DOI:
10.1039/D3SC00090G
(Edge Article)
Chem. Sci., 2023,
14, 2097-2106
An enzyme-powered microRNA discriminator for the subtype-specific diagnosis of breast cancer†
Received
6th January 2023
, Accepted 26th January 2023
First published on 31st January 2023
Abstract
Breast cancer, a disease with highly heterogeneous features, is the most common malignancy diagnosed in people worldwide. Early diagnosis of breast cancer is crucial for improving its cure rate, and accurate classification of the subtype-specific features is essential to precisely treat the disease. An enzyme-powered microRNA (miRNA, RNA = ribonucleic acid) discriminator was developed to selectively distinguish breast cancer cells from normal cells and further identify subtype-specific features. Specifically, miR-21 was used as a universal biomarker to discriminate between breast cancer cells and normal cells, and miR-210 was used to identify triple-negative subtype features. The experimental results demonstrated that the enzyme-powered miRNA discriminator displayed low limits of detection at fM levels for both miR-21 and miR-210. Moreover, the miRNA discriminator enabled the discrimination and quantitative determination of breast cancer cells derived from different subtypes based on their miR-21 levels, and the further identification of the triple-negative subtype in combination with the miR-210 levels. Therefore, it is hoped that this study will provide insight into subtype-specific miRNA profiling, which may have potential use in the clinical management of breast tumours based on their subtype characteristics.
Introduction
As a burden to healthcare systems around the globe, breast cancer is now the most frequent malignant tumour-related disease diagnosed worldwide, a condition that seriously threatens the lives of people around the globe.1–3 In recent decades, breast cancer mortality has significantly declined in line with advances made in therapeutics, but metastatic breast cancer remains a leading cause of cancer-related death.4,5 As the molecular levels alter in different breast tumours, the heterogeneity of the malignancy can be categorised into several subtypes based on the tumour expressions of the oestrogen receptor, progesterone receptor (PR), and the human epidermal growth factor receptor-2 (HER-2).6,7 According to biology-centred treatment concepts, accurate identification of the breast cancer subtype is necessary for precision management of breast tumours and is beneficial for predicting tumour behaviour.8 For example, triple-negative breast cancer (TNBC), in which these three biomarkers are not expressed, is highly aggressive; it has the worst prognosis and accounts for approximately one third of breast cancer-related deaths.9,10 Due to the lack of effective therapeutic targets, TNBC patients do not benefit from the currently available treatment options, and are more likely to develop lung or brain metastasis, even at an early stage of the disease.11–13 Therefore, breast cancer diagnosis is not exclusively related to the early discovery of breast tumours, the subtype-specific features of the cancer need to be identified, as well as the potential therapeutic targets for clinical treatment, which is especially important to improve the outcome in TNBC patients.
MicroRNAs (miRNAs, RNA = ribonucleic acid) are small non-coding RNA molecules that are involved in the regulation of gene expression through the interaction with the 3′ untranslated region (3′ UTR) of messenger RNA.14 Increasing evidence has revealed that dysregulated miRNA expression can be closely correlated with tumour development in many types of cancer. Therefore, the biological mechanism underpinning tumour initiation and progression makes miRNA a promising indicator for use in cancer diagnosis and prognosis, representing a potential therapeutic target and direction for the management of cancer.15–17 Several miRNAs have been discovered and identified as diagnostic biomarkers for breast cancer.18–22 For example, miR-21, which is commonly upregulated in solid tumours, functions as an oncogene in breast cancer and also contributes toward resistance to breast cancer therapies.18 Moreover, miR-210, which is highly expressed in hypoxic environments, promotes breast cancer proliferation, especially in the most aggressive TNBC subtype.21 In this view, miRNA profiling may not only provide predictive information for the early detection of breast cancer but also guide the management of subtype-specific cancers.
Due to its high programmability and controllable size, dynamic DNA assembly has received considerable attention and has exhibited distinct advantages in biological analysis.23–26 For example, in a toehold-mediated strand displacement reaction, a single-stranded overhanging region, that is, a toehold, mediates branch migration via a controllable strand invasion mechanism.27 Moreover, logic relationships can be established by the construction of particular computing-network architectures when DNA assembly reactions occur in an orderly, sequenced manner.28 On the basis of the dynamic DNA assembly, several nucleic acid-based computing-network architectures have been designed to evaluate multiple cell surface receptors and enabled the identification of a particular subpopulation of cancer cells.29–31 Recently, single miRNA biomarker (e.g. miR-21, miRNA-141, miRNA-155) has been used to manipulate entropy-driven DNA assembly and achieved signal amplification for low-abundant miRNA imaging in living cells.32–34 In the meanwhile, multiple analysis of endogenous miRNA biomarkers has also been realized and applied for the accurate discrimination of cell subtypes in cancers by making use of DNA-based nanodevices in a logic input–output signal correlation pattern.35–37 These methods permitted the automatic discrimination of non-tumorigenic, malignant, and metastatic cells, as well as the respective tumours, and facilitated the targeted cancer diagnosis and therapy. However, these methods were based on enzyme-free DNA assembly strategies, most of which required a sophisticated design of multiple DNA probes and exhibited an unremarkable limit of detection (e.g. pM level for miRNA biomarkers).
Alternatively, an enzyme-powered miRNA discriminator based on dynamic DNA assembly is herein reported. The miRNA discriminator incorporates two toehold-mediated strand displacement reactions, which are initiated sequentially with the miRNA biomarkers and fuelled by T7 exonuclease (Exo)-powered digestion. Taking the combination of miR-21 and miR-210 as an example, the miRNA discriminator is able to selectively identify breast cancer cells and also the TNBC subtype by revealing their miRNA signatures. Compared to the above-mentioned enzyme-free methods, the miRNA discriminator is simpler in design as it requires only one signal probe input and reveals an enhanced sensitivity at the fM level by virtue of enzyme-powered signal amplification. In the meanwhile, the miRNA discriminator is adaptable to the determination under a relatively steady condition in vitro, and can avoid the direct effect of intracellular environment in fluorescence imaging, such as pH and temperature fluctuation. Moreover, the design described in this study is based on an electrochemical technique, which has the unique advantages of convenient operation, high sensitivity, and low cost.24,26 Therefore, our miRNA discriminator may provide accurate diagnostic and therapeutic information for the in-clinic miRNA-targeted management of breast cancer.
