DOI:
10.1039/D2SD00005A
(Critical Review)
Sens. Diagn., 2022,
1, 387-404
Advancements and future prospects of wearable sensing technology for healthcare applications
Received
11th January 2022
, Accepted 17th March 2022
First published on 17th March 2022
Abstract
The realm of wearable technology is continually growing. Researchers are encouraged to design additional wearable monitoring devices as wearable technology advances and the need for real-time analyte monitoring grows. Wearable monitoring systems are, in fact, being hailed as the next generation of personal portable electronics. Wearable healthcare systems will usher in a new era for illness detection, treatment, and prevention as material science, integrated circuit construction, innovation for manufacturing, fabrication of integrated circuits, and structural design advancement. Wearables are used in a variety of industries, including agriculture, sports, and healthcare navigation systems. The relevance of wearable devices in the area of customised healthcare is discussed in this paper, as well as their future conceptual development. The last portion includes conclusions that focus on crucial challenges and emerging developments in the realm of wearable healthcare devices.
1. Introduction
Wearable technology is still in its early stages of development. Anything that can be worn to conduct everyday tasks is considered wearable. Wearable gadgets, wearables or wearable technology refers to smart and portable devices, as well as systems that can communicate wirelessly, that are integrated into equipment and peripherals, or clothing that can be placed on the body, or even intrusive versions like microchips or smart tattoos.1 In other words, any computer device outfitted with the requisite sensors to process, measure or analyse one or more health indicators for the individual wearing it is considered a wearable device. Wristbands, chest bands, smart watches and other sensors based on textile are examples of wearable gadgets. The devices are connected to gather data, monitor actions, and then tailor the experiences to meet the needs of the users depending on the obtained and recorded data. Agriculture, sports, and healthcare navigation systems are just a few of the industries where wearables may be found. Sensors, network connections, data processors, cameras, and other features are included in wearable gadgets. Multiple parameters may be monitored and synced using this method. Wearables that are built for a certain use may include multi-sensor capabilities that are configurable for that application. Wearables must be designed to be comfortable to wear and lightweight while being modest in appearance and versatile. Sensing, analysing, storing, communicating, and applying are the essential functions that wearables should fulfil. Data processing might take place either at the wearer's location or at a distant site.
Wearable gadgets in the healthcare industry have huge potential for contributing to better world health. A helpful and pleasant technology may be created by integrating the concepts of biosensors and wearability. Wearable technology in the health sector refers to technological gadgets that individuals may wear, like smartwatches and Fitbits, and that seek data from their own fitness and health. According to Accenture, consumer adoption of wearables increased from 9% in 2014 to 33% in 2018.2 Consumer-wearable gadgets have come a long way since their beginnings, but they are still in their initial stages. Most individuals utilize a smartphone connected with an activity tracker, as shown in Fig. 1(a),3 restricting their experience to the heart rate and number of steps. Truly, the diversity of statistics gathered and succeeded in a wearable network may provide mankind with a hitherto unheard-of user experience.4 Aside from traditional eyewear, smartwatches, sports trackers, heart rate metres and on-body cameras, advanced high-tech wearables will include mixed, virtual, augmented and enhanced-reality gadgets, as well as different smart garments and commercial wearable computing. The wearable industry is expected to continue to develop enormously in the future years from a financial standpoint. The market is anticipated to grow at a frequency of more than 20% every year, reaching approximately 40 billion EUR every year in the following 5 years and increasing to 150 billion EUR by 2028.5 Wearable shipments, which were anticipated to be 113.2 million in 2017 with a total market size of $70 billion in 2019, are expected to reach 222.3 million annually by 2021.6 Furthermore, the COVID-19 epidemic had a significant influence on the development of wearable devices, which was fuelled by the adoption of different crowd-sensing and contact-tracing platforms.7 Wearables are predicted to proliferate gradually over the next several decades, with a major shift away from wristbands and sport trackers toward smarter, more feature-rich wearables. Furthermore, the worldwide enterprise wearable market alone was worth over EUR 18 billion in 2017, and it is expected to increase at a CAGR of 11.8% during the projected period of 2019 to 2026.8Fig. 1(b)3 depicts the particularities of market movements as well as the prediction for the future. Wearable technologies might be utilised to boost employee physical activity, allow for more effective relaxing, and improve workplace safety and productivity.9 We have compiled a list of wearable healthcare systems that have been created in recent years, taking into account all of the factors.
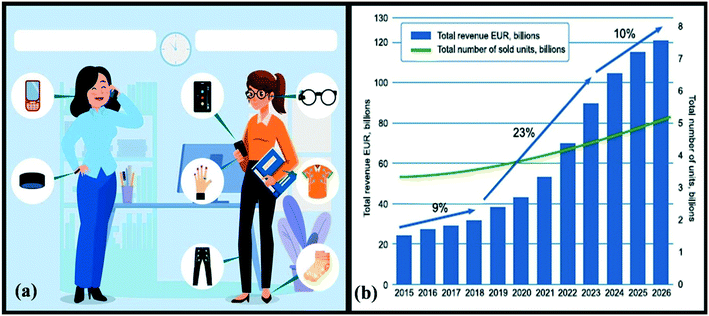 |
| Fig. 1 (a) A look at the future of personal wearable ecosystems; (b) projection of the wearables market. Reproduced from ref. 3 under creative commons attribution license, copyright @ Elsevier (2021). | |
2. Wearable healthcare devices and their historical perspective
Wearable devices are described as tools that may be attached to the human body or clothes and can be used for a variety of purposes.10 They are made up of a transducer and a target receptor. The target analyte is recognised by a receptor, which then reacts to it.11 When the transducer receives a signal from the receptor, it converts it into something usable.12Fig. 2 shows a simple schematic of a typical sensor. Wearable technologies have demonstrated promising outcomes in the healthcare industry owing to their deformability and compliance, according to a number of research investigations. It is hoped that these healthcare wearable devices will assist in preventing and curing illnesses by providing better knowledge of how the human body develops over time. People with limited access to physicians might benefit from wearable sensors throughout their recuperation time as well. Hospitalization is costly, and wearable sensors may allow continuous monitoring of health indicators without frequent hospital visits. However, real-time monitoring of parameters in each wearable sensor has limits. Therefore, a variety of sensors are required to monitor a wide range of health metrics. In order to produce this sensor family for health care, pathogen detection, and clinical reasons, many fabrication processes have been developed throughout the years.13–16 Wearable technology has grown in three phases, according to early studies: a first phase from the 1980s to 1997; a second phase between 1998 and 2000; and a third phase between 2001 and 2004.17 It is described as follows: the first period is driven by technology, with an emphasis on wearable computing applications; the second is a period of integration with the fashion and textiles sector, with more extensive garment integration; and the third is a period of growth in smart clothing and garment integration in the commercial sector. Recent fashion trends, on the other hand, have seen a shift in focus away from garment-based forms and toward accessory and jewellery forms. In combination with a fast reduction in size and power consumption of the supporting technology, smaller and more compact wearables have become more widespread in recent years. Smaller components have made it feasible to create smaller, accessory-type form factors that were before hard or impossible to achieve. This is shown by the observed growth in the popularity of jewellery-type wearables.18 One possible explanation for the developments between 2004 and 2016 is provided by a 2010 analysis,19 which identifies obstacles to garment-integrated development of wearable technologies that include manufacturing difficulties, application development difficulties, and cultural differences between sub-fields contributing to wearable technologies. The field of wearable devices has grown at an exponential rate over the last decade, owing to its ability to offer continuous and real-time physiological data via continual and non-invasive measurements of multiple biochemical markers in the most diverse body biofluids.20 A large number of novel tools have been introduced in a diverse range of applications.21–24 The early wearable gadgets gained popularity primarily in the field of physical activity monitoring. This information was gathered via the use of physical sensors, which were primarily used to monitor movement (step counter/calorie burning) and vital signs.21 Recently, technologies have been developed that are mainly focused on real-time insulin monitoring, resulting in less invasive procedures for diabetic patients. They may reduce the discomfort and danger associated with blood collection processes, enabling these devices to be more easily accepted, improved, and integrated into the everyday lives of their users.25 The confluence of technologies connected with the present development of nanotechnology has fuelled additional progress and encouraged design innovation as well as the advancement of healthcare wearable devices, among other things. Analytes for pathogen monitoring, testing, and assessment are provided in a cost-effective, more basic, disposable format for use in a variety of clinical situations. Such advancements have proven the great potential that wearable devices have for real-world applications.26 When it comes to wearable body sensors, early attempts focused on tracking physical signals by detecting changes in a physical stimulus and then converting that stimulus into a quantifiable and recordable signal. Thermal, pressure, obstacle, and strain sensors are among the types of wearable physical sensors that have gained a lot of interest recently, particularly in the sports industry. Body temperature, heart rate, and blood pressure are the three vital signs in physical sensing that are most commonly monitored in individual healthcare checkups. These three vital signs extract significant physiological information from the individual and are used to reflect the individual's health status on a daily basis.27,28
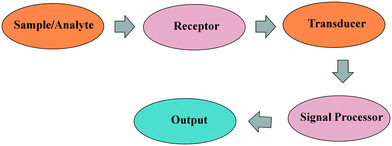 |
| Fig. 2 Schematic of a typical sensor. | |
Following a shift in research priorities in recent years, researchers have turned their attention away from traditional physical signals toward addressing critical obstacles in biomedicine and healthcare applications, such as early cancer detection and long-awaited diabetes therapy.29 When it comes to in situ sensing, wearable chemical and biological sensors have been intensively investigated as noninvasive alternatives to traditional in situ sensors.30 Sensors for chemical potentials and gases are available,31 as are sensors for humidity and ions.32 Wearable chemical sensors are primarily composed of wearable ion sensors, which are capable of detecting and monitoring a wide range of ions in a wide range of biological fluids.33 Wearable chemical sensors offer a portable and reliable detection system for continuous on-body analyses, ranging from simple device designs to integrated array systems. In essence, biosensors are chemical sensors, but the recognition system uses a biochemical process instead of a chemical mechanism. As a result, biosensors are composed of two fundamental components: a molecular recognition element and a physico-chemical transducer. Despite numerous preliminary medical screening tests in wearable sensing platforms that have been conducted in recent years, researchers are still far from achieving a comprehensive understanding of how this human–computer interaction technology can best contribute to biomedicine and the human healthcare enterprise in the future. There are a range of problems, such as the demand for high reliability, precision, and security in consumer wearables, as well as a shortage of technologies that use little power, that have slowed the advancement of commercial manufacture to date. Large-scale validation tests, mandatory equipment regulatory clearances, and a comprehensive marketing channel plan are all required to deal with this situation effectively and efficiently. Because of the complexity and variety of human physiology, many different types of sensors have been explored and produced extensively throughout the years.
2.1. Types of wearable devices
Wearable devices are broadly classified as skin-based wearable devices and biofluidic-based wearable devices.
Skin-based wearable devices.
Due to the fact that skin covers the majority of the human body, it is an ideal modality for noninvasive healthcare wearable devices. In addition to providing physiological and psychological monitoring, skin-based wearable sensors may be used to monitor the progression of various illnesses, such as cardiovascular and neuromuscular disorders. Additional applications include the detection of various disorders by the qualitative and quantitative study of skin secretions such as perspiration, among other things. Skin-based wearable devices may be classified as either textile-based or epidermal-based, depending on the sort of skin contact they have. Textile-based wearable devices include the incorporation of critical sensors into clothing, while epidermal-based wearable devices entail the direct attachment of wearables to the skin, similar to a tattoo, and are collectively referred to as electronic skin (e-skin).34
Biofluidic-based wearable devices.
