The attitude to learning chemistry instrument (ALChI): linking sex, achievement, and attitudes
Received
3rd February 2022
, Accepted 23rd April 2022
First published on 25th April 2022
Abstract
Attitudes towards learning chemistry have been little studied in secondary school students, especially regarding dimensions related to problem solving, the molecular atomic perspective of chemistry, and real-world connection of chemistry. In the present study, we first aimed to design and assess the psychometric properties of the attitude to learning Chemistry instrument (ALChI). A second aim of the study was to assess sex differences and the relation between achievement and attitudes. Participants were 503 secondary school students, from a central southern region of Chile, who answered a 23-item version of the ALChI. The results of the exploratory factor analysis (EFA) revealed that an 18-item scale was most appropriate grouped into four factors: real world connection (RWC), perceived difficulty in problem-solving (PDPS), confidence in problem solving (CPS), and atomic molecular perspective of chemistry (AMPCh). Confirmatory factor analysis (CFA) confirmed the four-factor structure of the model, with optimal fit indexes. Sex factorial invariance was also obtained for configural, metric, and scalar models, demonstrating that ALChI was invariant for sex. Reliability analysis showed moderate to good indices, which suggests good internal consistency of ALChI. On a descriptive level, the highest mean was for the CPS subscale, which entails that students showed a favourable disposition towards their ability to succeed in solving chemistry problems. A structural equation multiple indicators multiple causes (MIMIC) model was conducted to compare factor latent means for sex and assess the relationship between achievement and attitude latent variables. These analyses revealed that achievement was positively associated with all four latent factors. However, no difference by sex was observed across all four factor means. These findings are partially in line with the results from previous studies in more developed countries where attitudes towards chemistry have been consistently linked to academic performance and male students tend to have more positive attitudes than their female counterpart. Our findings are of relevance for researchers, school teachers, and secondary school students since attitudes towards learning chemistry play an important role in choosing a future career path.
Introduction
One of the most important goals in science education is to stimulate positive attitudes towards science, due to the impact they have on the decision-making about students’ future careers (Caleon and Subramaniam, 2008; Harrison et al., 2011) and even in their professional future (Archer et al., 2015). Furthermore, attitudes are considered to be fundamental to educate citizens about sustainable development and the responsibility of their actions (Bybee and McCrae, 2011; Burmeister and Eilks, 2012). In the particular case of chemistry, it has been observed that students’ attitudes significantly influence academic performance (Ali and Awan, 2013; Kanafiah and Jumadi, 2013; Xu et al., 2013). Likewise, numerous studies across the developed world have found attitudes differentiated by sex, that is, male students have more positive attitudes towards chemistry than female students (Jenkins and Nelson, 2005; George, 2006; Sorge, 2007; Barmby et al., 2008; Desy et al., 2011; Potvin and Hasni, 2014). However, in the case of Latin American countries, studies have not shown differences by sex (Montes et al., 2018). This suggests that there may be contextual differences to consider when explaining these attitudes.
To date, attitudes towards chemistry have been studied in university (Dalgety et al., 2003; Grove and Bretz, 2007; Barbera et al., 2008; Bauer, 2008) and secondary school (Salta and Tzougraki, 2004; Cheung, 2009) students across cognitive (utility, effort, the importance of chemistry course, and evaluative beliefs about school chemistry) and affective dimensions such as liking for chemistry theory lessons and chemistry learning experiences. These attitudinal dimensions have made it possible to describe more general aspects involved in the process of learning chemistry. However, more specific learning chemistry subscales such as the value of chemistry in everyday life, the attitude in solving chemical problems, and the microscopic nature of matter have been scantily studied, and even less in Latin American students. It is relevant to consider these attitude objects in relation to educational context and to more specific learning experiences. In fact, it has been demonstrated that when the school curriculum is implemented in the absence of phenomena students experience in their daily life, attitudes towards learning chemistry are negatively affected (Holbrook, 2005; Hofstein and Mamlok-Naaman, 2011; O’Dwyer and Childs, 2014). Additionally, it has been noted that problem solving is an aspect that students do not approach with liking and often overwhelms them (Reid and Yang, 2002; Overton et al., 2013; Rodríguez et al., 2019), which explains their low performance in the discipline (Surif et al., 2014; Randles and Overton, 2015; Mackatiani, 2017; Aydin-Günbatar and Kalender, 2019) and their negative attitudes (Adesoji, 2008; Overton and Potter, 2011). Furthermore, comprehension of microscopic-level processes and phenomena is poor, especially in the case of secondary school students (Ayas and Özmen, 2002; Ayas et al., 2010; Zarkadis et al., 2017), despite being a requirement in learning chemistry (Johnstone, 1993; 2006; Pinarbasi and Canpolat, 2003). It has been proposed that the lack of capacity to think abstractly about the subject matter at a microscopic level causes rejection and lack of interest in chemistry (Harrison and Treagust, 2002). Given that context seems to play an important role in the factors that influence attitudes, and that there is little knowledge about attitudes towards learning chemistry in Latin America, in this study we address attitude towards learning chemistry linked to problem solving, the atomic-molecular perspective of the discipline, and the association of chemistry to daily life in secondary school students.
Attitude towards learning chemistry
The attitude towards learning chemistry, as in the work of Cheung (2009, 2011), is conceptualized under the latent process model (Oskamp and Schultz, 2005). This model provides a broader explanatory framework of attitudes towards chemistry, including factors linked to learning chemistry. From this approach, chemistry lessons that involve aspects of problem solving, microscopic nature of matter and the real-world connection can trigger cognitive, affective and/or behavioral processes in the students, many of which are not observable (Oskamp and Schultz, 2005). These processes can produce an attitude towards the objects involved in the stimulus situation and this attitude is an unobservable or latent construction (see Fig. 1). However, the presence of an attitude can be measured in terms of observable responses to cognitive, affective and/or behavioral reactions to the attitude object (Eagly and Chaiken, 2005; Fabrigar et al., 2005; Oskamp and Schultz, 2005). Affective attitudinal responses are the feelings and emotions a person has towards the attitudinal object, whereas the cognitive attitudinal responses refer to the evaluative beliefs that a person has about the attitude object. The behavioral attitudinal responses are not behaviours per se but are the action tendencies of the person towards the object of the attitude (Cheung, 2011). In synthesis, chemistry lessons that involve aspects of problem solving, the microscopic nature of matter, and the real-world connection trigger hidden processes within the student, and this information results in a general evaluative summary that ultimately constitutes an attitude.
Chemistry course design engages various challenges that arise from the very nature of the discipline. Students must understand that all matter around them is the result of innumerable interactions of atoms and molecules, entities that cannot be seen with the naked eye. Furthermore, chemical phenomena are explained through chemical reactions that often involve mathematical calculations. Unfortunately, these challenges tend to generate overloaded curricula and with emphasis on the conceptual development of subjects, to the detriment of affective factors linked to learning, such as attitudes. The evidence suggests that favourable predisposition, interest, pleasant feelings and having positive attitudes facilitate the task of learning (Lord et al., 2009). In this sense, there is the need to develop instruments that measure attitudes towards specific aspects of learning chemistry and not only general attitudes.