Results and discussion
Principle of a T7 Exo-powered miRNA discriminator
Scheme 1 shows the design of the enzyme-powered miRNA discriminator employed in this study. As a proof-of-principle study, miR-21 was selected to identify breast cancer cells for the early detection of breast tumours, and miR-210 was selected to identify TNBC to reveal its subtype-specific features. An electroactive three-stranded signal probe, A/B/C, was prepared through the hybridisation of strands A, B, and C, in which strand A is labelled with electroactive methylene blue (MB) and ferrocene (Fc) molecules at both ends, separately. miR-21 binds to the toehold region within the signal probe, and initiates the first toehold-mediated strand displacement reaction, forming the assembly product A/C/miR-21. T7 Exo, which catalyses the stepwise hydrolysis of the mononucleotides from duplex DNA or a DNA
:
RNA hybrid in the 5′-to-3′ direction, is used to accelerate the displacement reaction and the release of end-labelled electroactive molecules.38 Once strand A hybridizes with miR-21 through a strand displacement reaction, strand A is partially segmented by T7 Exo, and releases the end-labelled MB molecules. T7 Exo digestion is terminated at the single-stranded region of strand A, and releases miR-21 to trigger a new round of strand displacement reactions. A large number of partially-digested signal probes, A1/C, are generated from the first T7 Exo-powered strand displacement reaction and expose the toehold regions to interact with miR-210, initiating the second strand displacement reaction. Strand A1 within the assembly product (A1/miR-210) is further digested by T7 Exo, and releases another end-labelled Fc molecule. Free MB and Fc molecules can be enriched at a cucurbit[7]uril (CB[7])-functionalised electrode through a host–guest interaction.39,40 The MB signal corresponding to miR-21 indicates the occurrence of breast cancer, and the Fc signal corresponding to miR-210 further identifies the TNBC subtype in breast cancer.
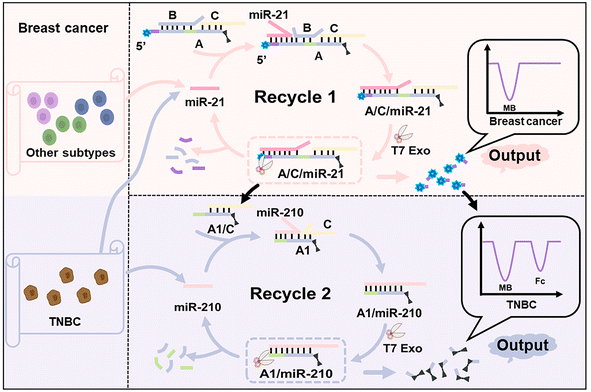 |
| Scheme 1 Schematic illustration of the enzyme-powered miRNA discriminator in the subtype-specific diagnosis of breast cancer. | |
Verification of the T7 Exo-powered miRNA discriminator
The formation of the three-stranded signal probe A/B/C was characterised by polyacrylamide electrophoresis gel analysis (Fig. S1†). The preparation of the three-stranded signal probe was also investigated using fluorescence spectrum after the assembly of strand A, carboxyfluorescein-labelled strand B (FAM-B), and black hole quencher-1-labelled strand C (BHQ1-C) in different ratios, and an optimised hybridisation ratio of 1
:
1
:
1 was identified through the observation of quenched fluorescence of FAM (Fig. S2†). Fig. 1A shows the sequential strand displacement reactions with miRNA inputs from miR-21 and miR-210. miR-21 binds to the toehold region of strand A within A/B/C, and initiates the first strand displacement, resulting in the release of strand B. In addition, the assembly product, A/C/miR-21, exposes the toehold region to trigger the second strand displacement reaction upon the binding of miR-210, resulting in the formation of the product A/miR-21/miR-210. Fig. 1B shows the fluorescence spectra of FAM obtained with different miRNA inputs, in which the fluorescent signal probe, A/FAM-B/BHQ1-C, was used. The fluorescence of FAM was quenched by BHQ1 without any inputs (curve a) or with miR-210 (curve c), but intense FAM fluorescence was observed with miRNA-21 (curve b) or with both miR-21 and miR-210 (curve d). The results revealed that the first strand displacement reaction was initiated when miR-21 was present regardless of whether miR-210 was present, which resulted in the release of the FAM-B strand and the recovery of the FAM fluorescence. Fig. 1C further shows the fluorescence spectra of carboxy-4′,5′-dichloro-2′,7′ dimethoxyfluorescein (JOE) obtained with different miRNA inputs, in which a fluorescent signal probe, JOE-A/B/BHQ1-C, was used. Quenched JOE fluorescence was observed without any inputs (curve a) or with miR-21 (curve b) or miR-210 (curve c), but intense JOE fluorescence was observed when both miR-21 and miR-210 were added (curve d). These results are in line with the design: the second strand displacement reaction only takes place in the presence of miR-210 and after miR-21-initiated strand displacement, which recovers the JOE fluorescence for the separation of the quencher-labelled strand C. The sequential strand displacement reactions were also confirmed using magnetic beads and another fluorescent signal probe, A/FAM-B/CY5-C. Magnetic beads loaded with A/FAM-B/CY5-C were characterised by flow cytometry analysis and fluorescence microscopy observation, showing the simultaneous intense fluorescence of FAM and CY5 (Fig. S3 and S4†). Fig. S5† schematically shows the strand displacement reactions at the magnetic beads. Fig. S6† shows the flow cytometry analysis of FAM and CY5 fluorescence at magnetic beads with different miRNA inputs. Only CY5 fluorescence was observed with the addition of miR-21, both FAM and CY5 fluorescence was observed with the addition of only miR-210, and no fluorescence was observed with the addition of both miR-21 and miR-210. These results are consistent with the experimental design. When miR-21 initiated the first strand displacement at the magnetic beads, FAM-B was replaced and released from the magnetic beads due to the generation of A/CY5-C/miR-21. When both miR-21 and miR-210 were present, the strand displacement reactions occurred sequentially at the magnetic beads, and released both FAM-B and CY5-C from the surface of the beads.