These devices utilize bodily secretions such as sweat, saliva, tears, and urine that include valuable biomarkers that are used for both monitoring and diagnostic reasons. Healthcare wearable devices may be employed directly or in conjunction with other platforms. For example, microfluidic systems can be combined to extract meaningful information from a variety of biofluids.35
On the basis of principle of their action, wearable sensors are classified as mechanical sensors, electrical sensors, optical sensors and chemical sensors.36
Wearable mechanical sensors.
A wearable mechanical sensor converts mechanical stimuli into electrical impulses, which may then be read. Mechanical sensors include piezoresistive, piezoelectric, capacitive, and iontronic sensors. Whenever conductive materials are exposed to mechanical deformation, the electrical characteristics of the materials are altered. The piezoresistive effect is the name given to this kind of electromechanical reaction. Capacitive sensors detect changes in an electrical property known as capacitance, and they operate by sensing these changes. Similarly, iontronic sensors respond to changes in pressure. The detecting mechanism of a piezoelectrical sensor is based on the piezoelectric effect of the materials, which causes electrical charges to be generated when they are subjected to external mechanical force, pressure, and strain. In the presence of mechanical stress, a change in electrical polarisation occurs inside the piezoelectric material. The change in polarisation causes a change in the surface charge (voltage) at the surface of the piezoelectric material as a consequence of the change in polarisation.37
Wearable electrical sensors.
These monitor changes in the electrical resistance of the skin or changes in capacitive or conductively linked charge at the skin surface using electrical resistance sensors or capacitive sensors. The majority of the time, high-input impedance circuits are utilised to detect these very minute changes in electrical charge.38
Wearable optical sensors.
Wearable optical sensors provide an optical signal in response to changes in the environment that are caused by biological, chemical, or physical changes.39 There are several types of optical sensor elements that are typically used to measure chemical or biological changes in a given environment. Colorimetric, plasmonic, and fluorometric sensing elements are examples of these types of optical sensor elements. Colorimetric-based sensing devices employ change in colour simply, when exposed to a specific analyte of interest, which may be achieved by a biological or chemical reaction.40 In most cases, an absorbance measurement is employed to detect such a colour shift. The sensing element is lighted, and the reflected or transmitted light is recorded and utilised to compute the sensor response.41,42 Plasmonic sensing makes use of metal-dielectric interfaces or nanostructures that display an optical resonance at a certain wavelength that is dependent on the geometry of the interface or nanostructure. When a plasmonic sensor comes into contact with a certain biological or chemical target, the wavelength at which this resonance occurs may also vary as a result.43 Fluorometric sensing, on the other hand, is differentiated by the use of fluorescent materials, such as organic dyes, fluorescent proteins, or quantum dots, to detect changes in the environment. In order for the fluorescent molecules to produce light at a certain emission wavelength via radiative electron transitions, these sensing components need an excitation light source to excite them to the appropriate level of excitation. Most of the time, the intensity of a fluorescent signal, or the change in the strength of a fluorescent signal over time, is connected to the concentration of an analyte under investigation. Because the excitation and emission wavelengths are separated in the optical spectrum, fluorometric sensing elements frequently require optical filters to reduce the background created by the excitation light in order to adequately read the significantly weaker emission signal.44
Wearable chemical sensors.
Chemical or biological sensors are typically composed of two elements: a recognition element (receptor) and a transduction element (conductor).45 If a wearable chemical sensor can be effectively connected with bio-fluids, it is possible to achieve signal transduction from chemical to electrical or chemical to optical signals. Colorimetric signal detection is often utilised in chemical-to-optical signal conversion, which is comparable to the method employed in urine-based pregnancy testing. Chemical-to-optical sensing has the potential to provide two major advantages:46 (1) ultra-low cost and high simplicity by eliminating the need for localised electronics, detectors, and other components; and (2) the ability to leverage some parts of the very large library of colorimetric or fluorometric assays used in conventional benchtop biofluid analyses. Chemical-to-electrical or electrochemical sensors are common in wearable chemical sensors due to the following reasons: (1) these sensors require no action from the user to observe or record the data; (2) in some cases these sensors can minimise the required technology (no light sources, optics, or detectors are required); many of these sensors are reagent and label-free so that they start working as soon as they are brought into contact with the biofluid.47 A subclass of chemical sensors that use an electrode as a transducer are electrochemical sensors. Analyte concentration is related to electrical values such as potential of current, which are measured by sensors that depend on the chemical–electric interaction. When compared to other forms of chemical tests, electrochemical reactions take place at the electrode/solution interface rather than in the bulk solution.
2.2. Advancement in wearable healthcare devices
Stretchability, ultra-thinness, biocompatibility, biodegradability, and self-healing are just a few of the desired properties of new wearable healthcare device design ideas. Wearable gadgets have transformed the healthcare system by reducing hospital workload and giving more accurate and timely data.48 Various kinds of wearable devices have been used for this purpose, including textile, flexible and epidermal-based wearables.49,50 Wrist, head, and eye-based wearables are examples of wearables that may be used for various body parts.51 These wearables track a variety of psychological and physiological variables that may be utilised to identify a variety of illnesses.52 Wearable devices, in fact, may be combined with various sample platforms to detect various chemical parameters in human fluids such as saliva, blood, urine, perspiration, and so on.50,53 Furthermore, in comparison to conventional medication delivery systems, these healthcare wearable devices may be employed to dispense pharmaceuticals in a more regulated and efficient way.54,55 Wearable technology that collects data on everyday activities, athletic performance, and health condition is propelled by the “quantified self” trend.56 Wearable gadgets, when used in conjunction with value-based healthcare systems and telemedicine, may help monitor high-risk patients, intervene early in illnesses, and save healthcare costs by predicting and preventing disease.57 Electronic/optical tattoos, hearing aids, wristbands, smartwatches, head mounted displays, electronic footwear, subcutaneous sensors, and electronic fabrics are examples of wearable technology (Fig. 3a). They may be smoothly put on the epidermis, introduced via skin or body orifices for biochemical or electrophysiological signal measurement, and medication delivery. Data may be collected from several wearable sensors and sent to a body area network using multiple sensor arrays.58
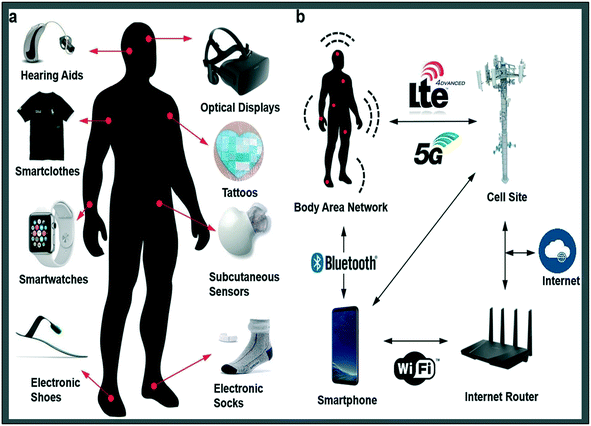 |
| Fig. 3 Medical wearables devices: a) wearable electronics come into contact with the skin in a conformal or loose manner, or are worn/inserted via bodily orifices. Interfacing devices that measure electrophysiological signals through optics and electrodes are the most prevalent interface. b) Data exchange from wearables. Body area networks (BAN), Bluetooth, WiFi, LTE, 3G, 4G, and 5G connections may be used to send data from wearable devices to the internet. It may be delivered to a healthcare professional for therapeutic feedback or acted on by other network devices. Reproduced from ref. 59 under creative commons attribution license, copyright @ WILEY-VCH Verlag GmbH & Co. KGaA, Weinheim (2018). | |
Fig. 3(b)
59 shows how this body area network utilizes 5G, 4G, 3G, Wi-Fi LTE or Bluetooth to transmit data (medical) to the Internet for additional analysis or input from a healthcare professional. Many healthcare wearable sensors based on various detection mechanisms have been created to gain early detection of problems during the pre-symptomatic period, including sensors like, colorimetric,41 electrochemical,47 optical,40 pressure/strain37 and many more. In this paper, we will concentrate on wearable electrochemical and colorimetric sensors on account of their advantages. The characteristics of electrochemical sensors, such as their ease of use, speed, portability, and intrinsic miniaturisation, make them ideal for the design and fabrication of flexible and wearable sensors.60 Colorimetric sensing has been used for both quantitative and qualitative analysis for wearable optical sensors. Colorimetric sensing is the process of inducing a colour change in a material that is visible to the naked eye and that can be correlated to the presence of a specific analyte in the material.61 This may be used for simple warning threshold signs that are only visible to the human eye, such as a change in colour, or it can be utilised for quantitative measurements of analyte concentrations that are measured using spectrophotometry. A significant benefit of colorimetric sensing is its capacity to detect without the usage of electrical connections, allowing for more design freedom as well as more convenient personal and portable use.61 The mechanism of wearable devices depends on the transfer of electrons either from the electrode to the electrolyte and vice versa.
2.3. Wearable electrochemical sensors
When an analyte is present, an electrode may be utilised as a transducer element. Electrochemical sensors fall under this category. For a wide range of factors in our daily lives, modern electrochemical sensors exploit a variety of features.62 Ease of fabrication, high sensitivity and rapid reaction are all features that may be found in electrochemical sensors.63 In the construction of wearable sensors, electrodes play a crucial role, especially via the electrochemical approach.64 Important problems still need to be addressed to enhance wearable biochemical sensor performance, such as the utilisation of functional materials and manufacturing technology for sensing electrodes.65 Wearable electrochemical sensors can detect target analytes in a variety of bodily fluids, including tears, saliva, perspiration, and interstitial fluid of the skin. The development of wearable chemical sensors that can easily monitor these biofluids has lately received significant attention from researchers. Human sweat offers a wealth of information about a person's health state, making it an ideal biofluid for non-invasive chemo-sensing applications. A variety of biomarkers, including metabolites (such as glucose, lactate, and urea, among others), proteins, nucleotides, and electrolytes (such as chlorine, sodium, and potassium, among others), are found in sweat and have significant diagnostic implications.66 A large number of glands are spread throughout the skin, making sweat a conveniently accessible source for chemical sensing. Consequently, sweat may be utilised to extract many chemical and biological characteristics utilising wearable electrochemical devices to offer monitoring and diagnostics in healthcare applications. Li et al.67 developed a highly integrated sensing paper (HIS paper) using a simple printing process, with MXene/methylene blue (Ti3C2Tx/MB) as the active materials and foldable all-paper substrates as sweat analysis patches. The HIS paper incorporates a signal processing system that can detect glucose and lactate in real time for sweat analysis (Fig. 4a–e). On the paper substrate, various effective parts of the HIS paper were printed before being folded into a three-dimensional (3D) structure. In the paper substrate, sweat may effectively move towards the perpendicular direction guided by capillary-driven force via vertically expanding the amount of hydrophilic region layer by layer. The three-independent electrode's 3D location improves enzyme decorating and fixation, as well as electrolyte accessibility. With a limit of detection (LOD) of 17.05 μM (S/N = 3), glucose revealed linear relationships in the range of 0.08–1.25 mM. The LOD is 3.73 μM and the sensor reacted linearly in the range of 0.3–20.3 mM to lactate concentrations. The sensor is a low-cost, versatile solution for biochemical systems, including wearable bioelectronics. Furthermore, the addition of methylene blue (MB) to Ti3C2Tx accelerates charge migration and increases performance electrochemically during sweat scrutiny due to the synergic impact. Low cost, compactness, and simplicity are all benefits of the all-paper sweat sensor. The unique 3D structural design facilitates perspiration absorption from the skin surface, preventing fluid collection and consequently minimising sweat-induced discomfort at the human–machine interface. Using PEDOT:PSS (poly(3,4-ethylenedioxythiophene):poly (styrene sulfonate)) hydrogels, Xu et al.68 successfully created a sensor (electrochemical) that relies on flexible microfluidics and has the capability to sense uric acid (UA) (Fig. 4(f and g)). On scanning throughout the concentration range of 2.0–250 μM, the sensor displayed an ultrahigh sensitivity of 0.875 μA μM−1 cm−2 and a low LOD of 1.2 μM (S/N = 3). It was tested on human sweat before and after a high-purine meal was consumed. The sensor's long-term UA monitoring of a genuine sweat sample was also tested, and it showed significant promise for use as an on-body wearable platform.
