For low Xgly (<0.4), the slope increases monotonically with increasing glycerol content in the mixture, which signifies an increase in the effective population of bound water molecules. The slope reaches its maximum value at Xgly = 0.4, indicating that all binding sites of glycerol molecules in the system are saturated. Further increasing glycerol content beyond this point leads to a decrease in the number of bound waters (quantified by the slope) since the number of water molecules in the system is reduced and glycerol–water interactions are progressively replaced by glycerol–glycerol interactions. These results are consistent with previous studies24,25 that proposed a progressive structural transition from a state where bulk-like water dominates and solvates glycerol molecules at high water content (where number of bound water per glycerol Nbound is high), to a state containing confined water populations, where there are not enough bound water molecules to solvate all glycerol molecules (Nbound decrease with Xgly).
To further characterize these structural transformations, we performed classical MD simulations and evaluated the relative mole fractions of wrap and bound water populations, as well as of bulk-like water not involved in the hydration of glycerol solutes (denoted hereafter non-shell water) as a function of Xgly. We identify these three populations in the simulations based on structural criteria, i.e., on their proximity with respect to OH and hydrophobic glycerol moieties and their H-bonding properties (see Methods section and ref. 35 and 37 for details). The simulated variations in the three populations with Xgly are shown in Fig. 3c, as obtained by direct counting of water molecules belonging to each identified population. Most notably, the bound water population follows most closely the trend observed experimentally for the slope, i.e., first increasing with Xgly for low glycerol content, saturating at around Xgly = (0.3–0.4), and then decreasing with further increasing the glycerol mole fraction. The simulated cavity wrap water population shows a similar non-monotonic behavior but reaches saturation for much lower glycerol content (Xgly = 0.15). This is easily understood by considering that wrap water interacts much more weakly than bound water with glycerol (since it is not H-bonded), and therefore it is the easiest part of the hydration layer that is sacrificed as soon as the glycerol molecules in the system become too many to be fully hydrated. The small number of wrap water above Xgly > 0.15 and its almost bulk-like character in glycerol–water mixtures below Xgly = 0.15 (see Fig. 4) might also be one aspect why cannot unambiguously identify this population in the THz-spectra. The non-shell water population shows a straightforward monotonic decrease with Xgly since increasing numbers of water molecules are involved in the solvation of glycerol molecules as their concentration increases.
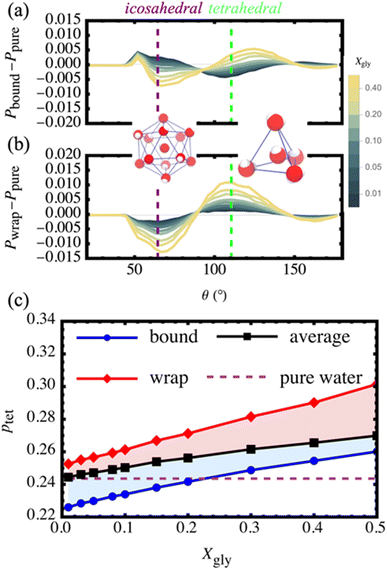 |
| Fig. 4 The three-body angle distributions for the (a) bound and (b) wrap waters exhibit a monotonic increase in the population of tetrahedrally-coordinated waters (θ = 109.5°) and a monotonic decrease in the population of icosahedrally-coordinated waters (θ = 64°) relative to pure water at 18 °C. (a) At low concentrations, the bound waters are more icosahedral and less tetrahedral than pure water. (b) Wrap waters show higher tetrahedral populations and lower icosahedral populations than pure water for the entire concentration range. (c) Here, we depict the population of tetrahedrally-coordinated waters ptet for wrap (red diamonds), sample-averaged (black squares), and bound (blue circles) as a function of glycerol mole faction. For reference, we indicate the pure water ptet with a horizontal dashed line. | |
To further corroborate our interpretation of the molecular arrangement of glycerol–water mixtures into bulk, bound and wrap populations, we performed Raman measurements in the mid-IR (1000–3550 cm−1) frequency range. The results are presented in Section 3 of the ESI.† We analyzed these spectra with a variant of the multivariate curve resolution – alternating least squares (MCR-ALS) technique pioneered by Ben Amotz and co-workers.48 The detailed analysis is given in the ESI.† The Raman spectra analysis is complementary to our approach.
Based on the results of the Raman spectra we deduce a decrease of the partial bulk water contribution and an increase of the bulk glycerol component. An additional third partial component has a maximum at a mole fraction Xgly = 0.2, which can be compared to the expected maximum of the wrap (at Xgly = 0.15) or the bound water (Xgly = 0.3), suggesting that this is reporting on wrap and bound water populations.
As detailed in the ESI,† these results from the MCR analysis of the Raman spectra are overall consistent with the simulated Xgly dependence for the bulk, wrap and bound water populations as well as with our results on the THz range.
Further, we leverage MD simulations to probe shifts in the underlying structure of hydration water around glycerol with increased glycerol concentration. It is well-known that changes in water tetrahedrality impact the solvation thermodynamics in aqueous solutions.47,49–54 The most ubiquitous measure of tetrahedral order in aqueous systems is the tetrahedral order parameter, q, of Debenedetti and Errington.55 However, the interpretation of q is difficult for water in crowded mixtures, under confinement, and at surfaces because the calculation requires all four nearest neighbors of a central water molecule which may not be in the first hydration shell of the central water. Instead, we compute the three-body angle distribution50,53,54 by cataloguing angles between each water molecule and its two nearest neighbors (excluding neighbors further than 3.4 Å from the central water molecule). In principle, this distribution can be used to compute point metrics like q, but ostensibly contains significantly more information about fluctuations in orientational ordering. To date, a number of works56–59 have demonstrated the utility of three-body angle distributions as a descriptor shifts in solvation thermodynamics and dynamics in aqueous environments.
