DOI:
10.1039/D3RA02702C
(Paper)
RSC Adv., 2023,
13, 19789-19802
Gingerol extract-stabilized silver nanoparticles and their applications: colorimetric and machine learning-based sensing of Hg(II) and antibacterial properties†
Received
24th April 2023
, Accepted 14th June 2023
First published on 3rd July 2023
Abstract
This study focused on synthesizing ginger-stabilized silver nanoparticles (Gin-AgNPs) using a more eco-friendly method that utilized AgNO3 and natural ginger solution. These nanoparticles underwent a color change from yellow to colorless when exposed to Hg2+, enabling the detection of Hg2+ in tap water. The colorimetric sensor had good sensitivity, with a limit of detection (LOD) of 1.46 μM and a limit of quantitation (LOQ) of 3.04 μM. Importantly, the sensor operated accurately without being affected by various other metal ions. To enhance its performance, a machine learning approach was employed and achieved accuracy ranging from 0% to 14.66% when trained with images of Gin-AgNP solutions containing different Hg2+ concentrations. Furthermore, the Gin-AgNPs and Gin-AgNPs hydrogels exhibited antibacterial effects against both Gram-negative and Gram-positive bacteria, indicating potential future applications in the detection of Hg2+ and in wound healing.
1. Introduction
Heavy metal pollution of the environment remains a pressing problem worldwide. Metal contamination occurs in industrial facilities like coal power plants, textile and plastic factories, and others. Heavy metals, especially mercury (Hg2+), affect the environment in many ways and are severely toxic to humans. Mercury (Hg2+) can damage the brain, nervous system, kidneys and endocrine system.1 In order to protect from and detect mercury contamination in the environment, scientists have used many techniques, including atomic absorption spectrometry (AAS),2 atomic fluorescence spectrometry (AFS),3 microwave-induced plasma-atomic emission (MIP-AES),4 inductively coupled plasma-mass spectrometry (ICP-MS),5 inductively coupled plasma-atomic emission spectrometry (ICP-AES),6 UV-vis spectroscopy,7 high-performance liquid chromatography (HPLC)8 and ion-selective electrodes (ISE).9
All of the above methods are expensive, time-consuming and require sophisticated equipment.10 In contrast, colorimetric sensors are simple, low-cost, on-site sensors with high sensitivity.11 For example, fluorometric and colorimetric sensor arrays have been used as an enzyme-triggered dual-signal system with gold/silver nanoparticles and carbon nanodots for the detection of glucose,12 and ForceSpun polydiacetylene nanofibers have been used as colorimetric sensors for detecting food spoilage.13 A variety of nanomaterials-based colorimetric probes, including those that use gold,14 silver15 and copper nanoparticles,16 as well as nanoclusters,17 have been developed for the detection of Hg2+.
Silver nanoparticles (AgNPs) are a popular choice due to their antibacterial and catalytic properties, and their applications in drug delivery, bio-sensing, biomedical engineering, nanomedicine, and also colorimetric detection of mercury.18,19 Silver nanoparticles are also widely used as colorimetric sensors for other heavy metal ions such as lead(II),20 copper(II),21 arsenic(III),22 and mercury(II).23 This success comes from functionalization of AgNPs by organic molecules leading to reliable, stable, and sensitive detection.24 Synthesis of silver nanoparticles can be performed by chemical and physical methods.25 Many green synthetic methods using organic molecules as stabilizing agents,26 for example, using an aqueous extract of Salacia chinensis (SC) bark27 and Lysiloma acapulcensis27 as green resources to reduce silver nitrate to silver nanoparticles and subsequently stabilize them.
Zingiber officinale, commonly known as ginger, has been used as a spice and herb worldwide28 and also used as a traditional medicine for treating vomiting, pain, and cold symptoms in China for at least 200 years.29 Ginger contains many bioactive compounds, such as phenolic and terpene, compounds that can be antioxidant, anti-inflammatory, antimicrobial and anticancer molecules.30 Beyond these medical applications, ginger can be used in the green synthesis of AgNPs for the inhibition of SARS-CoV-2,31 against food pathogens32 and additionally used as a colorimetric sensor for detecting mercury.33
In this study, a colorimetric sensor was developed from ginger-stabilized silver nanoparticles (Gin-AgNPs) to detect mercury(II) ions (Hg2+) based on redox interactions between Hg2+ and Gin-AgNPs (depicted in Scheme 1). The original yellow color of the Gin-AgNPs solution changes to colorless based on the concentration of Hg2+. In addition to being able to detect Hg2+, our Gin-AgNPs have been shown to have antibacterial action towards both Gram-positive (S. aureus) and Gram-negative (E. coli) bacteria. Gin-AgNPs are therefore of great interest to apply as selective and efficient visual Hg2+ sensors, and for potential biomedical applications.
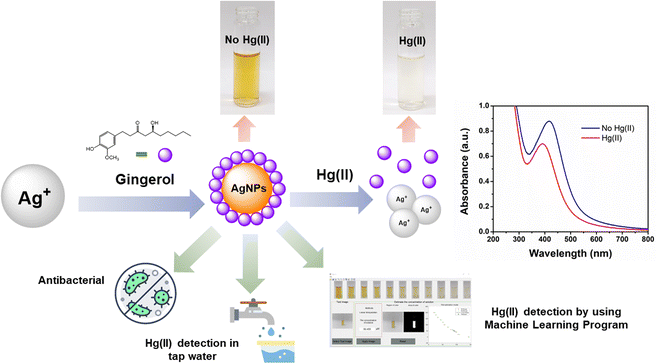 |
| Scheme 1 A schematic illustration of the Hg2+ and Gin-AgNPs sensing mechanism and application to real world drinking water samples, including utilizing machine learning software for the sensing analysis. Assessment of antibacterial activity is also undertaken. | |
2. Materials and methods
2.1 Materials
Silver nitrate (AgNO3, 99.9%) was purchased from POCH™, and the ginger extract capsule dietary supplements were purchased from a drugstore in Khon Kaen, Thailand. Fresh Cissampelos pareira L. was purchased from Khon Kaen, Thailand and ethanol (C2H6O) was purchased from V. S. Chemhouse, Thailand. Tris(hydroxymethyl)methylamine (C4H11NO3, 99.8%), sodium nitrate (NaNO3, 99%), magnesium nitrate (Mg(NO3)2, 99.4%), barium nitrate (Ba(NO3)2, 99%) and cobalt(II) nitrate hexahydrate (Co(NO3)2·6H2O, 98%) were purchased from Univar, Australia. Manganese(II) nitrate tetrahydrate (Mn(NO3)2·4H2O, 97%) was purchased from Sigma Aldrich, China. Sodium hydroxide anhydrous (NaOH, 99%), lead(II) nitrate (Pb(NO3)2, 99%), nickel(II) nitrate hexahydrate (Ni(NO3)2·6H2O, 99%), and cadmium nitrate tetrahydrate (Cd(NO3)2·4H2O, 99%) were purchased from Carlo Erba, Italy. Hydrochloric acid (37%) was purchased from RCI Labscan, Thailand, and potassium nitrate (K2NO3, 99%) was purchased from BDH, England. Calcium chloride (CaCl2, 95%) was purchased from Scharlau, Spain. Copper(II) nitrate trihydrate (Cu(NO3)2·3H2O, 99%) and zinc nitrate hexahydrate (Zn(NO3)2·6H2O, 99%) were purchased from Fluka, Switzerland. Dipotassium phosphate (K2HPO4, 99%), potassium dihydrogen phosphate (KH2PO4, 90%), and mercury(II) chloride (HgCl2, 99.5%) were purchased from QRec™, New Zealand. Deionized water (DI) with specific resistivity of 18.2 MΩ cm was obtained from a RiOs™ Type I Simplicity 185 (Millipore water purification system). Bacterial strains were obtained from the Biochemistry Laboratory, Biochemistry Department, Faculty of Science, Khon Kaen University, Thailand. Mueller Hinton Broth (MHB) was purchased from HiMedia, India. Gentamicin sulfate, 7-hydroxy-3H-phenoxazine-3-one-10-oxide sodium salt (C12H6NNaO4, ∼80%), and glycerol, were purchased from Sigma-Aldrich, St. Louis, Missouri, the United States. Antimicrobial activity assays were performed using Staphylococcus aureus (S. aureus) and Escherichia coli (E. coli) as Gram-positive and Gram-negative controls, respectively.
