Design of antimicrobial peptides containing non-proteinogenic amino acids using multi-objective Bayesian optimisation†
Received
19th May 2023
, Accepted 28th July 2023
First published on 1st August 2023
Abstract
Antimicrobial peptides (AMPs) have attracted attention as next-generation antimicrobial drugs. Designing AMPs while considering multiple properties, such as antimicrobial activities and toxicity, requires numerous trials and errors by chemists. In this study, we propose MODAN, a machine learning-assisted AMP design framework based on multi-objective Bayesian optimisation. The primary advantage of MODAN is its ability to handle various non-proteinogenic amino acids, which have recently shown the potential of activity enhancement, and this flexibility has not been achieved by previous studies. In addition, multi-objective Bayesian optimisation enables simultaneous improvement of antimicrobial activity and toxicity. We have succeeded in designing peptides that have potent antimicrobial and low haemolytic activities within two rounds of MODAN recommendation and experimentation, based on a strategy that chemists do not usually consider.
1. Introduction
Antimicrobial peptides (AMPs) have attracted attention as a next-generation drug modality because they can reduce the emergence of resistant bacteria.1,2 In general, amphiphilicity, which involves hydrophobic and cationic amino acids, and α-helical structural stability are crucial factors to consider when designing AMPs that can effectively interact with bacterial cell membranes.3–5 To improve these factors, the introduction of non-proteinogenic amino acids (NPAAs), containing α,α-disubstituted NPAAs (commonly referred to as α,α-disubstituted α-amino acids), rather than natural amino acids (NAAs) and a side-chain stapling strategy have been considered.6–13 An α,α-disubstituted NPAA and the side-chain stapling strategy can improve α-helical structural stability, which is one of the important factors of AMPs, because an α,α-disubstituted NPAA and the strategy restrict the movement within a peptide.
Designing AMPs still relies heavily on the intuition and experience of chemists.14 Chemists have designed AMPs to simultaneously satisfy multiple properties, such as antimicrobial activities and toxicity, which is a challenging task. Recently, to tackle such multi-objective optimisation problems, Bayesian optimisation has been widely used in various fields, such as powder metallurgy,15 epitaxial titanium nitride thin films,16 fluorescent proteins,17 and peptide substrates for enzymes.18 Bayesian optimisation is a method that uses machine learning to efficiently search for the best solution by repeating cycles of recommending promising new candidates from existing data and validating them.19–21 Regarding AMP designs, several studies have been reported using Bayesian optimisation combined with machine learning (ML)-based evaluations rather than experimentation.22,23 However, the optimisation potential of AMPs using Bayesian optimisation combined with experimental feedback has not been investigated.
In addition, efficient methods for designing AMPs to handle NPAAs, containing α,α-disubstituted NPAAs, and side-chain stapling have not been developed. For designing AMPs using only NAAs, many ML-based methods have been developed.24–28 Several ML-based design methods to incorporate NPAAs have also been proposed, but with various limitations.29–33 These methods compute input features using prepared descriptors such as z-scale,34 T-scale,35 and NNAA-index,36 which require laborious additional efforts when adding an unregistered amino acid. The z-scale requires experimental data of the amino acid. The T-scale and the NNAA-index require the calculations of physicochemical properties using specific software packages, such as ChemOffice 8.0,37 E-dragon,38 and Molecular Operating Environment (MOE),39 and analyses such as principal component analysis and factor analysis, respectively. Furthermore, the previous ML-based methods cannot handle the side-chain stapling strategy because they assume the calculation of the properties for each individual amino acid and do not expect a linking between two amino acids.
In this study, we developed a multi-objective Bayesian framework consisting of computational recommendations and experimental feedback for the design of AMPs that could consider various NPAAs containing α,α-disubstituted NPAAs and side-chain stapling, namely MODAN. To address the limitations of the previous ML-based methods, MODAN employed molecular fingerprints calculated from 2D peptide representations as input features to predict properties. For handling new amino acids and the side-chain stapling, the preparation of their simplified molecular input line entry system (SMILES)40 strings and the corresponding codes are only needed. Using this information, MODAN can assemble the SMILES string of a given peptide sequence and calculate its molecular fingerprints. The potential of this method was demonstrated through the optimisation of an AMP derived from magainin 2 (ref. 41) by considering six antimicrobial activities and toxicity. The source code and dataset used in this study are available at https://github.com/ycu-iil/MODAN.‡
2. Materials and methods
The optimisation round of MODAN consists of three processes: construction of surrogate models, recommendation of promising AMP candidates considering NPAAs, and experimentation (Fig. 1). By repeating these three processes, MODAN optimises an AMP based on the Bayesian optimisation framework.
