DOI:
10.1039/D2SD00010E
(Critical Review)
Sens. Diagn., 2022,
1, 343-375
Recent advances in liquid biopsy technologies for cancer biomarker detection
Received
19th January 2022
, Accepted 28th February 2022
First published on 2nd March 2022
Abstract
Gene mutation is associated with cancer and the dissemination of circulating biomarkers through the blood represents a vital transitional step that exemplifies the shift from localized to systemic disease. The detection and characterization of circulating tumor biomarkers at an early stage is therefore essential as a conventional approach to monitor and suppress the initiation of overt metastatic disease. Currently, the genetic profiles of solid tumors are obtained from needle biopsies, which provides a glimpse of tumor heterogeneity. Furthermore, some tumor entities such as lung cancer are situated at remote sites and a needle biopsy can be very difficult and can increase chances of tumor seeding. Thus, the analysis of circulating tumor cells (CTCs) and cell-free circulating nucleic acids (in particular circulating tumor DNA-ctDNA) and tumor derived exosomes released into the peripheral blood from the primary tumor and/or metastatic deposits by minimally invasive means, can provide the full genetic landscape of all cancerous lesions, and can enable systematic tracking of genomic evolution. Herein, we explore how circulating biomarkers (CBs) detectable in bodily fluids can be utilized for diagnosis, prognosis evaluation and prediction of response to treatments. We also discuss the current and emerging methods for analysing CBs as well as biological and technical challenges. Deficiencies of detection methods are identified, with subsequent critical overview of methods to address them.
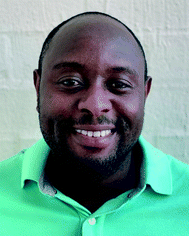 Narshone Soda | Narshone Soda is a Postdoctoral Research Fellow at the Shiddiky Research laboratory in the Queensland Micro- and Nanotechnology Centre at Griffith University. He obtained his PhD at Griffith University (Australia) in 2021. His research focuses on the development of new platform technologies and devices for liquid biopsies in genomic driven oncology. Currently he is involved in developing nanodetection technologies for diagnosis, monitoring, and treatment of cancer and infectious diseases such as Covid-19. Prior to his PhD, he obtained his M.Sc. in Chemistry at the University of Johannesburg, South Africa. |
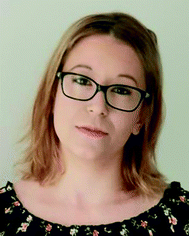 Kimberley Clack | Kimberley Clack is currently a Ph.D student at the Shiddiky Research laboratory in the School of Environment and Science. Her research interest lies in the development of nanobiosensors-particularly wearable and implantable, for the early detection of disease. Prior to starting her Ph.D, she obtained her first-class Bachelor of Science (B.Sc.) Honours majoring in chemistry at Griffith University (Australia), with the award of the university medal for academic excellence. |
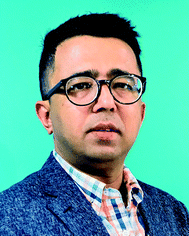 Muhammad J. A. Shiddiky | Muhammad J. A. Shiddiky is an Associate Professor in the School of Environment and Science and the Queensland Micro- and Nanotechnology Centre at Griffith University. He obtained his Ph.D. from Pusan National University in 2007. Following his Ph.D. work, he was a Postdoctoral Fellow at Monash University, Australia Research Council DECRA Fellow at the University of Queensland and NHMRC Career Development Fellow at Griffith University. He has published 155+ papers in international refereed journals with over 6400 citations (h-index > 47). His research focuses on the development of Omic technologies and portable devices for medical, agricultural and environmental applications. |
1. Introduction
The opportunity for early diagnosis of a pathological condition and for improving the efficacy of therapeutic treatments in the same instance is the main objective for many biomedical research and pharmaceutical industries. Cancer diagnosis and clinical genetic analyses require tissue sampling through tumor biopsy.1 This involves the extraction of a cancerous tissue sample or cells through invasive means and analyzing them. Although tumor tissue is currently the gold standard source for cancer diagnosis and characterization, major barriers still exist concerning acquisition and utility.2 Tissue-based tumor profiles are prone to sampling bias; they provide a spatially and temporally limited snapshot of a tumor and might fail to reflect its heterogeneity. Most importantly, tissue biopsies are not without complications with increased patient care cost and infeasible repeated sample collection. However, genomic profiling of cancer-derived materials in circulation commonly referred to, as ‘liquid biopsies’ has become an alternative approach for tumor genotyping. Liquid biopsies rely on the analysis of CBs isolated from bodily fluid samples such as blood, or urine to detect tumor specific genetic alterations. CBs have emerged as functional and non-invasive tools for prognosis and treatment monitoring of cancer due to their key role in various cell regulation processes. Thus, the identification of relevant CBs that reflect tumor behavior, through non-invasive approaches, represents a great paradigm shift in personalized clinical care.
Many studies have demonstrated the potential of liquid biopsy approaches to examine the genetic profiles of cancer patients, monitor responses to treatment and to determine the inception of therapy resistance.3,4 Molecular profiling of liquid biopsies in cancer biomarker discovery is a promising field of research because of the relatively low invasiveness of techniques employed to obtain the sample and the possibility of repeated sample collection from the same individuals at different time intervals. In recent years, the demand for molecular profiling tools has increased significantly due to the growing need and better understanding of genomic alterations and personalized treatment options. The clinical utility of circulating tumor biomarkers and their integration into routine clinical practice is of utmost importance. Thus, it is crucial to demonstrate the analytical specificity and clinical utility of CBs through large prospective multicentre studies to obtain optimum evidence required for their introduction into clinical environments. In addition, this will help to establish cancer-defining variants and identification of false positive signals through continuous follow ups.5
In recent years, several detection approaches have been developed extensively for CB analysis in both research and clinical purposes.6,7 These approaches mainly rely on coupling of liquid biopsies with novel miniaturized platforms, nanomaterials and electrochemical platforms suitable for point-of-care applications. Currently available technologies for ctDNA analysis are based on PCR and next-generation sequencing (NGS). Given the low ctDNA fraction in total cfDNA, more sensitive methods have been developed for ctDNA analysis including droplet digital PCR (ddPCR),8 digital PCR (dPCR),9 and beads, emulsion, amplification, magnetics (BEAMing).10 Despite the availability of multiple CTC technologies, only a handful of technologies have been translated for clinical usage because of the difficulties caused by CTC heterogeneity and isolation from blood.11 CellSearch® platform is currently the only FDA-approved clinical application of CTCs for enumeration of epithelial CTCs.12 Numerous detection approaches have been developed for analysis of exosomes and exosomal cargo including enzyme-linked immunosorbent assay (ELISA),13 flow cytometry14 and nanoparticle tracking analysis (NTA).15 More recently, many reviews have been published on the utility of CTCs, ctDNA and exosomes as cancer biomarkers16–18 and their detection methods.5,19,20 Herein, we detail the potential and clinical importance of CTCs, ctDNA and exosomes as key components of liquid biopsies highlighting new technologies and emerging concepts as well as existing challenges for liquid biopsy applications in clinical settings.
2. Biogenesis of common circulating biomarkers
Advances in genomics, proteomics and molecular pathology have produced many candidate biomarkers with potential clinical value. Their usage in cancer staging and personalized therapy during diagnosis could improve patient care. Liquid biopsies, mostly represented by circulating tumor nucleic acids (ctDNA and ctRNA), CTCs, tumor-derived extracellular vesicles (tdEVs), autoantibodies and tumor-educated platelets (TEPs), have the potential to assess various biomarkers for early cancer detection, carrying out genomic/immune profiling for not only selected appropriate therapy but for also monitoring the effect of therapy. Cancer antigens have been investigated as non-invasive biomarkers for early cancer detection since the 1970's, after the identification of cancer embryonic antigen (CEA) in colon tissue extracts by Gold and Freedman.21,22 Following this discovery, other clinically relevant cancer antigens such as prostate-specific antigen (PSA) and CA125, etc. were elucidated.23,24 Extensive discussion on the utility of protein based biomarkers in cancer detection is provided in the following reviews.25,26 In this review, we focus only on CTCs, ctDNA and exosomes.
CTCs
As dissemination through blood and lymph is a critical step of the metastatic cascade, CTCs are regarded as tumor surrogates with potential to monitor progression to metastatic disease and response to therapy.27 An Australian physician Thomas Ashworth first described CTCs in 1869 during a thorough comparison of CTC morphology to different tumor cells in the blood of cancer patients.28 Thereafter, it was concluded that tumor cells could penetrate vessel walls and the bloodstream. Cancer metastasis has been identified with specific genetic biomarkers such as mutations, chromosomal alterations, and gene expression patterns.29–31 Therefore, CTCs analysis is based on the assessment of cancer metastasis mechanisms. The dissemination of cancer cells from primary tumors and their subsequent seeding in distant tissues involves a multi-step process known as the invasion-metastasis cascade. This is a sequential process that includes the local invasion of primary tumor cells into surrounding tissues, intravasation through vascular walls into the circulation and parenchyma of distant tissues, the formation of micrometastatic colonies and subsequent colonization (Fig. 1).32 A leading hypothesis on processes underlying the intravasation involves epithelial-to-mesenchymal transition (EMT). This reversible phenotypic change involves a loss of intercellular adhesion and epithelial polarization and a gain of motility and invasiveness. EMT can be induced by paracrine signaling of TGF-beta, WNT, platelet-derived growth factors, or interleukin-6 (IL-6) but can also be triggered by nicotine, alcohol, and ultraviolet light.33–35
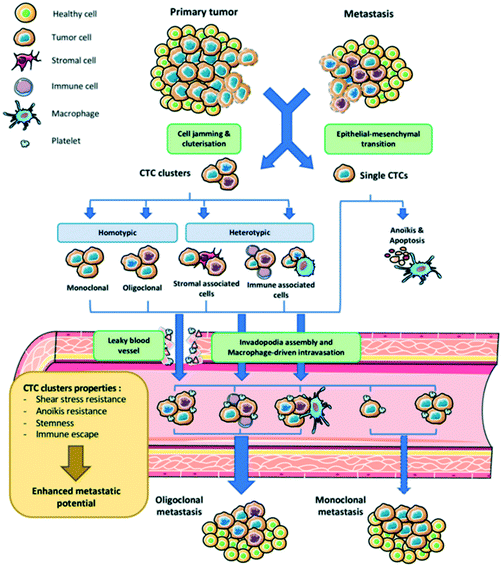 |
| Fig. 1 Schematic representation of tumor cell dissemination from its site of origin, entering the bloodstream, where they invade secondary sites and form metastatic tumors. Reproduced from ref. 32 with permission from MDPI, copyright [2020]. | |
Exosomes
The first and most notable study signifying the existence of membrane enclosed vesicles was reported in 1946.36 Initially, these membrane fragments were considered as platelet dust or cellular debris that directly emanated from the plasma membrane.37 In the 1980s, however, two independent research groups studying reticulocyte maturation described a more complex mode of extracellular vesicles secretion of small sized vesicles approximately 50 nm in diameter.38,39 The term ‘exosomes’ was then proposed in 1987 for the extracellular vesicles (EVs) of endosomal origin.40 The specific roles of these vesicles were undefined until the reports in 1996 that established the secretion of exosomes by B-immune cells with their ability to activate CD4+ T-cell clones in an antigen-specific manner.41,42 Following this, several EVs have been identified and classified based on their cellular genesis, functions and/or biogenesis.43,44 The biogenesis of exosomes entails numerous mechanisms, which facilitate the stratification of protein and RNA cargo to generate exosomes with peculiar biochemical compositions.45
Exosomes are secreted to the extracellular environment by almost every cell type and are defined and characterized by their endosomal origin small size (30–100 nm in diameter). Exosome biogenesis starts with the invagination of the plasma membrane resulting in the formation of primary endocytic vesicles, and the fusion of these vesicles with each other to generate early endosomal compartment.46 At this point, the endosome becomes a multivesicular body (MVB) consisting of a lipid bilayer and a small cytosol devoid of any cellular organelles, including various proteins and nucleic acid.47 Exosomes can also be discharged via the trans-Golgi network or inducible release (Fig. 2).48 The trans-Golgi network is a major secretory pathway sorting station that receives extracellular materials, recycled molecules from endocytic compartments, and directs them to different subcellular destinations. Various proteins from the Rab family of small GTPase proteins are essential for regulating different steps of intracellular vesicular trafficking, including vesicle budding, cytoskeletal transport and docking/fusion activities.49
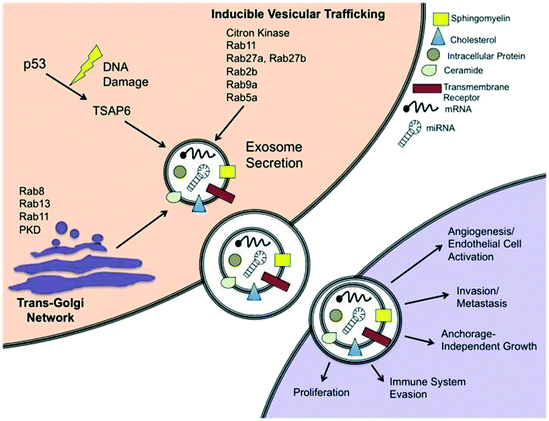 |
| Fig. 2 Schematic representation of exosome secretion in a cancer cell. Exosomes are generated within MVB subpopulations that upon maturation, fuse with the plasma membrane and discharge via an integral pathway comprising the trans-Golgi network and/or inducible pathways, which can be initiated by a p53-mediated response to DNA damage or Rab GTPases. Exosomes secreted from cancer cells can be absorbed by neighboring cells and are able to induce pathways involved in cancer initiation and progression. Reproduced from ref. 48 with permission from Frontiers Media S.A., copyright [2012]. | |
ctDNA
A group of French scientists first reported the discovery of cell-free nucleic acids in circulation in 1948 and multiple sequential studies have been performed to establish the underlying mechanism in the release of DNA fragments into the serum from the cells in their healthy, inflamed, or diseased states.50 DNA fragments are thought to be secreted into circulation like cell death, both via the apoptosis and necrosis pathways. However, the active release of DNA fragments into the bloodstream is still not completely understood.51 Some studies suggested that tumor cells shed micro-vesicles (exons) harboring fragments of double-stranded DNA (ctDNA); in contrast, this theory is still not universally accepted.3 Despite the fact that circulating cell-free DNA (cfDNA) can be detected in healthy individuals, their concentration is substantially increased in cancer patients.52 ctDNA is also released from primary and metastatic tumor sites into the circulation via the same mechanisms described above.
Cell-free DNA normally exist as double-stranded fragments of approximately 150 to 200 base pairs in length, analogous to nucleosome-associated DNA and is likely derived from apoptotic cells.53,54 The tumor-derived fraction of cfDNA is commonly termed ctDNA.55 In cancer patients, the high number of tumors affect the discharge of ctDNA, which may harbor similar mutations and genetic alterations as those of the primary tumor. Under normal physiological conditions, necrotic and apoptotic cell debris are released from the tissue by infiltrated phagocytes and are combined with an increased cellular turnover. This process rapidly generates large amounts of necrotic and apoptotic cell debris. As such, the withdrawal of the biological contents from necrotic and apoptotic cells into the bloodstream, including ctDNA, is significantly higher than in normal conditions. Despite the portion of ctDNA mirroring the tumor burden in cancer patients, significant variations have been noted among patients with similar cancer types, possibly indicating biological differences or differences in rates of cell death in individual tumors.56 In addition, patients with different tumor types exhibit substantial discrepancies in the prevalence of detectable ctDNA. As such, detecting and analyzing ctDNA amidst a background of normal germline cfDNA proffers a significant challenge.
3. Importance of CBs in diagnostics, prognostics and therapeutics
3.1 CTCs
CTC isolation plays a critical role in assisting oncologists in early cancer diagnosis and helps to determine the specific treatment thus, allowing for more personalized and effective treatments.
Clinical studies have recently demonstrated the potential of CTCs and CTC counting for treatment monitoring and prognosis prediction in early cancer detection.57,58 The combination of CTC counting with comprehensive genetic analysis using cfDNA profiling could elucidate a more distinct representation of cancer progression and prognosis as well as providing new information, such as sensitivity or resistance to certain treatment methods to further improve treatments.59–61
Following the isolation of single CTCs, various genomic profiling approaches can be adopted. Genomic analysis of single CTCs circumvents the limitation of leukocyte contamination thus, allowing the evaluation of CTC heterogeneity and assists in detecting co-existing mutations within a cell. Since a single cell contains approximately 6.6 pico grams of DNA, sensitive and reliable whole-genomic amplification (WGA) is required for mutational analyses.62 However, changes in gene expression, which are biologically relevant, and different to those effected by technical and biological noise, remains a big challenge in single-cell transcriptome analyses. To circumvent this hurdle, Ramskold et al.63 employed the Smart-Seq protocol with enhanced transcriptome coverage, high reproducibility and low technical variations as compared to previous mRNA-Seq protocols.64
3.2 Exosomes
Exosomes have been considered to be miniature versions of the parental cell for their complex composition in respect to their uniquely sorted proteins, lipids, nucleic acids, and respective elements that mostly relies on the status quo of the cell type of origin.65 Various constitutional elements in exosomes are derived from different cell types, including approximately 4400 proteins, 194 lipids, 1639 mRNAs, and 764 miRNAs, which exemplify their intricacy and potential functional sundry.66,67 Exosomal contents have been suggested to be potentially linked to particular activation or disease states. Shi et al. observed an increase in alpha-synuclein concentrations in plasma exosomes from Parkinson's disease patients compared to healthy controls68 where as Fiandaca et al. showed an increase in phosphorylated tau and beta amyloid (Aβ) proteins in Alzheimer's disease patients.69 Exosomes enclosed functional molecules, such as enzymes and miRNAs, have the ability to alter the function of target cells.70 Studies have also shown that exosomal miRNAs are capable of mediating silencing of downstream genes, which stimulates tumorigenesis in nontumorigenic epithelial cells.71
In previous years, many studies have demonstrated the diagnostic and therapeutic potential of exosomes in many diseases including cancer, neurodegenerative, infectious and cardiovascular diseases. The presence of exosome markers in human prostate and breast cancer cell lines was studied, and all samples reportedly tested positive for CD81 and CD9.72 Furthermore, significantly higher concentrations of exosomal melanoma inhibitory activity (MIA) and S100B were observed in melanoma patients than in healthy controls and disease-free patients.73 In non-small cell lung cancer plasma, exosomal NY-ESO-1 was the only marker that maintained a significant impact on inferior survival after Bonferroni correction.74
Many studies have described the enormous potential application of exosomes in tissue regeneration, drug delivery, vaccine development and gene silencing.20,43,75 Apart from cancer, the therapeutic potential of exosomes has already been shown in myocardial ischemia reperfusion and kidney injury.76 Plasma exosomes protect the myocardium from ischemia–reperfusion injury,77 arthritis,78 multiple sclerosis,79 and neurodegenerative diseases, such as Alzheimer's or Parkinson's.80 As such, exosomes have been regarded as essential cancer biomarkers because of their selective cargo loading and resemblance to parent cells.
3.3 ctDNA
CtDNA has now been widely assessed as a novel biomarker for liquid biopsy in cancer diagnosis and prognosis.81 In many cases, ctDNA is utilized for genotyping point mutations in a targeted approach to detect known druggable mutations with potential impact on therapy decision. CtDNA analysis overcomes limitations associated with tissue biopsies in genotyping by reflecting the genetic mutations of the whole tumor tissue. Henceforth, the analysis of ctDNA might improve tumor genotyping and targeted cancer therapy, which would significantly benefit personalized medicine. Multiple studies have documented an elevated level of ctDNA in patients with breast tumors and have noted their direct correlation with tumor burden.56,82 CtDNA also displays a promising diagnostic potential in hepatocellular carcinoma.83
With a short-lived half-life, ctDNA can be utilized to determine changes in the progression of tumor genomes in real time.84 Anticipation of treatment resistance before any visible clinical failure can be beneficial for patients likely to live with cancer for years. Garcia-Murillas et al.85 recently designed a personalized assay for monitoring mutations in early-stage breast cancer patients after receiving curative treatments, surgery, and chemotherapy. Mutation tracking in serial samples precisely forecasted metastatic relapse. Such remarkable early prediction could enable intervention before the reappearance of cancer in high-risk patients. More recently, research based on massively parallel sequencing (MPS) has demonstrated the potential to characterize personalized landscapes of chromosomal rearrangements within cancer patients, prognosis of metastasis and post-surgery recurrence.86,87 Molparia et al.88 described the possibility of screening copy number variations using ctDNA. This enables the application of ctDNA as a (cancer) biomarker not only for point mutation detection, but also for a galore of chromosomal aberrations.
CtDNA can also be employed to assess the efficiency of therapy via the detection of mutation-driven resistance.56,89,90 For instance, early detection of ESR1 mutations, which effect endocrine therapy resistance, may improve patient outcomes by changing to alternative treatment prior to clinical progression of metastatic breast cancer.91 Similarly, the evaluation of KRAS gene mutations in ctDNA of colorectal cancer patients may indicate resistance to epidermal growth factor receptor inhibitors.56
4. Isolation and purification of circulating biomarkers
Currently available technologies for enriching and isolating CTCs exploit their biological and physical properties that differentiate them from the surrounding normal blood cells, including size, density, deformability (physical properties) and surface protein expression, mostly EpCAM expression (biological properties)92 (Fig. 3).
