DOI:
10.1039/D2AY01771G
(Paper)
Anal. Methods, 2023,
15, 906-915
Quantitative and qualitative analyses of metal ions in food and water by using a multicolor sensor array and chemometrics†
Received
30th October 2022
, Accepted 13th December 2022
First published on 14th December 2022
Abstract
Rapid and accurate detection of toxic metal ions is the key to combating food contamination and environmental pollution. In sensor arrays, gold nanoparticles play a crucial role in monitoring metal ions based on surface plasmon resonance. However, identifying metal ions with unknown concentrations in a complex system through this assay is difficult because of its monotonous color change and weak anti-interference ability. To overcome these limitations, a sensitive, flexible, low-cost, and multicolor sensor array was designed herein. The applicability of the sensor array for the qualitative and quantitative analyses of metal ions in food and water was also verified. The developed sensor array could classify 14 metal ions (Cu2+, Fe2+, Fe3+, Mn2+, Ni2+, Zn2+, Cd2+, Cr3+, Co2+, Ba2+, K+, Tl+, Pb2+, and Hg2+) of unknown concentration with an accuracy of 100%. In addition, partial least squares models were established to quantify Tl+, Pb2+, and Hg2+ in water and rice samples, with square correlation coefficients (R2) of 0.9991, 0.9742, and 0.9731, respectively. This method can be used for accurate quantitative and qualitative analyses of heavy metal ions in water and food.
1. Introduction
Heavy metal ions are some of the major pollutants causing water contamination.1 Because of sewage irrigation, industrial wastewater discharge, and use of ineffective or damaged domestic filters, heavy metal ions accumulate in human bodies.2 Metal ions (Mn+) above a threshold limit are toxic, causing various life-threatening diseases. Therefore, methods for detecting metal contaminants in food and drinking water are urgently needed to allow their safe consumption.3,4
Quantitative and qualitative analyses of metal ions in food and water have been studied in-depth by using sophisticated techniques and instruments, such as atomic absorption spectroscopy (AAS), inductively coupled plasma atomic emission spectrometry (ICP-AES), and inductively coupled plasma-mass spectroscopy (ICP-MS).4,5 However, these techniques require expensive instrumentation, long analysis times, and complex operation procedures.6 In recent decades, nanotechnology-based colorimetry and biosensing platforms have emerged as viable technologies for the on-site detection of heavy metal ions.5,7,8 Gold nanoparticles (AuNPs) have played a prominent role in such systems because of the following advantages: (1) well-established synthesis routes; (2) capability for easy modification; (3) high stability; (4) low cost; (5) low toxicity; and (6) remarkable surface plasmon resonance (SPR) properties.9,10 SPR-based sensors, which employ visual read-out of a sensing result, have been widely developed for heavy metal ions analyses.11 However, these sensors can only identify different metal ions at the same concentration, making them unsuitable for practical applications in which the concentration of the metal ion is unknown.4 In addition, a highly selective aptamer is required to differentiate the metal ions; however, development of aptamers that bind to specific metal ions is challenging.12,13 Therefore, an aptamer-independent method that can distinguish various metal ions at different concentrations needs to be established. Optical sensor arrays are a promising approach to screening a wide range of analytes or mixtures. A colorimetric array enables the simultaneous detection of multiple analytes in a single test. The colorimetric signature of the array can be used to identify substances by their unique color combination. The technology can be customized by varying the array dimensions according to specific applications.2,14
In this study, AuNPs were employed to imitate horseradish peroxidase (HRP) activity such that the chromogenic substrate with a reductive state was converted to the chromogenic substrate with an oxidation state, leading to a color change. Considering that the analytical ability of a chromogenic array sensor depends on its color diversity, seven chromogenic substrates, namely, 4-chloro-1-naphthol (4-CN), 3-amino-9-ethylcarbazole (AEC), o-phenylenediamine (OPD), 2,2′-azinobis (3-ethylbenzthiazpline-6-sulfonic acid)-ammonium salt (ABTS), 3,3′-diaminobenzidine (DAB), benzidine, and 5-aminosalicylic acid (5-ASA), were used to build a sensor array. The color change of the chromogenic substrate is related to the catalytic activity of AuNPs, which depends on the composition, morphology, particle size, surface modification, valence change, charge, and charge density of the AuNPs. These AuNP properties can be regulated by the types and concentrations of metal ions. The color change of the chromogenic substrate can be recorded and correlated with the types and concentrations of the metal ions. Then, the information from the color photos, absorbance at the maximum absorption wavelength (at 605, 414, 443, 414, 559, 410, and 411 nm for 4-CN, AEC, OPD, ABTS, DAB, benzidine, and 5-ASA, respectively) and ultraviolet-visible (UV-Vis) spectra of the chromogenic solutions was used to establish multivariate calibration models to classify 14 metal ions (Cu2+, Fe2+, Fe3+, Mn2+, Ni2+, Zn2+, Cd2+, Cr3+, Co2+, Ba2+, K+, Tl+, Pb2+, and Hg2+). Based on multivariate analysis and the sensor array, the classification model could achieve 100% analytical accuracy. Finally, the principal component analysis-linear discriminant analysis (PCA-LDA) and partial least squares discriminant analysis (PLS-DA) were applied to identify the toxic metal ions in drinking water and rice. Meanwhile, the color map is the fingerprint that allows quantitative analysis of the specific metal ion. PLS models were also be built for quantitative Tl+, Pb2+, and Hg2+ in water and rice, and the R2 of PLS model are 0.9991, 0.9742 and 0.9731, respectively. The recovery of Tl+, Pb2+ and Hg2+ based on sensor array and PLS-DA models are 101.11%, 97.93% and 103.22%.
