DOI:
10.1039/D2AY01549H
(Paper)
Anal. Methods, 2023,
15, 916-924
Miniprep assisted proteomics (MAP) for rapid proteomics sample preparation†
Received
23rd September 2022
, Accepted 28th October 2022
First published on 14th November 2022
Abstract
Complete enzymatic digestion of proteins for bottom-up proteomics is substantially improved by use of detergents for denaturation and solubilization. Detergents however, are incompatible with many proteases and highly detrimental to LC-MS/MS. Recently; filter-based methods have seen wide use due to their capacity to remove detergents and harmful reagents prior to digestion and mass spectrometric analysis. We hypothesized that non-specific protein binding to negatively charged silica-based filters would be enhanced by addition of lyotropic salts, similar to DNA purification. We sought to exploit these interactions and investigate if low-cost DNA purification spin-filters, ‘Minipreps,’ efficiently and reproducibly bind proteins for digestion and LC-MS/MS analysis. We propose a new method, Miniprep Assisted Proteomics (MAP), for sample preparation. We demonstrate binding capacity, performance, recovery and identification rates for proteins and whole-cell lysates using MAP. MAP recovered equivalent or greater protein yields from 0.5–50 μg analyses benchmarked against commercial trapping preparations. Nano UHPLC-MS/MS proteome profiling of lysates of Escherichia coli had 99.3% overlap vs. existing approaches and reproducibility of replicate minipreps was 98.8% at the 1% FDR protein level. Label Free Quantitative proteomics was performed and 91.2% of quantified proteins had a %CV <20% (2044/2241). Miniprep Assisted Proteomics can be performed in minutes, shows low variability, high recovery and proteome depth. This suggests a significant role for adventitious binding in developing new proteomics sample preparation techniques. MAP represents an efficient, ultra-low-cost alternative for sample preparation in a commercially obtainable device that costs ∼$0.50 (USD) per miniprep.
Introduction
Effective sample preparation prior to LC-MS based proteomics requires the successful removal of all MS-incompatible reagents and contaminants while achieving reproducible and complete proteolytic digestion.1–3 Detergents, like sodium dodecyl sulfate (SDS), are ideal for the solubilization of aggregates and hydrophobic membrane proteins and the complete denaturation of polypeptides.4–6 However, detergents are typically incompatible with enzymatic digestion, and can cause signal suppression, adduct formation, column poisoning, and other instrumentation issues at even the smallest concentrations.7–9 Because of this, many bottom-up proteomic preparations avoid the use of detergents, leading to incomplete digests and poor accuracy.10–12 Some methods utilizing SDS require time-consuming or costly protocols to remove it via ion-exchange, filtration, or other means.13,14 Due to the growing use of SDS in bottom-up proteomic workflows, there is a need to develop robust low-cost methods that incorporate SDS removal from protein mixtures.
Filter-based methods are commonly used in bottom-up preparations because they allow for reliable detergent removal and protein isolation.15,16 Filters act as ‘reactor chambers’ where proteins are stored, detergents are removed via buffer exchange, and enzymatic digestion can occur (e.g. FASP).17 Early protocols stored proteins on molecular weight cutoff (MWCO) filters.18,19 Repetitive washes with urea disrupts protein–SDS micelles, and removes detergents and other harmful reagents as flow-through.17,18 However, this process requires time-consuming wash steps, and filters have shown to be inconsistent.20–22 More recent methods employ quartz or silica filters to trap proteins from a fine suspension.23 Protein-trapping using quartz or glass can be carried out in a fraction of the time and effort required for MWCO preparations.24 These filters can be costly, especially in resource-limited settings or when hundreds of samples need analysis. Silica filter traps can be inexpensively reproduced in-house for an ‘economical proteomics’ approach.25 However, these don't account for labor costs, and inherit inconsistencies passed down in ‘home-built’ devices. At present, no inexpensive; commercially available filter-trap for proteomics exists.
Protein binding and adsorption to glass or silica surfaces is well studied.26–28 These interactions occur rapidly and are influenced by temperature, pH, ionic strength, as well as the biochemical properties of protein.29–32 Johnson et al., demonstrated that at adequate ionic strength, precipitated protein will readily and non-specifically bind to glass beads for proteomics sample preparation.33 The conditions that drive protein binding to silica in solution appear similar to earlier strategies employed to optimize nucleic acid binding to silica substrates. Nucleic acid purifications are facilitated by binding to a silica surface bridged with lyotropic salts at a pH which maintains the protonation state of silanes.34–36 We hypothesized the strategies employed for nucleic acid purification via silica would readily optimize for protein-binding and digestion, and the spin filters used to purify nucleic acids can also be used to purify proteins for bottom-up proteomic studies without alteration. This is significant to the field, because spin filters used for DNA purification are commercially available, inexpensive, and potentially offer quicker detergent and salt removal than traditional bottom-up proteomic approaches.
In this study, we develop and optimize a strategy based on the chemical properties of DNA binding to silica substrates and instead utilize it to exploit adventitious protein binding to silica for rapidly isolating proteins from a mixture prior to bottom-up proteomic analysis. We are calling this method Miniprep Assisted Proteomics (MAP). Samples are denatured, reduced and alkylated in a buffered SDS solution before the addition of ammonium acetate to increase the ionic strength prior to binding on DNA Miniprep spin filters. In total it takes less than 15–30 minutes to prepare proteins for enzymatic digestion of which only 1–3 minutes is spent loading and spinning the minipreps. Sodium acetate, chlorate or guanidine thiocyanate are examples of lyotropic salts more commonly used to mediate DNA-silica binding, we investigated several of these including kosmotropic salts (data not shown). We quickly selected ammonium acetate as it is volatile and less hazardous than other options.36 Ammonium acetate was buffered to pH 5 because proteins exhibit stronger binding to silica below their isoelectric points.37
We determined the protein loading capacity, digestive efficiency, and reproducibility of MAP as compared to S-Traps. S-Traps are a benchmark trapping-methods for comparison and have been demonstrated high proteomic coverage relative to alternatives.38–41 Capacity was determined using digests of bovine serum albumin (BSA) followed by MALDI-TOF MS analysis and whole cell lysates of Escherichia coli (E. coli). Precision, accuracy, and suitability were determined using Escherichia coli (E. coli) lysates prepared with MAP and S-Traps in parallel. Nano UHPLC-MS/MS and Label Free Quantification (LFQ) [Flash-LFQ] in MetaMorpheus of the resulting peptide fragments was used to quantify protein yield and reproducibility across technical and biological triplicate samples.42,43 This is the first work to provide quantitative analysis of inexpensive DNA Miniprep spin filters as tools for filter-based protein digests.
