Digital research environments: a requirements analysis†
Received
8th November 2022
, Accepted 30th March 2023
First published on 4th April 2023
Abstract
Physical science depends on historical records as well as new ideas, results, and opportunities. In this increasingly digital era, in which much of modern day life is technology-driven, a significant amount of scientific data remains in forms that are inaccessible to current methods. Moreover, the creation and subsequent accessing of high quality reusable data continues to present challenges. As part of the pilot phase of the Physical Sciences Data Infrastructure (PSDI) Initiative we investigated the current landscape of digitisation in 2022, aiming to produce an outline of what the physical sciences community requires from a Digital Research Environment (DRE). Evidence suggests that while scientists are digitising diverse portions of their work, some research still remains lost in paper lab books, and many barriers (hardware, software and people) persist for the effective management of scientific data. Our studies build on previous research and include an informal survey of what the UK Physical Sciences community considered to be the key requirements for capturing, sharing, and accessing data. The paper ends by outlining future prospects for PSDI and provides some concrete actions for different stakeholders in the community.
1 Introduction
Research in the physical sciences is increasingly dominated by digital tools and experiences. Experiments often involve sophisticated technology and ultra-fast data capture, and the Internet provides access to vast amounts of reference data along with methods and results from the scientific literature. However, a significant amount of scientific data remains in forms that are inaccessible to current methods, thus presenting challenges for reusing and exploiting that data. Notionally, the concept of a Digital Research Environment (DRE) exists already, but it has yet to be fully realised in practice. This paper describes our preliminary investigations into what might be required to reify the concept.
Despite the ubiquity of digital tools to support scientists and the benefits that they bring, certain aspects of the scientific record are still commonly captured on paper, principally the day-to-day record of ideas, methods and results that come from the design and implementation of experimental work. Various digital information systems have been developed to capture the myriad of digital data and other assets that are produced as part of the research process.1 Within the lab, Laboratory Information Management Systems (LIMS) have been extended from sample management and reporting to include automated data collection, data mining, and analysis. Electronic Laboratory Notebooks (ELNs) have been developed to enable the capture of research notes in digital form, and in some cases to link these notes to the data produced in the lab. ELNs have historically been more popular in certain settings; many scientists have chosen to make use of generic note-booking software or standard office tools to capture their research. For some scientists, such as computational chemists, all experimental work takes place within the digital domain. For all, the generation of digital data from instruments or through simulations, models, workflows, or other forms of code creates a potential disconnect between the experiment record, the data, and the software used to generate them.2 This disconnect leads to challenges at many stages of the data lifecycle, causing the processes of creating manuscripts for publication, or preparing data for sharing to be manual and laborious. For data sharing, in particular, this means that data published with and supplementary to publications often lacks important context about how it was produced or access to the tools and source data needed to reproduce it, making it hard to be assessed and reused within the community.
Studies over the past two decades3–6 have demonstrated that limitations with current software solutions have failed to fully address these issues. No individual piece of software exists that can address these problems and there are additional challenges to be addressed. These challenges include attitudes towards the adoption of software, especially for notetaking; a lack of consistent standards for both data and software making it difficult to move and utilise data between different systems and integrating with existing software and instruments in the lab. In the physical sciences, each laboratory or facility is likely to have its own isolated data infrastructure, using different working practices and tools. It is however recognised that for effective progression in science there is a need for researchers to effectively share their data, methods, findings, and tools. Findings need to be validated and data needs to be reusable to ensure funding for research activities results in good value and high-quality results. Other domains, especially the life sciences, make use of data-centric infrastructures for collecting and reusing data.7–9 These infrastructures act as community hubs that drive sharing of new methods and discoveries. Key to creating such hubs for the physical sciences is providing tools that facilitate the preparation of data, along with high quality research records, in discoverable and reusable formats.
As part of the pilot phase of the Physical Sciences Data Infrastructure (PSDI) Initiative,10 a case study comprising several activities was carried out to assess the current capabilities of existing software and potential requirements from physical scientists for tools that would support these aims. This paper discusses the design and results of an informal survey to elicit information about current working practices in the physical sciences and requirements for a DRE. A primary motivator for the survey was to assess whether researcher behaviours and working practices had changed in response to the pandemic and whether this might inform a new understanding of the needs of these researchers. In addition to the survey, a variety of other methods were used to assess and compare currently available tools and to derive requirements based on working practices of researchers across multiple physical science domains; these will be discussed in future work.
This paper begins with a brief background to the key issues and our work on process recording, the generation of the scientific record, and the rationale behind the survey and need to assess the requirements for DREs. In Section 3 we discuss the content, participation, and findings of the survey. Section 4 discusses the requirements and desired features for a DRE as derived from previous research and updated considering the survey findings and engagement during the pilot phase of PSDI. Section 5 discusses some possible options for technologies and software that could form part of a DRE to support physical scientists based on the derived features. The final sections of the document discuss the conclusions, how the work fits into the bigger picture and recommendations for future work.
2 Background
Over the last two decades research at the University of Southampton has investigated how e-Science infrastructures and digital tools can help to make smart and intelligent laboratories. A significant part of this work has been the design, development, and assessment of digital notebooks to both support and facilitate the work within physical sciences and ensure the capture, storage, and usability of the experiment record. These activities have included systematic literature reviews, qualitative research, focus groups and ethnography to examine laboratory practices and scientists' experiences with electronic notebooks and other digital tools.5,11–18 Over the last decade the accessibility and reusability of scientific data has become paramount. As a result the focus of the research has shifted from simply providing desirable digital replacement of paper notebooks to a broader picture of putting together an ecosystem of tools that facilitate the productive capture, sharing and reuse of scientific information for researchers across the physical sciences.
Many groups, including within the PSDI initiative, are concerned with the design and implementation of tools for preparing, finding, and sharing data. There are many challenges to overcome in making data findable, accessible, interoperable, and reusable (FAIR),19 not least the myriad of current and legacy data formats used across scientific domains. However, an often-overlooked element of making data usable and reusable within the community is that the data alone, however well-structured and machine readable it is, does not provide enough context either to validate it or to reuse it. Examples of such context are the methods used to generate the data, along with code, software, calculations, workflows, and source data used to produce the results. The experiment or research record should completely and accurately capture the conditions and methods used to generate and prepare the data; it is also likely to include important additional information about the rationale behind the experiment, why certain decisions were made, observations made, and if any problems were encountered. Different kinds of research may need different kinds of tools, but a digital tool that can build upon and enhance the functionality of the paper notebook is an essential requirement for recording the processes and rationale behind scientific research. Designing the right tools can facilitate the production of high-quality data for publication and to ensure that the associated records and assets are also accurate and complete. Effective management of the data and the research record enable publications to be more easily generated and less laborious to adequately prepare data for sharing. Such tools can also be designed to make use of appropriate standards and to both automate and prompt the user to add metadata that can facilitate data discovery, accessibility, interoperability and shareability.
Electronic Laboratory Notebooks have provided a digital replacement for the paper notebook, and even at the most basic level provide functions beyond the notebook such as providing the ability to quickly enter, retrieve, locate, and share data; facilitating long term storage through the creation of backups and archives;20 eliminating the need for manual transcription, and being usable by widely distributed groups.21 The majority of ELNs enable users to be able to link to their data in some way, either by attaching data files to the record or by creating a link to an external source and automatically capture at least a small number of metadata to enable the records to be searched or ordered. The potential benefits of ELNs are very high; not just capturing the record in a digital format but providing ways to integrate the digital data with the methods and observations behind the experiment and allowing easy sharing of the research enabling others to verify and contribute to the research more easily. However, previous research has demonstrated that many researchers were not entirely enamoured with the original concept and even terminology of ELNs.17 Researchers did not necessarily desire a replacement for their paper notebook, with the perception that such replacements would be difficult to use, and due to other barriers such as cost, data compatibility and accessibility in the lab.5,22,23 Even if scientists are not making use of ELNs to capture their research, many are producing digital copies of their research in some form,17 with many making use of tools that could be considered simple generic digital notebooks such as OneNote24 or even Microsoft Word.25
Beyond digital notebooks, other tools for generating and potentially capturing the experiment record are more relevant to those using computational methods for their research. For these disciplines, scraps of paper are more likely to be used for recording their thoughts or calculations with notebook use much more sporadic. Despite the computer-based nature of the research it is critical that methods of generating data and developing models or simulations are still recorded; not just documentation about the intended purpose of the tools, but also ensuring that the code itself is understandable, so that others can verify the code does what it claims to do. Some tools are more effective at providing functionality to support integrated documentation than others. For example, Jupyter Notebooks26 combine code, data, results, and documentation, for those users who make the effort to include it, all within the same document.27 This makes it possible for others to understand what the author was attempting to do and alter both data and code to see the effects. The same requirement of documentation is also true for researchers using workflow software to process and generate data.11,28
ELNs and indeed the overall offerings of scientific software have progressed significantly over the last decade with the remit of many ELN systems evolving away from merely a replacement for the paper lab notebook, to become a more complete digital platform.2 For example, RSpace,29 a digital research platform originating from the eCat ELN comprises the RSpace ELN and inventory and integrates with a variety of services supporting the full data lifecycle.29,30 Companies such as Benchling,31 Starlims32 and Agilent33 all offer ELNs as a wider part of a digital research service that include data management systems in addition to inventories and other tools. Many companies still offer ELNs without integration with other systems, but even those have evolved over time.23 The past perception that ELNs could provide all desired technical functionality under one software umbrella has turned out to be an unrealistic expectation as demonstrated by the shift to the ELN as a vital part of a wider ecosystem.16 Apart from a limited number of open science notebooks, researchers are unlikely to be accessing and reusing data directly from an ELN outside of their own research groups. Without active efforts to create and integrate tools for the purpose and ensuring compatibility of both data and their associated context, the amount of effort involved in sharing one's own data with the community and discovering and making use of the data of others is laborious and time-consuming.