2. Experimental section
2.1 Materials and instruments
Hydrogen tetrachloroaurate(III) trihydrate (HAuCl4·3H2O, ≥99.99%), sodium citrate dihydrate (C6H5Na3O7·2H2O, ≥99%), CrCl3·6H2O, CuCl2·6H2O, FeCl2·4H2O, FeCl3·6H2O, MnCl2·4H2O, NiCl2·6H2O, ZnCl2, CdCl2·2.5H2O, CoCl3·6H2O, BaCl2·2H2O, KCl, Pb(NO3)2, and the mercury nitrate standard were purchased from Aladdin Industrial Corporation (Shanghai, China). TlNO3 was purchased from Sigma-Aldrich (St. Louis, MO, USA). Single-stranded DNAs (ssDNA, 5′-GTG GGT AGG GCG GGT TGG-3′ [T001], 5′-GGT TGG TGT GGT TGG-3′ [T002], 5′-GGG TGG GTG GGT GGG T-3′ [T003], 5′-GGG TAG GGC GGG TTG G-3′ [T004], and 5′-TTC TTT CCC CTT GTT TGT T-3′ [T005, Hg2+ aptamer]) were synthesized by Sangon Biotechnology Co. Ltd. (Shanghai, China). Analytical reagents were used without further purification, and ultrapure water provided by a Direct-Q3 system was used to freshly prepare all solutions.
The UV-Vis spectra were recorded on a UV-Vis spectrometer (UV5Nano Mettler Toledo Corporation). An oscillator (ST70-21ST60-4, Hangzhou Miu Instruments Co. Ltd.) was used to mix the solutions and heat them to 37 °C for 30 min.
2.2 Synthesis of AuNPs
AuNPs (13 nm) were synthesized by reducing a boiling solution of HAuCl4 using trisodium citrate. More details can be found in ref. 15. In accordance with the Beer–Lambert law, the concentration of AuNP colloid was estimated.
2.3 Sensor arrays
ssDNAs (T001, T002, T003, T004, and T005) were used as surface modification agents for the AuNPs. In our experiments, the recognition of metal ions based on different ssDNA are shown in Fig. S1.† The type of ssDNA did not affect the results of identifying metal ions in this study. Therefore, T002 (5′-GGT TGG TGT GGT TGG-3′) was selected for constructing the sensor array because it was easy to synthesize and did not spontaneously fold in solution.14 AuNPs (10 μL) and ssDNA (10 μL, 100 nM) were mixed and stored at 37 °C for 30 min. Next, Mn+ (10 μL, 10 μM) was added to the AuNPs–ssDNA solution and held at 37 °C for 10 min. Then, chromogenic solutions of 4-CN, AEC, OPD, ABTS, DAB, benzidine, and 5-ASA were added to the above mixed solution. After 30 min, the spectrum of each solution was recorded by UV-Vis spectrometry (Mettler Toledo Corporation) after the mixed solution was diluted to 600 μL with deionized water. Data analyses are described in the following section.
2.4 Chemometric methods
The entire dataset was randomly split into two subsets, i.e., a modeling set (about 80%) and an external test set (about 20%). The latent variables (LVs) and principal components (PCs) were selected using bootstrapping Latin partitions to build robust models. Moreover, the calibration models were evaluated by bootstrapping Latin partitions. With bootstrapping Latin partitions, the relative proportions of the class distributions were maintained between the training and prediction sets. The calibration model was evaluated in an unbiased manner because the dataset was randomly partitioned. Every object was used for prediction only once. To identify the metal ions, the evaluation of the classification model was based on the accuracy of cross validation and unassigned samples. To quantify the metal ions, the evaluation of the PLS model was based on the square correlation coefficient (R2), root-mean-square error of cross validation (RMSECV), and standard deviation-root-mean-square error of cross validation (SD-RMSECV) methods.
For building a model based on sensor array, data fusion was applied to construct an augmented matrix. The raw data of different sensor units directly construct a new dataset, which is low-level data fusion (data layer fusion).
PLS-DA and PCA-LDA were conducted using the classification toolbox (version 6.0), released by Milano Chemometrics and QSAR Research Group and shared at https://michem.disat.unimib.it/chm/. All model optimization and construction were carried out in MATLAB (The MathWorks Inc., USA).
2.5 Application
For practical applications, the metal ions in drinking water and rice were quantified using the developed sensor array. Tl+, Pb2+, and Hg2+ in drinking water and rice were also detected. Because the concentrations of Tl+, Pb2+ and Hg2+ were negligible in actual samples, a known amount of the analytes was spiked to the samples for measurement.