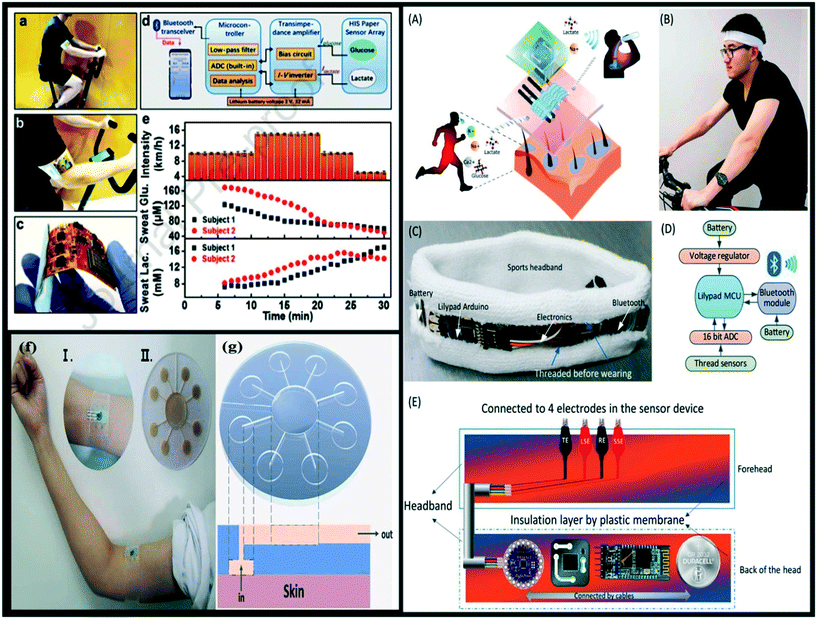 |
| Fig. 4 (a) Sweat analysis using the HIS paper-based device installed on the arm during physical exercise, as photographed. (b) Wireless detection procedure. (c) A close-up shot of a flexible circuit board. (d) A visual representation of the analytical system. (e) The sweat glucose (Glu.) and sweat lactate (Lac.) concentration curves of participants (reproduced with permission from ref. 67, copyright @ Elsevier (2021)); (f) the on-body device setup applied to the subject's upper arm. (g) Cross-sectional and top-down pictures of the microfluidic device for sweat extraction and skin operation. (Reproduced with permission from,68 copyright @ Elsevier (2021)); the wearable biosensor and its ‘smart’ headband for signal reading were designed. (A) Schematic of the wearable biosensor for sweat detection on the body during physical activities. (B) A user with a headband on. Photo of the signal readout headband (C). (D) The signal readout circuit's schematic design. (E) A cross-section of the headband. TE: trigger electrode (control electrode); LSE: lactate sensing electrode; SSE: sodium sensing electrode; RE: reference electrode. (Reproduced with permission from ref. 69, copyright @ Elsevier (2021)). | |
Zhao et al.69 proposed an electrochemical biosensor (wearable) based on sensing electrodes that are decorated with ZnO-NWs for on-body, simultaneous sodium ion and lactate detection in sweat during sweating (Fig. 4A–E). The biosensor, as well as its signal reading and transmission circuitry, was entirely incorporated into a sweat headband that can transmit data wirelessly to a smartphone. Low-temperature hydrothermal growth was used to directly synthesize ZnO NWs on thread carbon electrodes, and specialised sensing membranes were attached to the modified electrodes for sodium ion and lactate detection.
In order to attach with the skin, two thread-based electrochemical cells were fabricated with the ZnO-NW electrodes, which created output signals in electrical forms when sweat was carried to the sensor during user sweating. The detection of sodium ions and lactate in human sweat was performed simultaneously using this technology, with linear ranges of 0.1–100 mM and 0–25 mM, respectively, and LODs of 0.16 mM and 3.61 mM. These values encompass the sodium and lactate concentrations in human sweat that are clinically important. During vigorous activity, real-time on-body sweat collection and analysis were done on a volunteer, verifying the biosensor's accuracy and stability. This wearable, low-cost biosensing technology might be useful for monitoring human users' physiological conditions during exercise.
Non-invasive monitoring of essential physiological markers may be achieved by analysing tears for the concentrations of certain metabolites. In the past, wearable sensors based on tear biofluid have been described for glucose and lactate, two of the most common analytes.70 Electrochemical sensors have been incorporated into contact lens-based platforms with integrated electronic components enabling direct measurements of basal tears in these systems.71 The integration of suitable power sources and wireless data transmission, as well as sophisticated sensor designs, have been ongoing efforts to improve such tear detection devices. However, the successful functioning of fully integrated wireless tear-based wearable chemical sensor devices still faces substantial obstacles. Wearable sensing devices have huge potential in the field of alcohol since it is the most extensively used drug of abuse in the world, resulting in hundreds of billions of dollars in related expenditures in the United States each year.72 There has been a significant amount of study on the continuous real-time monitoring of a person's degree of intoxication as a result,73 and described technologies have drawbacks, such as poor specificity and time delay following alcohol ingestion. We have seen reports of wearable alcohol biosensors that can detect alcohol in sweat and interstitial fluid with improved specificity and near real-time monitoring.74,75 Despite these developments, there is still a strong need for new and better non-invasive alcohol monitoring systems. In the 1980s, Giles et al. first documented the presence of alcohol in tears.76
Sempionatto et al.77 demonstrated a positive response to the eyewear platform for measuring tear vitamins, alcohol and glucose, with no obvious carry-over effects across repeated assessments. They demonstrated that blood glucose and alcohol levels may be accurately measured using tear concentrations. They created a bioelectronic platform of wearable tear-based sensor utilizing a microfluidic detector (electrochemical) integrated into an eyewear nose-bridge pad (Fig. 5(i)A). The response of the tear alcohol-based biosensor was compared in sober state and after an alcoholic drink, with blood alcohol readings using a commercial breathalyser serving as validation (Fig. 5(i)B). Healthcare wearable devices are a handy and pleasant tool for testing blood sugar levels, and tears may be used to measure glucose levels instead of blood and urine. Several alternative techniques for measuring glucose levels in tears have been developed in the past. Tear glucose detection was done in the same way as tear alcohol detection. Immobilized GOx (glucose oxidase) was used to biocatalyze the transformation of glucose into hydrogen peroxide and gluconic acid (Fig. 5(i)C-a). In contrast, SWV was used to detect vitamin in tears, with the synthesized sensor used for signal coupling, and the proportional behaviour of oxidation peak current with the vitamin content (Fig. 5(i)C). Fig. 5(i)D depicts the creation of a fluidic gadget.
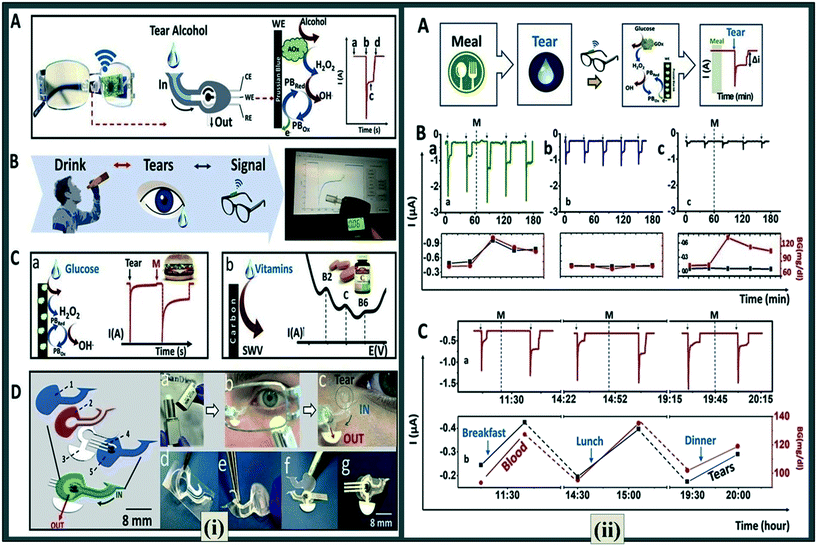 |
| Fig. 5 (i) A fluidic device for eyeglasses. (A) Schematic showing fluidic device's integration with eyewear platform, where (a) represents the baseline, (b) the current change owing to the captured tear, (c) the detected alcohol signal, and (d) the drying of the device. (B) Alcohol detection. (C) Glucose detection represented in inset a and vitamin detection representation in inset b. (D) Exploded view of fluidic device with (1) representing top polycarbonate membrane, (2) double adhesive spacer, (3) paper outlet, (4) electrochemical (bio)sensor, and (5) bottom polycarbonate membrane. Inset in D depicts stimulated tears: (a) menthol tear stick; (b) tear stick applied by a volunteer; (c) a tear enters the device's inlet; (d) adhesive spacer is removed from the PET substrate; (e) spacer placed on the bottom membrane; (f) electrode and outlet are placed on top of the spacer followed by top membrane; (g) the final device for the nose bridge pad of eyeglasses. (ii) Evaluation of a tear glucose biosensor on the body shown by (A). The technique for on-body glucose sensing tests shown by amperograms demonstrating non-invasive glucose testing in tears. (B) Graphs represent: a) a GOx sensor with a meal; b) GOx sensor without meal; c) Prussian blue sensor without enzyme after meal. In the bottom graphs of part (ii) B, the tear signal and the blood glucose level are correlated where black line shows current intensity received with the glasses wearing sensor and red line shows blood glucose levels collected with a glucometer. (C) Continuous glucose monitoring throughout the day after breakfast, lunch, and dinner. Reproduced with permission from ref. 77, copyright @ Elsevier (2019). | |
Fig. 5(ii) demonstrates amperograms taken after and before each meal, as well as on-body tracking of fluctuating tear glucose concentration. (Fig. 5B-a and C). Legitimate tear selection and precise alcohol assessments in triggered tears were facilitated by the alcohol-oxidase (AOx) biological recognition fluidic apparatus, resulting in the world's first wearable tear alcohol monitoring platform. The notion of tear alcohol sensing was proven in human subjects for monitoring alcohol consumption across many drinking courses, with excellent connections to parallel BAC (blood alcohol concentration) measures. It was the first time that someone showed the capacity to keep an eye on tear glucose peripheral of the eye, as well as the efficacy of wearables for vitamin supplement assessment in association with an enzyme circulation device and quick electroanalytical scanning. Such breakthroughs pave the foundation for the expansion of an operational eyewear mechanism that can really detect biochemical tears. Also, the totally wearable tear detecting framework was located outside the eye area, overcoming the constraint of early available sensor systems that were put in direct contact with the eye.