To better visualize the changes in the distributions, we examine the differential three-body angle distribution Pi(Xgly) − Ppure where, Pi(Xgly) is the distribution from population i at Xgly and Ppure is the reference distribution from pure water at 18 °C. We also provide the raw three-body angle distributions, Pi(Xgly), in ESI Fig. S7.† In aqueous environments we typically consider the tradeoff between the amplitudes of two characteristic regions of Pi(Xgly) − Ppure corresponding to icosahedral (simple) fluid and tetrahedral fluid behavior at θ = 64° and θ = 109.5°, respectively. Additionally, there is a low-probability region centered at θ ∼ 50° corresponding to the relative population of over-coordinated water environments.46 As shown in Fig. 4a and b, bound and wrap water populations exhibit distinct behavior for the entire range of compositions from Xgly = 0 to 0.5. For small Xgly, the bound waters display more icosahedral character compared to pure water, suggesting that glycerol–water HBs disrupt the water–water HB-network for bound waters. On the other hand, the wrap waters show enhanced tetrahedrality and decreased icosahedrality compared to pure water for the entire concentration range. This enhancement of tetrahedrality near the hydrophobic CHx groups is consistent with previous theoretical,60 computational,61,62 and experimental63 findings, showing that the presence of small hydrophobic solutes (<1 nm in radius) enhances the structure of the surrounding hydration waters.
We directly quantify changes in tetrahedrality by integrating over the tetrahedral region (100° < θ < 120°) of the raw three-body angle distributions to estimate the population of tetrahedrally-coordinated waters ptet for bound, wrap, and the system-averaged (all water molecules in the simulation box). In Fig. 4b, we demonstrate that the system-average ptet increases systematically by ∼10% from that of pure water upon increasing Xgly from 0.01 to 0.5. Increasing by 19%, the wrap water ptet exceeds both the system average and pure water for the entire range of Xgly. On the other hand, ptet for bound waters is 7% smaller than that of the system-average while increasing systematically by 15% increasing from Xgly = 0.01 to 0.50. Further, the bound water ptet eventually exceeds that of pure OPC water. These observations are consistent with the qualitative trends displayed in Fig. 4a.
These structural changes as revealed by MD simulations and spectroscopic fingerprints are expected to directly influence the thermodynamic properties of glycerol–water mixtures. Here, we are able to quantify these correlations directly from measured spectroscopic observables by means of THz-calorimetry (eqn (1)–(5)). In Fig. 5a, we plot the spectroscopically measured slope (for all the mixtures in the full Xgly range), i.e., the fingerprint of bound water molecules, against mixing enthalpy (ΔHmixing) data from the literature.19 Remarkably, the figure shows a nearly linear relationship, i.e., the larger the slope, the more negative (i.e., favorable) ΔHmixing is. In Fig. S6 of the ESI† we confirm the correlation by plotting several available calorimetry literature data of ΔHmixing (ref. 19, 64 and 65) as a function of the deduced slope.
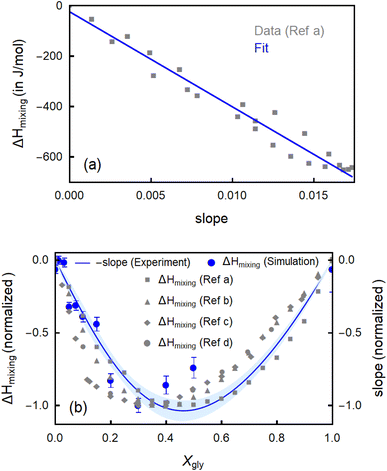 |
| Fig. 5 (a) Linear correlation between ΔHmixing (from Ref a,19 as an example) and the slope in the libration range of the εhydration spectra (Δεhydration/Δν), associated to the bound water population. The blue line is a linear fit. (b) The trend of the experimentally determined slope (Δεhydration/Δν) as a function of glycerol content is compared with that of mixing enthalpy values (ΔHmixing). ΔHmixing values are taken from four literature data sets: Ref a; calculated,19 Ref b; extrapolated,19 Ref c,65 Ref d,65 as well as from the present MD simulations. All data sets are scaled (from 0 to −1) for better comparison. | |
In the assumption that changes in the bound water contribution dominates the changes in mixing enthalpy, the ratio between ΔHmixing and the slope can be fitted by means of eqn (5). The absence of a detectable wrap spectroscopic fingerprint in the full miscibility range corroborates this assumption. We deduce a scaling factor of (Δ
bound = −35.9 ± 2.4 kJ) for the correlation factor between ΔHmixing (as averaged from all literature data from Fig. 5a and S6†) and the slope, which is as expected <0. To better investigate the correspondence between slope and ΔHmixing, in Fig. 5b, minus the slope (we multiply the slope by −1 because Δ
bound < 0) is displayed as a function of Xgly and compared with several ΔHmixing experimental datasets19,64,65 as well as the theoretical mixing enthalpy values from the present MD simulations. The scatter of the experimental ΔHmixing data sets gives an idea of the related confidence interval. Within this interval, we observe a very good agreement between simulated and measured ΔHmixing values. Their trend with Xgly can be quantitatively predicted from the trend of the THz slope, i.e., from the bound water population H-bonded to glycerol molecules. This signifies that the changes in mixing enthalpy with Xgly can be quantitatively explained by considering the enthalpic term due to the variations in the bound water population, only. More specifically, the decrement of ΔHmixing with Xgly (for Xgly < 0.4) is ascribed to an increase in the number of bound water molecules that enthalpically stabilize the mixture, while the further increase of ΔHmixing (for Xgly > 0.4) is caused by the progressive decrease in the number of bound water molecules. This result has two important implications. First, bound water molecules enthalpically stabilize glycerol–water mixtures by the HB they form with glycerol –OH groups. Second, and most important, the global trends in mixing enthalpy of heterogeneous glycerol–water binary mixtures can be understood by only considering the changes in one local structural hydration motif, the bound water population, without invoking contributions from more complex collective structural transformations occurring in the mixtures. This allows us to establish a well-defined structural and spectroscopic marker to track the changes in the thermodynamic properties of ubiquitously used glycerol–water solvents.