Sex differences, achievement, and attitudes towards chemistry
It has been demonstrated that attitudes towards chemistry are related to factors such as academic performance (Brandriet et al., 2011; Xu and Lewis, 2011; Xu et al., 2012; Ali and Awan, 2013; Kanafiah and Jumadi, 2013; Kahveci, 2015; Kousa et al., 2018; Montes et al., 2018) and sex (Salta and Tzougraki, 2004; Cousins, 2007; Cheung, 2009; Can, 2012). For example, in a study performed in secondary school students from four public schools in Turkey, a significantly positive correlation was found between attitudes towards chemistry and academic performance in the discipline. More specifically, students with more positive attitudes towards chemistry demonstrated a significantly higher performance in comparison to students with negative attitudes (Kahveci, 2015). In line with these findings, Vilia et al. (2017) performed a correlation analysis and multiple linear regressions to explain the relationship between attitudes towards chemistry and physics and achievement of ninth-grade students in Portugal. Vilia et al. (2017) used the Attitude toward physic-chemistry questionnaire (Neto et al., 2011), which consists of four sub-scales (positive emotions, negative emotions, competence, and utility) and 23 items. Their main results showed positive correlations between the three dimensions of the attitude scale and academic performance in physics and chemistry. Furthermore, in Chile, Montes et al. (2018) measured cognitive and affective components of attitudes towards chemistry with a semantic differential instrument based on the ASCI-V2 (Xu and Lewis, 2011). They showed that performance was positively associated with attitudes towards chemistry; cognitive attitudes were lower than affective ones and decreased at a higher rate over time. The researchers advised that it would be necessary to consider attitudes along with academic performance, so educators are informed of their students’ attitudes towards chemistry as part of the evaluation process. Emphasis should be placed specifically on negative attitudes that may affect learning making it less effective.
Concerning sex differences regarding attitudes towards chemistry, findings reported until now are not consistent across the different attitude objects that have been measured. Salta and Tzougraki (2004), for example, surveyed 576 secondary students in Greece using an attitude scale with four subscales: the difficulty of the chemistry course, the interest of the chemistry course, the usefulness of the chemistry course for their future career, and the importance of chemistry in their life. Salta and Tzougraki (2004) did not find sex differences regarding interest, utility, and importance of chemistry, but they did find that girls tend to express negative attitudes towards the difficulty of the chemistry course. Nonetheless, Cheung (2009) observed that Japanese boys between 16 and 17 years of age manifest a higher liking towards chemistry theory classes in comparison with their female counterparts. However, by the time they are 18, girls show significantly higher attitudes than boys in this dimension, with sex differences disappearing a year later. Likewise, in Australia, Cousins (2007) informed no differences in academic performance between males and females, but the latter reported feeling insecure about their ability to stand out in the discipline and showed less interest in enrolling on chemistry courses. In synthesis, the evidence seems to show that female students have lower perceptions of competence and success expectations than that of their male peers, which can result in unfavourable attitudes towards chemistry.
In the case of Latin American countries, studies regarding attitudes towards chemistry are generally very limited (Molina et al., 2011) and only one of them explored sex differences (Montes et al., 2018). Molina et al. (2011) studied university students in Colombia, demonstrating that students found chemistry interesting and useful, but difficult to study. They did not examine sex differences or academic performance. To the best of our knowledge, the only study about attitudes towards chemistry in secondary school students in Latin America is that of Montes et al. (2018). This work is also the first in Latin America to evaluate the role of sex in attitudes towards chemistry. Montes et al. (2018) measured cognitive and affective attitudes towards chemistry in 523 secondary school students and did not observe sex differences in any of these dimensions, which contrasts heavily with most evidence reporting more positive attitudes towards chemistry for male students (e.g., Cheung, 2009; Bybee and McCrae, 2011). While their study is conclusive regarding the non-existence of sex differences, it only measured general dimensions of chemistry; therefore, the effect of sex on specific dimensions of learning chemistry in Chilean students and Latin American students, in general, remains unknown. In particular, it is unknown in these contexts if there are sex differences in dimensions such as problem-solving, the atomic-molecular perspective of the discipline, and the association of chemistry with the environment.
Considering the effect of sex on the above dimensions is relevant because there are substantial sex differences regarding the pursuit of STEM degrees in Chile (Bordón et al., 2020). In particular, female students apply far more to health science than engineering and technology degrees, where male students take the lead. It is possible that these differences stem from their secondary school experience, as has been the case in other countries (Harrison et al., 2011; Riegle-Crumb and Peng, 2021). In synthesis, research on the role of sex in attitudes towards chemistry shows that studies using scales linked to learning chemistry are more frequently reported in developed countries (e.g., Salta and Tzougraki, 2004; Cousins, 2007; Cheung, 2009), where men demonstrate more favourable attitudes than women. Meanwhile, in less developed countries, it is not clear how male and female students value chemistry in aspects linked to their learning (e.g., Can, 2012). Therefore, it is necessary to carry out more research to better understand sex differences in attitudes towards chemistry learning, particularly among secondary school students, who face the daunting task of deciding a future career path.
The present study
The present study had two aims: (1) to design and assess the psychometric properties of the attitude to learning Chemistry instrument (ALChI) in a sample of secondary school students and (2) to assess sex differences and the relation between achievement and attitudes. To the best of our knowledge, there are still no previous instruments to measure attitudes towards learning chemistry in secondary students in Latin America. We used the Colorado Learning Attitudes about Science Survey for Use in Chemistry (CLASS-Chem) by Barbera et al. (2008) as a reference for the selection of items and factors relevant to secondary school students. The CLASS-Chem was based on a previous work created for use in physics and designed to approach a variety of subjects that educators consider important in the process of learning physics in university students (Adams et al., 2006). We report reliability and validity using exploratory factor analysis (EFA) and confirmatory factor analysis (CFA). We also report factorial invariance across sex to make sure that the instrument could be used to measure attitudes in male and female students. By means of a structural equation model, we then examine sex differences in attitudes towards learning chemistry and the relationship between achievement and attitude factors.
Method
Participants
Participants in this study were 503 secondary school students: 261 girls and 242 boys between 15 and 18 years of age, who were from 10th to 13th grade at four secondary schools located in a central southern region of Chile.