Wearable electronics has sparked significant interest in developing wearable and mobile sensor systems.78 While early efforts focused on physical sensors and wearable mobility, contemporary efforts have switched to the creation of mechanisms that can monitor (bio) chemical indicators non-invasively.79 Wearable sensors have therefore been created to be easily integrated into a subject's cloths, offering helpful insights about the subject's fitness and health status, as well as the wearer's environment.80 A great deal of interest has been generated in non-invasive portable synthetic detecting systems that operate in readily sampled biofluids such as tears, saliva or sweat. These platforms have the commitment to providing relevant insightful information regarding changes in biomarker's amount without the need for blood sampling. A great deal of interest has been generated in non-invasive portable synthetic detecting systems that operate in readily sampled biofluids such as tears, saliva or sweat. These platforms have the ability to generate useful real-time insights into changes in biomarker concentrations without the need for blood sampling. Consequently, biodegradable and straightforward to assemble wearable electrochemical sensors have been created as a consequence of the growing need for low-cost, scalable, simple and electrochemical sensors.
2.4. Wearable colorimetric sensors
Numerous wearable gadgets have colorimetric sensors integrated into them. Color changes may be observed with the naked eye,81,82 resulting in a device that is simple, cost-effective, and has great selectivity without the need for any extra equipment. Despite this, there are limits to this kind of sensing in terms of resolution (spatial) and sensitivity. Because the human eye is incapable of distinguishing the tiny colour changes induced by low concentrations, it's challenging to do quantitative analysis with them. Sensors combined with smart gadgets and high-resolution cameras, on the other hand, have the potential to fix this issue. It is possible to transform images of colour changes collected by a camera into RGB signals (red, green, and blue), which are then translated to various amounts, resulting in highly accurate examination.83
Zhou and et al.84 created a luminous wearable sweat tape (LWST) biosensor by inserting multi-component nanoprobes into microwell-patterned paper substrates of hollowed-out double-side tapes and then exposing the resulting sensor to light. The researchers employed enzyme-embedded AuNCs (gold nanoclusters) wrapped in nanosheets (NSs) of MnO2 as the probe for detecting UA, alcohol and glucose in the blood samples they collected. For alcohol, UA and glucose, the luminous detection signals may be identified by their coloration: green, red, and yellow, respectively. The LWST biosensors are unable to detect changes in the concentration of interesting substances in sweat, but more research will be carried out in the future to accomplish this aim. Furthermore, the authors used an app in a smartphone to record and digitally transform colorimetric photos as RGB (red, green, and blue) signals in order to improve the visual evaluation. For urine, glucose, and alcohol detection, researchers developed LWST biosensors that are wrapped in MnO2 NSs and enzyme-embedded AuNC assembles, which are used as the probe. Using coloured AuNCs and the matching enzymes, the mechanism of the integrated probe may be easily tuned to produce other emissive colours and different targeting analytes than those used in the original probe. Because of their permeable architectures and poor bonding within the framework, like van der Waals force, the enzyme-based nanogel framework may enhance related sweat analytes (Fig. 6(A–I)) The schematic design of a sweat sensor based on enzyme/AuNCs@PAH@MnO2 NSs nanoprobes is shown in Fig. 6J. This wearable gadget was made up of two identical double-sided tapes that were held together by the adhesive surface. Physical punching on one of the double-sided tapes produced the microwells. The functionalized filter sheets were strongly adhered in the microwells and acted as separate reaction chambers to detect target analytes, thanks to the exposed microwells' particular stickiness. Sharma et al.85 presented a non-invasive sensor (colorimetric) for distinguishing ketones. On a prepared cotton fabric, they alternately applied sodium nitroprusside (SNP) and poly(2-(dimethylamino ethyl) methacrylate) (pDMAEMA). The presence of the first layer (pDMAEMA) cationized the cotton surface, increasing the affinity for the second layer's absorption (SNP).
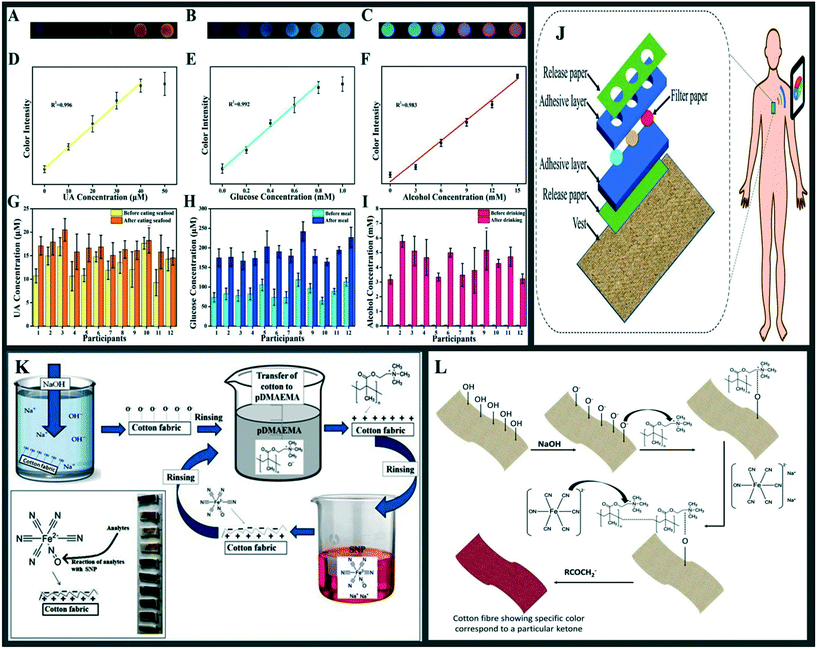 |
| Fig. 6 A) Under UV light, the colorimetric reactions of uricase/hGG-AuNCs@PAH@MnO2 NSs to UA at varied concentrations ranging from 0 to 50 M. B) Under UV light, the colorimetric responses of GOx/HG-AuNCs@PAH@MnO2 NSs to glucose concentrations ranging from 0 to 1 mM. C) Under UV light, the colorimetric responses of ADH/aGG-AuNCs@PAH@MnO2 NSs to alcohol concentrations ranging from 0 to 15 mM. D) Color intensity vs. UA concentration in the range of 0 to 50 M. E) Color intensity vs. glucose concentration in the range of 0 to 1 mM. F) Color intensity as a function of alcohol content, ranging from 0 to 15 mM. The designed LWST biosensor was used in human studies to detect G) UA, H) glucose, and I) alcohol in situ. (J) A diagram depicting the production of LWST biosensors and the smartphone device's sensing procedure. (Reproduced with permission from ref. 84, copyright @ Elsevier (2021)); (K) schematic diagram of layer-by-layer assembly on cotton fabric; the inset illustrates the colours achieved for various ketones on treated cotton fabric. (L) A schematic depiction of the colour sensing device used to identify various ketones. Reproduced from ref. 85 under creative commons attribution license, copyright @ Elsevier (2021). | |
GSH (glutathione); ADH (alcohol dehydrogenase); PAH (poly (allylamine hydrochloride)).
The colour of the treated cotton fabric is due to the integration of SNP ions on the cotton surface. The constructed sensor allows for immediate, inexpensive, repeatable, and convincing detection of specific analytes. Fig. 6K depicts the schematic design for manufacturing the sensor, whereas Fig. 6L depicts the probable sensing mechanism. The proposed sensor's precise sensing of ketones within a narrow range gives insights into the health state and helps to avoid numerous chronic illnesses. The fabricated sensor is an inexpensive, biodegradable, and portable device that does not need regular calibration. Cotton fabric-based colorimetric techniques have various benefits, including ease of fabrication, low cost, and the ability to examine and understand the signal with the naked eye. It also allows for continuous and real-time monitoring of the analyte to be detected. The produced sensor does not take up a lot of space, and its disposal does not endanger the environment.
The detection of dangerous H2S gas for environmental monitoring is becoming more important in both business and academics due to the fast growth in healthcare concerns. Colorimetric probes have gotten a lot of interest because of their benefits such quick sensing times, easy sensing with the naked eye, and inexpensive measurements. The majority of colorimetric examinations designed for H2S detection, however, work in the liquid form and have a lengthy reaction time on the scale of a few minutes, limiting practical everyday use.86 As a result, colorimetric H2S detection chemosensors with quick detection, appropriate selectivity, and usage under a variety of situations are needed. Zhang et al.87 developed a new colorimetric probe 1,4-phenylenebis(azaneylylidene) bis(6-bromoindolin-2-one) (C8-ISPBI) based on a Schiff base with a straightforward synthesis approach for effective naked-eye detection of H2S. Due to substantial UV–vis absorption spectrum shifts, C8-ISPBI changed colour quickly and dramatically in reaction to H2S. The sensing mechanism was discovered to be an efficient addition reaction (nucleophilic) after experimental and theoretical computations. C8-ISPBI has a fast reaction to H2S (less than 15 seconds), a low LOD (1.2 μM), and outstanding selectivity against competing species of the analyte. C8-ISPBI also demonstrated exceptional colorimetric sensing performance in a variety of states, including films, solutions and coloured fabrics. These advantages point to C8-ISPBI's usefulness as an H2S sensor in a variety of situations. Promphet et al.88 created a wearable colorimetric sensor for non-invasive and simultaneous sensing of urea and glucose in human perspiration. Fig. 7A depicts the sensor production process. Fig. 7B depicts the response process that causes the colour change. As illustrated in Fig. 7C, the sensor was used to sense urea and glucose in the fake sweat at the same time. Thread has emerged as a viable non-invasive wearable sensor substrate. The cotton thread surfaces were modified using cellulose nanofiber/chitosan-graphene oxide to improve enzymatic immobilisation efficiency and sensor performance. For glucose, the sensing platform has a linear range of 0.1–3 mM with an LOD of 0.1 mM, and for urea, the sensing platform has a linear range of 30–180 mM with an LOD of 30 mM.
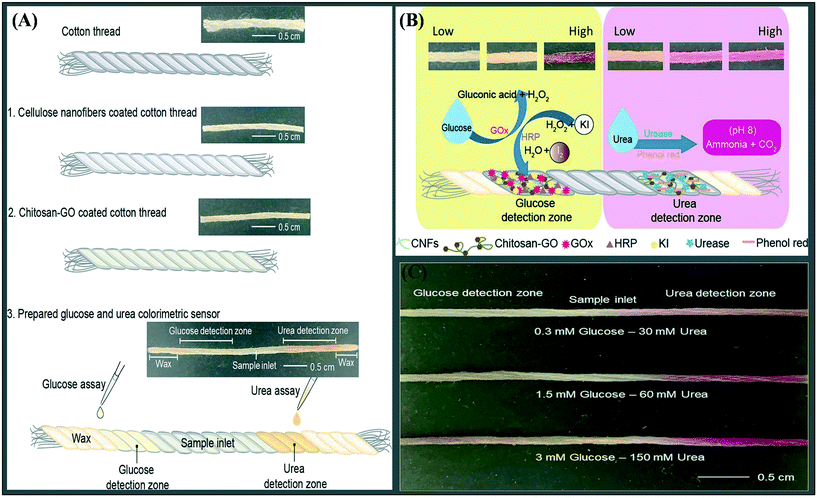 |
| Fig. 7 (A) Development of colorimetric sensors based on cotton thread, (B) schematic of the sensing mechanism and (C) simultaneous detection of urea and glucose. Reproduced with permission from ref. 88, copyright @ Elsevier (2021). | |
The disclosed colorimetric sensor can discriminate between normal and abnormal persons by determining the cut-off values for both urea (65 mM) and glucose (0.3 mM) in human sweat. This sensor might be easily incorporated with clothing or directly fixed with the skin of humans for monitoring of diabetes and renal failure from the subject's perspiration due to the thread's flexibility. Table 1 summarises further information on wearable electrochemical and colorimetric sensors.