Implications of our findings for cryopreservation
Cryopreservation traditionally requires the addition of organic solvents, glycerol being a gold-standard, to mitigate the damage caused by ice formation and growth, which would otherwise lead to cell death.66 From the spectroscopic and simulations results of the present work, we can identify a glycerol concentration window with optimal structural and thermodynamic conditions to fulfill such cryoprotective role. This corresponds to a range of Xgly from 0.15 to 0.4. In this range, glycerol–water direct H-bonding and mixing enthalpy are maximized due to bound water being the dominant population (reaching the maximum at Xgly = 0.4, Fig. 5), while the bulk-like water population is close to zero (Fig. 3c). The formation of such a glycerol–water network was proposed in a previous study to promote protein stabilization via preferential solvation.4 In contrast, at Xgly < 0.15, bulk water and wrap water (which has a bulk-like character) are predominant, which is expected to lead to unwanted ice crystallization and growth upon cooling, as confirmed by previous experiments.4 This offers a new light to explain the empirical observations that Xgly = 0.15 is sufficient to achieve cryoprotection of many macromolecules.67 We propose that this is due to Xgly = 0.15 being the lowest glycerol concentration at which bound water is the dominant water population, while the “bulk-like” water population drops to almost zero. For Xgly > 0.4, glycerol–water HBs are progressively replaced by glycerol–glycerol direct bonding as the dominant interactions, as marked spectroscopically by the decrease of the bound-water fingerprint, and thermodynamically by the decrease of the mixing enthalpy. This causes a progressive transition toward a glycerol “bulk-like” state. In such conditions, the cytotoxic effects of glycerol will manifest, possibly hindering the viability of too concentrated mixtures for specific applications.66 Following the procedure introduced here, THz spectroscopy can be now used to systematically study the partial contributions of local water populations to the thermodynamics of binary mixtures and optimize their composition to fulfill a specific role (cryoprotection in our case).
Conclusion
In summary the addition of glycerol to aqueous solutions goes beyond its well-known impact on water's macroscopic viscosity. It alters the local molecular structures of hydration water and thereby dictates the mixing thermodynamics. The basic understanding provided here on such structure–thermodynamics relations offers a basis for a rational optimization of glycerol–water mixtures solvation properties by tailoring the mixture composition. Depending on the application, a goldilocks regime may be the minimum glycerol concentration in which all bulk water is transformed into hydration water populations, while minimizing destabilizing effects of glycerol on the protein, or a much higher glycerol concentration in which water–water HB interactions are minimized, and rather replaced by water–glycerol interactions. Our study also highlights the importance of explicit inclusion of glycerol in simulation studies of proteins for the purpose of comparing computed conformational dynamics and interactions to experimental studies that may require glycerol as solution constituents, e.g., in the study of double electron electron resonance (DEER).68 By combining THz spectroscopy with ab initio and classical MD simulations and mixing enthalpy estimations, we characterized, at the molecular level, how the structural transformations occurring in glycerol–water mixtures dictate their thermodynamic properties. Based on a spectroscopic observable, we were able to quantify the bound water population, i.e. we can directly monitor of changes in the hydrophilic solvation mechanisms as a function of glycerol concentration. This allows us to unravel the molecular mechanism for the changes in solvation enthalpy based on local solvation structures in the entire miscibility range. We propose that the mixing enthalpy between water and glycerol – a macroscopic property – is dominated by local contributions from the (hydrophilic) hydration water populations directly bound to glycerol. For glycerol, the bound water and not the wrap water (hydrophobic hydration) population dictates mixing enthalpy since glycerol fits nicely into the water network and vice versa. By contrast, for primary alcohols, the cavity formation around the hydrophobic chains, as probed by the cavity wrap water population dominates the entropy, as is typical for hydrophobic molecules. The general picture emerging by combining these results is that the balance between local hydrophobic/wrap and hydrophilic/bound contributions dictates global mixing thermodynamics. The balance depends crucially on the size of the molecules that are mixed with water as well as on the number and distributions on polar groups.
Our results offer exciting opportunities to tune the enthalpy and entropy of polyol–water mixtures – ubiquitously used co-solvents in biological and technological processes – by rationally modulating the nature of the polyol. For example, from the present results we can anticipate that the balance between the released water that is lost by the cell during freezing and the bound water population H-bonded to CPA (glycerol) and biomolecules – a key parameter for cryoprotection – can be tailored by experimentally exploiting the bound water THz fingerprint and making direct connection to mixing enthalpy. Specifically, the mixing ratio and type of polyol can be tuned to find conditions in which the bound water THz fingerprint is maximized in the spectrum, and hence maximize the number of bound waters and minimize that of released free water during freezing that, presumably, will more readily crystalize. Moreover, we expect that increasing the size of the polyol with respect to glycerol will be an efficient strategy to manipulate mixing enthalpy, by shifting the balance between bound and wrap hydration populations toward the latter, due to an increased volume exclusion effect. Such a shift in the bound/wrap balance could be used for instance to promote the solubility of more hydrophobic biomolecules (i.e., containing large hydrophobic surface patches exposed to the solvent), for which one important unfavorable thermodynamic contribution to solvation is the enthalpic penalty required to break strong solvent–solvent HBs. Based on the present results, mixture compositions where such penalty is minimized could be efficiently designed with a high-throughput THz screening, by “simply” finding the mixture composition for which the THz/FIR fingerprint of bound waters is minimized. The proposed Ansatz is general and can be transferred even to complex mixtures, since the spectroscopic fingerprints of the structural motifs around hydrophobic and hydrophilic hydrated moieties are well resolved in the THz/FIR range between 50 and 600 cm−1, and less obscured by intramolecular modes (which dominate in the infrared). Therefore, this study provides a path to quantitatively interpret macroscopic thermodynamic properties at the molecular level, in terms of local hydration motifs as quantified from experimentally measured observables, only.
