Teaching stereoisomers through gesture, action, and mental imagery
Received
22nd November 2021
, Accepted 9th May 2022
First published on 10th May 2022
Abstract
Many undergraduate chemistry students struggle to understand the concept of stereoisomers, molecules that have the same molecular formula and sequence of bonded atoms but are different in how their atoms are oriented in space. Our goal in this study is to improve stereoisomer instruction by getting participants actively involved in the lesson. Using a pretest–instruction–posttest design, we instructed participants to enact molecule rotation in three ways: (1) by imagining the molecules’ movements, (2) by physically moving models of the molecules, or (3) by gesturing the molecules’ movements. Because gender differences have been found in students’ performance in chemistry (Moss-Racusin et al., 2018), we also disaggregated our effects by gender and examined how men and women responded to each of our 3 types of instruction. Undergraduate students took a pretest on stereoisomers, were randomly assigned to one of the 3 types of instruction in stereoisomers, and then took a posttest. We found that, controlling for pretest performance, both women and men participants made robust improvements after instruction. We end with a discussion of how these findings might inform stereoisomer instruction.
Introduction
Many undergraduates have negative experiences in chemistry courses. Why? Like all human problems, multiple factors contribute to overall success in chemistry coursework. Research has examined motivation and self-efficacy (Gibbons et al., 2018; Avargil, 2019; Gibbons and Raker, 2019), background preparation, spatial and mental rotation ability (Pribyl and Bodner, 1987; Stieff et al., 2014; Stieff and Uttal, 2015; Stieff et al., 2018), amount of time spent studying and method of studying (Lopez et al., 2013), faculty relationship quality (Barr et al., 2008), to name a few. Gender has also been a focus, given that men are overrepresented in STEM fields (National Science Foundation, 2019). Although the proportion of women earning bachelor's degrees in chemistry is approximately equal to men (Matson, 2013; Cheryan et al., 2017; National Science Foundation, 2019), women are less likely to complete graduate degrees in chemistry or enter chemistry-related professions (National Science Foundation, 2019). Gender differences in spatial skills relevant to chemistry might be one explanation (Stieff et al., 2014). However, these differences are small (typically about half a standard deviation, Peters et al., 2007). These differences can be eliminated with practice and training (Feng et al., 2007; Stieff et al., 2014), making sociocultural factors, such as attitudes and stereotypes, a potentially better explanation for these gender differences (Ceci et al., 2009; Sunny et al., 2017).
Given the complexity of this problem, researchers hoping to increase gender diversity in STEM fields have taken several approaches. Some focus on “pipeline” issues by increasing Advanced Placement enrollment (Corra et al., 2011) or parental support in high school (see, for example, Simpkins et al., 2015). Others focus on interventions that can benefit learners by meeting them where they are. Some focus on socio-emotional dimensions such as asking students to take an additional support course (Lee et al., 2018), or reducing anxiety or negative affect (Raker et al., 2019). Others focus on building skills (Pribyl and Bodner, 1987; Uttal et al., 2013) or teaching concepts more effectively by introducing specialized teaching tools (e.g., virtual models, Stull and Hegarty, 2016; transparent whiteboards, Stull et al., 2018, etc.). Our project takes the latter approach. We follow up on previous work by asking whether an active, embodied chemistry lesson is effective in improving learning.
Involving the body in active engagement supports learning in organic chemistry
Chemistry is generally taught using slides, textbooks, and animation programs. But there is reason to believe that engaging the body when explaining a chemistry concept is related to learning the concept. One of the challenges for STEM learning is that students have to represent complex phenomena that are often not detectable to the naked eye. This representational competence is essential for reasoning, indeed Stieff and colleagues (2020) suggest that representational competence developed through STEM classroom activities can benefit STEM learning more than focusing on spatial ability. This representational ability can be enhanced in a variety of ways, but at a minimum, requires the ability to visualize change in structures, to compare different vantage points of a structure and to translate representations between 2D and 3D versions. Developing this representational skill has been practiced in classrooms in a variety of ways. Imagining molecules’ movements has been found to help learners (particularly men) develop this representational skill (Stieff et al., 2014).
But research has also shown that embodying the dynamic changes can lead to representational competence in molecular chemistry (DeSutter and Stieff (2020)—although there are there are limits to this approach depending on the complexity of the visualization). Although a detailed review of embodied cognition is beyond the scope of this paper, the general argument is that the human mind is fundamentally shaped by having a human body and using that body to interact with the world (e.g., Gibbs, 2005). Many studies show that bodily experiences impact what were previously considered to be purely mental processes (see Castro-Alonso et al. (2019) and Niedenthal et al. (2005) for extensive examples). One core part of the embodied cognition argument is that when generating mental imagery (e.g., mentally rotating an object), we are engaging in an embodied simulation that is neurally similar to seeing mental rotation (see, e.g., Zwaan et al., 2002).
Stereoisomers in chemistry education
We focus here on students learning stereoisomers. Stereoisomers are molecules that have the same molecular formula and sequence of bonded atoms, but are different in how their atoms are oriented in space. That is, there is no degree of rotation that results in perfect superimposability (Michl, 2003). Our instruction centers on this core principle of non-superimposability of stereoisomers. Reading about or viewing 2-D representations of molecule rotation, and then making inferences about the 3-D molecules, is difficult for some students. Students are often asked to mentally rotate, but enacting the actual rotation of a molecule may be a more effective way to instruct students in stereoisomers. For example, requiring students to act on a physical or virtual model of molecules does, in fact, benefit learners (Stull and Hegarty, 2016).
Active learning and student engagement
Active Learning is a movement within the field of education. It argues that students learn more when they engage in activities (problem solving, discussion, using physical models), rather than passively absorbing material. Empirical support for the claims of active learning is emerging (Freeman et al., 2007; Freeman et al., 2014), but there is controversy. A recent meta-analysis of active learning in undergraduate STEM courses found a benefit for active learning in some studies, but not in all (Freeman et al., 2014). As Bernstein (2018) points out, a better question to ask is which active learning strategies work and why (see also Rau and Herder, 2021).
Lower-level cognition that could explain why active learning can benefit learners includes memory and affect. Active learning might reset attentional focus, allowing for an increase in sustained attention, which improves memory for the course materials (Cherney, 2008; Young et al., 2009; Fuller et al., 2018). Or active learning might increase positive affect (Steen-Utheim and Foldnes, 2018). The literature on affect and learning is large and complex, but generally finds that positive affect leads to better learning (typically mediated by, or in relationship with, other factors such as motivation and self-regulation; Brom et al., 2017). Some of this benefit may stem from changes in attention as a function of mood (Scrimin and Mason, 2015).
Higher-level explanations for the benefits of active learning are often grounded in constructivist theories of learning. Constructivism argues that knowledge is created through sensory, motor (Piaget, 1952; Liu and Matthews, 2005) and affective-social (Rieber, et al., 1987; Vygotsky, 1938, 1986) interactions (Bruner, 1966; Kolb and Kolb, 2005). For example, the way a young child approaches the task of navigating down a slope is shaped by her experience with crawling, the grade of the slope, and whether her caregiver is encouraging or discouraging (Adolph et al., 2010). For college-aged learners, the same general principles are expected to apply. According to these accounts, active learning is beneficial because it reshapes pedagogical practices so they are consistent with the way learning actually happens—as part of a dynamic system in which social, emotional, sensory-motor events are assimilated to existing experiences (see Osgood-Campbell, 2015, for discussion). There is good reason to believe in the arguments of constructivism (Bransford et al., 2000), if not necessarily in any direct link to active learning paradigms.
Embodied cognition and gesture
What is new to this array of teaching techniques is the use of gesture as a way to enact molecular rotation. Gesture is defined generally as “spontaneous movements of the hands and arms accompanying speech” (McNeill, 1992, p. 37). Ping and colleagues (2021) observed the hand gestures students spontaneously produced when asked to explain stereoisomers, a core principle of organic chemistry. Students who had never studied stereoisomers completed a set of problems that involved drawing molecules and explaining why (or why not) the molecule drawings represented stereoisomers. The students were then given a brief lesson in stereoisomers. There was not a single student who could solve the stereoisomer task and explain it correctly before the lesson. After the lesson, a small number of students succeeded, and their success was predicted by the responses they produced in gesture prior to the lesson—in particular, by responses in which gesture conveyed a relevant problem-solving strategy not found in the accompanying speech. The gestures enacted the appropriate molecular rotation needed to solve the problem; the accompanying speech did not. This finding suggests that engaging the body in a chemistry lesson could be beneficial to learning. However, the improvement students showed after the lesson in the Ping et al. (2021) study was small, perhaps not generalizable to performance in the classroom.
Our goal here was to harness the active engagement students display spontaneously in their gestures as a method to increase their ability to profit from a chemistry lesson. But gesturing is only one of many techniques that students can use to actively engage in a chemistry lesson.
A number of scholars have pointed out the implications of embodied cognition for education (Glenberg, 2008; Osgood-Campbell, 2015; Castro-Alonso et al., 2019; Shapiro and Stolz, 2019; Nathan, 2021), but Parrill (2020) provides a discussion of the connections between active learning, embodied cognition, and gesture specifically. To put it briefly, gesture can provide a bridge between real actions in the world (rotating an object) and abstract concepts (superimposability) because it allows for the creation of schematized motor representations that are linked to semantic representations. Exploring gestural embodiment is of particular interest for the study of stereochemistry because it is neither as direct as rotating a molecule model, nor is it as indirect as imagining rotating a molecule. As mentioned earlier, learners’ gestures are a critical source of information about the mental representations that underlie their concept of stereoisomers, and can indicate readiness to transition to a new understanding of the concept (Ping et al., 2021; see also Alibali et al., 1997). In addition, gestural embodiment may have greater impact on generalized or transfer learning (Novack and Goldin-Meadow, 2015) than more direct embodiment.