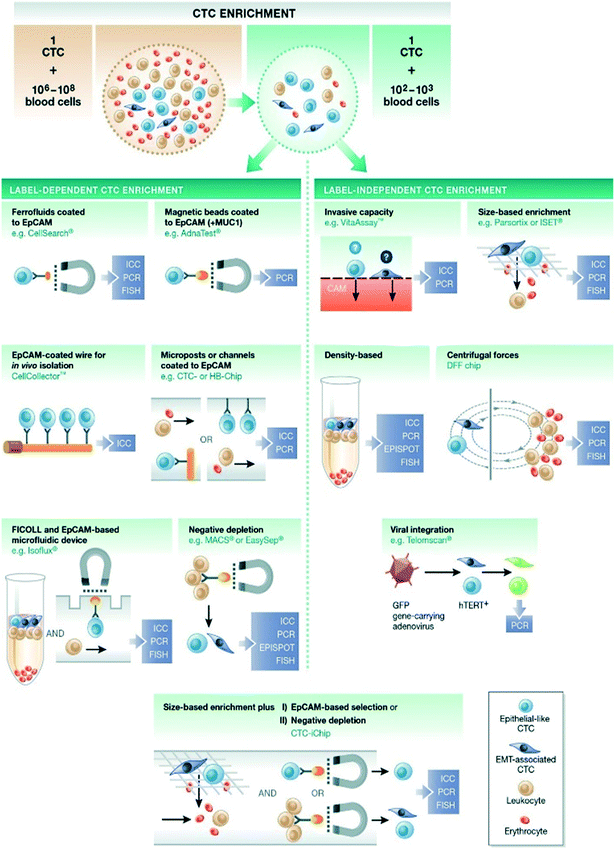 |
| Fig. 3 Enrichment of CTCs from peripheral blood of cancer patients based on physical or biological properties. Reproduced from ref. 92 with permission from John Wiley and Sons, copyright [2015]. | |
In order to facilitate the study and application of EVs, it is critically important to isolate exosomes precisely from a vast spectrum of cellular debris and interfering elements. The strategies used to isolate exosomes should demonstrate a high level of efficiency with the capability of separating exosomes from different sample matrices. At present, ultracentrifugation (including differential centrifugation) remains by far the most widely used primary isolation method for EVs due to its high processing capacity.93
As the amount of ctDNA in blood samples is finite and susceptible to contamination from high molecular DNA genomes from leucocytes, collection and isolation techniques play a key role in the detection of tumor specific mutations. Many extraction kits have been developed for cfDNA extraction and they are based on cfDNA binding to silica gel membrane-based columns or to magnetic beads. These include QIAamp circulating nucleic acid kit (Qiagen), NucleoSpin® Plasma XS kit (Macherey-Nagel) and Promega Maxwell RSC kits (Promega).94 The interaction effects between the cfDNA extraction approach and mutation detection method may result in increased artifacts, thus, careful comparison is warranted when establishing liquid biopsy procedures.17,95
4.1 Size-based enrichment and isolation technologies
Size-based CTC separation relies on exploiting different geometrical and mechanical properties between epithelial-derived cancer cells and blood cells. Size exclusion methods generally utilize membrane microfilters. The main benefit of size-based methods is that they generate viable and label free cells that allow subsequent analysis using downstream methods such as NGS to obtain more information on the specific cancer type and progression. One of the drawbacks of using the size-exclusion method for CTC isolation is that in real patient samples, most CTCs have similar sizes with that of circulating whole blood cells. To overcome this, an accuCyte-CyteFinder assay can be utilized.96 This method separates CTCs based on density. Another method for CTC isolation is the CTC iChip technology, which combines size-based enrichment with either EpCAM-based positive enrichment or CD45 negative depletion.97,98 However, this method is only suitable for single cells and up to four cell clusters and is not suitable for tumor microemboli.
4.2 Immunoaffinity based methods
A universally adopted approach for isolating CTCs utilizing epithelial lineage markers is magnetic bead separation, where antibody-labeled ferroparticles capture CTCs in a magnetic field. CTCs are subjected to positive or negative selection, normally by utilizing antibodies immobilized on inert surfaces. Positive selection often targets the EpCAM whereas negative selection usually targets cell-surface antigens not expressed in CTC such as leukocyte antigen CD45 for purified cell suspensions.99,100 Antibodies are bound to nanoparticles or microbeads. After antigen–antibody interaction, the sample is exposed to a non-uniform magnetic field to isolate labeled cells.101 However, the lack of reliable target antigens for cellular capture still presents a significant challenge to the method. Among the current EpCAM-based technologies, CellSearch is the only FDA approved method, whereby putative CTCs are positively captured based on EpCAM expression and are enumerated based on positivity for cytokeratins and lack of the white blood cells (WBC) marker CD45.102 Unfortunately, several drawbacks exist, such as EMT and disparate antigen expression. Further studies have indicated that EMT might affect stem cell-like properties in CTC subclones103 and current technologies based on epithelial antigens may miss the most aggressive CTC subpopulation.104
The presence of proteins and receptors in exosome membranes enables the development of highly specific approaches for exosome isolation via immunoaffinitive interactions. Recent studies have demonstrated the utility of antibody-coated magnetic beads for the efficient isolation of exosomes from antigen cells. It can be noted that the selection of an appropriate exosome membrane marker is one of the most essential steps in these immunoassays. Exosome immunoisolation biomarkers are bound on the membrane and are entirely expressed on the exosome surface. Zarovni et al.105 recently developed a microplate-based linked immunosorbent assay (ELISA) for isolation and quantification of exosomes derived in serum, plasma and urine. The comparison of the absorbance values deduced from ELISA results and the expression of known surface markers provided rapid results of highly specific exosomes. Compared to ultracentrifugation, substantial exosomal RNA was obtained using a microplate. Studies have also shown that members of the tetraspanin family, including CD9 and CD63 abundantly expressed in exosome membranes, can be employed for efficient immunocapture.106–108 The antibody-coated magnetic particle-based approach can be employed for isolating exosomes directly from body fluids, which avoids time-consuming centrifugation steps compared to other conventional methods. Koga et al.109 reported another approach for isolating breast cancer-specific exosomes based on HER-2 coated paramagnetic beads. The capture efficiency was validated using FACS analysis. Immunological approaches offer several benefits, and high specificity is facilitated by the antibody–antigen affinity interactions. However, this approach is relatively expensive and multiple combinations of antigen–antibody, and separation methods are required.
4.3 Microfluidic-based enrichment technologies
Microfabrication techniques allow for the establishment of structures at or below the cellular length scale, which enables easy cell separation. Microfluidic devices allow precise control of fluid flow, which is crucial for cell capture efficiency. Cell capture efficiency mostly depends on cell-antibody binding that can be examined via fluid flow velocity and direction. The first microfluidic device (the CTC-chip) comprised of an array of microposts chemically functionalized with anti-EpCAM antibodies and could enrich patients' blood with common epithelial tumors.110 Another device specific to CTCs, the cluster chip, uses bifurcating traps to capture CTCs and release them through flow reversal.111 The device flow rates are maintained at minimum levels, which aids to preserve cell viability. The herringbone-chip (HB-Chip) is a high-throughput microfluidic mixing device that provides a broad platform for isolating CTCs.112 Another promising technology, Ephesia CTC-chip, utilizes columns functionalized with self-assembled superparamagnetic beads in a microfluidic channel onto an array of magnetic traps. The Ephesia platform allows advanced cell biology testing on cancer cells.113 It integrates the microfluidic cell sorting merits which involves the utility of an ably regulated and flow-activated interaction between cells and beads, and those of immunomagnetic sorting, especially the application of batch-prepared, highly specific antibody-bearing beads. Acoustophoresis is another noncontact, label-free separation technique that relies entirely on three fundamental physical properties of the cells: size, density, and compressibility. The exposure of suspended particles to an acoustic standing wave results in varying movement trajectories, ultimately separating the cells. The advantages of microfluidic-based methods include ease of device fabrication, low reagent consumption, and non-invasiveness to cells.114 With advances in full automation and the ability to characterize cells on-chip, CTCs detection has become more and more efficient and accurate.
Microfluidics-based methods have recently become the prime techniques for microscale isolation, detection, and analysis of exosomes in bodily fluids. In microfluidics, micro- and nano-fabricated channels are utilized to manipulate minute fluid volumes, and this technique offers unique advantages of minimal processing times, high throughput and sensitivity with low reagent consumption, high surface-to-volume ratio, ease-of-use and the potential for portability. Although microfluidic-based technologies for isolating exosomes are still in their infancy, advantages of rapid processing, high sensitivity, and efficiency are currently driving the development of this field. Microfluidic-based separation technologies can be classified into two categories: (i) immunoaffinity-based isolation using specific biological markers, and (ii) combination of microfluidics with acoustic waves and dielectric electrophoresis based on exosomes electrical and physical properties. Lee et al.115 designed an acoustic nanofilter that utilizes ultrasound standing waves to isolate microvesicles based on size and density. Kanwar et al.116 developed an ExoChip that uses a polydimethylsiloxane (PDMS)-based microchannel array functionalized with exosome capture antibodies CD63. Exosomes were quantified by using a fluorescent assay method on a standard read-out plate reader.
4.4 Dielectrophoresis (DEP)
Dielectrophoresis is an innovative approach for cell separation that exploits the distinct electrical fingerprints of different cells. DEP relies on the cells' dielectric properties, which reflect the composition, morphology and phenotype of the cell.117,118 Isolation based on DEP can yield a relatively high purity output due to the specificity of the dielectric phenotypes of different cell types.119–121 Migration and retention are the two distinct strategies used in DEP for cell separation. In the migration strategy, the applied electrical field pushes cells in opposite directions by applying opposing forces. ApoStream (Apo Cell) is a typical commercial protocol for CTC enrichment that utilizes the migration strategy through the use of dielectrophoretic field-flow fractionation (DEP-FFF).122 The commercial strategy DEPArray applies the retention method of single cells in DEP cages generated via an array of single electrodes that can be regulated.123 Many clinical studies have reported the application of the DEPArray system to isolate CTCs for subsequent genetic analyses following enrichment using centrifugation or immunoaffinity.57,124–127 One of the limitations of the DEPArray system is the cell-loss for samples with a relatively high number of CTCs (e.g. metastatic carcinomas) during sample loading.127
4.5 Differential ultracentrifugation and density gradient centrifugation
With the capacity to generate centrifugal forces as high as 1
000
000 × g, ultracentrifugation is considered the gold standard approach for isolating exosomes.128 With this approach, no technical expertise or sample pre-treatments are required and it is relatively easy to use. There are two preparative ultracentrifugation methods – differential ultracentrifugation and gradient ultracentrifugation. Differential ultracentrifugation also referred to as the pelleting method, is the most common exosome isolation method that separates exosomes based on their size and density under a certain centrifugal force. Firstly, a low-speed centrifugation (400 × g) is performed to clean the human plasma/serum sample by removing large bioparticles followed by mixing with protease inhibitors to prevent degradation of exosomal proteins. The supernatant is then subjected to repeated centrifugations at progressively higher speeds that will fractionate homogenates of exosomes into their components. The exosome isolation is terminated following the last centrifugal spin (100
000–120
000 × g) to pellet the exosomes. It should be noted that extracellular fluids present high heterogeneity. Therefore, all sample components with buoyant density, size, and mass reaching a certain threshold can be precipitated under a certain centrifugal force. As such, this strategy often suffers from low purity, which limits the potential application of exosomes in downstream analysis. Gradient ultracentrifugation utilizes the size, mass and density of exosomes in a pre-built density gradient medium. The sample is introduced onto the top of the density gradient medium and subjected to longer ultracentrifugation (62–90 h).290 When the centrifugal force is applied, solutes in the sample passage through density gradient medium towards the bottom. This leads to distinct solute zones due to differing sedimentation rates of each solute. Fraction collection is therefore used to recover the separated exosomes. Although density gradient ultracentrifugation is limited to narrow load zones and requires extensive centrifugation times, it provides the purest exosome population compared to ultracentrifugation alone.129
4.6 Filtration
Ultrafiltration is one of the most vital approaches for size-based exosome isolation. This technique relies on nano-membranes with different molecular weight cut-offs for isolation of extracellular vesicles from clinical samples or cell culture medium.130 Filtration methods are usually combined with ultracentrifugation, where membranes are used to separate large debris and cells, after which separation of exosomes from proteins is achieved via ultracentrifugation. Merchant et al.131 described the microfiltration isolation method for isolating urine derived biomarkers using low-protein-binding size exclusion filters. This method utilizes a hydrophilized polyvinylidene difluoride membrane to isolate exosomes from fresh or frozen urine samples. Although filtration is relatively easy and rapid, optimization of the operating procedure is crucial for reducing detrimental consequences of clogging that can damage or deform exosomal vesicles and lower the yield.295
4.7 Polymer precipitation
Several commercial polymer-based kits have been produced based on exosome precipitation for isolation of exosomes from culture medium and various bodily fluids.132 Precipitation of exosomal vesicles is generally performed by dispersing a polymer, such as polyethylene glycol (PEG), into the sample to bind water molecules, causing other exosomes to precipitate out of solution.133 The recovered exosomes can be resuspended in phosphate-buffered saline (PBS) for further analysis. The precipitation method is generally easy to use with no specialized equipment required,295 and it enables easy integration into clinical settings by exploiting existing methods and can be scaled for larger samples. Recent studies have shown high exosome yields obtained using commercial kits compared to conventional methods.128,134 In addition, subsequent profiling analysis of exosomes isolated by precipitation yielded the highest amounts of mRNAs and miRNAs.135 Weng et al.136 recently developed a PEG-based precipitation method for exosome isolation and enrichment from cell culture supernatant for in-depth proteome profiling. The approach could record many exosome proteins that overlapped with 97% of the top 100 exosome marker proteins in the ExoCarta database. The major demerits of polymer precipitation are the interferences by co-precipitated proteins due to nonspecific interaction between polymer and protein (Table 1).137
Table 1 Isolation methods and techniques for circulating biomarkers with their merits and demerits
Biomarker |
Method |
Detection principle |
Advantages |
Disadvantages |
Ref. |
CTCs |
Immunomagnetic positive enrichment |
Based on the expression of single or multiple antigens on the cells surface either using traditional magnetic beads of geometrical enhanced surfaces |
Isolates cells with high specificity |
Only able to detect CTCs expressing high levels of EpCAM |
138–140
|
Semi-automated |
Recovery of EpCAM+ CTCs only |
Cocktail of antibodies available to increase CTC capture |
Immunomagnetic negative enrichment |
|
Recovery of heterogeneous population of CTCs |
Exclusion of CTC-WBC clusters |
141
|
Cocktail of antibodies used to maximize depletion |
Variable recovery rates |
May inadvertently remove CTCs |
Microfluidic immunocapture positive enrichment |
|
Large surface area for CTC capture |
Recovery of EpCAM+ CTCs only |
142, 143 |
High viability of recovered cells |
Slow processing rate |
Possibility to functionalize with alternative antibodies |
Complex geometry of chip difficult to scale up |
Not suitable for CTC clusters |
Size based enrichment |
Based on size difference between tumor cells and normal blood cells |
Fast, simple and economical |
There is an overlap in size and density between normal and tumor cells |
144, 145 |
Membrane filtration |
|
Heterogeneous CTC populations can be recovered |
Filter likely to clog |
146
|
Cheap and easy to produce |
Reduced capture efficiency because of fused pores |
Rapid processing time |
Deformability-based enrichment |
Based on the difference in biomechanical properties between tumor cells and normal blood cells |
Fast, simple and economical |
Loss of relatively small CTCs |
147
|
High sensitivity |
Electrical-charge-based electrophoresis |
Based on the assumption that tumor cells are significantly differentiated in dielectric properties from normal blood cells |
Heterogeneous CTC populations can be recovered |
Electrical properties of cells are not fully understood and validated as differentiating criteria between malignant and normal cells |
148
|
Rapid processing time |
Low purity in some devices |
High cell viability |
High efficiency |
Density gradient |
Density gradient separation of CTCs |
Parallel processing of multiple samples |
Low detection and recovery rates |
149
|
Heterogeneous population of CTCs can be recovered |
Loss of very small CTCs and cell aggregates |
High cell viability |
Low purity extra enrichment technologies required |
Inexpensive |
Subtraction/negative enrichment-iFISH |
Red blood cells depletion by centrifugation and cell separation matrix. Immunomagnetic white blood cells depletion by cocktail of multiple anti-leukocyte antibodies |
Efficient depletion of white blood cells |
Follow-up studies correlating clinical significance still required |
150
|
Capture enhancement by nanomaterials |
|
Simple chip design |
Recovery of EpCAM+ CTCs only |
151, 152 |
Large surface area for increased CTC capture |
Very low throughput |
Chip surface can be functionalized with a cocktail of antibodies for increased capture efficiency |
Time taken for optimal CTC adherence to substrate is too long |
Exosomes |
Differential centrifugation |
Based on the different size and density of cells, cell debris, and exosomes under centrifugal force |
Cost effective |
Potential damage as a result of high-speed centrifugation |
153
|
Low contamination risk |
Protein aggregation |
Large sample yields |
Tedious |
Density-gradient centrifugation |
Based on the discontinuous gradient of sucrose or iodoxinol |
High purity of products avoiding the contamination of proteins |
Long time for preparation |
154
|
High equipment required |
Labour intensive |
Not suitable for low sample diagnosis |
Ultrafiltration |
Uses filter membranes with defined molecular weight or size exclusion limits |
Fast procedure |
Possibility of clogging and vesicles trapping contributing to the loss of exosomes attaching to the membrane |
155
|
Portable |
Low-cost equipment |
Immunoaffinity capture |
Based on specific binding between exosome markers and immobilized antibodies (ligands) |
High efficiency of specific exosome subtypes isolation |
Costly reagents and only suitable for cell-free samples |
156
|
Easy to use |
No chemical contamination |
Precipitation |
Changing the solubility or dispersibility of exosomes with synthetic polymers or PEG |
Low timing-cost and high efficiency |
The co-precipitation and non-exosome contaminants involved in products of precipitation |
157
|
Size-exclusion chromatography |
Particles are eluted based on their size difference after the addition of porous materials |
High purity |
High device costs |
158
|
Fast preparation |
Require additional exosome enrichment |
Good reproducibility |
Can process all sample types |
Microfluidics-based techniques |
Based on different principles including immunoaffinity, size and density |
Highly efficient |
Low sample capacity |
155
|
Low cost |
Portable |
Easily automated & integrated with diagnosis |
ctDNA |
PCR |
Known point mutations such as KRAS, EGFR, and PIK3CA hotspot alterations |
Ease of use, lowest cost |
Susceptible to interference |
159
|
No bioinformatics required |
Need to strictly control the temperature |
Takes too long for the short half-life of ctDNA |
Analysis of point mutations is not very effective |
Digital PCR |
Known point mutations, genomic rearrangements |
High sensitivity |
Accuracy of deformation temperature control requires within ±0.3 °C |
160
|
Rapid, less expensive than other methods |
Low multiplexing capabilities low |
Targeted deep sequencing |
Point alterations such as PIK3CA, KRAS EGFR, TP53 |
High sensitivity, relatively inexpensive |
Less comprehensive than whole-genome sequencing methods |
161
|
Broad applications |
Whole-genome sequencing |
Genome-wide SNVs, CNVs |
Wide applicationin genome-wide or personalized-wide rearrangements without knowledge of the genetic information of the tumor |
Expensive |
162
|
Targeted hybridization based |
DNA point mutations |
Rapid |
No report on ctDNA in patients |
274
|
Multiplexing capabilities |
Inexpensive |
4.8 Comparative summary of isolation methods
In this section, we provide a further critique of the best methods of circulating biomarker isolation. However, the best approach is highly subjective and difficult to assess. For example, an approach that may have a high capture efficiency or high sample purity, may suffer from a low recovery amount or a low throughput. High performing approaches may require tedious preparation steps and may suffer from a high cost. As a general trend, combinatorial isolation approaches are arguably the best way forward but another issue exists in determining which approaches to combine.
4.8.1 CTCs.
In determining the best CTC isolation strategy, the overarching factors include capture efficiency, platform stability, recovery rate, purity and flow through/detection time, remaining subjective in nature. In determining which CTC isolation method to investigate first, however, depends largely on CTC EpCAM expression levels. The main challenge with EpCAM-based positive enrichment is that it risks missing EpCAM-negative CTCs. The size-based strategies can overcome this challenge. Zavridou et al.163 compared EpCAM-dependent positive selection and size-dependent microfluidic separation techniques in their CTC enrichment study for head and neck squamous cell carcinoma. The authors note that a lot more positive events for CTCs were detected with the Parsortix system than with the positive EpCAM enrichment approach. Rushton et al.164 also evaluated various CTC enrichment technologies, covering size-based microfluidics approaches, immunomagnetic negative enrichment and immunomagnetic positive enrichment strategies. Size-based membrane filtration achieved the highest recovery rate of CTCs (100%).
In another study, Adams et al. demonstrated a highly efficient CTC isolation method from whole blood using a microfluidic-based positive immunocapture (EpCAM) providing a 100% purity and 97% recovery.165 Negative immune enrichment in this instance was listed as a 79% capture efficiency with a 42% purity.166 Membrane-based size filtration offered a 90% capture efficiency with a 74% purity and a 90% recovery. Currently, DEPArray offers superior benefits resulting from its automated DEP field sorting which enable the recovery of single and pure CTCs from enriched samples within a short space of time.167
Zhao et al.168 reported on a label-free ferrohydrodynamic size-based CTC cell separation assay (from patient blood) that could separate low concentrations of CTCs (100 cells per ml) with an average cell recovery rate of 92.9%, and an average purity of 11.7%, with a throughput of 6 mL per hour. While this method is fast, it suffers from low purity. Kim et al. also reported on a highly stable, advanced tapered slit filter device for size-based CTC filtration with a novel low-stress design for reducing damage to CTCs.169 The assay facilitated viable CTC isolation (70% cell viability after 5 days of on-filter culture), with an 80% capture efficiency. Shen et al.,170 developed a stable size-based separation method that involves particle diameter and inertial focusing of particle migration around channels. They reported high throughput (3 mL min−1 of blood, 1.89 × 109 cells per min) and a highly efficient separation manner (up to 99.8% focusing) with 4 hour stability. When combining methods such as with the CTC-iChip EpCAM/CD45+ size approach, a very high capture efficiency (77–98%) and a very high recovery may be achieved (99.5%). The approach of combining the isolation may arguably provide the best solution to cater for the subjective performance criteria and EpCAM positive or EpCAM negative conditions.