Currently there is a disconnect between all the different tools that researchers are using making it hard to use them in conjunction to achieve the goals of creating findable, accessible, interoperable, usable, and reusable data for the scientific community. However, it is necessary to consider how to utilise the existing software used by physical scientists to create an optimum DRE, and what additional capabilities may be required. In addition to notebooks, physical scientists also use a vast range of domain-based software as part of the research lifecycle and their uses and capabilities need to be considered. Rather than attempting to specify or create a single software tool to encompass all processes, our survey looks to explore the community requirements of a DRE and understand how this could be achieved through existing tools and software. These investigations consider the diverse range of software and data formats that the physical sciences community currently work with, including the provisions for capturing metadata and details what work needs to be done to progress the lab of the future.
The survey was conducted as part of a range of other activities to investigate potential requirements for a DRE with these issues in mind. A key driver of the survey was to investigate whether the pandemic had changed any of the ways that researchers worked and whether resulting requirements for social distancing and increased remote working had driven more researchers to make use of ELNs or other digital notebooks to facilitate better communication or sharing with supervisors and co-workers. The survey was relatively informal and designed to act as a follow up to a series of focus groups conducted by Kanza et al. in 2017.5 Whilst many similar questions were asked by the new survey, several new areas of investigation required new questions. The informal nature of the survey allowed the use of many open questions to enable recipients to provide flexible responses. A full list of survey questions and details about the survey duration and participants can be found in the ESI† for this paper.
3 Results of the survey
Overall, 44 people from a mix of domains, experience and industry and academia participated in the survey; almost all the participants were UK based, due to the study being predominantly UK based. Over 50% of the respondents worked in the chemistry domain, with 30% working specifically in computational chemistry. 15% stated that they worked in physics departments with less than 5% working in the biology/life sciences sphere. The remaining participants were ambiguous about their specific research area.
3.1 Current working practices
The survey looked at current working practices for scientists, including how they record organise and link together their work.
3.1.1 Current use of paper and electronic.
In 2017, the responses from the focus groups and ethnography conducted by Kanza et al.5 demonstrated that researchers work in very different ways with different working patterns, and that they use a mix of paper and electronic methods to record their work, depending on the task at hand, with the highest use of paper for thinking about and recording work, and the highest use of electronic methods for analysing and writing up work. In the PSDI survey respondents were asked a similar question, and the results are displayed below in Fig. 1.
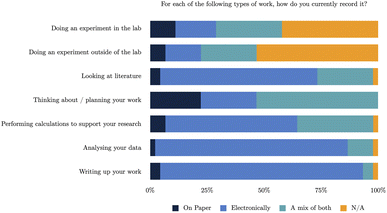 |
| Fig. 1 Results from Q1 regarding use of paper and electronic device in process recording. | |
Overall, this data demonstrates similar conclusions to five years ago. The use of purely paper based methods, compared to electronic or mixed approaches, is the least in each category, with the exception of thinking about and planning work. However, the overall split of the use of paper/electronic methods to record scientific research remains similar with respect to the different activities. Electronic methods are still heavily relied on at the intermediary and final stages of work, e.g. data analysis and writeup. However, the planning stages and recording data during experiments still remain quite split between the use of paper and electronic methods, as reflected in more recent work by Higgins et al.,6 and it is clear both from past research and these results that even now scientists still use paper-based methods frequently in these situations. This is not entirely surprising as the laboratory was always considered the most difficult location in which to record notes purely in an electronic form, due to barriers such as, access to hardware in the lab and being hostile environments for electronic devices.5,14,34 This suggests that whilst electronic methods are being used, despite the plethora of software solutions available, there is still much work to be done in providing suitable tools for those earlier stages in the research lifecycle.
3.1.2 Organising and linking work.
In addition to using a mixture of different methods to record their work, previous research also demonstrated that scientists organise and link their work together in very different ways. Shankar's studies in 2007 (ref. 35) concluded that taking notes and creating a standard method of data entry was personal to different scientists, and Nishida et al. noted that a key challenge in recording experiments was the potential for missing links between experiment records and their corresponding datasets.36 Kanza et al.'s 2017 studies5 demonstrated that this also holds true for organisational methods. It showed that scientists took different organisational strategies for paper and electronic notes, and used a variety of methods to link together paper and electronic notes, including using dates, codes, and adding hyperlinks of file paths into paper lab books. The user study participants described using a number of software packages to organise, and link together their work. Cloud storage tools such as Google Drive and Dropbox were mentioned with respect to having work all stored in one place. Additionally, some organisational tools were mentioned such as Google Keep37 and Google Tasks.38
Travelling forwards to today, the survey respondents were asked the same question, “How is your work organised and linked together?”. The results of these responses are categorised in Fig. 2, and show a level of similarity in approach to previous responses, but demonstrate an increase in both the use of software and range of software tools being used.
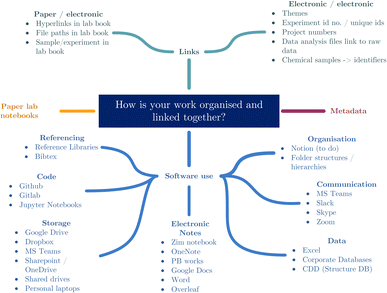 |
| Fig. 2 Categorised responses to questions about linking and organising work. | |
There has been no significant change in organising and linking paper and electronic research together. There is still a clear habit of using a linking piece of information to bridge between the two systems. The transcribing of hyperlinks and filepaths directly into paper lab notebooks shows that there is still a pattern of having paper notes linked to electronic data, and these links are being used to help the users find these related but disparate data sources.
Additionally, there remains a clear pattern of using unique ids for experiments and projects to signify which notes belong to which, both in the electronic and paper note systems. Unfortunately, the necessity to produce these between paper and electronic systems suggests that there are still portions of the scientific record that exist solely on paper. This leads back to the first stage of the remaining problem that “scientists need to digitise more of their work”. In terms of how work is electronically organised and linked, there is an obvious increase in the number of software packages used, including notably the use of software specifically for organisation. Fig. 2 shows categories of software that were noted by respondents with respect to organising and linking their work. This overall increase in the use of software will be discussed in further detail in the following section.
3.2 Software & data formats
Kanza et al.'s 2017 study5 concluded that despite some scientists preferring paper notebooks, they still frequently use a wide range of digital technology in their work. There are a number of generic notebook tools or even word processors available that can be used to capture the day-to-day scientific process. Indeed there have been many researchers that have published about using OneNote or Google Docs as an ELN.24,39–42 This has been a common theme across this initiative with most participants citing the use of electronic methods for different portions of their work.
3.2.1 Use of notebooking software.
Participants were asked about their use of software tools for data sharing and whether they used a Digital Research Notebook (DRN), a question that was deliberately left open to interpretation to gauge the range of responses that it would elicit. 55% said yes, they used a DRN and 45% said no they did not. The word cloud in Fig. 3 shows the range of responses given by those respondents who replied positively.
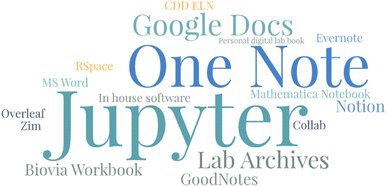 |
| Fig. 3 Word cloud depiction of the responses to use of digital research notebooks. | |
When questioned about ‘Digital Research Notebooks’, most of the respondents answers were not actually formal ELNs. A few were mentioned: Lab Archives,43 RSpace29 and Biovia Workbook.44 However, the rest were generic notebooking software such as MS Word, Google Docs, Zim,45 Overleaf,46 Evernote etc., with a clear preference for OneNote and Jupyter Notebooks. This is perhaps unsurprising with respect to Jupyter as 30% of respondents cited working in computational chemistry, but nonetheless these results demonstrate a range of software usage for notetaking.
3.2.2 Data sharing software.
Similarly, participants were asked about what software they used to share their data, the different categories of software identified are shown in Fig. 4.