Research also suggests that asking people to imitate gesture that represents advanced understanding of a concept can enhance learning of the concept (Stevanoni and Salmon, 2005; Broaders et al., 2007; Ping and Goldin-Meadow, 2008; Goldin-Meadow et al., 2009; Carlson et al., 2014; Novack et al., 2014). Meaningful gestures of a concept can create motor representations (Hostetter and Alibali, 2008), which help with retrieval of the concept (Kelly et al., 2009; Cook et al., 2010; Macedonia et al., 2011; Macedonia and von Kriegstein, 2012). The logic is that, because learners have both a semantic and a motor representation of a concept, they have multiple pathways for sense making.
Including gesture as a source of information about concepts in chemistry is becoming more common. Flood and colleagues (2015) argue for the necessity of including gestures produced by chemistry learners (who were studying molecular geometry) as a way of understanding how meaning is made. Abels (2016) illustrates how qualitative investigations of gesture during chemistry instruction (on atomic structure) can reveal important information about both interactive and conceptual dynamics. Rau and Herder (2021) compared physical and virtual training on energy diagrams. The most crucial of their findings (for our purposes) was that different kinds of gesture emerged depending on training. Rau and Herder argue that gestures index embodied schemas that are essential for the concept being acquired.
In a study on learning about molecular structure, Stieff and colleagues (2016) compared seeing gesture with seeing and doing gesture. They gave participants text training, training that asked them to observe gestural representations of a molecule, or training that asked them to observe and perform the gestural representations. Participants who observed and performed gestures scored better on an assessment than the other two groups. Stieff and colleagues (2016) also compared training in which students gestured to training in which students acted on three dimensional molecule models. During assessment, some learners had the models present; others did not. When the physical model was not present during assessment, learners who were trained on the model performed worse than they did with the model present. Learners who were trained to produce gestures did equally well whether the model was present or absent during the assessment. This finding suggests that instruction enabling abstract embodiment of molecule representations can lead to transferable or generalized learning that does not rely on a concrete model being present. This claim is in line with the general argument that different kinds of training support the acquisition of different dimensions of a concept (Rau and Herder, 2021).
Summary.
There are many reasons why undergraduate students struggle with chemistry, particularly organic chemistry. We suggest that actively engaging students in representing how the molecules of a stereoisomer move may be a strategy that improves learning in all populations. If so, it would have the potential to reduce disparities.
Here we compared three kinds of training that encourage students to actively represent molecule movement in stereoisomer problems. We asked students to directly rotate physical models of molecules, or to perform gestures about enacting rotation of molecules. These two conditions involve motor action. As a contrast for the physical movement conditions, we created a third condition in which a student was to imagine the rotation of a molecule, an activity that does not require motor engagement of the body. We constructed three stereoisomer lessons that could incorporate these three different types of active engagement; physically rotating a molecule model, gesturing the rotation of a molecule and imagining the rotation of a molecule.
We address the following two research questions:
(1) Do different types of active engagement in stereoisomer instruction benefit learning? We designed our instruction around stereoisomers because understanding stereoisomers is important for success in chemistry coursework, and because the spatial content of the relevant molecules can be enacted in a variety of ways. We chose a third condition that embodies rotation in the form of mental imagery with no motor engagement of the body. We compared this training to physically and gesturally rotating a model of stereoisomer molecules.
Learning may be facilitated by the active embodiment of molecular rotation regardless of the method of active engagement. Alternatively, acting on physical models may be the most effective training because action is familiar to students. Gesturing has the potential to benefit learners more than acting because it frees learners from details of the model and focuses them on the underlying principle of rotation. Imagining rotation has the potential to be the most effective training because it requires students to create an abstract mental representation.
(2) Given that there are gender differences in Science, Technology, Engineering and Mathematics fields, we also examine the impact of our training on men and women. Does the instruction we provide benefit men and women learners equally? If men are more likely to use imagery than women (Stieff et al., 2014; see also Wakefield et al. (2019a, 2019b)), we might expect them to perform better than women with the training that asks learners to imagine, but not enact, movement.
Methods
Participants
Our sample size was based on a priori power analyses conducted with G*Power3 (Faul et al., 2009) using an F family of tests. With a medium effect size, alpha set to 0.05, and 1-beta set to 0.80, we found that a sample size of at least 77 would be required. The goal was to collect data from about 25 to 26 individuals within each of the three experimental conditions. Table 1 shows the output of that effort. We nearly met our goal with N = 74 participants, with N = 26 in the Action condition, N = 25 in the Gesture condition, and N = 23 in the Imagine condition. Because we predicted that gender might interact with condition, we aimed toward a minimum of at least 10 individuals of each gender in each of the three conditions. Again, referring to Table 1, we met this goal in 5 of those six cases, with N = 9 male participants in the Gesture condition.
Table 1 Participants by condition, study site and gender
Condition |
Female |
Male |
Total |
Action |
16 |
10 |
26 |
CWRU |
7 |
6 |
13 |
UIC |
2 |
2 |
4 |
UChicago |
7 |
2 |
9 |
Gesture |
16 |
9 |
25 |
CWRU |
8 |
3 |
11 |
UIC |
2 |
4 |
6 |
UChicago |
6 |
2 |
8 |
Imagine |
13 |
10 |
23 |
CWRU |
9 |
4 |
13 |
UIC |
4 |
1 |
5 |
UChicago |
0 |
5 |
5 |
Total |
45 |
29 |
74 |
The study was approved by the university IRBs, which ensured that: confidentiality was protected through the use of an alphanumeric code on de-indentified data; students had alternatives to participating for extra credit; and informed consent was comprehensive. None of the researchers was an instructor in a course that participants were taking at the time of the study. Informed consent was obtained (written) and participants had the opportunity to ask questions and to withdraw from the study at any time.
In order to maximize external validity of our findings, we recruited as many participants as possible from Organic Chemistry courses across two sites—for a total of 52 participants (37 at Case Western Reserve University, CWRU, a small private university in northeast Ohio; 15 at University of Illinois at Chicago, UIC, a large public university in northern Illinois). We supplemented this pool by simultaneously running participants at the University of Chicago (UChicago), a small private university in northern Illinois (N = 22). These individuals were recruited through a list-serve of psychology study volunteers, and had at least one year of formal chemistry education at the high school or undergraduate level.
To maximize internal validity, we used purely random assignment at all three sites until about half of the data were collected, and then used pseudo-random assignment to balance the number of individuals from each site (within each gender) across each of the three conditions. Table 1 displays the number of individuals from each site in each condition by gender. We ended data collection at all three sites at the same time—when the Organic Chemistry students had mastered stereoisomers. Participants were compensated with either course credit or a monetary payment ($20).
Materials
We selected 6 molecules for pretest, 6 molecules for posttest, and 4 molecules for training. Criteria for selection were that the molecule does not have surface level traits that previous participants had found unnecessarily distracting or confusing in the past—no double bonds, no rings. Fig. 1 shows an example of a molecule that was used in pretest. For these representations, we used the wedge-and-dash representation with surface level modifications that highlight the geometric shape of the molecule. So, for example, in Fig. 1, we have modified by naming each of the molecules—even the carbon and hydrogen. We have also combined some substituent groups to simplify structure, presenting CH3 instead of C with H–H–H attached.
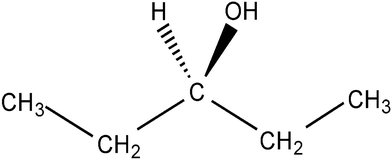 |
| Fig. 1 An example of a “trained molecule” at pretest and posttest. This molecule is analogous to the top left one in Fig. 2. | |
All participants were familiar with traditional wedge and dash representations, and were familiarized with our modifications at the beginning of the procedure. This is described in detail in Procedure subsection. Participants were reminded that the dark-colored triangles (wedges) indicate parts of the molecule coming out of the page in space towards the viewer, and that the light-colored triangles (dashes) indicate parts going into the page in space away from the viewer. In Fig. 1, the hydroxy group (OH) is coming out of the page towards the viewer, and the lone hydrogen (H) atom is going back into the page away from the viewer.
Materials for pretest and posttest
In each of the pretest and posttest, there were 6 molecules. Pretest and posttest questions were of two types: (a) four (4) trained problems with one potential chiral center; that is, enantiomers or potential enantiomers similar to the molecules used in the training (see Fig. 2 for trained molecules); and (b) two (2) transfer molecules with multiple potential chiral centers—one diastereomer and one meso compound. These problems required students to transfer what they had learned during training. See the online repository for the full set (link below). We will refer to pretest/posttest items with only one potential chiral center as “trained molecules”, and those with two potential stereocenters as “transfer molecules”. Items were presented in the same fixed order for everyone, and pretest and posttest problems were not counterbalanced. The images were presented in black and white, and affixed to the whiteboard.