The attitude to learning chemistry instrument (ALChI)
Carefully selected items from the Colorado Learning Attitudes about Science Survey for Use in Chemistry or CLASS-Chem (Barbera et al., 2008) were used in the design of the ALChI. The original instrument consists of 9 dimensions and 50 items designed for university students. In turn, this scale was based on the Colorado Learning Attitudes about Science Survey (CLASS), originally designed for use in physics (CLASS-Phis) (Adams et al., 2006). In order to validate the instrument for use in chemistry, the authors interviewed students and surveyed chemistry faculty members from several universities. They examined face validity and reliability of the instrument. They also employed an appropriate methodology for determining robustness, resulting in the labelling of distinct scales within the instrument. Heredia and Lewis (2012) carried out a psychometric evaluation of the instrument developed by Barbera et al. (2008) and found that each of the 9 categories proposed showed good model fit. However, this structure could not be confirmed in a CFA because it lacked discriminant validity. Heredia and Lewis then ran a CFA only on the scales that did not have repeated items: (1) personal interest; (4) problem solving: confidence; (7) conceptual connections; and (9) atomic-molecular perspective of chemistry, leading to a high correlation between problem solving: confidence and conceptual connections of 0.930. In light of those results, they ended up with a three-factor model and 16 items. In our study, we took a different approach to that of Heredia and Lewis (2012) but acknowledging the assessment they performed. We wanted to use scales and items from the original CLASS-CHEM to measure attitudes towards learning chemistry in secondary school students. Given the characteristics of our sample, we only selected items that were relevant for secondary school students and matched chemistry contents endorsed by the National School Curriculum for this level (Mineduc, 2009; 2019). The original instrument by Barbera et al. (2008) had items that were repeated across different scales, so we first focused on items from the scales that were confirmed in the work by Heredia and Lewis (2012). These were personal interest, problem solving: confidence, and atomic molecular perspective of chemistry. Additionally, we included items from personal interest, real-world connection, and other problem-solving scales since they also fit the contents relevant for secondary school students in Chile. In total, we selected 23 items. During the selection of items, we considered that secondary school students usually have trouble visualising abstract micro-phenomena because of the inherent complexity of chemistry concepts. In fact, research has shown that abstraction, low imaginative power, and underrepresentation are some of the factors responsible for poor performance in chemistry in both secondary school and higher education (Harle and Towns, 2011; Dries et al., 2016). These factors are also correlated with poor attitudes towards learning chemistry (Chua and Karpudewan, 2017). Hence, some items preliminarily classified under the atomic molecular perspective of chemistry were deemed very relevant (2, 11, 17, 29, and 33). All items from Personal Interest (4, 13, 16, 28, 34, and 36) and two from Real-World Connection (41, 43) were also selected given that they fit within the framework of school chemistry. Measuring attitudes towards chemistry that involve personal interest and real-world connection is useful because they involved information about meaningful and transferable scientific construction (Sanmarti Puig and Márquez Bargalló, 2017). Finally, a number of items from Problem Solving: General and Problem solving: Confidence were included (15, 18, 19, 21, 28, 30, 40, 47, 49, 50). Problem solving occupies a predominant place in science learning activities (Gómez-Ferragud et al., 2015; Martínez-Aznar et al., 2017). Schoenfeld (1983) was one of the first researchers who glimpsed that problem solving cannot be seen solely from an exclusively cognitive perspective and proposed the existence of a belief system, which includes attitudes that guide the actions carried out during problem solving. In particular, attitudes related to problem solving can be divided into will, perseverance and self-confidence (Lester et al., 1989; Zakaria and Yusoff, 2009; Zakaria and Ngah, 2011). Perseverance is defined as the voluntary pursuit of goal-oriented behaviour, even if this involves overcoming obstacles, difficulties, and disappointments (Peterson and Seligman, 2004). Confidence is an individual's belief in their ability to successfully solve even challenging problems, as well as an individual's belief in their own competence relative to their peers (Lester et al., 1989).
After all the items were selected, the ALChI was translated into Spanish by two language teaching experts fluent in both English and Spanish, using parallel translation. One of the authors, a Chemistry teacher, participated in the final form of the instrument to make sure the questions were clear and unambiguous to secondary school students.
Data collection
After receiving approval from the Universidad Católica de la Santísima Concepción (UCSC) Ethics Committee, we sent each school an email with a letter as an attachment in order to inform them about the purpose of the study and the data collection procedure. In the meantime, we prepared the consent and assent forms for parents and children to sign, respectively. Both documents clearly stated that participation was voluntary and no personally identifiable information was required from the students. Contact information about the researchers involved in the study and UCSC Ethics Committee was provided in the case of queries from parents or students. The data was collected in May (mid-term in Chile) at a time when there were no evaluations that could interfere with the survey. Before giving out copies of the instrument, we made sure that both the parents and students had signed their consent and assent forms, respectively. Participants were then asked to fill in the demographic survey including age, sex (male/female), and type of school they attended. Finally, they completed a paper-and-pencil version of the instrument in their usual classroom during a regular class. The entire data collection procedure took around 15 minutes on average.
Data analysis
The data were first coded and analysed for internal consistency and validity. R software (R Core Team, 2020) was used to process all data including descriptive statistics, internal consistency, construct validity, and structural equation model testing. In the first stage, we divided the sample randomly into two. The first half (n = 251) was used to conduct an exploratory factor analysis (EFA) using the principal axis method. Factors extracted were rotated using direct oblimin rotation. In the second stage, confirmatory factor analysis (CFA) was conducted on the other half of the sample (n = 252). Given that the collected data were derived from a five-point Likert scale, it was treated as ordinal data rather than continuous. In a factor analysis framework, this type of data is best modelled by using a robust, diagonally weighted least squares estimator, such as WLSMV (Finney and DiStefano, 2013). The recommendations for the values of the fit index with the WLSMV estimator are CFI ≥ 0.95 and RMSEA ≤ 0.05. SRMR is not recommended with the WLSMV estimator (Beauducel and Herzberg, 2006; DiStefano and Morgan, 2014).
After running the CFA, the estimated factor scores were extracted for use in subsequent analyses. In the third stage, as a reliability measure, the omega coefficient (ωh) was used (Komperda et al., 2018) instead of Cronbach's alpha coefficient since the items of our model were not tau-equivalent (McDonald, 1999; Cho, 2016). Then measurement invariance testing was performed for the configural, metric, and scalar models comparing female and male students. Establishing factorial invariance (FI) is an important step in demonstrating validity of an instrument (Ventura-León, 2017; Rocabado et al., 2019). Thus, verifying that measurement properties of the instruments are independent from the characteristics of the groups being compared, but not from the construct being measured, is an indispensable prerequisite for comparing groups with regard to a latent variable (Byrne, 2008; Rocabado et al., 2020). Each model (configural, metric, and scalar) adds a restriction to prove if the groups being compared share a similar measurement model and whether comparisons can be supported. Therefore, beside assessing the fit of each model of the invariance test, we also calculated and assessed the change in the fit of the data model between nested models. The X2 test statistic is commonly used, however, it has been shown to be very sensitive to sample size (Tomarken and Waller, 2003). Therefore, we also assessed model fit based on the following fit index cut-off points: ΔCFI (<0.01) and ΔRMSEA (<0.015) (Chen, 2007).
Once measurement invariant was achieved, a structural equation multiple indicators multiple causes (MIMIC) model (Finch and French, 2015) was conducted in order to compare attitude latent means for sex and assess the relationship between achievement (average of students’ Chemistry marks from the previous year) and attitude latent variables.
Results
Descriptive statistics
Descriptive statistics for the entire sample (n = 503) were obtained for each item score. Items Q05, Q10, Q23, and Q24 were negatively stated. Therefore, these four items were recoded in this analysis for ease of interpretation. The average score for each item ranged from 2.17 to 3.87, with standard deviation values ranging from 0.88 to 1.25. No item was found to have skewness and kurtosis with a magnitude larger than 1, which suggests normality of the data (see Table 1).