2.2 Preparation of ginger solution and ginger-stabilized silver nanoparticles (Gin-AgNPs)
Ginger solutions were prepared by adding 2.5 g of ginger powder to 50 mL of deionized (DI) water and then stirring for 15 minutes at 60 °C. The mixtures were cooled at room temperature and filtered through Whatman filter paper, then a nylon syringe filter (pore size 0.22 μM). The prepared ginger solutions were then kept in the refrigerator.
Ginger-stabilized silver nanoparticles (Gin-AgNPs) were synthesized by a green synthesis method, using the ginger solution as both a reductant and stabilizing agent. Firstly, the ginger solution was added to 0.0485 g (5 mM) of AgNO3 in a round bottom flask and then the mixture was refluxed. 7 mL of 70% v/v ethanol was added drop by drop at 80 °C. The mixture was kept refluxing at 85 °C for 3 hours, then left to cool to room temperature, before filtering using a syringe filter (diameter 25 mm, pore size 0.22 μm). The obtained ginger-stabilized silver nanoparticles solution was dark yellow in color.
2.3 Calculation of concentration of silver nanoparticles
The concentration of silver nanoparticles was calculated by a procedure reported elsewhere by using UV-vis spectroscopy method.34 First, the nanoparticles were characterized by TEM. In this work, the number of atoms per nanoparticle were firstly calculated using by the method described by Gurunathan et al.35 As shown in eqn (1)where ρ is the density of face centered, cubic (fcc) silver (10.5 g cm−3), d is the average diameter of nanoparticles (= 11.89 nm = 11.89 × 10−7 cm as determined by transmission electron microscopy), M is the atomic mass of silver = 107.86 u, assuming that all silver ions in the initial solution reacted to form silver nanoparticles, N = 84.04.
Secondly, molar concentration of the silver nanoparticles C in solution is then approximately as eqn (2)36
where “
Ntotal” is the silver atoms added to the reaction solution (10 × 10
−6 × 6.02 × 10
23), “
V” is the volume of the reaction solution (67 mL), “
NA” is the Avogadro's constant, and “
N” is the number of atoms per nanoparticle calculated from
eqn (1). In this case
C = 1.77 μM was calculated for the as-prepared neat Gin-AgNPs solution.
2.4 Instrumentation for characterization of ginger-stabilized silver nanoparticles
The morphology and size of the synthesized Gin-AgNPs were determined by a transmission electron microscope (TEM), Tecnai G2-20 (FEI, Netherland) system, at an acceleration voltage of 200 kV. The formation of silver nanoparticles was checked by the XRD technique using a Bruker device (Model: D8 Advance) and the diffraction pattern of the synthesized NPs was recorded in the range of 10–90 (2θ). Ultraviolet-visible (UV-vis) spectra of Gin-AgNPs were recorded using a UV-vis spectrophotometer (Agilent Technologies Cary 60, Germany) by measuring the absorbance with a 1.0 cm path length quartz cell. Spectra were recorded over a range of 200–800 nm. The zeta potential of silver nanoparticles in aqueous suspension was determined using a Zetasizer Nano ZS (Malvern Instrument, UK). The pH of the Gin-AgNPs solution was determined using a HI 5221 pH meter (Hanna Instruments, Thailand).
2.5 Colorimetric sensing of Hg2+ and other metal ions by UV-vis spectroscopy and Gin-AgNPs
A stock solution of 10 mM HgCl2 (Hg2+) was prepared by dilution with DI water. Other metal ion solutions (Na+, K+, Ca2+, Mg2+, Ba2+, Pb2+, Mn2+, Ni2+, Cu2+, Zn2+, Cd2+ and Co2+) were prepared using their respective metallic salts. The colorimetric detection was carried out by adding 0.12 μM (350 μL) of Gin-AgNPs into a 5 mL volumetric flask, followed by 1 mL of DI water. After that, 50 mM of Tris–HCl buffer solution (pH 8) and 50 mM standard metal ions were added to 5 mL volumetric flasks, respectively, and all the solutions were adjusted to 5 mL with DI water. Finally, UV-vis spectra of the prepared solutions were recorded by UV-vis spectroscopy. The selectivity of Gin-AgNPs for Hg2+ was confirmed by comparison with other metal ions under the same conditions. All experiments were repeated at least three times at room temperature.
2.6 Naked-eye detection of Hg2+ in water
For the naked-eye detection of Hg2+, 0.12 μM (350 μL) of Gin-AgNPs solution was added to a 5 mL volumetric flask. 50 mM of Tris–HCl buffer solution (pH 8) was added. 100 μL of each 200 μM metal ions solutions were added to the 5 mL volumetric flasks and the solutions were adjusted to 5 mL with DI water. For testing the sensitivity of naked-eye detection of Hg2+, various concentrations of Hg2+ from 10 mM stock solution were added to the mixture of Gin-AgNPs solution and Tris–HCl buffers (pH 8.0) at different concentrations. The various concentrations of Hg2+ were 0, 20, 40, 60, 80, 100, 120, 140, 160, 180 and 200 μM.
2.7 Preparation of Gin-AgNPs hydrogels for antibacterial properties
For the preparation of the Gin-AgNPs hydrogels, 5 g of fresh Cissampelos pareira L. branches were washed with DI water and cut into small pieces, then blended in a fruit blender with 125 mL DI water. Then, two different concentrations of Gin-AgNPs (15 μM and 25 μM) solutions were added until they turned into a gel. The obtained gel was filtered using a flour colander. The resulting Gin-AgNPs hydrogel was kept in the refrigerator for a night before using in antibacterial tests. The appearance of the Gin-AgNPs in hydrogel form is shown in Fig. S1.†
2.8 Determination of Hg2+ in real water samples
For the determination of Hg2+ in real water samples, 3 tap water samples were screened. The tap water was collected from the laboratory and then left for a night and microfiltered before sampling. The water samples were spiked by adding Hg2+ 25 μM and 50 μM in 1 mL of water sample and 50 mM of Tris–HCl buffer solution (pH 8) was added, then the samples were adjusted to 5 mL with DI water.
2.9 Machine learning, image processing and determination of Hg2+
The concept of detection and estimation for the determination of Hg2+ concentrations with Gin-AgNPs using image processing can be summarized in the following 4 steps.
2.9.1 Setting up the database. The database consisted of images of Gin-AgNPs solutions with different mercury concentrations. The available 11 levels of concentration were 0, 20, 40, 60, 80, 100, 120, 140, 160, 180 and 200 μM. The color intensities of the solutions differed according to the added concentrations of mercury. These solutions were put in test tubes and the imaged (Fig. S2†). For creating the database, images of each test tube were taken from three different angles and stored. The aspect ratio of the images was 1
:
1. The setup for recording images of the solutions at different angles is depicted in Fig. S3(a) and (b).†
2.9.2 Extracting image features. For image processing, an ‘image feature’, a piece of information representing a particular characteristic of the image, must be extracted, analyzed and interpreted. The image feature can be a sub-region, edge or ridge that appears in the image. For this work, the yellow sub-region in the test tube was defined as the image feature. To extract the yellow sub-region in the image, each image was converted to a pixel matrix, and each coefficient in the form of (r, g, b) represents the level of red, green and blue, respectively. Our image feature was defined by:
Then, each pixel was converted to a binary value (0 or 1) based on a threshold of the yellow_pixel value. An example of extracting the feature and converting to a binary image is shown in Fig. S3(c).†
Next, the reference image feature was estimated. Let xji refer to the reference point for each image, where j = 1, 2, …, 11 was the number of groups and i = 1, 2, 3 was the number of images with different positions for each group. The mean of image feature, Xj,
and its standard deviation, sj for each group were calculated. Each non-overlapping data range was set as interval Rj = [aj, bj] = [Xj − sj, Xj + sj].
2.9.3 Estimating the concentration. In this step, the concentration of test or unknown solutions was estimated using the linear approximation technique. We let xtest be a reference image feature of test image and Y = [Yj]1×11 = [0, 20, 40, 60, 80, 100, 120, 140, 160, 180, 200] be a vector of all concentrations in the database.(1) If xtest ∈ Rj then the concentration of xtest is in group j,
(2) If Rj < xtest < Rj+1 then the concentration Ytest of solution xtest is approximated by using the linear approximation
(3) Otherwise, the concentration of xtest was out of the range 0–200 μM.