4.8.2 Exosomes.
The most relevant characteristics to consider when determining the best method for exosome isolation are (i) the exosome recovery rate or yield of the method, (ii) the purity of the obtained exosome sample, and (iii) the efficiency of the method in terms of time and labor, as well as the cost.
Patel et al.,171 provided a comparative analysis of exosome isolation methods using culture supernatant (from a pancreatic cancer cell line) for optimum yield, purity and downstream applications. Based on yield, they reported that the precipitation-based total exosomes isolation kit (Invitrogen) had the maximum yield and serves as the best method for fast and efficient isolation of exosomes. This method was compared to gel-filtration chromatography (iZON, qEVSingle), PureExo kit (101Bio), and differential ultracentrifugation. Thus, the choice of EV isolation method should depend on the amount of starting material together with the downstream application, being influenced by the need to remove all or only distinct groups of non-EV serum components.172 As such, the best method of exosome isolation is still highly subjective.
PEG-based precipitation methods have been demonstrated to have the highest recovery rate in comparison to other methods (80–90%) with differential centrifugation and ultrafiltration having relatively low to medium yields.173,174 Density gradient ultracentrifugation also has the lowest exosome recovery rate at around only 10%. In terms of purity, density gradient ultracentrifugation is the gold-standard method for achieving the highest exosome purity, since the method can remove non-specifically bound proteins from vesicles. Concerning time and labor cost, density gradient centrifugation requires the most labor and time, with PEG-based precipitation requiring the least labor and lowest cost.175
High-speed ultracentrifugation remains the classical approach for exosome isolation, but it suffers limitations such as low throughput.176 The method also suffers from high levels of protein aggregate and lipoprotein contamination. As such, a standardized method of exosome isolation has yet to be realized, given that a single, best method of exosome isolation cannot be applied across various sample sources.93 Wu et al.,177 reported on an exosome separation method that utilized acoustofluidics and microfluidics to isolate exosomes directly from whole blood. The approach demonstrated the isolation of 110 nm particles from a mixture of micro- and nanosized particles with a yield greater than 99%. Exosomes were also isolated from an extracellular vesicle mixture with a purity of 98.4%. The method also achieved a blood flow removal rate of 99.99%. This approach appears to be superior in terms of purity and yield, but it may be criticized on speed and the inability to isolate smaller particles.
4.8.3 CtDNA.
The best method of ctDNA isolation is also subjective, and Lyu et al.,178 note that detection performance is based on the type and method of extraction kits for improving the diagnostic capabilities of ctDNA. Lim et al.,179 summarized both NGS and PCR-based techniques for detecting ctDNA mutation in non-small cell lung cancer, for liquid biopsy applications. For the NGS approaches, the authors noted that MiSeq offered 100% sensitivity, 100% specificity with a LOD of 0.028% (L858R). The greatest benefit of NGS over PCR-based approaches is that NGS offers the capability to discover new genetic mutations, with ultra-sensitive detection.
The PCR-based ddPCR is one of the most powerful techniques for cancer screening via genetic alterations in ctDNA. This technique features increased specificity and sensitivity (especially for rare target mutations) owing to the generation of a large number of droplets using a microfluidic chip for compartmentalizing particular DNA fragments. The newer ddPCR approach is recognized as one of the best-positioned techniques for detecting ctDNA at early tumor stages for blood screening tests.180
5. Detection technologies of circulating biomarkers
5.1 Amplification and sequencing-based approaches
The recent advances in the field of nanomaterials has witnessed new emerging approaches with significant short processing times for CTC detection in vivo with high sensitivity and feasibility compared to conventional methods, such as immunocytochemistry and RT-qPCR.181,182 Gold nanoparticles (AuNPs) have been widely used as effective substrates for surface-enhanced Raman scattering (SERS), which provides a unique fingerprint-like spectral pattern that enables researchers to differentiate from other interferences in complex blood media.183
The chimeric nature of cfDNA and the presence of tumor-derived DNA in blood plasma indicates the presence of the entire spectrum of tumor genome aberrations that enabled the development of applications in other fields. Several methods have been developed for detecting ctDNA and are classified into two groups, namely, targeted and untargeted approaches. Targeted strategies focus on the detection of specific mutations in a batch of predefined genes. Typical examples of targeted methods for quantifying genetic mutations for different cancers include next generation sequencing (NGS), single nucleotide polymorphism (SNP) analysis and genome-wide association studies (GWAS). In the SNP array, unique nucleotide sequences are used as probes for hybridization with various fragments of ssDNAs. The GWAS is based on the application of a chip-based microarray technology that allows the analysis of SNPs.184
PCR-based techniques are easy to operate with minimum cost for amplification of nucleic acids. Over the past years, several PCR based strategies have been described notably, custom-designed coamplification at lower denaturation temperature (COLD-PCR),185,186 and peptide nuclei acid-locked nucleic acid (PNA-LNA) PCR clamp.187 COLD-PCR selectively amplifies low-abundant DNA variants from mixtures of wild type, irrespective of mutation type or position on the amplicon. Advantages of conventional PCR-based methods include simplicity and cost effectiveness that allows their implementation in most clinical molecular pathology laboratories. However, there are high error rates associated with these methods and limited performance towards the detection of low allelic fraction (AF) alterations.
Digital PCR (dPCR) is a robust technique for detecting point mutations in ctDNA. This method comprises of droplet-based systems, microfluidic platforms for parallel PCR such as droplet digital PCR (ddPCR) and BEAMing.188 BEAMing is the first high-throughput ddPCR system reported for detecting and enumerating genetic variants.189 BEAMing involves a preamplification step of starting the DNA template with specific primers targeting the genomic loci of interest. PCR products are then mixed with beads coupled to fluorescently labeled dideoxynucleotide terminators to delineate mutant from wild-type DNA (Fig. 4).190 This method can identify genetic variations present in the original DNA population and can accurately measure their number in comparison to the number of wild-type sequences.89 The detection accuracy of this technique has been reported to be one mutant DNA molecule in a background of 10
000 wild-type molecules.191 However, BEAMing procedure is relatively cumbersome and complicated for routine clinical use.191,192
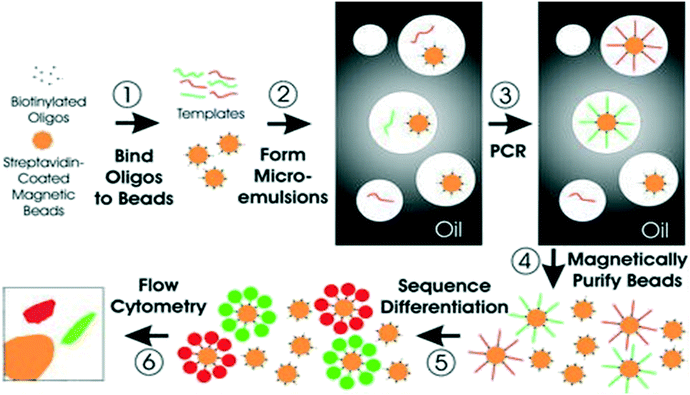 |
| Fig. 4 Schematic of BEAMing. Step 1: Magnetic beads coated with streptavidin are bound to biotinylated oligonucleotides. Step 2: Microemulsions are then created by mixing all components for PCR. Step 3: Temperature recycling of the microemulsions. Step 4: Breaking of emulsions, and purification of the beads with a magnet. Step 5: Incubation of beads with probes that can differentiate different sequences from various templates. Fluorescently labeled antibodies are then used to label the bound hybridization probes. Step 6: Application of flow cytometry to count the red and green beads. Reproduced from ref. 190 with permission from National Academy of Sciences, U. S. A., copyright [2003]. | |
Droplet digital PCR (ddPCR) has recently become one of the most precise and reliable tools for evaluating genetic alterations in various cancers due to its high sensitivity and specificity. This method relies on limiting the partition of the PCR volume, such that a positive result in any of many microreactions indicates the presence of a single target molecule in each reaction. The distribution of target sequences into partitions is represented by the Poisson distribution, thus enabling precise and absolute quantification of the target from the ratio of positive targets against all partitions at the end of the reaction. DdPCR has demonstrated its utility for examining archival tumor tissues, where poor DNA quality and insubstantial sample availability are key challenges for standard methods, providing less subjective and more automated quantitative results. DdPCR employs the same principle of dPCR, which involves the dispersion of single DNA molecules into thousands of droplets. Flow cytometry fluorescently labelled TaqMan probes are therefore used to capture and enumerate droplets consisting of mutated or wild-type DNA strands.193 The quantity of the droplets determines the sensitivity of ddPCR.194
Next generation sequencing also referred to as massively parallel sequencing technologies are revolutionizing the ability to characterize cancers at the genomic, transcriptomic and epigenetic levels. NGS has high throughput and can screen unknown variants. NGS can analyze several millions of short DNA sequences in parallel followed by either sequence alignment to a reference genome or de novo sequence assembly.89 There are several methods utilizing NGS to target panel, such as tagged-amplicon deep sequencing (TAm-seq), safe-sequencing system (Safe-SeqS) and cancer personalized profiling by deep sequencing (CAPP-Seq).71,195–197 Recently, Newman et al.197 utilized CAPP-Seq to quantify ctDNA in non–small-cell lung cancer (NSCLC). The method could detect ctDNA in 100% of patients with stage II–IV NSCLC and in 50% of patients with stage I, with 96% specificity for mutant allele fractions down to ∼0.02%.197 Amplicon sequencing is mostly used for the examination of gene mutations in specified genomic regions. The Ion-AmpliSeq method is one of the most widely used deep targeted sequencing platforms due to its rapidity, cost-effectiveness, and requires small amounts of input DNA.
5.2 Immunoassay methods
The most widely used CTC detection assays are based on immunomagnetic separation, flow cytometry, microfluidics and CTC filters. In immunomagnetic separation, CTCs are positively isolated from heterogeneous samples using specific antibodies coated on magnetic beads targeting epithelial specific antigens (cytokeratin), EpCAM, or human epithelial antigen (HEA) expressed on tumor and normal cells. They can also target tumor-specific cell surface antigens such as PSA, HER-2, CEA.
5.2.1 CellSearch.
At present, the CellSearch system is the only Food and Drug Administration (FDA) approved technique for CTC detection. It uses ferrofluids coated with epithelial cell specific EpCAM antibody to immunomagnetically enrich epithelial cells. Captured tumor cells are then permeabilized, prefixed and labeled with the fluorescent nuclear dye DAPI, a fluorescent antibody to CD45 specific to leucocytes and fluorescent antibodies to intracellular cytokeratins specific to epithelial cells. Non-specific staining of haematopoietic cells is measured by counterstaining with leukocyte marker (CD45) antibodies. Automated fluorescent imaging (Cell-Spotter Analyzer) which can classify epithelial cells positive for cytokeratin and negative CD45 to analyse the captured cells performs sample analysis. Several clinical studies based on the CellSearch system have been reported and have demonstrated the significant prognostic information provided for patients with metastatic colon, breast and prostate cancer.198–201
5.2.2 ELISA.
ELISA represents quantitative analytical methods that display antigen–antibody reactions through color change. The color change is achieved by using an enzyme-linked conjugate and enzyme substrate that serve to identify the presence and concentration of molecules in biological fluids.202,203 The ELISA mechanism has been widely used for analysis of various circulating biomarkers. Boriachek et al.204 designed a direct method for exosome isolation and subsequent detection using antibody (CD9 or CD63) functionalized magnetic nanoparticles for colorimetric and electrochemical detection of exosomes. We have recently developed a method for bioseparation, and electrochemical detection of exosomes based on bioengineered and self-assembled superparamagnetic polyhydroxybutyrate (PHB) nanobeads.205 In this approach, PHB nanobeads were functionalized with a tetraspanin antibody CD9 to capture bulk exosomes. Following magnetic capture and purification, the exosome-bound antibody was immobilized onto CA125 antibody-modified electrodes. The peroxidase activity of HRP via the hydrogen peroxide (H2O2)/HRP/hydroquinone (HQ) redox cycling system was then used to achieve an electrochemical quantification of CA125-specific exosomes present in the cell solution.
5.3 Microfluidic based methods
Microfluidic technologies allow in vitro cell sorting, quantification and single-cell analysis.206 Different materials including polymers (e.g., PDMS), ceramics, semi-conductors (e.g., silicon) and metals, have been used to develop microfluidic devices for CTC capture. Among these materials, PDMS has been widely used due to its optical properties, biological and chemical compatibility, rapid prototyping and low-cost.207 PDMS enable microfluidic devices to be easily fabricated using standard photolithography and can be integrated with other nanotechnologies. CTC chip is another microfluidic device that can efficiently and reproducibly isolate CTCs from the blood. The chip is made up of an array of microposts containing EpCAM antibodies.
Over the years, various microfluidic-based platforms have been developed for the efficient analysis of exosomes. Microfluidic devices manipulate small exosome sample volumes in their channels and allow fast and cost-effective separation and detection. Zhao et al.208 recently designed a microfluidic approach (ExoSearch) which provided enriched blood plasma exosomes for in situ, multiplexed detection using immunomagnetic beads (Fig. 5i). The ExoSearch chip exhibited significant diagnostic potential compared to the standard Bradford assay. Vaidyanathan et al.209 also designed a multiplexed microfluidic assay based on tuneable alternating current electrohydrodynamic for specific capture and quantification of exosomes (Fig. 5ii). In this approach, exosomes derived from cells expressing human epidermal growth factor receptor 2 (HER2) and prostate specific antigen (PSA) were detected simultaneously using the catalytic oxidation of TMB on the peroxidase-based exosome-antibody immunocomplex via colorimetry. Although microfluidic technologies offer several benefits such as automation and rapid analysis, they are still associated with several limitations, including batch-to-batch variations and high false positive rates from non-specific bindings.210
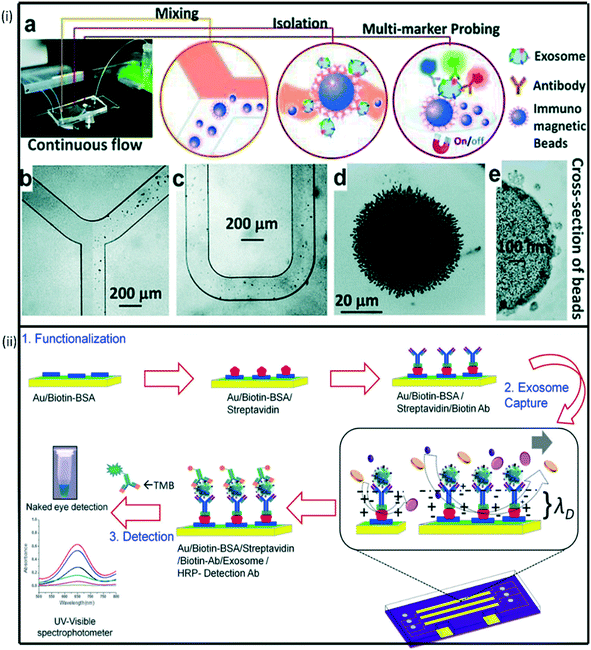 |
| Fig. 5 (i) Schematic representation of multiplexed device functionalization (a) ExoSearch chip workflow for multiplex exosome detection. (b)–(c) Manipulation of immunomagnetic beads in the microfluidic channel for mixing and separation of exosomes. (d) Agglomerated exosome-bound immunomagnetic beads in the microchamber with an on/off switchable magnet. (e) TEM image of an exosome-bound immunomagnetic bead in a cross-sectional view. Reproduced from ref. 208 with permission from Royal Society of Chemistry, copyright [2016]. (ii) ac-EHD induced tunable nanoshearing for the specific, exosome capture and colorimetric detection of captured exosomes. Reproduced from ref. 209 with permission from American Chemical Society, copyright [2014]. | |
5.4 Flow cytometry
Flow cytometry is one of the most used molecular approaches for quantifying and analyzing high throughput exosomes and can be used to analyze single particles and provide both cell counting and phenotyping of EV properties. The workhorse technique for high throughput analysis of exosomes relies on fluorescent labeling of exosomes, followed by passaging suspended exosomes in a fluid sheet as a single file to a laser beam. The scattered and fluorescent wavelengths are detected and analyzed or used for sorting of exosomes. The magnitude of scattered light depends on the presence of particles in the sample. Generally, a beam of laser light is directed at a hydrodynamically-focused stream of fluid that carries suspended particles.211 Several detectors are carefully placed around the stream, (at the point where the fluid passes through the light beam) measuring the forward and side scatter signals, and a maximum of nine fluorescence signals can be detected. However, the size detection of particles by flow cytometry has a limitation between 300 and 500 nm.212 In addition, over time, debris is aggregated, which might result in false positives as well as the sample pre-treatment, which includes staining, is a time-consuming process. In order to determine the detection limits of traditional flow cytometry, several researchers have examined the relationship between scatter signals and diameters of biological vesicles, polystyrene (PS), and silica beads.213,214 Since biological vesicles have a lower refractive index (RI) than polystyrene beads, they scatter light approximately 10-fold less efficiently than polystyrene beads.213 As a result, the smallest single vesicles that can be detected by flow cytometry must be larger than approximately 500 nm. Thus, It is difficult to capture and analyse single exosomes using flow cytometry due to their small sizes (≤150 nm). However, flow cytometry can be used to analyse exosome populations. Exosomes surface proteins can be stained with fluorochrome-conjugated antibodies to characterize populations originating from a particular cell type. The scattering intensity depends on the size, shape, refractive index, and absorption. The Kramers–Kronig relation provides a physical connection between the spectral variation of the refractive index and the absorption coefficient.215 However, this method has several limitations such as the aggregation of debris over time, which might result in false positives as well as the sample pre-treatment, which includes staining, is a time-consuming process.
5.5 Nanoparticle tracking analysis (NTA)
Nanoparticle tracking analysis (NTA) allows the determination of both the size distribution and relative concentration of microvesicles, including exosomes, in the supernatants of cultured cells and biological fluids. NTA uses the light-scattering characteristics of laser light on particles undergoing Brownian motion when in solution. NTA software analyzes many particles individually and simultaneously (particle-by-particle) and calculates the hydrodynamic diameters using the Stokes Einstein equation which depends on the temperature and viscosity of the suspended fluid.216 A video recording shows the tracking of particles and a mean squared displacement is calculated for each particle, allowing a hydrodynamic radius to be determined and displayed as a particle size distribution.
5.6 Electrochemical based approaches
Electrochemical methods have attracted considerable attention owing to their high sensitivity, rapid response, miniaturization capabilities, and low-cost operations.217,218 In the electrochemical detection approach for exosomes, a recognition element (e.g., antibody) interacts with the target exosomes to selectively recognize antigens that are present on the exosome surface. Electroactive molecules are tagged with a detection antibody and used as an electroactive transducer.219 Over the years, several platforms have been developed for exosomes detection based on the integration of nanotechnology with electrochemical biosensing techniques. Our group has recently reported on a proof-of-concept electrochemical method for quantifying exosomes using carboxyl group-functionalized iron oxide nanoparticles. In this method, bulk exosomes are first captured using tetraspanin CD9 antibody-functionalized iron oxide nanoparticles (C-IONPs) followed by the detection of ovarian cancer-related exosomes using biotinylated CA125 antibody.220 This method achieved a detection limit of 1.25 × 106 exosomes per mL.
Different electrochemical techniques have been used for the detection of tumor cells.221,222 Among these; voltammetry is the most used technique due to its wide applicability and high sensitivity. In voltammetry-based immunosensors, electron transfer can be hindered from electronic mediators by the surface bound cells. As such, increasing the number of electroactive mediators is an effective way to improve the current response. Infinite coordinate polymer (ICP) has widely been used as an electroactive mediator due to the unique morphology and highly tailorable properties suitable for efficient transduction of enzyme-based biorecognition events into electronic readout.223 Zhou et al.224 developed an amperometric immunosensor for HeLa cell detection via tyramide signal amplification (TSA) induced layer-by-layer deposited electroactive ICPs. In this approach, a nucleolin-targeting aptamer AS1411 (CP) was employed as a tumor cell catcher, and a TSA-based signal enrichment system comprised of Pt NPs@HRP@CP composite as a catalytic probe and tyramine functionalized ICPs (ICPs@Tyr) as electroactive signal tags for enhanced detection sensitivity (Fig. 6a). Wan et al.222 recently designed a multiplex chip for analysing cancer cells. This multi marker approach exploited the direct electrochemical oxidation of metal nanoparticles to report the specific surface marker present. The fabricated sensor chip contained an array of nanostructured gold electrodes coated with anti-EpCAM aptamer for specific capture of epithelial cancer cells (Fig. 6b).