Methods
Experimental section
Glycerol was purchased from Sigma-Aldrich and was used without further purification. All the aqueous binary mixtures were prepared using de-ionized ultrapure water. The temperature-controlled densities of all the mixtures were measured by Anton-Paar DMA 4500M density meter to calculate the concentrations of water and glycerol in the solutions (cwater,solution and cglycerol,solution). Far infrared (FIR/THz) spectra of glycerol aqueous solutions with various mole fractions were recorded in the frequency range from 30 cm−1 to 600 cm−1 (0.9–18 THz) at three different temperatures (5 °C, 20 °C and 40 °C) using Bruker (USA) Vertex 80v Fourier transform infrared (FTIR) spectrometer. A mercury lamp was used as the light source of the broadband THz radiation and an Infrared Laboratories liquid-helium-cooled silicon bolometer (HDL-5, Infrared Laboratories, USA) was employed as an external detector. A Mylar-multilayer beam splitter was utilized to generate the interferogram. All the FIR/THz experiments were measured using a temperature-controlled (T ±0.2 K) liquid transmission cell with diamond windows (0.5 mm thick) and a Kapton spacer with a nominal thickness of 26 ± 0.2 μm. The interference pattern of the empty cell was used to determine the exact sample thickness (d) and was used for further data analysis. During the measurements, the sample compartment was constantly purged with dry nitrogen to minimize the absorption arising from the residual water vapor. Further details about the spectrometer can be found elsewhere.42,69,70 Each spectrum was measured with a resolution of 2 cm−1. Each spectrum presented here is an average of 64 individual measurements. All the data were validated with repeated experiments.
Experimental data analysis protocol
The frequency-dependent absorption coefficient α(ν) of glycerol–water solutions are determined using the Lambert–Beer law, |  | (6) |
where d is the thickness of the sample, Isolution and Iwater are the transmitted intensities through the samples and pure water, respectively. Water is used as a reference to minimize “etalon effects”, i.e., standing waves. From the absorption coefficient we calculate the average molar extinction coefficients, | 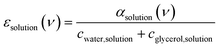 | (7) |
The molar effective extinction is deduced by subtracting the partial contributions from bulk water and bulk glycerol, respectively.
| εhydration(ν) = εsolution(ν) − Xglyεglycerol(ν) − (1 − Xgly)εwater(ν) | (8) |
where
εglycerol and
εwater are the molar extinction coefficient of pure glycerol and pure water, respectively.
εhydration(
ν) bears information solely about the inter-molecular interactions that are changed due to the mixing of water and glycerol (
i.e., water–water, water–glycerol, glycerol–glycerol interactions in the solvation shells). The glycerol content in the mixtures is quantified in terms of mole fraction:
|  | (9) |
where
ngly and
nw are the number of moles of glycerol and water, respectively, in the solutions.
MD simulation details
We model glycerol–water mixtures using Bliek–Chelli parameters for glycerol33,71 and the OPC 4-site water model.72 This combination of models reliably reproduces the equilibrium thermophysical properties of glycerol–water under standard temperature and pressure conditions.32,71,73 coulombic interactions are modelled using the particle-mesh Ewald summation scheme (PME).74 We simulate glycerol–water mixtures with Xgly = 0, 0.012, 0.033, 0.05, 0.075, 0.10, 0.15, 0.20, 0.30, 0.40, 0.50, and 1.0 at 18 °C and 1 bar using the GPU-optimized OpenMM package.75 All simulations are conducted in the NPT ensemble, with temperature and pressure held constant using a Langevin thermostat75 and Monte Carlo barostat,75 respectively. We generate initial configurations containing 729 glycerol molecules and vary the numbers of water molecules using Packmol software.76 We first minimize the energy of the initial configurations and equilibrate in the NPT ensemble for times ranging from 100 to 500 ns depending on the glycerol concentration. We conduct production runs for 100 ns with system coordinates saved every 100 ps. Following the simulations, we analyze the production run trajectories using the pytraj Python library77 and in-house python code. For each simulation-computed quantity, we estimate the standard error of the mean using block averaging.
We define HBs via the widely used geometric criteria of Luzar and Chandler78 namely, O–H distance, dO–H, and ∠O–OH angle, θ∠O–OH, criteria of dO–H ≤ 3.5 Å and θ∠O–OH ≥ 120° degrees, respectively. Tracking the waters participating in water-glycerol H-bonds, we directly quantify the mole fraction of bound waters (Xbound). We also calculate the mole fraction of wrap waters (Xwrap) by defining them as waters lying within a cut-off distance, rc, of the heavy atoms of glycerol molecules. Here, rc is set by the second hydration shell of glycerol molecules (∼4.2 Å, as defined by the second minima of the 2D radial distribution function between glycerol heavy atoms and water oxygens), but not H-bonded to the glycerol molecule. Non-shell waters are simply all waters residing more than 4.2 Å from glycerol heavy atoms. We compute concentration of non-shell waters on a molar basis via Xnon-shell = (1 − Xbound − Xwrap − Xgly). Using the simulation-averaged molar energy 〈E〉 and molar volume 〈V〉 for each simulated glycerol–water mixture, we also estimate the molar energy of mixing and molar volume of mixing,
| ΔEmixing = 〈E〉 − Xgly〈Eglycerol〉 − (1 − Xgly)〈Ewater〉 | (10) |
| ΔVmixing = 〈V〉 − Xgly〈Vglycerol〉 − (1 − Xgly)〈Vwater〉 | (11) |
where, 〈
Ywater〉 and 〈
Yglycerol〉 correspond to the average molar value of
Y for pure water and glycerol, respectively. The molar enthalpy of mixing Δ
Hmixing hence stems from the relation,
| ΔHmixing = ΔEmixing + PΔVmixing | (12) |
where
P is system pressure (1 bar).