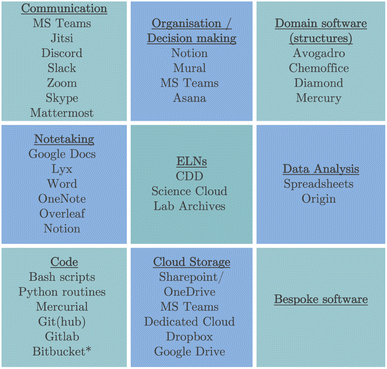 |
| Fig. 4 Categorised software responses for collaboration/sharing. All identified from Q9 except * from Q11. | |
This exemplifies the diverse use of software, even across a relatively small group of people. It is unsurprising that there has been a surge in the use of communication software as the COVID-19 pandemic made that a necessity. When participants were asked about what had changed since COVID-19, some mentioned an increase in digital sharing using cloud software. MS Teams47/OneNote/Sharepoint48 is clearly more prevalent than in earlier studies, as MS Teams only came into existence in 2017 (with a free version in 2018). In combination with Google Drive not adhering to the European Economic Area (EEA) requirements and many universities purchasing Office 365 subscriptions, this has meant a significant shift to the use of the Microsoft suite,24,40–42 although many still use other programs alongside that.
The use of software and scripts to share code has also increased as there has been greater emphasis on making these scripts available. Some domain-based software packages are also used for data sharing. This is almost certainly due to some data/work requiring specialist software to handle certain data types. This will be discussed further in the next section.
3.2.3 Other software.
Earlier studies also elicited that there is a wealth of domain-based software available for the physical sciences. Kanza (2018)16 conducted a study to ascertain what software packages existed in the domain of Chemistry and how much they were used. In the PSDI survey participants were asked what other software they used in their work. 206 different types of software were mentioned; these were then grouped, using both the categories identified in Kanza (2018)16 and some additional types that emerged from the data (as the initial list only pertained to chemistry software). The full list can be found in the ESI.†
Table 1 shows that there are many different types of software both generic and domain-specific being used across the physical sciences community. This demonstrates that users require a wide range of different features from software, ranging from data management, word processing and organisation, to more domain specific endeavours such as molecular modelling and crystallographic software. This demonstrates that scientists have a diverse set of needs with regards to using digital tools and it is increasingly unlikely that there will ever be one tool that encompasses all of these features. Thus work needs to be conducted to ascertain how to make the available tools work better together, and to address the second problem ‘improving data and record curation’, which needs to be taken into account when proposing any new solution.
Table 1 Categorised responses from the 206 software types identified in the PSDI survey on ‘use of other software’
Category |
Percentage (%) |
Crystallographic software |
12.44 |
Coding software |
10.53 |
Molecular Modelling & Simulation software |
10.53 |
Quantum Chemistry & Solid State Physics software |
10.05 |
Data Visualisation & Analysis |
9.09 |
General document processing |
8.61 |
Other |
6.22 |
Spectroscopic software |
4.78 |
Image processing software |
4.31 |
Chemical Database & Informatics software |
3.83 |
Organisational software |
3.35 |
Chemistry Bibliographic Databases |
2.39 |
Database software |
2.39 |
Instrument Control |
2.39 |
Simulation (non-chemical) |
2.39 |
Communication software |
1.91 |
Molecular Editor software |
1.44 |
Nanostructures Modelling software |
0.96 |
Machine Learning |
0.96 |
CAD software |
0.96 |
Workflow software |
0.48 |
3.3 Data formats
A key issue regarding the sheer volume of software in the physical sciences is the range of different data formats that have been created as a result. Experimental data can include files on safety information relating to the experiment, publications or methods that have been used as part of the experiment design, or images and data produced during and after the experiment. As demonstrated in Section 3.2.2, it is common for scientists to want to share their data. For example, users may wish to share a record with a collaborator or an auditor, or they might want to package the record and data for ESI.† For many researchers there are regulations that require that the records and the data be stored for the long term so content may need to be exported so that it can be backed-up or preserved. Unfortunately, it is far too common for all of these data to be produced in a variety of non-standard formats,49 which proves a major barrier to data sharing, both in terms of sharing between users and sharing between different software packages used to view data. There are ongoing efforts in the community to produce standard formats for the exchange of chemical information, such as AnIML,50 and chemical identifiers such as InChI.51 Indeed some ELN developers are part of the ELN file format initiative to try and promote interoperability between ELNs.52 However, despite these efforts, lack of adoption of standards, and data frequently being available in either non-standard formats or conflicting standard formats still presents barriers to digital research.
3.4 Barriers to digital research
In addition to understanding current research practices, it is also important to understand the current barriers to digital research that exist for today's physical science community. Previous research by the authors highlighted barriers to adoption, and limitations of ELNs.5,11–18 However, with the wide use of other software it was important to understand the overall barriers to all aspects of digital research, with the optimistic outlook that the new working practices many will have had to adopt as a result of COVID-19 might have accelerated some progress in this area. Participants were asked whether they encountered any restrictions in trying to share their data, and what barriers or limitations they faced that would prevent their research from reaching its full potential. A wealth of barriers and concerns were identified ranging from cost, hardware and software issues, problems with current systems, and people themselves. These have been grouped and described below.
3.4.1 Logistical barriers.
3.4.1.1 Cost.
This has been consistently listed as a barrier in previous research,5,22,53 and is an ongoing issue mentioned in the survey by multiple respondents. Researchers face issues of lack of funding, or running projects on small budgets, which are then further exacerbated by the cost of conducting research, e.g. cost of software licenses and open access publication fees. It is obvious that any DRE would involve the use of existing software that researchers already had access to via their institutions, or open-source software.
3.4.1.2 Time.
Whilst this is a hard problem to solve, time is a constant barrier, particularly in an increasingly busy world.5 Specifics raised in the survey that relate to this issue were: lack of time alongside other projects, the time it takes to use some systems and the time to find, ‘clean’, exploit data and to convert it between systems. One of the many reasons that scientists do not digitise all of their work, or why they do not digitise it to its full potential, is the time cost and the fact that the current systems in place make this a very arduous task.
3.4.2 People barriers.
3.4.2.1 Attitude.
The road to digitisation is a socio-technical task.5 In order to make improvements in this area the support of the users, systems and software are required. People are often afraid of change and it can be very challenging to persuade an entire research group to adopt a new piece of software, an issue echoed by some of the survey responses. There can also often be an unwillingness to share data and therefore an unwillingness to put the data in a format and location where it can be easily found, accessed and used.54
3.4.2.2 Training.
Whilst training was not specifically mentioned in response to our question about barriers, the importance of training was reflected in answers to other questions with respect to recommendations for the future, and discussing what data is and is not in a digital form. There will be a need for training to learn any new system, and researchers need to be adequately trained to manage their data well and digitise their work appropriately. This was emphasised by Ghannam et al.55 who postulated that there was a correlation between students not receiving proper training in notetaking and producing disorganised ill-formed notes. If users are unfamiliar with these concepts then they are unlikely to learn them overnight, and given the wealth of poorly digitised data, clearly further education is required in this area.
3.4.3 Data barriers.
3.4.3.1 (Un) FAIR data.
A key barrier for researchers is how much data does not adhere to FAIR standards,56,57 something that was noted in the feedback from our survey. Despite ongoing efforts in the community,57 researchers struggle to find data, both in terms of discovering it or locating it when references are incorrect. Accessibility is also an issue as often data cannot be accessed due to embargos. Data is not interoperable due to the many different data formats and conventions, and some data only being available in proprietary formats.58 Researchers have also cited that it is currently a very time-consuming endeavour to make data FAIR, producing a circular issue.
3.4.3.2 Metadata & provenance.
Metadata has been consistently mentioned by the survey respondents. It should be captured at all stages of the lifecycle such that data can be described, understood and re-used. However, it is hard to capture metadata and subsequently often researchers do not, or at least they do not record useful metadata which then means that data often lacks context. Further, it is also very important to researchers to be able to trace the source of the data, but often the original data are not made available, and there is a lack of appropriate historic data, making it hard to understand the origin of many experiments and papers.
3.4.3.3 Size of data.
Some participants mentioned that the size of the data they work with is an issue. Dealing with large datasets is a challenge, as is sharing them. There are a limited number of places to store large datasets and their size causes issues with uploads/downloads and collaboration.
3.4.4 Hardware & software barriers.
3.4.4.1 Storage.
Data storage options have been cited as another barrier both in the survey responses, and in previous research.49,59 Many universities provide Office 365 accounts and storage options. However, this clearly does not occur for all institutions as lack of cloud storage, limited data storage and poor cloud storage options have all been cited as limitations. There are alternative platforms such as Google Drive or Dropbox, however many universities do not allow the use of these services.