3. Results and discussion
3.1 Mechanisms
HRP-catalyzed coloration is an important diagnostic technique because of its straightforward labeling principle and small sample size.16,17 AuNP-based functional catalysts have gained significant interest recently because they can catalyze many reactions with industrial and environmental significance.18 The advantages of catalytically active nanomaterials (nanozymes) over natural enzymes include their controlled synthesis, low-cost, tunable catalytic properties, high effectiveness, and high stability under stringent conditions.19,20 In analytical chemistry, enzyme mimetic AuNPs can catalyze the reaction between 3,3′,5,5′-tetramethylbenzidine (TMB) and H2O2 to generate TMBOX with a color change (from light blue to dark blue). However, the application of this oxidation reaction is limited because of the monotonous color change.14 Several reports have revealed the strong dependence of the enzyme-like properties of AuNPs on their size, structure, morphology, surface modification, charge, and charge density.21–23 Our sensor unit is based on a non-crosslinking interaction mechanism. ssDNA can be absorbed on the surfaces of citrate-capped AuNPs by complexation.1 The surface AuNPs–ssDNA is negatively charged. When metal ions added into the AuNPs–ssDNA solution, they interact with ssDNA. Some metal ions (such as Hg2+, Pb2+, and Cr3+) form complexes with ssDNA, leading to the aggregation of AuNPs–ssDNA and consequently altering the size, structure, and morphology of the AuNPs.8,24–26 Some metal ions (such as K+, Ca2+, and Cu2+) adsorb to the surface of AuNPs–ssDNA owing to electrostatic action, resulting in changes of the surface modification, charge, and charge density of AuNPs.13,27,28 These properties of AuNPs can be regulated by different metal ion types and concentrations, which can be reflected by the color of the chromogenic substrate. Therefore, the color of the chromogenic substrate can be used for quantitatively and qualitatively analyzing metal ions based on the enzyme-like properties of AuNPs. In this study, seven chromogenic substrates (4-CN, AEC, OPD, ABTS, DAB, benzidine, and 5-ASA) were employed to build a sensor array for analyzing metal ions. 4-CN, AEC, OPD, ABTS, DAB, benzidine, and 5-ASA have different colors, as well as varying sensitivities and selectivities toward AuNPs–ssDNA–Mn+. First, AuNPs were modified by ssDNA, and the resulting AuNPs–ssDNA comprises several exposed nitrogenous ssDNA assimilated on the surfaces for metal ion binding. The ssDNA-modified AuNPs do not confer higher peroxidase catalytic ability (see Fig. S1†). The AuNPs–ssDNA combined with different metal ions exhibit different peroxidase catalysis abilities and allow the oxidation of the chromogenic substrates with H2O2, resulting in color change for analyzing metal ions (see Fig. 1A). Meanwhile, different ssDNAs were used to enhance the selectivity of the array. However, AuNPs–ssDNA–Mn+ with different ssDNAs have same color maps against different metal ions (the results shown in Fig. S2†). Finally, T002 was selected for constructing the array sensor because it was easy to synthesize and did not spontaneously fold in solution. Metal ions have been reported as catalytic active sites in many literature.25,29 To verify whether metal ions or AuNPs–ssDNA–Mn+ play a catalytic role in our experiment, metal ions (without AuNPs–ssDNA) were added to the chromogenic substrate (shown in Fig. S3†). Fig. S3† indicate that a few metal ions (Cu2+, Fe3+, and Cr3+) have catalytic activity, which cannot identify 14 metal ions. Therefore, the multi-color array sensor based on peroxidase catalytic ability of AuNPs–ssDNA–Mn+ can successfully analyze metal ions. The color map based on different peroxidase catalysis ability of AuNPs–ssDNA–Mn+ can identify metal ions, and the color degree of sensors can be used for quantitative analyses.