Table 1 Wearable electrochemical sensors
Analyte |
Electrode |
Platform |
Technique |
Linear range |
Matrix |
Ref. |
SWV = square wave voltammetry; AgNW = nanocomposite of Ag wires; OPH = organophosphorus hydrolase enzyme; PDMS = poly(dimethylsiloxane); PANi = polyaniline; LOx = lactate oxidase enzyme; MWCNT = multiwalled carbon nanotubes; CNTs = carbon nanotubes; DPV = differential pulse voltammetry; GOx = glucose oxidase enzyme. |
Abuse drug (fentanyl) |
MWCNT-PEI-IL/carbon printed electrode |
Glove |
SWV |
10–100 μM |
Liquid and powder forms |
89
|
Tetrahydrocannabinol (THC) |
MWCNT/carbon printed electrode |
Ring |
Amperometry |
1–6 μM THC |
Saliva |
90
|
Ethanol |
AOx/PPy/Au |
16-gauge syringe |
Amperometry |
1–50 mM |
Interstitial fluid |
91
|
Ethanol |
AOx/BSA/chitosan/carbon-PB-printed electrode |
Tattoo |
Amperometry |
0–36 mM |
Sweat |
92
|
Vitamins (C, B2, B6) |
AOx/BSA/carbon-PB-printed electrode |
Eyeglasses |
Amperometry |
— |
Tears |
93
|
Drug (caffeine) |
CNTs/Nafion/carbon printed electrode |
Wrist band |
DPV |
10–40 μM |
Sweat |
94
|
Glucose |
GOx membrane (MEMS)/Pt |
Mouthguard |
Amperometry |
5–1000 μM |
Saliva |
95
|
Glucose |
GOx/sol–gel membrane |
Contact Lens |
Differential module |
0–2 mM |
Tears |
96
|
Glucose |
Cerium oxide nanoparticles |
— |
Colorimetric |
— |
Tear |
97
|
Glucose |
Platinum/iridium coils |
Eyeglass |
Amperometry |
0–16 mM |
Tear |
98
|
Methyl paraoxon |
Carbon-PB-printed electrode |
Ring |
Amperometry |
0.25–1.25 mM |
Liquid phases |
99
|
Organophosphorus nerve agent |
OPH/Nafion/carbon-printed electrode |
Tattoo and textile |
SWV |
90–300 mg L−1 |
Vapor phases |
100
|
Na+ |
Ion selective membrane |
Mouthguard |
Potentiometry |
|
Saliva |
101
|
K+ |
Ion-selective membrane |
Eyeglass |
Amperometry |
0.1–100 mM |
Tears |
102
|
Diisopropyl fluorophosphate |
OPH/Nafion/PANi/carbon-printed electrode |
Tattoo |
Potentiometry |
10–120 mM |
Vapor and liquid phases |
103
|
Methyl parathion |
OPH/Nafion/carbon-printed electrode |
Nitrile glove |
SWV |
— |
Surfaces |
104
|
Latic acid |
PPD-LOx/carbon-PB-printed electrode |
Mouthguard |
CA |
0.1–0.5 mM |
Saliva |
105
|
Latic acid |
LOx/glutaraldehyde/BSA/PU/Pt |
Contact lens |
Amperometry |
0–1 mM |
Tears |
106
|
Capsaicin |
GOx/PB/carbonprinted electrode |
Nitrile glove |
Amperometry |
0.1–0.9 mM |
Food |
107
|
Dehydration |
AgNW/PDMS |
Skin |
Impedance |
|
|
108
|
Ascorbic acid |
Ascorbate oxidase |
Skin |
SWV |
— |
Sweat |
109
|
H2 |
Palladium oxide and zinc oxide hybrid nanoparticles |
— |
Colorimetric |
— |
— |
110
|
pH |
Bioactive silk ink |
— |
Colorimetric |
— |
Sweat |
111
|
H2S, Ammonia |
Lead acetate and ionic liquid |
— |
Colorimetric |
— |
Breath |
112
|
3. Recent development in wearable technology for detecting the spread of COVID-19
COVID-19, a new coronavirus generated by SARSCoV-2 that was initially diagnosed in Wuhan, China, in December 2019, has spread worldwide. COVID-19 is a worldwide health problem due to the exponential growth in people infected with the virus and the lack of a viable therapeutic treatment or vaccination.113 It is critical to keep track of a person's symptoms and fever while he or she is isolated. While this strategy is based mostly on the person's self-discipline, it is not completely controllable and is dependent on the individual's ability and amenability. Nevertheless, the advent of latest technology has made it possible to extract people’ biological data via wearable devices on a large scale, potentially altering the pandemic's course. COVID-19's appearance offers a chance to wearable technologies to mitigate the spread of disease. These wearables might provide symptom prediction and instantaneous remote monitoring based on blood pressure, body temperature, SpO2 levels, heart rate and lung sound. These wearables have been utilized to warn people about a possible COVID-19 contamination before the onset of serious symptoms,114 thanks to their excellent sensors. These wearables were also utilized to track multidimensional biological markers in patients to identify COVID-19 progression early.115
Face masks were discovered to be the primary protective item worn by everyone during the COVID 19 outbreak but the extended use of the facemask has a significant risk of infection and the user is more likely to get an infection as a result of the prolonged COVID-19 exposure.116 Rabiee et al.117 discussed the possibility of fabricating a face mask for early diagnosis along with self-protection. The wearable mask with a metal–organic framework coating was created for real-time detection of COVID-19 virus based on simple visual colour changes. The optical characteristics of the noble metal nanoparticles are altered to identify the presence of the virus in this study. Physisorption or chemisorption is used to dope the nanoparticles in a nanoporous matrix such as metal–organic frameworks (MOFs). This is followed by covering the mask with the nanoparticle doped MOF (Fig. 8). When the doped nanoparticles interact with the virus, they modify their optical characteristics, resulting in a noticeable colour shift. Kumar et al.118 studied drone-based systems and COVID-19 pandemic conditions, and presented an architecture for dealing with pandemic situations in diverse scenarios using real-time and simulation-based scenarios. A push–pull data fetching strategy is employed by the suggested architecture, which makes use of wearable sensors to capture observations in body area networks (BANs). The proposed architecture has been found to be effective in remote and heavily congested pandemic locations where either wireless or Internet access is a major issue or the likelihood of COVID-19 spread is high. It captures and retains a large amount of data in a short period of time, allowing for timely and appropriate action to be taken as and when it is necessary. A vast area may be covered for sanitization, thermal image collection, and patient identification in a short period of time (about 2 kilometres within 10 minutes) using an aerial route in a real-time drone-based healthcare system implementation for COVID-19 operations. In the simulation, the same statistics are observed as in the real world, with the addition of collision-resistant methods that have been proven to be effective in both indoor and outdoor healthcare operations.
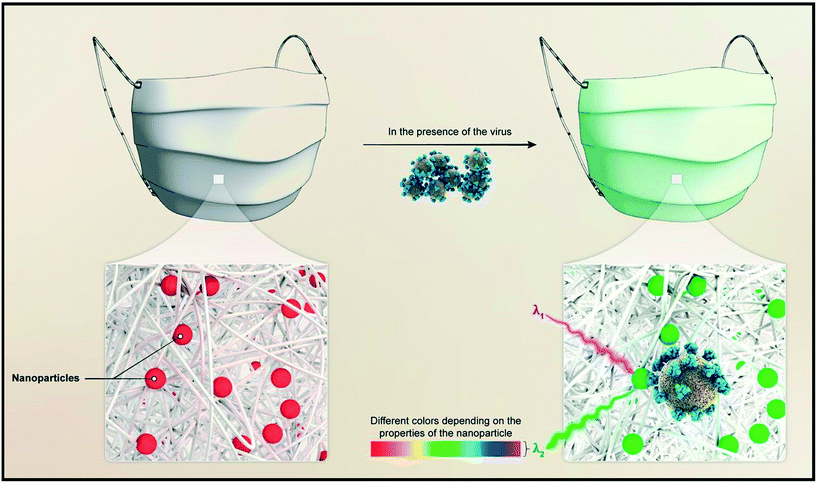 |
| Fig. 8 COVID-19 detection using a wearable mask. Reproduced from ref. 117 under creative commons attribution license, copyright @ MDPI (2020). | |
An IoT-enabled wearable monitoring device for COVID-19 has been developed by Bassam et al. as shown in Fig. 9.119 IoT cloud edge nodes process and evaluate the data from the wearable sensor in order to determine a person's current state of health. Even more importantly, if a possibly contaminated patient breaches quarantine conditions, the system instantly informs the appropriate medical authorities. The wearable IoT sensor layer, the cloud layer with the application peripheral interface (API), and the Android web layer for mobile phones are all part of the proposed system. First, the data from the IoT sensor layer is analysed to identify the health symptoms. Preventive measures, alarms, and quick responses are handled by the next layer, which is utilised to store data in a cloud database. In order to notify and inform the family members of patients who may have been infected, the Android mobile application layer is accountable. For forecasting and alerting the situation, the integrated system uses both an API and a mobile application that are synced with each other. In a nutshell, the wearable sensor layer measures temperature, heartbeat, SpO2, and cough count. It also provides real-time GPS position data of the patient to medical authorities and alert family members to lessen stress. The application peripheral interface layer stores, collects, and analyses data to monitor and govern social life during pandemics and emergencies. The 3D wearable design concept is relatively basic, ensuring the quality of life by decreasing COVID-19 spread. Hence, monitoring, treatment, and analysis of COVID-19 symptoms are all made easier with the help of the design.
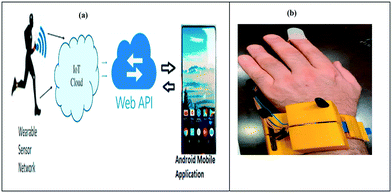 |
| Fig. 9 (a) Schematic of the healthcare system; (b) prototype of the wearable device. Reproduced from ref. 119 under creative commons attribution license, copyright @ Elsevier (2021). | |
4. Challenges
Wearable gadgets are transforming the healthcare profession, thanks to new smart materials. The healthcare paradigm is evolving away from acute, reactive, or preventive treatment and toward proactive care. Despite fast advancements in the area of healthcare wearables, obstacles must be addressed before they can be completely used in clinical settings. The potential advantages of using wearable technology in the health sector are accompanied with a slew of hurdles and unanswered problems. The duty for ensuring the accuracy, dependability, and accessibility of such fitness training structures for the patients who use them poses these issues. Furthermore, the bulk of those systems' core machine learning models are inaccurate. These models are critical because they provide a relationship between the device's sensors and the patient's health indicators. These and other problems have prompted the computer research community to develop new techniques to solve these new difficulties. Another obstacle in the way of wearable technology in the healthcare industry is its compatibility with the clinical environment. The discrepancy in the standards for storing, processing and measuring the data from the wearable is referred to as compatibility. Between the database of the wearable device and the clinical private databases, there is frequently a distinction. When the design of consumer-grade wearable devices devoted to health coaching fails to account for the fundamental features of clinical compliance, compatibility concerns develop. Machine learning model flaws constitute a significant difficulty in the wearables industry. With a few exceptions, dependent on infrastructure connection, today's wearables communicate using short-range wireless technologies. This trend is mostly due to current battery limits and the additional costs associated with employing longer-range communication technologies owing to increased power consumption. Nonetheless, the majority of wearables are still used for data collection, necessitating the use of advanced approaches to improve the overall effectiveness of the information processing life cycle. Nevertheless, owing to a lack of strong interoperability norms, tight coupling of diverse systems offered by different suppliers remains one of the most critical issues of wearables.