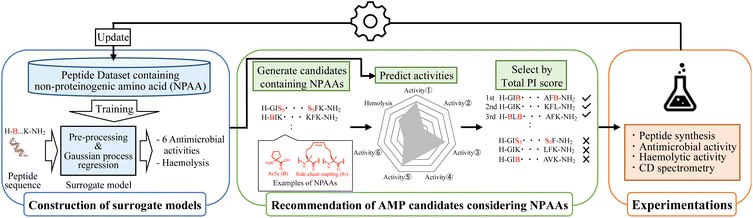 |
| Fig. 1 Outline of semi-automated AMP design based on MODAN. | |
2.1 Construction of surrogate models
Seven surrogate models for the six antimicrobial activities and haemolytic activity as toxicity were trained using the Gaussian process19,20 implemented in the PHYSBO package.42 As target bacteria, Escherichia coli NBRC 3972, E. coli DH5α (DH5α), Pseudomonas aeruginosa, multiple-drug resistant P. aeruginosa (MDRP), Staphylococcus aureus, and S. epidermidis were selected. The input for each surrogate model was a molecular fingerprint converted from a peptide sequence, and the outputs were the common logarithms of the mean and variance of the predicted activity value.
A peptide sequence was represented using the prepared amino acid codes, whose details are described in Section S1-1 (ESI†). Then, a peptide sequence was converted into two types of 2D peptide representations using the SMILES notation: standard and Skip-7. The details of both representations are shown in Section S1-2 (ESI†). The peptide representations were converted to molecular fingerprints using a Morgan fingerprint algorithm43 and molecular access system (MACCS) keys44 [Section S1-2 (ESI†)]. Two types of count-based Morgan fingerprints were generated under the conditions of the dimensions of 1024 and the maximal radius of two and four, respectively. The MACCS keys were 166 bits of structural key descriptors.
To identify the most effective combination of a peptide representation and a molecular fingerprint, 10-fold cross-validation was performed for each target. In this study, six combinations, consisting of two types of peptide representations and three types of molecular fingerprints, were validated in total. This study used an in-house dataset as the initial dataset, which is available at https://github.com/ycu-iil/MODAN/blob/main/data/Dataset_MODAN_initial.xlsx. The details of this dataset are described in Section S1-3 (ESI†). According to the evaluation based on a correlation coefficient, the best combination of a peptide representation and a molecular fingerprint was determined for each target. Each surrogate model used in the optimisation process was trained on the whole data using the best combination.
2.2 Recommendation of AMP candidates considering NPAAs
The recommendation of promising AMP candidates is performed in two steps: generation of AMP candidates containing NPAAs and recommendations of AMP candidates for experimentation based on the score calculated using the surrogate models and an acquisition function.
2.2.1 Generation of AMP candidates.
To generate AMP candidates, one or two amino acids in a lead peptide sequence were exhaustively converted into amino acids prepared for substitution. As the amino acids prepared for substitution, we selected three α,α-disubstituted NPAAs, α-aminoisobutyric acid (Aib), 1-aminocyclopentanecarboxylic acid (Ac5c), and 1-aminocyclohexanecarboxylic acid (Ac6c) (Fig. 2). Two α-NPAAs, diaminobutyric acid (Dab) and ornithine (Orn), were also used (Fig. 2). (S)-2-(4-Pentenyl)alanine (S5) and (R)-2-(7-octenly)alanine (R8) were amino acids that were prepared as the building blocks of side-chain stapling, the combination of two S5 (“S5,S5”) and that of R8 and S5 (“R8,S5”) (Fig. 2). Previous studies have reported that NPAAs and side-chain stapling improve helicity and antimicrobial activities.6,8,45,46 In addition, Val and Leu residues were also selected as amino acids prepared for substitution as a previous study reported that AMPs whose hydrophobic residues were converted into Val and Leu residues could suppress toxicity and exhibit potent antimicrobial activities.47
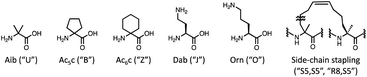 |
| Fig. 2 NPAAs and side-chain stapling used in this study. MODAN used the codes in brackets for the construction of surrogate models, and the generation of AMP candidates containing NPAAs. | |
2.2.2 Recommendation of AMP candidates for experimentation.
To recommend promising AMP candidates, MODAN sorted the AMP candidates in descending order based on the scores calculated by an acquisition function and then proposed them to us in that order. We selected the top-scoring AMP candidates and any other AMP candidates by considering the scores.48 Prior to calculating the scores, the antimicrobial and haemolytic activities and their variances in the generated AMP candidates were predicted using the seven trained surrogate models. In this study, we defined the total probability of improvement (TPI) score (eqn (1)), which is the product of the probability of improvement (PI) against the six antimicrobial (eqn (2)) and haemolytic activities (eqn (3)), as an acquisition function.49 PI is a well-known acquisition function used in Bayesian optimisation and it indicates the probability of exceeding or falling below a criterion. Here, PIk represents the PI of the kth target in the six antimicrobial activities, and the criterion ybac was set to 5 (μM). PItox indicates the PI of the haemolytic activity and the criterion ytox was set to 100 (μM). μk and μtox represent the mean values of the predicted antimicrobial and haemolytic activities, respectively. σk and σtox are the variance values of the predicted antimicrobial and haemolytic activities, respectively. The TPI score indicates the probability of meeting all activity criteria. | 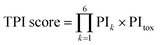 | (1) |
