DOI:
10.1039/D2SC02116A
(Edge Article)
Chem. Sci., 2022,
13, 12899-12905
Direct discrimination of cell surface glycosylation signatures using a single pH-responsive boronic acid-functionalized polymer†
Received
13th April 2022
, Accepted 5th October 2022
First published on 24th October 2022
Abstract
Cell surface glycans serve fundamental roles in many biological processes, including cell–cell interaction, pathogen infection, and cancer metastasis. Cancer cell surface have alternative glycosylation to healthy cells, making these changes useful hallmarks of cancer. However, the diversity of glycan structures makes glycosylation profiling very challenging, with glycan ‘fingerprints’ providing an important tool for assessing cell state. In this work, we utilized the pH-responsive differential binding of boronic acid (BA) moieties with cell surface glycans to generate a high-content six-channel BA-based sensor array that uses a single polymer to distinguish mammalian cell types. This sensing platform provided efficient discrimination of cancer cells and readily discriminated between Chinese hamster ovary (CHO) glycomutants, providing evidence that discrimination is glycan-driven. The BA-functionalized polymer sensor array is readily scalable, providing access to new diagnostic and therapeutic strategies for cell surface glycosylation-associated diseases.
Introduction
Mammalian cells are coated with complex network of interconnected proteoglycans and lipoglycans that perform crucial roles in multiple biological processes, such as the cell–cell adhesion,1–3 pathogen infection,4–6 and cancer metastasis.7–9 Glycosylation signatures from this glycocalyx can give a predictive readout of cellular behaviors, providing a unique target for cell surface-based diagnostics and therapeutics. For example, studies have shown that glycosylation changes in cancer cells can take multiple forms, including the increase in the amount of incomplete glycans and the generation of novel glycans, making glycosylation alteration a hallmark of cancer.10–12
The structures of glycans on cell surfaces are complex, making cell surface glycan profiling a very challenging task. Mass spectrometry (MS)-based approaches have been widely used to map glycoproteins on cell surface.13–16 However, MS-based methods require a large number of samples and multiple preparation steps. Moreover, they need specific analysis tools to interpretate the data, making these methods expensive and slow.17 Numerous studies have applied enzyme-linked immunosorbent assay (ELISA), especially lectin-based ELISA assays to detect cell surface glycans based on the high affinity of lectin with carbohydrates.18–22 Significantly, this approach generates fingerprints as opposed to discrete measurement of glycans due to lectin/antibody-glycan cross-reactivity.23
Array-based sensing can provide a highly sensitive sensing platform to discriminate complicated bio-analytes,24 including proteins,25–27 bacteria,28–30 and cell phenotypes,31–33 using selective and differential interaction between sensor elements and target analytes.34 Polymeric sensor array platforms have emerged as promising strategies due to their high stability, reproducibility, and scalability.35,36 Previously, our lab fabricated a three-channel sensor using gold nanoparticles featuring arginine ligand conjugated with fluorescent proteins to identify mammalian cells based on cell-surface glycosylation pattern.37 The use of gold nanoparticles and protein, however, limited the scalability and stability of this approach, preventing clinical and screening applications of this technology.
We hypothesized that a polymer-based sensing platform could provide a fully synthetic system suitable for high throughput-high content screening (HT-HCS) glycosylation profiling. Boronic acids (BA) can bind with diol motifs commonly present in glycans, providing a dynamic covalent recognition motif for cell surface glycan detection.38–40 In addition, the structure and water solubility of boronic acids are tunable by pH.41 BA exists as a boronate anion form when the solution pH is higher than the pKa of BA, becoming uncharged when the solution pH is below the pKa.42 These properties make the binding affinity between BA and diols pH-responsive.43 Hence, utilizing multiple pH environments provides high-content multidimensional data for glycosylation-based sensing. We report here the use of boronic acid-functionalized polymer to generate a new polymeric sensor (PONI-BA-pyrene) that can differentiate mammalian cells based on the glycan signatures on their surface (Fig. 1). We applied this sensing platform to successfully identify cancer cells with 100% classification accuracy and 93% correct unknown identification. We hypothesized that the discrimination of cancer cells arose from their unique glycosylation patterns. Testing the sensor against Chinese hamster ovary (CHO) glycomutants supported this contention, with the classification accuracy between CHO lines 100%, matched by unknown identification accuracy of 100%. Taken together, a single polymer sensing platform presents a new strategy for discriminating cell surface glycosylation changes that is readily scalable to HT-HCS applications.