3.4.4.2 Software.
Software was raised as a huge issue for researchers for many different reasons. There is a call for more modern software, as many are still using outdated software that lacks proper documentation. A notable quote from one of the survey participants was “There is no ELN that combines experimental flexibility with data storage in multiple places”. There are also issues with system and software compatibility, for example trying to use Teams on Linux. Much software still only works for either Windows only, or Windows and Mac. Additionally, a lot of software programs that researchers need to use do not work well with one another.
3.4.4.3 Hardware.
Many laboratories need an equipment overhaul as they contain legacy equipment that only works with certain older operating systems, and often use outdated data formats.5 This must be addressed to improve the digital scientific record, although these changes would need to come from the institutions themselves, and in many cases are not financially viable.
3.5 Changes since COVID-19
Nothing has had as much potential to disrupt scientific working practices as the COVID-19 pandemic and subsequent lockdowns that turned almost everybody's working practices upside down. For many, going into work, sharing physical copies of data/notes and indeed at times accessing the laboratory simply was not an option. However, how much did this really change things in terms of digitisation, and will these changes remain now that the world is getting back to a “new normal”?
One of the main aims of this informal survey was to ascertain if COVID-19 had initiated any significant changes. It was hypothesised that requirements to work from home and communicate via online methods could have had a powerful impact on the digitisation of work, and the use of digital tools to achieve this. This has occurred in some ways, although not perhaps in the expected way.
Participants were asked: “Since the COVID-19 pandemic have you changed your research methods with respect to recording and sharing your work? If so, what aspects are digital that did not used to be?” which received some mixed answers. For some, nothing had changed; whilst others described an increased use of digital technologies although most predated COVID-19. Some respondents noted changes, stating that more of their work was digital, but it is worth noting that less than 10% mentioned the use of an ELN as part of this. Most participants who cited a change described having more data in a digital format, or an increased effort towards versioning work and syncing files between work and home computers. A more significant but expected change was the increasing use of virtual technologies to accommodate meetings, or the use of more note taking and organisational software to manage work.
It appears from the survey results that COVID-19 has led to some increase in the use of digital tools to communicate, digitise work and share data in an electronic form. However, this has not increased ELN adoption. It is clear from this question and the overall results, that whilst scientists have looked to other software to aid their research process. This has been in the form of either generic software to enable notes, task management, literature linking and supporting the use of code, or they have looked to domain-based software for specific solutions pertaining to their research.
These results show that while some participants have seen a greater shift towards digital technologies since COVID-19, many barriers still remain that hinder scientific research. Overall, this survey demonstrates that there is still no viable solution for improving the digitisation of scientific research, and strengthens the hypothesis that this cannot be solved by one piece of software. A platform approach where users can pick and choose their desired features would be a much more viable solution. The research conducted as part of this case study, in conjunction with the data gathered through historic studies,5,11–18 was used to identify features that are required by the physical sciences community for a DRE. These will be discussed in more detail in Section 4.
4 Requirements for a digital research environment
Previous research and the conclusions drawn from the survey analysis have reinforced the view that there is no simple solution to creating a tool to digitise scientific research, and that a future solution will not involve creating the ‘right’ piece of software that every scientist will want to use. The physical sciences are such a diverse wide-ranging set of disciplines that it would be close to impossible to capture all of the features required in one piece of software. The following subsections provide a summary of the key features desired by the community. It is arguably self-evident that it would not be practical to adequately satisfy all of these requirements, therefore Section 5 discusses how existing tools might be deployed, adapted, and extended to cover a broad range of the required features that would then form a DRE.
4.1 Required features
Kanza et al.5 conducted studies to identify the range of features that a scientist would want from an Electronic Lab Notebook. This was explored through the medium of a 3-layered approach: The Notebook Layer, the Domain Layer and the Semantic Layer.
However, having continued this research it has become clear that even though this approach was working along the right lines, it is not entirely appropriate. It has become apparent that even more features are required than initially identified. Scientists want generic notebooking features, but also want a range of features afforded by other software, including both domain-based and other types of useful software such as referencing and organisation. There is also a high requirement for supporting FAIR data and offering a wide range of data management tools. A substantial amount of these features exist in different software packages, although some still require creation or refinement. The authors propose that the best way of addressing these needs would be to identify the different software programs that already offer some of the required features, and where necessary, create the appropriate infrastructures (be that middleware, data conversion services, data management services that are compatible with other software) to enable them to interface with one another.
The required features identified throughout the last decade of research were collated and categorised, including those found through several systematic literature reviews, surveys and focus groups from the authors' previous work,5,11–18 the PSDI survey, and the all-partners meetings that brought together stakeholders. Fig. 5 demonstrates a potential DRE system working with a Physical Sciences Data Infrastructure at its core to enable these features. The functionality of the high-level categories for the features (represented by nodes on the diagram) are displayed in Fig. 5 and note which ones should be facilitated by existing software. It is inevitable that users will all have slightly different needs for their DRE, but ideally they would be able to use the PSDI services/infrastructures to harmonise their environment such that the different software they use can work together where necessary. The full breakdown of the individual features for each category can be found in the ESI† for this paper.
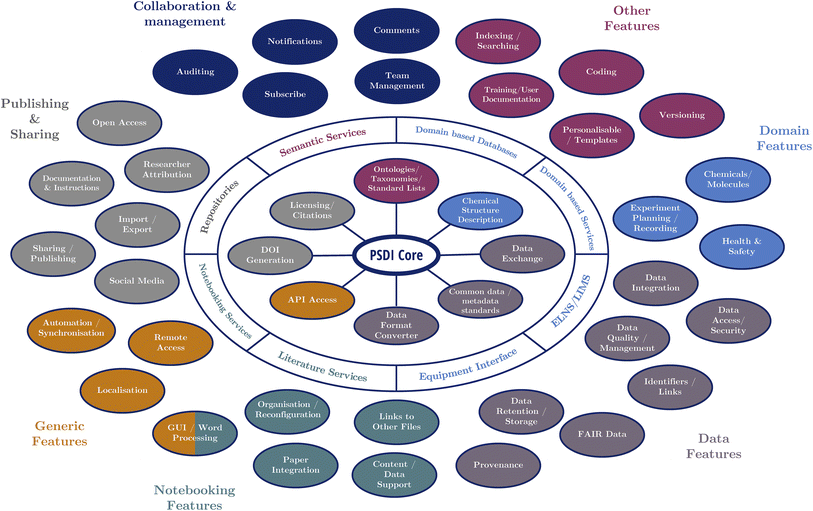 |
| Fig. 5 Example of the desired features of a DRE system, demonstrating the conceptual framework of a PSDI core interfacing with different services and features. | |
4.2 Generic features
Feature list: API access, automation, GUI, localisation, remote access, synchronisation.
There are several desired features for the high-level characteristics of a DRE. These all fall in line with the expectations of modern software, that it will be scalable, usable in their own language across all their devices, remotely accessible and have inbuilt automated features such as file saving and updates.
4.3 Notebooking features
Feature list: content support, interaction/access, file links, organisation/reconfiguration, paper integration, referencing/literature, word processing.
There are a number of desired features that either exist as part of generic notebooking software or would naturally extend onto notebooking software. Previous research has demonstrated that some of the required features for scientists are those that are synonymous with generic notebooking software, which is why the use of tools such as MSWord, OneNote and Google Docs remains high. Further, there is a strong desire for the entire workflow to interface with the use of paper (or at least devices that provide the functionality of paper), as one of the biggest drivers for the continued use of paper is the strong ease of use factor.
4.4 Data features
Feature list: access, conversion, exchange, integration, management, quality, retention, security, standards, support, FAIR, identifiers, provenance.
Data are at the cornerstone of scientific research, and as the amount of data generated (predominantly electronically but sometimes not) increases, researchers have ever growing needs for digital tools to manage and curate their data. Scientists want the capacity to integrate data, link it together using identifiers and capture its provenance. Users need to be able to work with their required domain data standards, and need features to ensure data integrity and consistent use of standards.
4.5 Publishing & sharing features
Feature list: documentation & instructions, DOIs, export, licensing, open access, publishing, sharing, social media, researcher attribution, repositories.
One of the desired end products of a scientific research project is creating publications. Users require features that will aid with this process: which includes mechanisms to create and supply DOIs, create data licenses, share data with others and to enable a direct link to relevant domain repositories. One of the concerns of using ELNs has always been that there would be an unnecessary duplication of effort. Users of these systems are reluctant to spend a long-time curating notes and data when they have to then re-perform half the work to produce the final publication. There has long been a call for a “generate report” or “generate thesis” button that would enable data and notes to be pulled together in at least a ‘semi-publication-ready’ state.
4.6 Collaboration & management features
Feature list: auditing, comments, notifications, subscribe, team management.
The global pandemic necessitated an increase in the use of management software. There are a number of features of collaboration and project/team management that are imperative for todays scientists. One of the features that users have cited that they need to be able to replicate in any digital system is the audit trail that is vital both from a health and safety perspective but also when it comes to claiming patents. Team management is vital to any group project. Many project management programs exist (Teams, Trello, Notion etc.) and these should be used as part of a DRE such that large scale projects can be managed, tasks can be allocated and work can be organised appropriately.