|  | (2) |
|  | (3) |
2.3 Experimentation
2.3.1 Peptide synthesis.
The recommended peptides were synthesized by Fmoc-based solid-phase methods using SyroI (Biotage, Tokyo, Japan). The H-Rink-Amide-ChemMatrix was swollen in dichloromethane for 30 min. After washing the resin with dimethylformamide (DMF), Fmoc-amino acid (4 equiv.), 1-[(dimethylamino)(dimethyliminio)methyl]-1H-benzo[d][1,2,3]triazole 3-oxide hexafluorophosphate (HBTU) (4 equiv.), 1-hydroxybenzotriazole monohydrate (HOBt) (4 equiv.), and N,N-diisopropylethylamine (DIPEA) (10 equiv.) were added. The Fmoc-protective groups were deprotected using 40% piperidine in DMF. After elongation of the sequence, the resins were dipped in a cleavage cocktail (95% trifluoroacetic acid (TFA), 2.5% water, and 2.5% triisopropylsilane) for 3 h at room temperature, and then the crude peptides were cleaved from them. The TFA was evaporated to a small volume under a stream of N2 and dripped into cold ether to precipitate the peptides. The peptides were dissolved in dimethyl sulfoxide and purified using reverse-phase HPLC using a Discovery®BIO Wide Pore C18 column (Supelco, Bellefonte, PA, USA) (25 cm × 21.2 mm solvent A: 0.1% TFA/water, solvent B: 0.1% TFA/MeCN, flow rate: 10.0 mL × mL−1, and gradient: 20–50% gradient of solvent B over 30 min).
2.3.2 Evaluation of antimicrobial activity.
In this study, the antimicrobial activities against DH5α, MDRP, and S. aureus were investigated. DH5α was purchased from BioDynamics Laboratory, Inc. (Tokyo, Japan). MDRP and S. aureus were obtained from the Biological Resource Center, NITE (NBRC, Tokyo, Japan). The antimicrobial activities of the peptides were evaluated using a standard broth microdilution method. The bacteria were inoculated and grown overnight at 37 °C on agar media and then collected with liquid media, according to the Japanese Pharmacopoeia, 17th edition. Sample peptides were diluted from an initial concentration of 500 μM to a final concentration of 1 μM using phosphate-buffered saline (PBS). Then, 10 μL of the peptide solutions were added to each well of a 96-well plate. 104 colony-forming units were added to each well. The plate was incubated for 16 h at 35 °C. The minimal inhibitory concentration (MIC) was defined as the lowest concentration of the peptide at which bacterial growth was completely inhibited, based on visual observations at 595 nm.
2.3.3 Evaluation of haemolytic activity.
Human red blood cells (RBCs) were kindly supplied by the Japanese Red Cross Society (Tokyo, Japan) after obtaining informed consent from the volunteers. The experimentation was conducted according to the procedure described in a previous study.8,50 The RBCs were washed and suspended in 172 mM Tris–HCl buffer (pH = 7.6). Then, 50 μL of the RBC solution was added to 50 μL of the peptide solution and incubated for 30 min at 37 °C. The suspension was then centrifuged at 400 rpm for 5 min. The absorbance of the supernatant was measured at 535 nm. M-lycotoxin51 was used as a positive control.
2.3.4 CD spectrometry.
The circular dichroism (CD) spectral analyses were performed using a J-1100 CD spectrometer (Jasco, Tokyo, Japan) with a 1.0 mm path length cell at 25 °C. The data were expressed in terms of [θ]; that is, total molar ellipticity (deg cm2 dmol−1). Peptides were dissolved in 20 mM phosphate-buffered solution (pH = 7.4) with 1% SDS at a concentration of 100 μM.
3. Results and discussion
Based on MODAN, we designed AMPs that considered NPAAs and side-chain stapling through two optimisation rounds using the magainin 2 derivative peptide (H-GIKKFLKSAKKFVKAFK-NH2; initial peptide)41 as a lead peptide sequence. Magainin 2 is a well-known natural AMP,52,53 and its derivatives have relatively high antimicrobial activity and low haemolytic activity.41 Improving these properties is challenging, but valuable.
3.1 First round
The surrogate models were trained using an in-house dataset, which included 82 peptides, as the initial dataset [Section S1-3 (ESI†)]. The trained models achieved relatively high performances, with correlation coefficients ranging from 0.70–0.88 [Section S1-4 (ESI†)]. Next, MODAN generated 123
390 AMP candidates and recommended promising peptides based on the TPI scores. The top three peptides with the highest TPI scores (peptides 1-1, 1-2, and 1-3), which consisted of only NAAs, were selected for experimentation. To consider peptides containing NPAAs, the top three, including Aib substitution with TPI scores (peptides 1-4, 1-6, and 1-7), and the top one, including Orn substitution with TPI scores (peptide 1-5), were also selected. The selected peptides and their TPI scores are listed in Table 1, and the helical wheel diagrams are shown in Fig. 3.