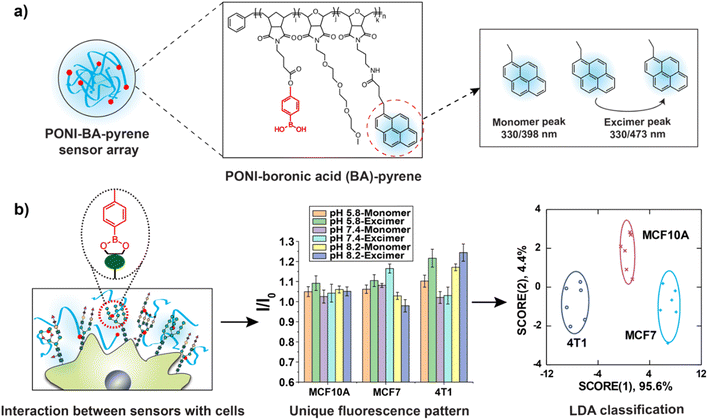 |
| Fig. 1 Phenotyping cancer cells using PONI-BA-pyrene sensor array. (a) Chemical structure of the polymeric sensor. Boronic acid serves as the recognition element and pyrene serves as the transducer with the monomer emission of 398 nm and excimer emission of 473 nm at the excitation of 330 nm. (b) The BA-pyrene sensors are incubated with cells at three different pH phosphate buffers (pH 5.8, pH 7.4 and pH 8.2), generating six fluorescent channels. The selective interactions of sensor elements with cell surface glycans result in unique fluorescent pattern for each cell line, and the fluorescence patterns were analyzed using LDA. | |
Results and discussion
Design and characterization of the sensor array
The polymer used a “semiarthritic” poly (oxanorborneneimide) (PONI) random copolymer scaffold. The scaffold has the PONI backbone which assumes a semi-flexible architecture in solution to provide structural advantages, such as being readily pre-organized to prevent strong intrachain aggregation and ease of modification.44 Phenylboronic acid was conjugated to the polymer backbone as the recognition element due to the high affinity of BA with diols contained in cell surface glycans.45 Tetra (ethylene glycol) (TEG) sidechains were used to increase the solubility of sensors, and pyrene was used as the signal transducer to form PONI-BA-pyrene sensor. The synthesized polymer was characterized by GPC (Fig. S1†) and the molecular weight was 30 kDa with a polydispersity of 1.01. The limited aggregation of the polymer was validated through transmission electron microscopy (Fig. S2†). The fluorescence properties were determined using a standard plate reader, with a two-channel fluorescence output generated from the monomeric pyrene and the pyrene excimer (Fig. 2).
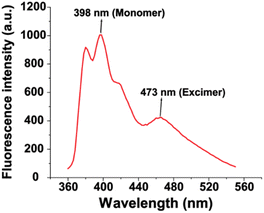 |
| Fig. 2 Fluorescence spectrum of PONI-BA-pyrene polymer. | |
The hydrodynamic size of the polymer under different pH was determined through dynamic light scattering (DLS), with diameters of 14.0 ± 5.0 nm (pH 5.8), 19.4 ± 4.7 nm (pH 7.4), and 15.6 ± 2.7 nm (pH 8.2) (Fig. S3†). The results demonstrated that the change of pH value did not noticeably alter the size of PONI-BA-pyrene polymer.
The sensor solution was generated by diluting PONI-BA-pyrene using 5 mM phosphate buffers with three different pH values (pH 5.8, pH 7.4 and pH 8.2) to the final concentration of 40 μg L−1. Six characteristic fluorescent channels were generated from the three representative pH values (monomer and excimer, at pH 5.8, 7.4 and 8.2) (Fig. S4†).