2.9.4 Determination of Hg2+ using a machine learning program. For the determination of Hg2+ using a machine learning program, we firstly screened 4 concentrations of Hg2+. Each sample was prepared by adding 0.12 μM (350 μL) of Gin-AgNPs solution to a 5 mL volumetric flask. Then the different concentrations of Hg2+ from 10 mM stock solution were added to the mixture of Gin-AgNPs solution and Tris–HCl buffers (pH 8.0). The concentrations of Hg2+ were 50, 90, 130 and 170 μM.
2.10 Antimicrobial activity
2.10.1 Bacterial strain and culture media. E. coli O157:H7 and S. aureus ATCC 25923 were obtained from the Protein and Proteomics Research Group, Khon Kaen University. These bacteria were stored at −70 °C in 20% glycerol until used. To grow bacteria, the bacteria were streaked on Mueller Hinton Agar (MHA) and incubated at 37 °C for 24 hours. The colonies of these bacteria were picked and inoculated in 5 mL of MHB at 37 °C overnight. Then, bacteria were subcultured in 5 mL of the same medium at 37 °C in a 180 rpm shaker incubator for 3 hours to yield a mid-logarithmic growth phase culture.
2.10.2 Antimicrobial susceptibility of Gin-AgNPs. The diffusion method was performed to evaluate the antimicrobial susceptibility of Gin-AgNPs. The overnight culture of E. coli and S. aureus was sub-cultured in MH broth for 3 hours. Then, the bacteria were diluted to OD600 = 0.01 (107 CFU mL−1) with the same media. The bacteria were swabbed on a three-dimensional MH agar plate. Then, wells were cut into the surface of the agar using a sterile yellow tip. 30 μL of Gin-AgNPs was dropped into the wells and incubated at 37 °C for 24 hours. Gentamicin and polymyxin b were used as the positive control and deionized water was used as the negative control, respectively. Inhibition of bacterial growth was evaluated by measuring the diameter (mm) of each zone of inhibition.
2.10.3 Antimicrobial assay of Gin-AgNPs. Minimum inhibitory concentrations (MICs) and minimum bactericidal concentrations (MBCs) were performed to evaluate the antimicrobial activity of Gin-AgNPs by the broth microdilution method. Briefly, a range of concentrations of Gin-AgNPs were prepared by serial dilutions of 2-fold. The solutions were then added to an equal volume of bacterial suspension (50 μL) in each well of a 96-well plate with the final cell concentration of 105 CFU mL−1. The plates were incubated at 37 °C for 18–20 h. After overnight incubation, 10 μL of 0.01% resazurin solution (7-hydroxy-3H-phenoxazine-3-one-10-oxide; Sigma-Aldrich) was added to each well and incubated at 37 °C for 2–3 hours. A color change was observed. The lowest concentration before the color change was determined as the MIC, the blue color represented no growth of bacteria and the pink meant bacterial survival.37 The MBC value was determined when there was no colony growth from the directly plated contents of the wells. The tests were performed in two independent experiments in triplicates.
2.10.4 Antimicrobial test of Gin-AgNPs hydrogel. The diffusion method was performed to assess the antimicrobial activity of Gin-AgNPs hydrogel. The overnight culture of E. coli and S. aureus was subcultured in MH broth for 3 hours. Then, the bacteria were diluted to OD600 = 0.01 (107 CFU mL−1) with the same media. The bacteria were swabbed onto a three-dimensional MH agar plate. Then, 4 mm diameter wells were cut into the surface of the agar using a sterile tip. The Gin-AgNPs hydrogel was dropped into the wells at 0.05 g and incubated at 37 °C for 24 hours. Gentamicin and polymyxin b were used as the positive control and deionized water was used as the negative control. Inhibition of bacterial growth was evaluated by measuring the diameter (mm) of each zone of inhibition.
3. Results and discussion
3.1 Characterization of ginger silver nanoparticles (Gin-AgNPs)
The synthesis of Gin-AgNPs was performed by the green synthesis method, using silver nitrate as a starting material and ginger solution as a reductant and stabilizing agent for improving the properties of the silver nanoparticles. The optical properties of Gin-AgNPs were characterized by UV-vis spectroscopy. The absorption (extinction) spectrum of the Gin-AgNPs showed the characteristic strong surface plasmon resonance (SPR) band at 420 nm (Fig. 1(a)). The size and morphologies of the AgNPs were investigated by transmission electron microscopy (TEM). The particles are well dispersed with homogeneous spherical shapes as depicted in Fig. 1(b). The average diameter of the AgNPs was calculated using the ImageJ program, revealing an average size of 11.89 ± 4.17 nm (n = 130) as shown in Fig. 1(c). The X-ray diffraction pattern of the AgNPs is shown in Fig. 1(d). The diffraction peaks were observed at 2θ of 34.98°, 38.20°, 46.34°, 64.80° and 77.34°, corresponding to the (111), (111), (200), (220) and (311) lattice planes, respectively. The observed diffraction peaks are in good agreement with the Joint Committee on Power Diffraction Standards (JCPDS) profile of the face-centered cubic (fcc) structure of silver.38
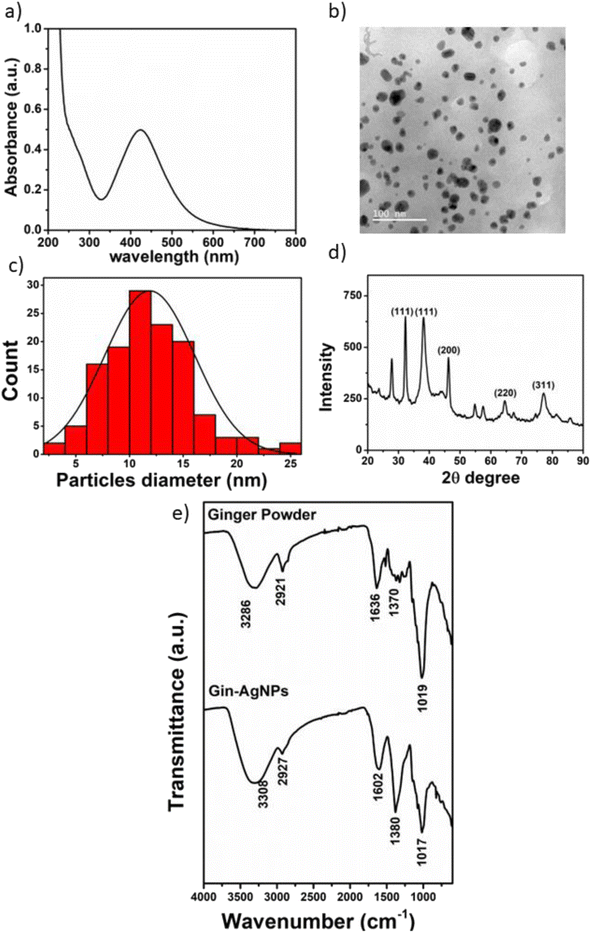 |
| Fig. 1 (a) UV-vis absorption spectra of Gin-AgNPs, (b) TEM image of Gin-AgNPs (11.89 ± 4.17 nm; n = 130), (c) size distribution plot of Gin-AgNPs, (d) XRD pattern of Gin-AgNPs and (e) FTIR spectra of ginger powder and Gin-AgNPs. | |
The functional groups present in ginger powder and the Gin-AgNPs were characterized by Fourier-transform infrared spectroscopy (FTIR), as shown in Fig. 1(e). The broad peak at 3286 cm−1 was attributed to the O–H stretching of hydrogen-bonds, suggesting that there were hydroxyl groups on the surface of the AgNPs. The bands at 2921, 1636, 1370 and 1263 cm−1 correspond to stretching vibrations of CH2, and C
O in amide, aromatic skeletons combined with C–H and C
O respectively. Finally, the peak at 1019 cm−1 is attributed to amino acid groups.39 These results confirm that the molecules in the ginger powder are also present on the surface of the Gin-AgNPs.