4.7 Domain-based features
Feature list: chemical/molecules, default lists, equipment interface, experiment planning/recording, health & safety, LIMS/ELN, link to domain-based databases & software.
The physical science domain contains a wide range of different disciplines, which within themselves (e.g. chemistry) have a large amount of subdomains. This means that there are a great deal of domain specific formats, standards, databases, software packages etc. (as demonstrated in Section 3.2). It would be entirely unrealistic to re-build all of these software packages within one piece of software, therefore there is a requirement to provide mechanisms to enable scientists working across different domains to use their required software alongside their notes and data.
4.8 Coding support
Feature list: coding, versioning.
As the amount of data generated by the physical sciences community continues to increase, there has been a vast surge in using computational methods to handle and analyse this data. Support for coding, and recording the process of developing code is essential for many scientists. Software such as Jupyter notebooks have become incredibly popular as data and code can be stored side by side and users can collaborate on coding projects, and any viable DRP would need to interlink with Jupyter notebooks and other software that offers similar functionality. Hand in hand with coding comes the notion of version control. In order to record the process of developing code, different versions of the code at different points need to be stored and there must be a capacity to roll back to different versions. Ideally users need to be able to interface between their notebooks/domain based software and GitHub and other popular version control services.
4.9 Metadata, semantics & AI
Feature list: AI tools/integration, metadata, semantics.
Computational techniques to manage data have increased, resulting in a growing desire to capture and store metadata, and to use intelligent technologies to improve data handling. Users have requested integration with ML packages for data analysis, and the capacity to store ML training data. Digital tools can automatically capture vast amounts of valuable metadata and the provenance trail. Where automatic capture is possible, they can prompt the user to add metadata as they proceed, rather than forcing a burdensome process of metadata generation and curation at the end of each process. There is also a demand for the inclusion of semantics, for interoperability and to add context and meaning to data. Users want their data to be annotated semantically where appropriate, and given links to established ontologies and vocabularies. This will enable experiments, data, code etc. to be classified, and will facilitate semantic search on ontology defined concepts which is a superior method compared to text-based searches. This also enables projects and notebooks to be linked together, and for inferences to be made about similar work.
4.10 Searching
Feature list: search by: domain, characteristics search, keyword/concept via content types, literature & notebook, indexing.
One of the key things that computers can do immeasurably better than humans is search efficiently through large datasets. Many of the features requested by users are related to search. Users want to be able to search different aspects of their work (e.g. datasets, notebooks, literature) and search in different ways (intelligent search via concepts, datasets with specific characteristics, similarity searches on content or even a visual search using images or to search for specific information in images or graphs). For example, chemists also want the capacity to perform domain specific searches such as searching by structure, reaction scheme or chemical etc.
4.11 Customisation & extension
Feature list: personalisable, templates.
Notetaking is very personal, and yet individuals have their own standard methods of working. Users want a DRE that is tailored to their own specific research and domain area, that enables them to create their own personalised template for models, code, experiment plans, notes etc. They also want the capacity to share these templates and use ones created by others.
4.12 Training & user support
Feature list: training, user documentation.
It is not enough to just provide software for different scientific endeavours, it is also important to provide scientists with support to enable them to use this software effectively. Training should be provided to both demonstrate the different types of functionalities the software within a DRE has to offer, and the most effective ways of using one. Further, any software should come equipped with a decent level of user documentation to aid users in using the system, and train them in concepts and approaches that are unfamiliar to them e.g. curation.
5 Technologies & software
To enable the overall connected research environment outlined within the example DRE system demonstrated in Fig. 5 there are a number of different technologies and software that could be used to aid development and implementation. This section touches on some of the areas of technology and software that would be required. This is not an exhaustive list of the technology/software areas that may be required, but shows some of the current technologies that could be employed to achieve more connected research recording. Each section will note the key next steps, both in terms of suggested research areas, and some suggestions for concrete actions to demonstrate where the community needs to move forward in this work.
5.1 Integration software/middleware
Integration with other services and systems is a crucial element of the overall research system. In particular, for the examples raised in Section 4.7, users may want to communicate with a chemical information service, bibliographic service or domain repository, among many other possibilities e.g. more generic software such as data visualisation and analytics software, or software that enables coding such as Jupyter notebooks. Users also want to be able to use software for taking notes, organisation and communication. Some of these software packages naturally stand alone, and don't necessarily require integration (e.g. your software for task management could usefully link with your calendar, but doesn't necessarily need to link with your chemical databases), whereas others would benefit highly from integration (e.g. if your ELN or Notebook could link with different data files, literature databases, Jupyter Notebook or data visualisation software). This type of flexible integration is slowly starting to surface on some scale, for example RSpace29 facilitates integration with office documents, repositories, domain based software and allows the import of a variety of generic and domain based data. It also provides an API for others to integrate with their systems. This approach aligns with our evaluation of user needs and should be more widely encouraged to enable this type of mass integration. However, given that this does not widely exist, ideally the community needs some infrastructures and frameworks to enable integration on a larger scale.
5.1.1 Prospects for PSDI – software analysis & prototyping.
Further work is required to ascertain what software is available, which software is most widely used, and which would be the most practical to link together based on how well they meet the user required features, and other aspects including: license, cost, data formats (import/export), platform and API access. This could be achieved through a combination of systematic investigation and surveying the wider community.
5.1.2 Community recommendations.
Software developers must provide API access and should facilitate integration with generic tools and enable import/export of data in common formats.
5.2 Data standards
As demonstrated in Section 5.1, users want to be able to integrate with many different services, and import/export their data into different formats, which will require common data standards to be in place. Data standards are a critical element to facilitate interoperability, allowing exchange of data between different systems and researchers. As detailed in Section 3.3 there is a plethora of file formats in the physical sciences, both generic and domain specific. There is a need for data format conversion between different data types in order to facilitate data exchange between different services, and to allow users to collaborate using common formats. There are existing software programs that facilitate some conversions such as OpenBabel,60 which converts between many of the different chemical formats with a specific focus on structural representation. There are also format converters for very specific domain formats such as WinSPEDAC,61 which is a software package that allows you to convert spectral data from one instrument manufacturer's format to another with some limitations. With respect to more generic data formats, there are a wide range of online format converters that exist.
5.2.1 Prospects for PSDI – investigate data standards & data format converters.
There needs to be an investigation of data standards that have already been widely adopted within the community, or defined by the governing bodies within a domain, and identification of where appropriate standards do not currently exist. Existing data format converters should be compared and evaluated to see if particular ones are more viable than others, with rigorous checks to ensure that data are not lost between different format conversions. Where suitable format converters do not exist, the optimum strategies for creating these should be investigated. These could include: creating an ‘intermediary-format’ that can convert any file into its format, and vice versa, using an existing generic format (e.g. XML,62 JSON63) as that intermediary format, building specialist data converters, interfacing with existing software programs that already facilitate conversions, and writing additional code to facilitate conversions for formats that are not represented. This conversion process should be well documented, allowing extension by the community to support further formats.
5.2.2 Community recommendations.
Researchers across both academia and industry should identify and actively work on areas in their disciplines where data format conversion is required. Where certain disciplines are lacking centralized bodies, the community as a whole need to consider setting up one to curate and manage the range of data formats used within a discipline.
5.3 Metadata
Another vital requirement clearly demonstrated by the case study results is the need for metadata and metadata standards. Metadata needs to be captured to describe documents/data/code (e.g. author, date, file size) much of which can be done using bibliometric standards such as Dublin Core64 and DCAT.65 Domain-based metadata also needs to be captured to describe the context and content of scientific documents (e.g. experiment links, equipment links, chemicals represented).
Whilst it is widely agreed that the capturing of metadata is vital, and indeed some electronic systems such as word processing software, ELNs and instrument based software do capture some information automatically (e.g. date, time, author). However, not all software/systems offer this service, and generally the metadata that is automatically captured is only that of the generic type, rather than additional domain specific data.
The manual task of adding comprehensive metadata to all documents, data, code etc. is an arduous task, and therefore one that is frequently ignored. Methods of automatically capturing metadata upon document and data creation need to be considered. Some work is being done in this area, such as the Materials Research Data Alliance (Marda)'s Metadata Extractor Working Group66 which is working on “connecting and advancing interoperability of efforts on automated extraction of metadata from materials files”. However, this is an ongoing and wide-ranging effort that is required across the whole of the physical sciences.
There are a number of automatic tagging services such as Refinitiv's Intelligent Tagging Service,67 and OntoText's Semantic Tagging Service.68 These perform relatively well at providing tags which could be used as metadata for more generic terms, but there is a lot of work to be done to provide automatic tagging in different domain specific areas. Some projects (such as ChemicalTagger69) have made a start in this area, but it still requires extensive further work. User research looking at these services suggested that whilst users would want a tool that did most of the work for them, they would also want to be able to edit the results after completion to make corrections. Therefore, users require methods of automatically capturing metadata in such a way that it could be customised and edited by different users.