pH-responsive binding of BA-based sensor array with cell surface glycans
The general binding affinity of boronic acids for diols in saccharides is as follows: cis-1,2-diol > 1,3-diol ≫ trans-1,2-diol.46 To confirm that boronic acid motifs of PONI-BA-pyrene bind with diols present in glycans, we studied the effects of 500 nM galactose, lactose and sucrose on the fluorescence of PONI-BA-pyrene (40 μg L−1) at pH 7.4. Galactose and lactose have cis-1,2-diols in their structure while sucrose only contains trans-1,2-diols. After incubating with galactose and lactose, the fluorescence spectrum of PONI-BA-pyrene changed substantially, especially at the pyrene excimer wavelength (Fig. 3a). In contrast, no significant change was observed with sucrose. In addition, we also checked the variation of fluorescence intensity of PONI-BA-pyrene with increases in concentration of the above saccharides. Fig. S5† shows that the fluorescence intensity of sensor increased with increased concentration of galactose and lactose, while the concentration of sucrose did not significantly affect the fluorescence of the sensor. The above results indicate that the binding of PONI-BA-pyrene with glycans was primarily from the interactions between boronic acid motifs with cis-1,2-diols.
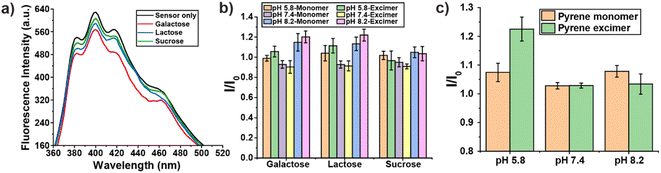 |
| Fig. 3 The fluorescent responses of BA-based sensor array. (a) Fluorescence intensity of 40 μg L−1PONI-BA-pyrene after incubating with 500 nM galactose, lactose or sucrose at pH 7.4 for 30 min. Each value is the average of three parallel measurements (n = 3). (b) Fluorescence response of PONI-BA-pyrene after 30 min incubation with galactose, lactose or sucrose at different pH values (n = 3). (c) Fluorescence response of PONI-BA-pyrene after 30 min incubation with HeLa cells (n = 8). | |
Fourier transform infrared spectroscopy (FT-IR) spectra was performed on norbornene imide-based boronic acid monomer (NI-BA) with and without the presence of galactose to further study the binding of the boronic acids to the diols of the saccharides (Fig. S6 and S7†). Mannose pentaacetate was taken as a control without diols. The results showed a change in the transmittance in the O–H stretching region between galactose (Fig. S7a†) and mannose pentaacetate (Fig. S7b†) which due to the absence of the O–H in the control. The broad spectra difference of NI-BA after adding galactose also suggested that the O–H vibration from the boronic acid moiety of NI-BA slightly shifted frequency relative to the O–H vibrations from the alcohol in the saccharide.47,48 Fig. S7c and d† showed the region of B–O stretching modes and C–O stretching modes from the sugars. The trough ∼1100 cm−1 was consistent with a shift in the B–O stretching mode from the free boronic acid to the diol bound boronic ester, along with the appearance of C–O stretching frequency from the saccharide.49
Binding affinities between boronic acid with cis-diol have been shown to be pH dependence (Scheme S1†).41,50 To validate the pH responsiveness of binding between PONI-BA-pyrene and cis-diol containing glycans, we further studied the fluorescence response of PONI-BA-pyrene after incubation with galactose, lactose or sucrose (500 nM) at different pH values. The ratio of the fluorescence intensity of sensor incubated with saccharides for 30 min to the fluorescence intensity of sensor only (I/I0) was recorded as fluorescence responses. Fig. 3b shows that galactose and lactose had measurable changes in fluorescent intensity. As expected, however, no significant change was observed at different pH with sucrose. This finding further indicates that the interactions between the BA-based polymer and glycans were primarily driven by the binding of boronic acid with cis-1,2-diols.
We next determined the fluorescent responses of the sensor array to HeLa cells after 30 min incubation at 37 °C (Fig. 3c). Our sensor generated unique fluorescent signals to HeLa cells at each pH, confirming that the interaction between BA-based polymer with cell surface glycans is pH dependent.