Data availability
The data that support the findings of this study are available from the corresponding author upon reasonable request.
Author contributions
M. H., M. S. S. and S. H. designed research. D. D. M. conducted the THz measurements, D. D. M., S. P., G. S. and M. H. analysed the experimental data and derived thermodynamic quantities from spectra. The molecular dynamics simulations were performed and analysed by D. R. B. and M. S. S. All authors discussed the results and wrote the manuscript.
Conflicts of interest
There are no conflicts to declare.
Acknowledgements
M. H. acknowledge financial support by European Research Council (ERC) Advanced Grant 695437 THz-Calorimetry. This study is funded by the Deutsche Forschungsgemeinschaft (DFG, German Research Foundation) under Germany's Excellence Strategy EXC2033 390677874 RESOLV. We thank the Mercator Research Center Ruhr (MERCUR) for funding. M. S. S. and D. R. B. acknowledge support from the Center for Materials for Water and Energy Systems, an Energy Frontier Research Center funded by the US Department of Energy, Office of Science, Basic Energy Sciences under Award DE-SC0019272. Use was made of computational facilities purchased with funds from the National Science Foundation (OAC-1925717) and administered by the Center for Scientific Computing (CSC). The CSC is supported by the California NanoSystems Institute and the Materials Research Science and Engineering Center (MRSEC; NSF DMR 1720256) at UC Santa Barbara.
References
- L. Weng, S. L. Stott and M. Toner, Exploring Dynamics and Structure of Biomolecules, Cryoprotectants, and Water Using Molecular Dynamics Simulations: Implications for Biostabilization and Biopreservation, Annu. Rev. Biomed. Eng., 2019, 21, 1–31 CrossRef CAS PubMed.
- J. J. Towey, A. K. Soper and L. Dougan, Molecular Insight into the Hydrogen Bonding and Micro-Segregation of a Cryoprotectant Molecule, J. Phys. Chem. B, 2012, 116, 13898–13904 CrossRef CAS PubMed.
- J. J. Towey, A. K. Soper and L. Dougan, What happens to the structure of water in cryoprotectant solutions?, Faraday Discuss., 2013, 167, 159–176 RSC.
- J. L. Dashnau, N. V. Nucci, K. A. Sharp and J. M. Vanderkooi, Hydrogen Bonding and the Cryoprotective Properties of Glycerol/Water Mixtures, J. Phys. Chem. B, 2006, 110, 13670–13677 CrossRef CAS PubMed.
- K. Gekko and S. N. Timasheff, Mechanism of protein stabilization by glycerol: preferential hydration in glycerol-water mixtures, Biochemistry, 1981, 20, 4667–4676 CrossRef CAS PubMed.
- N. Chéron, M. Naepels, E. Pluhařová and D. Laage, Protein Preferential Solvation in Water:Glycerol Mixtures, J. Phys. Chem. B, 2020, 124, 1424–1437 CrossRef PubMed.
- M. Hirai, S. Ajito, M. Sugiyama, H. Iwase, S.-i. Takata, N. Shimizu, N. Igarashi, A. Martel and L. Porcar, Direct Evidence for the Effect of Glycerol on Protein Hydration and Thermal Structural Transition, Biophys. J., 2018, 115, 313–327 CrossRef CAS PubMed.
- L. Weng, S. L. Stott and M. Toner, Exploring Dynamics and Structure of Biomolecules, Cryoprotectants, and Water Using Molecular Dynamics Simulations: Implications for Biostabilization and Biopreservation, Annu. Rev. Biomed. Eng., 2019, 21, 1–31 CrossRef CAS PubMed.
- L. Weng, C. Chen, J. Zuo and W. Li, Molecular Dynamics Study of Effects of Temperature and Concentration on Hydrogen-Bond Abilities of Ethylene Glycol and Glycerol: Implications for Cryopreservation, J. Phys. Chem. A, 2011, 115, 4729–4737 CrossRef CAS PubMed.
- C. Weeraratna, C. Amarasinghe, W. Lu and M. Ahmed, A Direct Probe of the Hydrogen Bond Network in Aqueous Glycerol Aerosols, J. Phys. Chem. Lett., 2021, 12, 5503–5511 CrossRef CAS PubMed.
- A. Charkhesht, D. Lou, B. Sindle, C. Wen, S. Cheng and N. Q. Vinh, Insights into Hydration Dynamics and Cooperative Interactions in Glycerol–Water Mixtures by Terahertz Dielectric Spectroscopy, J. Phys. Chem. B, 2019, 123, 8791–8799 CrossRef CAS PubMed.
- C. Osei-Bempong, A. E. Ghareeb, M. Lako, F. C. Figueiredo and W. J. Armitage, Defining the optimal cryoprotectant and concentration for cryopreservation of limbal stem cells, Cryobiology, 2018, 84, 98–102 CrossRef CAS PubMed.
- L. Zaniboni, M. Madeddu, F. Mosca, A. Abdel Sayed, S. P. Marelli, M. Di Iorio, N. Iaffaldano and S. Cerolini, Concentration dependent effect of dimethylacetamide and N-methylacetamide on the quality and fertility of cryopreserved chicken semen, Cryobiology, 2022, 106, 66–72 CrossRef CAS PubMed.
- D. Whaley, K. Damyar, R. P. Witek, A. Mendoza, M. Alexander and J. R. Lakey, Cryopreservation: An Overview of Principles and Cell-Specific Considerations, Cell Transplant., 2021, 30, 963689721999617 Search PubMed.
- J. J. Towey, A. K. Soper and L. Dougan, What happens to the structure of water in cryoprotectant solutions?, Faraday Discuss., 2013, 167, 159–176 RSC.