Table 1 Descriptive statistics for all preliminary ALChI items
Items |
Mean |
SD |
Skewness |
Kurtosis |
Q01 |
3.183 |
1.025 |
−0.227 |
−0.190 |
Q02 |
2.757 |
1.245 |
0.126 |
−0.954 |
Q03 |
2.875 |
1.102 |
−0.065 |
−0.556 |
Q04 |
2.169 |
1.059 |
0.811 |
0.158 |
Q05 |
3.139 |
1.143 |
−0.098 |
−0.717 |
Q06 |
2.952 |
1.171 |
−0.056 |
−0.757 |
Q07 |
3.740 |
0.944 |
−0.702 |
0.466 |
Q08 |
3.728 |
1.104 |
−0.730 |
−0.141 |
Q09 |
3.871 |
1.138 |
−0.900 |
0.106 |
Q10 |
3.280 |
1.105 |
−0.207 |
−0.683 |
Q11 |
2.481 |
1.189 |
−0.026 |
−0.800 |
Q12 |
2.811 |
1.118 |
0.060 |
−0.659 |
Q13 |
3.451 |
0.935 |
−0.306 |
−0.055 |
Q14 |
3.483 |
0.883 |
−0.497 |
0.521 |
Q15 |
3.294 |
1.178 |
−0.389 |
−0.638 |
Q16 |
3.294 |
1.128 |
−0.346 |
−0.606 |
Q17 |
3.384 |
1.023 |
−0.418 |
−0.297 |
Q18 |
3.247 |
1.185 |
−0.314 |
−0.676 |
Q19 |
2.688 |
1.147 |
0.202 |
−0.745 |
Q21 |
3.219 |
0.953 |
−0.435 |
0.141 |
Q22 |
3.517 |
1.025 |
−0.408 |
−0.269 |
Q23 |
3.561 |
1.086 |
−0.569 |
−0.297 |
Q24 |
3.340 |
1.173 |
−0.373 |
−0.667 |
Exploratory factor analysis
In the first stage of the exploratory factor analysis (EFA), a correlation matrix was generated. Then, Bartlett's test was applied, which resulted to be highly significant (p < 0.001), confirming that the items in the questionnaire were related and were not independent from one another (Field et al., 2012). The KMO test was used next, yielding an overall result of 0.87. After evaluating the eigenvalues, scree plot, and parallel analysis, a first EFA of the 23 items selected was carried out. In line with all objective indexes, four factors were extracted. After examining factor loadings in this first instance, we proceeded to eliminate those items that had very low loadings (below 0.35) and/or presented cross loadings: Q04, Q07, Q11, Q13 and Q21. A rule of thumb for factor loadings is that loadings <0.40 are considered weak while those ≥0.60 are considered strong (Garson, 2010).
After eliminating the said items, a new EFA was performed on the remaining 18 items with oblimin rotation, ensuring that every item had factor loadings above 0.35 and that there were no items with factor loadings above this value in more than one factor.
Confirmatory factor analysis
After specifying the structure of the model with four factors based on the EFA and on relevant theory, the goodness of fit of this four-factor, 18-item model was tested. The results of the confirmatory factor analysis (CFA) yielded optimal fit indexes χ2 (n = 252, df = 129, p < 0.01) = 200.35; CFI = 0.98; RMSEA = 0.05. Therefore, the final instrument, the Attitude to learning Chemistry (ALChI), ended up consisting of 18 items and four factors that we named as follows: Real-World connection (RWC), Perceived difficulty in problem-solving (PDPS), Confidence in problem solving (CPS), and Atomic molecular perspective of chemistry (AMPCh). See Table 2 for the English version and Appendix 1 for the Spanish version of the ALChI.
Table 2 Attitude to learning Chemistry (ALChI)'s factors and items
Real-world connection (RWC) |
Perceived difficulty in problem-solving (PDPS) |
Confidence in problem solving (CPS) |
Atomic molecular perspective of chemistry (AMPCh) |
Q02: I think about the chemistry I experience in everyday life. |
Q05: I do not expect equations to help my understanding of the ideas in chemistry; they are just for doing calculations. |
Q08: If I get stuck on a chemistry problem on my first try, I usually try to figure out a different way that works. |
Q01: To understand a chemical reaction, I think about the interactions between atoms and molecules. |
|
Q06: I study chemistry to learn knowledge that will be useful in my life outside of school. |
Q10: Why chemicals react the way they do does not usually make sense to me; I just memorize what happens. |
Q09: Nearly everyone is capable of understanding chemistry if they work at it. |
Q03: I think about how the atoms are arranged in a molecule to help my understanding of its behaviour in chemical reactions. |
|
Q15: Learning chemistry changes my ideas about how the world works. |
Q23: If I get stuck on a chemistry problem, there is no chance I'll figure it out on my own. |
Q17: I can usually figure out a way to solve chemistry problems. |
Q12: When I see a chemical formula, I try to picture how the atoms are arranged and connected. |
|
Q16: Reasoning skills used to understand chemistry can be helpful to me in my everyday life. |
Q24: When I'm solving chemistry problems, I often don't really understand what I am doing. |
Q22: When studying chemistry, I relate the important information to what I already know rather than just memorizing it the way it is presented. |
Q14: The arrangement of the atoms in a molecule determines its behaviour in chemical reactions. |
Q18: The subject of chemistry has relation to what I experience in the real world. |
|
Q19: To understand chemistry, I sometimes think about my personal experiences and relate them to the topic being analysed. |
|
|
|
Measurement invariance by sex
Given that the CFA yielded a well-defined four-factor structure, configural, metric, and scalar invariance by sex was undertaken. Fit indexes obtained for the configural model showed that the general structure of items and factors was similar across males and females, so configural invariance between groups was well-established. Metric invariance testing followed. This step involved testing whether the linear relationship between items and factors was similar across groups (Gregorich, 2006). The results indicated that the fit statistics for this model were within the cutoffs (ΔCFI ≤ 0.01 and ΔRMSEA ≤ 0.015) (Chen, 2007), so the metric invariance model was accepted. Finally, the scalar invariance model was evaluated, incorporating unstandardised equal intercepts, in addition to equal loadings across female and male groups. The results showed that differences in fit statistics for metric and scalar invariance models were acceptable (ΔCFI = 0.003 and ΔRMSEA = 0.002), so the hypothesis that the intercepts are invariant between both groups was confirmed. Fit indexes for configural, metric and scalar invariance models are presented in Table 3.
Table 3 Sex invariance adjustment indices for the ALChI (N = 252)
Model |
χ
2
|
df |
p
|
CFI |
TLI |
RMSEA |
Δχ2(p) |
ΔCFI |
ΔRMSEA |
Note: the chi-square difference test was not used due to its sensitivity to minor parameter changes in large samples. |
Configural |
384.955 |
258 |
<0.001 |
0.955 |
0.946 |
0.063 |
— |
— |
— |
Metric |
395.624 |
272 |
<0.001 |
0.956 |
0.950 |
0.060 |
|
0.001 |
0.003 |
Scalar |
436.032 |
322 |
<0.001 |
0.959 |
0.961 |
0.053 |
|
0.011 |
0.007 |
Reliability analysis
The reliability for each factor was calculated using McDonald's Omega. The indices for each of the factors was as follows: RWC = 0.87; PDPS = 0.66; CPS = 0.70; AMPCh = 0.67. These indices are considered moderate to good since each dimension contains items directed to the same underlying construct (Trizano-Hermosilla and Alvarado, 2016), which demonstrate good internal consistency of the ALChI.
Structural equation multiple indicators multiple causes (MIMIC) model for attitude, sex, and achievement
After gathering validity evidence for the internal structure of the ALChI, we compared attitude latent means for sex and assessed the relationship between achievement and attitude latent variables (RWC, PDPS, CPS, and AMPCh). All fit results for the model were very good, χ2 (n = 252, df = 157, p < 0.0001) = 234.471; CFI = 0.987; TLI = 0.988; RMSEA = 0.044; and SRMR = 0.061. Each item loaded significantly in their respective factors with most loadings ranging from 0.45 to 0.82. Item 5 was the exception displaying a factorial loading <0.30. A significant and moderate correlation (r > 0.42) was also observed between the four ALChI scales. All standardized path coefficients between achievement and attitude latent variables were significant; however, none of the four latent factors showed significant sex differences. The standardized parameter estimates from the model are shown in Fig. 2.