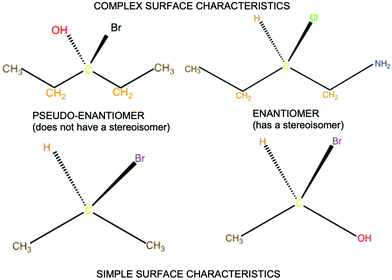 |
| Fig. 2 The four molecules used in the training section of the experiment. The two on the right have enantiomers. The two on the left share surface similarity with the enantiomers, but they do not have a stereoisomer because they are symmetric. For this paper we have labelled them pseudo-enantiomer. Note participants only heard the general term “stereoisomer”. | |
Materials for training
Training molecules consisted of four items—see Fig. 2. Given that we only had about 8–10 minutes for training, we used the principles of analogical reasoning to maximize the power of comparing and contrasting in extracting deep structural similarity between comparators (Gentner et al., 2016). Fig. 2 displays the four molecules. As you can see, the top two share surface similarity, but the one on the right has a stereoisomer while the one of the left does not. The two with more complex surface level characteristics (see Fig. 2, top) were aligned with two examples with simple surface level characteristics (see Fig. 2, bottom). The simple molecules also had one enantiomer (Fig. 2, bottom right) and one symmetric non-stereoisomer that looks like it could be an enantiomer (Fig. 2, bottom left). The examples were chosen so that the learner could extract the structural characteristics (chirality) without getting bogged down in surface level characteristics (the shape of the molecule).
Along with the 2-D representations used in the training, participants also saw a ball-and-stick 3-D representation of the molecule, which mapped onto the colors in the 2D representation (see Fig. 3 for an example). Substituent groups were several different sizes of Styrofoam ball, and sticks of uniform length served as representations of the bonds connecting the groups. All sticks were strongly affixed to the balls except the top two holes in the central carbon, which were left unaffixed so that participants in the action condition could switch their locations. So, for example in Fig. 3, participants in the action condition would pluck the brown and blue ball (along with stick) from their slots in the yellow ball, and reverse them so that the brown ball was in front of the carbon and the blue ball was behind. The central carbon of each molecule was affixed to a screwdriver, which was placed, handle first, in a plastic jar of pennies. Participants in the action condition were thus able to easily rotate the entire model 180 degrees in the horizontal plane.
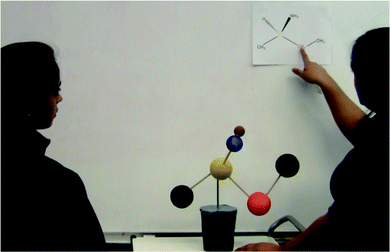 |
| Fig. 3 A researcher explaining how the 2D and 3D models correspond to one another. This is the example from the introduction to the task, but the training was set up in the same way. | |
Procedure overview
Participants followed these five steps: they (1) were familiarized with the materials and the problem; (2) took a pre-test on stereoisomers; (3) participated in instruction in line with one of three conditions (action, gesture, or imagine); (4) took a post-test on stereoisomers, and (5) answered demographic questions.
Detailed procedure
Participants were recorded for the entire experiment, and the camera was placed so that both the gestures during explanations, and the drawings on the whiteboard, were captured on video.
Introduction to the materials and the problem.
First, participants were informed about the graphical conventions used in the study. The researcher placed a 2D image on the white board and a 3-D molecule on the table, and pointed out how one mapped onto the other (see Fig. 3). She pointed out the small changes between our 2D molecules and traditional wedge-and-dash models—described in detail in the materials section. After this explanation, the participant answered two multiple choice questions on paper to ensure that she or he had understood the instructions.
Next, the researcher explained the basic concept of a stereoisomer (the non-superimposable nature of variants of the molecule), and placed a sign on the whiteboard reminding participants that if two molecules are superimposable, they are not stereoisomers. The goal here was to reduce the ‘load’ of having to remember that non-stereoisomers are superimposable, and stereoisomers are non-superimposable. The researcher then showed the participant a brief video on a laptop. This video (available via our Open Science Framework repository, link below) was designed to give participants a simple demonstration of the key differences. Fig. 4 captures a still from the video demonstrating two stereoisomers.
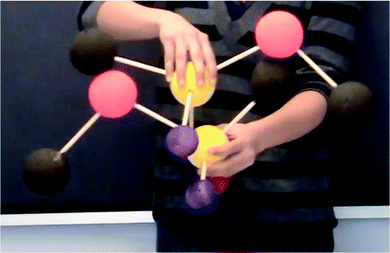 |
| Fig. 4 A still from the video introducing the concept of non-superimposability in stereoisomers. All participants saw this video before the pretest. | |
Pretest.
After watching the video, participants took the pre-test. Participants completed six problems according to the following procedure. The researcher attached a problem to the whiteboard, and instructed the participant to “draw a stereoisomer of this molecule if any exist”.
When the participant appeared to be finished, the researcher ensured that their drawing had all the required atoms. If any part of the molecule was missing, the researcher said “I’m sorry but the molecule must remain bonded in the exact same manner. Your drawing appears to be missing a part.” If the participant attempted to create a simplified drawing of the molecule (by replacing substituents or chains with variables or simpler symbols), the researcher said, “Please draw the substituents shown in the original molecule.” The participant was prompted to erase and correct the model.
When the participant completed the drawing, the researcher prompted her or him to put the marker down, and said, “Please explain why your drawing is a different non-superimposable spatial arrangement of the original molecule.” If the participant claimed there were no stereoisomers, the researcher said, “Can you please explain why you think there are no stereoisomers for this molecule.” If participants started to explain their diagram while drawing, the researcher said, “Please wait until you have finished drawing before explaining your diagram.” This exact procedure was repeated for the remaining five pretest trials. No feedback was provided.
Training.
Participants then received training according to their condition (Action, Gesture, or Imagine). In the training problems in all three conditions, participants were taught three steps to solve the problem: (1) two parts of the molecule were switched, (2) the entire molecule was rotated 180 degrees, and (3) the changed molecule was compared to the original. Participants were shown this three-step process and the researcher emphasized the need to attend to the changes after each step. A sign reading switch, rotate, compare was attached to the whiteboard to decrease the load of remembering the order.
Action condition.
In the action condition, participants acted on physical models made out of colored foam balls. They completed four training problems. In each, they were instructed to remove the two ball-and-sticks representing the substituent groups lying along the Z-axis (e.g., in Fig. 3, the blue and brown balls), and switch (placing one where the other had been). Then, they were told to physically rotate the entire model 180 degrees—rotate. Finally, they were asked to draw the molecule on the board and compare it against the original. The researcher had the participant try out the switching and rotating procedures on a practice problem. The participant then received the first training example, which was a simple stereoisomer (Fig. 2, bottom right). The researcher prompted the participant to switch, rotate, and compare. The researcher then attached a drawing of the stereoisomer (the correct new molecule) to the whiteboard and pointed out the relationship between the two. She emphasized that the new molecule was a stereoisomer because the two were not superimposable. This procedure was repeated for the second training problem, which was a complex stereoisomer (Fig. 2, top right). The third training problem was a simple molecule with no stereoisomer (Fig. 2, bottom left); the procedure was identical except that, after the participant drew the new molecule, the researcher attached the correct drawing to the whiteboard, pointed out the superimposability of the two, and emphasized that the new version was not a stereoisomer of this molecule. The final problem was a complex molecule with no stereoisomer (Fig. 2, top left); the procedure was the same as in the previous problem.
Gesture condition.
As in the action condition, a physical model was placed on the table, and the researcher explained the three-step process. In this condition, participants learned specific switch and rotate gestures to perform. The switch gesture was modelled, such that in Fig. 4, the forefinger was pointing toward the white board, representing the brown ball, and the middle finger was pointing away from the whiteboard, representing the blue ball. The researcher modelled the switch gesture, by pointing the fingers in the opposite direction so that middle finger was going into board and the pointer finger was going away from board, and asked the participant to produce the gesture. In the rotate step, the fingers maintained the V shape and the entire hand was rotated at the wrist around 180 degrees. The comparison step was identical to the previous condition; however, the 3D model was not altered in the gesture condition as it was in the action condition. The participants completed the training problems as described above, except that the researcher prompted them to perform gestures rather than physically moving the model.
Imagine condition.
As in the other conditions, a physical model was placed on the table, and the researcher explained the three-step process. She then prompted the participant to imagine how each step would change the model. She performed the switch and rotate steps and, during each, prompted the participant to imagine the changes. The comparison step was identical to the gesture condition. Participants completed the training problems as described above, except that the researcher prompted them to imagine changing the molecule rather than physically moving the model or gesturing.
The imagine condition was designed to control for actual movement. Mental rotation (simulation of rotation) is considered an active, embodied strategy (students are asked to do something, and are engaging in mental simulation). However, mental rotation does not involve actual movement of the body.
Posttest drawing and explanation.
Participants then completed 6 posttest problems, following the same draw and explain methodology used in the pretest. Participants were not allowed to use physical models during the posttest. No feedback was provided.
Relationship between pre/posttest and training.
We chose a draw-and-explain assessment of learning rather than a paper test without explanation for two reasons. First, the core competency we are attempting to measure is whether learners can translate between 2D conventional drawings (of the sort they will see in classes and texts) and a 3D understanding of the molecule, necessary to determine whether it has a stereoisomer. The trainings we provide support both aspects of this competency and we wanted the assessment to measure both. Second, our previous work suggested that there were multiple ways to be wrong about the binary question (stereoisomer or not). Explanations offered a better way to detect truly correct understanding. We provide greater detail below in our description of speech coding and our dependent variable.
Measures of spatial ability.