Discrimination of different cancer cell lines
Glycan changes in cancer cells take a variety of forms, including under expression and/or overexpression of specific glycans, the increase of incomplete or truncated glycans, and the generation of novel glycans.10 We hypothesized that each cancer cell line has a distinct glycosylation pattern that could be discriminated with the BA-based sensing platform. Initial experiment focused on the discrimination of different cancer cell types using our sensor array. Three human cell lines and two mouse cell lines with different organ origins and cell status were used: MCF 10A (human breast, non-tumorigenic cell), MCF-7 (human breast, tumorigenic cell), HeLa (human cervix, tumorigenic cell), 3T3 (mouse embryonic fibroblasts, non-tumorigenic cell), and 4T1 (mouse breast, tumorigenic cell). We tested these five cell types against the sensor array using six replicates, and the fluorescent patterns were shown in Fig. 4a. Linear discriminant analysis (LDA) is a robust classification method that can be used to find a linear combination of features that best separates two or more classes of object.51 After obtaining the fluorescent fingerprints of each cell lines, LDA was then used to observe whether the sensor array could discriminate each cell line based on their fluorescent signatures. The LDA plot revealed five distinct clusters with a correct classification of 100% (Fig. 4b, Tables S1 and S2†). These results indicate that our sensing platform can distinguish different cancer cell types with high sensitivity.
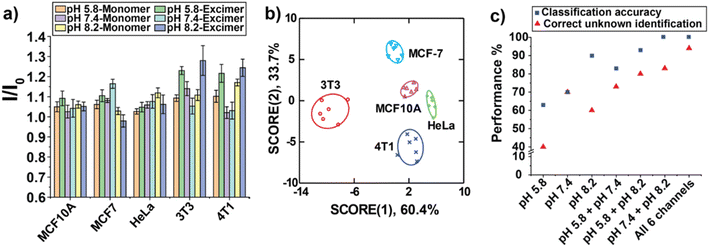 |
| Fig. 4 Discrimination of different cancer cell lines using PONI-BA-pyrene polymer. (a) Fluorescence intensities of sensor array outputs after 30 min incubation with each cell line, normalizing against sensor only (n = 6). (b) Fluorescence patterns were analysed using LDA and the first two canonical scores were plotted with 95% confidence ellipses shown. (c) Classification accuracy and correct unknown identification of cell types through different combinations of sensor channels. | |
Unknown identification was performed to determine the robustness and efficiency of our system for phenotyping cancer cells.52 A high percentage of correct unknown identification (93%) was obtained (Table S3†). Next, we determined the necessity for using all three pH conditions to generate six channels of information from the sensor. The performance of classification and unknown identification under individual pH and different combinations were compared. Fig. 4c showed that both of classification accuracy and correct unknown identification increased with increasing number of pH conditions. Significantly, while using “pH 7.4 + pH 8.2” the classification accuracy was 100%, the correct unknown identification was only 83%, indicating the importance of performing unknown sample identification for evaluating the performance of sensing platforms. Taken together, the above results confirmed the high sensitivity and reliability of our BA-based sensor array to differentiate cancer cell types phenotypically.
Discrimination of Chinese hamster ovary (CHO) glycosylation mutants
We next wanted to directly test the ability of our sensor array to detect glycosylation changes in cells. CHO glycosylation mutants have been particularly useful for glycosylation studies since their surfaces feature a range of different glycosaminoglycans (GAGs).53 We chose six GAG-mutated CHO cell lines as our analytes (Table 1), which were generated due to the deficiency of different enzymes that play important structural roles at the cell surface for the synthesis of GAGs.54,55 Upon incubation of sensor with GAG mutant cells cultured on the 96-well microplates for 30 min, fluorescent signals were measured at three pH values. The six-channel readout generated a unique fluorescence pattern for each cell type (Fig. 5a). LDA was further used to characterize the fluorescence responses (Fig. 5b). The six different mutants were clustered into six nonoverlapping groups with 100% correct classification (Tables S4 and S5†). 100% of correct unknown identification confirmed the high sensitivity, robustness and reproducibility of our sensor array for directly profiling cell surface glycosylation signatures (Table S6†). In addition, Fig. 5c demonstrated that the classification accuracies of “pH 7.4” channel and “pH 7.4 + pH 8.2” channel were also 100%, whereas their correct unknown identifications were very low, 31% and 64%, further indicating the necessity of using all three different pH values to generate multidimensional fluorescence response for more efficient unknown identification.