Table 1 Activities evaluated for the recommended peptides in the first round. Antimicrobial activities against three bacteria and haemolytic activity against red blood cells were investigated. U and O indicate Aib and Orn residues, respectively. The values in the brackets are the predicted activities by the surrogate modelsa
Peptide |
Sequence |
Total PI score |
MIC (μM) |
Haemolysis (μM) |
DH5α |
MDRP |
S. aureus
|
MIC, minimal inhibitory concentration; DH5α, Escherichia coli DH5α; MDRP, multiple-drug resistant Pseudomonas aeruginosa; S. aureus, Staphylococcus aureus. Activity values written in bold indicate that each criterion is met. The criteria of antimicrobial and haemolytic activities were set to 5 and 100 (μM), respectively.
|
Initial |
H-GIKKFLKSAKKFVKAFK-NH2 |
|
3.13 |
12.5 |
12.5 |
>200 |
1-1 |
H-GIKLLLKSAKKFVKAFK-NH2 |
0.284 |
6.25 (2.34) |
25.00 (2.94) |
25.00 (2.10) |
>200 (302) |
1-2 |
H-GIKLFLKSAKKFVKAFK-NH2 |
0.281 |
6.25 (2.85) |
25.00 (4.87) |
25.00 (2.63) |
>200 (219) |
1-3 |
H-GIKLVLKSAKKFVKAFK-NH2 |
0.277 |
12.50 (2.29) |
50.00 (2.32) |
>50.00 (2.49) |
>200 (268) |
1-4 |
H-GIKLULKSAKKFVKAFK-NH2 |
0.275 |
6.25 (1.89) |
25.00 (2.02) |
50.00 (3.43) |
>200 (333) |
1-5 |
H-GIOLFLKSAKKFVKAFK-NH2 |
0.257 |
6.25 (2.63) |
25.00 (4.60) |
25.00 (3.01) |
200 (241) |
1-6 |
H-GIKKULKSAKLFVKAFK-NH2 |
0.254 |
3.13 (1.83) |
50.00 (2.01) |
25.00 (3.67) |
>200 (317) |
1-7 |
H-GIKKFLKSAKLUVKAFK-NH2 |
0.244 |
1.56 (1.87) |
25.00 (1.95) |
6.25 (3.94) |
>200 (278) |
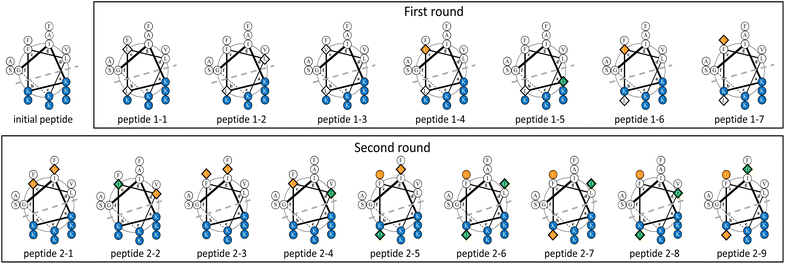 |
| Fig. 3 Helical wheel diagrams of the initial and recommended peptides in the first and second rounds. A diamond shape represents a substituted amino acid. Blue, green, and orange represent a cationic NAA, a cationic NPAA, and a hydrophobic NPAA, respectively. U and O indicate Aib and Orn residues, respectively. A dotted line shows the boundary line for amphiphilicity. | |
The seven selected peptides were synthesized, and their antimicrobial and haemolytic activities are listed in Table 1. The antimicrobial activities of peptide 1-7, in which the Lys and Phe residues at the 11th and 12th positions from the N-terminus in the initial peptide were converted to Leu and Aib respectively, exhibited enhanced antimicrobial activity against both DH5α and S. aureus. All seven peptides also showed low levels of haemolytic activity. Their secondary structures were analysed using CD spectroscopy, which revealed that peptide 1-7 had the highest helicity among the others [Fig. S2 (ESI†)].
3.2 Second round
The experimental data for the seven peptides in the first round were added to the initial dataset, and the surrogate models were then reconstructed. Their performances showed no significant numerical changes compared to those of the first round [Table S3 (ESI†)]. In this round, the initial peptide and peptide 1-7 were used as lead peptide sequences, and over 120
000 AMP candidates were generated from the initial peptide. Four peptides (peptides 2-1, 2-2, 2-3, and 2-4) from the top 10 candidates with the TPI scores were selected to assess cation NPAA substitution, predicted values against MDRP, and sequence isomers. Approximately 120
000 AMP candidates were generated from peptide 1-7. Five peptides (peptides 2-5, 2-6, 2-7, 2-8, and 2-9) from the top ten candidates with the TPI scores were selected to assess cation NPAA substitution, predicted values against MDRP, and sequence isomers. The selected peptides are listed in Table 2, and their helical wheel diagrams are shown in Fig. 3.