Participants completed two measures of spatial ability, Guay's visualization of viewpoints (Guay and McDaniels, 1976) and the Vandenberg and Kuse Mental Rotation Task (Vandenberg and Kuse, 1978). Because the data were lost for most participants, we were not able to analyze this factor and will not provide further details about these measures.
Debriefing.
Participants were asked to complete a demographic sheet asking for age, race/ethnicity, handedness and a guess about the purpose of the experiment. They were also asked to provide their gender. In an effort to recognize the non-binary nature of gender, we provided the following choices: male, female, or other. This list would not be best practice today (see, e.g., Cameron and Stinson, 2019), but the data were collected at a time when it was standard to offer only male and female categories. Other than requiring that students have one year of instruction in chemistry, and that students not have had instruction in stereoisomers, we did not assess prior chemistry knowledge. After the study, participants were informed about its purpose and had an opportunity to ask questions. They then received their compensation.
Coding
Scoring drawings.
A drawing was scored as either correct or incorrect. A correct drawing completely illustrated a possible stereoisomer of the molecule. For molecules without stereoisomers, a response was considered correct if the participant did not produce a drawing and instead stated that the molecule lacked a stereoisomer.
Speech coding.
We coded speech according to a system developed in Ping et al. (2021), shown in Table 2. This system categorizes verbal responses into four levels, based on the strategy expressed. We categorized strategies into those highlighting components irrelevant to the stereoisomer problem (level 0 and 1 strategies) and those highlighting components relevant to the problem (levels 2, 3, and 4). The relevant strategies are shown in Table 2: (a) correctly switching the orientation of any two substituents at the stereocenter, (b) rotating the entire molecule until the substituents are in their original locations, and (c) comparing the non-manipulated substituents of the molecule to see whether they superimpose on one another. Level 2 strategies express either (a) or (b): switch the relative orientation of any two substituents (a), or Rotate the drawn molecule to compare it to the original (b). Those two strategies, when expressed together [(a) + (b)], make up the level 3 strategy, Switch Combined with Rotation. The other level 3 strategy, Mirror Image, is an alternative version of Switch + Rotate. The level 4 strategy, Compare Non-Manipulated Substituents, adds the last component, checking the remaining substituents to see whether they superimpose (c). Speech could not be coded on trials where participants did not give a contentful spoken explanation (e.g., “I am not really sure how I got this but I think it's right”; “I just did what I did last time”), nor on trials where participants repeated the definition of a stereoisomer (e.g., “my drawing is not superimposable on the original molecule”). The experimenter gave a “why?” prompt in response to both kinds of answers; responses were classified as not codable if participants did not respond to the prompts. This coding system has been shown to be reliable across coders (Ping et al., 2021).
Table 2 Strategies expressed in speech
Strategy name |
Description (speech indicates that…) |
Example |
Level 2: Relevant Switch |
Two substituent groups were switched with one another |
“I exchanged the groups on the Carbon so now the Br is going into the board and the OH is coming out” |
|
Level 2: Relevant Rotate |
The entire molecule was rotated in space |
“No matter how you rotate the top or the bottom there is always some combination of rotation on this original one that can match up with the rotation of this.” |
|
Level 3: Switch plus Rotate |
Both switch and rotate included in explanation. |
“I don't think one exists because when you switch the OH and the Cl and you rotate it making the same molecule so they are superimposable” |
|
Level 3: Mirror Image |
By creating the original molecule's mirror image they have created a stereoisomer, or the mirror image of the molecule would be superimposable on the original. |
“So yeah this carbon has two of the same groups attached to it so if we were to draw a mirror image we could actually rotate it back to the original molecule” |
|
Level 4: Level 3 Explanation plus Check Non-Manipulated Substituents |
A molecule manipulated by either Mirror Image or Relevant Switch & Relevant Rotation must be compared to the original to check super-imposability. Typically participants checked manipulated substituents against their original orientations, then checked the two non-manipulated substituents’ locations against their original orientations |
“You notice after the 180 rotation here that the CH3 is on the left hand side as compared to the right hand side here (point to original molecule). Hence the two are not the same.” |
Dependent variable: correct drawing + level 4 speech
The dependent variable used in this study is Correct Drawing + Level 4 Speech. This decision was informed by Ping et al. (2021), which used the same training design. We use this variable because students can have a correct drawing but an incomplete understanding; combining the two measures of performance provides better information than measuring correct drawings or measuring fully correct explanations (level 4 speech) on their own This criterion for performance leaves no doubt that the individual explicitly understands the problem. Performance on each pretest and each posttest item was scored as 0 or 1. A response received a score of 1 when it had both: (a) a correct drawing and (b) a level 4 explanation in speech, and 0 otherwise.
Results
Data have been uploaded to Open Science Framework: https://osf.io/h35bw/. Data were analyzed using R version 4.0.0 (2020-04-24), known as “Arbor Day” (R Core Team, 2020). Utility packages used for data manipulation, cleaning, and reporting include KnitR (Xie, 2020), haven (Wickham and Bryan, 2019; Wickham and Miller, 2019), readxl (Wickham and Bryan, 2019), dplyr (Wickham et al., 2020), and tidyr (Wickham and Henry, 2020). Visualizations and tables were created with ggplot2 (Wickham, 2016) and flextable (Gohel, 2020). Statistical analysis and modelling were completed with nlme (R Core Team, 2020; Pinheiro et al., 2015), lme4 (Bates et al., 2015), psych (Revelle, 2019), car (Fox and Weisberg, 2019), and emmeans (Lenth, 2020). When binomial data are analyzed (e.g., correct vs. incorrect as the dependent variable), we used mixed method logistic regression analysis. Where change data were analyzed (e.g., gain from pretest to posttest), we used Gaussian linear regression models. Where contrasts between more than two levels are reported, Tukey's correction was applied. These analyses all use the dependent variable “Correct Drawing + Level 4 Speech”.
Study site
We first asked whether students from the different research sites performed differently. We collapsed across pretest and posttest for students from each test site. There was no statistically significant difference between the proportion of problems correct for each site, with students from each site correctly responding to roughly 1/3 of problems: CWRU (M = 0.28, SD = 0.45), UIC (M = 0.21, SD = 0.41), and UChicago (M = 0.30, SD = 0.46), χ2(2) = 1.54, p = 0.46. Table 3 displays the model summary table for the proportion correct. With CWRU set as comparison, there was no significant effect of being at test site UIC (β = −0.392, SE = 0.386, Wald z = −1.016, p = 0.310), or at test site UChicago (β = 0.114, SE = 0.332, Wald z = 0.344, p = 0.731; AIC = 983 on 883 degrees of freedom). Planned contrasts also showed no difference between UIC and U of C (β = −0.51, SE = 0.42, Wald z = −1.21, p = 0.45). Given that we found no difference as a function of site, we collapsed across data from the three study sites.
Table 3 Logistic regression model summary table for study site
|
Estimate |
SE |
z value |
Pr(>|z|) |
(Intercept) |
−1.157 |
0.207 |
−5.591 |
0 |
Test site UIC |
−0.392 |
0.386 |
−1.016 |
0.31 |
Test site U of C |
0.114 |
0.332 |
0.344 |
0.731 |
Does training lead to learning?
Our first question was whether a brief training on stereoisomers led to learning. We elected to include gender in this analysis to determine whether male and female participants performed differently. We looked for an effect of time, an effect of gender, and an interaction between time and gender. Table 4 shows descriptive statistics for time and gender: Fig. 5 shows a boxplot by time and gender.
Table 4 Mean proportion correct by time and gender
Gender |
Time |
Mean |
SD |
Male |
Pre |
0.13 |
0.33 |
Post |
0.47 |
0.50 |
Female |
Pre |
0.10 |
0.30 |
Post |
0.41 |
0.49 |
With only time (pretest to posttest) in the model, there was a statistically significant difference between the proportion of problems correct on the pretest (M = 0.10, SD = 0.31), compared to the posttest (M = 0.35, SD = 0.50), χ2(2) = 116.72, p < 0.0001 (see Table 5). With pretest set as comparison, there was a significant effect of time (β = 2.41, SE = 0.22, Wald z = 10.80, p < 0.001; AIC = 823.62 on 884 degrees of freedom). Our brief training significantly improved the participants’ understanding of stereoisomers.
Table 5 Logistic regression model summary table for time
|
Estimate |
SE |
z value |
Pr(>|z|) |
(Intercept) |
−2.77 |
0.26 |
−10.73 |
0.00 |
Time (post) |
2.41 |
0.22 |
10.8 |
0.00 |
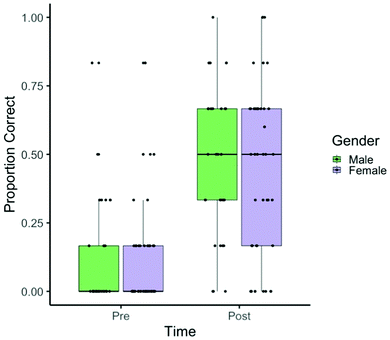 |
| Fig. 5 Mean proportion correct by time and gender. | |
With only gender in the model, there was no statistically significant difference between proportion correct in males (M = 0.30, SD = 0.46) and females (M = 0.25, SD = 0.44), χ2(2) = 0.83, p = 0.36 (see Table 6). With male set as comparison, there was no significant effect of gender (β = −0.27, SE = 0.30, Wald z = −0.91, p = 0.36; AIC = 9813.74 on 884 degrees of freedom). Contrary to past research, female and male participants in our study displayed the same level of stereoisomer understanding both before and after training.