Experimental
Reagents and equipment
LB broth and LB agar were from Amresco (Solon, OH). Urea was from Teknova (Hollister, CA). LC-MS pure reagents (water, ethanol, acetonitrile, and methanol) were purchased from J.T. Baker (Radnor, PA). Gel-electrophoresis reagents were from Bio-Rad (Berkley, CA). Iodoacetamide (IAA) was purchased from MP Biomedicals (Solon. OH). All other reagents are from Sigma-Aldrich (St. Louis, MO) unless specified. The gels used for electrophoresis were from Genscript Biotech Corporation (Piscataway, NJ). Separation occurred on a Mighty Small II from Hoefer Inc. (Holliston, MA) and gels were imaged with a Bio-Rad Gel-Doc EZ Imager. DNA Miniprep spin filters were purchased from Bioneer Corporation (Oakland, CA) and the S-Trap mini devices were from Protifi (Huntington, NY). At the time of publication 200 Miniprep columns from Bioneer have a US price of $115 including DNA reagents and bulk miniprep columns from Epoch Life Science (http://www.epochlifescience.com/) can be obtained for $0.42 each without reagents. Hydrophilic–lipophilic balance (HLB) solid phase extraction (SPE) cartridges (10 mg) from Waters (Milford, MA) were used to desalt peptide samples prior to analysis on a Q-Exactive HF from Thermo Fisher Scientific (San Jose, CA) or an ultrafleXtreme MALDI-TOF from Bruker Scientific LLC (Billerica, MA).
Bacteria culture and protein extraction
Single colonies of E. coli MG1655 were struck to LB agar and incubated overnight at 37 °C. A single colony was selected and placed into 5 mL of LB broth and incubated, shaking at 37 °C overnight (250 rpm). Cells were diluted 1
:
100 with fresh LB media in an Erlenmeyer flask and incubated until an OD600 0.5 was reached (∼3–4 hours). Cultures were centrifuged at 5000 × g for 10 min at 4 °C to pellet bacteria. LB broth was decanted and the cell pellet was re-suspended in cold PBS before being centrifuged again at 5000 × g for 10 min at 4 °C. This was repeated again, and the cells were pelleted and stored at −80 °C until lysis. The cell pellet was re-suspended in 1 mL of lysis buffer consisting of 50 mM Tris HCl (pH 7.5), 150 mM sodium chloride, 1 mM phenylmethylsulfonyl fluoride (PMSF), 1% SDS, and 0.1% Triton X-100. Cells were lysed with a bead beater (BioSpec) in three thirty-second intervals with 0.5 mL 0.1 mm zirconia beads. Samples were placed on ice between intervals. Cells and debris were clarified by centrifugation at 12
000 × g for 12 min. Protein concentration was determined via BCA assay (Thermo Scientific).
MAP sample preparation
Protein samples; 5 mg BSA or 1.0 mg E. coli lysate (capacity experiments) (50 μg nLC-MS/MS) were dissolved in 50 mM ammonium bicarbonate (ABC) and 6% SDS to ∼2 mg mL−1. Proteins were reduced with 10 mM tris(2-carboxyethyl)phosphine (TCEP) and denatured at 95 °C for 3 min. Alkylation was performed by addition of IAA to 10 mM and proceeded in the dark for 30 min. Following alkylation, 1
:
10 v/v 5 M ammonium acetate pH 5.0 was added (to 0.5 M) and 0.5–500 μg of protein was aliquoted to separate microcentrifuge tubes. Samples were diluted to maintain a constant volume of 50 μL in 50 mM ABC. A seven-fold further addition of 95
:
5 methanol
:
500 mM ABC was performed to maximize non-specific binding.33 Samples were then pipetted onto a Bioneer DNA Miniprep spin column and centrifuged at 1200 × g for 30 s. The flow-through was saved for PAGE analysis. Bound samples were washed twice with the 95
:
5 methanol–buffer and spun as above. Wash steps were collected for PAGE analysis. Trypsin was resuspended in a 50 mM ABC buffer and added on the filter at a 1
:
50 (enzyme
:
protein w/w) ratio, additional ABC was added to cover miniprep (∼50 μL), then incubated for 8 hours at 37 °C. After digestion, samples were centrifuged to collect flow-through (1200 × g for 30 s) and peptides were further eluted with washes of 100 μL 0.1% formic acid in water and 150 μL 0.2% formic acid in 1
:
1 acetonitrile and water. Peptide solutions were concentrated to near dryness in a vacuum centrifuge (SP Genevac miVac, Warminster, PA) and then re-suspended in 0.1% formic acid in water prior to desalting via solid phase extraction (performed as in Cronin et al.)44 and dried prior to mass spectrometric analysis.
Capacity testing/electrophoresis
Flow-through and washes were dried down in a vacuum concentrator and resuspended in SDS-PAGE 5× sample loading buffer. Tris-MOPS-SDS gels were used; 20 μL of sample was loaded and 20 μL of intact BSA control (1 mg mL−1) or E. coli lysate (1 mg mL−1) was loaded as a control. Electrophoresis occurred at 150 V for 60 min. Gels were stained for 1 h with Coomassie Brilliant Blue R-250 and left to destain overnight, then imaged on a Gel-Doc EZ Image (BioRad).