Table 1 CHO glycosylation mutants studied using PONI-BA-pyrene sensor array
CHO line |
Biochemical defect |
Cell status |
CCL-61 |
None |
Tumorigenic |
CRL-1735 |
N-Acetylglucosamine transferase deficient |
Tumorigenic |
CRL-1736 |
Sialic acid deficient |
Tumorigenic |
CRL-2241 |
Galactosyltransferase I deficient |
Tumorigenic |
CRL-2242 |
Xylosyltransferase I deficient |
Non-tumorigenic |
CRL-2244 |
Heparan sulfate deficient |
Non-tumorigenic |
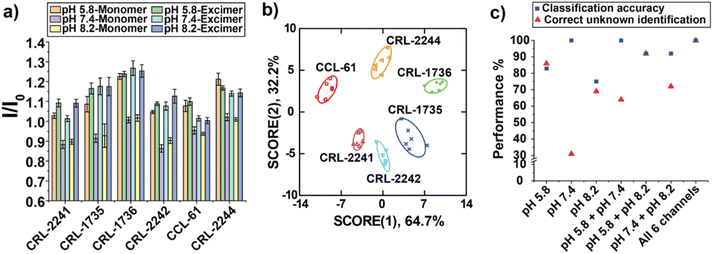 |
| Fig. 5 Discrimination of CHO glycosylation mutants using BA-based sensing platform. (a) Fluorescence intensities of PONI-BA-pyrene incubated with each CHO cell line. Each value is the average of six parallel measurements. (b) Canonical score plot for two factors of normalized fluorescence patterns obtained with 95% confidence ellipse. (c) Classification accuracy and correct unknown identification of CHO glycosylation mutants using different combinations of sensor channels. | |
Conclusions
In summary, boronic acid-functionalized PONI polymers provide a highly sensitive and robust multichannel polymeric sensor array for cell surface glycosylation-based discrimination. Due to the pH-responsive binding affinity between boronic acids with cell surface glycans, a six-channel high-content sensor array was generated from a single polymer using three representative pH values. This high-content sensor array accurately discriminated cancer cells with different organ origins and status. CHO cells with different glycosylation patterns were also effectively distinguished using the BA-based sensing platform, consistent with cell surface glycosylation being a primary driver of sensor response. Taken together, the ability of our sensing platform to rapidly distinguish cell surface glycan patterns provides access to new strategies for rapid diagnosis of glycan signature-based disease stage, and achieve the high-throughput screening of glycosylation-associated therapeutics.
Experimental section
Materials
All chemicals and solvents were purchased from Fisher Scientific or Sigma-Aldrich except where otherwise noted. For assays requiring absorbance and fluorescence measurements, a SpectraMax M2 plate reader was used (Molecular Devices, San Jose, CA).
PONI-BA-pyrene synthesis
The detailed synthesis and characterizations of PONI-BA-pyrene can be found in the ESI.†
Cell culture
Cell lines were purchased from American Type Culture Collection (ATCC, Manassas, VA) and were maintained according to the recommended guidelines. 3T3 and MCF-7 were cultured in Dulbecco's Modified Eagle Medium (DMEM) high glucose media with 10% fetal bovine serum (FBS) and 1% antibiotics. HeLa cells were cultured in DMEM low glucose media with 10% FBS and 1% antibiotics. 4T1 cells were cultured in RPMI-1640 medium supplemented with 10% FBS and 1% antibiotics at the same condition. MCF 10A cells were cultured in DMEM/F-12 medium supplemented with 5% horse serum, 20 ng mL−1 human epidermal growth factor, 500 ng mL−1 hydrocortisone, 100 ng mL−1 cholera toxin and 10 μg mL−1 insulin. CCL-61, CRL-2241, CRL-2242, and CRL-2244 cells were cultured in Ham's nutrient mixture F12 supplemented with FBS and 1% antibiotics. CRL-1735 and CRL-1736 cells were cultured in alpha minimum essential medium eagle supplemented with 10% FBS and 1% antibiotics. All the cell lines were maintained at 37 °C under a humidified atmosphere containing 5% CO2 and sub-cultured when reaching 80–90% confluence.
Array-based sensing procedures
The sensor was prepared by diluting PONI-BA-pyrene using 5 mM phosphate buffers with three different pH values (pH 5.8, pH 7.4 and pH 8.2) to the final concentration of 40 μg L−1. After 30 min incubation in dark, 150 μL of each sensor solution was added into 96-well plate with 104 cells in each well. After 30 min of incubation with different types of cells at 37 °C incubator, fluorescence intensities were recorded using the microplate reader at 25 °C. The fluorescence channels of the sensor were 330/398 nm (Pyrene monomer) and 330/473 nm (Pyrene excimer).