- M. Toner, E. G. Cravalho and M. Karel, Thermodynamics and kinetics of intracellular ice formation during freezing of biological cells, J. Appl. Phys., 1990, 67, 1582–1593 CrossRef.
- J. O. M. Karlsson, E. G. Cravalho and M. Toner, A model of diffusion-limited ice growth inside biological cells during freezing, J. Appl. Phys., 1994, 75, 4442–4455 CrossRef.
- G. I. Egorov, D. M. Makarov and A. M. Kolker, Volume properties of liquid mixture
of water+glycerol over the temperature range from 278.15 to 348.15 K at atmospheric pressure, Thermochim. Acta, 2013, 570, 16–26 CrossRef CAS.
- Y. Marcus, Some thermodynamic and structural aspects of mixtures of glycerol with water, Phys. Chem. Chem. Phys., 2000, 2, 4891–4896 RSC.
- Y. Hayashi, A. Puzenko and Y. Feldman, Slow and fast dynamics in glycerol–water mixtures, J. Non-Cryst. Solids, 2006, 352, 4696–4703 CrossRef CAS.
- E. C. H. To, J. V. Davies, M. Tucker, P. Westh, C. Trandum, K. S. H. Suh and Y. Koga, Excess Chemical Potentials, Excess Partial Molar Enthalpies, Entropies, Volumes, and Isobaric Thermal Expansivities of Aqueous Glycerol at 25°C, J. Solution Chem., 1999, 28, 1137–1157 CrossRef CAS.
- R. Grasso, F. Musumeci, M. Gulino and A. Scordino, Exploring the behaviour of water in glycerol solutions by using delayed luminescence, PLoS One, 2018, 13, e0191861 CrossRef PubMed.
- F. Mallamace, D. Mallamace, S.-H. Chen, P. Lanzafame and G. Papanikolaou, Hydrophilic and Hydrophobic Effects on the Structure and Themodynamic Properties of Confined Water: Water in Solutions, Int. J. Mol. Sci., 2021, 22, 7547 CrossRef CAS PubMed.
- C. Weeraratna, C. Amarasinghe, W. Lu and M. Ahmed, A Direct Probe of the Hydrogen Bond Network in Aqueous Glycerol Aerosols, J. Phys. Chem. Lett., 2021, 12, 5503–5511 CrossRef CAS PubMed.
- A. Charkhesht, D. Lou, B. Sindle, C. Wen, S. Cheng and N. Q. Vinh, Insights into Hydration Dynamics and Cooperative Interactions in Glycerol–Water Mixtures by Terahertz Dielectric Spectroscopy, J. Phys. Chem. B, 2019, 123, 8791–8799 CrossRef CAS PubMed.
- R. Behrends, K. Fuchs, U. Kaatze, Y. Hayashi and Y. Feldman, Dielectric properties of glycerol/water mixtures at temperatures between 10 and 50°C, J. Chem. Phys., 2006, 124, 144512 CrossRef CAS PubMed.
- Y. Hayashi, A. Puzenko, I. Balin, Y. E. Ryabov and Y. Feldman, Relaxation Dynamics in Glycerol−Water Mixtures. 2. Mesoscopic Feature in Water Rich Mixtures, J. Phys. Chem. B, 2005, 109, 9174–9177 CrossRef CAS PubMed.
- A. Puzenko, Y. Hayashi, Y. E. Ryabov, I. Balin, Y. Feldman, U. Kaatze and R. Behrends, Relaxation Dynamics in Glycerol–Water Mixtures: I. Glycerol-Rich Mixtures, J. Phys. Chem. B, 2005, 109, 6031–6035 CrossRef CAS PubMed.
- A. Leavesley, C. B. Wilson, M. Sherwin and S. Han, Effect of water/glycerol polymorphism on dynamic nuclear polarization, Phys. Chem. Chem. Phys., 2018, 20, 9897–9903 RSC.
- Y. Nagasaki, T. Ishii, Y. Sunaga, Y. Watanabe, H. Otsuka and K. Kataoka, Novel Molecular Recognition via Fluorescent Resonance Energy Transfer Using a Biotin-PEG/Polyamine Stabilized CdS Quantum Dot, Langmuir, 2004, 20, 6396–6400 CrossRef CAS PubMed.
- B. Zelent, N. V. Nucci and J. M. Vanderkooi, Liquid and Ice Water and Glycerol/Water Glasses Compared by Infrared Spectroscopy from 295 to 12 K, J. Phys. Chem. A, 2004, 108, 11141–11150 CrossRef CAS.
- R. Chelli, P. Procacci, G. Cardini and S. Califano, Glycerol condensed phases part II. A molecular dynamics study of the conformational structure and hydrogen bonding, Phys. Chem. Chem. Phys., 1999, 1, 879–885 RSC.
- R. Chelli, P. Procacci, G. Cardini, R. G. Della Valle and S. Califano, Glycerol condensed phases part I. A molecular dynamics study, Phys. Chem. Chem. Phys., 1999, 1, 871–877 RSC.
- S. Gómez, N. Rojas-Valencia, S. A. Gómez, C. Cappelli, G. Merino and A. Restrepo, A molecular twist on hydrophobicity, Chem. Sci., 2021, 12, 9233–9245 RSC.
- V. Conti Nibali, S. Pezzotti, F. Sebastiani, D. Galimberti, G. Schwaab, M. Heyden, M.-P. Gaigeot and M. Havenith, Wrapping up hydrophobic hydration: locality matters, J. Phys. Chem. Lett., 2020, 11, 4809–4816 CrossRef CAS PubMed.
- T. Morawietz, O. Marsalek, S. R. Pattenaude, L. M. Streacker, D. Ben-Amotz and T. E. Markland, The Interplay of Structure and Dynamics in the Raman Spectrum of Liquid Water over the Full Frequency and Temperature Range, J. Phys. Chem. Lett., 2018, 9, 851–857 CrossRef CAS PubMed.