Suspension trapping (S-Trap) sample preparation
50 μg E. coli lysate was prepared according to the manufacturer's instructions. Trypsin was resuspended in a 50 mM ABC buffer and added on the filter at a 1
:
50 (enzyme
:
protein w/w) ratio and incubated for 8 hours at 37 °C. After the digestion, peptides were eluted according to the manufacturer's protocol and vacuum centrifuged to dryness prior to desalting.
Solid phase extraction (SPE)
All samples were desalted prior to MS analysis with either C18 ZipTips or 10 mg HLB cartridges according to manufacturer's instructions as in Smith et al. and Cronin et al.44,45 Samples were dried prior to analysis and stored at −80 °C until re-suspension for LC-MS/MS.
MALDI-TOF MS
0.25 μL of sample was spotted onto a stainless steel MALDI target and then overlaid in 0.25 μL of a saturated α-cyano-4-hydroxycinnamic acid (CHCA) in a 1
:
1 mixture of acetonitrile and water with 0.1% trifluoroacetic acid. Data were acquired in reflectron mode with a mass range of 800–2100 m/z. 3000 spectra were summed per sample at a pulse rate of 500 Hz. Peptide mass fingerprinting (PMF) was performed using Mascot (Matrix Sciences) from peak lists generated in flexAnalysis (Bruker). Briefly, smoothed and deisotoped peaks (2
:
1 S/N) were subjected to PMF with the following parameters: bovine proteome was the database, trypsin was the enzyme selected, and our search was sensitive to two missed cleavage sites. We searched with a mass tolerance of 60 ppm,46 carbamidomethylation of cysteine was selected as a fixed modification, and oxidation of methionine as a variable modification.
Analysis via nano UHPLC-MS/MS
Dried digests were resuspended in 0.1% formic acid in water to a concentration of 500 ng μL−1. 2 μL of solution was injected onto a 100 mm × 100 μm C18 BEH reverse phase chromatography column with an autosampler (nanoACQUITY, Waters Corporation) as in Sun et al.47 Peptides were separated over a 90 minutes segmented gradient from 4–35% B (A = H2O + 0.1% FA, B = acetonitrile + 0.1% FA) LC-MS/MS was performed on a Q-Exactive HF mass spectrometer via electrospray ionization (Thermo Fisher Scientific). Samples were collected in biological triplicate, and data was acquired in technical triplicate injections using a TOP17 data-dependent method (DDA).48
Peptide-spectrum matching (PSM)
RAW files from technical and biological triplicates were processed for peptide identification, protein inference, and false-discovery rate using the MetaMorpheus search engine.42,43 An E. coli FASTA from UniProt (proteome ID UP000000625) was used with a common contaminant file. Data were first calibrated prior to search (‘Traditional’) using the default parameters. For ‘Main’ search, additional parameters included a maximum of two missed cleavages, two variable modifications per peptide, and a minimum peptide length of seven amino acids. The precursor mass tolerance was set to 5 ppm and the product mass tolerance was set to 20 ppm. The ‘common’ fixed and ‘common’ variable modifications were selected, and identifications were filtered to a q-value of <0.01. RAW data files are available through MassIVE ftp://massive.ucsd.edu/MSV000088657/(ftp://MSV000088657@massive.ucsd.edu password until publication <DNA4Prot>).
Results & discussion
In this study, we hypothesized that DNA Miniprep filters can be used in bottom-up proteomics sample preparations with similar efficacy to other widely-used tools in a method we are calling MAP. To begin testing this, we digested an experimentally relevant amount of a standard protein, BSA, on Minipreps using the MAP protocol. MAP generated peptides were analyzed via MALDI-TOF MS (ESI Fig. 1†). Resulting spectra were subject to Peptide Mass Fingerprint (PMF) search using Mascot to determine the efficacy of the digest.49,50 For all digested samples, scores were nearly 3-fold higher than the significance threshold (<0.02) and sequence coverage was ∼45% or higher (ESI Table 1†). The positive identification informed us that MAP can be used for filter-based digestion of simple protein mixtures.
In order to determine the capacity and recovery of Minipreps we first prepared a bulk suspension of denatured, reduced, alkylated BSA, added ammonium acetate and aliquoted to 0–500 μg then addition of MeOH
:
ABC buffer prior to loading on Miniprep columns. Columns were microcentrifuged to bind; then washed twice with a MeOH
:
ABC buffer to remove excess salt and detergent. The combined flow-through and washes are expected to contain any unbound protein due to the sample exceeding filter capacity, or not binding. Combined flow-through and wash samples were concentrated and analyzed via SDS-PAGE (ESI Fig. 2†). We did not observe any breakthrough of BSA at any measured concentration. Next, we repeated this testing with a complex protein sample. Volume-normalized aliquots of E. coli lysate from 0–500 μg were loaded onto Minipreps and centrifuged. Flow-through samples were analyzed by SDS-PAGE. No protein bands were visible from the combined flow-through and wash samples (ESI Fig. 2†). From this, we conclude that the columns effectively bind protein in the presence of a lyotropic salt to at least 500 μg. The capacity appears only limited by clogging of the filter-substrate which would prevent extraction.
To test peptide recovery from mass-limited samples, we digested sub-microgram amounts of protein using the MAP protocol. 500 ng of BSA was prepared as previously described, and peptides were again analyzed via MALDI-TOF MS, with the resulting spectra searched using Mascot (ESI Fig. 1†). From the 500 ng digest, 28 peptides were identified resulting in a protein score of 132 and 46% coverage (ESI Table 1†). From these data, we conclude that the MAP is sufficient for mass-limited protein samples.