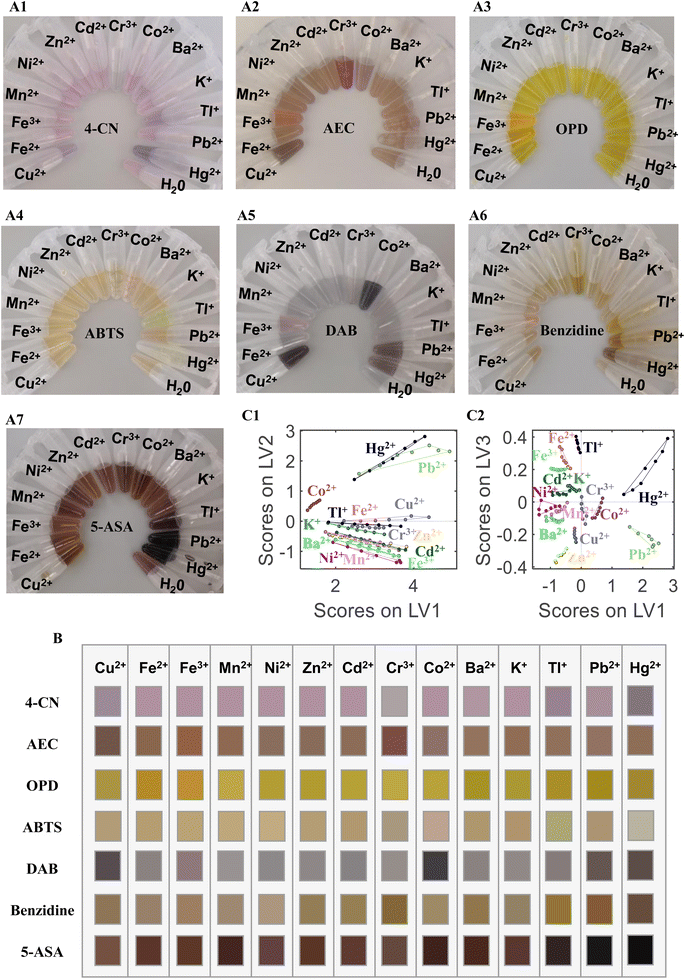 |
| Fig. 1 (A) Photographs of the colorimetric sensor array used to detect 14 metal ions (10 μM) based on seven sensor units: (A1) 4-CN, (A2) AEC, (A3) OPD, (A4) ABTS, (A5) DAB, (A6) benzidine, and (A7) 5-ASA. (B) Color map corresponding to (A). (C) Scatter plots of the score combination of different LVs included in the PLS-DA model: (C1) LV 1 vs. LV 2, and (C2) LV 1 vs. LV 3. | |
3.2 Identification of metal ions based on the color map
Fourteen metal ions (Cu2+, Fe2+, Fe3+, Mn2+, Ni2+, Zn2+, Cd2+, Cr3+, Co2+, Ba2+, K+, Tl+, Pb2+, and Hg2+) were employed to test the identification ability of the sensor array. Fig. 1 shows the photograph of the sensor array response toward the 14 metal ions (10 μM each) based on seven sensor units (A1) 4-CN, (A2) AEC, (A3) OPD, (A4) ABTS, (A5) DAB, (A6) benzidine, and (A7) 5-ASA. All sensor units exhibited different colors when AuNPs–ssDNA was mixed with different metal ions because the catalytic activity of the AuNPs–ssDNA was regulated by the metal ions. Based on the color change of the chromogenic substrate, the catalytic activity of AuNPs–ssDNA can classify the metal ions into different groups. Fig. 1B shows the color map corresponding to Fig. 1A. The color variation suggests that our sensor array contains fingerprint information for identifying the metal ions. This system can be used for the on-site detection of metal ions based on the color reaction and can be observed by the naked eye. To further prove the detection ability, the photographs of the colorimetric response were taken by a smartphone (HUAWEI P20), and PLS-DA was employed to classify the 14 metal ions using the photographs. The error rate of cross validation (CV) was used to select the number of LVs. When the number of LVs was 3, both the error rate of CV and unassigned samples reached the minimum. Fig. 1C shows the scatter plot of the score combinations of LV 1 vs. LV 2 and LV 1 vs. LV 3 included in the PLS-DA model, in which each metal ion forms a tight cluster with a substantial separation from the other clusters. These results demonstrate the feasibility of the chromogenic sensor array for metal ion discrimination by using the peroxidase-like activity of AuNPs.
3.3 Identification of metal ions based on the absorbance at the maximum absorption wavelength
Classification of metal ions at different concentrations using PLS-DA models was difficult to achieve by using photographs of our sensor array. To improve the identification ability, the absorbance at the maximum absorption wavelength (at 605, 414, 443, 414, 559, 410, and 411 nm for 4-CN, AEC, OPD, ABTS, DAB, benzidine, and 5-ASA, respectively) was measured by a UV-Vis spectrometer. In Fig. 2A, the absorbance of the chromogenic solution formed a fingerprint map for each metal ion (10 μM). The classification model was established using PLS-DA and PCA-LDA. To improve the robustness of the model, mean centering, autoscaling, variance scaling, and range scaling were conducted to preprocess the variables. The results of the PLS-DA and PCA-LDA models are listed in Table 1.