Table 2 Activities evaluated for the recommended peptides in the second round. Antimicrobial activities against three bacteria and haemolytic activity against red blood cells were investigated. U and O indicate Aib and Orn residues, respectively. The values in the brackets are the predicted activities by the surrogate models
Peptide |
Sequence |
Total PI score |
MIC (μM) |
Haemolysis (μM) |
DH5α |
MDRP |
S. aureus
|
2-1 |
H-GIKKULKSUKKFVKAFK-NH2 |
0.064 |
1.56 (2.04) |
25 (5.95) |
50 (3.49) |
>100 (83.15) |
2-2 |
H-GIKKOUKSAKKFVKAFK-NH2 |
0.052 |
50 (2.06) |
>50 (8.05) |
>50 (3.53) |
>100 (137.30) |
2-3 |
H-GIKKFLKSUKKUVKAFK-NH2 |
0.049 |
1.56 (2.12) |
25 (6.36) |
25 (3.29) |
>100 (66.07) |
2-4 |
H-GIKKUOKSAKKFVKAFK-NH2 |
0.040 |
>50 (2.15) |
>50 (10.74) |
>50 (2.96) |
>100 (161.89) |
2-5 |
H-GIKKFLKSUKOUVKAFK-NH2 |
0.048 |
1.56 (1.86) |
25 (6.53) |
25 (4.08) |
>100 (76.88) |
2-6 |
H-GIKKFLKSAKOUOKAFK-NH2 |
0.033 |
25 (1.72) |
>50 (9.58) |
>50 (4.64) |
>100 (119.21) |
2-7 |
H-GIKKFLKSAKUUOKAFK-NH2 |
0.031 |
12.5 (2.17) |
>50 (12.60) |
>50 (4.32) |
>100 (172.98) |
2-8 |
H-GIKKFOKSAKOUVKAFK-NH2 |
0.029 |
>50 (1.97) |
>50 (10.94) |
>50 (5.01) |
>100 (158.88) |
2-9 |
H-GIKKFLKSOKUUVKAFK-NH2 |
0.028 |
>50 (2.68) |
>50 (12.47) |
>50 (4.57) |
>100 (129.92) |
Nine selected peptides were synthesised, and their antimicrobial activities against DH5α, MDRP, and S. aureus were investigated (Table 2). Peptides 2-1, 2-3, and 2-5 showed potent antimicrobial activity against DH5α, comparable to the activity of peptide 1-7. The other peptides showed weak antimicrobial activity against any of all tested bacteria. The nine peptides also exhibited low haemolytic activity. Secondary structure analysis with CD spectral analysis indicated that peptides 2-1, 2-3, and 2-5 with high antimicrobial activity against DH5α had higher helicity than the other peptides [Fig. S3 (ESI†)]. During the optimisation process, some predicted MIC values were different from those of experimental activities, especially for S. aureus and MDRP. In terms of prediction performances evaluated by cross-validation, as for S. aureus, the whole prediction performance was lower than that for the other investigated targets, DH5α, MDRP, and haemolysis [Section S1-4 (ESI†)]. In addition, the prediction performance for S. epidermidis, which belongs to the same family Staphylococcaceae as S. aureus, was also relatively low. These results may indicate that the family Staphylococcaceae is a relatively difficult target to predict. For MDRP, the number of initial experimental data was relatively small [Section S1-3 (ESI†)], which generally makes the development of prediction models difficult. These factors may have led to the discrepancies between the predicted and experimental MIC values for MDRP and S. aureus.
3.3 Characteristics of the designed peptides
In the AMPs designed by MODAN, peptide 1-7 was particularly interesting because it had potent antimicrobial activity and low haemolytic activity, despite incomplete amphiphilicity due to the conversion from the cationic Lys residues to the hydrophobic Leu residues (Fig. 3). In AMP research, the perturbation of amphiphilicity via the conversion of cationic residues into hydrophobic residues is uncommon.4,8,41,54 Peptide 1-7, which had low cationic charge and incomplete amphiphilicity, showed higher antimicrobial activity than the initial peptide. This suggested that peptide 1-7 strongly disrupted the bacterial cell membranes as it easily formed an α-helical structure [Fig. S2 (ESI†)]. The helical wheel diagrams of the second-round peptides showed that peptides 2-1, 2-3, and 2-5 with potent antimicrobial activity and low haemolytic activity maintained amphiphilicity, whereas the other peptides pulled it down (Fig. 3). The results confirmed that the α-helical structure and amphiphilicity influenced the antimicrobial activity, as reported in previous studies.3,4
4. Conclusions
We have developed MODAN that enabled AMP design considering various NPAAs based on multi-objective Bayesian optimisation. A small peptide dataset was used to successfully design peptides 1-7, 2-1, 2-3, and 2-5, which contain α,α-disubstituted NPAAs with more potent antimicrobial activities and low haemolytic activity, within only two optimisation rounds. These results demonstrate the potential of MODAN for practical and efficient design considering NPAAs.