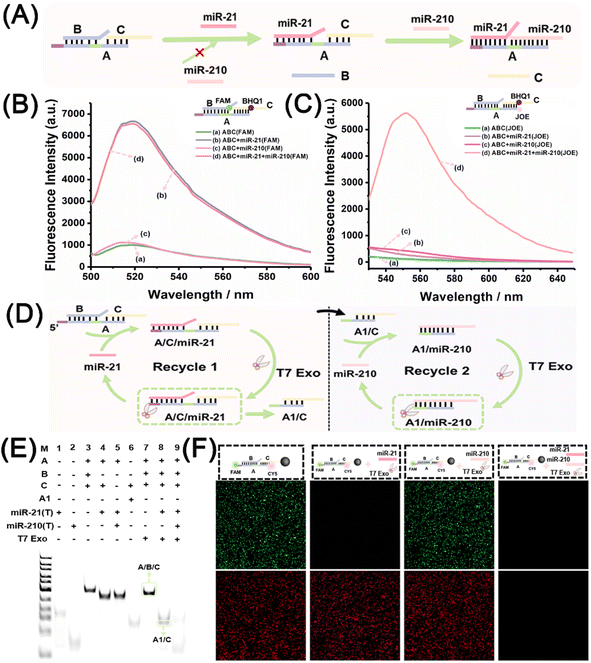 |
| Fig. 1 (A) Schematic diagram of the sequential strand displacement reactions with the input of miR-21 and miR-210 using A/B/C as a signal probe. (B) Fluorescence spectra of FAM obtained using different miRNA inputs. (C) Fluorescence spectra of JOE obtained using different miRNA inputs. (D) Schematic diagram of the working principles of the T7 Exo-powered miRNA discriminator. (E) Polyacrylamide electrophoresis gel analysis results of the T7 Exo-powered miRNA discriminator under different conditions. (F) Fluorescence microscope characterisation of the T7 Exo-powered miRNA discriminator at magnetic beads. | |
Successful design of the sequential strand displacement reactions lays the foundation for the T7 Exo-powered miRNA discriminator. Fig. 1D schematically illustrates the working principles of the discriminator, and Fig. 1E shows the polyacrylamide electrophoresis gel analysis results of the discriminator under different conditions. The band for A/B/C in Lane 7 confirms the resistance of the signal probe to T7 Exo digestion due to the presence of the 5′-overhang domain in strands A, B, and C. The emergence of the band for A1/C and the disappearance of the band for A/B/C in Lane 8 confirmed that T7 Exo powered the first strand displacement, while the disappearance of the bands of A/B/C and A1/C in Lane 9 confirmed the complete digestion of strand A after the sequential reactions with both miR-21 and miR-210. The T7 Exo-powered discriminator was also investigated by fluorescence microscopy observation of the magnetic beads loaded with A/B/C, in which strand A was labelled by FAM and CY5 at both ends, separately (Fig. 1F). Both FAM and CY5 fluorescence was observed surrounding the magnetic beads; only CY5 fluorescence was observed after the miR-21-initiated strand displacement occurred with the assistance of T7 Exo digestion; no fluorescence was observed after the complete digestion of strand A with the input of both miR-21 and miR-210. In contrast, when only miR-210 was present, dual fluorescence remained stable surrounding the magnetic beads, confirming the inherent order of the miRNA discriminator in the design. Moreover, T7 Exo digestion was utilized to accelerate the displacement efficiency, and resulted in greatly enhanced fluorescence compared to that without digestion using the fluorescent probes A/FAM-B/BHQ1-C and JOE-A/B/BHQ1-C (Fig. S7†). Overall, the fluorescence results demonstrated the design and feasibility of the T7 Exo-powered miRNA discriminator.
T7 Exo-powered miRNA discriminator for electrochemical measurement of miRNA biomarkers
To achieve the electrochemical measurement of miRNA biomarkers, electroactive A/B/C was prepared by the dual labelling of strand A with MB and Fc molecules. Free MB and Fc molecules were able to enrich a CB[7]-functionalised electrode through the host–guest interaction, enhancing the electrochemical signals (Fig. S8†). Fig. 2A schematically illustrates the electrochemical measurements conducted using the designed miRNA discriminator. miR-21 binds to the exposed toehold region within A/B/C, producing A/C/miR-21 via the first strand displacement. The partial digestion of A strand by T7 Exo not only leads to the release of end-labelled MB molecules and produces a quantitative signal corresponding to miR-21 but also accelerates strand displacement with the recycling of miR-21. Moreover, the partially digested product A1/C exposes another toehold region to interact with miR-210, initiating the second strand displacement reaction with the aid of T7 Exo. The complete digestion of the A1 strand by T7 Exo leads to the release of another end-labelled electrochemical tag, the Fc molecule, and produces another quantitative signal corresponding to miR-210. Fig. 2B shows the electrochemical responses obtained with or without T7 Exo digestion. The peak currents of MB and Fc were observed after T7 Exo-powered strand displacement with the addition of both miRNAs, but almost no responses were observed without T7 Exo digestion, even in the presence of miR-21 and miR-210. The electrochemical results were found to be in good agreement with the fluorescence analysis, and reconfirmed that T7 Exo digestion was essential to release both end-labelled electroactive tags.