Comparison of MAP and S-Trap
We sought to benchmark the performance of MAP to that of S-Traps, a commercial suspension-trapping based proteomics tool. 50 μg E. coli cell lysate was in triplicate was prepared and bound to DNA Minipreps using our protocol and three S-Trap minis following the manufacturer's protocol. The samples were digested with trypsin and eluted peptides were desalted using SPE. Each sample was analyzed by nLC-MS/MS in technical triplicate. Peptide-spectral matching, protein inference and Label Free Quantification (LFQ) was performed using FlashLFQ in MetaMorpheus.42 Data were filtered to an FDR of 1.0% and exported as text for analysis. An overview of the experimental design is visualized as Fig. 1.
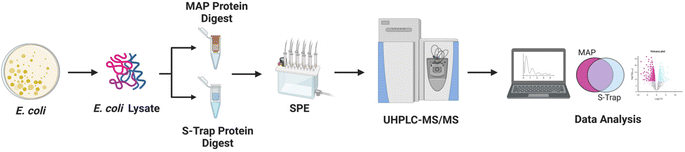 |
| Fig. 1 Experimental design. E. coli cell pellets were harvested and lysed in a buffer containing 1% SDS. Additional SDS was added prior to reduction and alkylation. Adventitious binding to the MAP was enhanced with ammonium acetate, contaminants and detergents were removed by washing on both DNA miniprep purification columns (MAP) and S-Trap minis. Proteins were digested with trypsin; peptides collected and desalted using SPE cartridges. Digests were analyzed by Nano UHPLC-MS/MS. Peptide spectral mass-matching and FLASH LFQ label free quantitative proteomics of the raw data was performed. | |
Proteins were sorted from highest to lowest average intensity and were counted as positively identified if they appeared in at least two out of three technical replicates for a single sample. Using these parameters, 2262 proteins were identified from all samples. 2247 proteins were identified in the MAP digested samples and 2082 proteins were identified from the S-Trap digested samples. Of the 2262 total identified proteins, 2067 were found in both the MAP samples and the S-Trap samples. 180 proteins were unique to the MAP digests and 15 proteins were unique to the S-Trap digests (Fig. 2A). To better visualize the comparison, we generated a heat map of the proteins organized from highest to lowest based on the log10 value of their average LFQ intensities (Fig. 2B). More abundant (log10(a.u.) >104) proteins appear in a shade of blue (darker blues indicate higher LFQ abundance) while less abundant (log10(a.u.) <104) proteins will appear yellow (dark yellow indicating a low abundance or unidentified protein). Many of the lower abundant proteins (log10(a.u.) < 104) are present in the three S-Trap samples. There is significant overlap in protein identifications between the two methods, however, overall protein abundance is higher in the MAP protocol, particularly among the lower-abundance proteome (Fig. 2B, proteins >1500). Interestingly, measured abundance of the most abundant proteins in the lysate were higher in the S-Trap digested samples (Fig. 2B, darker blue proteins 1–250). This is also reflected in ESI data Fig. 3.† From this data we conclude MAP successfully recovers proteins without significant bias from abundance.
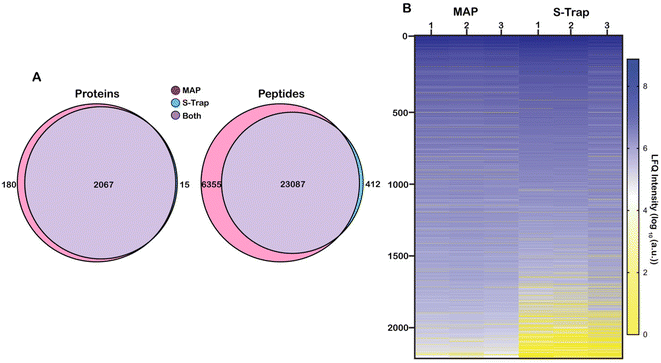 |
| Fig. 2 Protein and peptide identifications for each digestion method. (A) Venn diagrams of the overlap of protein (left) or peptide (right) identifications for the filter digestion methods. Overlap between the two methods is significant. 180 of 2262 proteins were identified uniquely to MAP vs. 15 to S-Trap. (B) Heatmap of all proteins identified from MAP or S-Trap approaches ordered by decreasing LFQ intensity. Each column is the average of three technical replicates. Minipreps more consistently detect proteins from the lowest abundances determined from LFQ. | |
We next applied this analysis to the measured and identified peptides. 29
854 unique peptide spectral matches (PSM) were identified across both methods filtered to a 1% FDR. 29,442 (98.6%) peptides were identified with MAP and 23
499 (78.7%) were identified with S-Traps. 23
087 (77.3%) peptides were shared between both filter methods. 6355 (21.3%) peptides were unique to the MAP and 412 (1.4%) peptides were unique to S-Traps (Fig. 2A).
Reproducibility
In order to assess the reproducibility of the MAP method we performed technical and biological replication and analyzed that data. Fig. 3A shows protein alignment between triplicate sets of replicates for MAP (left) and benchmark digestion (right) scored as described in experimental methods. We plotted the overlap of protein identifications for the replicate injections for either method. In terms of identifications, both methods were highly reproducible. Of the 2247 proteins identified with MAP, 2220 (98.8%) were identified in all three samples. Of the 2082 proteins identified with S-Traps, 2008 (96.5%) were identified in all three samples, demonstrating both methods are largely reproducible at the protein level. From these data, we conclude that Minipreps are highly reproducible (98.8% protein replication) and nearly identical to suspension based proteomics preparation (96.5%). Fig. 2B also illustrates the reproducibility between biological replicates; the majority of visible points in the heat map align across all three biological replicates. Next, the coefficient of variation (CV) was calculated for both digest methods to determine statistical run-to-run reproducibility. CVs for each digest method were calculated from (LFQ abundance) of individual proteins with each sample's replicate injections. These CVs were then averaged for each biological sample to determine an overall CV for both approaches. The average CV for S-Traps was 17.64% (SD for all reps ±0.63) and for MAP it was 8.48% (±0.87). The run-to-run variability was significantly lower for MAP (Ftest ⌠ >1.00 @ 95%CI), but both methods produced proteins with the majority of CVs below 20% (Fig. 3B).