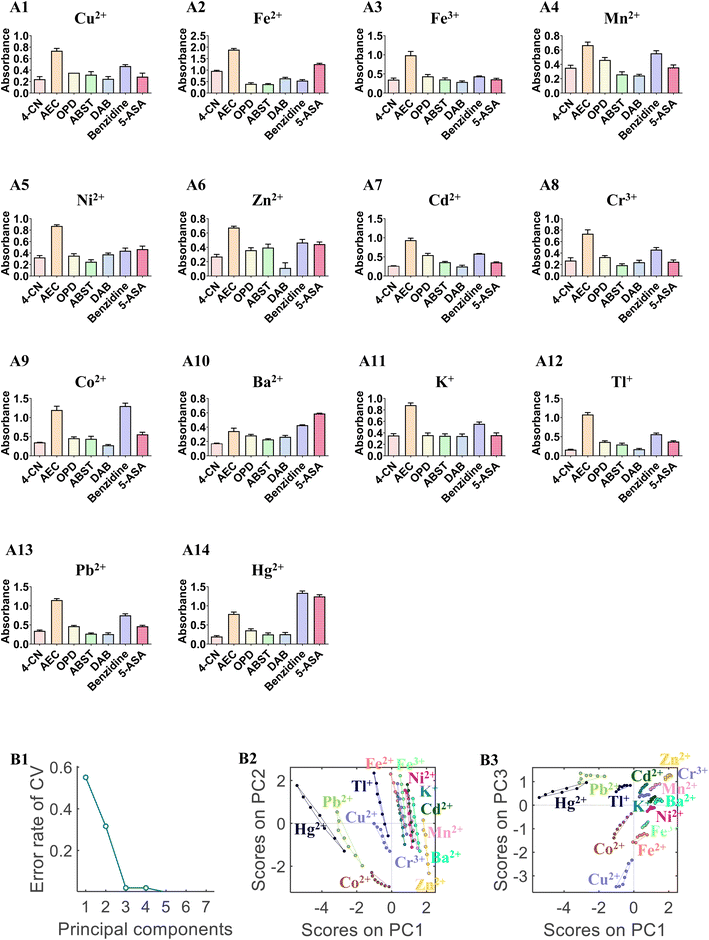 |
| Fig. 2 (A) Absorbance at the maximum absorption wavelength in the colorimetric sensor array (4-CN, AEC, OPD, ABTS, DAB, benzidine, and 5-ASA) in the presence of 14 metal ions (10 μM) (A1) Cu2+, (A2) Fe2+, (A3) Fe3+, (A4) Mn2+, (A5) Ni2+, (A6) Zn2+, (A7) Cd2+, (A8) Cr3+, (A9) Co2+, (A10) Ba2+, (A11) K+, (A12) Tl+, (A13) Pb2+, and (A14) Hg2+. (B) Results of PCA-LDA models based on the absorbance at the maximum absorption wavelength of the colorimetric sensor array, (B1) cross-validation error rate as a function of the number of PCs; scatter plots of the score combination of different components included in the PCA-LDA model: (B2) PC 1 vs. PC 2, and (B3) PC 1 vs. PC 3. | |
Table 1 Classification results based on the absorbance at the maximum absorption wavelength of the sensor array
Pre-processing |
PCA-LDA models |
PLS-DA models |
PCs |
Error rate of CV |
LVs |
Error rate of CV |
Rate of unassigned samples |
None |
6 |
0.01 |
7 |
0.29 |
0.64 |
Mean centering |
6 |
0.01 |
6 |
0.57 |
0.69 |
Autoscaling |
5 |
0 |
6 |
0.35 |
0.66 |
Variance scaling |
5 |
0 |
6 |
0.50 |
0.79 |
Range scaling |
6 |
0 |
6 |
0.64 |
0.78 |
The PLS-DA and PCA-LDA models can distinguish all metal ions with accuracies of 71.00% and 100.00%, respectively. The most robust model is displayed in Fig. 2B. The error rate of CV was used to select the number of PCs. The final PCA-LDA model was calculated by selecting 5 PCs and 5 CV groups for internal validation. When 5 PCs were selected, 100% variance was explained, and the predicted accuracy of the PCA-LDA model was 100%. Fig. 2B2 and B3 display the scatter plots of different component scores. The plots of couples PC 1 vs. PC 2 or PC 1 vs. PC 3 exhibited better clusters graphically.
Therefore, the method can identify metal ions at different concentrations using absorbance at the maximum absorption wavelength in a viable manner.
3.4 Identification of metal ions based on UV-Vis spectra
Compared with the absorbance at the maximum absorption wavelength, the UV-Vis spectra provide more fingerprint information related to the identification ability of the method, especially in a complex system. The UV-Vis spectra of various chromogenic solutions (4-CN from 400 nm to 800 nm, AEC from 400 nm to 700 nm, OPD from 350 nm to 800 nm, ABTS from 400 nm to 750 nm, DAB from 500 nm to 800 nm, benzidine from 350 nm to 800 nm, and 5-ASA from 400 nm to 800 nm) were measured to classify the 14 metal ions. In Fig. 3A, the spectra of the chromogenic solution were different for each metal ion (10 μM). The classification model was established using PLS-DA and PCA-LDA based on the UV-Vis spectra. To improve the robustness of the model, the standard normal variate (SNV), multiplicative scatter correction (MSC), and the first and second derivatives were used to preprocess the UV-Vis spectra. The results of PLS-DA and PCA-LDA models based on UV-Vis spectra are listed in Table 2. All 14 metal ions can be distinguished by the PLS-DA and PCA-LDA models with 100.00% accuracy. The results indicate that the classification models based on the UV-Vis spectra are more robust than those based on absorbance at the maximum or based on photographs. The most robust PLS-DA model is displayed in Fig. 3B. Although the first seven LVs can be explained more variably, the scatter plot of LV2 vs. LV4 shows better clusters graphically, indicating that the PLS-DA model can classify each metal ion into different groups under high dimensions.