- S. Pezzotti, F. Sebastiani, E. P. van Dam, S. Ramos, V. Conti Nibali, G. Schwaab and M. Havenith, Spectroscopic Fingerprints of Cavity Formation and Solute Insertion as a Measure of Hydration Entropic Loss and Enthalpic Gain, Angew. Chem., Int. Ed., 2022, 61, e202203893 CrossRef CAS PubMed.
- D. Das Mahanta, A. Patra, N. Samanta, T. Q. Luong, B. Mukherjee and R. K. Mitra, Non-monotonic dynamics of water in its binary mixture with 1,2-dimethoxy ethane: a combined THz spectroscopic and MD simulation study, J. Chem. Phys., 2016, 145, 164501 CrossRef PubMed.
- S. Chakraborty, P. Pyne, R. Kumar Mitra and D. Das Mahanta, A subtle interplay between hydrophilic and hydrophobic hydration governs butanol (de)mixing in water, Chem. Phys. Lett., 2022, 807, 140080 CrossRef CAS.
- E. M. Adams, S. Pezzotti, J. Ahlers, M. Rüttermann, M. Levin, A. Goldenzweig, Y. Peleg, S. J. Fleishman, I. Sagi and M. Havenith, Local Mutations Can Serve as a Game Changer for Global Protein Solvent Interaction, JACS Au, 2021, 1, 1076–1085 CrossRef CAS PubMed.
- J. Ahlers, E. M. Adams, V. Bader, S. Pezzotti, K. F. Winklhofer, J. Tatzelt and M. Havenith, The key role of solvent in condensation: mapping water in liquid-liquid phase-separated FUS, Biophys. J., 2021, 120, 1266–1275 CrossRef CAS PubMed.
- C. Y. Ma, S. Pezzotti, G. Schwaab, M. Gebala, D. Herschlag and M. Havenith, Cation enrichment in the ion atmosphere is promoted by local hydration of DNA, Phys. Chem. Chem. Phys., 2021, 23, 23203–23213 RSC.
- S. Funke, F. Sebastiani, G. Schwaab and M. Havenith, Spectroscopic fingerprints in the low frequency spectrum of ice (Ih), clathrate hydrates, supercooled water, and hydrophobic hydration reveal similarities in the hydrogen bond network motifs, J. Chem. Phys., 2019, 150, 224505 CrossRef PubMed.
- V. Conti Nibali, S. Pezzotti, F. Sebastiani, D. R. Galimberti, G. Schwaab, M. Heyden, M. P. Gaigeot and M. Havenith, Wrapping Up Hydrophobic Hydration: Locality Matters, J. Phys. Chem. Lett., 2020, 11, 4809–4816 CrossRef CAS PubMed.
- H. Vondracek, S. Alfarano, C. Hoberg, I. Kolling, F. Novelli, F. Sebastiani, J.-B. Brubach, P. Roy, G. Schwaab and M. Havenith, Urea's match in the hydrogen-bond network? A high pressure THz study, Biophys. Chem., 2019, 254, 106240 CrossRef CAS PubMed.
- F. Böhm, G. Schwaab and M. Havenith, Mapping Hydration Water around Alcohol Chains by THz Calorimetry, Angew. Chem., Int. Ed., 2017, 56, 9981–9985 CrossRef PubMed.
- T. Starciuc, Y. Guinet, A. Hedoux and E. Shalaev, Water content thresholds in glycerol/water system: low- and high-wavenumber Raman spectroscopy study, J. Mol. Liq., 2021, 321, 114678 CrossRef CAS.
- P. Perera, M. Wyche, Y. Loethen and D. Ben-Amotz, Solute-Induced Perturbations of Solvent-Shell Molecules Observed Using Multivariate Raman Curve Resolution, J. Am. Chem. Soc., 2008, 130, 4576–4577 CrossRef CAS PubMed.
- N. Galamba, Water's Structure around Hydrophobic Solutes and the Iceberg Model, J. Phys. Chem. B, 2013, 117, 2153–2159 CrossRef CAS PubMed.
- A. Chaimovich and M. S. Shell, Tetrahedrality and structural order for hydrophobic interactions in a coarse-grained water model, Phys. Rev. E: Stat., Nonlinear, Soft Matter Phys., 2014, 89, 022140 CrossRef PubMed.
- J. N. Dahanayake and K. R. Mitchell-Koch, Entropy connects water structure and dynamics in protein hydration layer, Phys. Chem. Chem. Phys., 2018, 20, 14765–14777 RSC.
- X. Wu, W. Lu, L. M. Streacker, H. S. Ashbaugh and D. Ben-Amotz, Temperature-Dependent Hydrophobic Crossover Length Scale and Water Tetrahedral Order, J. Phys. Chem. Lett., 2018, 9, 1012–1017 CrossRef CAS PubMed.
- P. Stock, J. I. Monroe, T. Utzig, D. J. Smith, M. S. Shell and M. Valtiner, Unraveling Hydrophobic Interactions at the Molecular Scale Using Force Spectroscopy and Molecular Dynamics Simulations, ACS Nano, 2017, 11, 2586–2597 CrossRef CAS PubMed.
- J. I. Monroe and M. S. Shell, Decoding signatures of structure, bulk thermodynamics, and solvation in three-body angle distributions of rigid water models, J. Chem. Phys., 2019, 151, 094501 CrossRef PubMed.
- J. R. Errington and P. G. Debenedetti, Relationship between structural order and the anomalies of liquid water, Nature, 2001, 409, 318–321 CrossRef CAS PubMed.
- J. I. Monroe and M. S. Shell, Decoding signatures of structure, bulk thermodynamics, and solvation in three-body angle distributions of rigid water models, J. Chem. Phys., 2019, 151, 094501 CrossRef PubMed.