Table 6 Logistic regression model summary table for gender
|
Estimate |
Std error |
z value |
Pr(>|z|) |
(Intercept) |
−1.04 |
0.23 |
−4.49 |
0.00 |
Gender (female) |
−0.27 |
0.30 |
−0.91 |
0.36 |
With time and gender both included in the model, we replicated the main effect of time and no main effect of gender, and found no significant time × gender interaction χ2(1) = 0.01, p = 0.90 (see Table 7). With male pretest set as comparison, there was no significant interaction between time and gender: β = 0.05, SE = 0.43, Wald z = 0.12, p = 0.906; AIC = 826.77 on 882 degrees of freedom. In other words, both genders learned equally well from our instruction.
Table 7 Logistic regression model summary table for time and gender
|
Estimate |
SE |
z value |
Pr(>|z|) |
(Intercept) |
−2.54 |
0.38 |
−6.70 |
0.00 |
Gender (female) |
−0.39 |
0.49 |
−0.79 |
0.43 |
Time (post) |
2.38 |
0.33 |
7.21 |
0.00 |
Gender × time (female, post) |
0.05 |
0.43 |
0.12 |
0.90 |
Effects of training conditions
We next addressed the effect of training condition. With time and condition in the model, we found no significant interaction (χ2(1) = 0.15, p = 0.93), and no main effect of condition (χ2(2) = 1.98, p = 0.37). See Table 8 for proportion correct and Table 9 for regression summary table.
Table 8 Mean proportion correct by time and condition
Condition |
Time |
Mean |
SD |
Imagine |
Pre |
0.09 |
0.28 |
Post |
0.41 |
0.49 |
Action |
Pre |
0.15 |
0.36 |
Post |
0.49 |
0.50 |
Gesture |
Pre |
0.08 |
0.27 |
Post |
0.40 |
0.49 |
To reduce the number of dimensions in our model, we converted pre and post test data to a difference score (post–pre). This manipulation allowed us to control for variation in knowledge of stereoisomers before instruction when determining change after exposure to instruction. We could then ask whether male and female participants performed differently as a function of condition. We analysed the simple problems on which the participants had been trained first, followed by the complex problems, which required them to transfer what they had learned from the training.
Looking first at the trained problems, Table 10 shows mean difference scores by gender and condition; Fig. 6 displays box plots in which each individual is represented by a dot. With male imagine set as comparison, there was no statistically significant difference in pretest to posttest change for gender (χ2(1) = 0.53, p = 0.47) or condition (χ2(2) = 0.06, p = 0.97), and no interaction between gender and condition (χ2(2) = 4.60, p = 0.10), AIC = 242.03 on 68 degrees of freedom. Table 11 shows the model summary for change scores. In essence, both female and male students learned, regardless of the type of active engagement training they received.
Table 9 Logistic regression model summary table
|
Estimate |
SE |
t
|
Pr(>|t|) |
(Intercept) |
−3.04 |
0.46 |
−6.57 |
0.00 |
Condition (action) |
0.67 |
0.59 |
1.14 |
0.25 |
Condition (gesture) |
0.08 |
0.62 |
0.13 |
0.90 |
Time (post) |
2.54 |
0.41 |
6.21 |
0.00 |
Time × condition (post, action) |
−0.20 |
0.53 |
−0.38 |
0.70 |
Time × condition (post, gesture) |
−0.14 |
0.55 |
−0.25 |
0.80 |
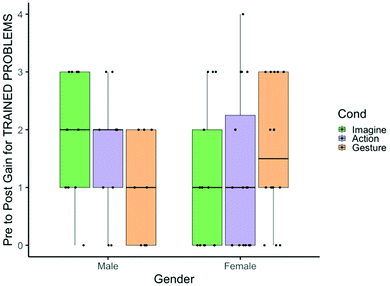 |
| Fig. 6 Change by condition and gender, trained problems. | |
Table 10 Mean pre–post difference by gender and condition, trained problems
Gender |
Cond |
Mean |
SD |
Male |
Imagine |
1.90 |
1.10 |
Action |
1.60 |
1.07 |
Gesture |
1.00 |
0.87 |
Female |
Imagine |
1.08 |
1.26 |
Action |
1.19 |
1.38 |
Gesture |
1.63 |
1.15 |
Finally, we asked how training condition impacted performance on the problems on which the participants had not been trained. Table 12 shows descriptive statistics for transfer problems, and Fig. 7 shows the mean change according to gender and condition. With male imagine set as comparison, there was no statistically significant difference in pretest to posttest change for gender (χ2(1) = 0.002, p = 0.96) or condition (χ2(2) = 1.05, p = 0.47), and no interaction between gender and condition (χ2(2) = 2.46, p = 0.29), AIC = 181.36 on 68 degrees of freedom. Table 13 shows the model summary for change scores. Regardless of the type of active engagement training and gender of the learner, students generalized their learning to new stereoisomer forms not included in the instruction (Tables 12 and 13).
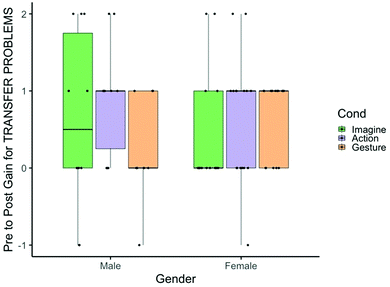 |
| Fig. 7 Change by condition and gender, transfer problems. | |
Table 11 General linear model for change, trained problems
|
Estimate |
SE |
t
|
Pr(>|t|) |
(Intercept) |
1.90 |
0.37 |
5.10 |
0.00 |
Gender (female) |
−0.82 |
0.50 |
−1.66 |
0.10 |
Condition (action) |
−0.30 |
0.53 |
−0.57 |
0.57 |
Condition (gesture) |
−0.90 |
0.54 |
−1.66 |
0.10 |
Gender × condition (female, action) |
0.41 |
0.69 |
0.60 |
0.55 |
Gender × condition (female, gesture) |
1.448 |
0.698 |
2.08 |
0.04 |
Table 12 Mean change by gender and condition, transfer problems
Gender |
Condition |
Mean |
SD |
Male |
Imagine |
0.60 |
1.17 |
Action |
0.90 |
0.74 |
Gesture |
0.22 |
0.67 |
Female |
Imagine |
0.46 |
0.78 |
Action |
0.63 |
0.81 |
Gesture |
0.63 |
0.50 |
Table 13 General linear model for change, transfer problems
|
Estimate |
SE |
t value |
Pr(>|t|) |
(Intercept) |
0.60 |
0.25 |
2.43 |
0.02 |
Gender (female) |
−0.14 |
0.33 |
−0.42 |
0.68 |
Condition (action) |
0.30 |
0.35 |
0.86 |
0.39 |
Condition (gesture) |
−0.38 |
0.36 |
−1.05 |
0.30 |
Gender × condition (female, action) |
−0.14 |
0.46 |
−0.30 |
0.77 |
Gender × condition (female, gesture) |
0.54 |
0.46 |
1.17 |
0.25 |
Discussion
The focus of this study
We compare instructions that require a student to rotate a molecule in 3 different ways: (1) imagined rotation, (2) physical rotation, or (3) gestural rotation. We base our instructional approaches on a body of research arguing that active engagement benefits learners (Active Learning), and that active engagement using the body can be particularly effective (embodied cognition). The choice of gesture as an instructional technique comes from a number of studies suggesting that gesture is a powerful embodied learning strategy, particularly for scientific concepts. Thus, the main question of our study is whether incorporating these three types of active engagement into instruction benefits learning about stereoisomers, and, if so, whether bringing in body movement further improves the learning. In what follows, we consider the impact that these types of instruction have on learning about stereoisomers.
Our project also has a second question. Because gender equity in STEM fields is such a crucial goal, and in light of existing research on spatial differences across women and men, we ask whether the instruction we provide benefits women and men equally.
A brief training can improve understanding of stereoisomers
We asked whether a brief training on stereoisomers led to learning, and found that it did. Given the significant obstacles involved in designing short interventions that are beneficial, this is an important finding. For example, Stieff and colleagues (2014) find that gender differences can be eliminated with training, but that women benefit from training in analytic strategies more than men do. Habig (2020) found that men benefitted more from instruction using augmented reality. In short, it is not straightforward to design a training that benefits all learners.
In all three of our training conditions, students were actively engaged in thinking about or doing the movements of the molecules. Although we cannot directly compare these results to our previous work, it is interesting to note that Ping and colleagues (2021) gave students the same training but without the active component. In that earlier study, where passive rather than active training was used, only 9 of the 52 participants solved any of the problems correctly after training, and none of these participants solved more than 2 of the 6 problems on the posttest correctly. In other words, 17% of Ping et al.'s participants were successful after their training, compared to 30% of our participants; their success rate was 24% of the 6 problems, compared to 57% for our participants. Although statistical comparison is not possible, the difference in effectiveness between the two studies suggests that adding an active component to stereoisomer training may be a promising approach.
Our training builds on work suggesting that active rather than passive engagement facilitates learning spatial concepts that are not detectable to the eye. A useful next step would be to prepare the training we designed for use in classroom teaching and learning. The training would fit nicely into a one-hour lab session, but some streamlining would be necessary to make it feasible for instructors to deliver in different instructional contexts.