3.2 UV-vis detection and proposed mechanism for detection of Hg2+ with Gin-AgNPs
In this work, UV-vis spectroscopy was used to investigate the interaction between Gin-AgNPs and Hg2+. The absorption peak of Gin-AgNPs was observed at 420 nm. The absorption peak was reduced when Hg2+ was added into the Gin-AgNPs solution, and the yellow color of Gin-AgNPs turned colorless as depicted in Fig. S4(a) and (b),† attributed to the redox reaction between Gin-AgNPs and Hg2+. It was previously reported that silver nanoparticles have the potential to detect Hg2+ because silver nanoparticles have surface plasmon resonance (SPR) properties that can change by modifying the size, shape, composition and dielectric constant of the system.40 In our experiment, Gin-AgNPs showed a strong surface plasmon resonance at 420 nm, and the presence of Hg2+ decreased that signature and caused it to blue-shift. This is attributed to hydrogen bonding of Hg2+ to the stabilizer on AgNPs surface,41,42 which causes the removal of the stabilizer because gingerols are phenolic compounds with the ability to form complexes with heavy metals through the interaction between hydroxyl groups and the heavy metal.43 In the context of this study, silver nanoparticles were capped by gingerol molecules containing hydroxyl groups. The addition of Hg2+ to the solution of gingerol-capped silver nanoparticles leads to an electro-ionic interaction42 between the surface of the nanoparticles and Hg2+, followed by redox reactions on the AgNP surface. The redox reaction is spontaneous with the standard reduction potential of silver (Ag+/Ag (0.80 V)) being lower than that of mercury (Hg2+/Hg (0.85 V)).44 The result is an Ag–Hg amalgam,42,45 when Hg2+ react with silver nanoparticles (AgNPs), the size of the AgNPs increases, resulting in the formation of Ag+ ions. Hg0, on the other hand, forms Hg–Ag colloidal amalgams with the AgNPs. This Hg–Ag amalgam then coats the surface of the AgNPs, damping the surface plasmon and causing a noticeable change in the color of the colloidal solution. Consequently, the colloidal solution loses its original color and becomes colorless. These observation changes indicate that all the available Ag0 in the colloidal suspension has been completely oxidized by the added mercury. In addition, the deposition of Hg0 on the surface of Ag+ drove the blue-shift and damped of the surface plasmon resonance (and thus the colour change of the Gin-AgNPs solution from yellow to colorless, Fig. 2). Parameters such as Gin-AgNPs solution volume, pH, and reaction time, were examined next in order to optimize this visual detection of the presence of Hg2+.
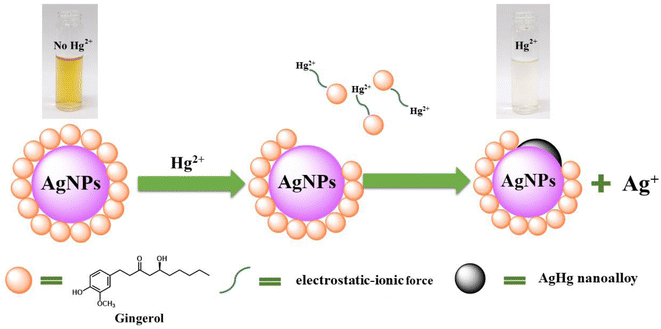 |
| Fig. 2 Schematic representation of the colorimetric sensing of Hg2+ by Gin-AgNPs. The proposed mechanism is an interaction between the ions and the nanoparticle (NP) surface, resulting in both NP stabilizer removal and Hg–Ag amalgam formation at the NP surface. The latter damps the surface plasmon resonance and changes the colour of the solution. | |
3.3 Effect of pH value
The potential of Gin-AgNP solutions for Hg2+ sensing was investigated at different pH values. Buffer solutions from pH 3 to 11 were employed; acidic (HOAc buffers, pH 3.0, 4.0, 5.0 and 6.0), neutral (phosphate buffer, pH 7.0), and basic (Tris–HCl buffers (pH 8.0, 9.0, 10.0 and 11.0)) as depicted in Fig. 3(a) and (b). For each pH, the observed changes to the SPR absorption band at 420 nm was estimated as A0 − A, where ‘A0’ was the absorbance of the neat Gin-AgNPs solution and ‘A’ was the Gin-AgNPs solution absorbance in the presence of 50 μM Hg2+. The A0 − A value was highest at pH 8. The bioactive stabilizer on Gin-AgNPs could be potentially reduced by Hg2+ in this alkaline condition,43 maximizing the A0 − A value. Moreover, the pH 8 buffer condition optimized dispersion of the Gin-AgNPs, as shown in Fig. S6(b)† compared to neat Gin-AgNPs (Fig. S6(a)†) and Gin-AgNPs in the presence of Hg2+ (Fig. S6(c)†). Therefore, pH 8.0 was selected as optimal for this sensing system.
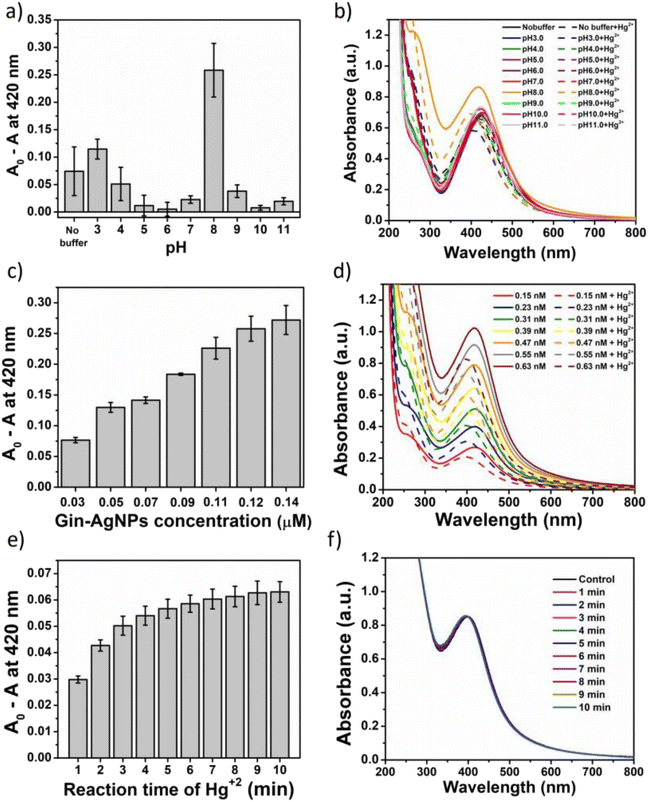 |
| Fig. 3 (a) Relative change in the absorbance of Gin-AgNPs in the presence of Hg2+ (50 μM) and 50 mM of buffer solution at different pHs from 3.0 to 11.0, (b) the corresponding UV-vis absorption spectra for the data in panel (a), (c) relative change in the absorbance of Gin-AgNPs as a function of Gin-AgNPs volume in the presence of Hg2+ (50 μM) and 50 mM of Tris–HCl buffer solution at pH 8.0, (d) the corresponding UV-vis absorption spectra for the data in panel (c), (e) relative change of the absorbance of Gin-AgNPs as a function of time in the presence of Hg2+ (50 μM) and 50 mM of Tris–HCl buffer solution at pH 8.0, (f) the corresponding UV-vis absorption spectra for the data in panel (e). | |
3.4 Effect of Gin-AgNPs concentration
The effect of Gin-AgNPs concentrations for optical sensing experiments was evaluated as shown in Fig. 3(c) and (d). In this experiment, various concentrations of Gin-AgNPs (0.03, 0.05, 0.07, 0.09, 0.11, 0.12, and 0.14 μM). The experiments were conducted in the presence of Hg2+ (50 μM) and Tris–HCl buffer solution (50 mM) with a pH of 8.0. Absorption spectra of Gin-AgNPs at various concentrations in the absence and presence of Hg2+ are depicted in Fig. 3(d). The change in absorbance ratio increased when increasing the volume of Gin-AgNPs. However, a similar absorbance ratio could be seen when 0.12 μM (350 μL) and 0.14 μM (400 μL) of Gin-AgNPs were used. Thus, 0.12 μM was selected as the optimum concentration of Gin-AgNPs for further experiments.