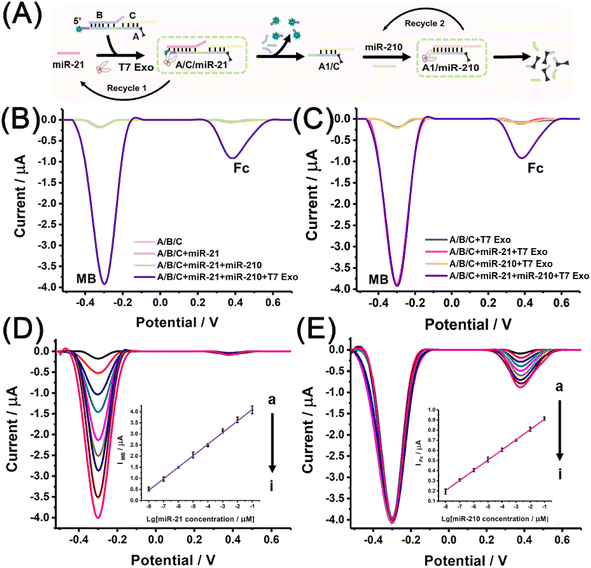 |
| Fig. 2 (A) Schematic illustration of the electrochemical measurement of miRNA biomarkers using the T7 Exo-powered miRNA discriminator. (B) Electrochemical responses obtained with miRNA inputs (miR-21 or both miR-21 and miR-210) and that with both miRNAs after T7 Exo digestion. (C) Electrochemical responses obtained with different miRNA inputs (miR-21, miR-210 and both miRNAs) after T7 Exo digestion. (D) Electrochemical responses obtained with miR-21 at different concentrations (from a to i: 0, 10 fM, 100 fM, 1 pM, 10 pM, 100 pM, 1 nM, 10 nM, and 100 nM). The inset shows the linear relationship between IMB and the logarithmic value of miR-21 concentration. (E) Electrochemical responses obtained with the co-existence of miR-21 (100 nM) and miR-210 at different concentrations (from a to i: 0, 10 fM, 100 fM, 1 pM, 10 pM, 100 pM, 1 nM, 10 nM, and 100 nM). The inset shows the linear relationship between IFc and the logarithmic value of miR-210 concentration. | |
Fig. 2C shows the electrochemical responses obtained after T7 Exo digestion with different miRNA inputs. The MB signal was observed when miR-21 was added, both MB and Fc signals were observed simultaneously when both miRNAs were added, but almost no peak current was observed in the absence of miRNAs or the presence of only miR-210. Overall, the electrochemical results demonstrated the feasibility of using a dual signals-based miRNA discriminator to identify miR-21 and miR-210 in sequence.
To ensure the high sensitivity of the miRNA discriminator, experiments were performed to optimize the reaction conditions, including the concentration of signal probe, the reaction time for T7 Exo-powered strand displacement reactions, the pH and the temperature (Fig. S9–S14†). The quantitative determination of miRNA biomarkers was performed under the optimised conditions. Fig. 2D shows the electrochemical responses attained using different miR-21 concentrations. As expected, the MB signal increased with the enhancement in the miR-21 concentration. The correlation between the peak current of MB (IMB) and the logarithmic value of miR-21 concentration (CmiR-21) revealed a linear relationship in the range from 10 fM to 100 nM. The regression equation was determined to be IMB (μA) = 0.521 × lg
CmiR-21 + 4.63 (coefficient of determination, R2 = 0.998), and the detection limit was estimated to be 3.0 fM for miR-21 at a signal-to-noise ratio of 3. Fig. 2E shows the electrochemical responses attained with the co-existence of miR-21 (100 nM) and miR-210 at different concentrations. The MB signal remained stable due to the fixed miR-21 concentration, while the Fc signal increased with the enhancement in the miR-210 concentration. The inset of Fig. 2E shows a linear relationship between the peak current of Fc (IFc) and the logarithmic value of the miR-210 concentration (CmiR-210) in the range from 10 fM to 100 nM. The regression equation was determined to be IFc (μA) = 0.101 × lg
CmiR-210 + 1.01 (R2 = 0.999), and the detection limit was calculated to be 1.81 fM for miR-210 determination at a signal-to-noise ratio of 3. Table S1† shows the comparison of the method developed in this study and the methods currently available for the quantification of miRNA.41–46 The method developed in this study showed an improved sensitivity with a relatively wider linear range and a lower detection limit compared to the existing methods.
The selectivity of the miRNA discriminator was then examined. Fig. 3A shows the electrochemical responses attained with the different miRNA inputs of miR-210, miR-188, miR-375, and miR-21 after T7 Exo digestion. An intense MB signal was observed upon the addition of miR-21, but low-intensity MB signals approaching that of the background were observed upon the addition of the other control miRNAs. It is worth mentioning here that almost no MB signal was observed, even for miR-210. Fig. 3B shows the electrochemical responses obtained with the co-existence of miR-21 (100 nM) and the different miRNAs miR-210, miR-188, and miR-375 after T7 Exo digestion. The MB signal remained stable at a high level, but an intense Fc signal was only observed in the presence of miR-210. These results were reasonable and proved the selectivity of the discriminator. In the first T7 Exo-powered strand displacement reaction, the end-labelled MB molecules were released and enriched at the electrode to produce an MB signal corresponding to miR-21; in the second strand displacement reaction, the end-labelled Fc molecules were released to produce an Fc signal corresponding to miR-210. The selectivity of the method was also investigated using mismatched miRNAs. As shown in Fig. 3C, a decreased IMB was observed upon the addition of mismatched miR-21, and the IMB obtained with the control miRNA containing three mismatched bases (TM) was almost as low as the background. The statistical analysis revealed that the peak current attained with the target miRNA was significantly higher than that even with only single mismatched base (SM). A similar trend was also revealed by tracing the Fc signal with the mismatched miR-210 (Fig. 3D). Notably, the position and the type of the single mismatched base was also proven to adversely affect the stability of the hybridisation with the probe in both control experiments, which was in line with previous reports and confirmed high selectivity of our discriminator.47,48