5. Future prospects
To address the criteria of sophisticated wearable sensors, such as ultrasensitivity, high recognition correctness, complete integration, and mass manufacturing, innovative materials and enhanced fabrication procedures must be created from a material science standpoint. Combining two or more functional qualities into a single innovative multi-functional material might provide healthcare wearable device developers greater options. Combining nanofibers with antiviral characteristics, for example, might provide a possible substrate material for long-term anti-COVID-19 health monitoring masks that don't need to be cleaned or disinfected. Smart wearable glasses, on the other hand, might be used to detect the spread of COVID-19, whether in air or in human bodies, by fitting them with sensors that detect COVID-19 cell movement in the same way that a microscope does. These wearables might potentially be utilised to lower infection rates by achieving early detection of people who are at risk of infection. Wearable technology might be utilised to reduce the incidence of COVID-19 transmission among patients, doctors, and other healthcare professionals, thanks to the availability of real-time data. Through their biosensors, such wearables might potentially be used to give telehealth and telemedicine services. Wearable sensors, from the standpoint of healthcare providers, play an important role in bridging the gap between users and providers, so they should not only monitor users' physiological parameters with high accuracy, but also provide multiple aspects of health status assessment in an easy-to-access manner. While wearable technology has other uses, it has not yet been employed during the epidemic. Researchers should devise new techniques or improve on current ones in order to extract sweat on demand and to employ these sensors in a range of applications. Sweat biomarkers have a lot of promise for diagnosis since sweat is a readily accessible fluid in the body. However, since such sensors rely on sweat, their uses are restricted to circumstances that need physical excretion. To employ these sensors for a variety of applications, on-demand sweat extraction, such as iontophoresis, is required. The eyewear biosensing device has a lot of promise and adaptability for non-invasive tear biomarker monitoring. Analyses are still needed to improve the system's accuracy and precision, as well as to learn more about the effects of tear stimulation and collection on analyte concentration and detector geometry on tear dilution. These investigations may lead to improved non-invasive tear analysis while also resolving the shortcomings of the contact-lenses sensing platform. These novel capabilities of the tear bioelectronic concept based on spectacles show great promise for non-invasive detection of a broad range of biomarkers, opening the path for widespread use of tear-based non-invasive wearable biosensing diagnostic systems. Prior to broad use, extensive validation tests and blood concentration correlations are required to prove the clinical and forensic value of the novel wearable tear-sensor device. To report the appropriate concentration values, appropriate algorithms will be necessary.
Wearable technology has enormous promise in the near future for flattening the healthcare expense curve. Computing in healthcare research has the potential to lead clinical development in the delivery of healthcare in the near future. The use of computers in healthcare will drive the development of new artificial intelligence models that are appropriate for wearable devices. These artificial intelligence models will play an important role in the management and prediction of patient health variations. Finally, as healthcare evolves, a rising number of academics from many areas will get together to form an interdisciplinary community focused on discovering solutions for wearable devices for health coaching. In addition, power supply is critical in the case of wearable sensors. Rigid batteries are unable to keep up with the present demands due to the trend toward downsizing and integration of devices, as well as the increased demand for in situ monitoring. It is possible to investigate battery-free and wireless sensing systems, with near-field communication (NFC) being a viable option. By connecting the circuit with the NFC module, it is possible to transmit data wirelessly and remove the need for batteries altogether. When the NFC-enabled circuit is coupled to the wearable sensor, it is possible to achieve real-time, in situ, and long-term detection of a variety of parameters at the same time. In previous research, it was discovered that the nanomaterial-enabled NFC antenna could be printed on a flexible substrate and connected with a wearable sensor, allowing for battery-free and wireless sensing to be achieved. Although the sensor and the receiving phone are only a short distance apart, it is possible that this will be a constraint. More emphasis should be made to the development of efficient algorithms for data transmission as well as the understanding of the features of data transmission over long distances and at high frequencies.
Since the introduction of lab-on-a-chip analytical devices, the creation of health monitoring devices, and the introduction of wearable bioelectronics with human skin and tissue interfaces, a plethora of new technologies have emerged. It is vitally crucial to increase the safety, stability, and dependability of the test equipment in order to create a flexible wearable device, which is a promising step forward. The enhancement of clinical features as well as analytical identification in the event of chronic disease, and its continuous real-time health monitoring, are both possible at the same time. It is also advised that wearable gadgets be quick, resilient, and dependable, as well as being comfortable to wear. A significant progress and development have been made in wearable biosensors/devices. Now-a-days there are significant reports showing current state of the art of wearable biosensing that is characterised by the demonstration of a proof-of-concept of wearable devices for the identification of various biomarkers. There have, however, been just a few advances taken in this subject in the direction of practical applications and commercialization. The characteristics taken into consideration include stability, precision, accuracy, stability, communication, and so forth. Wearable biosensors have confronted several basic obstacles and technological limitations that are connected to their scope. Successful expansion and extensive commercialization are dependent on the ability to overcome these difficulties.
In order to achieve effective validation of healthcare data from heterogeneous devices in tailored healthcare environments via the Internet of Things, more sophisticated smart algorithms must be created. Other than that, flexible pressure sensors with a low detection limit, a quick reaction speed, high sensitivity, a high density, and a wide sensing range are being developed. Unique and better knowledge of the process should be studied in order to produce an enhanced performance pressure sensor with regard to overall performance metrics, which remains a significant issue notwithstanding the above-mentioned advancements. In addition, sensor literature that addresses particular issues such as power consumption, hysteresis, slip, and force vectors has been seldom reported on. In order to further improve slip and force vector sensors, it is necessary to further develop the innovative mechanism and structure. The underlying cause of hysteresis has not yet been completely explored, and techniques of mitigation should be investigated further. Various materials, including active materials, electrode materials, and substrate materials that are flexible and intrinsically stretchable have been created for use in sensor construction. It is necessary to continue developing self-healing and stretchy insulators, conductors, semiconductors, and their composites that have high interfacial compatibility and processability. As a result, in order to develop a skin-like multifunctional integrated system, it is necessary to integrate various electronic components and high-density sensors (including pressure sensors) into a tiny soft substrate, necessitating the development of large-area and low-cost integration as well as fabrication techniques. Furthermore, because these sensors will create a large amount of information, it will be necessary to investigate how to gather, organise, manage, analyse, retain, and comprehend the massive amounts of data that will be generated.
More advanced smart algorithms must be developed in order to provide successful validation of healthcare data from heterogeneous devices in personalised healthcare environments via the Internet of Things. Flexible pressure sensors with a low detection limit, a rapid reaction time, high sensitivity, a high density of sensors, and a wide sensing range, among other characteristics, are currently being developed. A stupendous understanding of the process should be investigated in order to generate a pressure sensor with improved performance metrics in terms of sensitivity and selectivity that continues to be a serious concern despite the other mentioned improvements. Furthermore, sensor research that tackles specific concerns such as power consumption, hysteresis, slip, and force vectors has been relatively underreported on in the literature. It is vital to continue developing the unique mechanism and structure in order to further improve slip and force vector sensors. Because the fundamental cause of hysteresis has not yet been fully studied, it is necessary to research potential mitigation strategies in greater depth. Sensor building involves the use of a variety of flexible and inherently stretchy materials, including active materials, electrode materials, and substrate materials. Active materials, electrode materials, and substrate materials have all been developed for this purpose. It is essential to continue research and development of self-healing and stretchable insulators, conductors, semiconductors, and their composites that have excellent interfacial compatibility and processability, as well as other novel materials and processes. Because of this, in order to develop a skin-like multifunctional integrated system, it is necessary to integrate a variety of electronic components and high-density sensors (including pressure sensors) into a small soft substrate. As a result, large-area and low-cost integration, as well as fabrication techniques, must be developed. Because these sensors will generate enormous amounts of data, it will be required to examine how to acquire, organise, manage and analyse the immense amounts of data that will be generated, as well as how to store and grasp this data.
Wearable sensor systems with energy producing and storage components are urgently needed for personal healthcare monitoring as well as human activity monitoring, and an integrated wearable sensor system with energy generating and storage components is urgently needed. The development of low-power sensor integrated wearable platforms, high-output efficiency wearable generators, and large-capacity energy storage equipment, among other things, continues to have significant development potential. Wearable health monitoring systems that are systematic and intelligent are getting closer to the market. More auxiliary components, such as a signal processing and display unit, a treatment feedback mechanism, and so on, should be integrated into the system. In the early stages of intelligence, wearable devices are combined with personal computers or cellphones, which is the first step of computing. Users may simply obtain sufficient health information by incorporating relevant applications into their cellphones. Some next-generation healthcare systems may already feature display and processing modules; however, these modules need be completely rebuilt to fulfil the demands for flexibility, reduction, and integration. Next-generation intelligent systems may already have processing and display modules that will need to be redesigned in order to meet the demands for flexibility, reduction, and integration in the future. At the moment, prototypes of flexible screens, TFT displays, and other modules are being explored; nevertheless, they still require further reduction and integration.
6. Conclusion
Wearable sensors and associated healthcare systems have shown to be a viable solution to the challenge of providing efficient real-time medical services. Preventive treatment and assisting individuals in changing unhealthy lifestyles are becoming more crucial as a result of global issues such as an ageing population and growing health expenses. The urgent worldwide need for better healthcare quality, availability, and affordability will inevitably necessitate continual accurate and thorough monitoring of patients through wearable devices for health coaching. Wearable devices analyse and transmit a variety of data, including signals connected to human bodies and activities. Wearable health coaching technologies present a slew of new research issues while also providing solutions to certain previously voiced concerns. The issues of privacy, accuracy of sensor readings, and compatibility with medical ecosystems are only a few of them. Along with these issues, the research community has significant duties in the healthcare field. As a result, the wearables will take some time to emerge on store shelves. Fundamentals of electrochemistry in wearable conditions, software algorithms to treat signal artefacts in real-time wearable detection, reliable sensors for long-term wearable sensing in the body fluid, platforms for testing newly developed sensors, validation of test results, reproducible and standardised sampling methods, and the clinical significance of wearable testing data are all issues that need to be addressed.
Conflicts of interest
The authors declare that there is no conflict of interest.
References
- T. Luczak, R. Burch, E. Lewis, H. Chander and J. Ball, Int. J. Sports Sci. Coach, 2020, 15(1), 26 CrossRef.
-
P. Price, The Benefits of Wearable Technology in Tech. Rep. Revation Systems, 2020 Search PubMed.
- A. Ometov, V. Shubina, L. Klus, J. Skibinska, S. Saafi, P. Pascacio, L. Flueratoru, D. Q. Gaibor, N. Chukhno, O. Chukhno, A. Ali, A. Channa, E. Svertoka, W. B. Qaim, R. C. Marques, S. Holcer, J. T. Sospedra, S. Casteleyn, G. Ruggeri, G. Araniti, R. Burget, J. Hosek and E. S. Lohan, Comput. Netw., 2021, 193, 108074 CrossRef.
- J. Zhou, Z. Cao, X. Dong, N. Xiong and A. V. Vasilakos, Inf. Sci., 2015, 314, 255 CrossRef.