3.5 Effect of reaction time
In this experiment, the effect of reaction time was investigated using 0.12 μM (350 μL) of Gin-AgNPs, 50 μM of Hg2+ and 50 mM of Tris–HCl buffers (pH 8.0), respectively. Various incubation times between 1 and 10 min were monitored and recorded as shown in Fig. 3(e) and (f). The absorbance change ratio (A0 − A) where ‘A0’ was the absorbance of the Gin-AgNPs solution with Hg2+ at pH 8 and ‘A’ was absorbance of same solution measure at the period of time (1–10 minutes) as depicted in Fig. 3(e). After mixing Hg2+ with the Gin-AgNPs, the color of the solution changed slowly from yellow to colorless according with the increasing time, and then the absorbance change ratio for reaction time was stable after 8 min. Thus, a reaction time of 8 min was selected and applied in all further experiments.
3.6 Selectivity studies
The selectivity of Gin-AgNPs for reaction with Hg2+ relative to other transition, alkali, and alkaline earth metal ions (Na+, K+, Ca2+, Mg2+, Ba2+, Pb2+, Mn2+, Ni2+, Cu2+, Zn2+, Cd2+ and Co2+) was investigated by UV-vis spectroscopy and the naked eye (Fig. 4(e)). The results showed that the Gin-AgNPs SPR signal changed significantly after the addition of Hg2+ compared with the other metal ions (Fig. 4(a) and (b), respectively). To assess the selectivity studies of Gin-AgNPs for Hg2+ sensing, 250 μM of the other metal ions were added to the Gin-AgNPs solution to assess their level of interference in the sensing process. There were no remarkable changes observed upon the addition of 250 μM of the other cations together with Hg2+ as shown in Fig. 4(c) and (d). The A0 − A values of the Gin-AgNPs in the presence of Hg2+ alone and with the presence of other metal ions did not show any changes, except for Pb2+ that showed a slight decrease. Moreover, the color of the Gin-AgNPs solution noticeably changed from yellow to colorless when Hg2+ was added, but colour changes were imperceptible for the addition of the other metal ions.
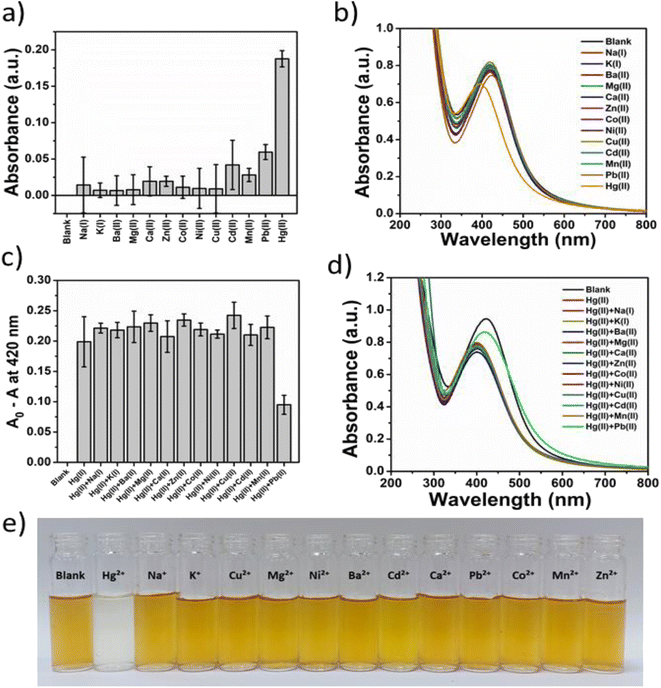 |
| Fig. 4 (a) Relative change in the absorbance of 0.12 μM of Gin-AgNPs in the presence of Hg2+ and various metal ions (200 μM), (b) the UV-vis absorption spectra for the data in panel (a), (c) relative change in the absorbance of 0.12 nM of Gin-AgNPs in the presence of Hg2+ together with various metal ions (200 μM), (d) the related UV-vis absorption spectra for the data in panel (c), (e) the image showing the changes in the color of 0.12 μM of Gin-AgNPs with the presence of 200 μM of various metal ions and Hg2+. | |
3.7 Analytical performance of the optical sensor for the detection of Hg2+
The quantitative detection of Hg2+ with Gin-AgNPs was examined next. The change in absorbance values (A0 − A) of Gin-AgNPs at 420 nm with the different concentrations of Hg2+ was performed under the previously optimized conditions. As depicted in Fig. 5(a) and (b), the change of absorbance values increased following the increasing concentrations of Hg2+. The optical sensor using Gin-AgNPs showed a linear response over the concentration range of 0–160 μM of Hg2+ (Fig. 5(a)). A linear equation of (A0 − A) = (0.1302 ± 0.0067)log
Hg2+ − (0.0039 ± 0.0113) was obtained with a correlation coefficient (R2) of 0.9921. The achieved limit of detection (LOD) and limit of quantitation (LOQ) were 1.46 μM and 3.04 μM, respectively (the concentration of Hg2+ required to give an absorbance change ratio (A − A0) at 420 nm equal to 3 standard deviations (3σ; 10 replicate measurements of the blank sample (A0)) and 10 standard deviations (10σ) of A0 for LOQ).
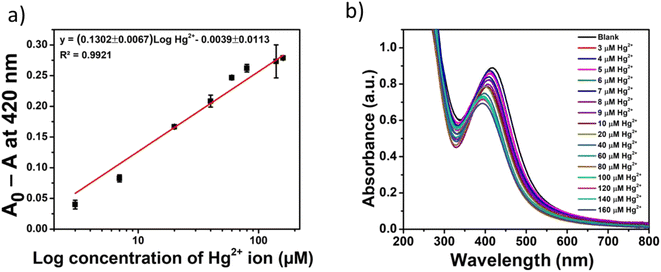 |
| Fig. 5 (a) The absorbance ratio (A0 − A) at 420 nm as a function of Hg2+ concentrations, (b) the UV visible absorption spectra of Gin-AgNPs (0.55 nM) with different concentrations of Hg2+ ranging from 0 to 160 μM in buffer solution (pH 8.0). | |
3.8 Colorimetric sensing of Hg2+ based on naked eye detection and the determination of Hg2+ in real water samples
Naked eye detection of Hg2+ at various concentrations ranging from 0–200 μM using Gin-AgNPs is examined in this section (Fig. 6). As discussed, the color of the solution changed from yellow to colorless with increasing concentration of Hg2+. A complete color change occurred at the Hg2+ concentration of about 200 μM. This may be a useful experiment, therefore, for rapid screening for the presence of Hg2+ water samples in field studies. The potential of Gin-AgNPs to detect Hg2+ in tap water was investigated by UV-vis spectroscopy by adding 25 μM and 50 μM of standard HgCl2 to un-spiked tap water. The concentration of Hg2+ present was then calculated from the standard addition curve as shown in Fig. S7.† The results shown in Table 1 indicate the good agreement between added and determined values with 98.78–107.54% of recovery and 2.14–2.32% of relative standard deviation (RSD).
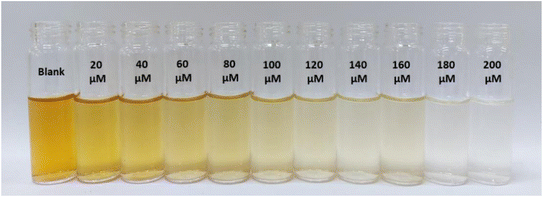 |
| Fig. 6 Colorimetric response of Gin-AgNPs to different concentrations of Hg2+ (from left to right: 0, 20, 40, 60, 80, 100, 120, 140, 160, 180, and 200 μM where blank refers to 0 μM). | |
Table 1 Determination of Hg2+ concentrations in a real water sample
Water sample |
Added Hg2+ (μM) |
Proposed method (n = 3) |
Found Hg2+ (μM) |
% recovery ± SD |
RSD (%) |
Tap water |
25 |
24.70 ± 0.57 |
98.78 ± 2.95 |
2.32 |
50 |
53.76 ± 1.15 |
107.54 ± 2.30 |
2.14 |
3.9 A machine learning and image processing procedure for the detection of Hg2+
To test the accuracy of the developed algorithm, the data sets of solution images were separated into 3 cases. The first data set was the solutions with the same concentrations as the database and the properties of their images such as ratio, brightness and position were set the same as the database. The second data set was the solutions with the same concentrations as the database, but their images had different ratios, brightness and position, and only one image was taken for each group. The last data set was the solutions with different concentrations of 70 μM, 110 μM, 150 μM and 190 μM. Their image properties were the same as in the database. During the implementation process of our technique for these data sets, the application was developed using the MATLAB programming language and a graphic user interface (GUI) with the purpose of being user-friendly, simple and comfortable. An example of the GUI is depicted in Fig. 7.