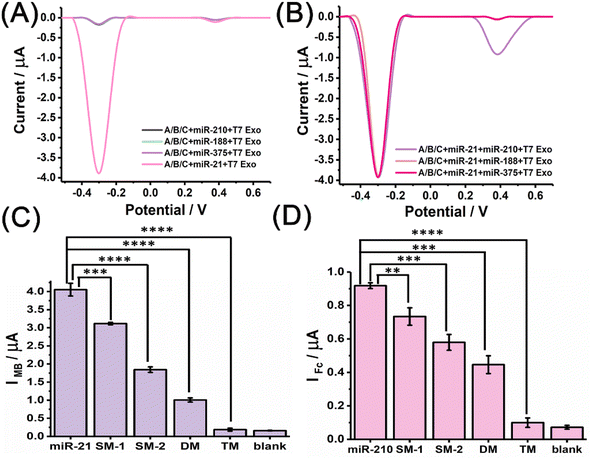 |
| Fig. 3 (A) Electrochemical responses obtained with the different miRNAs miR-210, miR-188, miR-375, and miR-21 after T7 Exo-powered strand displacement. (B) Electrochemical responses attained with the co-existence of miR-21 (100 nM) and the different miRNAs miR-210, miR-188, and miR-375 after T7 Exo-powered strand displacement. (C) IMB attained with miR-21 (100 nM) and mismatched miR-21 containing single mismatched (SM), double mismatched (DM), and triple mismatched (TM) bases. (D) IFc obtained with miR-210 (100 nM) and the mismatched miR-210 containing single SM, DM, and TM bases. Statistical significance was determined by two-tailed Student's t-test; **p < 0.01, ***p < 0.001, ****p < 0.0001. | |
The anti-interference performance of the miRNA discriminator was investigated in a complex serum environment. Fig. S15 and S16† show the comparison of the IMB and IFc values obtained in buffer and those obtained in the serum samples, respectively. The peak currents attained in the serum samples were all comparable to those in the buffer, and the recoveries observed in the serum samples were calculated to be 101.0–108.2% for miR-21 and 96.07–99.33% for miR-210 (Tables S2 and S3†). Overall, the electrochemical results showed the satisfactory sensitivity, specificity, and anti-interference properties of the miRNA discriminator, even in a complex environment.
T7 Exo-powered miRNA discriminator for electrochemical analysis of breast cancer cells with different subtypes
Encouraged by its performance in the electrochemical measurement of the miRNA biomarkers, the miRNA discriminator was further applied to analyse different breast cancer cells, including ER-positive MCF-7, HER-2-positive BT-474, and triple-negative MDA-MB-231 cells. A breast cell line, MCF-10A, was used as the normal control. Fig. 4A schematically illustrates the application of the T7 Exo-powered miRNA discriminator in the subtype-specific detection of breast cancer. RNA extracts were obtained from different cell samples by using the standard extract kits that contained RNase inhibitors. The gold-standard method, quantitative reverse transcription polymerase chain reaction (qRT-PCR), was carried out to determine the miR-21 and miR-210 levels in the different cells. Fig. 4B shows the miR-21 levels in different breast cancer cells relative to that in the normal control MCF-10A cells, suggesting up-regulated miR-21 expression in all the breast cancer cell lines. Fig. 4C shows the miR-210 levels in different breast cancer cells relative to the normal control MCF-10A cells, suggesting elevated miR-210 expression exclusively in the MDA-MB-231 cell line that has TNBC features. Fig. 4D shows the MB signals attained for the MCF-10A, MCF-7, BT-474, and MDA-MB-231 cells. MB signals corresponding to miR-21 levels were found to significantly increase in the breast cancer cell lines. As a comparison, the MB signal observed for the MCF-10A cells was as low in intensity as the background. Fig. 4E further shows the Fc signals observed for the MCF-10A, MCF-7, BT-474, and MDA-MB-231 cells. A greatly enhanced Fc signal was observed only in the case of MDA-MB-231 cells. The electrochemical results demonstrated that the miR-21 levels could be used to distinguish breast cancer cells from normal cells and the miR-210 levels could be used to further identify TNBC subtype features.
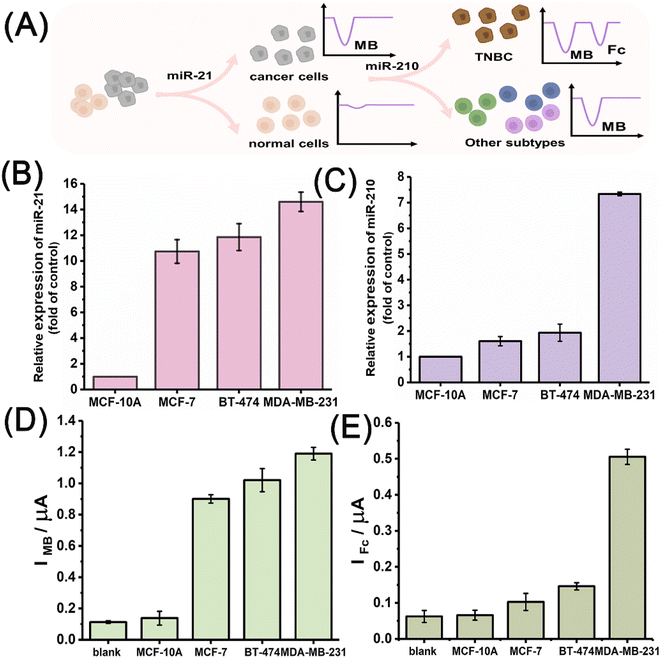 |
| Fig. 4 (A) Schematic illustration of the use of the miRNA discriminator in the subtype-specific detection of breast cancer. (B) miR-21 expressions determined by qRT-PCR in different cancer cells (MCF-7, BT-474, and MDA-MB-231 cells) relative to that in MCF-10A cells. (C) miR-210 expressions determined by qRT-PCR in different cancer cells (MCF-7, BT-474, and MDA-MB-231 cells) relative to that in MCF-10A cells. (D) IMB attained for MCF-10A, MCF-7, BT-474, and MDA-MB-231 cells. (E) IFc attained for MCF-10A, MCF-7, BT-474, and MDA-MB-231 cells. | |