Linear discriminant analysis (LDA)
The fluorescence change (I/I0) patterns were subjected to linear discriminant analysis (LDA) using SYSTAT (version 13, Systat Software, Richmond, CA, U.S.A.) to classify cells with different states. LDA is a revised multivariate method used to find a linear combination of features that characterizes or separates two or more classes of objects. All variables were used in the complete mode and the tolerance was set as 0.001. The raw fluorescence response patterns were transformed to canonical patterns where the between-class variance was maximized while the within-class variance was minimized.51
Unknown identification
The identity of unknown cell groups was predicted by computing their Mahalanobis distance to the center of the training groups, followed by determining the probability of cells belonging to its closest cluster using an appropriate F-distribution for the minimum distance.52
Data availability
The data supporting the findings of this study are available within the article and in the ESI.†
Author contributions
M. Jiang and A. Chattopadhyay led the conceptualization, investigation and data analysis of the work and the writing of the original draft. C. Li, Y. Geng and R. Huang supported the conceptualization, investigation and data analysis of the work. D. C. Luther supported the writing of the original draft. V. M. Rotello helped the supervision of the work and led the review and editing of the draft.
Conflicts of interest
There are no conflicts to declare.
Acknowledgements
This research was supported by NIH (DK121351).
Notes and references
- J. Gu, T. Isaji, Q. Xu, Y. Kariya, W. Gu, T. Fukuda and Y. Du, Glycoconjugate J., 2012, 29, 599–607 CrossRef CAS PubMed.
- M. K. Hall, D. A. Weidner, S. Dayal and R. A. Schwalbe, FEBS Open Bio, 2014, 4, 892–897 CrossRef CAS PubMed.
- Y. Choi, J. Kim, J. Chae, J. Hong, J. Park, E. Jeong, H. Kim, M. Tanaka, M. Okochi and J. Choi, J. Controlled Release, 2022, 342, 321–336 CrossRef CAS PubMed.
- C. J. Day, E. N. Tran, E. A. Semchenko, G. Tram, L. E. Hartley-Tassell, P. S. K. Ng, R. M. King, R. Ulanovsky, S. McAtamney, M. A. Apicella, J. Tiralongo, R. Morona, V. Korolik and M. P. Jennings, Proc. Natl. Acad. Sci. U. S. A., 2015, 112, E7266–E7275 CrossRef CAS PubMed.
- D. Shi, A. Sheng and L. Chi, Front. Mol. Biosci., 2021, 8, 1–15 Search PubMed.
- J. D. Allen, Y. Watanabe, H. Chawla, M. L. Newby and M. Crispin, J. Mol. Biol., 2021, 433, 2–3 CrossRef PubMed.
- B. Adamczyk, T. Tharmalingam and P. M. Rudd, Biochim. Biophys. Acta, Gen. Subj., 2012, 1820, 1347–1353 CrossRef CAS PubMed.
- H. Jiang, B. P. English, R. B. Hazan, P. Wu and B. Ovryn, Angew. Chem., Int. Ed., 2015, 54, 1765–1769 CrossRef CAS PubMed.
- R. Gupta, F. Leon, S. Rauth, S. K. Batra and M. P. Ponnusamy, Cells, 2020, 9, 446 CrossRef CAS PubMed.
- A. F. Costa, D. Campos, C. A. Reis and C. Gomes, Trends Cancer, 2020, 6, 757–766 CrossRef CAS PubMed.
- J. Munkley and D. J. Elliott, OncoTargets Ther., 2016, 7, 35478–35489 CrossRef PubMed.
- A. Peixoto, M. Relvas-Santos, R. Azevedo, L. Lara Santos and J. A. Ferreira, Front. Oncol., 2019, 9, 1–24 CrossRef PubMed.
- J. Hofmann and K. Pagel, Angew. Chem., Int. Ed., 2017, 56, 8342–8349 CrossRef CAS PubMed.
- L. Veillon, Y. Huang, W. Peng, X. Dong, B. G. Cho and Y. Mechref, Electrophoresis, 2017, 38, 2100–2114 CrossRef CAS PubMed.