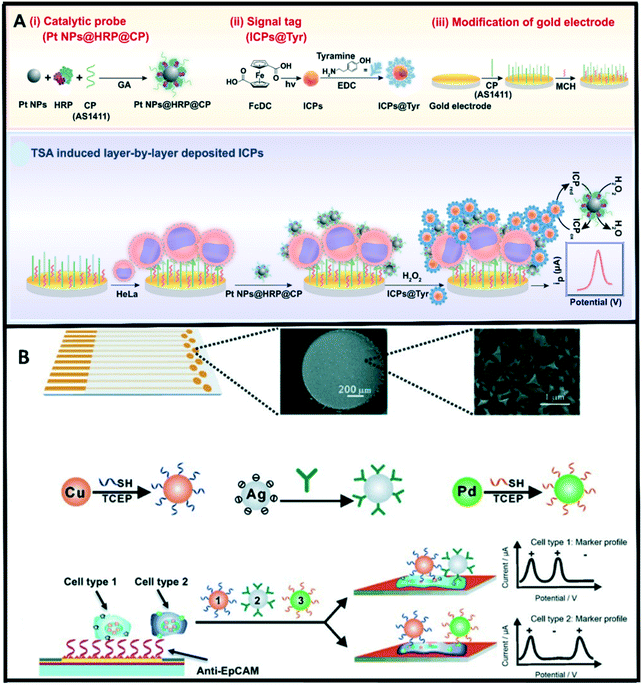 |
| Fig. 6 (A) Illustration of the assay principle of an electrochemical immunosensor for CTCs (HeLa cell as a model) detection based on TSA induced layer-by-layer deposited ICPs. Reproduced from ref. 224 with permission from Elsevier, copyright [2019]. (B) Overview of the multi-nanoparticle approach to specific cancer cell detection. Reproduced from ref. 222 with permission from Wiley-VCH, copyright [2014]. | |
Electrochemical detection approaches for ctDNA often rely on hybridization between the surface bound reciprocal receptor probe and target ctDNA sequences on the electrode surface and this hybridization generates a quantifiable signal. The development of surface modification engineering and its application in electrochemical detection techniques helps improve the detection sensitivity and limit of detection. Many studies have reported the use of sandwich-type nanostructures that possess high surface-to-volume ratios for immobilization of (more) capture probes, which serves to enhance the conductivity and sensitivity of the sensing platform.225 Chen et al.226 has recently developed a hybridization-based method for ctDNA detection in whole blood. This method utilized probe DNA modified gold-coated magnetic nanoparticles (DNA-Au@MNPs) for selective detection of short- and long-strand DNA targets. Enzymes have been successfully utilized as labels due to their capability to generate numerous molecules that could be detected from a single binding event. Recently, we designed a versatile technology platform based on bioengineered polymer nanobeads for efficient capture and electrochemical detection of DNA methylation in ovarian cancer patient samples.227 Engineered polyhydroxybutyrate (PHB) nanobeads were utilized as electrode surface modifiers that facilitated the adsorption of 5 mC antibody (specific antibody for methylated DNA). This method could detect methylation changes as low as 5% in ovarian cancer cell lines and plasma DNA samples.
5.7 Summary of detection methods
The determination of the best method of CB detection is subjective, and may be assessed on various needs such as the ability to analyse a low sample volume, particle size analysis capability, sensitivity, speed, cost, ease of use, multiplex capabilities, etc. (Table 2).
Table 2 Current technologies for CB detection
Biomarker |
Method |
Detection principle |
Advantages |
Disadvantages |
Ref. |
CTCs |
CellSearch |
Semiautomated immunomagnetic separation system for capturing epithelial cells (EpCAM positive) by ferrofluid |
FDA cleared for patient care diagnostics |
Not likely to capture CTCs expressing low EpCAM levels |
228, 229 |
Maintained cell structure that enables additional evaluation studies |
EpCAM negative CTCs may be missed |
Multiple large validation studies |
EpCAM-positivity dependent |
CTC-chip |
Unique microfluidic approach |
Controlled flow conditions should prevent trapping |
Clinical validation not yet available |
110
|
Array of microposts in a specific nongeometric pattern and coated with antibodies to EpCAM |
Maintains viability of cells |
Not available outside research setting |
Controlled laminar flow conditions |
High detection rate |
Limited data |
Further analysis possible |
CTC cluster-chip |
Uses bifurcating traps for capturing CTCs and release via flow reversal |
Isolation of CTC clusters |
Lower CTCs |
111
|
Eliminate the usage of tumor specific markers for isolation |
Preservation of cell viability due to low flow rates |
AdnaTest |
Isolation based on combining immunomagnetic separation and qRT-PCR assay |
Diverse markers (antibodies) enable simultaneous cells characterization for multiple markers |
No flexibility |
200
|
Maintained cell structures for additional analysis |
False negatives resulting from the depletion of CTC specific antigens |
Coupled with RT-PCR for additional cell analysis |
Only EpCAM negative CTCs experience EMT |
Unviable cells following detection |
Not automated |
Acoustophoresis |
Uses ultrasound waves for separation of suspended cells based on their acoustophysical properties |
The strength of the acoustic radiation force relies on the density and volume of the particle |
Unstable direct pressure source can result in deviations within the flow lines |
230
|
Gentle cell manipulation |
Low tumor cell purity and low throughput |
CAM assay |
CTCs identification via CAM uptake criteria |
Sensitive and specific |
Isolation step requires more than 12 hours |
231
|
Downstream analysis is possible |
Biomarker dependent |
CTC-iChip |
Microfluidic design for rapid cell sorting using magnetically labelled targets |
High throughput |
Limited to single or small 2–4 cell clusters, not tumor microemboli |
232
|
Inertial based capture platform |
Rapid assay time |
High sensitivity for low level CTC detection compared to commercial technology |
Capable of isolating CTCs based on tumor membrane epitopes, allowing the method to be applied in all cancers |
Dielectrophoresis (DEP) |
Separation based on polarizability and size in the field |
Selective isolation of cells |
Simultaneous control of large number of parameters |
233
|
High cell viability |
High efficiency |
DynaBeads |
Magnetic separation and isolation based on binding to desired target and beads responding to magnetic field |
Pre-coupling of biomolecules with an affinity for the target |
Only 3 types of DynaBeads are available for human tumor cell isolation |
234
|
Ephesia CTC chip |
Separation based on immunomagnetic sorting coupled with microfluidics |
High capture efficiency |
Not suitable for large volumes of blood |
235
|
Flexible platform |
Low sample volumes are required |
EPISPOT |
Separation of leukocytes via CD45 depletion |
Detection of viable CTCs and DTCs (disseminated tumor cells) |
Requires efficient antigen binding and specific epitope presentation |
236
|
Dissemination of CTC/DTC protein fingerprint using multi-parameter analysis |
Demands high antigen levels |
In vitro culture transitions may reduce cell viability and decrease detection rates |
GILUPI CellCollector Nanodetector |
In vivo isolation based on ex vivo (FSMW) |
Overcomes sample blood volume limitations |
Only suitable for direct CTC extraction from patient's bloodstream |
237
|
Functionalized structured medical wire coated with antibody |
No evident side effects |
More studies are required to ascertain its clinical utility |
ISET |
Isolation of epithelial cells using filter-based, size exclusion approach |
Isolation of CTCs without any specific epithelial surface markers antibodies |
Multi-step processing can result in CTCs damage or fragments |
238
|
High sensibility |
CTC heterogeneity with regards to size and morphology |
Herringbone CTC-chip |
An advanced CTC-chip using herringbones to generate microvortices and increase interactions between target CTCs and the antibody-coated chip surface |
Captures CTC clusters |
Particulate properties of cells are not considered |
239
|
Unviable cells |
NanoVelcro microfluidic device |
Microfluidic device uses tiny rods coated with antibodies and 3 color immunofluorescences for CTCs detection |
More advanced prototype with new additional transparent substrate for CTCs isolation |
Only suitable EpCAM-positive CTCs detection |
142
|
Exosomes |
Nanoparticle tracking analysis |
Measures exosome size distribution using both light scattering and Brownian motion |
Convenient and provides real-time feedback on the aggregation of particles |
Not suitable for exosome phenotype determination |
240
|
Unable determine cellular origin or biochemical composition |
Contamination during sample preparation |
Dynamic light scattering |
Measures particles relative size distribution in fluid |
Suitable for monodisperse samples |
Not suitable for measuring complex exosome samples with large size range |
241
|
Flow cytometry |
Measure scattered light and fluorescence of individual exosomal vesicles present in the suspension |
Subpopulation confirmation by the surface markers of exosomes; fluorescence |
Difficult to recover weak signals |
242
|
Debris aggregation resulting in false positives |
Time consuming sample pre-treatment process |
Electron microscopy |
High resolution fluorescence microscopy |
Electron microscope can directly observe the morphological structure of exosomes |
Not suitable for quantitative analysis |
243
|
Enzyme-linked immunosorbent analysis (ELISA) |
Detection of the expression of exosomal marker proteins |
High specificity |
Complicated and time-consuming procedures |
244
|
Rapid detection |
High-throughput analysis |
Transmission electron microscopy (TEM) |
Uses electrons to create images to determine size and morphology of microvesicles |
Can provide biochemic information when labelled |
Complicated sample preparation |
245
|
Western blot (WB) |
Detection of the expression of exosomal marker proteins |
Can qualitatively and quantitatively analyse marker proteins |
Complicated and time-consuming operations |
246
|
Not suitable for detecting exosomal marker proteins in biological fluids |
ctDNA |
qPCR based
|
Hotspot alterations such as KRAS, EGFR, and PIK3CA |
Simple |
Lower sensitivity |
247–250
|
ARMS |
Inexpensive |
High amplification error-rates |
PCR-SSCP |
Time effectiveness |
Only detect limited genomic loci |
Bi-PAP amplification |
PNA-mediated PCR |
COLD-PCR |
Droplet digital PCR
|
Hotspot mutations, genomic rearrangements such as KRAS, ESR1 mutations |
High sensitivity |
Only detect limited genomic loci |
250, 251 |
Digital PCR |
BEAMing |
Chip digital PCR |
Targeted hybridization based
|
DNA point mutations such KRAS, PIK3CA, or EGFR |
Fast |
No report on ctDNA in patients |
85, 252–254 |
SERS |
Multiplexing capabilities |
LSRP |
Inexpensive |
Electrochemical chip-based methods |
Microfluidic approach |
Targeted deep sequencing
|
Point alterations such as PIK3CA, EGFR, TP53 |
High sensitivity |
Less comprehensive than WGS methods |
255–257
|
TAm-Seq |
Cost effectiveness |
Safe-Seq |
SiMSen-Seq |
Ion-AmpliSeq™ |
CAPP-Seq |
Whole-genome sequencing
|
|
Wide application in genome-wide or personalized-wide rearrangements without knowledge of the genetic information of the tumor |
Expensive |
258
|
Digital karyotyping |
PARE |
5.7.1 CTCs.
Due to a lack of comparative investigations and the lack of specificity of current markers in CTC detection, there is no ideal method available.259 However, when deciding on the appropriate CTC detection method, the most important parameter to note is whether the detection method recognize clinically relevant subsets of CTCs. Politaki et al.139 noted that the CellSearch method was superior to the AdnaTest in predicting clinical outcome in patients with advanced breast cancer. However, the major limitation of CellSearch is that it fails to detect EpCAM-negative cells. CellSearch is also limited due to its high cost and low sensitivity towards some cancer types such as hepatocellular carcinoma.
Hugenschmidt et al., noted that the frequency of CTC-detection varies between detection technologies, due to differences in enrichment strategies and detection efficiency, as well as biological differences between the subtypes of CTCs.260 They note that CellSearch is currently ranked above other methods such as immunodetection and PCR in a systematic comparison of methods. Nagrath et al.,261 developed the CTC chip to overcome these limitations. The CTC chip utilizes antibody-coated microposts under laminar flow conditions to facilitate selective and efficient isolation of CTCs from whole blood. The capture efficiency of CTCs in this device was 65–80% in a PBS suspension, for several cell lines.
Isolation by size of epithelial tumor cells (ISET) technique is a highly sensitive microfiltration technique that has an isolation sensitivity threshold close to one carcinoma cell per millilitre. Tan et al., demonstrated a novel ISET-based assay which possessed at least 80% of isolation efficiency and purity for breast and colon cancer cells. ISET has the fantastic advantage of the ability to isolate CTCs without damaging cell morphology, which allows immunocytochemistry and immunofluorescence technique analysis to investigate individual CTCs.262
Another strategy for early CTC detection is to combine both CellSearch with ISET. Ramirez et al., examined the prognostic relevance of viable circulating tumor cells detected by EPISPOT in metastatic breast cancer patients. Using the EPISPOT assay, CTCs were detected in 59% of metastatic breast cancer patients. The overall patient survival was linked with the CTC status as measured by the EPISPOT method.263
5.7.2 Exosomes.
Nanoparticle tracking analysis, ELISA, flow cytometry and digital detection are established methods of exosome detection. These methods require a low volume of sample and the feature high sensitivity; however, the approaches are vulnerable to contamination and are time and labor intensive.264 It is not possible to detail a “best” method of exosome detection, largely due to size difference of exosomes. Van der pol et al., studied the particle size distribution of exosomes and microvesicles determined by transmission electron microscopy, flow cytometry, nanoparticle tracking analysis, and resistive pulse sensing, noting minimal detectable vesicle sizes (for the detection of single vesicles in suspension): TEM (1 nm); conventional flow cytometry (270–600 nm); dedicated flow cytometry (150–190 nm); NTA (70–90 nm) and RPS (70–100 nm).265 This study was conducted because most single vesicles are below the detection range of many techniques, owing to the small vesicle size and low refractive index. This leads to the misrepresentation of data and reported concentrations in the range 104 to 1012 vesicles per mL in plasma. The authors note in summary that, while TEM is able to detect the smallest vesicles present, this was possible after surface adhesion. Dedicated flow cytometry was the most accurate method for determining the size of the reference beads, but due to the heterogeneity of the refractive index of vesicles, it is expected to be less accurate on vesicles. The authors also report that of the detection methods, dedicated flow cytometry is relatively fast and it allows multiplex fluorescence detection, which makes it more applicable to clinical research.
5.7.3 CtDNA.
PCR and qPCR are the most widely used methods for quantifying nucleic acids, but they suffer from insensitivity for detecting low concentrations of mutant alleles. Scorpion ARMS integrated with real-time qPCR was developed to overcome this challenge, followed by the creation of ddPCR. While the detection capability of various forms of cancer is quite high for methods such as Scorpion ARMS, ddPCR and BEAMing, these methods are only applicable to known mutations, and they are complex to operate.266
Digital PCR methods including BEAMing and magnetics have been effectively utilized to detect a limited number of specific target variants, including KRAS, EGFR and PIK3CA mutations across cancers. Despite the high sensitivity of digital PCR, the detection of the KRAS mutation has often failed, with the ctDNA detection rate average being only 50%. NGS methods have been developed to overcome these prior limitations. NGS offers the detection by parallel sequencing of hundreds of millions of DNA fragments from a single sample, being used to assist clinical decision-making, ranging from biomarker detection, analysis for diagnosis, prognosis, and monitoring of cancer treatments.267
More advanced technologies such as TAm-Seq have emerged, resulting in higher sensitivities and more personalized cancer therapies. Forshew et al., developed a method for tagged-amplicon deep sequencing (TAm-Seq), screening 5995 genomic bases for low-frequency mutations. They identified cancer mutations present in circulating DNA at allele frequencies as low as 2%, with sensitivity and specificity of >97%.196 Furthermore, they also used the Tam-Seq method to monitor tumor dynamics, tracking 10 contaminant mutations in plasma of a metastatic breast cancer patient over 16 months. As an added benefit, TAm-Seq is also a low-cost and high-throughput method. Han et al.,268 provided a critical review of ctDNA as a tumor biomarker for detection, comparing the various methods of ctDNA detection with detection limits (% ctDNA). Among the reviewed methods, digital PCR has been noted to have the highest sensitivity (0.01), but it is limited to testing a small number of genomic positions in a sample.
CAPP-Seq has an equally high sensitivity (0.01) but it is less comprehensive than whole genome sequencing. The sensitivity of amplicon based NGS ranges between (0.01–2.00), but is less comprehensive than other NGS methods. Allele-specific-PCR is able to preferentially amplifying rare mutant DNA molecules, being easy to use and being lower in cost but it has a lower sensitivity (0.10–1.00) and it is only able to test a small number of genomic positions in a sample. Whole-exome sequencing (WES) offers the ability to interrogate the entire exome, but it suffers from the lowest sensitivity of the reviewed methods (5.00). It is also expensive.
6. Challenges associated with CB analysis
6.1 Biological challenges of CBs
Although many technologies have been developed for circulating biomarker detection, the lack of standard and variable technologies bring forth dynamic challenges for integration into clinical settings. The basic requirement for developing circulating biomarker-based diagnostics is the ability for detection in bodily fluids (serum, plasma etc.) with acceptable accuracy and sensitivity. Accurate detection of CBs has greatly been affected by several challenges, including those related to pre-analytical disparities and data analysis.269
6.1.1 Low abundance.
The consensus on blood samples withdrawn from veins is that statistically they represent the whole blood of the entire body. However, this may be untrue for detecting CTCs, because of their low frequency in a high background of normal blood cells (estimated to comprise only one cell per billion blood cells)270 which may not reflect the entire cell population. This extremely low concentration of CTCs poses a great challenge for their characterization and detection, analogous to figuratively looking for a needle in a haystack. However, CTC detection rate can be improved by increasing the sample volume within the clinically allowable range. The CTC frequency can also be increased by including an enrichment step before detection.
The relative low abundance of cfDNA; usual estimates being in the range of 5–10 ng per mL plasma, makes ctDNA detection immensely difficult; with more blood samples required.271 In addition, the purification rate of blood samples requires a great improvement. The dilution of tumor DNA fragments with normal DNA in circulation may inhibit subsequent analysis.
6.1.2 High fragmentation.
CfDNA analysis is greatly hampered by the highly fragmented nature of cfDNA, which affects its isolation as well as the accuracy of quantification. Furthermore, the amount of intact DNA that can easily be analyzed is significantly reduced during DNA fragmentation and thus analysis with techniques such as amplification and sequencing becomes difficult.272,273 CfDNA fragments commonly show prominent mode around 180 bp, suggesting release from apoptotic cells.274 Another astonishing attribute of the ctDNA fraction of cfDNA in circulation is the wide variability in the fragment sizes. It has been noted that cfDNA fragments derived from tumor sites are shorter than those from healthy cells of the same origin.275 In addition, studies have recently indicated that the cfDNA fragmentation form is not unsystematic. As cfDNA degradation is steered by nucleosome patterns within the loci,276 continuous insufficient representation of some regions in cfDNA presents systematic bias in PCR-based enrichment of target amplicons and compromises the sensitivity at a local scale. An increase in tumor size may also increase the extent of fragmentation.277 As such, a loss or decrease in DNA molecules fostering the genetic marker of interest may occur, thereby compromising the precise assessment of ctDNA. Furthermore, the possible loss of DNA characteristics during the analysis design, affects the isolation and precise quantification of circulating DNA.
6.1.3 Difficulties in direct analysis.
Amplification and hybridization-based approaches are often used to analyse nucleic acids. Hybridization provides high sequence specificity and is well suited for cancer specific molecular alteration-based analysis of ctDNA. However, this method involves tedious and time-consuming steps especially when denaturing double stranded DNA structures into ssDNA molecules required for efficient hybridization analysis. Furthermore, renaturation of ssDNA also hinders the hybridization-based analyses efficiency.278 Various methods have been proposed to circumvent this challenge. Noh et al.278 proposed a direct detection method that utilizes alkaline phosphatase labelled Zn-finger proteins to detect dsDNA by electrochemistry. More so, DNA clutch probes (DCPs) have recently been applied for ctDNA detection (Fig. 7). DCPs enables efficient hybridization analysis by inhibiting DNA molecules from renaturing.279
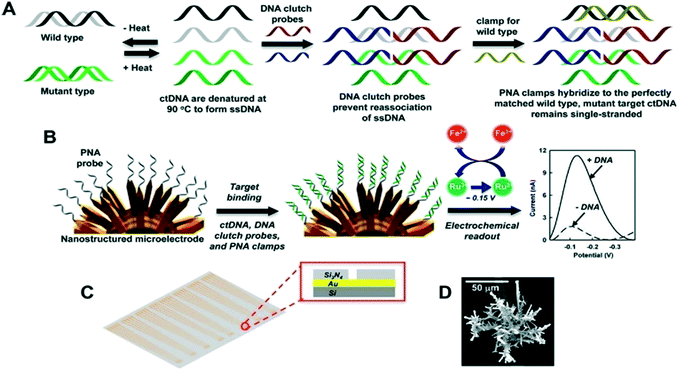 |
| Fig. 7 Schematic illustration of direct detection of ctDNA based on DNA clutch separation probes. (A) SsDNA is generated after double stranded DNA denaturation, with the following introduction of DNA clutch probes to inhibit the renaturation of ssDNA. The PNA clump then hybridizes with the wild type target ssDNA, leaving the mutant target ssDNA unhybridized. (B) Nanostructured microelectrodes (NME) are immobilized with PNA probes complementary to mutant target DNA, with only complimentary targets binding to the probe. The generated signal is recorded in the presence of a reporter system. (C) Sensor design illustration whereby the sensors are deposited in arrays on the microchip with the inset showing the cross-sectional hole in the chip. (D) Illustration of the NME sensor using scanning electron microscopy. Reproduced from ref. 279 with permission from American Chemical Society, copyright [2016]. | |
6.1.4 Low stability.
The rate at which ctDNA is released into the circulation relies mostly upon the location, size, and vascularity of the tumor, resulting in varying ctDNA levels among patients. CtDNA has a very short half-life in peripheral blood, approximately 1.5 to 2 h (ref. 85) and exhibit different levels of stability.280,281 With several steps involved in cfDNA secretion, degradation and clearance, the differing levels of DNase activity and cfDNA attaching on blood cells surface affects their stability and clearance time.282,283 Several processes and organs are cognate of cfDNA clearance, uptake and degradation by phagocytes.284,285 Therefore, measuring cfDNA levels using diagnostic strategies presents irregular and inadequate results.286
6.1.5 Sample heterogeneity.
Several factors related to sample heterogeneity including gene variation and physiological conditions could affect the analysis of exosomes. Disease-specific exosomes in healthy individuals can be found to be in higher or lower concentrations than normal as a result of various factors including gender, age, body mass index (BMI), and immunity, differing among individuals.287 Consequently, selecting an ideal benchmark for heterogeneous samples is extremely challenging, as the control cannot be used interchangeably to screen exosomes derived from different age groups. Therefore, routine studies are required to assess the influence of sample heterogeneity on the quantity, function and development of exosomes. As a prerequisite, sample control cohorts that constitute controls from all potential variants such as different sexes, ages, races, physiological conditions etc. need to be developed. Despite the recent advancements in the isolation of exosomes from other extracellular vesicles, only a few methods have been demonstrated to efficiently detect disease-specific exosomes obtained from both normal and disease cells of the same subject.288,289
6.2 Technical challenges of CBs
6.2.1 Sample preparation and choice of sample source.
Since the discovery of exosomes, numerous technologies have been developed to investigate exosomes from biological specimen. Despite these discoveries, the clinical application of exosomes remains problematic. One of the major limitations is their method of isolation. Currently, no gold standard method for exosome isolation has been established.290,291 The most common method for isolating exosomes from body fluids and culture media is differential ultracentrifugation, due to the tiny size and low density of exosomes.292 However, studies have indicated that ultracentrifugation is not only labor-intensive, but also requires excessive time and costly equipment, thus limiting its clinical usage.20 More so, exosomes are exposed to high pressure during processing and there is lack of specificity during precipitation.293 In addition, reproducible isolation in different settings or places is difficult. Ultrafiltration is another method commonly used for exosome isolation which is size based exclusive. Separation is based on size, and exosome isolation can be performed using membrane filters with distinct molecular weight or size exclusion limits.294 Ultrafiltration is relatively fast compared to ultracentrifugation and no specialized equipment is required.293 However, the high force used may deform or break up vesicles and renders them unsuitable for downstream applications that require high amounts of exosomes.295 Recently, many commercial kits based on precipitation have been developed for exosome isolation and are compatible with body fluids.293 Commercial kits are ideally fast and reproducible and can further be applied in future diagnostics, particularly in miRNA-based tests.