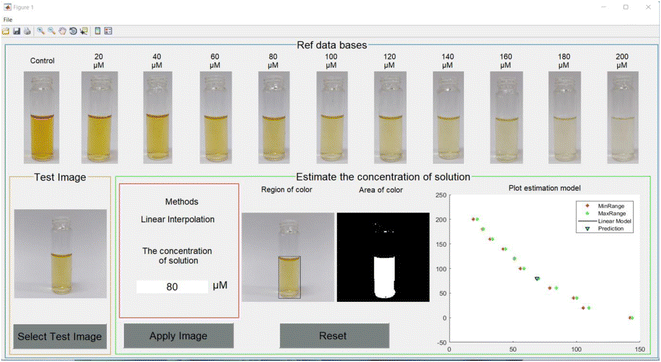 |
| Fig. 7 Image processing application with GUI interface. | |
The numerical results for the first data set are shown in Table S1.† The results estimated using the application provide very precise results. The minimum and maximum errors were 0% and 1.7%, respectively. The maximum error appeared at the concentration of 20 μM. For the second data set, the results are shown in Table S2.† The minimum and maximum errors were 0.15% for 20 μM concentration and 12.90% for 200 μM concentration, respectively, excluding the case of 0 μM concentration. The error increased along with the increasing concentration. For the last data set, the results are shown in Table S3.† The minimum and maximum errors are 3.66% and 14.66%, respectively. However, these error levels are still acceptable. From the experimental results, the application of machine learning using image processing could help to estimate the concentration of Hg2+ in the Gin-AgNPs solution. The accuracy of the prediction was acceptable, with an error rate of less than 13% for the solution whose concentration matched the given database. For the solution whose concentration did not match the given database, the accuracy of prediction was increased but the error rate was still less than 15%. The factors that played an important role in the accuracy of our algorithm might be the position of the test tube, brightness and background setting. Machine learning showed some ability and usefulness in the prediction of the concentration of mercury in the Gin-AgNPs solution instead of the practical measurements in the laboratory. However, practical measurement was still required for the most reliable and precise estimation.
3.10 Application of Gin-AgNPs for the determination of Hg2+ by a machine learning program
The potential of Gin-AgNPs for the detection of Hg2+ by the machine learning program was performed using Gin-AgNPs solutions with different concentrations of Hg2+ being 50, 90, 130 and 170 μM. Then the images of sample solutions were taken and the machine learning program was used to determine the quantity of Hg2+ as shown in Table 2.
Table 2 Determination of Hg2+ concentrations in a real water sample
Hg2+ added (μM) |
Proposed method (n = 3) |
Hg2+ found (μM) |
RSD (%) |
50 |
50.56 ± 0.32 |
0.62 |
90 |
92.10 ± 1.40 |
1.53 |
130 |
131.14 ± 0.21 |
0.16 |
170 |
170.66 ± 1.14 |
0.67 |
3.11 Comparison with other methods for Hg2+ determination
Mercury is a heavy metal that is toxic to the environment and the human body. It exists in an inorganic form which includes metallic mercury, mercury vapor (Hg0) and mercurous (Hg22+) or mercuric (Hg2+) salts.46,47 Mercuric ions are the most stable inorganic form and they commonly contaminate water because of their solubility in water. Mercury causes many negative effects and diseases in the human body including neurological, nephrological, immunological, cardiac, motor, reproductive and genetic effects.45 Minamata disease, a neurological disorder in people who consume mercury at a high level, is one of the diseases caused by mercury.48,49 Therefore, the detection of Hg2+ in water is important for the environment and to avoid mercury damage to humans. In 1976, the World Health Organization (WHO) reported that drinking water should contain less than 0.4 μg of Hg2+.50 In Thailand, the laws of mercury control in water recommend that drinking water must have less than 0.002 mg L−1 Hg2+. Wastewater that drains from a factory must have mercury at less than 0.005 mg L−1 Hg2+, except for zinc smelting plants which must have wastewater with less than 0.002 mg L−1 of Hg2+. Water sources in nature should have total Hg2+ content of less than 0.002 mg L−1.51
Therefore, many detection methods for Hg2+ in water at low concentrations have been developed, as summarized in Table S4,† including electrochemistry, fluorescence spectroscopy, and colorimetry. Among these techniques, the colorimetric method is exceptional because of its simplicity, ease of use, low-cost, on-site capability, and high selectivity. In addition, our colorimetric sensor shows a change of color that can be observed by the naked eye and thus has great potential for field-based detection of Hg2+.
3.12 Antimicrobial susceptibility of Gin-AgNPs
Antimicrobial susceptibility to Gin-AgNPs was investigated for S. aureus and E. coli by diffusion assay. The diffusion tests on agar plates of Gin-AgNPs are reported in Fig. 8 against S. aureus (Fig. 8(a)) and E. coli (Fig. 8(b)). In addition, the zones of inhibition in millimeters of Gin-AgNPs are exhibited in Table 3. The results show that Gin-AgNPs could inhibit the growth of both bacteria. The inhibition zones of Gin-AgNPs to S. aureus and E. coli were 9.33 ± 0.58 mm and 10.97 ± 0.40 mm, respectively. Gin-AgNPs were more inhibitive for E. coli than S. aureus. These results indicate that both S. aureus and E. coli are susceptible to Gin-AgNPs. Our results were similar to a previous study, in which synthesized ginger extract-AgNPs exhibited high potential antimicrobial activity against several strains of bacteria and fungi.52
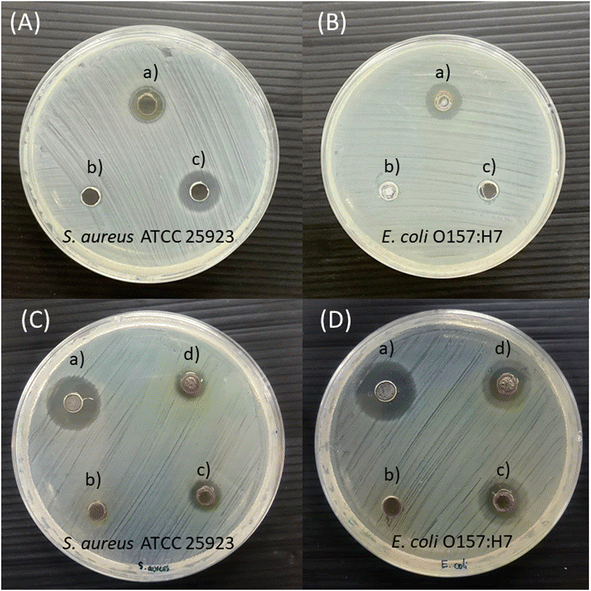 |
| Fig. 8 Antimicrobial susceptibility of Gin-AgNPs against S. aureus and E. coli. (a) Gin-AgNPs, (b) deionized water, and (c) 16 μg mL−1 of gentamicin or polymyxin b were tested against S. aureus ATCC 25923 (A), E. coli O157:H7 (B) and antimicrobial susceptibility of Gin-AgNPs against S. aureus and E. coli, (a) 16 μg mL−1 of gentamicin or polymyxin b, (b) 0 μg mL−1 Gin-AgNPs (c) 15 μg mL−1 Gin-AgNPs hydrogel, and (d) 25 μg mL−1 Gin-AgNPs hydrogel were tested against S. aureus ATCC 25923 (C), E. coli O157:H7 (D). | |
Table 3 Inhibition zone of Gin-AgNPs against Gram-positive and Gram-negative bacteria
Agents |
Inhibition zone (mm) |
Staphylococcus aureus |
Escherichia coli |
Gin-AgNPs |
9.33 ± 0.58 |
10.97 ± 0.40 |
Gentamicin |
12.00 ± 1.00 |
— |
Polymyxin b |
— |
6.03 ± 0.38 |
Deionized water |
0.00 |
0.00 |
3.13 Antimicrobial assay of Gin-AgNPs
After antimicrobial screening to evaluate the antimicrobial susceptibility of Gin-AgNPs, the antimicrobial activity of Gin-AgNPs was tested by the microdilution method. MIC and MBC values were measured to determine the lowest concentration of Gin-AgNPs for inhibition and killing bacteria. MIC and MBC of Gin-AgNPs against Gram-positive and Gram-negative bacteria are exhibited in Table 4. The MIC value and the MBC value of Gin-AgNPs against S. aureus ATCC 25923 were 0.4 μg mL−1 and 1.5 μg mL−1, whereas the MIC value and the MBC values toward E. coli O157:H7 were 0.2 μg mL−1 and 1.5 μg mL−1, respectively. The MIC value and the MBC values of gentamicin against S. aureus ATCC 25923 were 0.5 μg mL−1 and 1 μg mL−1 whereas the MIC value and the MBC values toward E. coli O157:H7 were ≤0.25 μg mL−1. Gin-AgNPs could inhibit E. coli O157:H7 at lower concentrations when compared with S. aureus ATCC 25923. However, the killing activity of Gin-AgNPs against both bacteria was equal at 1.5 μg mL−1. In addition, a comparison of the antimicrobial activity of Gin-AgNPs and gentamicin indicated that the MIC and MBC of the agents were similar against S. aureus ATCC 25923. This indicates that the antimicrobial activity of Gin-AgNPs and gentamicin against S. aureus ATCC 25923 are no different and thus Gin-AgNPs have high potential antimicrobial activity.