- Y. Zhang, Z. Hu, C. Zhang, B. F. Liu and X. Liu, Talanta, 2020, 219, 121356 CrossRef CAS PubMed.
- A. Messina, A. Palmigiano, F. Esposito, A. Fiumara, A. Bordugo, R. Barone, L. Sturiale, J. Jaeken and D. Garozzo, Glycoconjugate J., 2021, 38, 201–211 CrossRef CAS PubMed.
- C. L. Woodin, M. Maxon and H. Desaire, Analyst, 2013, 138, 2793–2803 RSC.
- R. Thompson, A. Creavin, M. O'Connell, B. O'Connor and P. Clarke, Anal. Biochem., 2011, 413, 114–122 CrossRef CAS PubMed.
- J. Wu, J. Zhu, H. Yin, R. J. Buckanovich and D. M. Lubman, J. Proteome Res., 2014, 13, 2197–2204 CrossRef CAS PubMed.
- L. Zhang, S. Luo and B. Zhang, mAbs, 2016, 8, 524–535 CrossRef CAS PubMed.
- M. P. Lenza, I. Oyenarte, T. Diercks, J. I. Quintana, A. Gimeno, H. Coelho, A. Diniz, F. Peccati, S. Delgado, A. Bosch, M. Valle, O. Millet, N. G. A. Abrescia, A. Palazón, F. Marcelo, G. Jiménez-Osés, J. Jiménez-Barbero, A. Ardá and J. Ereño-Orbea, Angew. Chem., Int. Ed., 2020, 59, 23763–23771 CrossRef CAS PubMed.
- M. Kimani, S. Beyer, Z. El-Schich, K. Gawlitza, A. Gjörloff-Wingren and K. Rurack, ACS Appl. Polym. Mater., 2021, 3, 2363–2373 CrossRef CAS.
- L. Pang, P. Li, H. He, S. Xu and Z. Liu, Chem. Sci., 2022, 13, 4589–4597 RSC.
- M. S. Hizir, N. M. Robertson, M. Balcioglu, E. Alp, M. Rana and M. V. Yigit, Chem. Sci., 2017, 8, 5735–5745 RSC.
- X. Wei, Y. Wang, Y. Zhao and Z. Chen, Biosens. Bioelectron., 2017, 97, 332–337 CrossRef CAS PubMed.
- X. Zhao, Y. Gao, J. Wang, Y. Zhan, X. Lu, S. Xu and X. Luo, Chem. Commun., 2020, 56, 13828–13831 RSC.
- R. L. Pérez, M. Cong, S. R. Vaughan, C. E. Ayala, W. I. S. Galpothdeniya, J. K. Mathaga and I. M. Warner, ACS Sens., 2020, 5, 2422–2429 CrossRef PubMed.
- J. Han, H. Cheng, B. Wang, M. S. Braun, X. Fan, M. Bender, W. Huang, C. Domhan, W. Mier, T. Lindner, K. Seehafer, M. Wink and U. H. F. Bunz, Angew. Chem., Int. Ed., 2017, 56, 15246–15251 CrossRef CAS PubMed.
- L. Zheng, P. Qi and D. Zhang, Sens. Actuators, B, 2019, 286, 206–213 CrossRef CAS.
- M. Yin, C. Jing, H. Li, Q. Deng and S. Wang, J. Nanobiotechnol., 2020, 18, 1–14 CrossRef PubMed.
- Y. Geng, A. N. Chattopadhyay, X. Zhang, M. Jiang, D. C. Luther, S. Gopalakrishnan and V. M. Rotello, Small, 2020, 2002084 CrossRef CAS PubMed.
- Y. Geng, J. Hardie, R. F. Landis, J. A. Mas-Rosario, A. N. Chattopadhyay, P. Keshri, J. Sun, E. M. Rizzo, S. Gopalakrishnan, M. E. Farkas and V. M. Rotello, Chem. Sci., 2020, 11, 8231–8239 RSC.
- M. Jiang, A. N. Chattopadhyay, Y. Geng and V. M. Rotello, Chem. Commun., 2022, 58, 2890–2893 RSC.