We anticipate that augmented and virtual reality (AR/VR) will become a key method for teaching this highly spatial concept. If women and men solve these problems using different strategies, AR may play an important role in closing gaps. A recent study compared AR to traditional representations in teaching stereochemistry (Habig, 2020). Interestingly, this study found that men performed better than women on problems supplemented with AR. However, the study did not incorporate action or gesture. Action and gesture have the potential to be core parts of instruction using augmented reality. Thus, we see our research as providing a useful starting point for the design of AR and VR instruction that uses the body.
Gender differences in understanding stereoisomers are not inevitable
We also asked whether males and females came to the study with different levels of knowledge, and whether they benefited differently from our three kinds of training. We found neither pretest nor posttest differences between male and female students, an encouraging finding in light of previous research that has found gender differences in chemistry performance (e.g., Moss-Racusin et al., 2018).
Our findings indicate that gender differences on this task are not inevitable. Perhaps the impact of negative stereotypes about women and STEM performance is fading. Our findings are encouraging in another sense—training that involves active engagement in rotating the molecules of a potential stereoisomer can lead to equal improvement in male and female students. Previous studies have found gender differences in strategy effectiveness (Habig, 2020; Stieff et al., 2014), but our findings suggest that encouraging the right kind of training may help mitigate gender differences.
Does the type of enactive engagement matter?
Finally, we asked whether our three different kinds of training led to comparable levels of learning, and found that they did. Participants improved the same amount regardless of the type of activity they performed. We chose these trainings because each facilitates the embodiment of molecular rotation and has been shown to be effective in students’ mastery of chemistry, in general, and stereoisomers, in particular.
It is worth noting that, although they did not reach significance, the improvement patterns for males vs. females in our study mirror patterns found in an earlier study in which 4 to 6 year-old children were given instruction in mental rotation (Wakefield et al., 2019a, 2019b). The boys in that study profited equally from instruction to imagine or to gesture the rotation of the object, and more than from instruction to act. In contrast, the girls profited more from instruction to gesture the rotation than from instruction to imagine or to act. The patterns in Fig. 6 show this same trend. Our relatively small sample size may have contributed to the lack of significance of these trends. In addition, the fact that the students volunteered and many participated for course extra credit may have resulted in a highly motivated population. We might have gotten a different result if all students in a particular course had been required to participate. It is also likely that university students, although the appropriate population for a study on undergraduate chemistry instruction, are not representative of the general population. Future research involving larger samples of males and females drawn from different populations would be useful.
We focused here on the benefits of gesture instruction because it a new technique for teaching math and science concepts, and because previous research has suggested that gesturing leads to better transfer than acting (Novack et al., 2014). In contrast to other studies, we did not find an overall benefit of gesture instruction. Although robust effects of producing gesture have been found in the domain of mathematical equivalence, and there is converging evidence for using gesture to teach foreign (e.g., Macedonia, Müller, and Friederici, 2011) and nonsense (Wakefield et al., 2018) words, gesture is not necessarily the superior instructional choice for all concepts and training paradigms. For example, a recent study using gesture to teach brain anatomy also found no benefit of gesture over and above other kinds of training (Parrill et al., submitted).
Our trainings were based on converging research arguing that (1) instruction that actively rather than passively engages students (even if that engagement is mental simulation and not motor action) helps them understand scientific entities undetectable to the naked eye, and (2) using the body during learning can support the acquisition of key competencies, such as representational ability. An extension of this second argument is the finding that gesture can provide a bridge between real actions in the world (rotating an object) and abstract concepts (superimposability) because it allows for the creation of schematized motor representations. However, as noted in our introduction, there are inconsistent findings within each of these bodies of literature. Active learning is not always better than passive learning (Bernstein, 2018). Action doesn’t always lead to improved learning (Steffens et al., 2015), nor does gesture.
In addition, we may have seen no differences across training because determining how to support representational competence through models, actions and imagined action is not straightforward. For example, Fyfe and colleagues’ work (Fyfe et al., 2014; Fyfe et al., 2015) suggests that starting with manipulatives (models) and moving to symbolic representations (called concreteness fading) is particularly helpful. It may be that gesture would work better only after learners have had an opportunity to use models. Future research is needed to determine the conditions under which action and gesture are, and are not, effective teaching tools for this concept.
We may also have seen no effect of different types of training because not all participants improved. The fact that the gain was zero for many participants may reflect the difficulty of the concept, or the training itself. A condition that used a more standard lesson would be needed to tease these possibilities apart.
Limitations
One limitation of our study was the inability to explore individual differences that may have separated our learners. Spatial ability has been shown to be related to success in chemistry (Stieff and Uttal, 2015; Stieff et al., 2018), and demographic factors including race and handedness may have played a role. Although we did intend to explore the role of spatial ability, our data on this variable were lost for most participants, so we were not able to address this factor. We also did not collect finer grained information about prior knowledge (beyond requiring students to have had one year of study and no formal instruction on stereoisomers). However, because students were randomly assigned to our instructional conditions, we can assume that individual variation was equally dispersed across our conditions.
Another limitation for drawing conclusions about gender differences was our sample size. As suggested earlier, there were some gender differences that echoed previous work but did not reach statistical significance; these trends might have been significant had we used a larger sample size.
Although this experimental design was chosen to target our interest in different active, embodied strategies, it is important to acknowledge that the cognitive processes occurring in these three types of training cannot be entirely separated and cannot be fully captured based on external behavior. For example, a learner who is instructed to mentally rotate the molecule may be imagining herself physically turning it, which is thought to evoke motor activity in premotor areas of the brain (see, e.g., Macedonia, Müller and Friederici, 2011). In short, she is mentally simulating the process a learner in the physical model or gesture conditions is performing. Similarly, a learner who is asked to physically rotate the molecule may also be imagining the rotation. Fairly intensive experimental controls (typically interference tasks, see Wagner et al., 2004) are necessary to ensure that only the desired mental operations are taking place. We therefore see our study as a first step that focuses more on whether students can learn than on adjudicating among models of mental processing. We believe the learners were at least performing mental imagery, action, and gesture in their respective conditions, although they may have been performing more than one.
Finally, although we attempted to provide ecologically valid instructional techniques, we were not comparing these active methods to a more passive lesson, such as one involving animations, whiteboards, or slides. It is possible our training was worse than this kind of more typical lesson. Indeed, we saw no improvement between pre-test and post-test for many participants. This may be because the concept is difficult, because our training was too complicated, or because our brief intervention did not have the depth and breadth of a lesson in chemistry. Future work could compare these strategies to a lesson using slides or animations, and also use different trainings at different points in a learner's understanding. Each intervention may affect learners differently at different points in their learning trajectories. Future research needs to address this possibility. But note that lengthy and complex lessons may be difficult for students to process and sustain focus. Brief instruction emphasizing essential material might be more effective under certain conditions.
Conclusions
Our findings are good news for educators. First, we found that the brief training we designed, all versions of which were grounded in active student engagement (both physical and imagined), led to improved performance on a concept that is crucial for success in organic chemistry, stereoisomers.
Second, we found that women and men students at three different institutions performed equivalently at pretest and benefitted equally from our brief instruction. This finding is consistent with research suggesting that differences in aptitude are not what keep women from entering the STEM “pipeline” (see, e.g., Barr et al., 2008).
Finally, we found that all three types of training involving student engagement were associated with better understanding of stereoisomers, although we did not compare these trainings to a lesson involving animations or slides. However, there may be practical advantages to using gesture (as opposed to action, imagination, or animations) to engage students in the lesson. Having a model that students can manipulate, one for each member of the class, can become expensive. Moreover, instructing students on rotating the molecules in the model could itself become a production that gets in the way of students understanding the principle of rotation (see Novack et al., 2014, for discussion). In contrast, for those students who have use of their hands, getting them to focus on how their hands are moving could highlight the rotation principle (Stieff et al., 2016). Unlike models, gesture can be used during an exam, which has the potential to promote transfer of lessons learned during training to the test.
Along the same lines, asking students to imagine moving the molecules does seem to work, particularly for men (Stieff et al., 2014). But making sure that a class full of students is actually doing the imagining could complicate a teacher's task. In contrast, it is easy to see when students are gesturing and it is even possible to correct their gestures if they are making incorrect movements.
For adults learning complex spatial concepts, any instructional technique that concretizes spatial events not visible to the naked eye (like biological, chemical, matter and energy events) may improve understanding (see, e.g., Castro-Alonso et al., 2019). Gesture may not provide a unique advantage, but it can be easily employed during verbal explanations of understanding when physical models are not available. Gesture is a representation that is portable and less context-reliant, and thus offers an important alternative instructional technique.
Our data suggest that instructors ought to make use of active participation by their students in classes focusing on spatial concepts like stereoisomers. In addition, instructors ought to consider using gesture as an economical and easily monitored tool that can help all students in undergraduate chemistry courses.
Author contributions
Ping: conceptualization, methodology, investigation, writing – review and editing, formal analysis, data curation. Parrill: investigation, resources, supervision, writing – original draft. Church: writing – review and editing, project administration. Goldin-Meadow: conceptualization, methodology, resources, writing – review and editing supervision, project administration, funding acquisition.
Conflicts of interest
There are no conflicts to declare.
Acknowledgements
We wish to thank Mike Stieff for guidance on stimuli and assistance with recruitment and data collection at UIC, Rekha Srinivasan and Marisha Kazi for assistance with data collection at CWRU, and Aarati Singh and Kristel Dupaya for assistance with data collection and coding. This research was supported by funding from NSF (SBE 0541957) to the Spatial Intelligence and Learning Center (Goldin-Meadow was a co-PI).
References
- Abels S., (2016), The role of gestures in a teacher-student-discourse about atoms, Chem. Educ. Res. Pract., 17(3), 618–628.