Fig. 5A shows the MB signals observed for the different cells with enhanced numbers from 10 to 105 cells using the T7 Exo powered miRNA discriminator. In line with an increase in the number of cells, the MB signal gradually increased in intensity regardless of the breast cancer subtype, but stayed at an extremely low level for the MCF-10A cells. The MB signal corresponding to miR-21 was highly sensitive for identifying breast cancer, even with only 10 cells. Fig. 5B further shows the Fc signals obtained for the different cells in line with increase in the number of cells from 10 to 105 cells. The Fc signal exhibited an obvious increase with an increase in the number of MDA-MB-231 cells, whereas only a slight increase in intensity of the Fc signal was observed for the MCF-7 and BT-474 cells. These results were in good agreement with miRNA expression determined by qRT-PCR. miR-21 was highly expressed in all the breast cancer cells, while miR-210 expression in the TNBC subtype MDA-MB-231 cells was much higher than that in luminal-like MCF-7 and HER-2 positive BT-474 cells. In order to clarify the reliable and accurate miRNA discriminator, a miR-21 mimic was used to pre-treat the normal MCF-10A cells. The peak current was observed to increase evidently due to the up-regulated miR-21 level after the treatment with miR-21 mimic, which was in accordance with qRT-PCR results (Fig. S17†). Furthermore, when MCF-7, BT-474, and MDA-MB-231 cells were treated with miR-21 inhibitor,49 the MB signals sharply decreased in intensity to a negligible level in the presence of increased inhibitor concentrations due to the inhibition of miR-21-initiated strand displacement (Fig. 5C). Interestingly, Fc signals were also found to decrease with the addition of miR-21 inhibitors (Fig. S18†). This result was reasonable and reconfirmed the order of the strand displacement, in which the miR-210-initiated strand displacement was on the basis of miR-21-initiated strand displacement. Fig. 5D shows Fc signals attained for MDA-MB-231 cells after treatment with different concentrations of the miR-210 inhibitor.20 A high-intensity Fc signal was observed for the MDA-MB-231 cells due to high miR-210 expression, but gradually decreased in intensity to quite a low level in line with increased inhibitor concentration, which was consistent with the qRT-PCR determination (Fig. S19†). Overall, these results demonstrated that miR-21 could be used to discriminate between breast cancer and normal cells, and miR-210 was used to further identify the subtype-specific features of TNBC among different breast cancer cells.
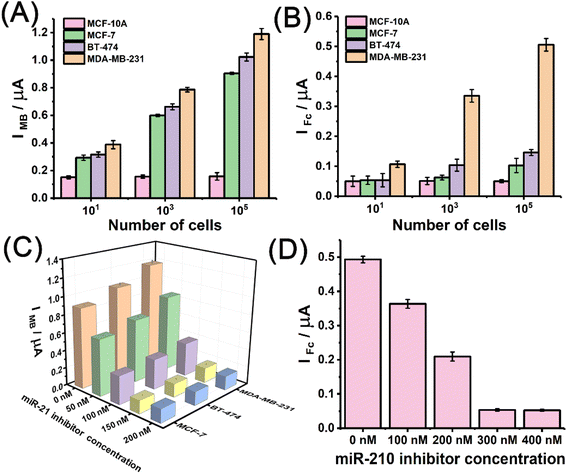 |
| Fig. 5 (A) IMB obtained for different numbers of MCF-10A, MCF-7, BT-474, and MDA-MB-231 cells. (B) IFc obtained for different numbers of MCF-10A, MCF-7, BT-474, and MDA-MB-231 cells. (C) IMB obtained for MCF-7, BT-474, and MDA-MB-231 cells that were pre-treated with different concentrations of miR-21 inhibitor. (D) IFc obtained for MDA-MB-231 cells that were pre-treated with different concentrations of miR-210 inhibitor. | |
Finally, the feasibility of the designed miRNA discriminator was verified in the analysis of real tissue samples. To do so, a tumour xenograft mouse model was established via the subcutaneous injection of MDA-MB-231 cells, and the tumour and normal breast tissues were finally excised for analysis after the mice were sacrificed. As shown in Fig. S20,† both MB and Fc signals were found to evidently increase in intensity in the tumour tissue compared to in the normal breast tissue, confirming the stability and practicability of the designed discriminator in complex environments.
Conclusions
An enzyme-powered miRNA discriminator was designed to facilitate the detection of breast tumours and also reveal the subtype-specific features in breast cancer on the basis of highly-ordered strand displacement reactions. Two endogenous miRNA biomarkers, miR-21 and miR-210, were selected to identify breast cancer cells and TNBC features, respectively, which triggered strand displacement reactions in sequence. miR-21 triggered the first enzyme-powered strand displacement, and the generated MB signal corresponding to miR-21 allowed the distinguishing between breast cancer and normal cells, even at a fairly low concentration. Thereafter, miR-210 triggered the second strand displacement using the newly-digested product, and the generated Fc signal corresponding to miR-210 further revealed the subtype-specific features of breast cancer cells, taking the TNBC subtype as an example. As important biomarkers in cancer diagnosis, miRNAs play a key role in cancer occurrence and development, thus miRNA profiling may provide more precise information for the implementation of targeted treatment and prediction of prognosis. In future studies, more miRNA biomarkers could be combined and introduced to the miRNA discriminator for a detailed understanding of tumour features in breast cancer, offering new options for the management of cancer, especially for subtypes with poor prognosis, such as HER-2-positive and TNBC subtypes. Moreover, well-organised strand displacement may also provide new insight into the development of an automatous DNA nanomachine and elucidate the complicated logic relationship involved in cancer-related mechanisms, thus promoting cancer diagnosis and therapy.
Data availability
All relevant data is presented in the article and the ESI.‡
Author contributions
Huiru Mao: methodology, validation, investigation, writing – original draft. Ya Cao: methodology, validation, investigation. Zihan Zou: investigation, Jianan Xia: investigation. Jing Zhao: conceptualisation, writing – review & editing, supervision.