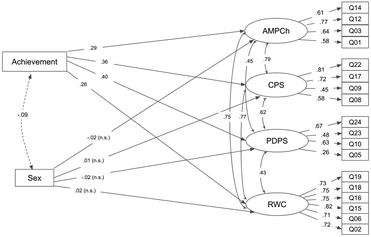 |
| Fig. 2 MIMIC model for attitude, sex, and achievement. | |
Discussion
The aims of the present study were to design and assess the psychometric properties of the Attitude to Learning Chemistry Instrument (ALChI) for use in secondary school students, and to measure students’ attitudes towards learning chemistry and their relationship with sex and academic performance. For this purpose, the ALChI, a new instrument based on CLASS-Chem scale (Barbera et al., 2008) was created and assessed psychometrically. The EFA yielded four dimensions and 18 items, which we named: Real-World Connection (RWC), Perceived difficulty in problem solving (PDPS), Confidence in problem solving: (CPS), and atomic molecular perspective of chemistry (AMPCh). The CFA confirmed the four-factor structure of the Class-Chem for secondary school students. In addition, the instrument was successfully tested for sex invariance, so it is appropriate to measure attitudes towards learning chemistry across male and female students. The factors that emerged after the EFA were similar to those expected and were part of the original instrument by Barbera et al. (2008). Personal Interest and Real-World Connection (originally 8 items) were grouped into a single factor that we labelled Real-World Connection (RWC) (6 items). Atomic Molecular Perspective of Chemistry was reduced from 6 to 4 items, so we kept the original name since it did not include new items. General Problem Solving was divided into two subscales which we named Perceived Difficulty in Problem Solving and Confidence in Problem Solving. This is aligned with the framework of attitudes towards problem solving described previously in the Methodology section (Lester et al., 1989; Zakaria and Yusoff, 2009; Zakaria and Ngah, 2011). The Perceived Difficulty in Problem Solving items denote a characteristic of perseverance as soon as the student opts for a behaviour that, given the difficulties and/or disappointments, does not decide to persist (Peterson and Seligman, 2004). The Confidence in Problem Solving factor corresponds to an individual's belief in their ability to successfully solve even challenging problems, as well as an individual's belief in their own competence with respect to their peers (Lester et al., 1989).
Descriptive data showed that the highest mean was for the CPS subscale, which revealed that students showed a favourable disposition towards their ability to succeed in solving chemistry problems. This conforms to the study by Navarro and Förster (2012), who measured attitudes towards science in 15-year-old Chilean students and obtained similar results in their dimension called ‘scientific attitude adoption’. Both dimensions can be compared since they evaluate aspects of the students’ performance facing activities that have to do with science. This suggests a similar pattern in Chilean secondary school students in both studies. The subscale with the lowest mean was AMPCh, which indicates that secondary school students do not find it very useful to think about the microscopic nature of matter when they learn chemistry. As it is known, processes and phenomena addressed by chemistry inherently arrive to microscopic-level explanations; therefore, if students do not have positive attitudes towards this specific dimension of chemistry, they might be likely to manifest rejection, apathy, and disinterest towards learning chemistry.
The results of the MIMIC model revealed no sex differences across all four attitude factors, but a significant association between achievement and each of the latent variables. Concerning academic performance, our results matched previous studies that observed a relationship between academic performance and attitudes towards chemistry, where dimensions like competence and utility (Vilia et al., 2017), task value and expectation of success (Else-Quest et al., 2013), and importance of discipline and personal relevance (Nieswandt, 2007) were measured.
The fact that achievement was positively related to each of the attitude factors means that low-achievement students had more negative attitudes towards learning chemistry than their high-achievement peers. Kousa et al. (2018) observed something similar when measuring attitudes towards chemistry in 15-year-old Finnish students. Kousa et al. (2018) also informed that students with low academic performance found chemistry more difficult than their high-performance peers, and thought chemistry was not useful (cognitive dimension) for their future. Our results also matched those obtained by Dierks et al. (2016), who reported that 12- to-19-year-old German students with high academic performance showed higher interest towards science than students with low academic performance, specifically towards activities that link science with intellectual tasks or practical tasks related to the environment. Several authors have argued that students with positive attitude towards science use more learning strategies than those with negative attitudes (Farooq et al., 2011; Awang et al., 2013). This implies that students with a favourable or positive attitude towards class contents or science courses are more likely to obtain better results because they are more likely to persist and are more willing to read and learn about subjects that interest them than those students with less favourable attitudes.
Regarding the lack of differences between female and male attitudes towards learning chemistry in these subscales, our results contrast with those of more developed countries, whose students show sex differences in subscales that measure specific attitude objects of chemistry (e.g., Salta and Tzougraki, 2004; Cheung, 2009; Can, 2012). For instance, Cousins (2007) reported that Australian secondary school females were not comfortable with the mathematical calculations that chemistry problems involved, and they felt insecure about their ability to stand out academically in the course. In contrast to females, males perceived chemistry as a ‘manly’ subject, and more males chose higher level chemistry because it offered them a challenge and because the subject matter seen in class interested them. Studies in Latin America about attitudes towards science (Molina et al., 2013) or chemistry alone (Montes et al., 2018) are very few, but have been consistent in showing no sex differences in attitude. For instance, Molina et al. (2013) researched attitudes towards science in students aged 10 to 18 in Colombia and did not find sex differences in the ‘learning science in school’ subscale. Likewise, Montes et al. (2018) in Chile failed to find significant differences between male and female secondary school students for either cognitive or affective attitudes towards chemistry. Our results extend previous findings in Latin America to attitudes towards the learning of chemistry and are consistent in showing no sex differences. This suggests that attitude towards learning chemistry and science are modulated by contextual factors, with less developed (Latin American) countries displaying no sex differences, whereas more developed countries showed more positive attitudes for males than females. It is worth noting that attitudes towards learning chemistry had not been previously studied in Latin American secondary school students and are linked to the cognitive domain of attitudes. Our findings provide information on the role these attitudinal dimensions may play not even in secondary school but also in post-secondary school academic pathways involving chemistry. If students do not value learning chemistry (e.g., problem-solving, real-world connection, and atomic molecular perspective), they may develop intentions of not pursuing studies related to science in higher education (Ardura and Pérez-Bitrián 2018).
Conclusions and implications for instruction
The present study provides validity evidence for the ALChI, which measures attitudes towards learning chemistry across male and female secondary school students. It is a short four-factor scale which can be easily used by both secondary school teachers and researchers. We replicated the strong positive link between achievement and attitudes towards chemistry found earlier and extended these findings to other subscales associated with the learning of chemistry. While sex differences in attitudes are consistently absent in Latin American students, low attitudes towards learning chemistry seem to be an issue for low-achievement students. This should be approached efficiently in the classroom since it can impact future student career choices. We encourage teachers to search for opportunities to develop research projects in small groups, where students could develop problem solving and scientific reasoning skills. Research shows these strategies have an impact on academic performance (Sababha et al., 2016) and on attitudes towards learning chemistry (Chase et al., 2013). Furthermore, teachers can use molecular visualisation software to represent molecules’ shape and size, as well as their interactions, thus helping students in their transition towards the particulate nature of matter (e.g., Pymol, Schrodinger, 2012). So far interventions both in university and secondary school students have proven effective in impacting students’ performance and attitudes (Bethel and Lieberman, 2014; Lazarowitz and Naim, 2013; Abualia et al., 2016). Finally, we recommend engaging students in activities outside the classroom; for instance, visits to parks, museums, or industries, so they can associate chemistry concepts with daily activities and familiar environments. The evidence is clear in pointing out that these events can foster positive attitudes towards chemistry (Hofstein and Mamlok-Naaman, 2011; O’Dwyer and Childs, 2014).
Author contributions
Lilian H. Montes, conceptualization, data curation, formal analysis, investigation, methodology, validation, visualization, writing – original draft. Roberto A. Ferreira, conceptualization, data curation, formal analysis, funding acquisition, methodology, project administration, resources, supervision, validation, visualization, writing – original draft, writing – review & editing. Cristina Rodriguez, conceptualization, data curation, formal analysis, methodology, project administration, supervision, validation, writing – review & editing.