-
J. Hayward, Wearable Sensors 2018–2028: Technologies, Markets & Players, IDTechEx Ltd, 2018 Search PubMed.
-
J. Smith, Wearables Shipments Will Double By 2021, Insider Inc, 2017 Search PubMed.
- V. Shubina, A. Ometov and E. S. Lohan, Proc. of 12th International Congress on Ultra Modern Telecommunications and Control Systems and Workshops (ICUMT), IEEE, 2020, 229 Search PubMed.
-
R. T. Llamas, Enterprise Wearable Market: Information By Technology (IoT, Bluetooth, BLE), Product (Wristwear, Footwear, Eyewear), Application (Infotainment, IT), and Regional Outlook – Forecast Till 2026, Tech. Rep. SR1195, Straits Research, 2019.
-
The Benefits of Wearable Technology in the Workplace, DVV Media HR Group Ltd, 2018 Search PubMed.
- J. Xie, Q. Chen, H. Shen and G. Li, J. Electrochem. Soc., 2020, 167, 037541 CrossRef CAS.
- A. N. Kozitsina, T. S. Svalova, N. N. Malysheva, A. V. Okhokhonin, M. B. Vidrevich and K. Z. Brainina, Biosensors, 2018, 8, 1 CrossRef PubMed.
- N. Bhalla, P. Jolly, N. Formisano and P. Estrela, Essays Biochem., 2016, 60, 1 CrossRef PubMed.
- M. R. Khosravani and T. Reinicke, Sens. Actuators, A, 2020, 305, 111916 CrossRef CAS.
- Madhuprasad, M. P. Bhat, H. Y. Jung, D. Losic and M. D. Kurkuri, Chem. – Eur. J., 2016, 22, 6148 CrossRef CAS PubMed.
- M. P. Bhat, Y. Madhuprasad, P. Patil, S. K. Nataraj, T. Altalhi, H. Y. Jung, D. Losic and M. D. Kurkuri, Chem. Eng. J., 2016, 303, 14 CrossRef CAS.
- M. P. Bhat, M. Kurkuri, D. Losic, M. Kigga and T. Altalhi, Anal. Chim. Acta, 2021, 1159, 338439 CrossRef CAS.
- B. Ariyatum, R. Holland, D. Harrison and T. Kazi, J. Text. Inst., 2005, 96(4), 199 CrossRef.
-
Y. Silina and H. Haddadi, in Proc. of the 19th International Symposium on Wearable Computers, Osaka Japan, 2015 Search PubMed.
- L. E. Dunne, Fash. Pract. J. Des. Creat. Process Fash. Ind., 2010, 2(1), 41–66 Search PubMed.
- A. Salim and S. Lim, Biosens. Bioelectron., 2019, 141, 111422 CrossRef CAS PubMed.
- B. Noah, M. S. Keller, S. Mosadeghi, L. Stein, S. Johl, S. Delshad, V. C. Tashjian, D. Lew, J. T. Kwan, A. Jusufagic and B. M. R. Spiegel, NPJ Digit. Med., 2017, 2018, 1 Search PubMed.
- M. Gray, J. Meehan, C. Ward, S. P. Langdon, I. H. Kunkler, A. Murray and D. Argyle, Vet. J., 2018, 239, 21 CrossRef CAS PubMed.
- V. Scognamiglio and F. Arduini, TrAC, Trends Anal. Chem., 2019, 120, 115642 CrossRef CAS.
- B. Purohit, A. Kumar, K. Mahato and P. Chandra, Curr. Opin. Biomed. Eng., 2020, 13(2020), 42 CrossRef.
- A. K. C. Neelam and J. S. Rana, Anal. Biochem., 2019, 581, 113345 CrossRef CAS PubMed.
- A. W. Zaibudeen and J. Philip, Sens. Actuators, B, 2018, 268(1), 338 CrossRef CAS.
- P. J. Colvonen, NPJ Digit. Med., 2021, 4, 38 CrossRef.
- Y. Chen, P. Zhang, Y. Li, K. Zhang, J. Su and L. Huang, J. Appl. Phys., 2021, 54, 155101 CAS.
- J. Kim, A. S. Campbell and J. Wang, Talanta, 2018, 177, 163 CrossRef CAS PubMed.
- G. He, Y. C. Zhang, D. C. Tandt and J. Stiens, J. Phys. D: Appl. Phys., 2020, 53, 095404 CrossRef CAS.
- X. Wu, S. Mao, J. Chen and J. Huang, Adv. Mater., 2018, 30, 1705642 CrossRef.
- F. Wen, T. He, H. Liu, H. Y. Chen, T. Zhang and C. Lee, Nano Energy, 2020, 78, 105155 CrossRef CAS.
- M. A. A. Mamun and M. R. Yuce, Adv. Funct. Mater., 2020, 30, 2005703 CrossRef CAS.
- M. A. Sheikh Iqbal, I. Mahgoub, E. Du, M. A. Leavitt and W. Asghar, npj Flex. Electron., 2021, 5(1), 9 CrossRef.
- S. Li, Z. Ma, Z. Cao, L. Pan and Y. Shi, Small, 2020, 16, 1903822 CrossRef CAS.
- J. Heikenfeld, A. Jajack, J. Rogers, P. Gutruf, L. Tian, T. Pan, R. Li, M. Khine, J. Kim, J. Wang and J. Kim, Lab Chip, 2018, 18, 217 RSC.
- K. I. Park, J. H. Son, G. T. Hwang, C. K. Jeong, J. Ryu, M. Koo, I. Choi, S. H. Lee, M. Byun, Z. L. Wang and K. J. Lee, Adv. Mater., 2014, 26, 2514 CrossRef CAS PubMed.
- T. K. Bera, J. Med. Eng., 2014, 2014, 28 Search PubMed.
- F. Taffoni, D. Formica, P. Saccomandi, G. D. Pino and E. Schena, Sensors, 2013, 13(10), 14105 CrossRef.
- H. N. Kim, W. X. Ren, J. S. Kim and J. Yoon, Chem. Soc. Rev., 2012, 41(8), 3210 RSC.
- M. P. Bhat, S. Vinayak, J. Yu, H. Y. Jung and M. Kurkuri, ChemistrySelect, 2020, 5, 13135 CrossRef CAS.
- M. Finnegan, E. Duffy and A. Morrin, Sens. Bio-Sens. Res., 2022, 35, 100473 CrossRef.
- L. Guo, J. A. Jackman, H. H. Yang, P. Chen, N. J. Cho and D. H. Kim, Nano Today, 2015, 10(2), 213 CrossRef CAS.
- J. Wu, W. Liu, J. Ge, H. Zhang and P. Wang, Chem. Soc. Rev., 2011, 40(7), 3483 RSC.
- J. Heikenfeld, Electroanalysis, 2016, 28, 1242 CrossRef CAS.
- A. Koh, D. Kang, Y. Xue, S. Lee, R. M. Pielak, J. Kim, T. Hwang, S. Min, A. Banks, P. Bastien, M. C. Manco, L. Wang, K. R. Ammann, K. I. Jang, P. Won, S. Han, R. Ghaffari, U. Paik, M. J. Slepian, G. Balooch, Y. Huang and J. A. Rogers, Sci. Transl. Med., 2016, 8, 366ra165 Search PubMed.
- A. J. Bandodkar and J. Wang, Trends Biotechnol., 2014, 32, 363–371 CrossRef CAS PubMed.
- D. R. Seshadri, R. T. Li, J. E. Voos, J. R. Rowbottom, C. M. Alfes, C. A. Zorman and C. K. Drummond, NPJ Digit. Med., 2019, 2, 71 CrossRef PubMed.
- N. Bhalla, P. Jolly, N. Formisano and P. Estrela, Biochemistry, 2016, 60, 1 Search PubMed.
- J. Kim, A. S. Campbell, B. E. F. de Avila and J. Wang, Nat. Biotechnol., 2019, 37, 389 CrossRef CAS PubMed.
- C. G. Nunez, L. Manjakkal and R. Dahiya, npj Flex. Electron., 2019, 3, 1 CrossRef.
- M. M. Rodgers, V. M. Pai and R. S. Conroy, IEEE Sens. J., 2015, 15, 3119 Search PubMed.
- A. Salim and S. Lim, Biosens. Bioelectron., 2019, 141, 111422 CrossRef CAS PubMed.
- Q. Ouyang, X. Feng, S. Kuang, N. Panwar, P. Song, C. Yang, G. Yang, X. Hemu, G. Zhang, H. S. Yoon, J. P. Tam, B. Liedberg, G. Zhu, K. T. Yong and Z. L. Wang, Nano Energy, 2019, 62, 610 CrossRef CAS.
- V. R. Jayaneththi, K. Aw, M. Sharma, J. Wen, D. Svirskis and A. J. McDaid, Sens. Actuators, B, 2019, 297, 126708 CrossRef CAS.
- M. Swan, Int. J. Environ. Res. Public Health, 2009, 6, 492 CrossRef PubMed.
- S. R. Steinhubl, E. D. Muse and E. J. Topol, Sci. Transl. Med., 2015, 7, 283rv3 Search PubMed.
- J. A. Levine, Technology and Investment, 2017, 8, 33 CrossRef.
- A. K. Yetisen, J. L. M. Hurtado, B. Unal, A. Khademhosseini and H. Butt, Adv. Mater., 2018, 30, 1706910 CrossRef PubMed.
- H. Gao, L. Wen, J. Tian, Y. Wu, F. Liu, Y. Lin, W. Hua and G. Wu, Biosens. Bioelectron., 2019, 142, 111504 CrossRef CAS PubMed.
- E. Schoolaert, R. Hoogenboom and K. D. Clerck, Adv. Funct. Mater., 2017, 27, 1–26 Search PubMed.
- A. Singh, A. Sharma, A. Ahmed, A. K. Sundramoorthy, H. Furukawa, S. Arya and A. Khosla, Biosensors, 2021, 11(9), 336 CrossRef CAS PubMed.
- J. Wang, Nucleic Acids Res., 2000, 28(16), 3011 CrossRef CAS PubMed.
- E. M. Brynda, J. P. Sęk, A. Kasprzak, A. Kroikowska, M. Donten, M. Patrzalek, M. Poplawska and A. M. Nowicka, Biosens. Bioelectron., 2019, 128, 23 CrossRef PubMed.
- Y. Yu, H. Y. Y. Nyein, W. Gao and A. Javey, Adv. Mater., 2020, 32(15), 1902083 CrossRef CAS PubMed.
- T. Someya and M. Amagai, Nat. Biotechnol., 2019, 37, 382 CrossRef CAS PubMed.
- M. Li, L. Wang, R. Liu, J. Li, Q. Zhang, G. Shi, Y. Li, C. Hou and H. Wang, Biosens. Bioelectron., 2021, 174, 112828 CrossRef CAS PubMed.
- Z. Xu, J. Song, B. Liu, S. Lv, F. Gao, X. Luo and P. Wang, Sens. Actuators, B, 2021, 348, 130674 CrossRef CAS.
- C. Zhao, X. Li, Q. Wu and X. Liu, Biosens. Bioelectron., 2021, 188, 113270 CrossRef CAS PubMed.
- M. Senior, Nat. Biotechnol., 2014, 32, 856 CrossRef CAS PubMed.
- M. Falk, V. Andoralov, M. Silow, M. D. Toscano and S. Shleev, Anal. Chem., 2013, 85, 6342 CrossRef CAS PubMed.
- B. F. Grant, S. P. Chou, T. D. Saha, R. P. Pickering, B. T. Kerridge, W. J. Ruan, B. Huang, J. Jung, H. Zhang, A. Fan and D. S. Hasin, JAMA Psychiatry, 2017, 74, 911 CrossRef PubMed.