Conflicts of interest
There are no conflicts to declare.
Acknowledgements
This work was supported by the National Natural Science Foundation of China (Grant No. 81972799, 81871449, and 81671781).
Notes and references
- A. N. Giaquinto, H. Sung, K. D. Miller, J. L. Kramer, L. A. Newman, A. Minihan, J. Ahmedin and R. L. Siegel, Ca-Cancer J. Clin., 2022, 72, 524–541 CrossRef.
- O. Ginsburg, F. Bray, M. P. Coleman, V. Vanderpuye, A. Eniu, S. R. Kotha, M. Sarker, T. T. Huong, C. Allemani, A. Dvaladze, J. Gralow, K. Yeates, C. Taylor, N. Oomman, S. Krishnan, R. Sullivan, D. Kombe, M. M. Blas, G. Parham, N. Kassami and L. Conteh, Lancet, 2017, 389, 847–860 CrossRef.
- R. Hong and B. Xu, Cancer Commun., 2022, 42, 913–936 CrossRef.
- D. Zardavas, A. Irrthum, C. Swanton and M. Piccart, Nat. Rev. Clin. Oncol., 2015, 12, 381–394 CrossRef CAS PubMed.
- D. Barba, A. León-Sosa, P. Lugo, D. Suquillo, F. Torres, F. Surre, L. Trojman and A. Caicedo, Crit. Rev. Oncol. Hematol., 2021, 157, 103174 CrossRef.
- S. K. Yeo and J.-L. Guan, Trends Cancer, 2017, 3, 753–760 CrossRef CAS PubMed.
- K. L. Britt, J. Cuzick and K. A. Phillips, Nat. Rev. Cancer, 2020, 20, 417–436 CrossRef CAS PubMed.
- J. Bange, E. Zwick and A. Ullrich, Nat. Med., 2001, 7, 548–552 CrossRef CAS.
- H. Huang, J. Hu, A. Maryam, Q. Huang, Y. Zhang, S. Ramakrishnan, J. Li, H. Ma, V. W. S. Ma, W. Cheuk, G. Y. K. So, W. Wang, W. C. S. Cho, L. Zhang, K. M. Chan, X. Wang and Y. R. Chin, Nat. Commun., 2021, 12, 2242 CrossRef CAS.
- Y. Gong, P. Ji, Y. Yang, S. Xie, T. Yu, Y. Xiao, M. Jin, D. Ma, L. Guo, Y. Pei, W. Chai, D. Li, F. Bai, F. Bertucci, X. Hu, Y. Jiang and Z. Shao, Cell Metab., 2021, 33, 51–64 CrossRef CAS PubMed.
- G. Bianchini, C. De Angelis, L. Licata and L. Gianni, Nat. Rev. Clin. Oncol., 2022, 19, 91–113 CrossRef CAS PubMed.
- G. Peluffo, A. Subedee, N. W. Harper, N. Kingston, B. Jovanović, F. Flores, L. E. Stevens, F. Beca, A. Trinh, C. S. R. Chilamakuri, E. K. Papachristou, K. Murphy, Y. Su, A. Marusyk, C. S. D'Santos, O. M. Rueda, A. H. Beck, C. Caldas, J. S. Carroll and K. Polyak, Cancer Res., 2019, 79, 4173–4183 CrossRef CAS PubMed.
- B. D. Lehmann, A. Colaprico, T. C. Silva, J. J. Chen, H. B. An, Y. G. Ban, H. Huang, L. Wang, J. L. James, J. M. Balko, P. I. G. Ericsson, M. E. Sanders, B. Zhang, J. A. Pietenpol and X. S. Chen, Nat. Commun., 2021, 12, 6276 CrossRef CAS.
- M. Ha and V. N. Kim, Nat. Rev. Mol. Cell Biol., 2014, 15, 509–524 CrossRef CAS.
- B. M. Hussen, H. J. Hidayat, A. Salihi, D. K. Sabir, M. Taheri and S. Ghafouri-Fard, Biomed. Pharmacother., 2021, 138, 111528 CrossRef CAS.
- Y. Xing, G. Ruan, H. Ni, H. Qin, S. Chen, X. Gu, J. Shang, Y. Zhou, X. Tao and L. Zheng, Front. Immunol., 2021, 12, 624725 CrossRef CAS PubMed.
- S. Cai, T. Pataillot-Meakin, A. Shibakawa, R. Ren, C. L. Bevan, S. Ladame, A. P. Ivanov and J. B. Edel, Nat. Commun., 2021, 12, 3515 CrossRef CAS PubMed.
- D. Bautista-Sánchez, C. Arriaga-Canon, A. Pedroza-Torres, I. A. De La Rosa-Velázquez, R. González-Barrios, L. Contreras-Espinosa, R. Montiel-Manríquez, C. Castro-Hernández, V. Fragoso-Ontiveros, R. María Álvarez-Gómez and L. A. Herrera, Mol. Ther. Nucleic Acids, 2020, 20, 409–420 CrossRef.
- S. Zhu, H. Wu, F. Wu, D. Nie, S. Sheng and Y. Mo, Cell Res., 2008, 18, 350–359 CrossRef CAS PubMed.
- M. G. Costales, C. L. Haga, S. P. Velagapudi, J. L. Childs-Disney, D. G. Phinney and M. D. Disney, J. Am. Chem. Soc., 2017, 139, 3446–3455 CrossRef CAS.
- Y. Du, N. Wei, R. Ma, S. Jiang and D. Song, Cell Death Dis., 2020, 11, 731 CrossRef CAS PubMed.
- R. P. Arun, H. F. Cahill and P. Marcato, Biomedicines, 2022, 10, 651 CrossRef CAS PubMed.
- S. Jiang, Z. Ge, S. Mou, H. Yan and C. Fan, Chem, 2021, 7, 1156–1179 CAS.