5.3.1 Prospects for PSDI – investigate standards & metadata.
To improve metadata capture both in terms of automation, and identifying what should be captured. There needs to be a thorough investigation into automating metadata capture, and how to integrate this process with existing software used to create, store and manage data. The community needs to understand what benefits and capabilities different metadata schemas and services can provide. Available metadata ontologies/schemas (e.g. Dublin Core) should be investigated to see what terms they cover and identify any gaps, in addition to identifying best practices for capturing metadata.
5.3.2 Community recommendations.
Software developers should expose what metadata is automatically captured and what isn't by their tools, and researchers in different disciplines need to work together to agree on best practice guidelines for metadata capture in their work.
5.4 Semantic enrichment
Users have demonstrated a need for semantic enrichment of their research, through the requests for better search capabilities, consistency in data terminology and the desire to be able to link with and locate similar work. This can be achieved through marking up and annotating their documents and data semantically with links to established ontologies and vocabularies. This will in turn facilitate a semantic search whereby data can be traversed and retrieved using the graph structure of RDF. This would provide a much wider range of data paths than, for example, performing SQL queries on standard relational databases, and will also enable projects and notebooks to be linked together and for inferences about similar work to be made.
There are a number of ontologies that exist within the physical sciences. Some of the more popular ones are the three created by the Royal Society of Chemistry, RXNO – Named Reactions Ontology,70 CMO – Chemical Methods Ontology71 and MOP – Molecular Processes Ontology.72 There is also the ChEBI – Chemical Entities of Biological Interest73,74 and CHEMINF – terms commonly used in cheminformatics.75,76 Many others exist, Strömert et al. produced a comprehensive review of ontologies in Chemistry,77 and many others can be viewed on BioPortal78 (such as the Gene Ontology79,80 or the BioAssay Ontology81,82) and other ontology repositories. In order to identify the usefulness and relevance of different ontologies an evaluation would need to be performed, much like Kanza and Frey83 undertook in the domain of Drug Discovery ontologies.
The Semantic Web also has several annotation data formats for web-based documents. Resource Description Framework in Attributes (RDFa),84 Microdata85 and Java-Script Object Notation for Linked Data (JSON-LD).86 Each of these formats are HTML extensions that permit embedding rich metadata within HTML pages to provide additional information to the browser about the meaning of the pages and their context, meaning that search engines are able to access this metadata to retrieve more accurate search results. Additionally, this could also be achieved by creating a knowledge graph about different documents and datasets in RDF. By creating these annotations and descriptions, this will enable the classification of the different data, documents, notebooks etc., and facilitate semantic search on concepts.
5.4.1 Prospects for PSDI – evaluate semantic tools & ontology.
It would be useful to investigate and evaluate relevant ontologies and semantic systems for use in the physical sciences community, and identify gaps where new ontologies need to be created. Relevant taxonomies that could be converted into ontologies should also be investigated. Further, there needs to be experimentation of different methods for semantic annotation to ascertain which approaches work best, and whether any of the formats have particular advantages or not.
5.4.2 Community recommendations.
Ontology creators should either allocate resource to maintain and update their ontologies, or they should be handed over to a centralised service to manage this task. Hand in hand with this, ontology lookup services/ontology databases should also ensure that they are regularly maintained to ensure that researchers understand which ontologies remain active and in development. Additionally, software developers and researchers should work on lightweight methods of incorporating semantic web technologies into services in a useful manner, as often transforming an entire dataset into an RDF graph isn't necessarily the answer.
5.5 Hybrid & voice technologies
The outcomes of this case study have illustrated that there are many affordances of paper that still entice scientists to use it for several note-taking endeavours as opposed to using an electronic device. Further, scientists find it incredibly intrusive to use a keyboard in the laboratory, both due to the slower data entry than paper and a frequent requirement to remove gloves and sanitise before touching a computer. Therefore other non keyboard based methods are required to record data and indeed interface with any laboratory systems or software. An alternative to paper is hybrid notebook devices that allow users to write on them in a manner akin to paper, whilst also digitally saving and preserving the notes, and in some cases automatically converting the handwritten notes into electronic text. Examples of these devices are the reMarkable,87 and Boox.88 There are also smart paper and pen systems e.g. the RocketBook.89 This will obviously still produce data in an arguably “analogue” digital form, but it will be digital nonetheless, meaning that via digital devices users will be able to store it, locate it, and protect it against loss.
Another alternative method of recording notes and interfacing with the laboratory is through voice technologies. Companies such as LabTwin90 and Lab Voice91 have been working on voice powered smart assistants that enable data capture, instrument control, and interfacing with different pieces of software all through voice commands. Research by Knight et al.92 has demonstrated the great potential of voice and smart laboratories, and as such it would be advantageous for future notebooking software or ELNs to be able to work with these different types of technologies.
It would be also interesting to explore different methods of increasing digitisation through unconventional methods such as taking photographs of lab pages (as tried by Lang and Botstein in 2011 (ref. 93)) and automatically saving them to notebooking software such as Google Drive or OneNote. Simple software could be written to automatically organise lab notebook pages into dated folders, which would only require users to take a photograph of each lab page using a phone app. This could then link to users' notebooking software of choice so that whilst writing up work they could easily access photographs of their lab books.
5.5.1 Prospects for PSDI – enabling the future lab.
Work needs to be conducted to understand how best to make use of hybrid and voice technologies. This should involve evaluating the hybrid notebook tools available to see how well they work overall and how/if hybrid notebook tools can be linked with existing software. Investigations should also be conducted into creating Smart Labs, including what software currently facilitates voice control and the technological logistics of enabling this.
5.5.2 Community recommendations.
Software developers of lab management software should work with those implementing voice solutions and hybrid notebook devices (e.g. tablets/smart notebooks) to see how integration between their systems is possible.
6 Conclusions
The results of this case study support many of the original conclusions that have been formed in recent years.5,16,17 There is a clear requirement for better digital tools to enable scientists to conduct, share and publish their research, and yet there is still no one single system that supports this endeavour. This is namely because, based on the diverse and extensive physical sciences community and the sheer level of different software requirements and tools available, this is extremely unlikely to ever be possible. The community needs to move away from the idea that the solution is to create “one piece of software to rule them all” and focus on how the available tools can be best used, improved and integrated.
Many of the results from the research and survey demonstrate that scientists use a wide breadth of generic and domain-based software tools to support their research. There is a calling for these tools to play better together, and for common data standards to be adopted such that different software tools can be used in conjunction with each other. There is also an overwhelming call for improving metadata creation and usage. Further, this is a people issue as much as a hardware and software issue. Many of the hurdles that scientists encounter in their research also centre round user adoption and attitude.
There are two main areas that need to be considered: improving how much information is captured in a digital form, and enhancing how this digital data is managed. Both of these need to be addressed (separately and in conjunction with one another) in order to reach a point of a cohesive digital physical sciences community. The authors therefore propose that rather than trying to reinvent the wheel, more effort should be expended on training and educating our community, and applying user centered design methodologies to develop methods to enable a functional DRE that supports the digitisation and management of scientific research. Work should also be undertaken to facilitate links between existing domain-based software, and improve metadata design and capture.
7 The bigger picture and PSDI
The aim of PSDI is to enable researchers in the physical sciences to handle data more easily by connecting the different data infrastructures they use. PSDI will link up and enhance existing Physical Sciences infrastructures in the UK. A significant part of this is to facilitate the use of digital technologies and provide a DRE for the physical sciences.
PSDI will have a core element that offers a range of services. Those most relevant to this paper are: links between notebooking software (e.g. OneNote, ELNs), and domain-based software, common data and metadata standards that can be used across different software, ontologies/taxonomies to describe scientific data, data format conversion and data exchange. It will also offer access to high quality open scientific literature, adequate descriptions of chemical structures, DOI generation and linking, APIs to access different types of data, licenses for data and scientific research and citations and attributions for all datatypes.
The PSDI next steps outlined in Section 5 will be initiated in the next phase of PSDI (phase 1b). These will go a long way to not only enabling DREs for the physical sciences community, but also in producing many of the core features envisioned for PSDI.
The community recommendations are actions that the authors believe are important steps forward for the physical sciences and require the efforts of the whole community including software creators and developers, researchers, data curators, service managers, and the breadth and knowledge across academia and industry.
Ethical statement
Ethics approval was obtained from the University of Southampton Ethics and Research Governance Team through application ERGO/FEPS/70431 and the participants were provided with a participant information sheet and data protection plan prior to their participation in the survey.
Data availability
The following data is available for this paper relating to the Survey and ELN Landscape Assessment.
https://doi.org/10.5258/SOTON/D2437 – this record details the survey participants and duration and contains the following files:
PSDI_Survey_Questions.pdf – survey questions.