- Adolph K. E., Karasik L. B. and Tamis-LeMonda C. S., (2010), Using social information to guide action: Infants’ locomotion over slippery slopes, Neural Networks, 23(8–9), 1033–1042 DOI:10.1016/j.neunet.2010.08.012.
- Alibali M. W., Flevares L. M. and Goldin-Meadow S., (1997), Assessing knowledge conveyed in gesture: Do teachers have the upper hand? J. Educ. Psychol., 89, 183–193.
- Avargil S., (2019), Learning chemistry: Self-efficacy, chemical understanding, and graphing skills, J. Sci. Educ. Technol., 28, 285–298.
- Barr D. A., Gonzalez M. E. and Wanat S. F., (2008), The leaky pipeline: Factors associated with early decline in interest in premedical studies among underrepresented minority undergraduate students, Acad. Med., 83, 503–511.
- Bates D., Maechler M., Bolker B. and Walker S., (2015), Fitting linear mixed-effects models using lme4, J. Stat. Softw., 67.
- Bernstein D. A., (2018). Does active learning work? A good question, but not the right one, Scholarship Teach. Learn. Psychol., 4(4), 290–307 DOI:10.1037/stl0000124.
- Bransford J. J., Brown A. L. and Cocking R. R., (2000), How people learn: Brain, mind, experience, and school, Washington: National Academy Press.
- Broaders S. C., Cook S. W., Mitchell Z. and Goldin-Meadow S., (2007), Making children gesture brings out implicit knowledge and leads to learning, J. Exp. Psychol.: Gen., 136, 539–550.
- Brom C., Dechterenko F., Frollová N., Stárková T., Bromová E. and D’Mello S. K., (2017). Enjoyment or involvement? Affective-motivational mediation during learning from a complex computerized simulation, Comput. Educ., 114, 236–254 DOI:10.1016/j.compedu.2017.07.001.
- Bruner J. S., (1966), Toward a Theory of Instruction, Cambridge, MA: Belkapp Press.
- Cameron J. J. and Stinson D. A., (2019). Gender (mis)measurement: Guidelines for respecting gender diversity in psychological research, Soc. Person. Psychol. Compass, 13(11), e12506 DOI:10.1111/spc3.1250613:e12506.
- Carlson C., Jacobs S., Perry M. and Church R. B., (2014), The effect of gestured instruction on the learning of physical causality problems, Gesture, 14, 26–45.
- Castro-Alonso J. C., Paas F. and Ginns P., (2019), Embodied cognition, science education, and visuospatial processing, in J. C. Castro-Alonso (ed.), Visuospatial Processing for Education in Health and Natural Sciences, Springer, pp. 175–205.
- Ceci S. J., Williams W. M. and Barnett S. M., (2009), Women's underrepresentation in science: Sociocultural and biological considerations, Psychol. Bull., 135, 218–261.
- Cherney I., (2008). The effects of active learning on students’ memories for course content, Active Learn. High. Educ., 9, 2 DOI:10.1177/1469787408090841.
- Cheryan S., Ziegler S. A., Montoya A. K. and Jiang L., (2017), Why are some STEM fields more gender balanced than others? Psychol. Bull., 143, 1–35.
- Cook S. W., Yip T. K. and Goldin-Meadow S., (2010), Gesturing makes memories that last, J. Memory Lang., 63, 465–475.
- Corra M., Carter J. S. and Carter S. K., (2011), The interactive impact of race and gender on high school advanced course enrollment, J. Negro Educ., 80, 33–34.
- DeSutter D. and Stieff M., (2020), Designing for spatial thinking in stem: Embodying perspective shifts does not lead to improvements in the imagined operations, in Proceedings of the 14th International Conference of the Learning Sciences (ICLS) 2020, Nashville, TN.
- Faul F., Erdfelder E., Buchner A. and Lang A. G., (2009), Statistical power analyses using G*Power 3.1: Tests for correlation and regression analyses, Behav. Res. Methods, 41(4), 1149–1160 DOI:10.3758/BRM.41.4.1149.
- Feng J., Spence I. and Pratt J., (2007), Playing an action video game reduces gender differences in spatial cognition, Psychol. Sci., 18, 850–855.
- Flood V. J., Amar F. G., Nemirovsky R., Harrer B. W., Bruce M. R. M. and Wittmann M. C., (2015), Paying attention to gesture when students talk chemistry: Interactional resources for responsive teaching, J. Chem. Educ., 92, 11–22.
- Fox J. and Weisberg S., (2019), An R Companion to Applied Regression, Thousand Oaks, CA: SAGE.
- Freeman S., O’Connor E., Parks J. W., Cunningham M., Hurley D., Haak D. and Wenderoth M. P., (2007), Prescribed active learning increases performance in introductory biology, Cell Biol. Educ.: Life Sci. Educ., 6(132–139) DOI:10.1187/cbe.06-09-0194.
- Freeman S., Eddy S. L., McDonough M., Smith M. K., Okoroafor N., Jordt H. and Wenderoth M. P., (2014), Active learning increases student performance in science, engineering, and mathematics, Proc. Natl. Acad. Sci. U. S. A., 111, 8410–8415 DOI:10.1073/pnas.1319030111.
- Fuller K. A., Karunaratne N. S., Naidu S., Exintaris B., Short J. L., Wolcott M. D. and White P. J., (2018), Development of a self-report instrument for measuring in-class student engagement reveals that pretending to engage is a significant unrecognized problem, PLoS One, 13(10), e0205828 DOI:10.1371/journal.pone.0205828.
- Fyfe E. R., McNeil N. M., Son J. Y. and Goldstone R. L., (2014). Concreteness fading in mathematics and science instruction: A systematic review, Educ. Psychol. Rev., 26, 9 DOI:10.1007/s10648-014-9249-3.
- Fyfe E. R., McNeil N. M. and Borjas S., (2015), Benefits of “concreteness fading” for children's mathematics understanding, Learn. Instruct., 35, 104–120 DOI:10.1016/j.learninstruc.2014.10.004.
- Gentner D., Levine S. C., Ping R., Isaia A., Dhillon S., Bradley C. and Honkeg G., (2016). Rapid learning in a children's museum via analogical comparison. Cogn. Sci., 40, 224–240.
- Gibbons R. E. and Raker J. R., (2019), Self-beliefs in organic chemistry: Evaluation of a reciprocal causation, cross-lagged model, J. Res. Sci. Teach., 56, 598–618.
- Gibbons R. E., Xu X., Villafañe S. M. and Raker J. R., (2018), Testing a reciprocal causation model between anxiety, enjoyment and academic performance in postsecondary organic chemistry, Educational Psychologist, 38, 838–856.
- Gibbs R., (2005), Embodiment and Cognitive Science, Cambridge: Cambridge University Press.
- Glenberg A., (2008), Embodiment for education, in Calvo P. and Gomila A. (ed.), Handbook of Cognitive Science: An Embodied Approach, London: Elsevier Science, pp. 355–371.
- Gohel D., (2020), Flextable: Functions for tabular reporting, R package version 0.5.9.
- Goldin-Meadow S., Cook S. W. and Mitchell Z. A., (2009), Gesturing gives children new ideas about math, Psychol. Sci., 20(3), 267–272 DOI:10.1111/j.1467-9280.2009.02297.x.
- Guay R. and McDaniels E., (1976), The visualization of viewpoints, The Purdue Research
Foundation.
- Habig S., (2020). Who can benefit from augmented reality in chemistry? Sex differences in solving stereochemistry problems using augmented reality, Br. J. Educ. Technol., 51(3), 629–644 DOI:10.1111/bjet.12891.
- Hostetter A. B. and Alibali M. W., (2008), Visible embodiment: Gesture as simulated action, Psychon. Bull. Rev., 15, 495–514.
- Kelly S. D., McDevitt T. and Esch M., (2009), Brief training with co-speech gesture lends a hand to word learning in a foreign language, Lang. Cogn. Proc., 24, 313–334.
- Kolb A. Y. and Kolb D. A., (2005), Learning styles and learning spaces: Enhancing experiential learning in higher education, Acad. Manage. Learn. Educ., 4(2), 193–212.
- Lee S., Crane B. R., Ruttledge T., Guelce D., Yee E. F., Lenetsky M., Caffrey M., De Ath Johnsen W., Lin A., Lu S., Rodriguez M.-A., Wague A. and Wu K., (2018), Patching a leak in an R1 university gateway STEM course, PLoS One, 13.
- Lenth R., (2020), Emmeans: Estimated Marginal Means, aka Least-Squares Means, R package version 1.4.6.
- Liu C. H. and Matthews R., (2005). Vygotsky's philosophy: Constructivism and its criticisms examined. Int. Educ. J., 6(3), 386–399.
- Lopez E. J., Nandagopal K., Shavelson R. J., Szu E. and Penn J., (2013), Self-regulated learning study strategies and academic performance in undergraduate organic chemistry: An investigation examining ethnically diverse students, J. Res. in Sci. Teach., 50, 660–676.
- Macedonia M. and von Kriegstein K., (2012), Gestures enhance foreign language learning, Biolinguistics, 6, 393–416.
- Macedonia M., Müller K. and Friederici A. D., (2011), The impact of iconic gestures on foreign language word learning and its neural substrate, Hum. Brain Map., 32, 982–998.
- Matson J., (2013), Women are earning greater share of STEM degrees, but doctorates remain gender skewed, Sci. Am., 308.