- A. C. Campbell, J. Kim and J. Wang, Curr. Opin. Electrochem., 2018, 10, 126 CrossRef CAS PubMed.
- M. Gamella, S. Campuzano, J. Manso, G. G. de Rivera, F. L. Colino, A. J. Reviejo and J. M. Pingarron, Anal. Chim. Acta, 2014, 806, 1 CrossRef CAS PubMed.
- A. Hauke, P. Simmers, Y. R. Ojha, B. D. Cameron, R. Ballweg, T. Zhang, N. Twine, M. Brothers, E. Gomez and J. Heikenfeld, Lab Chip, 2018, 18, 3750 RSC.
- H. G. Giles, S. Sandrin, V. Saldivia and Y. Israel, J. Clin. Exper. Res., 1988, 12, 255 CrossRef CAS PubMed.
- J. R. Sempionatto, L. C. Brazaca, L. G. Carmona, G. Bolat, A. S. Campbell, A. Martin, G. Tang, R. Shah, R. K. Mishra, J. Kim, V. Zucolotto, A. Escarpa and J. Wang, Biosens. Bioelectron., 2019, 137, 161 CrossRef CAS PubMed.
- J. Kim, A. S. Campbell and J. Wang, Talanta, 2018, 177, 163 CrossRef CAS PubMed.
- Y. Yang and W. Gao, Chem. Soc. Rev., 2019, 48, 1465 RSC.
- J. R. Sempionatto, T. Nakagawa, A. Pavinatto, S. T. Mensah, S. T. Imani, P. Mercier and J. Wang, Lab Chip, 2017, 17, 1834 RSC.
- G. Matzeu, L. M. Soldevila, W. Li, A. Naidu, T. H. Turner, R. Gu, P. R. Blumeris, P. Song, D. G. Pascal, G. Guidetti, M. Li and F. G. Omenetto, Adv. Mater., 2020, 32, 2001258 CrossRef CAS PubMed.
- D. H. Kim, J. H. Cha, J. Y. Lim, J. Bae, W. Lee, K. R. Yoon, C. Kim, J. S. Jang, W. Hwang and I. D. Kim, ACS Nano, 2020, 14, 16907 CrossRef CAS PubMed.
- N. Promphet, S. Ummartyotin, W. Ngeontae, P. Puthongkham and N. Rodthongkum, Anal. Chim. Acta, 2021, 1179, 338643 CrossRef CAS PubMed.
- Z. Zhou, T. Shu, Y. Sun, H. Si, P. Peng, L. Su and X. Zhang, Biosens. Bioelectron., 2021, 192, 113530 CrossRef CAS PubMed.
- A. Sharma, A. Singh, A. Khosla and S. Arya, J. Saudi Chem. Soc., 2021, 25, 101340 CrossRef CAS.
- H. Wang, D. Yang, R. Tan, Z. J. Zhou, R. Xu, J. F. Zhang and Y. Zhou, Sens. Actuators, B, 2017, 247, 883–888 CrossRef CAS.
- J. Zheng, H. L. Noh, H. W. Chun, B. M. Oh, J. Lee, S. K. Choi, E. Kim, D. Jung, W. S. Lee and J. H. Kim, Sens. Actuators, B, 2021, 341, 130013 CrossRef CAS.
- N. Prompheta, J. P. Hinestroza, P. Rattanawaleedirojn, N. Soatthiyanon, K. Siralertmukul, P. Potiyaraja and N. Rodthongkum, Sens. Actuators, B, 2020, 321, 128549 CrossRef.
- A. Barfidokht, R. K. Mishra, R. Seenivasan, S. Liu, L. J. Hubble, J. Wang and D. A. Hall, Sens. Actuators, B, 2019, 296, 126422 CrossRef CAS PubMed.
- R. K. Mishra, J. R. Sempionatto, Z. Li, C. Brown, N. M. Galdino, R. Shah, S. Liu, L. J. Hubble, K. Bagot, S. Tapert and J. Wang, Talanta, 2020, 211, 120757 CrossRef CAS PubMed.
- H. Jiang, X. Zhou, S. Kulkarni, M. Uranian, R. Seenivasan and D. A. Hall, IEEE, 2018, 1–4 Search PubMed.
- J. Kim, I. Jeerapan, S. Imani, T. N. Cho, A. Bandodkar, S. Cinti, P. P. Mercier and J. Wang, ACS Sens., 2016, 1, 1011 CrossRef CAS.
- J. R. Sempionatto, L. C. Brazaca, L. G. Carmona, G. Bolat, A. S. Campbell, A. Martin, G. Tang, R. Shah, R. K. Mishra, J. Kim, V. Zucolotto, A. Escarpa and J. Wang, Biosens. Bioelectron., 2019, 137, 161 CrossRef CAS PubMed.
- L. C. Tai, W. Gao, M. Chao, M. Bariya, Q. P. Ngo, Z. Shahpar, H. Y. Y. Nyein, H. Park, J. Sun, Y. Jung, E. Wu, H. M. Fahad, D. H. Lien, H. Ota, G. Cho and A. Javey, Adv. Mater., 2018, 30, 1707442 CrossRef PubMed.
- T. Arakawa, Y. Kuroki, H. Nitta, P. Chouhan, K. Toma, S. I. Sawada, S. Takeuchi, T. Sekita, K. Akiyoshi, S. Minakuchi and K. Mitsubayashi, Biosens. Bioelectron., 2016, 84, 106 CrossRef CAS PubMed.
- H. Yao, Y. Liao, A. R. Lingley, A. Afanasiev, I. Lähdesmäki, B. P. Otis and B. A. Parviz, J. Micromech. Microeng., 2012, 22, 075007 CrossRef.
- S. Park, J. Hwang, H. J. Jeon, W. R. Bae, I. K. Jeong, T. G. Kim, J. Kang, Y. G. Han, E. Chung and D. Y. Lee, ACS
Appl. Nano Mater., 2021, 4, 5198 CrossRef CAS.
- A. E. Kownacka, D. Vegelyte, M. Joosse, N. Anton, B. J. Toebes, J. Lauko, I. Buzzacchera, K. Lipinska, D. A. Wilson, N. G. Duijvestijn and C. J. Wilson, Biomacromolecules, 2018, 19, 4504 CrossRef CAS PubMed.
- J. R. Sempionatto, R. K. Mishra, A. Martín, G. Tang, T. Nakagawa, X. Lu, A. S. Campbell, K. M. Lyu and J. Wang, ACS Sens., 2017, 2, 1531 CrossRef CAS PubMed.
- R. K. Mishra, A. Martín, T. Nakagawa, A. Barfidokht, X. Lu, J. R. Sempionatto, K. M. Lyu, A. Karajic, M. M. Musameh, I. L. Kyratzis and J. Wang, Biosens. Bioelectron., 2018, 101, 227 CrossRef CAS PubMed.
- Y. Lee, C. Howe, S. Mishra, D. S. Lee, M. Mahmood, M. Piper, Y. Kim, K. Tieu, H. S. Byun, J. P. Coffey, M. Shayan, Y. Chun, R. M. Costanzo and W. H. Yeo, Proc. Natl. Acad. Sci. U. S. A., 2018, 115, 5377 CrossRef CAS PubMed.
- J. R. Sempionatto, T. Nakagawa, A. Pavinatto, S. T. Mensah, S. Imani, P. Mercier and J. Wang, Lab Chip, 2017, 17, 1834 RSC.
- R. K. Mishra, A. Barfidokht, A. Karajic, J. R. Sempionatto, J. Wang and J. Wang, Sens. Actuators, B, 2018, 273, 966 CrossRef CAS.
- R. K. Mishra, L. J. Hubble, A. Martín, R. Kumar, A. Barfidokht, J. Kim, M. M. Musameh, I. L. Kyratzis and J. Wang, ACS Sens., 2017, 2, 553 CrossRef CAS PubMed.
- J. Kim, G. V. Ramírez, A. J. Bandodkar, W. Jia, A. G. Martinez, J. Ramírez, P. Mercier and J. Wang, Analyst, 2014, 139, 1632 RSC.
- N. Thomas, I. Lähdesmäki and B. A. Parviz, Sens. Actuators, B, 2012, 162, 128 CrossRef CAS.
- B. Ciui, A. Martin, R. K. Mishra, T. Nakagawa, T. J. Dawkins, M. Lyu, C. Cristea, R. Sandulescu and J. Wang, ACS Sens., 2018, 3, 2375 CrossRef CAS PubMed.
- S. Yao, A. Myers, A. Malhotra, F. Lin, A. Bozkurt, J. F. Muth and Y. Zhu, Adv. Healthcare Mater., 2017, 6, 1601159 CrossRef.
- J. R. Sempionatto, A. A. Khorshed, A. Ahmed, D. L. Silva, A. Barfidokht, L. Yin, K. Y. Goud, M. A. Mohamed, E. Bailey, J. May and C. Aebischer, ACS Sens., 2020, 5, 1804 CrossRef CAS PubMed.
- S. H. Hwang, Y. K. Kim, S. M. Jeong, C. Choi, K. Y. Son, S. K. Lee and S. K. Lim, Text. Res. J., 2020, 90, 2198 CrossRef CAS.
- G. Matzeu, L. M. Soldevila, W. Li, A. Naidu, T. H. Turner, R. Gu, P. R. Blumeris, P. Song, D. G. Pascal, G. Guidetti, M. Li and F. G. Omenetto, Adv. Mater., 2020, 32, 2001258 CrossRef CAS PubMed.
- D. H. Kim, J. H. Cha, J. Y. Lim, J. Bae, W. Lee, K. R. Yoon, C. Kim, J. S. Jang, W. Hwang and I. D. Kim, ACS Nano, 2020, 14, 16907 CrossRef CAS PubMed.
- S. Whitelaw, M. A. Mamas, E. Topol and H. G. C. V. Spall, Lancet Digital Health, 2020, 2, E435–E440 CrossRef.
- D. R. Seshadri, E. V. Davies, E. R. Harlow, J. J. Hsu, S. C. Knighton, T. A. Walker, J. E. Voos and C. K. Drummond, Front. Digit. Health, 2020, 2, 1 CrossRef PubMed.
- C. K. Wong, D. T. Y. Ho, A. R. Tam, M. Zhou, Y. M. Lau, M. O. Y. Tang, R. C. F. Tong, K. S. Rajput, G. Chen, S. C. Chan, C. W. Siu and I. F. N. Hung, BMJ Open, 2020, 10(7), 1 CrossRef PubMed.
- E. Atangana and A. Atangana, Results Phys., 2020, 19, 103425 CrossRef PubMed.
- N. Rabiee, M. Bagherzadeh, A. Ghasemi, H. Zare, S. Ahmadi, Y. Fatahi, R. Dinarvad, M. Rabiee, S. Ramakrishna, M. Shokouhimehr and R. S. Varma, Int. J. Mol. Sci., 2020, 21, 5126 CrossRef CAS PubMed.
- A. Kumar, K. Sharma, H. Singh, S. G. Naugriya, S. S. Gill and R. Buyya, Future Gener. Comput. Syst., 2021, 115, 1 CrossRef PubMed.
- N. Al Bassam, S. A. Hussain, A. Al Qaraghuli, J. Khan, E. P. Sumesh and V. Lavanya, Inform. Med. Unlocked, 2021, 24, 100588 CrossRef PubMed.
|
This journal is © The Royal Society of Chemistry 2022 |
Click here to see how this site uses Cookies. View our privacy policy here.