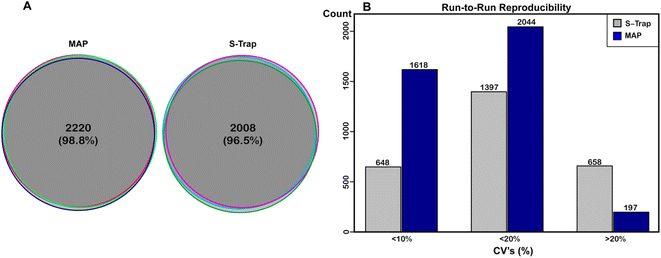 |
| Fig. 3 Reproducibility of protein identifications from filter approaches. (A) The reproducibility of protein identifications per sample for either filter method, shown by Venn diagrams depicting protein overlaps for each biological replicate. (B) The reproducibility of protein abundance per sample for either filter method based on the coefficient of variation (CV) of LFQ intensities. | |
Missed cleavages (MC) were also calculated for the MAP method and compared to the benchmark. For MAP digests, 63.45% ± 1.33% of the reported peptides had 0 MC, 32.02% ± 0.95% reported 1 MC and 4.53% ± 0.37% had 2. S-Traps were nearly identical with 63.88% ± 1.75%, 31.03% ± 0.69%, and 5.08% ± 1.10% respectively. These are not significantly different (t-test = >0.50).
LFQ intensities of proteins from each digestion method were plotted against each other (ESI Fig. 3†). The correlation between filter methods showed a strong linear trend, having an R2 value of 0.80 (ESI Fig. 3†). Both methods demonstrate low variability between runs, and are highly reproducible as 91% of proteins were identified in all biological replicates for either method, and >96% were identified in all replicates within an individual method. There is a strong linear correlation between individual intensities from both preparation methods. Higher LFQ protein abundance is observed in a greater number S-Trap based preparation (‘above the line’ ESI Fig. 3†). Interestingly these are almost exclusively from proteins with reported LFQ intensities >5 × 105; which corresponds to the blue abundances reported in Fig. 2B. There is no overall bias in recovered abundances of proteins from Miniprep prepared proteomics samples. This further demonstrates DNA Miniprep spin filters as a useful tool for bottom-up proteomic experiments.
In order to describe the origin of potential difference in recovered protein abundance across MAP and S-Traps, we visualized our data using a volcano plot (Fig. 4A) and predicted MW and pI (Fig. 4B). For each protein, significance values (y-axis) are reported as the −log10 value of a p-value determined by t-test (Benjamini Hochberg corrected to a 0.05 FDR).51 The x-axis is LFQ abundance from average S-Trap protein intensity divided by its corresponding average MAP intensity (log2). Significance of −log10 (p-value) >1.50, seen in the dotted lines. 429 proteins were found to be at over two-fold intensity on S-Traps relative to MAP (blue). 368 proteins (magenta) were more abundant in MAP. There is a narrow distribution of log2 fold change ratio, with 60% of all proteins identified showing little or no statistical difference. This indicates that while there are proteins that are selectively enriched by either filter, the majority remain statistically unchanged.
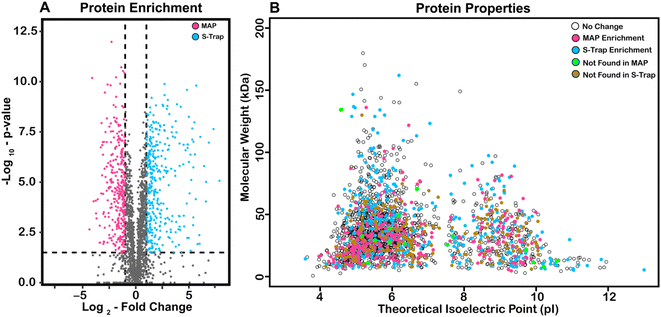 |
| Fig. 4 Predicted pI and MW distributions of statistically enriched and missed proteins. (A) Volcano plot of fold-change S-Trap/MAP. Significant differential protein expression is highlighted in magenta increased in Miniprep or blue increased in S-Trap (significance = −log10 >1.5 Benjamini–Hochberg). (B) Scatter plot of the molecular weight (MW) vs. predicted isoelectric point (pI) of observed proteins. Proteins with significant differential expression between the two methods are indicated with magenta (increase in Miniprep) or blue (increase in S-Trap). Proteins that are solely detected in one method only are indicated as green (undetected in Minipreps) or brown (undetected in S-Traps). | |
We hypothesized that biochemical characteristics of the proteins might explain differences in abundance.52–54 To characterize proteins that are undetected or enriched by a certain filter, we plotted the molecular weight of all proteins against their theoretical isoelectric points (Fig. 4B). These data points are color-coded for their properties. Proteins enriched by S-Traps are blue, enriched by MAP are magenta, exclusively found with S-Traps are green, and exclusively found with MAP are orange. All other proteins were labeled white. Proteins that were enriched and only detected with MAP are significantly smaller than those from other conditions (31.37 kDa). Proteins that were only detected with S-Traps are more basic (theoretical pI = 7.16) than the non-enriched or MAP enriched proteins (pI's of 6.25 and 6.36 respectively). One conclusion that is consistent with this observation is that DNA minipreps, which purify highly acidic nucleic acid, might have biases against basic proteins. This might be demonstrated for a specific example, but we observe no biases for or against the ribosomal proteins, which are both abundant and highly basic, and might be expected to magnify that difference.54 We next performed GO Gene Ontology enrichment analysis for the 161 and 97 proteins >log2 2.0 for both S-Trap and MAP respectively as compared to the E. coli genome (http://geneontology.org/). For proteins >4-fold enriched in S-Trap based proteomics we observed a 7.94–11.18-fold enrichment (p = 5.84 × 10−6–4.61 × 10−5) in the biological process of DNA duplex unwinding/chromosome organization (28 proteins expected 2.96). This enrichment is nearly identical if we also include proteins exclusively identified in S-Trap preparations. We observe no statistical enrichment in any GO category for proteins significantly represented in Miniprep proteomics. Based on these data we conclude that there might be a small recovery bias against certain categories of nucleic acid binding proteins, but overall recovery across all functional, abundance and cellular localization data is excellent and exhibits no measureable bias.