Another common challenge faced during sample collection is the presence of impurities generated by activated platelet-derived vesicles resulting from physical forces involved with drawing blood. To circumvent shear stress and resulting platelet activation, there is a need for standardized sampling sites and larger needles can be used as well as careful blood drawing.287 It is also suggested to avoid the usage of heparin-based anticoagulants in sample collection tubes. Recent reports have indicated that heparin prevents extracellular vesicle uptake by recipient cells.296 Alternative anticoagulants such as ethylenediaminetetraacetic acid (EDTA), sodium fluoride, or sodium citrate have generally been employed in collection tubes.
Various CTCs during EMT display different phenotypes, as such, a comprehensive enrichment spectrum using specific composition of cell surface epithelial and mesenchymal markers is required.297 Nevertheless, the combination of different markers that contain all promising CTC phenotypes may increase the chances of individual blood cells expressing at least one of these markers, resulting in false positive responses. To circumvent this, actin bundling protein plastin-3 has frequently been targeted and is neither expressed in blood cells nor downregulated by CTCs during their EMT. Recently, a microfluidic CTC-iChip has been developed for sorting epithelial and non-epithelial cancer cells using a label-dependent or a label-free process.298,299 CTC-iChip can capture and culture CTCs with improved sensitivity and efficiency for downstream characterization.298 Following enrichment, some CTCs may be retained to background cells, resulting in the reduction efficiency of analysis and sequencing efficiency of the cell pools.300 However, with the advances in NGS and single-cell-sequencing techniques, scientists can implement technologies such as flow cytometry analysis (FACS) and laser capture microdissection (LCM) for additional isolation of CTCs from background cell pools.301,302
Various preanalytical elements such as sample collection and processing, the choice of matrix, storage and biases in enriching genomic regions can influence cfDNA analysis results.303–306 Delays between venipuncture and plasma separation can generate high background of intact DNA caused by lysis of white blood cells, thus affecting PCR and tagmentation-based sequencing strategies by reducing the available effective template for analysis.307 However, to surpass this, special cell-stabilization tubes containing a preservative are used to prevent lysis of peripheral blood cells for many days at room temperature. In addition, fast processing of the sample, ambient temperatures and double plasma centrifugation, can significantly increase the chance of collecting high-quality specimens.
Despite the recent advances in the design of cfDNA/ctDNA purification and isolation protocols, considerable amounts of cfDNA/ctDNA are still lost during the purification step. Previously, studies have highlighted that by exposing samples to high-speed centrifugation, DNA can be discharged ex vivo by blood cells.308 However, microfabrication technologies have presented new ways for isolating cfDNA that circumvents time-consuming procedures for purifying cfDNA. Campos et al.309 developed a suitable polymer-based microfluidic device that can extract cfDNA/ctDNA directly from plasma with high efficiency. Hence, these advances have high potential for enhancing the overall sensitivity of ctDNA detection strategies, thus enabling implementation in clinical applications.
6.2.2 Limited comparability of different quantification methods..
Over the years, numerous cfDNA quantification strategies have been reported. These include spectrophotometric methods and sensitive fluorometric approaches that utilize a wide variety of dyes in addition to several PCR-based assays with various targets.310–312 Nevertheless, different results are obtained because only total or amplifiable DNA is targeted. For instance, fluorometric analysis targets total cfDNA levels without a DNA length limit313 whereas PCR-based techniques require short DNA strands to permit ctDNA fragment detection.314,315 Presently, there are insufficient studies directly comparing various cfDNA analysis approaches; as such, optimum strategies for efficient and definitive cfDNA detection and recovery of cfDNA for molecular assessment are inexplicit.316 Thus, there is a desperate need to design general standard operating procedures and standardized approaches for analyzing cfDNA/ctDNA analysis, considering all probable pre-analytic elements that may impact the test results.317
7. Conclusions and future perspectives
The studies assessed here highlight the potential of liquid biopsies and the development of new technologies that enable characterization of each element of the tumor circulome with increased accuracy. Liquid biopsies are being posited as a game-changing tool in personalized cancer management. The potential of liquid biopsies in translational cancer research is well recognized and these assays have been utilized in designing various clinical trials. Liquid biopsies provide a rapid, reliable and minimally invasive source for diagnosing and monitoring cancer at early stages. Clonal heterogeneity can also be captured using liquid biopsies as compared to conventional tissue biopsies. Diverse liquid biopsy samples can be incorporated to enhance cancer diagnosis, and successive real-time biopsies will further provide evidence to identify therapy-resistant tumors at an early stage. Moreover, the analysis of liquid biopsies can improve the detection and characterization of minimal residual disease after initial therapy. However, multiple limitations still need to be addressed to demonstrate the role of liquid biopsies in clinical practices.
One of the most prominent challenges in this field is the lack of standardized preanalytical and analytical variables. An ideal liquid biopsy should ensure the sample integrity, be reproducible, rapid and cost-effective. This can be achieved by using automated chip-based devices that allow biomarker analysis directly from whole blood and obviates lengthy and costly purification steps. Automated chip-based devices are suitable for high throughput analysis of biomarkers from body fluids. Although additional studies are still required to universally define the future role of liquid biopsies in cancer management, the promising results reported so far substantiate the potential utility of liquid biopsies to transform the current paradigms of cancer management.
Several studies have demonstrated the viability of ctDNA utility in tracking and monitoring tumor dynamics, drug response, and therapy resistance. Although multiple approaches have exhibited extremely high sensitivities with detection rates as low as single mutated DNA molecules, ctDNA application as a liquid biopsy marker still lacks standardization in several aspects such as blood collection, processing and storage, DNA extraction and quantification, and validation in large prospective clinical studies, is necessary. As illustrated in this study, one of the difficulties of implementing ctDNA as a liquid biopsy substrate is the commonly low sample yield from plasma extraction. To ensure sufficient starting material for further downstream analyses such as deep sequencing, whole genome amplification may be utilized.
Over the past few years, great strides have been made in translating the enigmas of exosomes. However, limitations associated with efficient exosome isolation are still yet to be solved. This is majorly due to the complex nature of biological fluids, significant overlapping, as well as their heterogeneous nature.318 As such, no specific exosome isolation method has currently been accepted as suitable for all studies.319 Given these conditions, the integration of two or more techniques would increase the exosome isolation efficiency.
Detection of CTCs in the peripheral blood provides insights into the complex biology of micrometastasis and has been described as a reliable surrogate for prognosis in several cancers. This is essentially crucial for guiding molecularly targeted therapies and to assess the effects of cancer therapy. The lack of technologies for efficient isolation and detection of CTCs poses a major challenge to the understanding and utilization of CTC biomarkers in clinical practices. Other fundamental issues include heterogeneity of the CTC populations, detection of CTC with tumor-initiating capacity, as well as hallmarks of DTC growth and dormancy at metastatic sites. The continuous development of liquid biopsy techniques requires the optimization and standardization of new methods of enrichment and targets for detection with improved sensitivity and specificity. There is a need to understand further molecular mechanisms of cancer progress, recurrence, and metastasis using genetic profiling of CTCs. Further research in this field could potentially contribute to the interpretation of the metastatic process and lead to new treatment interventions. In addition, the use of multimarker assays and real-time PCR should be further explored. All studies in these fields will prompt both cancer biology research and clinical cancer management, thus improving the life quality and expectancy for patients with tumors.
Author contributions
N. Soda and K. Clark completed the literature survey and wrote the original draft. N. Soda reviewed and edited the manuscript. M. J. A. Shiddiky involved in conceptualization, funding acquisition and administration of this project. All authors have given approval to the final version of the manuscript.
Conflicts of interest
There are no conflicts to declare.
Acknowledgements
K. C. thanks the support from Griffith University Higher Degree Research Scholarship. This work was partially supported by ARC DP Grant (DP190102944). M. J. A. S. also acknowledges funding from the Australian Research Council via Linkage Projects (LP200100016 and LP200200758). These grants significantly contributed to the environment to stimulate the research described here.
References
- G. Siravegna, S. Marsoni, S. Siena and A. Bardelli, Nat. Rev. Clin. Oncol., 2017, 14, 531–548 CrossRef CAS PubMed.
- E. Heitzer, I. S. Haque, C. E. S. Roberts and M. R. Speicher, Nat. Rev. Genet., 2019, 20, 71–88 CrossRef CAS PubMed.
- C. Alix-Panabières and K. Pantel, Cancer Discovery, 2016, 6, 479–491 CrossRef PubMed.
- F. Diehl, K. Schmidt, M. A. Choti, K. Romans, S. Goodman, M. Li, K. Thornton, N. Agrawal, L. Sokoll, S. A. Szabo, K. W. Kinzler, B. Vogelstein and L. A. Diaz Jr, Nat. Med., 2008, 14, 985–990 CrossRef CAS PubMed.
- L. Gorgannezhad, M. Umer, M. N. Islam, N. T. Nguyen and M. J. A. Shiddiky, Lab Chip, 2018, 18, 1174–1196 RSC.
- K. M. Koo, N. Soda and M. J. A. Shiddiky, Curr. Opin. Electrochem., 2021, 25, 100645 CrossRef CAS.
- J. D. Cohen, L. Li, Y. Wang, C. Thoburn, B. Afsari, L. Danilova, C. Douville, A. A. Javed, F. Wong, A. Mattox, R. H. Hruban, C. L. Wolfgang, M. G. Goggins, M. Dal Molin, T. L. Wang, R. Roden, A. P. Klein, J. Ptak, L. Dobbyn, J. Schaefer, N. Silliman, M. Popoli, J. T. Vogelstein, J. D. Browne, R. E. Schoen, R. E. Brand, J. Tie, P. Gibbs, H. L. Wong, A. S. Mansfield, J. Jen, S. M. Hanash, M. Falconi, P. J. Allen, S. Zhou, C. Bettegowda, L. A. Diaz, Jr., C. Tomasetti, K. W. Kinzler, B. Vogelstein, A. M. Lennon and N. Papadopoulos, Science, 2018, 359, 926–930 CrossRef CAS PubMed.
- Y. Takayama, K. Suzuki, Y. Muto, K. Ichida, T. Fukui, N. Kakizawa, H. Ishikawa, F. Watanabe, F. Hasegawa, M. Saito, S. Tsujinaka, K. Futsuhara, Y. Miyakura, H. Noda, F. Konishi and T. Rikiyama, Oncotarget, 2018, 9, 24398–24413 CrossRef PubMed.
- G. Herbreteau, A. Vallée, A.-C. Knol, S. Théoleyre, G. Quéreux, E. Varey, A. Khammari, B. Dréno and M. G. Denis, Oncotarget, 2018, 9, 25265–25276 CrossRef PubMed.
- J. García-Foncillas, E. Alba, E. Aranda, E. Díaz-Rubio, R. López-López, J. Tabernero and A. Vivancos, Ann. Oncol., 2017, 28, 2943–2949 CrossRef PubMed.
- D. A. Haber and V. E. Velculescu, Cancer Discovery, 2014, 4, 650–661 CrossRef CAS PubMed.
- S. Riethdorf, L. O'Flaherty, C. Hille and K. Pantel, Adv. Drug Delivery Rev., 2018, 125, 102–121 CrossRef CAS PubMed.
- S. Khodashenas, S. Khalili and M. Forouzandeh Moghadam, Biotechnol. Lett., 2019, 41, 523–531 CrossRef CAS PubMed.
- Y. Tian, M. Gong, Y. Hu, H. Liu, W. Zhang, M. Zhang, X. Hu, D. Aubert, S. Zhu, L. Wu and X. Yan, J. Extracell. Vesicles, 2020, 9, 1697028 CrossRef CAS PubMed.
- P. Carnell-Morris, D. Tannetta, A. Siupa, P. Hole and R. Dragovic, Methods Mol. Biol., 2017, 1660, 153–173 CrossRef CAS PubMed.
- S. Manier, J. Park, M. Capelletti, M. Bustoros, S. S. Freeman, G. Ha, J. Rhoades, C. J. Liu, D. Huynh, S. C. Reed, G. Gydush, K. Z. Salem, D. Rotem, C. Freymond, A. Yosef, A. Perilla-Glen, L. Garderet, E. M. Van Allen, S. Kumar, J. C. Love, G. Getz, V. A. Adalsteinsson and I. M. Ghobrial, Nat. Commun., 2018, 9, 1691 CrossRef CAS PubMed.
- J. C. M. Wan, C. Massie, J. Garcia-Corbacho, F. Mouliere, J. D. Brenton, C. Caldas, S. Pacey, R. Baird and N. Rosenfeld, Nat. Rev. Cancer, 2017, 17, 223–238 CrossRef CAS PubMed.
- J. C. Contreras-Naranjo, H. J. Wu and V. M. Ugaz, Lab Chip, 2017, 17, 3558–3577 RSC.
- Y. Zhang, X. Mi, X. Tan and R. Xiang, Theranostics, 2019, 9, 491–525 CrossRef CAS PubMed.
- N. Soda, B. H. A. Rehm, P. Sonar, N. T. Nguyen and M. J. A. Shiddiky, J. Mater. Chem. B, 2019, 7, 6670–6704 RSC.
- P. Gold and S. O. Freedman, J. Exp. Med., 1965, 121, 439–462 CrossRef CAS PubMed.
- H. Koprowski, M. Herlyn, Z. Steplewski and H. F. Sears, Science, 1981, 212, 53–55 CrossRef CAS PubMed.
- R. J. Ablin, W. A. Soanes, P. Bronson and E. Witebsky, J. Reprod. Fertil., 1970, 22, 573–574 CrossRef CAS PubMed.
- T. L. Klug, R. C. Bast Jr., J. M. Niloff, R. C. Knapp and V. R. Zurawski Jr., Cancer Res., 1984, 44, 1048–1053 CAS.
- C. A. K. Borrebaeck, Nat. Rev. Cancer, 2017, 17, 199–204 CrossRef CAS PubMed.
- D. J. Brennan, D. P. O'connor, E. Rexhepaj, F. Ponten and W. M. Gallagher, Nat. Rev. Cancer, 2010, 10, 605–617 CrossRef CAS PubMed.
- M. Braun, A. Markiewicz, R. Kordek, R. Sądej and H. Romańska, Cancers, 2019, 11, 143 CrossRef CAS PubMed.
- T. Ashworth, Med. J. Aust., 1869, 14, 146 Search PubMed.
- I. J. Fidler, Eur. J. Cancer, 1973, 9, 223–227 CrossRef CAS PubMed.
- D. R. Coman, R. P. deLong and M. McCutcheon, Cancer Res., 1951, 11, 648 CAS.
- I. Zeidman, Cancer Res., 1957, 17, 157–162 CAS.
- S. Amintas, A. Bedel, F. Moreau-Gaudry, J. Boutin, L. Buscail, J.-P. Merlio, V. Vendrely, S. Dabernat and E. Buscail, Int. J. Mol. Sci., 2020, 21, 2653 CrossRef CAS PubMed.
- J. P. Thiery, H. Acloque, R. Y. Huang and M. A. Nieto, Cell, 2009, 139, 871–890 CrossRef CAS PubMed.
- W. L. Tam and R. A. Weinberg, Nat. Med., 2013, 19, 1438–1449 CrossRef CAS PubMed.
- S. Kishi, P. E. Bayliss and J. Hanai, Transl. Res., 2015, 165, 241–249 CrossRef CAS PubMed.
- E. Chargaff and R. West, J. Biol. Chem., 1946, 166, 189–197 CrossRef CAS.
- P. Wolf, Br. J. Haematol., 1967, 13, 269–288 CrossRef CAS PubMed.
- B.-T. Pan, K. Teng, C. Wu, M. Adam and R. M. Johnstone, J. Cell Biol., 1985, 101, 942–948 CrossRef CAS PubMed.
- C. Harding, J. Heuser and P. Stahl, J. Cell Biol., 1983, 97, 329–339 CrossRef CAS PubMed.
- R. M. Johnstone, M. Adam, J. Hammond, L. Orr and C. Turbide, J. Biol. Chem., 1987, 262, 9412–9420 CrossRef CAS.
- G. Raposo, H. W. Nijman, W. Stoorvogel, R. Liejendekker, C. V. Harding, C. J. Melief and H. J. Geuze, J. Exp. Med., 1996, 183, 1161–1172 CrossRef CAS PubMed.
- K. Boriachek, M. N. Islam, A. Moller, C. Salomon, N. T. Nguyen, M. S. A. Hossain, Y. Yamauchi and M. J. A. Shiddiky, Small, 2018, 14, 1702153 CrossRef PubMed.
- E. van der Pol, A. N. Boing, P. Harrison, A. Sturk and R. Nieuwland, Pharmacol. Rev., 2012, 64, 676–705 CrossRef CAS PubMed.
- G. Raposo and W. Stoorvogel, J. Cell Biol., 2013, 200, 373–383 CrossRef CAS PubMed.
- Y. Zhang, Y. Liu, H. Liu and W. H. Tang, Cell Biosci., 2019, 9, 19–19 CrossRef PubMed.
- S. Gurunathan, M.-H. Kang, M. Jeyaraj, M. Qasim and J.-H. Kim, Cell, 2019, 8, 307 CrossRef CAS PubMed.
- M. Ostrowski, N. B. Carmo, S. Krumeich, I. Fanget, G. Raposo, A. Savina, C. F. Moita, K. Schauer, A. N. Hume, R. P. Freitas, B. Goud, P. Benaroch, N. Hacohen, M. Fukuda, C. Desnos, M. C. Seabra, F. Darchen, S. Amigorena, L. F. Moita and C. Thery, Nat. Cell Biol., 2010, 12, 19–30 CrossRef CAS PubMed.
- M. C. Henderson and D. O. Azorsa, Front. Oncol., 2012, 2, 38, DOI:10.3389/fonc.2012.00038.
- H. Stenmark, Nat. Rev. Mol. Cell Biol., 2009, 10, 513–525 CrossRef CAS PubMed.
- M. Filipska and R. Rosell, Mol. Oncol., 2021, 15, 1667–1682 CrossRef PubMed.
- H. Schwarzenbach, D. S. Hoon and K. Pantel, Nat. Rev. Cancer, 2011, 11, 426–437 CrossRef CAS PubMed.
- C. Alix-Panabières, H. Schwarzenbach and K. Pantel, Annu. Rev. Med., 2012, 63, 199–215 CrossRef PubMed.
- C. Alix-Panabières and K. Pantel, Cancer Discovery, 2016, 6, 479 CrossRef PubMed.
- J. V. Canzoniero and B. H. Park, Biochim. Biophys. Acta, 2016, 1865, 266–274 CAS.
- L. Keller, Y. Belloum, H. Wikman and K. Pantel, Br. J. Cancer, 2021, 124, 345–358 CrossRef PubMed.
- C. Bettegowda, M. Sausen, R. J. Leary, I. Kinde, Y. Wang, N. Agrawal, B. R. Bartlett, H. Wang, B. Luber, R. M. Alani, E. S. Antonarakis, N. S. Azad, A. Bardelli, H. Brem, J. L. Cameron, C. C. Lee, L. A. Fecher, G. L. Gallia, P. Gibbs, D. Le, R. L. Giuntoli, M. Goggins, M. D. Hogarty, M. Holdhoff, S. M. Hong, Y. Jiao, H. H. Juhl, J. J. Kim, G. Siravegna, D. A. Laheru, C. Lauricella, M. Lim, E. J. Lipson, S. K. Marie, G. J. Netto, K. S. Oliner, A. Olivi, L. Olsson, G. J. Riggins, A. Sartore-Bianchi, K. Schmidt, M. Shih, S. M. Oba-Shinjo, S. Siena, D. Theodorescu, J. Tie, T. T. Harkins, S. Veronese, T. L. Wang, J. D. Weingart, C. L. Wolfgang, L. D. Wood, D. Xing, R. H. Hruban, J. Wu, P. J. Allen, C. M. Schmidt, M. A. Choti, V. E. Velculescu, K. W. Kinzler, B. Vogelstein, N. Papadopoulos and L. A. Diaz, Jr., Sci. Transl. Med., 2014, 6, 224ra224 Search PubMed.