- McNeill D., (1992), Hand and Mind: What Gestures Reveal about Thought, Chicago: University of Chicago Press.
- Michl J., (2003), Organic chemical systems, theory, in Meyers R. A. (ed.), Encyclopedia of Physical Science and Technology (Third Edition), Tarzana, California: Academic Press, pp. 435–457.
- Moss-Racusin C. A., Sanzari C., Caluori N. and Rabasco H., (2018), Gender bias produces gender gaps in STEM engagement, Sex Roles: J. Res., 79, 651–670.
- Nathan M. J., (2021), Foundations of Embodied Learning: A Paradigm for Education, Routledge.
- National Science Foundation, (2019), Women, minorities, and persons with disabilities in science and engineering.
- Niedenthal P. M., Barsalou L. W., Winkielman P., Krauth-Gruber S. and Ric F., (2005). Embodiment in attitudes, social perception, and emotion, Person. Soc. Psychol. Rev., 9(3), 184–211.
- Novack M. and Goldin-Meadow S., (2015). Learning from gesture: How our hands change our minds, Educ. Psychol. Rev., 27(3), 405–412.
- Novack M. A., Congdon E. L., Hemani-Lopez N. and Goldin-Meadow S., (2014), From action to abstraction: Using the hands to learn math, Psychol. Sci., 25, 903–910.
- Osgood-Campbell E., (2015). Investigating the educational implications of embodied cognition: A model interdisciplinary inquiry in mind, brain, and education curricula, Mind, Brain Educ., 9(1), 3–9.
- Parrill F., (2020). Using cognitive science to teach cognitive science: Embodied teaching and learning in the cognitive science classroom, Scholarship Teach. Learn. Psychol., online first DOI:10.1037/stl0000196.
- Parrill F., Wagner Cook S. and Shymanskyi J., (submitted), Using the hands to learn about the brain: Testing action-based instruction in brain anatomy.
- Peters M., Manning J. T. and Reimers S., (2007), The effects of sex, sexual orientation, and digit ratio (2D:4D) on mental rotation performance, Arch. Sex. Behav., 36, 251–260.
- Piaget J., (1952), The Origins of Intelligence in Children (M. Cook, Trans.), New York: International Universities Press.
- Ping R. and Goldin-Meadow S., (2008), Hands in the air: Using ungrounded iconic gestures to teach children conservation of quantity, Dev. Psychol., 44, 1277–1287.
- Ping R., Church R. B., Decatur M.-A., Larson S. W., Zinchenko E. and Goldin-Meadow S., (2021), Unpacking the gestures of chemistry learners: What the hands tell us about correct and incorrect conceptions of stereochemistry, Discourse Processes, 58, 213–232.
- Pinheiro J., Bates D., DebRoy S. and Sarkar D., (2015), Nlme: linear and nonlinear mixed effects models, R Package Version 3.1-147.
- Pribyl J. R. and Bodner G. M., (1987), Spatial ability and its role in organic chemistry: A study of four organic courses, J. Res. Sci. Teach., 24, 229–240.
- Raker J. R., Gibbons R. E. and Cruz R. d A. D., (2019), Development and evaluation of the organic chemistry-specific Achievement Emotions Questionnaire (AEQ-OCHEM), J. Res. Sci. Teach., 56, 163–183.
- Rau M. A. and Herder T., (2021). Under which conditions are physical versus virtual representations effective? Contrasting conceptual and embodied mechanisms of learning. J. Educ. Psychol., 1–23, advance online publication DOI:10.1037/edu0000689.
- R Core Team, (2020), R: A language and environment for statistical computing.
- Revelle W., (2019), Psych: Procedures for personality and psychological research, Software.
- Rieber R. W., Carton A. S. and Carton A. S., (1987), The Collected Works of L. S. Vygotsky, New York: Plenum PressRieber, RW.
- Scrimin S. and Mason L., (2015). Does mood influence text processing and comprehension? Evidence from an eye-movement study, Br. J. Educ. Psychol., 85, 387–406 DOI:10.1111/bjep.12080.
- Shapiro L. and Stolz S. A., (2019), Embodied cognition and its significance for education, Theor. Res. Educ., 17(1), 19–39 DOI:10.1177/1477878518822149.
- Simpkins S. D., Price C. D. and Garcia K., (2015), Parental support and high school students’ motivation in biology, chemistry, and physics: Understanding differences among Latino and Caucasian boys and girls, J. Res. Sci. Teach., 52, 1386–1407.
- Steen-Utheim A. T. and Foldnes N., (2018), A qualitative investigation of student engagement in a flipped classroom, Teach. High. Educ., 23(3), 307–324 DOI:10.1080/13562517.2017.1379481.
- Steffens M. C., Stülpnagel R. V. and Schult J. C., (2015), Memory recall after “learning by doing” and “learning by viewing”: Boundary conditions of an enactment benefit, Front. Psychol., 6(1907), 1–10 DOI:10.3389/fpsyg.2015.01907.
- Stevanoni E. and Salmon K., (2005), Giving memory a hand: Instructing children to gesture enhances their recall, J. Nonverbal Behav., 29, 217–233.
- Stieff M. and Uttal D., (2015), How much can spatial training
improve STEM achievement? Educ. Psychol. Rev., 27, 607–615.
- Stieff M., Dixon B. L., Ryu M., Kumi B. C. and Hegarty M., (2014), Strategy training eliminates sex differences in spatial problem solving in a STEM domain, J. Educ. Psychol., 106, 390–402.
- Stieff M., Lira M. E. and Scopelitis S., (2016), Gesture supports spatial thinking in STEM, Cogn. Instruct., 34, 80–99.
- Stieff M., Origenes A., DeSutter D., Lira M. E., Banevicius L., Tabang D. and Cabel G., (2018), Operational constraints on the mental rotation of STEM representations, J. Educ. Psychol., 110(8), 1160.
- Stieff M., Werner S., DeSutter D., Franconeri S. and Hegarty M., (2020), Visual chunking as a strategy for spatial thinking in STEM, Cognitive Research: Principles and Implications, 5, 1–15.
- Stull A. T. and Hegarty M., (2016), Model manipulation and learning: Fostering representational competence with virtual and concrete models, J. Educ. Psychol., 108, 509–527.
- Stull A. T., Fiorella L., Gainer M. J. and Mayer R. E., (2018), Using transparent whiteboards to boost learning from online STEM lectures, Comput. Educ., 120, 146–159.
- Sunny C. E., Taasoobshirazi G., Clark L. and Marchand G., (2017), Stereotype threat and gender differences in chemistry, Instruct. Sci., 45, 157–175.
- Uttal D. H., Meadow N. G., Tipton E., Hand L. L., Alden A. R., Warren C. and Newcombe N. S., (2013). The malleability of spatial skills: A meta-analysis of training studies, Psychol. Bull., 139, 352–402 DOI:10.1037/a0028446.
- Vandenberg S. G. and Kuse A. R., (1978). Mental rotations, a group test of three-dimensional spatial visualization, Perceptual Motor Skills, 47, 599–604.
- Vygotsky L. S., (1938), Mind in Society: The Development of Higher Psychological Processes, Cambridge, MA: Harvard University Press.
- Vygotsky L. S., (1986), Thought and Language, Cambridge, Mass.: MIT Press.
- Wagner S., Nusbaum H. and Goldin-Meadow S., (2004). Probing the mental representation of gesture: Is handwaving spatial? J. Mem. Lang., 50, 395–407 DOI:10.1016/j.jml.2004.01.002.
- Wakefield E., Hall C., James J. and Goldin-Meadow S., (2018), Gesture for generalization: Gesture facilitates flexible learning of words for actions on objects, Dev. Sci., 21(5), 1–14 DOI:10.1111/desc.12656.
- Wakefield E., Congdon E. L., Novack M. A., Goldin-Meadow S. and James K. H., (2019a), Learning math by hand: The neural effects of gesture-based instruction in 8-year-old children, Attention, Percept., Psychophys., 81(7), 2343–2353.
- Wakefield E. M., Foley A. E., Ping R., Villarreal J. N., Goldin-Meadow S. and Levine S. C., (2019b), Breaking down gesture and action in mental rotation: Understanding the components of movement that promote learning, Dev. Psychol., online first DOI:10.1037/dev0000697.
- Wickham H., (2016), Ggplot2: Elegant Graphics for Data Analysis, New York: Springer-Verlag.
- Wickham H. and Bryan J., (2019), Readxl: Read Excel files, R package version 1.3.1.
- Wickham H. and Henry L., (2020), Tidyr: Tidy messy data, R package version 1.0.2.
- Wickham H. and Miller E., (2019), Haven: Import and export ‘SPSS’, ‘Stata’ and ‘SAS’ Files.
- Wickham H., François R., Henry L. and Müller K., (2020), Dplyr: A grammar of data manipulation, R package version 0.8.5.
- Young M. S., Robinson S. and Alberts P., (2009), Students pay attention! Combating the vigilance decrement to improve learning during lectures, Act. Learn. High. Educ., 10(1), 41–55 DOI:10.1177/1469787408100194.
- Xie Y., (2020), knitr: A general-purpose package for dynamic report generation in R, R package version 1.28.
- Zwaan R. A., Stanfield R. A. and Yaxley R. H., (2002). Language comprehenders mentally represent the shapes of objects, Psychol. Sci., 136, 168–171.
|
This journal is © The Royal Society of Chemistry 2022 |
Click here to see how this site uses Cookies. View our privacy policy here.