- M. Jiang, A. N. Chattopadhyay and V. M. Rotello, Int. J. Mol. Sci., 2022, 23, 3672 CrossRef CAS PubMed.
- K. D. Shimizu and C. J. Stephenson, Curr. Opin. Chem. Biol., 2010, 14, 743–750 CrossRef CAS PubMed.
- J. Tropp, M. H. Ihde, A. K. Williams, N. J. White, N. Eedugurala, N. C. Bell, J. D. Azoulay and M. Bonizzoni, Chem. Sci., 2019, 10, 10247–10255 RSC.
- S. Ngernpimai, Y. Geng, J. M. Makabenta, R. F. Landis, P. Keshri, A. Gupta, C. H. Li, A. Chompoosor and V. M. Rotello, ACS Appl. Mater. Interfaces, 2019, 11, 11202–11208 CrossRef CAS PubMed.
- A. Schiller, R. A. Wessling and B. Singaram, Angew. Chem., Int. Ed., 2007, 46, 6457–6459 CrossRef CAS PubMed.
- W. L. A. Brooks, C. C. Deng and B. S. Sumerlin, ACS Omega, 2018, 3, 17863–17870 CrossRef CAS PubMed.
- S. Chatterjee, E. V. Anslyn and A. Bandyopadhyay, Chem. Sci., 2021, 12, 1585–1599 RSC.
- J. Yan, G. Springsteen, S. Deeter and B. Wang, Tetrahedron, 2004, 60, 11205–11209 CrossRef CAS.
- W. L. A. Brooks and B. S. Sumerlin, Chem. Rev., 2016, 116, 1375–1397 CrossRef CAS PubMed.
- A. Matsumoto and Y. Miyahara, Sci. Technol. Adv. Mater., 2018, 19, 18–30 CrossRef CAS PubMed.
- A. E. Madkour, A. H. R. Koch, K. Lienkamp and G. N. Tew, Macromolecules, 2010, 43, 4557–4561 CrossRef CAS PubMed.
- A. Galstyan, R. Schiller and U. Dobrindt, Angew. Chem., Int. Ed., 2017, 56, 10362–10366 CrossRef CAS PubMed.
- Y. Nakagawa and Y. Ito, Adv. Carbohydr. Chem. Biochem., 2012, 68, 1–58 CrossRef CAS PubMed.
- S. H. Brewer, A. M. Allen, S. E. Lappig, T. L. Chasse, K. A. Briggman, C. B. German and S. Franzen, Langmuir, 2004, 20, 5512–5520 CrossRef CAS PubMed.
- F. Wang, Q. Liao, G. Xiang and S. Pan, J. Mol. Struct., 2014, 1060, 176–181 CrossRef CAS.
- L. Balachander, G. Ramadevudu, M. Shareefuddin, R. Sayanna and Y. C. Venudharc, ScienceAsia, 2013, 39, 278–283 CAS.
- Z. Liu and H. He, Acc. Chem. Res., 2017, 50, 2185–2193 CrossRef CAS PubMed.
- C. Moulin, C. Largeron, C. Ducottet, M. Géry and C. Barat, Pattern Recognit., 2014, 47, 260–269 CrossRef.
- S. Rana, N. D. B. Le, R. Mout, K. Saha, G. Y. Tonga, R. E. S. Bain, O. R. Miranda, C. M. Rotello and V. M. Rotello, Nat. Nanotechnol., 2015, 10, 65–69 CrossRef CAS PubMed.
- S. J. North, H. H. Huang, S. Sundaram, J. Jang-Lee, A. T. Etienne, A. Trollope, S. Chalabi, A. Dell, P. Stanley and S. M. Haslam, J. Biol. Chem., 2010, 285, 5759–5775 CrossRef CAS PubMed.
- S. K. Patnaik and P. Stanley, Methods Enzymol., 2006, 416, 159–182 CAS.
- J. Gottschalk and L. Elling, Curr. Opin. Chem. Biol., 2021, 61, 71–80 CrossRef CAS PubMed.
Footnotes |
† Electronic supplementary information (ESI) available. See DOI: https://doi.org/10.1039/d2sc02116a |
‡ M. Jiang and A. N. Chattopadhyay contributed equally to the work. |
|
This journal is © The Royal Society of Chemistry 2022 |
Click here to see how this site uses Cookies. View our privacy policy here.