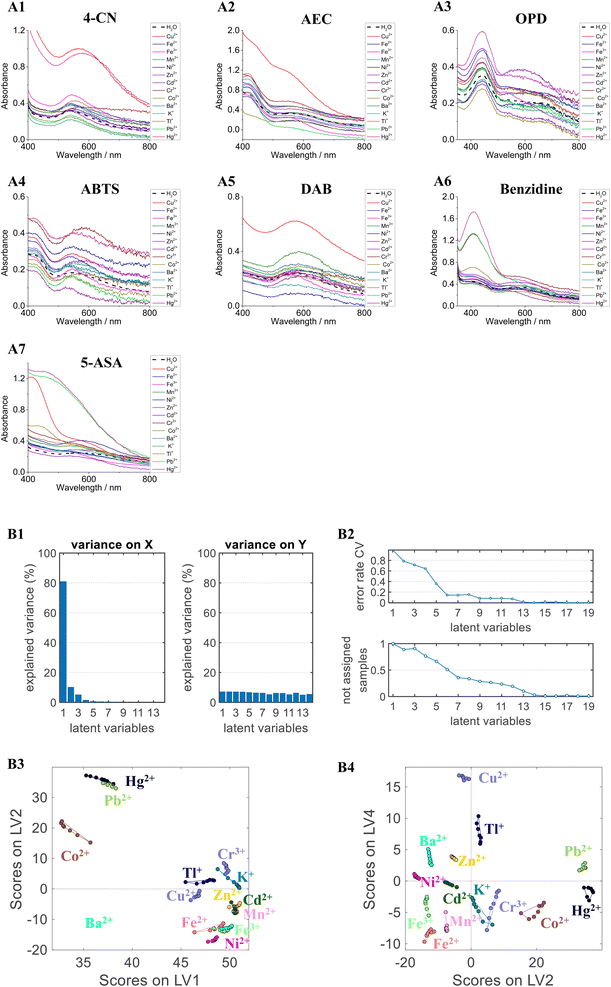 |
| Fig. 3 UV-Vis spectra for the classification of 14 metal ions (10 μM) based on seven sensor units: (A1) 4-CN, (A2) AEC, (A3) OPD, (A4) ABTS, (A5) DAB, (A6) benzidine, and (A7) 5-ASA. (B) Results of the PLS-DA models based on UV-Vis spectra of the colorimetric sensor array, (B1) explained variance on X (spectra) and explained variance on Y (metal ions type) as a function of the number of LVs; (B2) error rate of CV and the rate of unassigned samples as a function of the number of LVs; scatter plots of the score combination of different LVs included in the PLS-DA model: (B3) LV 1 vs. LV 2, and (B4) LV 2 vs. LV 4. | |
Table 2 Classification results based on the UV-Vis spectra of the sensor array
Pre-processing |
PCA-LDA models |
PLS-DA models |
PCs |
Error rate of CV |
LVs |
Error rate of CV |
Rate of unassigned samples |
None |
4 |
0.01 |
17 |
0 |
0 |
SNV |
8 |
0 |
14 |
0 |
0 |
MSC |
7 |
0 |
14 |
0.01 |
0 |
First derivative |
7 |
0.04 |
10 |
0.37 |
0.19 |
Second derivative |
3 |
0.12 |
10 |
0.37 |
0.25 |
3.5 Quantitation of Tl+, Pb2+, and Hg2+
According to the above-mentioned experimental results, our sensor array can identify metal ions using fingerprint information. Thus, we speculate that this assay can be used to quantitatively analyze specific metal ions. Tl+, Pb2+, Hg2+ and their compounds are some of the most toxic heavy-metal species, with poisoning events and environmental pollution posing serious harm to human health. Because Tl+, Pb2+, and Hg2+ salts are colorless and tasteless, they are difficult to detect. Thallium salts perform a primary role in toxic poisoning or terrorist attacks in many countries. Lead and mercury salts are the most common highly toxic metal ions in environmental pollution. At present, no standard clinical treatments against Tl+, Pb2+, and Hg2+ salts poisoning exist. Therefore, the rapid and precise detection of these ions is essential in preventing water poisoning and food contamination. Herein, our sensor array was employed to quantify toxic Tl+, Pb2+, and Hg2+ in various samples.
The UV-Vis spectra of Tl+, Pb2+, and Hg2+ collected from each sensor unit are shown in Fig. 4A–C. According to the UV-Vis spectra, PLS models were constructed using the data fusion dataset for quantitative analysis (Fig. 4D). Fig. 4D also provides the precision described by the 95% confidence interval obtained by 10 bootstraps. The predicted values of ion concentrations are highly correlated to the reference values in the PLS model. The RMSECV values of Tl+, Pb2+, and Hg2+ were 1.52 ± 0.07, 3.23 ± 0.02, and 7.44 ± 0.05 μM, with R2 values of 0.9991, 0.9742, and 0.9731, respectively. Based on the International Union of Pure and Applied Chemistry (IUPAC) definition, the limits of detection (LODs) of Tl+, Pb2+, and Hg2+ were calculated as 36.87, 26.55, and 20.35 nM, respectively. The linear dynamic ranges of Tl+, Pb2+, and Hg2+ were 10–100, 2–40, and 3–60 μM, respectively. The different forces between metal ions (Tl+, Pb2+, and Hg2+) and ssDNA (T002) lead to different linear dynamic ranges of quantitative experiments.