PSDI_CSSurvey_Responses.xlsx – anonymised survey results.
https://doi.org/10.5258/SOTON/D2438 – this record contains data analysis and categorisations of data from the survey. It contains the following files:
PSDI_FullListofFeatures.pdf – full list of features taken extrapolated from the survey and previous research with detailed explanations.
PSDI_CategorisedSoftware.pdf – categorised list of features extrapolated from the survey and previous research.
PSDI_CategorisedFeatures.pdf – categorised list of software taken from the survey.
ELNLandscapeAssessment.xlsx – ELN data collected as part of this project.
Author contributions
SK, CW, NK and CB undertook the main research for this article. SJC, JGF and NK organised the initiative and reviewed the material. SK, CW, NK & CB wrote the bulk of the manuscript. All authors reviewed and approved the manuscript pre-submission.
Conflicts of interest
There are no conflicts to declare.
Acknowledgements
This work was funded by EPSRC through grants EP/W032252/1 – PSDI (Physical Sciences Data Infrastructure (PSDI) Phase 1 Pilot), EP/S000356/1 – AI3SD Network+ (Artificial Intelligence and Augmented Intelligence for Automated Investigations for Scientific Discovery) and EP/S020357/1 – PSDS (Physical Sciences Data Science Service). This work also builds on research supported by the following grants: Web Science Centre for Doctoral Training at the University of Southampton funded by EPSRC under Grant No. EP/G036926/1, and the e-Science and digital economy activities funded under EPSRC Grants GR/R67729/01, EP/C008863/1 and EP/G026238/1 and EP/K003569/1.
Notes and references
-
J. G. Frey, D. D. Roure, M. C. Schraefel, H. Mills, H. Fu, S. Peppe, G. Hughes, G. Smith and T. R. Payne, Proceedings of First International Workshop on Hypermedia and the Semantic Web, 2003, p. 9 Search PubMed.
- M. Ulbrich and V. Aggarwal, Journal of Business Chemistry, 2019, 2, 76 Search PubMed.
- H. K. Machina and D. J. Wild, J. Lab. Autom., 2013, 18, 264–268 CrossRef.
-
G. Oleksik, N. Milic-Frayling and R. Jones, Proceedings of the 17th ACM conference on computer supported cooperative work & social computing, New York, NY, USA, 2014, pp. 120–133 Search PubMed.
- S. Kanza, C. Willoughby, N. Gibbins, R. Whitby, J. G. Frey, J. Erjavec, K. Zupančič, M. Hren and K. Kovač, J. Cheminf., 2017, 9, 31 Search PubMed.
- S. G. Higgins, A. A. Nogiwa-Valdez and M. M. Stevens, Nat. Protoc., 2022, 17, 179–189 CrossRef CAS PubMed.
- T. Kirsten, A. Gross, M. Hartung and E. Rahm, J. Biomed. Semant., 2011, 2, 6 CrossRef PubMed.
- R. Drysdale, C. E. Cook, R. Petryszak, V. Baillie-Gerritsen, M. Barlow, E. Gasteiger, F. Gruhl, J. Haas, J. Lanfear and R. Lopez,
et al.
, Bioinformatics, 2020, 2636–2642 CrossRef CAS PubMed.
- L. C. Crosswell and J. M. Thornton, Trends Biotechnol., 2012, 30, 241–242 CrossRef CAS PubMed.
-
Physical Sciences Data Infrastructure, https://www.psdi.ac.uk/, Accessed: 2022-10-27 Search PubMed.
- S. J. Coles, J. G. Frey, C. L. Bird, R. J. Whitby and A. E. Day, J. Cheminf., 2013, 5, 52 Search PubMed.
-
S. J. Coles, R. J. Whitby, A. Day, C. Willoughby, V. Tkachenko, J. G. Frey and A. J. Williams, Abstracts of Papers of the American Chemical Society, 2013 Search PubMed.
- A. J. Milsted, J. R. Hale, J. G. Frey and C. Neylon, PLoS One, 2013, 8, e67460 CrossRef CAS PubMed.
- K. A. Badiola, C. Bird, W. S. Brocklesby, J. Casson, R. T. Chapman, S. J. Coles, J. R. Cronshaw, A. Fisher, J. G. Frey, D. Gloria, M. C. Grossel, D. Brynn Hibbert, N. Knight, L. K. Mapp, L. Marazzi, B. Matthews, A. Milsted, R. S. Minns, K. T. Mueller, K. Murphy, T. Parkinson, R. Quinnell, J. S. Robinson, M. N. Robertson, M. Robins, E. Springate, G. Tizzard, M. H. Todd, A. E. Williamson, C. Willoughby, E. Yang and P. M. Ylioja, Chem. Sci., 2015, 6, 1614–1629 RSC.
- A. E. Day, S. J. Coles, C. L. Bird, J. G. Frey, R. J. Whitby, V. E. Tkachenko and A. J. Williams, J. Chem. Inf. Model., 2015, 55, 501–509 CrossRef CAS PubMed.
-
S. Kanza, Doctoral Thesis, University of Southampton, 2018.
- S. Kanza, N. Gibbins and J. G. Frey, J. Cheminf., 2019, 11, 23 Search PubMed.
-
C. Willoughby, Recording Science in the Digital Era: From Paper to Electronic Notebooks and Other Digital Tools, Royal Society of Chemistry, 2019 Search PubMed.
- M. D. Wilkinson, M. Dumontier, I. J. Aalbersberg, G. Appleton, M. Axton, A. Baak, N. Blomberg, J.-W. Boiten, L. B. da Silva Santos and P. E. Bourne,
et al.
, Sci. Data, 2016, 3, 1–9 Search PubMed.
- C. Voegele, B. Bouchereau, N. Robinot, J. McKay, P. Damiecki and L. Alteyrac, Bioinformatics, 2013, 29, 1710–1712 CrossRef CAS PubMed.
- J. D. Myers, Simulation Series, 2003, 35, 13–22 Search PubMed.
- E. D. Foster, E. C. Whipple and G. R. Rios, J. Med. Libr. Assoc., 2022, 110, 222 CrossRef PubMed.
-
B. Gerlach, C. Untucht and A. Stefan, Good Research Practice in Non-Clinical Pharmacology and Biomedicine, Springer, Cham, 2019, pp. 257–275 Search PubMed.
- S. Guerrero, A. López-Cortés, J. M. García-Cárdenas, P. Saa, A. Indacochea, I. Armendáriz-Castillo, A. K. Zambrano, V. Yumiceba, A. Pérez-Villa, P. Guevara-Ramírez, O. Moscoso-Zea, J. Paredes, P. E. Leone and C. Paz-y Miño, PLoS Comput. Biol., 2019, 15, e1006918 CrossRef CAS PubMed.
-
M. Cardenas, 2014 ASEE Annual Conference & Exposition, 2014, pp. 24–164 Search PubMed.
-
Jupyter Notebook, https://jupyter.org/, Accessed: 2022-10-27 Search PubMed.
- J. M. Perkel, Nature, 2018, 563, 145–146 CrossRef CAS PubMed.
- M. Monteiro, Computer Supported Cooperative Work, 2010, 19, 335–354 CrossRef.
-
RSpace ELN & Inventory, https://www.researchspace.com/, Accessed: 2022-10-27 Search PubMed.
- N. H. Goddard, R. Macneil and J. Ritchie, Automated Experimentation, 2009, 1, 1–7 CrossRef PubMed.
-
Cloud-based platform for biotech R&D | Benchling, https://www.benchling.com/, Accessed: 2023-01-26 Search PubMed.
-
LIMS | Laboratory Information Management System, https://www.starlims.com/, Accessed: 2023-01-26 Search PubMed.
-
Chemical Analysis, Life Sciences, and Diagnostics | Agilent, https://www.agilent.com/, Accessed: 2023-01-26 Search PubMed.
- C. L. Bird, C. Willoughby and J. G. Frey, Chem. Soc. Rev., 2013, 42, 8157–8175 RSC.
- K. Shankar, J. Am. Soc. Inf. Sci. Technol., 2007, 58, 1457–1466 CrossRef.
- E. Nishida, E. Ishita, Y. Watanabe and Y. Tomiura, Proceedings of the Association for Information Science and Technology, 2020, 57, e388 CrossRef.
-
Google Keep, https://www.google.com/keep/, Accessed: 2022-10-27 Search PubMed.
-
Google Tasks, https://apps.apple.com/us/app/google-tasks-get-things-done/id1353634006, Accessed: 2023-02-03 Search PubMed.
-
K. Colabroy and J. K. Bell, Biochemistry education: from theory to practice, ACS Publications, 2019, pp. 173–195 Search PubMed.
-
R. L. Johnson, A. M. Parsons and H. D. Tran, Electronic Lab Notebooks: Sandia Pilot Project, Sandia National Lab, Albuquerque, NM, USA, 2020, technical report Search PubMed.