- S. Jiao, D. M. Rivera Mirabal, A. J. DeStefano, R. A. Segalman, S. Han and M. S. Shell, Sequence Modulates Polypeptoid Hydration Water Structure and Dynamics, Biomacromolecules, 2022, 23, 1745–1756 CrossRef CAS PubMed.
- J. Monroe, M. Barry, A. DeStefano, P. A. Gokturk, S. Jiao, D. Robinson-Brown, T. Webber, E. J. Crumlin, S. Han and M. S. Shell, Water Structure and Properties at Hydrophilic and Hydrophobic Surfaces, Annu. Rev. Chem. Biomol. Eng., 2020, 11, 523–557 CrossRef CAS PubMed.
- B. C. Dallin, A. S. Kelkar and R. C. Van Lehn, Structural features of interfacial water predict the hydrophobicity of chemically heterogeneous surfaces, Chem. Sci., 2023, 14, 1308–1319 RSC.
- K. Lum, D. Chandler and J. D. Weeks, Hydrophobicity at small and large length scales, J. Phys. Chem. B, 1999, 103, 4570–4577 CrossRef CAS.
- D. M. Huang and D. Chandler, The Hydrophobic Effect and the Influence of Solute–Solvent Attractions, J. Phys. Chem. B, 2002, 106, 2047–2053 CrossRef CAS.
- D. M. Huang, P. L. Geissler and D. Chandler, Scaling of Hydrophobic Solvation Free Energies, J. Phys. Chem. B, 2001, 105, 6704–6709 CrossRef CAS.
- W. Di, X. Gao, W. Huang, Y. Sun, H. Lei, Y. Liu, W. Li, Y. Li, X. Wang, M. Qin, Z. Zhu, Y. Cao and W. Wang, Direct Measurement of Length Scale Dependence of the Hydrophobic Free Energy of a Single Collapsed Polymer Nanosphere, Phys. Rev. Lett., 2019, 122, 047801 CrossRef PubMed.
- R. Zehioua, C. Coquelet, C.-B. Soo, D. Richon and A.-H. Meniai, Experimental and predicted excess molar enthalpies of some working pairs for absorption cycles, Thermochim. Acta, 2009, 495, 72–80 CrossRef CAS.
- D. V. Batov, A. M. Zaichikov, V. P. Slyusar and V. P. Korolev, Enthalpies of Mixing and State of Components in Aqueous-Organic Mixtures with Nets of Hydrogen Bonds, Russ. J. Gen. Chem., 2001, 71, 1208–1214 CrossRef CAS.
- M. Hasan, A. E. R. Fayter and M. I. Gibson, Ice Recrystallization Inhibiting Polymers Enable Glycerol-Free Cryopreservation of Microorganisms, Biomacromolecules, 2018, 19, 3371–3376 CrossRef CAS PubMed.
- E. F. Garman and R. L. Owen, Cryocooling and radiation damage in macromolecular crystallography, Acta Crystallogr., Sect. D: Biol. Crystallogr., 2006, 62, 32–47 CrossRef PubMed.
- E. R. Georgieva, A. S. Roy, V. M. Grigoryants, P. P. Borbat, K. A. Earle, C. P. Scholes and J. H. Freed, Effect of freezing conditions on distances and their distributions
derived from Double Electron Electron Resonance (DEER): a study of doubly-spin-labeled T4 lysozyme, J. Magn. Reson., 2012, 216, 69–77 CrossRef CAS PubMed.
- F. Böhm, G. Schwaab and M. Havenith, Mapping Hydration Water around Alcohol Chains by THz Calorimetry, Angew. Chem., Int. Ed., 2017, 56, 9981–9985 CrossRef PubMed.
- G. Schwaab, F. Sebastiani and M. Havenith, Ion Hydration and Ion Pairing as Probed by THz Spectroscopy, Angew. Chem., Int. Ed., 2019, 58, 3000–3013 CrossRef CAS PubMed.
- J. Blieck, F. Affouard, P. Bordat, A. Lerbret and M. Descamps, Molecular dynamics simulations of glycerol glass-forming liquid, Chem. Phys., 2005, 317, 253–257 CrossRef CAS.
- S. Izadi, R. Anandakrishnan and A. V. Onufriev, Building Water Models: A Different Approach, J. Phys. Chem. Lett., 2014, 5, 3863–3871 CrossRef CAS PubMed.
- D. A. Jahn, F. O. Akinkunmi and N. Giovambattista, Effects of Temperature on the Properties of Glycerol: A Computer Simulation Study of Five Different Force Fields, J. Phys. Chem. B, 2014, 118, 11284–11294 CrossRef CAS PubMed.
- T. Darden, D. York and L. Pedersen, Particle mesh Ewald: an N·log(N) method for Ewald sums in large systems, J. Chem. Phys., 1993, 98, 10089–10092 CrossRef CAS.
- P. Eastman and V. Pande, OpenMM: A Hardware-Independent Framework for Molecular Simulations, Comput. Sci. Eng., 2010, 12, 34–39 CAS.
- L. Martínez, R. Andrade, E. G. Birgin and J. M. Martínez, PACKMOL: a package for building initial configurations for molecular dynamics simulations, J. Comput. Chem., 2009, 30, 2157–2164 CrossRef PubMed.
- D. R. Roe and T. E. Cheatham, PTRAJ and CPPTRAJ: Software for Processing and Analysis of Molecular Dynamics Trajectory Data, J. Chem. Theory Comput., 2013, 9, 3084–3095 CrossRef CAS PubMed.
- A. Luzar and D. Chandler, Structure and hydrogen bond dynamics of water–dimethyl sulfoxide mixtures by computer simulations, J. Chem. Phys., 1993, 98, 8160–8173 CrossRef CAS.
|
This journal is © The Royal Society of Chemistry 2023 |
Click here to see how this site uses Cookies. View our privacy policy here.