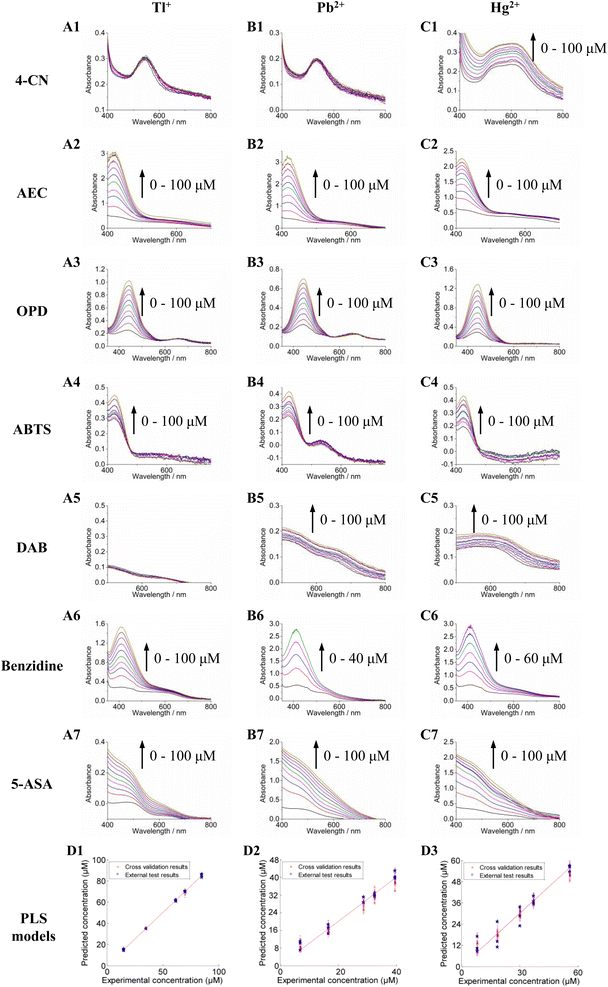 |
| Fig. 4 UV-Vis spectra for the quantification of Tl+ (A), Pb2+ (B), and Hg2+ (C) (each metal ion concentration from 0 to 100 μM) based on seven sensor units: (1) 4-CN, (2) AEC, (3) OPD, (4) ABTS, (5) DAB, (6) benzidine, and (7) 5-ASA. (D) Results of the PLS models based on the UV-Vis spectra of the colorimetric sensor array for the quantitative analyses of Tl+ (D1), Pb2+ (D2), and Hg2+ (D3). | |
3.6 Application
This work aims to develop a colorimetric sensor array for quantitative and qualitative analyses of metal ions in water and food. Drinking water and rice samples were used to demonstrate the identification ability of this sensor array for practical application. The accuracies of the predictive results obtained from the PCA-LDA (based on the absorbance at the maximum absorption wavelength) and PLS-DA models (based on the UV-Vis spectra) were 98.46% and 100%, respectively. The classification results indicate that our sensor array can identify metal ions in water and rice. To demonstrate quantitation capability, Tl+, Pb2+, and Hg2+ in drinking water and rice were analyzed using the PLS models. Recoveries based on the PLS-DA models were 101.11%, 97.93%, and 103.22%. The quantitation results indicate that this method possesses excellent identification ability and reproducibility for the quantitative analysis of Tl+, Pb2+ and Hg2+ in water and food.
4. Conclusion
In this study, we designed a multicolor sensor array based on the peroxidase-like activity of AuNPs and chemometrics. Color image, absorbance at the maximum absorption wavelength, and UV-Vis spectra were utilized to determine the peroxidase-like activity of AuNPs–ssDNA–Mn+. Chemometric methods were applied to analyze the collected information for qualitative and quantitative analyses of the metal ions. The 14 metal ions at 10 μM were classified with 100% accuracy by the PLS-DA model using the color reaction photos. The PCA-LDA models based on the absorbance at the maximum absorption wavelength and based on the UV-Vis spectra could classify the 14 metal ions at different concentrations with 100% accuracy. In addition, Tl+, Pb2+, and Hg2+ were detected in water samples with a LOD at the micromole level. Because of its identification ability, this sensor array can be extended to the detection of metal ions other than those discussed in this study.
Conflicts of interest
There are no conflicts to declare.
Acknowledgements
This work was supported by the Special Fund for Military Science (grant number BWS16J007, AWS17J009).
References
- Z. Li, J. R. Askim and K. S. Suslick, Chem. Rev., 2019, 119, 231–292 CrossRef CAS PubMed.
- L. Huang, L. Wang, Z. Nie and Y. Wang, Food Chem., 2020, 319, 126543 CrossRef CAS PubMed.