- M. M. Ferreira, V. C. Ramani and S. S. Jeffrey, Mol. Oncol., 2016, 10, 374–394 CrossRef CAS PubMed.
- M. Ilie, V. Hofman, E. Long-Mira, E. Selva, J.-M. Vignaud, B. Padovani, J. Mouroux, C.-H. Marquette and P. Hofman, PLoS One, 2014, 9, e111597 CrossRef PubMed.
- E. Heitzer, M. Auer, C. Gasch, M. Pichler, P. Ulz, E. M. Hoffmann, S. Lax, J. Waldispuehl-Geigl, O. Mauermann and C. Lackner, Cancer Res., 2013, 73, 2965–2975 CrossRef CAS PubMed.
- G. Galletti, M. S. Sung, L. T. Vahdat, M. A. Shah, S. M. Santana, G. Altavilla, B. J. Kirby and P. Giannakakou, Lab Chip, 2014, 14, 147–156 RSC.
- M. Pestrin, S. Bessi, F. Galardi, M. Truglia, A. Biggeri, C. Biagioni, S. Cappadona, L. Biganzoli, A. Giannini and A. Di Leo, Breast Cancer Res. Treat., 2009, 118, 523–530 CrossRef CAS PubMed.
- C. Gasch, T. Bauernhofer, M. Pichler, S. Langer-Freitag, M. Reeh, A. M. Seifert, O. Mauermann, J. R. Izbicki, K. Pantel and S. Riethdorf, Clin. Chem., 2013, 59, 252–260 CrossRef CAS PubMed.
- D. Ramskold, S. Luo, Y. C. Wang, R. Li, Q. Deng, O. R. Faridani, G. A. Daniels, I. Khrebtukova, J. F. Loring, L. C. Laurent, G. P. Schroth and R. Sandberg, Nat. Biotechnol., 2012, 30, 777–782 CrossRef PubMed.
- F. Tang, C. Barbacioru, Y. Wang, E. Nordman, C. Lee, N. Xu, X. Wang, J. Bodeau, B. B. Tuch, A. Siddiqui, K. Lao and M. A. Surani, Nat. Methods, 2009, 6, 377–382 CrossRef CAS PubMed.
- Y. Zhang, Y. Liu, H. Liu and W. H. Tang, Cell Biosci., 2019, 9, 19 CrossRef PubMed.
- S. Mathivanan, C. J. Fahner, G. E. Reid and R. J. Simpson, Nucleic Acids Res., 2012, 40, D1241–D1244 CrossRef CAS PubMed.
- D.-K. Kim, B. Kang, O. Y. Kim, D.-s. Choi, J. Lee, S. R. Kim, G. Go, Y. J. Yoon, J. H. Kim and S. C. Jang, J. Extracell. Vesicles, 2013, 2, 20384 CrossRef PubMed.
- M. Shi, C. Liu, T. J. Cook, K. M. Bullock, Y. Zhao, C. Ginghina, Y. Li, P. Aro, R. Dator, C. He, M. J. Hipp, C. P. Zabetian, E. R. Peskind, S. C. Hu, J. F. Quinn, D. R. Galasko, W. A. Banks and J. Zhang, Acta Neuropathol., 2014, 128, 639–650 CrossRef CAS PubMed.
- M. S. Fiandaca, D. Kapogiannis, M. Mapstone, A. Boxer, E. Eitan, J. B. Schwartz, E. L. Abner, R. C. Petersen, H. J. Federoff, B. L. Miller and E. J. Goetzl, Alzheimer's Dementia, 2015, 11, 600–607.e601 CrossRef PubMed.
- H. Valadi, K. Ekström, A. Bossios, M. Sjöstrand, J. J. Lee and J. O. Lötvall, Nat. Cell Biol., 2007, 9, 654–659 CrossRef CAS PubMed.
- S. A. Melo, H. Sugimoto, J. T. O'Connell, N. Kato, A. Villanueva, A. Vidal, L. Qiu, E. Vitkin, L. T. Perelman, C. A. Melo, A. Lucci, C. Ivan, G. A. Calin and R. Kalluri, Cancer Cell, 2014, 26, 707–721 CrossRef CAS PubMed.
- G. E. Rice, K. Scholz-Romero, E. Sweeney, H. Peiris, M. Kobayashi, G. Duncombe, M. D. Mitchell and C. Salomon, J. Clin. Endocrinol. Metab., 2015, 100, E1280–E1288 CrossRef CAS PubMed.
- E. Alegre, L. Zubiri, J. L. Perez-Gracia, M. González-Cao, L. Soria, S. Martín-Algarra and A. González, Clin. Chim. Acta, 2016, 454, 28–32 CrossRef CAS PubMed.
- B. Sandfeld-Paulsen, N. Aggerholm-Pedersen, R. Bæk, K. R. Jakobsen, P. Meldgaard, B. H. Folkersen, T. R. Rasmussen, K. Varming, M. M. Jørgensen and B. S. Sorensen, Mol. Oncol., 2016, 10, 1595–1602 CrossRef CAS PubMed.
- X. Luan, K. Sansanaphongpricha, I. Myers, H. Chen, H. Yuan and D. Sun, Acta Pharmacol. Sin., 2017, 38, 754–763 CrossRef CAS PubMed.
- P. Duan, J. Tan, Y. Miao and Q. Zhang, Am. J. Transl. Res., 2019, 11, 1184–1201 CAS.
- J. M. Vicencio, D. M. Yellon, V. Sivaraman, D. Das, C. Boi-Doku, S. Arjun, Y. Zheng, J. A. Riquelme, J. Kearney, V. Sharma, G. Multhoff, A. R. Hall and S. M. Davidson, J. Am. Coll. Cardiol., 2015, 65, 1525–1536 CrossRef CAS PubMed.
- O. J. Arntz, B. C. Pieters, M. C. Oliveira, M. G. Broeren, M. B. Bennink, M. de Vries, P. L. van Lent, M. I. Koenders, W. B. van den Berg, P. M. van der Kraan and F. A. van de Loo, Mol. Nutr. Food Res., 2015, 59, 1701–1712 CrossRef CAS PubMed.
- M. Sáenz-Cuesta, I. Osorio-Querejeta and D. Otaegui, Front.
Cell. Neurosci., 2014, 8, 100 Search PubMed.
- M. J. Haney, N. L. Klyachko, Y. Zhao, R. Gupta, E. G. Plotnikova, Z. He, T. Patel, A. Piroyan, M. Sokolsky, A. V. Kabanov and E. V. Batrakova, J. Controlled Release, 2015, 207, 18–30 CrossRef CAS PubMed.
- K. Mäbert, M. Cojoc, C. Peitzsch, I. Kurth, S. Souchelnytskyi and A. Dubrovska, Int. J. Radiat. Biol., 2014, 90, 659–677 CrossRef PubMed.
- R. Agassi, D. Czeiger, G. Shaked, A. Avriel, J. Sheynin, K. Lavrenkov, S. Ariad and A. Douvdevani, Am. J. Clin. Pathol., 2015, 143, 18–24 CrossRef CAS PubMed.
- Z. Zhang, P. Chen, H. Xie and P. Cao, Cancer Med., 2020, 9, 1349–1364 CrossRef CAS PubMed.
- E. Yong, Nature News, 2014, 511, 524 CrossRef CAS PubMed.
- I. Garcia-Murillas, G. Schiavon, B. Weigelt, C. Ng, S. Hrebien, R. J. Cutts, M. Cheang, P. Osin, A. Nerurkar, I. Kozarewa, J. A. Garrido, M. Dowsett, J. S. Reis-Filho, I. E. Smith and N. C. Turner, Sci. Transl. Med., 2015, 7, 302ra133 Search PubMed.
- E. Olsson, C. Winter, A. George, Y. Chen, J. Howlin, M. H. Tang, M. Dahlgren, R. Schulz, D. Grabau, D. van Westen, M. Ferno, C. Ingvar, C. Rose, P. O. Bendahl, L. Ryden, A. Borg, S. K. Gruvberger-Saal, H. Jernstrom and L. H. Saal, EMBO Mol. Med., 2015, 7, 1034–1047 CrossRef CAS PubMed.
- F. R. Harris, I. V. Kovtun, J. Smadbeck, F. Multinu, A. Jatoi, F. Kosari, K. R. Kalli, S. J. Murphy, G. C. Halling, S. H. Johnson, M. C. Liu, A. Mariani and G. Vasmatzis, Sci. Rep., 2016, 6, 29831 CrossRef CAS PubMed.
- B. Molparia, E. Nichani and A. Torkamani, PLoS One, 2017, 12, e0180647 CrossRef PubMed.
- M. Elazezy and S. A. Joosse, Comput. Struct. Biotechnol. J., 2018, 16, 370–378 CrossRef CAS PubMed.
- K. S. Thress, C. P. Paweletz, E. Felip, B. C. Cho, D. Stetson, B. Dougherty, Z. Lai, A. Markovets, A. Vivancos and Y. Kuang, Nat. Med., 2015, 21, 560–562 CrossRef CAS PubMed.
- C. Fribbens, B. O'Leary, L. Kilburn, S. Hrebien, I. Garcia-Murillas, M. Beaney, M. Cristofanilli, F. Andre, S. Loi and S. Loibl, J. Clin. Oncol., 2016, 34, 2961–2968 CrossRef CAS PubMed.
- S. A. Joosse, T. M. Gorges and K. Pantel, EMBO Mol. Med., 2015, 7, 1–11 CrossRef CAS PubMed.
- D. Yang, W. Zhang, H. Zhang, F. Zhang, L. Chen, L. Ma, L. M. Larcher, S. Chen, N. Liu, Q. Zhao, P. H. L. Tran, C. Chen, R. N. Veedu and T. Wang, Theranostics, 2020, 10, 3684–3707 CrossRef CAS PubMed.
- A. L. Volckmar, H. Sültmann, A. Riediger, T. Fioretos, P. Schirmacher, V. Endris, A. Stenzinger and S. Dietz, Genes, Chromosomes Cancer, 2018, 57, 123–139 CrossRef CAS PubMed.
- C. Pérez-Barrios, I. Nieto-Alcolado, M. Torrente, C. Jiménez-Sánchez, V. Calvo, L. Gutierrez-Sanz, M. Palka, E. Donoso-Navarro, M. Provencio and A. Romero, Transl. Lung Cancer Res., 2016, 5, 665–672 CrossRef PubMed.
- E. E. van der Toom, V. P. Groot, S. A. Glavaris, G. Gemenetzis, H. J. Chalfin, L. D. Wood, C. L. Wolfgang, J. de la Rosette, T. M. de Reijke and K. J. Pienta, Prostate, 2018, 78, 300–307 CrossRef CAS PubMed.
- N. M. Karabacak, P. S. Spuhler, F. Fachin, E. J. Lim, V. Pai, E. Ozkumur, J. M. Martel, N. Kojic, K. Smith, P. I. Chen, J. Yang, H. Hwang, B. Morgan, J. Trautwein, T. A. Barber, S. L. Stott, S. Maheswaran, R. Kapur, D. A. Haber and M. Toner, Nat. Protoc., 2014, 9, 694–710 CrossRef CAS PubMed.
- E. Ozkumur, A. M. Shah, J. C. Ciciliano, B. L. Emmink, D. T. Miyamoto, E. Brachtel, M. Yu, P. I. Chen, B. Morgan, J. Trautwein, A. Kimura, S. Sengupta, S. L. Stott, N. M. Karabacak, T. A. Barber, J. R. Walsh, K. Smith, P. S. Spuhler, J. P. Sullivan, R. J. Lee, D. T. Ting, X. Luo, A. T. Shaw, A. Bardia, L. V. Sequist, D. N. Louis, S. Maheswaran, R. Kapur, D. A. Haber and M. Toner, Sci. Transl. Med., 2013, 5, 179ra147 Search PubMed.
- J. W. Po, A. Roohullah, D. Lynch, A. DeFazio, M. Harrison, P. R. Harnett, C. Kennedy, P. de Souza and T. M. Becker, J. Circ. Biomarkers, 2018, 7, 1849454418782617 Search PubMed.
- J. W. Po, Y. Ma, B. Balakrishna, D. Brungs, F. Azimi, P. de Souza and T. M. Becker, PLoS One, 2019, 14, e0211866 CrossRef CAS PubMed.
- D. Lynch, B. Powter, J. W. Po, A. Cooper, C. Garrett, E.-S. Koh, M. Sheridan, J. van Gelder, B. Darwish and S. Mckechnie, Appl. Sci., 2020, 10, 3338 CrossRef CAS.
- W. J. Allard, J. Matera, M. C. Miller, M. Repollet, M. C. Connelly, C. Rao, A. G. Tibbe, J. W. Uhr and L. W. Terstappen, Clin. Cancer Res., 2004, 10, 6897–6904 CrossRef PubMed.
- S. A. Mani, W. Guo, M. J. Liao, E. N. Eaton, A. Ayyanan, A. Y. Zhou, M. Brooks, F. Reinhard, C. C. Zhang, M. Shipitsin, L. L. Campbell, K. Polyak, C. Brisken, J. Yang and R. A. Weinberg, Cell, 2008, 133, 704–715 CrossRef CAS PubMed.
- N. Bednarz-Knoll, C. Alix-Panabières and K. Pantel, Cancer Metastasis Rev., 2012, 31, 673–687 CrossRef CAS PubMed.
- N. Zarovni, A. Corrado, P. Guazzi, D. Zocco, E. Lari, G. Radano, J. Muhhina, C. Fondelli, J. Gavrilova and A. Chiesi, Methods, 2015, 87, 46–58 CrossRef CAS PubMed.
- A. Clayton, J. Court, H. Navabi, M. Adams, M. D. Mason, J. A. Hobot, G. R. Newman and B. Jasani, J. Immunol. Methods, 2001, 247, 163–174 CrossRef CAS PubMed.
- K. R. Jakobsen, B. S. Paulsen, R. Baek, K. Varming, B. S. Sorensen and M. M. Jorgensen, J. Extracell. Vesicles, 2015, 4, 26659 CrossRef PubMed.
- M. P. Oksvold, A. Neurauter and K. W. Pedersen, Methods Mol. Biol., 2015, 1218, 465–481 CrossRef CAS PubMed.
- K. Koga, K. Matsumoto, T. Akiyoshi, M. Kubo, N. Yamanaka, A. Tasaki, H. Nakashima, M. Nakamura, S. Kuroki, M. Tanaka and M. Katano, Anticancer Res., 2005, 25, 3703–3707 CAS.
- S. Nagrath, L. V. Sequist, S. Maheswaran, D. W. Bell, D. Irimia, L. Ulkus, M. R. Smith, E. L. Kwak, S. Digumarthy and A. Muzikansky, Nature, 2007, 450, 1235–1239 CrossRef CAS PubMed.
- A. F. Sarioglu, N. Aceto, N. Kojic, M. C. Donaldson, M. Zeinali, B. Hamza, A. Engstrom, H. Zhu, T. K. Sundaresan and D. T. Miyamoto, Nat. Methods, 2015, 12, 685–691 CrossRef CAS PubMed.
- S. L. Stott, C. H. Hsu, D. I. Tsukrov, M. Yu, D. T. Miyamoto, B. A. Waltman, S. M. Rothenberg, A. M. Shah, M. E. Smas, G. K. Korir, F. P. Floyd, Jr., A. J. Gilman, J. B. Lord, D. Winokur, S. Springer, D. Irimia, S. Nagrath, L. V. Sequist, R. J. Lee, K. J. Isselbacher, S. Maheswaran, D. A. Haber and M. Toner, Proc. Natl. Acad. Sci. U. S. A., 2010, 107, 18392–18397 CrossRef CAS PubMed.
- A. E. Saliba, L. Saias, E. Psychari, N. Minc, D. Simon, F. C. Bidard, C. Mathiot, J. Y. Pierga, V. Fraisier, J. Salamero, V. Saada, F. Farace, P. Vielh, L. Malaquin and J. L. Viovy, Proc. Natl. Acad. Sci. U. S. A., 2010, 107, 14524–14529 CrossRef CAS PubMed.
- W. Liang, J. Liu, X. Yang, Q. Zhang, W. Yang, H. Zhang and L. Liu, Microfluid. Nanofluid., 2020, 24, 26 CrossRef.
- K. Lee, H. Shao, R. Weissleder and H. Lee, ACS Nano, 2015, 9, 2321–2327 CrossRef CAS PubMed.
- S. S. Kanwar, C. J. Dunlay, D. M. Simeone and S. Nagrath, Lab Chip, 2014, 14, 1891–1900 RSC.
- F. F. Becker, X. B. Wang, Y. Huang, R. Pethig, J. Vykoukal and P. R. Gascoyne, Proc. Natl. Acad. Sci. U. S. A., 1995, 92, 860–864 CrossRef CAS PubMed.
- R. D. Stoy, K. R. Foster and H. P. Schwan, Phys. Med. Biol., 1982, 27, 501–513 CrossRef CAS PubMed.
- S. Y. Ng, J. Reboud, K. Y. Wang, K. C. Tang, L. Zhang, P. Wong, K. T. Moe, W. Shim and Y. Chen, Biosens. Bioelectron., 2010, 25, 1095–1101 CrossRef CAS PubMed.
- C. Jin, S. M. McFaul, S. P. Duffy, X. Deng, P. Tavassoli, P. C. Black and H. Ma, Lab Chip, 2014, 14, 32–44 RSC.
- M. Poudineh, E. H. Sargent, K. Pantel and S. O. Kelley, Nat. Biomed. Eng., 2018, 2, 72–84 CrossRef CAS PubMed.
- V. Gupta, I. Jafferji, M. Garza, V. O. Melnikova, D. K. Hasegawa, R. Pethig and D. W. Davis, Biomicrofluidics, 2012, 6, 24133 CrossRef PubMed.
- N. Manaresi, A. Romani, G. Medoro, L. Altomare, A. Leonardi, M. Tartagni and R. Guerrieri, IEEE J. Solid-State Circuits, 2003, 38, 2297–2305 CrossRef.
- E. L. Carpenter, J. Rader, J. Ruden, E. F. Rappaport, K. N. Hunter, P. L. Hallberg, K. Krytska, P. J. O'Dwyer and Y. P. Mosse, Front. Oncol., 2014, 4, 201–201 Search PubMed.
- B. Mesquita, D. G. Rothwell, D. J. Burt, F. Chemi, F. Fernandez-Gutierrez, D. Slane-Tan, J. Antonello, M. Carter, L. Carter, M. Parry, L. Franklin, R. Marais, F. Blackhall, C. Dive and G. Brady, Mol. Oncol., 2017, 11, 1687–1697 CrossRef CAS PubMed.
- B. Polzer, G. Medoro, S. Pasch, F. Fontana, L. Zorzino, A. Pestka, U. Andergassen, F. Meier-Stiegen, Z. T. Czyz, B. Alberter, S. Treitschke, T. Schamberger, M. Sergio, G. Bregola, A. Doffini, S. Gianni, A. Calanca, G. Signorini, C. Bolognesi, A. Hartmann, P. A. Fasching, M. T. Sandri, B. Rack, T. Fehm, G. Giorgini, N. Manaresi and C. A. Klein, EMBO Mol. Med., 2014, 6, 1371–1386 CrossRef CAS PubMed.
- D. J. Peeters, B. De Laere, G. G. Van den Eynden, S. J. Van Laere, F. Rothe, M. Ignatiadis, A. M. Sieuwerts, D. Lambrechts, A. Rutten, P. A. van Dam, P. Pauwels, M. Peeters, P. B. Vermeulen and L. Y. Dirix, Br. J. Cancer, 2013, 108, 1358–1367 CrossRef CAS PubMed.
-
C. Théry, S. Amigorena, G. Raposo and A. Clayton, Curr. Protoc. Cell Biol., 2006, ch. 3, Unit 3.22 Search PubMed.
- H. G. Lamparski, A. Metha-Damani, J.-Y. Yao, S. Patel, D.-H. Hsu, C. Ruegg and J.-B. Le Pecq, J. Immunol. Methods, 2002, 270, 211–226 CrossRef CAS PubMed.
- E. Zeringer, T. Barta, M. Li and A. V. Vlassov, Cold Spring Harb. Protoc., 2015, 2015 DOI:10.1101/pdb.top074476.
- M. L. Merchant, D. W. Powell, D. W. Wilkey, T. D. Cummins, J. K. Deegens, I. M. Rood, K. J. McAfee, C. Fleischer, E. Klein and J. B. Klein, Proteomics: Clin. Appl., 2010, 4, 84–96 CAS.
- A. Jemal, R. Siegel, E. Ward, T. Murray, J. Q. Xu and M. J. Thun, Ca-Cancer J. Clin., 2007, 57, 43–66 CrossRef PubMed.
- A. G.-E. Ibrahim, K. Cheng and E. Marbán, Stem Cell Rep., 2014, 2, 606–619 CrossRef CAS PubMed.
- E. van der Pol, A. N. Boing, E. L. Gool and R. Nieuwland, J. Thromb. Haemostasis, 2016, 14, 48–56 CrossRef CAS PubMed.
- M. L. Alvarez, M. Khosroheidari, R. Kanchi Ravi and J. K. DiStefano, Kidney Int., 2012, 82, 1024–1032 CrossRef CAS PubMed.
- Y. Weng, Z. Sui, Y. Shan, Y. Hu, Y. Chen, L. Zhang and Y. Zhang, Analyst, 2016, 141, 4640–4646 RSC.
- M. F. Peterson, N. Otoc, J. K. Sethi, A. Gupta and T. J. Antes, Methods, 2015, 87, 31–45 CrossRef CAS PubMed.
- D.-M. Zhu, L. Wu, M. Suo, S. Gao, W. Xie, M.-H. Zan, A. Liu, B. Chen, W.-T. Wu and L.-W. Ji, Nanoscale, 2018, 10, 6014–6023 RSC.