Conflicts of interest
There are no conflicts to declare.
Appendix
Conexión con el mundo real |
Dificultad percibida en la resolución de problemas |
Confianza en la resolución de problemas |
Perspectiva atómica molecular de la química |
Q02: Pienso sobre la química en situaciones de la vida cotidiana. |
Q05: No espero que las ecuaciones ayuden a mi comprensión de las ideas de química; tan sólo son para hacer cálculos. |
Q08: Si me quedo bloqueado en un problema de química en mi primer intento, por lo general trato de encontrar una manera diferente que funcione |
Q01: Para entender una reacción química, pienso en las interacciones entre átomos y moléculas. |
|
Q06: Yo estudio química para aprender el conocimiento que será útil en mi vida fuera de la escuela. |
Q10: El por qué los productos químicos reaccionan de la manera que lo hacen por lo general no tiene sentido para mí; simplemente memorizo lo que sucede. |
Q09: Casi todo el mundo es capaz de entender la química si hacen un esfuerzo |
Q03: Pienso en cómo los átomos están dispuestos en una molécula para ayudar a mi comprensión de su comportamiento en las reacciones químicas. |
|
Q15: Aprender química cambia mis ideas acerca de cómo funciona el mundo. |
Q23: Si me quedo bloqueado en un problema de química, no hay posibilidad de que lo averigüe por mi cuenta. |
Q17: Por lo general puedo encontrar una manera de resolver problemas de química. |
Q12: Cuando veo una fórmula química, trato de imaginar cómo están organizados y conectados los átomos. |
|
Q16: Las habilidades de razonamiento utilizadas para comprender la química pueden ser útiles para mí en mi vida cotidiana. |
Q24: Cuando estoy resolviendo un problema de química, a menudo no entiendo lo que estoy haciendo. |
Q22: Cuando estudio química, relaciono la información importante con lo que ya sé, en vez de sólo memorizarla en la forma en que se presenta. |
Q14: La organización de los átomos en una molécula determina su comportamiento en las reacciones químicas. |
|
Q18: La asignatura de química tiene relación con lo que vivo en el mundo real. |
|
|
|
|
Q19: Para comprender la química, a veces pienso sobre mis experiencias personales y las relaciono con el tema que se está analizando. |
|
|
|
References
- Abualia M., Schroeder L., Garcia M., Daubenmire P. L., Wink D. J. and Clark G. A., (2016), Connecting protein structure to intermolecular interactions: a computer modeling laboratory, J. Chem. Educ., 93(8), 1353–1363.
- Adams W. K., Perkins K. K., Podolefsky N. S., Dubson M., Finkelstein N. D. and Wieman C. E., (2006), New instrument for measuring student beliefs about physics and learning physics: The Colorado Learning Attitudes about Science Survey, Phys. Rev. Special Top.-Phys. Educ. Res., 2(1), 1–14.
- Adesoji F. A., (2008), Managing students’ attitude towards science through problem–solving instructional strategy, Anthropologist, 10(1), 21–24.
- Ali S. and Awan S., (2013), Attitude towards science and its relationship with students' achievement in science, Interdiscip. J. Contemp. Res. Bus., 4(10), 707–718.
- Archer L., Dewitt J. and Osborne J., (2015), Is science for us? Black students’ and parents’ views of science and science careers, Sci. Educ., 99(2), 199–237.
- Ardura D. and Pérez-Bitrián A., (2018), The effect of motivation on the choice of chemistry in secondary schools: Adaptation and validation of the science motivation questionnaire II to Spanish students, Chem. Educ. Res. Pract., 19(3), 905–918.
- Awang M. M., Ahmad A. R., Bakar N. A. A., Ghani S. A., Yunus A. N. M., Ibrahim M. A. H. and Rahman M. J. A., (2013), Students' Attitudes and Their Academic Performance in Nationhood Education, Int. Educ. Stud., 6(11), 21–28.
- Ayas A. and Özmen H., (2002), A study of students’ level of understanding of the particulate nature of matter at secondary school level, Bogazici Univ. J. Educ., 19, 45–60.
- Ayas A., Özmen H. and Çalik M., (2010), Students’ Conceptions of the Particulate Nature of Matter at Secondary and Tertiary Level, Int. J. Sci. Math. Educ., 8(1), 165–184.
- Aydin-Günbatar S. and Kalender N., (2019), Comparison of Learners' Problem Solving Approaches and Success in Stoichiometry, Sci. Educ. Int., 30(3), 169–180.
- Barbera J., Wieman C. E., Perkins K. K. and Adams W., (2008), Modifying and validating the Colorado Learning Attitudes about Science Survey for use in chemistry, J. Chem. Educ., 85(10), 1435.
- Barmby P., Kind P. M. and Jones K., (2008), Examining changing attitudes in secondary school science, Int. J. Sci. Educ., 30(8), 1075–1093.
- Bauer C. F., (2008), Attitude toward chemistry: A semantic differential instrument for assessing curriculum impacts, J. Chem. Educ., 85(10), 1440.
- Beauducel A. and Herzberg P. Y., (2006), On the performance of maximum likelihood versus means and variance adjusted weighted least squares estimation in CFA. Struct. Equation Model., 13(2), 186–203.
- Bethel C. M. and Lieberman R. L., (2014), Protein structure and function: An interdisciplinary multimedia-based guided-inquiry education module for the high school science classroom, J. Chem. Educ., 91(1), 52–55.
- Bordón P., Canals C. and Mizala A., (2020), The gender gap in college major choice in Chile, Economics of Education Review, 77, 1–27.
- Brandriet A. R., Xu X., Bretz S. L. and Lewis J. E., (2011), Diagnosing changes in attitude in first-year college chemistry students with a shortened version of Bauer's semantic differential, Chem. Educ. Res. Pract., 12(2), 271–278.
- Burmeister M. and Eilks I., (2012), An example of learning about plastics and their evaluation as a contribution to Education for Sustainable Development in secondary school chemistry teaching, Chem. Educ. Res. Pract., 13(2), 93–102.
- Byrne B. M., (2008), Testing for multigroup equivalence of a measuring instrument: a walk through the process, Psicothema, 20(4), 872–882.
- Bybee R. and McCrae B., (2011), Scientific literacy and student attitudes: Perspectives from PISA 2006 science, Int. J. Sci. Educ., 33(1), 7–26.
- Caleon S. and Subramaniam R., (2008), Attitudes towards science of intellectually gifted and mainstream upper primary students in Singapore, J. Res. Sci. Teach., 45(8), 940–954.
- Can H., (2012), Students’ attitudes toward school chemistry: The effect of interaction between gender and grade level, Asia-Pacific Forum Sci. Learn. Teach., 13(1), 11–16.
- Chase A., Pakhira D. and Stains M., (2013), Implementing process-oriented, guided-inquiry learning for the first time: Adaptations and short-term impacts on students’ attitude and performance, J. Chem. Educ., 90(4), 409–416.
- Chen F. F., (2007), Sensitivity of goodness of fit indexes to lack of measurement invariance, Struct. Equation Model., 14(3), 464–504.
- Cheung D., (2009), Students’ attitudes toward chemistry lessons: The interaction effect between grade level and gender, Res. Sci. Educ., 39(1), 75–91.
- Cheung D., (2011), Evaluating student attitudes toward chemistry lessons to enhance teaching in the secondary school, Educ. Quím., 22(2), 117–122.
- Cho E., (2016), Making Reliability Reliable: A Systematic approach to reliability coefficients, Org. Res. Methods, 19(4), 651–682.