The improvement of surrogate models and the approach to generating AMP candidates will make MODAN more practical and efficient. To improve prediction performances, we plan to modify input features, considering electric charge, amphiphilicity, and peptide conformation. Moreover, we also investigate other ML methods that can take account of variance, such as Bayesian neural networks55 and Gradient Boosted Decision Trees.56
In this study, MODAN only explored the sequences around the initial sequences by substituting two amino acids. The current version of MODAN cannot cope with a combinatorial explosion when the number of substitution positions and the number of amino acids prepared for the substitution increase further. To address this issue, for example, the total number of combinations could be reduced based on chemists' knowledge of AMP designs, such as fixing hydrophobic or hydrophilic amino acids to keep amphiphilicity. Moreover, reinforcement learning techniques, such as upper confidence bounds applied to trees (UCT),57 will enable MODAN to efficiently search for promising mutation positions and amino acids to replace for generating AMP candidates.
Data availability
The source code used in this study are available at https://github.com/ycu-iil/MODAN. This study used an in-house dataset as the initial dataset, which is available at https://github.com/ycu-iil/MODAN/blob/main/data/Dataset_MODAN_initial.xlsx.
Author contributions
Yuki Murakami: investigation, software, and writing – original draft; Shoichi Ishida: methodology, software, and writing – review & editing; Yosuke Demizu: supervision, conceptualization, writing – review & editing, and funding acquisition; Kei Terayama: methodology, software, supervision, conceptualization, writing – review & editing, and funding acquisition.
Conflicts of interest
There are no conflicts to declare.
Acknowledgements
The authors would like to express their deepest appreciation to Ms Megumi Kurashima (National Institute of Health Sciences) for aiding in the evaluation of the antimicrobial activity. This work was conducted as a Research Support Project for Life Science and Drug Discovery (Grant Numbers: 23mk0101197j0003 (to Y. D.) and 23ak0101185j0302 (to Y. D.)) and “Development of a Next-generation Drug Discovery AI through Industry-academia Collaboration (DAIIA)” (Grant Number: JP22ama121023 (to K. T.)) supported by the Japan Agency for Medical Research and Development (AMED). This work was also supported by the Ministry of Education, Culture, Sports, Science and Technology (MEXT) as a “Program for Promoting Researches on the Supercomputer Fugaku (MD-drive Precision Medicine)” to K. T., “Feasibility studies for the next-generation computing infrastructure” to K. T., KAKENHI Grant Numbers 21K05320 to Y. D., and Data Creation and Utilisation Type Material Research and Development Project Grant Number JPMXP1122683430 (to K. T.). This study was supported in part by grants from the Chugai Foundation for Innovative Drug Discovery Science: C-FINDs (to Y. D.).
Notes and references
- P. Cardoso, H. Glossop, T. G. Meikle, A. Aburto-Medina, C. E. Conn, V. Sarojini and C. Valery, Biophys. Rev., 2021, 13, 35–69 CrossRef CAS PubMed.
- M. F. Mohamed, A. Abdelkhalek and M. N. Seleem, Sci. Rep., 2016, 6, 29707 CrossRef PubMed.
- Y. Liang, X. Zhang, Y. Yuan, Y. Bao and M. Xiong, Biomater. Sci., 2020, 8, 6858–6866 RSC.
- A. G. Elliott, J. X. Huang, S. Neve, J. Zuegg, I. A. Edwards, A. K. Cain, C. J. Boinett, L. Barquist, C. V. Lundberg, J. Steen, M. S. Butler, M. Mobli, K. M. Porter, M. A. T. Blaskovich, S. Lociuro, M. Strandh and M. A. Cooper, Nat. Commun., 2020, 11, 3184 CrossRef CAS PubMed.
- S. H. Gellman, Acc. Chem. Res., 1998, 31, 173–180 CrossRef CAS.
- M. Hirano, C. Saito, C. Goto, H. Yokoo, R. Kawano, T. Misawa and Y. Demizu, ChemPlusChem, 2020, 85, 2731–2736 CrossRef CAS PubMed.
- O. Makoto, J. Pharm. Soc. Jpn., 2019, 139, 599–608 CrossRef PubMed.
- M. Hirano, C. Saito, H. Yokoo, C. Goto, R. Kawano, T. Misawa and Y. Demizu, Molecules, 2021, 26, 444 CrossRef CAS PubMed.
- L. D. Walensky, A. L. Kung, I. Escher, T. J. Malla, S. Burbuto, R. D. Wright, G. Wagner, G. L. Verdine and S. Korsmeyer, Science, 2004, 305, 1466–1470 CrossRef CAS PubMed.
- G. Basu, K. Bagchi and A. Kuki, Biopolymers, 1991, 31, 1763–1774 CrossRef CAS PubMed.
- C. Toniolo, A. Polese, F. Formaggio, M. Crisma and J. Kamphuis, J. Am. Chem. Soc., 1996, 118, 2744–2745 CrossRef CAS.