-
S. R. Soltau, Integrating Professional Skills into Undergraduate Chemistry Curricula, ACS Publications, 2020, pp. 259–279 Search PubMed.
- C. Patrick Jr, Biomedical Engineering Education, 2022, 2, 305–317 CrossRef.
-
LabArchives, https://www.labarchives.com/, Accessed: 2022-08-16 Search PubMed.
-
BIOVIA Workbook, https://www.3ds.com/products-services/biovia/products/laboratory-informatics/electronic-lab-notebooks/biovia-workbook/, Accessed: 2023-01-26 Search PubMed.
-
Zim – a desktop wiki, https://zim-wiki.org/, Accessed: 2022-10-27 Search PubMed.
-
Overleaf, https://www.overleaf.com, Accessed: 2022-10-27 Search PubMed.
-
Microsoft Teams, https://www.microsoft.com/en-us/microsoft-teams/group-chat-software, Accessed: 2022-10-27 Search PubMed.
-
Microsoft SharePoint, https://www.microsoft.com/en-gb/microsoft-365/sharepoint/collaboration, Accessed: 2022-10-27 Search PubMed.
- C. Willoughby and J. G. Frey, Digital Discovery, 2022, 183–194 RSC.
-
AnIML, https://www.animl.org/, Accessed: 2023-01-26 Search PubMed.
- S. R. Heller, A. McNaught, I. Pletnev, S. Stein and D. Tchekhovskoi, J. Cheminf., 2015, 7, 23 Search PubMed.
-
ELN file format (.eln), 2023, https://github.com/TheELNConsortium/TheELNFileFormat/blob/8535be4a7484ee167cf3167f25caf9f5357e70b1/SPECIFICATION.md, Accessed: 2023-01-26 Search PubMed.
-
S. Boobier, J. Davies, I. Derbenev, C. Handley and J. Hirst, AI4 Green: An Open-Source ELN for Green and Sustainable Chemistry, 2023, https://nottingham-repository.worktribe.com/output/16802329 Search PubMed.
- S. Herres-Pawlis, F. Bach, I. J. Bruno, S. J. Chalk, N. Jung, J. C. Liermann, L. R. McEwen, S. Neumann, C. Steinbeck and M. Razum,
et al.
, Angew. Chem., Int. Ed., 2022, 61, e202203038 CrossRef CAS PubMed.
- R. Ghannam, S. Hussain, H. Fan and M. Á. C. González, IEEE Access, 2021, 9, 43241–43252 Search PubMed.
- S. Stall, L. Yarmey, J. Cutcher-Gershenfeld, B. Hanson, K. Lehnert, B. Nosek, M. Parsons, E. Robinson and L. Wyborn, Nature, 2019, 570, 27–29 CrossRef CAS PubMed.
- S. J. Coles, J. G. Frey, E. L. Willighagen and S. J. Chalk, Data Intelligence, 2020, 2, 131–138 CrossRef.
- K. Jeffery, P. Wittenburg, L. Lannom, G. Strawn, C. Biniossek, D. Betz and C. Blanchi, Data Intelligence, 2021, 3, 116–135 CrossRef.
- C. Tenopir, N. M. Rice, S. Allard, L. Baird, J. Borycz, L. Christian, B. Grant, R. Olendorf and R. J. Sandusky, PLoS One, 2020, 15, e0229003 CrossRef CAS PubMed.
- N. M. O'Boyle, M. Banck, C. A. James, C. Morley, T. Vandermeersch and G. R. Hutchison, J. Cheminf., 2011, 3, 33 Search PubMed.
-
SPEDAC, http://www-naweb.iaea.org/napc/physics/PS/Softwares/Spedac.htm, Accessed: 2022-10-27 Search PubMed.
-
XML: Extensible Markup Language, https://developer.mozilla.org/en-US/docs/Web/XML/XML_introduction, Accessed: 2022-10-27 Search PubMed.
-
JSON, https://www.json.org/json-en.html, Accessed: 2022-10-27 Search PubMed.
-
DublinCore, https://www.dublincore.org/, Accessed: 2022-10-27 Search PubMed.
-
DCAT, https://dcat.org/, Accessed: 2022-10-27 Search PubMed.
-
GitHub – MardaAlliance, https://github.com/marda-alliance/metadata_extractors, Accessed: 2023-01-26 Search PubMed.
-
Text Analytics Service – Intelligent Tagging, https://www.refinitiv.com/en/products/intelligent-tagging-text-analytics, Accessed: 2022-10-27 Search PubMed.
-
Ontotext – Semantic Tagging, https://www.ontotext.com/solutions/semantic-tagging/, Accessed: 2022-10-27 Search PubMed.
-
Chemical Tagger, https://chemicaltagger.ch.cam.ac.uk/, Accessed: 2022-10-27 Search PubMed.
-
Name Reaction Ontology, https://obofoundry.org/ontology/rxno.html, Accessed: 2022-10-27 Search PubMed.
-
Chemical Methods Ontology, https://obofoundry.org/ontology/chmo.html, Accessed: 2022-10-12 Search PubMed.
-
Molecular Process Ontology, https://obofoundry.org/ontology/mop.html, Accessed: 2022-10-27 Search PubMed.
- K. Degtyarenko, P. de Matos, M. Ennis, J. Hastings, M. Zbinden, A. McNaught, R. Alcántara, M. Darsow, M. Guedj and M. Ashburner, Nucleic
Acids Res., 2008, 36, D344–D350 CrossRef CAS PubMed.
-
Chemical Entities of Biological Interest, https://obofoundry.org/ontology/chebi.html, Accessed: 2022-10-27 Search PubMed.
- J. Hastings, L. Chepelev, E. Willighagen, N. Adams, C. Steinbeck and M. Dumontier, PLoS One, 2011, 6, e25513 CrossRef CAS PubMed.
-
Chemical Information Ontology, https://obofoundry.org/ontology/cheminf.html, Accessed: 2022-10-27 Search PubMed.
- P. Strömert, J. Hunold, A. Castro, S. Neumann and O. Koepler, Pure Appl. Chem., 2022, 605–622 CrossRef.
-
Welcome to the NCBO BioPortal | NCBO BioPortal, https://bioportal.bioontology.org/, Accessed: 2022-10-27 Search PubMed.
- M. Ashburner, C. A. Ball, J. A. Blake, D. Botstein, H. Butler, J. M. Cherry, A. P. Davis, K. Dolinski, S. S. Dwight, J. T. Eppig, M. A. Harris, D. P. Hill, L. Issel-Tarver, A. Kasarskis, S. Lewis, J. C. Matese, J. E. Richardson, M. Ringwald, G. M. Rubin and G. Sherlock, Nat. Genet., 2000, 25, 25 CrossRef CAS PubMed.
-
Gene Ontology – Summary | NCBO BioPortal, https://bioportal.bioontology.org/ontologies/GO, Accessed: 2022-10-27 Search PubMed.
- U. Visser, S. Abeyruwan, U. Vempati, R. P. Smith, V. Lemmon and S. C. Schürer, BMC Bioinf., 2011, 12, 257 CrossRef PubMed.
-
BioAssay Ontology – Summary | NCBO BioPortal, https://bioportal.bioontology.org/ontologies/BAO, Accessed: 2022-10-27 Search PubMed.
-
S. Kanza and J. G. Frey, Systems Medicine, Academic Press, Oxford, 2021, pp. 129–144 Search PubMed.
-
W3C, RDFa Core 1.1 – Third Edition, 2015, https://www.w3.org/TR/rdfa-core/, Accessed: 2018-12-07 Search PubMed.
-
W3C, HTML Standard, 2022, https://html.spec.whatwg.org/multipage/#toc-microdata, Accessed: 2022-04-26 Search PubMed.
-
W3C, JSON-LD 1.1, 2018, https://www.w3.org/2018/jsonld-cg-reports/json-ld/, Accessed: 2022-04-26 Search PubMed.
-
reMarkable, https://remarkable.com/, Accessed: 2022-10-27 Search PubMed.
-
BOOX, The Official BOOX Site, https://www.boox.com/, Accessed: 2022-10-27 Search PubMed.
-
Rocketbook – Smart Notebook – Reusable Notepads, https://getrocketbook.co.uk/, Accessed: 2022-10-27 Search PubMed.
-
LabTwin | The Leading Voice Powered Digital Lab Assistant, https://www.labtwin.com/, Accessed: 2022-10-13 Search PubMed.
-
LabVoice | voice-enabling scientific laboratories, https://www.labvoice.ai/, Accessed: 2022-10-27 Search PubMed.
- N. J. Knight, S. Kanza, D. Cruickshank, W. S. Brocklesby and J. G. Frey, IEEE Internet of Things Journal, 2020, 7, 8631–8640 Search PubMed.
- G. I. Lang and D. Botstein, PLoS One, 2011, 6, e25290 CrossRef CAS PubMed.
|
This journal is © The Royal Society of Chemistry 2023 |
Click here to see how this site uses Cookies. View our privacy policy here.