- Chua K. E. and Karpudewan M., (2017), The role of motivation and perceptions about science laboratory environment on lower secondary students’ attitude towards science, Asia-Pacific Forum Sci. Learn. Teach., 18(2), 1–16.
- Cousins A., (2007), Gender inclusivity in secondary chemistry: A study of male and female participation in secondary school chemistry, Int. J. Sci. Educ., 29(6), 711–730.
- Dalgety J., Coll R. K. and Jones A., (2003), Development of chemistry attitudes and experiences questionnaire (CAEQ). J. Res. Sci. Teach., 40(7), 649–668.
- Desy E. A., Peterson S. A. and Brockman V., (2011), Gender Differences in Science-Related Attitudes and Interests among Middle School and High School Students, Sci. Educ., 20(2), 23–30.
- DiStefano C. and Morgan G. B., (2014), A comparison of diagonal weighted least squares robust estimation techniques for ordinal data, Struct. Equation Model., 21(3), 425–438.
- Dries D. R., Craig P. A., Dean D. M., Listenberger L., Novak W. R. and Franzen M. A., (2016), Molecular Visualization in the Classroom: Learning Goals and Competencies, FASEB J., 30, 666.
- Dierks P. O., Höffler T. N. and Parchmann I., (2016), Profiling interest of students in science: Learning in school and beyond, Res. Science and Technological Education, 32(2),97–114.
- Eagly A. H. and Chaiken S., (2005), Attitude research in the 21st century: The current state of knowledge, in D. Albarracin, B. T. Johnson and M. P. Zanna (ed.), The handbook of attitudes, Mahwah, NJ: Lawrence Erlbaum Associates, pp. 743–767.
- Else-Quest N. M., Mineo C. C. and Higgins A., (2013), Math and science attitudes and achievement at the intersection of gender and ethnicity, Psychol. Women Q., 37(3), 293–309.
- Fabrigar L. R., MacDonald T. K. and Wegener D. T., (2005), The structure of attitudes, in The handbook
of attitudes, ed. D. Albarracin, B. T. Johnson and M. P. Zanna, Mahwah, NJ: Lawrence Erlbaum Associates, pp. 79–124.
- Farooq S., Chaundhry H. and Berhanu G., (2011), Factors Affecting Students’ Quality of Academic Performance: A Case of Secondary School Level, J. Quality Technol. Manage., 7(2), 1–14.
- Field A. P., Miles J. and Field Z., (2012), Discovering statistics using R/Andy Field, Jeremy Miles, Zoë Field.
- Finch W. H. and French B. F., (2015), Latent variable modeling with R. Routledge.
- Finney S. J. and DiStefano C., (2013), Non-normal and categorical data in structural equation modeling, in Hancock G. R. and Mueller R. O. (ed.), Structural equation modeling: a second course. Charlotte, NC: Information Age Publishing, pp. 439–492.
- Garson D., (2010), Statnotes: Topics in Multivariate Analysis: Factor Analysis. Retrieved from http://faculty.chass.ncsu.edu/garson/pa765/statnote.htm.
- George R., (2006), A cross-domain analysis of change in students’ attitudes toward science and attitudes about the utility of science, Int. J. Sci. Educ., 28(6), 571–589.
- Gómez Ferragud C. B., Solaz Portolés J. J. and Sanjosé López V., (2015), Effects of topic familiarity on analogical transfer in problem-solving: A think-aloud study of two singular cases, Eur. J. Math., Sci. Technol. Educ., 11,(4), 875–887.
- Gregorich S. E., (2006), Do self-report instruments allow meaningful comparisons across diverse population groups? Testing measurement invariance using the confirmatory factor analysis framework, Med Care, 44(11 suppl 3), S78–S94.
- Grove N. and Bretz S. L., (2007), CHEMX: An instrument to assess students' cognitive expectations for learning chemistry, J. Chem. Educ., 84(9), 1524.
- Harle M. and Towns M., (2011), A review of spatial ability literature, its connection to chemistry, and implications for instruction, J. Chem. Educ., 88(3), 351–360.
- Harrison A. G. and Treagust D. F., (2002), The particulate nature of matter: Challenges in understanding the submicroscopic world, Chemical education: Towards research-based practice. Dordrecht: Springer, pp. 189–212.
- Harrison M., Dunbar D., Ratmansky L., Boyd K. and Lopatto D., (2011), Classroom-based science research at the introductory level: Changes in career choices and attitude, CBE Life Sci. Educ., 10, 279–286.
- Heredia K. and Lewis J. E., (2012), A psychometric evaluation of the colorado learning attitudes about science survey for use in chemistry, J. Chem. Educ., 89(4), 436–441.
- Hofstein A. and Mamlok-Naaman R., (2011), High-School students’ attitudes toward and interest in learning chemistry, Educ. Quím., 22(2), 90–102.
- Holbrook J., (2005), Making chemistry teaching relevant, Chem. Educ. Int., 6(1), 1–12.
- Jenkins E. and Nelson W., (2005), Important but not for me: Students’ attitudes towards secondary school science in England, Res. Sci. Technol. Educ., 23(1), 41–57.
- Johnstone A. H., (1993), The development of chemistry teaching: A changing response to changing demand, J. Chem. Educ., 70(9), 701–705.
- Johnstone A. H., (2006), Chemical education research in Glasgow in perspective, Chem. Educ. Res. Pract., 7(2), 49–63.
- Kahveci A., (2015), Assessing high school students’ attitudes toward chemistry with a shortened semantic differential, Chem. Educ. Res. Pract., 16(2), 283–292.
- Kanafiah M. and Jumadi A., (2013), Students’ perception towards mathematics: attitudes, interests and lecturers’ teaching, International Symposium on Mathematical Sciences and Computing Research, 6–7.
- Komperda R., Hosbein K. N. and Barbera J., (2018), Evaluation of the influence of wording changes and course type on motivation instrument functioning in chemistry, Chem. Educ. Res. Pract., 19, 184–198.
- Kousa P., Kavonius R. and Aksela M., (2018), Low-achieving students’ attitudes towards learning chemistry and chemistry teaching methods, Chem. Educ. Res. Pract., 19(2), 431–441.
- Lazarowitz R. and Naim R., (2013), Learning the cell structures with three-dimensional models: Students achievement by methods, type of school and questions’ cognitive level, J. Sci. Educ. Technol., 22, 500–508.
- Lester F. K., Garofalo J. and Kroll D. L., (1989), Self-confidence, interest, beliefs, and metacognition: key influences on problem-solving behavior, in D. B. McLeod and V. M. Adams (ed.) Affect and mathematical problem solving, Berlin, Germany: Springer-Verlag, pp. 75–88.
- Lord P., Straw S., Hart R., Springate I. and Harland J., (2009), Evaluation of chemistry for our future: Extension phase report. Slough: National Foundation for Educational Research.
- Mackatiani C. I., (2017), Influence of Examinations Oriented Approaches on Quality Education in Primary Schools in Kenya, J. Educ. Pract., 8(14), 51–58.
- Martínez-Aznar M. M., Rodríguez-Arteche I. and Gómez-Lesarri P., (2017), La resolución de problemas profesionales como referente para la formación inicial del profesorado de física y química, Revista Eureka sobre Enseñanza y Divulgación de las Ciencias, 14(1), 162–180.
- Mineduc, (2009), Marco Curricular: Objetivos Fundamentales y Contenidos Minimos Obligatorios de la Educación Básica y Media, Santiago: Unidad de Curriculum y Evaluación.