Conclusions
“Economical proteomics” suggests there is a desire in the field for more affordable yet reliable tools for bottom-up proteomics sample preparation. Past research in this field has focused on self-assembled ‘in-house’ filter tools to create inexpensive and reusable devices. In this work, we conclusively demonstrate that a more cost-effective alternative already exists. MAP, which utilizes inexpensive DNA miniprep consumables used in large quantities for nucleic acid purification, is capable of binding protein for bottom-up proteomics sample preparation, and significantly reduces the cost and time associated with bottom-up proteomics sample preparation. We exploit the adventitious binding of proteins for silica-base materials and optimized it using a priori knowledge of nucleic acid purification protocols. Quantitative protein yield as determined by normalized LFQ data and reproducibility between runs as determined by the CV prove that MAP is a reliable method of sample preparation. This is significant because the DNA Miniprep spin filters used in these experiments can be obtained in bulk for cents as opposed to dollars, and the protocols are less time-consuming than other approaches like FASP or in-gel digests. Future work can be dedicated to optimizing a procedure or adapting existing protocols for these filters in bottom-up studies.
Conflicts of interest
There are no conflicts to declare.
Acknowledgements
We thank Dr William Boggess in the Notre Dame Mass Spectrometry and Proteomics Facility for his assistance. We thank Dr Brett Phinney at UC Davis for advice and assistance. We thank Simon Weaver at Notre Dame for assistance generating figures. Portions of figures were made using BioRender (Toronto, ONT, CAN) Funding was provided in part by NIH R03AI149147, R01AI106872, R01GM139277.
References
- Y. Zhang, B. R. Fonslow, B. Shan, M. C. Baek and J. R. Yates, Protein Analysis by Shotgun/Bottom-up Proteomics, Chem. Rev., 2013, 113(4), 2343–2394 CrossRef CAS PubMed.
- P. Feist and A. B. Hummon, Proteomic challenges: sample preparation techniques for microgram-quantity protein analysis from biological samples, Int. J. Mol. Sci., 2015, 16(2), 3537–3563 CrossRef CAS PubMed.
-
M. M. Champion, A. D. Sheppard, S. S. Rund, S. A. Freed, J. E. O'Tousa, G. E. Duffield, Qualitative and quantitative proteomics methods for the analysis of the Anopheles gambiae mosquito proteome, in Short Views on Insect Genomics and Proteomics, Springer, 2016, p. 37–62 Search PubMed.
- D. Elinger, A. Gabashvili and Y. Levin, Suspension trapping (S-Trap) is compatible with typical protein extraction buffers and detergents for bottom-up proteomics, J. Proteome Res., 2019, 18(3), 1441–1445 CrossRef CAS PubMed.
- U. Kragh-Hansen, F. Hellec, B. de Foresta, M. Le Maire and J. V. Møller, Detergents as probes of hydrophobic binding cavities in serum albumin and other water-soluble proteins, Biophys. J., 2001, 80(6), 2898–2911 CrossRef CAS PubMed.
- A. Tanca, G. Biosa, D. Pagnozzi, M. F. Addis and S. Uzzau, Comparison of detergent-based sample preparation workflows for LTQ-Orbitrap analysis of the Escherichia coli proteome, Proteomics, 2013, 13(17), 2597–2607 CrossRef CAS PubMed.
- E. I. Chen, D. Cociorva, J. L. Norris and J. R. Yates, Optimization of Mass Spectrometry-Compatible Surfactants for Shotgun Proteomics, J. Proteome Res., 2007, 6(7), 2529–2538 CrossRef CAS PubMed.
- J. Funk, X. Li and T. Franz, Threshold values for detergents in protein and peptide samples for mass spectrometry, Rapid Commun. Mass Spectrom., 2005, 19(20), 2986–2988 CrossRef CAS PubMed.
- R. O. Loo, N. Dales and P. Andrews, Surfactant effects on protein structure examined by electrospray ionization mass spectrometry, Protein Sci., 1994, 3(11), 1975–1983 CrossRef CAS PubMed.
- L. C. Gillet, A. Leitner and R. Aebersold, Mass Spectrometry Applied to Bottom-Up Proteomics: entering the High-Throughput Era for Hypothesis Testing, Annu. Rev. Anal. Chem., 2016, 9(1), 449–472 CrossRef PubMed.
- T. Rabilloud, Membrane proteins ride shotgun, Nat. Biotechnol., 2003, 21(5), 508–510 CrossRef CAS PubMed.
- M. R. Wilkins, E. Gasteiger, J. Sanchez, A. Bairoch and D. F. Hochstrasser, Two-dimensional gel electrophoresis for proteome projects: the effects of protein hydrophobicity and copy number, Electrophoresis, 1998, 19(8–9), 1501–1505 CrossRef CAS PubMed.
- T. Masuda, M. Tomita and Y. Ishihama, Phase Transfer Surfactant-Aided Trypsin Digestion for Membrane Proteome Analysis, J. Proteome Res., 2008, 7(2), 731–740 CrossRef CAS PubMed.