- T. S. Yokum, T. J. Gauthier, R. P. Hammer and M. L. McLaughlin, J. Am. Chem. Soc., 1997, 119, 1167–1168 CrossRef CAS.
- F. Formaggio, M. Crisma, P. Rossi, P. Scrimin, B. Kaptein, Q. B. Broxterman, J. Kamphuis and C. Toniolo, Chemistry, 2000, 6, 4498–4504 CrossRef CAS PubMed.
- P. G. A. Aronica, L. M. Reid, N. Desai, J. Li, S. J. Fox, S. Yadahalli, J. W. Essex and C. S. Verma, J. Chem. Inf. Model., 2021, 61, 3172–3196 CrossRef CAS PubMed.
- R. Tamura, T. Osada, K. Minagawa, T. Kohata, M. Hirosawa, K. Tsuda and K. Kawagishi, Mater. Des., 2021, 198, 109290 CrossRef CAS.
- I. Ohkubo, Z. Hou, J. Lee, T. Aizawa, M. Lippmaa, T. Chikyow, K. Tsuda and T. Mori, Mater. Today Phys., 2021, 16, 100296 CrossRef CAS.
- Y. Saito, M. Oikawa, H. Nakazawa, T. Niide, T. Kameda, K. Tsuda and M. Umetsu, ACS Synth. Biol., 2018, 7, 2014–2022 CrossRef CAS PubMed.
- L. Tallorin, J. Wang, W. E. Kim, S. Sahu, N. M. Kosa, P. Yang, M. Thompson, M. K. Gilson, P. I. Frazier, M. D. Burkart and N. C. Gianneschi, Nat. Commun., 2018, 9, 5253 CrossRef CAS PubMed.
-
C. E. Rasmussen and C. K. I. Williams, Gaussian processes for machine learning, MIT Press, 2006 Search PubMed.
- J. Q. Candela and C. Rasmussen, J. Mach. Learn. Res., 2005, 6, 1939–1959 Search PubMed.
- K. Terayama, M. Sumita, R. Tamura and K. Tsuda, Acc. Chem. Res., 2021, 54, 1334–1346 CrossRef CAS PubMed.
-
L. Feng, P. Nouri, A. Muni, Y. Bengio and P. L. Bacon, arXiv, 2022, preprint, arXiv:2209.06259, DOI:10.48550/arXiv.2209.06259.
- A. Tučs, F. Berenger, A. Yumoto, R. Tamura, T. Uzawa and K. Tsuda, ACS Med. Chem. Lett., 2023, 14, 577–582 CrossRef PubMed.
- P. Das, T. Sercu, K. Wadhawan, I. Padhi, S. Gehrmann, F. Cipcigan, V. Chenthamarakshan, H. Strobelt, C. Santos, P. Chen, Y. Y. Yang, J. P. K. Tan, J. Hedrick, J. Crain and A. Mojsilovic, Nat. Biomed. Eng., 2021, 5, 613–623 CrossRef CAS PubMed.
- W. F. Porto, L. Irazazabal, E. S. F. Alves, S. M. Ribeiro, C. O. Matos, Á. S. Pires, I. C. M. Fensterseifer, V. J. Miranda, E. F. Haney, V. Humblot, M. D. T. Torres, R. E. W. Hancock, L. M. Liao, A. Ladram, T. K. Lu, C. Fuente-Nunez and O. L. Franco, Nat. Commun., 2018, 9, 1490 CrossRef PubMed.
- A. Tucs, D. P. Tran, A. Yumoto, Y. Ito, T. Uzawa and K. Tsuda, ACS Omega, 2020, 5, 22847–22851 CrossRef CAS PubMed.
- A. Capecchi, X. Cai, H. Personne, T. Köhler, C. Delden and J. Reymond, Chem. Sci., 2021, 12, 9221–9232 RSC.
- M. H. Cardoso, R. Q. Orozco, S. B. Rezende, G. Rodrigues, K. G. N. Oshiro, E. S. Cândido and O. L. Franco, Front. Microbiol., 2020, 10, 3097 CrossRef PubMed.
- X. Wang, X. Yang, Q. Wang and D. Meng, Crit. Rev. Microbiol., 2022, 1–25 Search PubMed.
- Y. Wang, Y. J. Yang, Y. N. Chen, H. Y. Zhao and S. Zhang, Comput. Methods Progr. Biomed., 2016, 134, 215–223 CrossRef PubMed.
- G. Maccari, M. D. Luca, R. Nifosí, F. Cardarelli, G. Signore, C. Boccardi and A. Bifone, PLoS Comput. Biol., 2013, 9, e1003212 CrossRef CAS PubMed.
- M. Xiong, M. Chen and J. Zhang, Chem. Biol. Drug Des., 2016, 88, 404–410 CrossRef CAS PubMed.
- Y. He and X. He, Pept. Sci., 2016, 106, 746–756 CrossRef CAS PubMed.