- Y. Cao, X. Yu, B. Han, L. Dong, J. Xu, Y. Dai, G. Li and J. Zhao, J. Am. Chem. Soc., 2021, 143, 16078–16086 CrossRef CAS PubMed.
- Y. Hu, Y. Wang, J. Yan, N. Wen, H. Xiong, S. Cai, Q. He, D. Peng, Z. Liu and Y. Liu, Adv. Sci., 2020, 7, 2000557 CrossRef CAS PubMed.
- Y. Cao, X. Yu, T. Zeng, Z. Fu, Y. Zhao, B. Nie, J. Zhao, Y. Yin and G. Li, J. Am. Chem. Soc., 2022, 144, 13475–13486 CrossRef CAS.
- F. C. Simmel, B. Yurke and H. R. Singh, Chem. Rev., 2019, 119, 6326–6369 CrossRef CAS PubMed.
- J. Chen, S. Fu, C. Zhang, H. Liu and X. Su, Small, 2022, 18, 2108008 CrossRef CAS PubMed.
- M. Rudchenko, S. Taylor, P. Pallavi, A. Dechkovskaia, S. Khan, V. P. Butler Jr, S. Rudchenko and M. N. Stojanovic, Nat. Nanotechnol., 2013, 8, 580–586 CrossRef CAS PubMed.
- M. You, G. Zhu, T. Chen, M. J. Donovan and W. Tan, J. Am. Chem. Soc., 2015, 137, 667–674 CrossRef CAS PubMed.
- K. Yuan, H. Meng, Y. Wu, J. Chen, H. Xu, L. Qu, L. Li and Z. Li, CCS Chem., 2022, 4, 1597–1609 CrossRef CAS.
- F. Li, G. Li, S. Cao, B. Liu, X. Ren, N. Kang and F. Qiu, Biosens. Bioelectron., 2021, 172, 112757 CrossRef CAS PubMed.
- C. Xing, Q. Lin, X. Gao, T. Cao, J. Chen, J. Liu, Y. Lin, J. Wang and C. Lu, ACS Appl. Mater. Interfaces, 2022, 14, 39866–39872 CrossRef CAS.
- S. He, S. Yu, R. Li, Y. Chen, Q. Wang, Y. He, X. Liu and F. Wang, Angew. Chem., Int. Ed., 2022, 61, e202206529 CAS.
- M. Bai, F. Chen, X. Cao, Y. Zhao, J. Xue, X. Yu, C. Fan and Y. Zhao, Angew. Chem., Int. Ed., 2020, 59, 13267–13272 CrossRef CAS PubMed.
- Y. Wu, Y. He, M. Han, D. Zhao, B. Liu, K. Yuan, H. Sun, H. Meng and Z. Li, CCS Chem., 2022, 1–13 Search PubMed.
- X. Gong, J. Wei, J. Liu, R. Li, X. Liu and F. Wang, Chem. Sci., 2019, 10, 2989–2997 RSC.
- P. Miao, T. Zhang, J. Xu and Y. Tang, Anal. Chem., 2018, 90, 11154–11160 CrossRef CAS PubMed.
- Y. Cao, D. Chen, W. Chen, J. Yu, Z. Chen and G. Li, Anal. Chim. Acta, 2014, 812, 45–49 CrossRef CAS PubMed.
- W. S. Jeon, K. Moon, S. H. Park, H. Chun, Y. H. Ko, J. Y. Lee, E. S. Lee, S. Samal, N. Selvapalam, M. V. Rekharsky, V. Sindelar, D. Sobransingh, Y. Inoue, A. E. Kaifer and K. Kim, J. Am. Chem. Soc., 2005, 127, 12984–12989 CrossRef CAS.
- Y. Xu, C. Wang, G. Liu, X. Zhao, Q. Qian, S. Li and X. Mi, Biosens. Bioelectron., 2022, 217, 114671 CrossRef CAS.
- Z. Wang, Y. Zhang, X. Wang and L. Han, Biosens. Bioelectron., 2022, 206, 114120 CrossRef CAS.
- X. Xie, Z. Wang, M. Zhou, Y. Xing, Y. Chen, J. Huang, K. Cai and J. Zhang, Small Methods, 2021, 5, 2101072 CrossRef CAS PubMed.
- H.-Y. Wang, Y.-T. Xu, B. Wang, S.-Y. Yu, X.-M. Shi, W.-W. Zhao, D. Jiang, H.-Y. Chen and J.-J. Xu, Angew. Chem., Int. Ed., 2022, e202212752 CAS.
- S. Cai, T. Pataillot-Meakin, A. Shibakawa, R. Ren, C. L. Bevan, S. Ladame, A. P. Ivanov and J. B. Edel, Nat. Commun., 2021, 12, 3515 CrossRef CAS PubMed.
- F. Meng, W. Yu, C. Chen, S. Guo, X. Tian, Y. Miao, L. Ma, X. Zhang, Y. Yu, L. Huang, K. Qian and J. Wang, Small, 2022, 18, 2200784 CrossRef CAS PubMed.
- C. Lou, A. Dallmann, P. Marafini, R. Gao and T. Brown, Chem. Sci., 2014, 5, 3836–3844 RSC.
- R. Liu, Y. Hu, Y. He, T. Lan and J. Zhang, Chem. Sci., 2021, 12, 9022–9030 RSC.
- J. Yi, T. Chen, J. Huo and X. Chu, Anal. Chem., 2017, 89, 12351–12359 CrossRef CAS PubMed.
Footnotes |
† Electronic supplementary information (ESI) available: Experimental details, electrophoresis analysis results, flow cytometry analysis results, fluorescence images, scheme of strand displacement reactions at magnetic beads, electrochemical responses of free electroactive molecules, optimisation experiments, peak currents of buffer and serum samples, comparison of currently available methods, quantification results in serum samples, and sequences of oligonucleotides used in this work. See DOI: https://doi.org/10.1039/d3sc00090g |
‡ These authors contributed equally to this work. |
|
This journal is © The Royal Society of Chemistry 2023 |