- N. Zhang, R. Chen, N. Young, D. Wishart, P. Winter and J. H. Weiner,
et al., Comparison of SDS- and methanol-assisted protein solubilization and digestion methods for Escherichia coli membrane proteome analysis by 2-D LC-MS/MS, Proteomics, 2007, 7(4), 484–493 CrossRef CAS PubMed.
- C. R. Thompson, M. M. Champion and P. A. Champion, Quantitative N-terminal footprinting of pathogenic mycobacteria reveals differential protein acetylation, J. Proteome Res., 2018, 17(9), 3246–3258 CrossRef CAS PubMed.
- J. Erde, R. R. O. Loo and J. A. Loo, Enhanced FASP (eFASP) to increase proteome coverage and sample recovery for quantitative proteomic experiments, J. Proteome Res., 2014, 13(4), 1885–1895 CrossRef CAS PubMed.
- J. R. Wiśniewski, Filter aided sample preparation–a tutorial, Anal. Chim. Acta, 2019, 1090, 23–30 CrossRef PubMed.
- J. R. Wiśniewski, A. Zougman, N. Nagaraj and M. Mann, Universal sample preparation method for proteome analysis, Nat. Methods, 2009, 6(5), 359–362 CrossRef PubMed.
- L. L. Manza, S. L. Stamer, A. L. Ham, S. G. Codreanu and D. C. Liebler, Sample preparation and digestion for proteomic analyses using spin filters, Proteomics, 2005, 5(7), 1742–1745 CrossRef CAS PubMed.
-
J. Erde, R. R. O. Loo, J. A. LooImproving Proteome Coverage and Sample Recovery with Enhanced FASP (eFASP) for Quantitative Proteomic Experiments, in Proteomics: Methods and Protocols [Internet], ed. Comai L., Katz J. E. and Mallick P., Springer New York, New York, NY, 2017, pp. 11–18. DOI: DOI:10.1007/978-1-4939-6747-6_2.
- M. Hernandez-Valladares, E. Aasebø, O. Mjaavatten, M. Vaudel, Ø. Bruserud and F. Berven,
et al., Reliable FASP-based procedures for optimal quantitative proteomic and phosphoproteomic analysis on samples from acute myeloid leukemia patients, Biol. Proced. Online, 2016, 18(1), 1–10 CrossRef PubMed.
- J. R. Wiśniewski, D. F. Zielinska and M. Mann, Comparison of ultrafiltration units for proteomic and N-glycoproteomic analysis by the filter-aided sample preparation method, Anal. Biochem., 2011, 410(2), 307–309 CrossRef PubMed.
- A. Zougman, P. J. Selby and R. E. Banks, Suspension trapping (STrap) sample preparation method for bottom-up proteomics analysis, Proteomics, 2014, 14(9), 1006–1010 CrossRef CAS PubMed.
- M. HaileMariam, R. V. Eguez, H. Singh, S. Bekele, G. Ameni and R. Pieper,
et al., S-Trap, an Ultrafast Sample-Preparation Approach for Shotgun Proteomics, J. Proteome Res., 2018, 17(9), 2917–2924 CrossRef CAS PubMed.
- Y. H. Lin, R. V. Eguez, M. G. Torralba, H. Singh, P. Golusinski and W. Golusinski,
et al., Self-Assembled STrap for Global Proteomics and Salivary Biomarker Discovery, J. Proteome Res., 2019, 18(4), 1907–1915 CrossRef CAS PubMed.
- S. K. Parida, S. Dash, S. Patel and B. K. Mishra, Adsorption of organic molecules on silica surface, Adv. Colloid Interface Sci., 2006, 121(1), 77–110 CrossRef CAS PubMed.
- Y. Lewin, M. Neupärtl, V. Golghalyani and M. Karas, Proteomic Sample Preparation through Extraction by Unspecific Adsorption on Silica Beads for ArgC-like Digestion, J. Proteome Res., 2019, 18(3), 1289–1298 CrossRef CAS PubMed.
- A. Bouamrani, Y. Hu, E. Tasciotti, L. Li, C. Chiappini and X. Liu,
et al., Mesoporous silica chips for selective enrichment and stabilization of low molecular weight proteome, Proteomics, 2010, 10(3), 496–505 CrossRef CAS PubMed.
- P. Zhu, G. Long, J. Ni and M. Tong, Deposition Kinetics of Extracellular Polymeric Substances (EPS) on Silica in Monovalent and Divalent Salts, Environ. Sci. Technol., 2009, 43(15), 5699–5704 CrossRef CAS PubMed.
- Y. Wang and P. L. Dubin, Protein binding on polyelectrolyte-treated glass: effect of structure of adsorbed polyelectrolyte, J. Chromatogr. A, 1998, 808(1), 61–70 CrossRef CAS PubMed.
- K. Tsumoto, D. Ejima, A. M. Senczuk, Y. Kita and T. Arakawa, Effects of salts on protein–surface interactions: applications for column chromatography, J. Pharm. Sci., 2007, 96(7), 1677–1690 CrossRef CAS PubMed.
- A. Kondo and H. Fukuda, Effects of Adsorption Conditions on Kinetics of Protein Adsorption and Conformational Changes at Ultrafine Silica Particles, J. Colloid Interface Sci., 1998, 198(1), 34–41 CrossRef CAS.
- H. E. Johnston, K. Yadav, J. M. Kirkpatrick, G. S. Biggs, D. Oxley and H. B. Kramer,
et al., Solvent Precipitation SP3 (SP4) Enhances Recovery for Proteomics Sample Preparation without Magnetic Beads, Anal. Chem., 2022, 94(29), 10320–10328 CrossRef CAS PubMed.
- K. A. Melzak, C. S. Sherwood, R. F. B. Turner and C. A. Haynes, Driving Forces for DNA Adsorption to Silica in Perchlorate Solutions, J. Colloid Interface Sci., 1996, 181(2), 635–644 CrossRef CAS.