- Mineduc, (2019), Bases curriculares: Tercer y Cuarto año medio, Unidad de Currículum y Evaluación, Santiago, Chile: Mineduc.
- McDonald R. P., (1999), Test theory: A unified treatment. Mahwah, NJ: Lawrence Erlbaum Associates.
- Molina F., Carriazo G. and Farías M. (2011), Actitudes hacia la química de estudiantes de diferentes carreras universitarias en Colombia, Química Nova, 34, 1672–1677.
- Molina M., Carriazo J. and Casas J., (2013), Estudio transversal de las actitudes hacia la ciencia en estudiantes de grados quinto a undécimo. Adaptación y aplicación de un instrumento para valorar actitudes, Tecné, Episteme y Didaxis: TED, (33), 45–60.
- Montes L. H., Ferreira R. A. and Rodríguez C., (2018), Explaining secondary school students’ attitudes towards chemistry in Chile, Chem. Educ. Res. Pract., 533–542.
- Navarro M. B. and Förster C. E., (2012), Nivel de alfabetización científica y actitudes hacia la ciencia en estudiantes de secundaria: comparaciones por sexo y nivel socioeconómico. Pensamiento Educativo, Revista de Investigación Latinoamericana (PEL), 49(1), 1–17.
- Neto A., Candeias A., Pomar C., Costa P., Oliveira M. and Silva S., (2011), Questionários de atitudes face à língua portuguesa (QAFLP), matemática (QAFM), ciências da natureza (QAFCdN), ciências naturais (QAFCN) e ciências físico-químicas (qafcfq) em alunos portugueses do ensino básico: estudo psicométrico, Proceedings of the XI Congreso Internacional Galego-Portugués de Psicopedagoxía, Coruña: Universidade da Coruña.
- Nieswandt
M., (2007), Student affect and conceptual understanding in learning chemistry, J. Res. Sci. Teach., 44(7), 908–937.
- O’Dwyer A. and Childs P., (2014), Organic chemistry in action! Developing an intervention program for introductory organic chemistry to improve learners’ understanding, interest, and attitudes, J. Chem. Educ., 91(2), 987–993.
- Oskamp S. and Schultz P. W., (2005), Attitudes and opinions, 3rd edn, Mahwah, New Jersey: Lawrence Erlbaum, 2005.
- Overton T. and Potter N., (2011), Investigating students’ success in solving and attitudes towards context-rich open-ended problems in chemistry, Chem. Educ. Res. Pract., 12(3), 294–302.
- Overton T., Potter N. and Leng C., (2013), A study of approaches to solving open-ended problems in chemistry, Chem. Educ. Res. Pract., 14(4), 468–475.
- Peterson C. and Seligman M. E. P., (2004), Character strengths and virtues: a handbook and classification. Oxford, United Kingdom: Oxford University Press.
- Pinarbasi T. and Canpolat N., (2003), Students' understanding of solution chemistry concepts, J. Chem. Educ., 80(11), 1328–1332.
- Potvin P. and Hasni A., (2014), Analysis of the Decline in Interest Towards School Science and Technology from Grades 5 Through 11. J. Sci. Educ. Technol., 23(6), 784–802.
- R Core Team, (2020), A language and environment for statistical computing, Vienna, Austria: R Foundation for Statistical Computing.
- Randles C. A. and Overton T. L., (2015), Expert vs. novice: approaches used by chemists when solving open-ended problems, Chem. Educ. Res. Pract., 16(4), 811–823.
- Reid N. and Yang M. J., (2002), The Solving of Problems in Chemistry: The more open-ended problems, Res. Sci. Technol. Educ., 20(1), 83–98.
- Riegle-Crumb C. and Peng M., (2021), Examining High School Students’ Gendered Beliefs about Math: Predictors and Implications for Choice of STEM College Majors, Soc. Educ., 94(3) 227–248.
- Rocabado G. A., Kilpatrick N. A., Mooring S. R. and Lewis J. E., (2019), Can we compare attitude scores among diverse populations? An exploration of measurement invariance testing to support valid comparisons between Black female students and their peers in an organic chemistry course, J. Chem. Educ., 96(11), 2371–2382.
- Rocabado G. A., Komperda R., Lewis J. E. and Barbera J., (2020), Addressing diversity and inclusion through group comparisons: a primer on measurement invariance testing, Chem. Educ. Res. Pract., 21(3), 969–988.
- Sababha H., Alqudah A., Abualbasal A. and AlQaralleh A., (2016), Project-based learning to enhance teaching embedded systems, Eurasia Journal of Mathematics, Sci. Technol. Educ., 12(9), 2575–2585.
- Salta K. and Tzougraki C., (2004), Attitudes toward chemistry among 11th grade students in high schools in Greece, Sci. Educ., 88(4), 535–547.
- Sanmarti Puig N. and Márquez Bargalló C., (2017), Aprendizaje de las ciencias basado en proyectos: del contexto a la acción, Ápice, 1(1), 3–16.
- Schoenfeld A. H., (1983), The wild, wild, wild, wild, wild world of problem solving (A review of sorts), For the learning of mathematics, 3(3), 40–47.
- Schrodinger L., (2012), The PyMOL Molecular Graphics System, version 1.7.
- Sorge C., (2007), What Happens? Relationship of Age and Gender with Science Attitudes from Elementary to Middle School, Sci. Educ., 16(2), 33–37.
- Surif J., Ibrahim N. H. and Dalim S. F., (2014), Problem solving: Algorithms and conceptual and open-ended problems in chemistry, Proc. –Soc. Behav. Sci., 116, 4955–4963.
- Tomarken A. J. and Waller N. G., (2003), Potential problems with “well fitting” models, J. Abnorm. Soc. Psychol., 112(4), 578.
- Trizano-Hermosilla I. and Alvarado J. M., (2016), Best alternatives to Cronbach's alpha reliability in realistic conditions: congeneric and asymmetrical measurements, Front. Psychol., 7, 769.
- Ventura-León J. L., (2017), Intervalos de confianza para coeficiente Omega: propuesta para el cálculo, Adicciones, 30(1), 77–78.
- Vilia N., Candeias A., Neto S., Franco S. and Melo M., (2017), Academic Achievement in Physics-Chemistry: The Predictive Effect of Attitudes and Reasoning Abilities, Front. Psychol., 8, 1064.
- Xu X. and Lewis J. E., (2011), Refinement of a chemistry attitude measure for college students, J. Chem. Educ., 88(5), 561–568.
- Xu X., Southam D. and Lewis J. (2012), Attitude toward the subject of Chemistry in Australia: An ALIUS and POGIL collaboration to promote cross-national comparisons, Aust. J. Educ. Chem., 72, 32–36.
- Xu X., Villafane S. M. and Lewis J. E., (2013), College students' attitudes toward chemistry, conceptual knowledge and achievement: structural equation model analysis, Chem. Educ. Res. Pract., 14(2), 188–200.
- Zakaria E. and Ngah N., (2011), A preliminary analysis of students’ problem-posing ability and its relationship to attitudes towards problem solving, Res. J. Appl. Sci., Eng. Technol., 3(9), 866–870.
- Zakaria E. and Yusoff N., (2009), Attitudes and problem-solving skills in algebra among Malaysian matriculation college students, Eur. J. Soc. Sci., 8(2), 232–245.
- Zarkadis N., Papageorgiou G. and Stamovlasis D., (2017), Studying the consistency between and within the student mental models for atomic structure, Chem. Educ.: Res. Pract. Eur., 18(4), 893–902.
|
This journal is © The Royal Society of Chemistry 2022 |
Click here to see how this site uses Cookies. View our privacy policy here.