- E. Politaki, S. Agelaki, S. Apostolaki, D. Hatzidaki, A. Strati, F. Koinis, M. Perraki, G. Saloustrou, G. Stoupis and G. Kallergi, Cell. Physiol. Biochem., 2017, 44, 594–606 CrossRef PubMed.
- K. Xiong, W. Wei, Y. Jin, S. Wang, D. Zhao, S. Wang, X. Gao, C. Qiao, H. Yue and G. Ma, Adv. Mater., 2016, 28, 7929–7935 CrossRef CAS PubMed.
- A. Drucker, E. M. Teh, R. Kostyleva, D. Rayson, S. Douglas and D. M. Pinto, PLoS One, 2020, 15, e0237308 CrossRef CAS PubMed.
- Y. J. Jan, J.-F. Chen, Y. Zhu, Y.-T. Lu, S. H. Chen, H. Chung, M. Smalley, Y.-W. Huang, J. Dong and L.-C. Chen, Adv. Drug Delivery Rev., 2018, 125, 78–93 CrossRef CAS PubMed.
- M. S. Loeian, S. M. Aghaei, F. Farhadi, V. Rai, H. W. Yang, M. D. Johnson, F. Aqil, M. Mandadi, S. N. Rai and B. Panchapakesan, Lab Chip, 2019, 19, 1899–1915 RSC.
- J. Mong and M.-H. Tan, Trends Biotechnol., 2018, 36, 511–522 CrossRef CAS PubMed.
- S.-J. Hao, Y. Wan, Y.-Q. Xia, X. Zou and S.-Y. Zheng, Adv. Drug Delivery Rev., 2018, 125, 3–20 CrossRef CAS PubMed.
- G. Kallergi, E. Politaki, S. Alkahtani, C. Stournaras and V. Georgoulias, Cell. Physiol. Biochem., 2016, 40, 411–419 CrossRef CAS PubMed.
- M. Hakim, F. Khorasheh, I. Alemzadeh and M. Vossoughi, Anal. Chim. Acta, 2021, 1186, 339115 CrossRef CAS PubMed.
- H. S. Rugo, J. Cortes, A. Awada, J. O'Shaughnessy, C. Twelves, S.-A. Im, A. Hannah, L. Lu, S. Sy and K. Caygill, Clin. Cancer Res., 2018, 24, 3348–3357 CrossRef CAS PubMed.
- D. E. Campton, A. B. Ramirez, J. J. Nordberg, N. Drovetto, A. C. Clein, P. Varshavskaya, B. H. Friemel, S. Quarre, A. Breman and M. Dorschner, BMC Cancer, 2015, 15, 1–13 CrossRef CAS PubMed.
- F. Ge, H. Zhang, D. D. Wang, L. Li and P. P. Lin, Oncotarget, 2015, 6, 27049 CrossRef PubMed.
- M.-H. Park, E. Reátegui, W. Li, S. N. Tessier, K. H. Wong, A. E. Jensen, V. Thapar, D. Ting, M. Toner and S. L. Stott, J. Am. Chem. Soc., 2017, 139, 2741–2749 CrossRef CAS PubMed.
- H. J. Yoon, A. Shanker, Y. Wang, M. Kozminsky, Q. Jin, N. Palanisamy, M. L. Burness, E. Azizi, D. M. Simeone and M. S. Wicha, Adv. Mater., 2016, 28, 4891–4897 CrossRef CAS PubMed.
- M. A. Livshits, E. Khomyakova, E. G. Evtushenko, V. N. Lazarev, N. A. Kulemin, S. E. Semina, E. V. Generozov and V. M. Govorun, Sci. Rep., 2015, 5, 1–14 Search PubMed.
-
F. Momen-Heravi, in Extracellular Vesicles, 2017, pp. 25–32 Search PubMed.
- P. Li, M. Kaslan, S. H. Lee, J. Yao and Z. Gao, Theranostics, 2017, 7, 789 CrossRef CAS PubMed.
- P. Sharma, S. Ludwig, L. Muller, C. S. Hong, J. M. Kirkwood, S. Ferrone and T. L. Whiteside, J. Extracell. Vesicles, 2018, 7, 1435138 CrossRef PubMed.
- T. Shtam, V. Evtushenko, R. Samsonov, Y. Zabrodskaya, R. Kamyshinsky, L. Zabegina, N. Verlov, V. Burdakov, L. Garaeva and M. Slyusarenko, PLoS One, 2020, 15, e0242732 CrossRef CAS PubMed.
- A. D. Foers, S. Chatfield, L. F. Dagley, B. J. Scicluna, A. I. Webb, L. Cheng, A. F. Hill, I. P. Wicks and K. C. Pang, J. Extracell. Vesicles, 2018, 7, 1490145 CrossRef PubMed.
- K. Heider, J. C. M. Wan, J. Hall, J. Belic, S. Boyle, I. Hudecova, D. Gale, W. N. Cooper, P. G. Corrie, J. D. Brenton, C. G. Smith and N. Rosenfeld, Clin. Chem., 2020, 66, 697–705 CrossRef PubMed.
- D. Sefrioui, L. Beaussire, A. Perdrix, F. Clatot, P. Michel, T. Frebourg, F. Di Fiore and N. Sarafan-Vasseur, Clin. Biochem., 2017, 50, 963–966 CrossRef CAS PubMed.
- Z. Cai, Z. Wang, C. Liu, D. Shi, D. Li, M. Zheng, H. Han-Zhang, A. Lizaso, J. Xiang and J. Lv, J. Mol. Diagn., 2020, 22, 860–870 CrossRef CAS PubMed.
- C. Bourrier, J.-Y. Pierga, L. Xuereb, H. Salaun, C. Proudhon, M. R. Speicher, J. Belic, E. Heitzer, B. P. Lockhart and N. Guigal-Stephan, Cancers, 2020, 12, 1481 CrossRef CAS PubMed.
- M. Zavridou, S. Mastoraki, A. Strati, G. Koutsodontis, A. Klinakis, A. Psyrri and E. Lianidou, Sci. Rep., 2020, 10, 6551 CrossRef CAS PubMed.
- A. J. Rushton, G. Nteliopoulos, J. A. Shaw and R. C. Coombes, Cancers, 2021, 13 Search PubMed.
- A. A. Adams, P. I. Okagbare, J. Feng, M. L. Hupert, D. Patterson, J. Göttert, R. L. McCarley, D. Nikitopoulos, M. C. Murphy and S. A. Soper, J. Am. Chem. Soc., 2008, 130, 8633–8641 CrossRef CAS PubMed.
- C. E. Peters, D. Maestre-Battle, S. M. Woodside, T. E. Thomas and A. C. Eaves, Cancer Res., 2016, 76, 511 CrossRef PubMed.
- F. Fontana, C. Rapone, G. Bregola, R. Aversa, A. de Meo, G. Signorini, M. Sergio, A. Ferrarini, R. Lanzellotto, G. Medoro, G. Giorgini, N. Manaresi and A. Berti, Forensic Sci. Int.: Genet., 2017, 29, 225–241 CrossRef CAS PubMed.
- W. Zhao, R. Cheng, B. D. Jenkins, T. Zhu, N. E. Okonkwo, C. E. Jones, M. B. Davis, S. K. Kavuri, Z. Hao, C. Schroeder and L. Mao, Lab Chip, 2017, 17, 3097–3111 RSC.
- Y. J. Kim, Y. T. Kang and Y. H. Cho, Anal. Chem., 2016, 88, 7938–7945 CrossRef CAS PubMed.
- S. Shen, C. Tian, T. Li, J. Xu, S.-W. Chen, Q. Tu, M.-S. Yuan, W. Liu and J. Wang, Lab Chip, 2017, 17, 3578–3591 RSC.
- G. K. Patel, M. A. Khan, H. Zubair, S. K. Srivastava, M. d. Khushman, S. Singh and A. P. Singh, Sci. Rep., 2019, 9, 5335 CrossRef PubMed.
- K. Brennan, K. Martin, S. P. FitzGerald, J. O'Sullivan, Y. Wu, A. Blanco, C. Richardson and M. M. Mc Gee, Sci. Rep., 2020, 10, 1039 CrossRef CAS PubMed.
- Y.-T. Tang, Y.-Y. Huang, L. Zheng, S.-H. Qin, X.-P. Xu, T.-X. An, Y. Xu, Y.-S. Wu, X.-M. Hu and B.-H. Ping, Int. J. Mol. Med., 2017, 40, 834–844 CrossRef CAS PubMed.
- J. Van Deun, P. Mestdagh, R. Sormunen, V. Cocquyt, K. Vermaelen, J. Vandesompele, M. Bracke, O. De Wever and A. Hendrix, J. Extracell. Vesicles, 2014, 3, 24858 CrossRef PubMed.
- M. Zhou, S. R. Weber, Y. Zhao, H. Chen and J. M. Sundstrom, Exosomes, 2020, 23–38 Search PubMed.
- C. S. Hong, S. Funk, L. Muller, M. Boyiadzis and T. L. Whiteside, J. Extracell. Vesicles, 2016, 5, 29289 CrossRef PubMed.
- M. Wu, Y. Ouyang, Z. Wang, R. Zhang, P.-H. Huang, C. Chen, H. Li, P. Li, D. Quinn, M. Dao, S. Suresh, Y. Sadovsky and T. J. Huang, Proc. Natl. Acad. Sci. U. S. A., 2017, 114, 10584 CrossRef CAS PubMed.
- M. Lyu, J. Zhou, K. Ning and B. Ying, OncoTargets Ther., 2019, 12, 2539–2552 CrossRef CAS PubMed.
- M. Lim, C.-J. Kim, V. Sunkara, M.-H. Kim and Y.-K. Cho, Micromachines, 2018, 9 Search PubMed.
- A. Junca, G. Tachon, C. Evrard, C. Villalva, E. Frouin, L. Karayan-Tapon and D. Tougeron, Cancers, 2020, 12 Search PubMed.
- B. J. Green, T. Saberi Safaei, A. Mepham, M. Labib, R. M. Mohamadi and S. O. Kelley, Angew. Chem., Int. Ed., 2016, 55, 1252–1265 CrossRef CAS PubMed.
- J. H. Myung, K. A. Tam, S. j. Park, A. Cha and S. Hong, Wiley Interdiscip. Rev.: Nanomed. Nanobiotechnol., 2016, 8, 223–239 Search PubMed.
- N. D. Israelsen, C. Hanson and E. Vargis, Sci. World J., 2015, 2015 Search PubMed.
- J. Xu, Z. Mo, D. Ye, M. Wang, F. Liu, G. Jin, C. Xu, X. Wang, Q. Shao, Z. Chen, Z. Tao, J. Qi, F. Zhou, Z. Wang, Y. Fu, D. He, Q. Wei, J. Guo, D. Wu, X. Gao, J. Yuan, G. Wang, Y. Xu, G. Wang, H. Yao, P. Dong, Y. Jiao, M. Shen, J. Yang, J. Ou-Yang, H. Jiang, Y. Zhu, S. Ren, Z. Zhang, C. Yin, X. Gao, B. Dai, Z. Hu, Y. Yang, Q. Wu, H. Chen, P. Peng, Y. Zheng, X. Zheng, Y. Xiang, J. Long, J. Gong, R. Na, X. Lin, H. Yu, Z. Wang, S. Tao, J. Feng, J. Sun, W. Liu, A. Hsing, J. Rao, Q. Ding, F. Wiklund, H. Gronberg, X.-O. Shu, W. Zheng, H. Shen, L. Jin, R. Shi, D. Lu, X. Zhang, J. Sun, S. L. Zheng and Y. Sun, Nat. Genet., 2012, 44, 1231–1235 CrossRef CAS PubMed.
- M. B. Freidin, D. V. Freydina, M. Leung, A. Montero Fernandez, A. G. Nicholson and E. Lim, Clin. Chem., 2015, 61, 1299–1304 CrossRef CAS PubMed.
- E. Castellanos-Rizaldos, P. Liu, C. A. Milbury, M. Guha, A. Brisci, L. Cremonesi, M. Ferrari, H. Mamon and G. M. Makrigiorgos, Clin. Chem., 2012, 58, 1130–1138 CrossRef CAS PubMed.
- Y. Nagai, H. Miyazawa, Huqun, T. Tanaka, K. Udagawa, M. Kato, S. Fukuyama, A. Yokote, K. Kobayashi, M. Kanazawa and K. Hagiwara, Cancer Res., 2005, 65, 7276–7282 CrossRef CAS PubMed.
- S. Volik, M. Alcaide, R. D. Morin and C. Collins, Mol. Cancer Res., 2016, 14, 898–908 CrossRef CAS PubMed.
- G. R. Oxnard, K. S. Thress, R. S. Alden, R. Lawrance, C. P. Paweletz, M. Cantarini, J. C.-H. Yang, J. C. Barrett and P. A. Jänne, J. Clin. Oncol., 2016, 34, 3375 CrossRef CAS PubMed.
- D. Dressman, H. Yan, G. Traverso, K. W. Kinzler and B. Vogelstein, Proc. Natl. Acad. Sci. U. S. A., 2003, 100, 8817–8822 CrossRef CAS PubMed.
- M. Li, F. Diehl, D. Dressman, B. Vogelstein and K. W. Kinzler, Nat. Methods, 2006, 3, 95–97 CrossRef CAS PubMed.
- F. Diehl, M. Li, D. Dressman, Y. He, D. Shen, S. Szabo, L. A. Diaz, S. N. Goodman, K. A. David and H. Juhl, Proc. Natl. Acad. Sci. U. S. A., 2005, 102, 16368–16373 CrossRef CAS PubMed.
- L. Dong, Y. Meng, Z. Sui, J. Wang, L. Wu and B. Fu, Sci. Rep., 2015, 5, 13174 CrossRef CAS PubMed.
- E. Takai and S. Yachida, World J. Gastroenterol., 2016, 22, 8480–8488 CrossRef CAS PubMed.
- Z. X. Yeo, M. Chan, Y. S. Yap, P. Ang, S. Rozen and A. S. G. Lee, PLoS One, 2012, 7, e45798 CrossRef CAS PubMed.
- T. Forshew, M. Murtaza, C. Parkinson, D. Gale, D. W. Tsui, F. Kaper, S. J. Dawson, A. M. Piskorz, M. Jimenez-Linan, D. Bentley, J. Hadfield, A. P. May, C. Caldas, J. D. Brenton and N. Rosenfeld, Sci. Transl. Med., 2012, 4, 136ra168 CrossRef PubMed.
- A. M. Newman, S. V. Bratman, J. To, J. F. Wynne, N. C. Eclov, L. A. Modlin, C. L. Liu, J. W. Neal, H. A. Wakelee, R. E. Merritt, J. B. Shrager, B. W. Loo, Jr., A. A. Alizadeh and M. Diehn, Nat. Med., 2014, 20, 548–554 CrossRef CAS PubMed.
- S. Riethdorf, H. Fritsche, V. Muller, T. Rau, C. Schindlbeck, B. Rack, W. Janni, C. Coith, K. Beck, F. Janicke, S. Jackson, T. Gornet, M. Cristofanilli and K. Pantel, Clin. Cancer Res., 2007, 13, 920–928 CrossRef CAS PubMed.
- D. R. Shaffer, M. A. Leversha, D. C. Danila, O. Lin, R. Gonzalez-Espinoza, B. Gu, A. Anand, K. Smith, P. Maslak, G. V. Doyle, L. W. Terstappen, H. Lilja, G. Heller, M. Fleisher and H. I. Scher, Clin. Cancer Res., 2007, 13, 2023–2029 CrossRef CAS PubMed.
- T. M. Gorges, A. Stein, J. Quidde, S. Hauch, K. Röck, S. Riethdorf, S. A. Joosse and K. Pantel, PLoS One, 2016, 11, e0155126 CrossRef PubMed.
- A. Kuske, T. M. Gorges, P. Tennstedt, A.-K. Tiebel, R. Pompe, F. Preißer, S. Prues, M. Mazel, A. Markou, E. Lianidou, S. Peine, C. Alix-Panabières, S. Riethdorf, B. Beyer, T. Schlomm and K. Pantel, Sci. Rep., 2016, 6, 39736 CrossRef CAS PubMed.
- B. K. Van Weemen and A. H. Schuurs, FEBS Lett., 1971, 15, 232–236 CrossRef CAS PubMed.
- E. Engvall and P. Perlmann, Immunochemistry, 1971, 8, 871–874 CrossRef CAS PubMed.
- K. Boriachek, M. K. Masud, C. Palma, H. P. Phan, Y. Yamauchi, M. S. A. Hossain, N. T. Nguyen, C. Salomon and M. J. A. Shiddiky, Anal. Chem., 2019, 91, 3827–3834 CrossRef CAS PubMed.
- N. Soda, Z. J. Gonzaga, S. Chen, K. M. Koo, N.-T. Nguyen, M. J. A. Shiddiky and B. H. A. Rehm, ACS Appl. Mater. Interfaces, 2021, 13, 31418–31430 CrossRef CAS PubMed.
- R. N. Zare and S. Kim, Annu. Rev. Biomed. Eng., 2010, 12, 187–201 CrossRef CAS PubMed.
- P. N. Nge, C. I. Rogers and A. T. Woolley, Chem. Rev., 2013, 113, 2550–2583 CrossRef CAS PubMed.
- Z. Zhao, Y. Yang, Y. Zeng and M. He, Lab Chip, 2016, 16, 489–496 RSC.
- R. Vaidyanathan, M. Naghibosadat, S. Rauf, D. Korbie, L. G. Carrascosa, M. J. A. Shiddiky and M. Trau, Anal. Chem., 2014, 86, 11125–11132 CrossRef CAS PubMed.
- C. D. Chin, V. Linder and S. K. Sia, Lab Chip, 2012, 12, 2118–2134 RSC.
- U. Erdbrügger and J. Lannigan, Cytometry, Part A, 2016, 89, 123–134 CrossRef PubMed.
- E. van der Pol, A. G. Hoekstra, A. Sturk, C. Otto, T. G. van Leeuwen and R. Nieuwland, J. Thromb. Haemostasis, 2010, 8, 2596–2607 CrossRef CAS PubMed.
- W. L. Chandler, W. Yeung and J. F. Tait, J. Thromb. Haemostasis, 2011, 9, 1216–1224 CrossRef CAS PubMed.
- E. van der Pol, M. J. van Gemert, A. Sturk, R. Nieuwland and T. G. van Leeuwen, J. Thromb. Haemostasis, 2012, 10, 919–930 CrossRef CAS PubMed.
- D. J. Faber, M. C. G. Aalders, E. G. Mik, B. A. Hooper, M. J. C. van Gemert and T. G. van Leeuwen, Phys. Rev. Lett., 2004, 93, 4 CrossRef PubMed.
- T. G. Mason, K. Ganesan, J. H. van Zanten, D. Wirtz and S. C. Kuo, Phys. Rev. Lett., 1997, 79, 3282–3285 CrossRef CAS.
- Y. Song, T. Xu, L.-P. Xu and X. Zhang, Chem. Commun., 2019, 55, 1742–1745 RSC.
- T. Xu, Y. Song, W. Gao, T. Wu, L.-P. Xu, X. Zhang and S. Wang, ACS Sens., 2018, 3, 72–78 CrossRef CAS PubMed.
- Y. G. Zhou, R. M. Mohamadi, M. Poudineh, L. Kermanshah, S. Ahmed, T. S. Safaei, J. Stojcic, R. K. Nam, E. H. Sargent and S. O. Kelley, Small, 2016, 12, 727–732 CrossRef CAS PubMed.
- F. Z. Farhana, M. Umer, A. Saeed, A. S. Pannu, M. Shahbazi, A. Jabur, H. J. Nam, K. Ostrikov, P. Sonar, S. H. Firoz and M. J. A. Shiddiky, ACS Appl. Nano Mater., 2021, 4, 1175–1186 CrossRef CAS.
- J. Cao, X.-P. Zhao, M. R. Younis, Z.-Q. Li, X.-H. Xia and C. Wang, Anal. Chem., 2017, 89, 10957–10964 CrossRef CAS PubMed.
- Y. Wan, Y.-G. Zhou, M. Poudineh, T. S. Safaei, R. M. Mohamadi, E. H. Sargent and S. O. Kelley, Angew. Chem., Int. Ed., 2014, 53, 13145–13149 CrossRef CAS PubMed.
- C. Zhang, Y. Che, Z. Zhang, X. Yang and L. Zang, Chem. Commun., 2011, 47, 2336–2338 RSC.
- X. Zhou, Y. Li, H. Wu, W. Huang, H. Ju and S. Ding, Biosens. Bioelectron., 2019, 130, 88–94 CrossRef CAS PubMed.
- M. Rahman, D. Cui, S. Zhou, A. Zhang and D. Chen, Anal. Methods, 2020, 12, 440–447 RSC.
- D. Chen, Y. Wu, S. Hoque, R. D. Tilley and J. J. Gooding, Chem. Sci., 2021, 12, 5196–5201 RSC.
- N. Soda, Z. J. Gonzaga, A. S. Pannu, N. Kashaninejad, R. Kline, C. Salomon, N.-T. Nguyen, P. Sonar, B. H. A. Rehm and M. J. A. Shiddiky, Cancers, 2021, 13 Search PubMed.
- M. Tamminga, K. C. Andree, T. J. N. Hiltermann, M. Jayat, E. Schuuring, H. van den Bos, D. C. J. Spierings, P. M. Lansdorp, W. Timens, L. Terstappen and H. J. M. Groen, Cancers, 2020, 12, 896 CrossRef CAS PubMed.
- N. Beije, A. Jager and S. Sleijfer, Cancer Treat. Rev., 2015, 41, 144–150 CrossRef PubMed.
- F. Guo, Z. Mao, Y. Chen, Z. Xie, J. P. Lata, P. Li, L. Ren, J. Liu, J. Yang and M. Dao, Proc. Natl. Acad. Sci. U. S. A., 2016, 113, 1522–1527 CrossRef CAS PubMed.