Table 4 MIC and MBC of Gin-AgNPs against Gram-positive and Gram-negative bacteriaa
Agents |
Gram-positive bacteria |
Gram-negative bacteria |
Staphylococcus aureus |
Escherichia coli |
MIC (μg mL−1) |
MBC (μg mL−1) |
MIC (μg mL−1) |
MBC (μg mL−1) |
MIC, minimum inhibitory concentration; MBC, minimum bactericidal concentration. Gentamicin was used as a standard antibacterial agent. MIC represents the lowest concentration of agent that inhibited bacterial growth >99%; MBC represents 100% inhibition. |
Gin-AgNPs |
0.4 |
1.5 |
0.2 |
1.5 |
Gentamicin |
0.5 |
1.0 |
≤0.25 |
≤0.25 |
3.14 Antimicrobial tests of Gin-AgNPs hydrogel
Gin-AgNPs were incorporated into a hydrogel and evaluated for antibacterial activity. The Gin-AgNPs hydrogel exhibited antimicrobial activity in all bacterial tests as indicated by the inhibition zone described in Table 5. The concentration of Gin-AgNPs at 15 μg mL−1 in the hydrogel showed inhibition zones at 8.92 ± 0.45 mm and 8.95 ± 0.41 against S. aureus and E. coli, respectively. In the same way, the concentration of Gin-AgNPs at 25 μg mL−1 in hydrogel exhibited inhibition zones at 10.08 ± 0.28 mm and 10.67 ± 0.24 mm against S. aureus and E. coli, respectively. These observations suggest an enhanced antimicrobial activity for the hydrogel when Gin-AgNPs concentrations are increased. The Gin-AgNPs hydrogel showed antimicrobial activity against both S. aureus, the representative Gram-positive bacterium, and E. coli; the representative Gram-negative bacterium.
Table 5 Inhibition zones of Gin-AgNP hydrogels against Gram-positive and Gram-negative bacteria
Agents |
Inhibition zone (mm) |
Staphylococcus aureus |
Escherichia coli |
0 μg mL−1 Gin-AgNPs hydrogel |
0.00 |
0.00 |
15 μg mL−1 Gin-AgNPs hydrogel |
8.92 ± 0.45 |
8.95 ± 0.41 |
25 μg mL−1 Gin-AgNPs hydrogel |
10.08 ± 0.28 |
10.67 ± 0.24 |
16 μg mL−1 gentamicin |
17.80 ± 0.26 |
18.65 ± 0.88 |
4. Conclusions
In this work, ginger-stabilized silver nanoparticles (Gin-AgNPs) were successfully synthesized with a green synthesis method and used in the detection of Hg2+ based on the interaction of Hg2+ with silver nanoparticles. The color changes from yellow to colorless are obvious to the naked eye for environmental pollutant-relevant levels of Hg2+. Quantification of Hg2+ over a linear range of 3–160 μM with a limit of detection (LOD) and limit of quantitation (LOQ) of 1.46 μM and 3.04 μM, respectively, were proved by UV-vis spectroscopy. Furthermore, the synthesized Gin-AgNPs were highly selective to Hg2+ compared with other metal ions. The proposed colorimetric sensor was applied Hg2+ detection in real water samples using a standard addition method with satisfactory results. Furthermore, a machine learning-based image processing program was developed for determining Hg2+ concentrations, again with satisfactory results. Finally, an additional application of the Gin-AgNPs, antimicrobial activity towards both Gram-positive and Gram-negative bacteria, was demonstrated. As such these green-synthesized nanomaterials may find applications both in environmental sensing and in biomedicine in the future.
Author contributions
Conceptualization, S. K., K. P., R. K. and Y. K.; methodology, S. K., K. P., R. K., Y. K.; validation, S. K.; formal analysis, S. K., K. P., R. K. and Y. K.; investigation, K. P., R. K., Y. K., R. P., C. T., A. S., S. T., K. L., S. K., and N. K.; writing – original draft preparation, S. K. and K. P.; writing – review and editing, visualization, supervision, project administration, funding acquisition, S. K. All authors have read and agreed to the published version of the manuscript.
Conflicts of interest
We wish to confirm that there are no known conflicts of interest associated with this publication.
Acknowledgements
K. P. and all authors sincerely thank the financial support of the Materials Chemistry Research Center (MCRC), Department of Chemistry and Center of Excellence for Innovation in Chemistry (PERCH-CIC), Faculty of Science (FoS), Khon Kaen University (KKU), Khon Kaen, Thailand. Thanks for the laboratory support from the Faculty of Science, Khon Kaen University. We thank Miss Kunthaya Ratchaphonsaenwong for TEM measurements at the Research Instrument Center Khon Kaen University.