- M. Sandberg, L. Eriksson, J. Jonsson, M. Sjöström and S. Wold, J. Med. Chem., 1998, 41, 2481–2491 CrossRef CAS PubMed.
- F. Tian, P. Zhou and Z. Li, J. Mol. Struct., 2007, 830, 106–115 CrossRef CAS.
- G. Liang, Y. Liu, B. Shi, J. Zhao and J. Zheng, PLoS One, 2013, 23, e67844 CrossRef PubMed.
-
ChemOffice, PerkinElmer Inc., https://www.perkinelmer.com/product/chemoffice-chemoffice, last access: July 13, 2023.
- I. V. Tetko, J. Gasteiger, R. Todeschini, A. Mauri, D. Livingstone, P. Ertl, V. A. Palyulin, E. V. Radchenko, N. S. Zefirov, A. S. Makarenko, V. Y. Tanchuk and V. V. Prokopenko, J. Comput.-Aided Mol. Des., 2005, 19, 453–463 CrossRef CAS PubMed.
-
Molecular Operating Environment (MOE), Chemical Computing Group, Inc.: http://www.chemcomp.com/, last access: July 13, 2023.
- D. Weininger, J. Chem. Inf. Comput. Sci., 1988, 28, 31–36 CrossRef CAS.
- C. Goto, M. Hirano, K. Hayashi, Y. Kikuchi, Y. Hara-Kudo, T. Misawa and Y. Demizu, ChemMedChem, 2019, 14, 1911–1916 CrossRef CAS PubMed.
- Y. Motoyama, R. Tamura, K. Yoshimi, K. Terayama, T. Ueno and K. Tsuda, Comput. Phys. Commun., 2022, 278, 108405 CrossRef CAS.
- H. L. Morgan, J. Chem. Doc., 1965, 5, 107–113 CrossRef CAS.
- J. L. Durant, B. A. Leland, D. R. Henry and J. G. Nourse, J. Chem. Inf. Comput. Sci., 2002, 42, 1273–1280 CrossRef CAS PubMed.
- W. Kang, H. Liu, L. Ma, M. Wang, S. Wei, P. Sun, M. Jiang, M. Guo, C. Zhou and J. Dou, Eur. J. Pharm. Sci., 2017, 105, 169–177 CrossRef CAS PubMed.
- M. Xiong, M. Chen and J. Zhang, Chem. Biol. Drug Des., 2016, 88, 404–410 CrossRef CAS PubMed.
- A. R. D'Souza, M. R. Necelis, A. Kulesha, G. A. Caputo and O. V. Makhlynets, Biomolecules, 2021, 11, 421 CrossRef PubMed.
-
A. Tiihonen, L. Filstroff, P. Mikkola, E. Lehto, S. Kaski, M. Todorović and P. Rinke, AI for Accelerated Materials Design NeurIPS 2022 Workshop, 2022 Search PubMed.
- A. Takahashi, Y. Kumagai, H. Aoki, R. Tamura and F. Oba, Sci. Technol. Adv. Mater., 2022, 2, 55–66 Search PubMed.
- E. Strandberg, J. Zerweck, D. Horn, G. Pritz, M. Berditsch, J. Bürck, P. Wedhwani and S. A. Ulrich, J. Pept. Sci., 2015, 21, 436–445 CrossRef CAS PubMed.
- M. Akishiba, T. Takeuchi, Y. Kawaguchi, K. Sakamoto, H. H. Yu, I. Nakase, T. Takatani-Nakase, F. Madani, A. Gräslund and S. Futaki, Nat. Chem., 2017, 9, 751–761 CrossRef CAS PubMed.
- M. K. Kim, N. H. Kang, S. J. Ko, J. Park, E. Park, D. W. Shin, S. H. Kim, S. A. Lee, J. I. Lee, S. H. Lee, E. G. Ha, S. H. Jeon and Y. Park, Int. J. Mol. Sci., 2018, 19, 3041 CrossRef PubMed.
- Y. Li, H. Wu, P. Teng, G. Bai, X. Lin, X. Zuo, C. Cao and J. Cai, J. Med. Chem., 2015, 58, 4802–4811 CrossRef CAS PubMed.
- N. Pathak, R. Salas-Auvert, G. Ruche, M. H. Janna, D. McCarthy and R. G. Harrison, Proteins, 1995, 22, 182–186 CrossRef CAS PubMed.
- J. Bergstra, R. Bardenet, Y. Bengio and B. Kégl, Adv. Neural Inf. Process. Syst., 2011, 24, 2546–2554 Search PubMed.
- L. Prokhorenkova, G. Gusev, A. Vorobev, A. V. Dorogush and A. Gulin, Adv. Neural Inf. Process. Syst., 2018, 31, 6638–6648 Search PubMed.
-
L. Kocsis and C. Szepesvári, European conference on machine learning, 2006, pp. 282–293 Search PubMed.
|
This journal is © The Royal Society of Chemistry 2023 |
Click here to see how this site uses Cookies. View our privacy policy here.