- J. Lu, T. Fan, Q. Zhao, W. Zeng, E. Zaslavsky, J. J. Chen, M. A. Frohman, M. G. Golightly, S. Madajewicz and W.-T. Chen, Int. J. Cancer Res., 2010, 126, 669–683 CrossRef CAS PubMed.
- N. M. Karabacak, P. S. Spuhler, F. Fachin, E. J. Lim, V. Pai, E. Ozkumur, J. M. Martel, N. Kojic, K. Smith, P.-i. Chen, J. Yang, H. Hwang, B. Morgan, J. Trautwein, T. A. Barber, S. L. Stott, S. Maheswaran, R. Kapur, D. A. Haber and M. Toner, Nat. Protoc., 2014, 9, 694–710 CrossRef CAS PubMed.
- W. Waheed, A. Alazzam, B. Mathew, N. Christoforou and E. Abu-Nada, J. Chromatogr., B, 2018, 1087, 133–137 CrossRef PubMed.
- J. E. Hardingham, D. Kotasek, B. Farmer, R. N. Butler, J. X. Mi, R. E. Sage and A. Dobrovic, Cancer Res., 1993, 53, 3455–3458 CAS.
- A.-E. Saliba, L. Saias, E. Psychari, N. Minc, D. Simon, F.-C. Bidard, C. Mathiot, J.-Y. Pierga, V. Fraisier, J. Salamero, V. Saada, F. Farace, P. Vielh, L. Malaquin and J.-L. Viovy, Proc. Natl. Acad. Sci. U. S. A., 2010, 107, 14524 CrossRef CAS PubMed.
- L. Cayrefourcq, A. De Roeck, C. Garcia, P.-E. Stoebner, F. Fichel, F. Garima, F. Perriard, J.-P. Daures, L. Meunier and C. Alix-Panabières, Cell, 2019, 8, 755 CrossRef CAS PubMed.
- K. Luecke, L. Gasiorowski, S. Herold, N. Brychta, G. Gallerani, T. Krahn, F. Fabbri, W. Dyszkiewicz and C. Schumann, J. Clin. Oncol., 2015, 33, e22035 Search PubMed.
- V. De Giorgi, P. Pinzani, F. Salvianti, M. Grazzini, C. Orlando, T. Lotti, M. Pazzagli and D. Massi, Arch. Dermatol., 2010, 146, 1120–1124 Search PubMed.
- S. Wang, A. Thomas, E. Lee, S. Yang, X. Cheng and Y. Liu, Analyst, 2016, 141, 2228–2237 RSC.
- M. Dehghani, S. M. Gulvin, J. Flax and T. R. Gaborski, Sci. Rep., 2020, 10, 1–10 CrossRef PubMed.
- T. S. Lyu, Y. Ahn, Y.-J. Im, S.-S. Kim, K.-H. Lee, J. Kim, Y. Choi, D. Lee, E. Kang and G. Jin, PLoS One, 2021, 16, e0231994 CrossRef CAS PubMed.
- M. N. Theodoraki, C. S. Hong, V. S. Donnenberg, A. D. Donnenberg and T. L. Whiteside, Cytometry, Part A, 2021, 99, 372–381 CrossRef CAS PubMed.
- A. Emelyanov, T. Shtam, R. Kamyshinsky, L. Garaeva, N. Verlov, I. Miliukhina, A. Kudrevatykh, G. Gavrilov, Y. Zabrodskaya and S. Pchelina, PLoS One, 2020, 15, e0227949 CrossRef CAS PubMed.
- H. Shao, H. Im, C. M. Castro, X. Breakefield, R. Weissleder and H. Lee, Chem. Rev., 2018, 118, 1917–1950 CrossRef CAS PubMed.
- M. K. Jung and J. Y. Mun, J. Visualized Exp., 2018, 131, e56482 Search PubMed.
- P. Chen, A. Ruan, J. Zhou, L. Huang, X. Zhang, Y. Ma and Q. Wang, J. Orthop. Surg. Res., 2020, 15, 1–8 CrossRef PubMed.
- P. Ye, P. Cai, J. Xie and Y. Wei, PLoS One, 2021, 16, e0248775 CrossRef CAS PubMed.
- S. Li, G. Zhang, X. Li, X. Li, X. Chen, Y. Xu and H. Ren, Pharmacogenomics, 2021, 12, 735260 Search PubMed.
- A. Billaud, V. Verriele, J. Dauvé, L.-M. Chevalier and A. Morel, Diagnostics, 2020, 10, 527 CrossRef CAS PubMed.
- S. Galbiati, F. Damin, V. Burgio, A. Brisci, N. Soriani, B. Belcastro, C. Di Resta, L. Gianni, M. Chiari and M. Ronzoni, Clin. Chim. Acta, 2019, 489, 136–143 CrossRef CAS PubMed.
- C. Cordova, M. M. Syeda, B. Corless, J. M. Wiggins, A. Patel, S. C. Kurz, M. Delara, Z. Sawaged, M. Utate and D. Placantonakis, J. Clin. Oncol., 2019, 37, 2026 Search PubMed.
- Z. Zou, P. Qi, Z. Qing, J. Zheng, S. Yang, W. Chen and R. Yang, TrAC, Trends Anal. Chem., 2017, 97, 36–49 CrossRef CAS.
- N. Lyu, V. K. Rajendran, R. J. Diefenbach, K. Charles, S. J. Clarke, A. Engel, Sydney Colorectal Cancer Study I., H. Rizos, M. P. Molloy and Y. Wang, Nanotheranostics, 2020, 4, 224–232 CrossRef PubMed.
- A. H. Nguyen and S. J. Sim, Biosens. Bioelectron., 2015, 67, 443–449 CrossRef CAS PubMed.
- E. Lau, P. McCoy, F. Reeves, K. Chow, M. Clarkson, E. M. Kwan, K. Packwood, H. Northen, M. He, Z. Kingsbury, S. Mangiola, M. Kerger, M. A. Furrer, H. Crowe, A. J. Costello, D. J. McBride, M. T. Ross, B. Pope, C. M. Hovens and N. M. Corcoran, Genome Med., 2020, 12, 72 CrossRef CAS PubMed.
- A. Ståhlberg, P. M. Krzyzanowski, J. B. Jackson, M. Egyud, L. Stein and T. E. Godfrey, Nucleic Acids Res., 2016, 44, e105 CrossRef PubMed.
- T. Noguchi, K. Sakai, N. Iwahashi, K. Matsuda, H. Matsukawa, T. Yahata, S. Toujima, K. Nishio and K. Ino, Oncol. Lett., 2020, 19, 2713–2720 Search PubMed.
- I. S. Tiong, C. Wilson, S. Yerneni, J. Markham, K. Dun, A. Bajel, E. R. Thompson, D. A. Westerman and P. Blombery, Blood, 2020, 136, 39–40 CrossRef.
- K. Jacob, C. Sollier and N. Jabado, Expert Rev. Proteomics, 2007, 4, 741–756 CrossRef CAS PubMed.
- H. Hugenschmidt, K. J. Labori, E. Borgen, C. Brunborg, C. B. Schirmer, L. T. Seeberg, B. Naume and G. Wiedswang, Cancers, 2021, 13, 485 CrossRef CAS PubMed.
- S. Nagrath, L. V. Sequist, S. Maheswaran, D. W. Bell, D. Irimia, L. Ulkus, M. R. Smith, E. L. Kwak, S. Digumarthy, A. Muzikansky, P. Ryan, U. J. Balis, R. G. Tompkins, D. A. Haber and M. Toner, Nature, 2007, 450, 1235–1239 CrossRef CAS PubMed.
- S. J. Tan, L. Yobas, G. Y. Lee, C. N. Ong and C. T. Lim, Biomed. Microdevices, 2009, 11, 883–892 CrossRef PubMed.
- J. M. Ramirez, T. Fehm, M. Orsini, L. Cayrefourcq, T. Maudelonde, K. Pantel and C. Alix-Panabières, Clin. Chem., 2014, 60, 214–221 CrossRef CAS PubMed.
- R. Luo, M. Liu, Q. Yang, H. Cheng, H. Yang, M. Li, X. Bai, Y. Wang, H. Zhang, S. Wang, T. Xie and Q. Tian, J. Cancer, 2021, 12, 5035–5045 CrossRef CAS PubMed.
- E. van der Pol, F. A. W. Coumans, A. E. Grootemaat, C. Gardiner, I. L. Sargent, P. Harrison, A. Sturk, T. G. van Leeuwen and R. Nieuwland, J. Thromb. Haemostasis, 2014, 12, 1182–1192 CrossRef CAS PubMed.
- S. Gauri and M. R. Ahmad, J. Sens., 2020, 2020, 1–10 CrossRef.
- G. Park, J. K. Park, D.-S. Son, S.-H. Shin, Y. J. Kim, H.-J. Jeon, J. Lee, W.-Y. Park, K. H. Lee and D. Park, Sci. Rep., 2018, 8, 11631 CrossRef PubMed.
- X. Han, J. Wang and Y. Sun, Genomics, Proteomics Bioinf., 2017, 15, 59–72 CrossRef PubMed.
- A. Sita-Lumsden, D. A. Dart, J. Waxman and C. L. Bevan, Br. J. Cancer, 2013, 108, 1925–1930 CrossRef CAS PubMed.
- M. Yu, S. Stott, M. Toner, S. Maheswaran and D. A. Haber, J. Cell Biol., 2011, 192, 373–382 CrossRef CAS PubMed.
- M. Ignatiadis, M. Lee and S. S. Jeffrey, Clin. Cancer Res., 2015, 21, 4786–4800 CrossRef CAS PubMed.
- L. Gorgannezhad, M. Umer, M. N. Islam, N.-T. Nguyen and M. J. A. Shiddiky, Lab Chip, 2018, 18, 1174–1196 RSC.
- M. Ivanov, P. Chernenko, V. Breeder, K. Laktionov, E. Rozhavskaya, S. Musienko, A. Baranova and V. Mileyko, Front. Genet., 2019, 10, 194 CrossRef CAS PubMed.
- R. Aarthy, S. Mani, S. Velusami, S. Sundarsingh and T. Rajkumar, Mol. Diagn. Ther., 2015, 19, 339–350 CrossRef CAS PubMed.
- P. Jiang, C. W. Chan, K. A. Chan, S. H. Cheng, J. Wong, V. W.-S. Wong, G. L. Wong, S. L. Chan, T. S. Mok and H. L. Chan, Proc. Natl. Acad. Sci. U. S. A., 2015, 112, E1317–E1325 CAS.
- M. Ivanov, A. Baranova, T. Butler, P. Spellman and V. Mileyko, BMC Genomics, 2015, 16, S1 CrossRef PubMed.
- M. Lapin, S. Oltedal, K. Tjensvoll, T. Buhl, R. Smaaland, H. Garresori, M. Javle, N. I. Glenjen, B. K. Abelseth, B. Gilje and O. Nordgård, J. Transl. Med., 2018, 16, 300 CrossRef CAS PubMed.
- S. Noh, D. T. Ha, H. Yang and M.-S. Kim, Analyst, 2015, 140, 3947–3952 RSC.
- J. Das, I. Ivanov, E. H. Sargent and S. O. Kelley, J. Am. Chem. Soc., 2016, 138, 11009–11016 CrossRef CAS PubMed.
- B. K. Thakur, H. Zhang, A. Becker, I. Matei, Y. Huang, B. Costa-Silva, Y. Zheng, A. Hoshino, H. Brazier, J. Xiang, C. Williams, R. Rodriguez-Barrueco, J. M. Silva, W. Zhang, S. Hearn, O. Elemento, N. Paknejad, K. Manova-Todorova, K. Welte, J. Bromberg, H. Peinado and D. Lyden, Cell Res., 2014, 24, 766–769 CrossRef CAS PubMed.
- S. Holdenrieder, J. Von Pawel, D. Nagel and P. Stieber, Anticancer Res., 2010, 30, 1613–1615 CAS.
- S. N. Tamkovich, A. V. Cherepanova, E. V. Kolesnikova, E. Y. Rykova, D. V. Pyshnyi, V. V. Vlassov and P. P. Laktionov, Ann. N. Y. Acad. Sci., 2006, 1075, 191–196 CrossRef CAS PubMed.
- O. E. Bryzgunova, S. N. Tamkovich, A. V. Cherepanova, S. V. Yarmoshchuk, V. I. Permyakova, O. Y. Anykeeva and P. P. Laktionov, Acta Naturae, 2015, 7, 115–118 CrossRef CAS PubMed.
- S. C. Yu, S. W. Lee, P. Jiang, T. Y. Leung, K. C. Chan, R. W. Chiu and Y. M. Lo, Clin. Chem., 2013, 59, 1228–1237 CrossRef CAS PubMed.
- K. C. A. Chan, P. Jiang, C. W. M. Chan, K. Sun, J. Wong, E. P. Hui, S. L. Chan, W. C. Chan, D. S. C. Hui, S. S. M. Ng, H. L. Y. Chan, C. S. C. Wong, B. B. Y. Ma, A. T. C. Chan, P. B. S. Lai, H. Sun, R. W. K. Chiu and Y. M. D. Lo, Proc. Natl. Acad. Sci. U. S. A., 2013, 110, 18761–18768 CrossRef CAS PubMed.
- S. Salvi, G. Gurioli, U. De Giorgi, V. Conteduca, G. Tedaldi, D. Calistri and V. Casadio, OncoTargets Ther., 2016, 9, 6549–6559 CrossRef CAS PubMed.
- K. W. Witwer, E. I. Buzás, L. T. Bemis, A. Bora, C. Lässer, J. Lötvall, E. N. Nolte-'t Hoen, M. G. Piper, S. Sivaraman, J. Skog, C. Théry, M. H. Wauben and F. Hochberg, J. Extracell. Vesicles, 2013, 2, 20360 CrossRef PubMed.
- S. Yadav, K. Boriachek, M. N. Islam, R. Lobb, A. Möller, M. M. Hill, M. S. A. Hossain, N.-T. Nguyen and M. J. A. Shiddiky, ChemElectroChem, 2017, 4, 967–971 CrossRef CAS.
- X. Doldan, P. Fagundez, A. Cayota, J. Laiz and J. P. Tosar, Anal. Chem., 2016, 88, 10466–10473 CrossRef CAS PubMed.
- D. D. Taylor and S. Shah, Methods, 2015, 87, 3–10 CrossRef CAS PubMed.
- B. Mateescu, E. J. K. Kowal, B. W. M. van Balkom, S. Bartel, S. N. Bhattacharyya, E. I. Buzás, A. H. Buck, P. de Candia, F. W. N. Chow, S. Das, T. A. P. Driedonks, L. Fernández-Messina, F. Haderk, A. F. Hill, J. C. Jones, K. R. Van Keuren-Jensen, C. P. Lai, C. Lässer, I. d. Liegro, T. R. Lunavat, M. J. Lorenowicz, S. L. N. Maas, I. Mäger, M. Mittelbrunn, S. Momma, K. Mukherjee, M. Nawaz, D. M. Pegtel, M. W. Pfaffl, R. M. Schiffelers, H. Tahara, C. Théry, J. P. Tosar, M. H. M. Wauben, K. W. Witwer and E. N. M. Nolte-'t Hoen, J. Extracell. Vesicles, 2017, 6, 1286095 CrossRef PubMed.
- J. Lin, J. Li, B. Huang, J. Liu, X. Chen, X. M. Chen, Y. M. Xu, L. F. Huang and X. Z. Wang, Sci. World J., 2015, 2015, 657086 Search PubMed.
- P. Li, M. Kaslan, S. H. Lee, J. Yao and Z. Gao, Theranostics, 2017, 7, 789–804 CrossRef CAS PubMed.
- J. F. Quintana, B. L. Makepeace, S. A. Babayan, A. Ivens, K. M. Pfarr, M. Blaxter, A. Debrah, S. Wanji, H. F. Ngangyung, G. S. Bah, V. N. Tanya, D. W. Taylor, A. Hoerauf and A. H. Buck, Parasites Vectors, 2015, 8, 58 CrossRef PubMed.
- E. V. Batrakova and M. S. Kim, J. Controlled Release, 2015, 219, 396–405 CrossRef CAS PubMed.
- C. A. Maguire, L. Balaj, S. Sivaraman, M. H. Crommentuijn, M. Ericsson, L. Mincheva-Nilsson, V. Baranov, D. Gianni, B. A. Tannous, M. Sena-Esteves, X. O. Breakefield and J. Skog, Mol. Ther., 2012, 20, 960–971 CrossRef CAS PubMed.
- M. Yu, A. Bardia, B. S. Wittner, S. L. Stott, M. E. Smas, D. T. Ting, S. J. Isakoff, J. C. Ciciliano, M. N. Wells, A. M. Shah, K. F. Concannon, M. C. Donaldson, L. V. Sequist, E. Brachtel, D. Sgroi, J. Baselga, S. Ramaswamy, M. Toner, D. A. Haber and S. Maheswaran, Science, 2013, 339, 580–584 CrossRef CAS PubMed.
-
E. Lianidou and D. Hoon, in Principles and applications of molecular diagnostics, 2018, ch. 9, pp. 235–281 Search PubMed.
- P. P. Praharaj, S. K. Bhutia, S. Nagrath, R. L. Bitting and G. Deep, Biochim. Biophys. Acta, Rev. Cancer, 2018, 1869, 117–127 CrossRef CAS PubMed.
- M. Umer, R. Vaidyanathan, N. T. Nguyen and M. J. A. Shiddiky, Biotechnol. Adv., 2018, 36, 1367–1389 CrossRef CAS PubMed.
- Y. Zhu, J. Podolak, R. Zhao, A. K. Shukla, R. J. Moore, G. V. Thomas and R. T. Kelly, Anal. Chem., 2018, 90, 11756–11759 CrossRef CAS PubMed.
- N. Bhagwat, K. Dulmage, C. H. Pletcher, L. Wang, W. DeMuth, M. Sen, D. Balli, S. S. Yee, S. Sa and F. Tong, Sci. Rep., 2018, 8, 1–14 CAS.
- G. Sozzi, L. Roz, D. Conte, L. Mariani, F. Andriani, P. Verderio and U. Pastorino, J. Natl. Cancer Inst., 2005, 97, 1848–1850 CrossRef CAS PubMed.
- T. H. Lee, L. Montalvo, V. Chrebtow and M. P. Busch, Transfusion, 2001, 41, 276–282 CrossRef CAS PubMed.
- X. Xue, M. D. Teare, I. Holen, Y. M. Zhu and P. J. Woll, Clin. Chim. Acta, 2009, 404, 100–104 CrossRef CAS PubMed.
- J. Jen, L. Wu and D. Sidransky, Ann. N. Y. Acad. Sci., 2000, 906, 8–12 CrossRef CAS PubMed.
- H. Markus, T. Contente-Cuomo, M. Farooq, W. S. Liang, M. J. Borad, S. Sivakumar, S. Gollins, N. L. Tran, H. D. Dhruv, M. E. Berens, A. Bryce, A. Sekulic, A. Ribas, J. M. Trent, P. M. LoRusso and M. Murtaza, Sci. Rep., 2018, 8, 7375 CrossRef PubMed.
- F. Mauger, C. Dulary, C. Daviaud, J. F. Deleuze and J. Tost, Anal. Bioanal. Chem., 2015, 407, 6873–6878 CrossRef CAS PubMed.
- C. D. M. Campos, S. S. T. Gamage, J. M. Jackson, M. A. Witek, D. S. Park, M. C. Murphy, A. K. Godwin and S. A. Soper, Lab Chip, 2018, 18, 3459–3470 RSC.
- J. B. de Kok, J. C. M. Hendriks, W. W. van Solinge, H. L. Willems, E. J. Mensink and D. W. Swinkels, Clin. Chem., 1998, 44, 2201–2204 CrossRef CAS.
- H. Lee, M. Choi, J. Lim, M. Jo, J. Y. Han, T. M. Kim and Y. Cho, Theranostics, 2018, 8, 505–517 CrossRef CAS PubMed.
- F. Vitzthum, G. Geiger, H. Bisswanger, H. Brunner and J. Bernhagen, Anal. Biochem., 1999, 276, 59–64 CrossRef CAS PubMed.
- A. S. Devonshire, A. S. Whale, A. Gutteridge, G. Jones, S. Cowen, C. A. Foy and J. F. Huggett, Anal. Bioanal. Chem., 2014, 406, 6499–6512 CrossRef CAS PubMed.
- M. Horlitz, A. Lucas and M. Sprenger-Haussels, PLoS One, 2009, 4, e7207 CrossRef PubMed.
- T. deVos, R. Tetzner, F. Model, G. Weiss, M. Schuster, J. Distler, K. V. Steiger, R. Grützmann, C. Pilarsky, J. K. Habermann, P. R. Fleshner, B. M. Oubre, R. Day, A. Z. Sledziewski and C. Lofton-Day, Clin. Chem., 2009, 55, 1337–1346 CrossRef CAS PubMed.
- K. G. Spindler, Acta Oncol., 2017, 56, 7–16 CrossRef CAS PubMed.
- S. El Messaoudi, F. Rolet, F. Mouliere and A. R. Thierry, Clin. Chim. Acta, 2013, 424, 222–230 CrossRef CAS PubMed.
- B.-Y. Chen, C. W.-H. Sung, C. Chen, C.-M. Cheng, D. P.-C. Lin, C.-T. Huang and M.-Y. Hsu, Clin. Chim. Acta, 2019, 493, 14–19 CrossRef CAS PubMed.
- N. Ludwig, T. L. Whiteside and T. E. Reichert, Int. J. Mol. Sci., 2019, 20, 4684 CrossRef CAS PubMed.
Footnote |
† These authors contributed equally to the current work. |
|
This journal is © The Royal Society of Chemistry 2022 |
Click here to see how this site uses Cookies. View our privacy policy here.