References
- K. Farhadi, M. Forough, R. Molaei, S. Hajizadeh and A. Rafipour, Sens. Actuators, B, 2012, 161, 880–885 CrossRef CAS
. - J. Švehla, R. Žídek, T. Ružovič, K. Svoboda and J. Kratzer, Spectrochim. Acta, Part B, 2019, 156, 51–58 CrossRef
. - M. L. Astolfi, C. Protano, E. Marconi, D. Piamonti, L. Massimi, M. Brunori, M. Vitali and S. Canepari, Microchem. J., 2019, 150, 104186 CrossRef CAS
. - R. Gras, J. Luong and R. A. Shellie, ACS Earth Space Chem., 2018, 2, 471–478 CrossRef CAS
. - I. S. Denmark, E. Begu, Z. Arslan, F. X. Han, J. M. Seiter-Moser and E. M. Pierce, Anal. Chim. Acta, 2018, 1041, 68–77 CrossRef CAS PubMed
. - Z. H. Fernández, L. A. V. Rojas, A. M. Álvarez, J. R. E. Álvarez, J. A. dos Santos Júnior, I. P. González, M. R. González, N. A. Macias, D. L. Sánchez and D. H. Torres, Food Control, 2015, 48, 37–42 CrossRef
. - B. Janani, A. Syed, A. M. Thomas, A. H. Bahkali, A. M. Elgorban, L. L. Raju and S. S. Khan, Optik, 2020, 204, 164160 CrossRef CAS
. - O. Linhart, A. Kolorosová-Mrázová, J. Kratzer, J. Hraníček and V. Červený, Anal. Lett., 2019, 52, 613–632 CrossRef CAS
. - A. Elgamouz, I. Shehadi, A. Assal, A. Bihi and A.-N. Kawde, J. Electroanal. Chem., 2021, 895, 115443 CrossRef CAS
. - H. Xiao-wei, Z. Xiao-bo, S. Ji-yong, L. Zhi-hua and Z. Jie-wen, Trends Food Sci. Technol., 2018, 81, 90–107 CrossRef CAS
. - S. Raj and D. R. Shankaran, Sens. Actuators, B, 2016, 226, 318–325 CrossRef CAS
. - W. Liu, F. Ding, Y. Wang, L. Mao, R. Liang, P. Zou, X. Wang, Q. Zhao and H. Rao, Sens. Actuators, B, 2018, 265, 310–317 CrossRef CAS
. - M. Valdez, S. K. Gupta, K. Lozano and Y. Mao, Sens. Actuators, B, 2019, 297, 126734 CrossRef CAS
. - W. Chansuvarn, T. Tuntulani and A. Imyim, TrAC, Trends Anal. Chem., 2015, 65, 83–96 CrossRef CAS
. - S. Balasurya, A. Syed, A. M. Thomas, N. Marraiki, A. M. Elgorban, L. L. Raju, A. Das and S. S. Khan, Spectrochim. Acta, Part A, 2020, 228, 117712 CrossRef CAS PubMed
. - V. Chakrapani, K. B. Ayaz Ahmed, V. V. Kumar, V. Ganapathy, S. P. Anthony and V. Anbazhagan, RSC Adv., 2014, 4, 33215–33221 RSC
. - G.-L. Wang, L.-Y. Jin, X.-M. Wu, Y.-M. Dong and Z.-J. Li, Anal. Chim. Acta, 2015, 871, 1–8 CrossRef CAS PubMed
. - P. Verma and S. K. Maheshwari, Int. J. Nano Dimens., 2019, 10, 18–36 CAS
. - S. H. Lee and B.-H. Jun, Int. J. Mol. Sci., 2019, 20(4) DOI:10.3390/ijms20040865
. - L. Qi, Y. Shang and F. Wu, Microchim. Acta, 2012, 178, 221–227 CrossRef CAS
. - J. Y. Cheon and W. H. Park, Int. J. Mol. Sci., 2016, 17(12) DOI:10.3390/ijms17122006
. - F. Divsar, K. Habibzadeh, S. Shariati and M. Shahriarinour, Anal. Methods, 2015, 7, 4568–4576 RSC
. - M. L. Firdaus, I. Fitriani, S. Wyantuti, Y. W. Hartati, R. Khaydarov, J. A. Mcalister, H. Obata and T. Gamo, Anal. Sci., 2017, 33, 831–837 CrossRef CAS PubMed
. - A. Aminu and S. A. Oladepo, Arabian J. Sci. Eng., 2021, 46, 5477–5487 CrossRef CAS
. - H. Xu, X. Shi, H. Ma, Y. Lv, L. Zhang and Z. Mao, Appl. Surf. Sci., 2011, 257, 6799–6803 CrossRef CAS
. - M. Rafique, I. Sadaf, M. S. Rafique and M. B. Tahir, Artif. Cells, Nanomed., Biotechnol., 2017, 45, 1272–1291 CrossRef CAS PubMed
. - D. Garibo, H. A. Borbón-Nuñez, J. N. D. de León, E. García Mendoza, I. Estrada, Y. Toledano-Magaña, H. Tiznado, M. Ovalle-Marroquin, A. G. Soto-Ramos, A. Blanco, J. A. Rodríguez, O. A. Romo, L. A. Chávez-Almazán and A. Susarrey-Arce, Sci. Rep., 2020, 10, 12805 CrossRef CAS PubMed
. - Y. Han, C.-W. Song, W. Koh, G. Yon, Y. Kim, S. Ryu, H. Kwon and K. Lee, Phytother. Res., 2013, 27 DOI:10.1002/ptr.4847
. - M. H. Shahrajabian, Acta Agric. Scand., 2019, 69, 546–556 CAS
. - M. H. Shahrajabian, S. U. N. Wenli and Q. Cheng, Not. Sci. Biol., 2019, 11, 309–319 CrossRef CAS
. - M. M. Al-Sanea, N. Abelyan, M. A. Abdelgawad, A. Musa, M. M. Ghoneim, T. Al-Warhi, N. Aljaeed, O. J. Alotaibi, T. S. Alnusaire, S. F. Abdelwahab, A. Helmy, U. R. Abdelmohsen and K. A. Youssif, Antibiotics, 2021, 10(7) DOI:10.3390/antibiotics10070824
. - P. Velmurugan, K. Anbalagan, M. Manosathyadevan, K.-J. Lee, M. Cho, S.-M. Lee, J.-H. Park, S.-G. Oh, K.-S. Bang and B.-T. Oh, Bioprocess Biosyst. Eng., 2014, 37 DOI:10.1007/s00449-014-1169-6
. - M. Ahmed, S. al-amri and A. Mohammed noori jassim, Plant Arch., 2020, 20, 5505–5515 Search PubMed
. - D. Paramelle, A. Sadovoy, S. Gorelik, P. Free, J. Hobley and D. G. Fernig, Analyst, 2014, 139, 4855–4861 RSC
. - K. Kalishwaralal, S. BarathManiKanth, S. R. K. Pandian, V. Deepak and S. Gurunathan, Colloids Surf., B, 2010, 79, 340–344 CrossRef CAS PubMed
. - P. Wimuktiwan, J. Shiowatana and A. Siripinyanond, J. Anal. At. Spectrom., 2015, 30, 245–253 RSC
. - M. Elshikh, S. Ahmed, S. Funston, P. Dunlop, M. McGaw, R. Marchant and I. M. Banat, Biotechnol. Lett., 2016, 38, 1015–1019 CrossRef CAS PubMed
. - M. A. Majeed Khan, S. Kumar, M. Ahamed, S. A. Alrokayan and M. S. AlSalhi, Nanoscale Res. Lett., 2011, 6, 434 CrossRef PubMed
. - X. Zhao, H. Zhu, J. Chen and Q. Ao, J. Food Process. Preserv., 2015, 39, 2017–2026 CrossRef CAS
. - H. Kaur, H. Kaur and A. Sharma, Mater. Today: Proc., 2021, 37, 3574–3576 CAS
. - D. Mandal, M. E. Bolander, D. Mukhopadhyay, G. Sarkar and P. Mukherjee, Appl. Microbiol. Biotechnol., 2006, 69, 485–492 CrossRef CAS PubMed
. - A. Devadiga, K. Vidya Shetty and M. B. Saidutta, Mater. Lett., 2017, 207, 66–71 CrossRef CAS
. - D. Karthiga and S. P. Anthony, RSC Adv., 2013, 3, 16765 RSC
. - A. Saenchoopa, W. Boonta, C. Talodthaisong, O. Srichaiyapol, R. Patramanon and S. Kulchat, Spectrochim. Acta, Part A, 2021, 251, 119433 CrossRef CAS PubMed
. - A. Jeevika and D. R. Shankaran, Mater. Res. Bull., 2016, 83, 48–55 CrossRef CAS
. - S. S. Ravi, L. R. Christena, N. SaiSubramanian and S. P. Anthony, Analyst, 2013, 138, 4370–4377 RSC
. - R. A. Bernhoft, J. Environ. Public Health, 2011, 2012, 460508 Search PubMed
. - F. Zahir, S. J. Rizwi, S. K. Haq and R. H. Khan, Environ. Toxicol. Pharmacol., 2005, 20, 351–360 CrossRef CAS PubMed
. - S. Ekino, M. Susa, T. Ninomiya, K. Imamura and T. Kitamura, J. Neurol. Sci., 2007, 262, 131–144 CrossRef CAS PubMed
. - B.-J. Ye, B.-G. Kim, M.-J. Jeon, S.-Y. Kim, H.-C. Kim, T.-W. Jang, H.-J. Chae, W.-J. Choi, M.-N. Ha and Y.-S. Hong, Ann. Occup. Environ. Med., 2016, 28, 5 CrossRef PubMed
. - E. A. by E. Ltd, Water Management in Thailand, Enviliance ASIA, https://enviliance.com/regions/southeast-asia/th/th-water, accessed March 9, 2023 Search PubMed.
- N. AlMasoud, T. S. Alomar, M. A. Awad, M. F. El-Tohamy and D. A. Soliman, Green Chem. Lett. Rev., 2020, 13, 316–327 CrossRef CAS
.
|
This journal is © The Royal Society of Chemistry 2023 |
Click here to see how this site uses Cookies. View our privacy policy here.