- X. Li, J. Zhang and H. Gu, Study on the Adsorption Mechanism of DNA with Mesoporous Silica Nanoparticles in Aqueous Solution, Langmuir, 2012, 28(5), 2827–2834 CrossRef CAS PubMed.
- B. Shi, Y. K. Shin, A. A. Hassanali and S. J. Singer, DNA Binding to the Silica Surface, J. Phys. Chem. B, 2015, 119(34), 11030–11040 CrossRef CAS PubMed.
- S. T. Moerz and P. Huber, pH-dependent selective protein adsorption into mesoporous silica, J. Phys. Chem. C, 2015, 119(48), 27072–27079 CrossRef CAS.
- K. R. Ludwig, M. M. Schroll and A. B. Hummon, Comparison of in-solution, FASP, and S-trap based digestion methods for bottom-up proteomic studies, J. Proteome Res., 2018, 17(7), 2480–2490 CrossRef CAS PubMed.
- K. Mikulášek, H. Konečná, D. Potěšil, R. Holánková, J. Havliš and Z. Zdráhal, SP3 Protocol for Proteomic Plant Sample Preparation Prior LC-MS/MS, Front. Plant Sci., 2021, 12, 635550 CrossRef PubMed.
- D. M. Marchione, I. Ilieva, K. Devins, D. Sharpe, D. J. Pappin and B. A. Garcia,
et al., HYPERsol: High-quality data from archival FFPE tissue for clinical proteomics, J. Proteome Res., 2020, 19(2), 973–983 CrossRef CAS PubMed.
- K. Hayoun, D. Gouveia, L. Grenga, O. Pible, J. Armengaud and B. Alpha-Bazin, Evaluation of sample preparation methods for fast proteotyping of microorganisms by tandem mass spectrometry, Front. Microbiol., 2019, 10, 1985 CrossRef PubMed.
- R. J. Millikin, S. K. Solntsev, M. R. Shortreed and L. M. Smith, Ultrafast Peptide Label-Free Quantification with FlashLFQ, J. Proteome Res., 2018, 17(1), 386–391 CrossRef CAS PubMed.
- L. Lu, R. J. Millikin, S. K. Solntsev, Z. Rolfs, M. Scalf and M. R. Shortreed,
et al., Identification of MS-cleavable and noncleavable chemically cross-linked peptides with MetaMorpheus, J. Proteome Res., 2018, 17(7), 2370–2376 CrossRef CAS PubMed.
- R. M. Cronin, M. J. Ferrell, C. W. Cahir, M. M. Champion and P. A. Champion, Proteo-genetic analysis reveals clear hierarchy of ESX-1 secretion in Mycobacterium marinum, Proc. Natl. Acad. Sci. U. S. A., 2022, 119(24), e2123100119 CrossRef CAS PubMed.
- C. Smith, J. G. Canestrari, A. J. Wang, M. M. Champion, K. M. Derbyshire and T. A. Gray,
et al., Pervasive translation in Mycobacterium tuberculosis. Kana BD, Shell S, editors, eLife, 2022, 11, e73980 CrossRef CAS PubMed.
- S. Lemeer, E. Kunold, S. Klaeger, M. Raabe, M. W. Towers and E. Claudes,
et al., Phosphorylation site localization in peptides by MALDI MS/MS and the Mascot Delta Score, Anal. Bioanal. Chem., 2012, 402(1), 249–260 CrossRef CAS PubMed.
- L. Sun, M. M. Bertke, M. M. Champion, G. Zhu, P. W. Huber and N. J. Dovichi, Quantitative proteomics of Xenopus laevis embryos: expression kinetics of nearly 4000 proteins during early development, Sci. Rep., 2014, 4, 4365 CrossRef PubMed.
- J. G. Canestrari, E. Lasek-Nesselquist, A. Upadhyay, M. Rofaeil, M. M. Champion and J. T. Wade,
et al., Polycysteine-encoding leaderless short ORFs function as cysteine-responsive attenuators of operonic gene expression in mycobacteria, Mol. Microbiol., 2020, 114(1), 93–108 CrossRef CAS PubMed.
- T. Koenig, B. H. Menze, M. Kirchner, F. Monigatti, K. C. Parker and T. Patterson,
et al., Robust prediction of the MASCOT score for an improved quality assessment in mass spectrometric proteomics, J. Proteome Res., 2008, 7(9), 3708–3717 CrossRef CAS PubMed.
- D. N. Perkins, D. J. Pappin, D. M. Creasy and J. S. Cottrell, Probability-based protein identification by searching sequence databases using mass spectrometry data, Electrophor. Int. J., 1999, 20(18), 3551–3567 CrossRef CAS.
- Y. B. Hochberg and Y. Benjamini, Controlling the false discovery rate: a practical and powerful approach to multiple testing, J. R. Stat. Soc., 1995, 57(1), 289–300 Search PubMed.
- J. R. Wiśniewski and D. Rakus, Multi-enzyme digestion FASP and the ‘Total Protein Approach’-based absolute quantification of the Escherichia coli proteome, J. Proteomics, 2014, 109, 322–331 CrossRef PubMed.
- M. Sielaff, J. Kuharev, T. Bohn, J. Hahlbrock, T. Bopp and S. Tenzer,
et al., Evaluation of FASP, SP3, and iST protocols for proteomic sample preparation in the low microgram range, J. Proteome Res., 2017, 16(11), 4060–4072 CrossRef CAS PubMed.
- M. M. Champion, C. S. Campbell, D. A. Siegele, D. H. Russell and J. C. Hu, Proteome analysis of Escherichia coli K-12 by two-dimensional native-state chromatography and MALDI-MS, Mol. Microbiol., 2003, 47(2), 383–396 CrossRef CAS PubMed.
|
This journal is © The Royal Society of Chemistry 2023 |
Click here to see how this site uses Cookies. View our privacy policy here.