- L. Huang, X. Zhang and Z. Zhang, Anal. Chim. Acta, 2018, 1044, 119–130 CrossRef CAS PubMed.
- E. Priyadarshini and N. Pradhan, Sens. Actuators, B, 2017, 238, 888–902 CrossRef CAS.
- N. Ratnarathorn, O. Chailapakul and W. Dungchai, Talanta, 2015, 132, 613–618 CrossRef CAS PubMed.
- L. Zhang, D. Peng, R.-P. Liang and J.-D. Qiu, TrAC, Trends Anal. Chem., 2018, 102, 280–289 CrossRef CAS.
- L. Yu, L. Zhang, G. Ren, S. Li, B. Zhu, F. Chai, F. Qu, C. Wang and Z. Su, Sens. Actuators, B, 2018, 262, 678–686 CrossRef CAS.
- W. Chen, F. Cao, W. Zheng, Y. Tian, Y. Xianyu, P. Xu, W. Zhang, Z. Wang, K. Deng and X. Jiang, Nanoscale, 2015, 7, 2042–2049 RSC.
- D. Liu, Z. Wang and X. Jiang, Nanoscale, 2011, 3, 1421–1433 RSC.
- Z. Krpetic, L. Guerrini, I. A. Larmour, J. Reglinski, K. Faulds and D. Graham, Small, 2012, 8, 707–714 CrossRef CAS PubMed.
- L. Huang, X. Zhang and Z. Zhang, Anal. Chim. Acta, 2018, 1044, 119–130 CrossRef CAS PubMed.
- V. K. Upadhyayula, Anal. Chim. Acta, 2012, 715, 1–18 CrossRef CAS PubMed.
- Z. Wang and L. Ma, Coord. Chem. Rev., 2009, 253, 1607–1618 CrossRef CAS.
- L. Huang, L. Xiang, Y. Zhang, Y. Wang and Z. Nie, Spectrochim. Acta, Part A, 2020, 238, 118392 CrossRef CAS PubMed.
- J. Turkevich, P. C. Stevenon and J. Hiller, Spec. Discuss. Faraday Soc., 1951, 11, 55–75 RSC.
- A. K. Dutta, S. Das, S. Samanta, P. K. Samanta, B. Adhikary and P. Biswas, Talanta, 2013, 107, 361–367 CrossRef CAS PubMed.
- Y. L. Liu, X. J. Zhao, X. X. Yang and Y. F. Li, Analyst, 2013, 138, 4526–4531 RSC.
- L. Fan, D. Lou, Y. Zhang and N. Gu, Analyst, 2014, 139, 5660–5663 RSC.
- A. A. Vernekar, T. Das, S. Ghosh and G. Mugesh, Chem.–Asian J., 2016, 11, 72–76 CrossRef CAS PubMed.
- A. L. Hu, H. H. Deng, X. Q. Zheng, Y. Y. Wu, X. L. Lin, A. L. Liu, X. H. Xia, H. P. Peng, W. Chen and G. L. Hong, Biosens. Bioelectron., 2017, 97, 21–25 CrossRef CAS PubMed.
- H. H. Deng, G. L. Hong, F. L. Lin, A. L. Liu, X. H. Xia and W. Chen, Anal. Chim. Acta, 2016, 915, 74–80 CrossRef CAS.
- Y. Zhang, C. Dai, W. Liu, Y. Wang, F. Ding, P. Zou, X. Wang, Q. Zhao and H. Rao, Mikrochim. Acta, 2019, 186, 340 CrossRef PubMed.
- S. Ge, M. Sun, W. Liu, S. Li, X. Wang, C. Chu, M. Yan and J. Yu, Sens. Actuators, B, 2014, 192, 317–326 CrossRef CAS.
- X. Zheng, Q. Liu, C. Jing, Y. Li, D. Li, W. Luo, Y. Wen, Y. He, Q. Huang, Y. T. Long and C. Fan, Angew. Chem., Int. Ed., 2011, 50, 11994–11998 CrossRef CAS PubMed.
- Z. Chen, C. Zhang, Q. Gao, G. Wang, L. Tan and Q. Liao, Anal. Chem., 2015, 87, 10963–10968 CrossRef CAS PubMed.
- Y. Guo, Z. Wang, W. Qu, H. Shao and X. Jiang, Biosens. Bioelectron., 2011, 26, 4064–4069 CrossRef CAS PubMed.
- L. Tan, Z. Chen, Y. Zhao, X. Wei, Y. Li, C. Zhang, X. Wei and X. Hu, Biosens. Bioelectron., 2016, 85, 414–421 CrossRef CAS PubMed.
- Y. Wang, D. Li, W. Ren, Z. Liu, S. Dong and E. Wang, Chem. Commun., 2008, 2520–2522, 10.1039/b801055b.
- J. Dong, H. Zhao, F. Zhou and B. Li, Anal. Bioanal. Chem., 2016, 408, 1863–1869 CrossRef CAS PubMed.
|
This journal is © The Royal Society of Chemistry 2023 |
Click here to see how this site uses Cookies. View our privacy policy here.