Development of the Assessment of Student Knowledge of Green Chemistry Principles (ASK-GCP)
Received
25th October 2021
, Accepted 14th February 2022
First published on 15th February 2022
Abstract
As implementation of green chemistry into university-level courses increases, it is vital that educators have a tool to rapidly measure student knowledge of green chemistry principles. We report the development of the Assessment of Student Knowledge of Green Chemistry Principles (ASK-GCP) and evaluation of its sensitivity and effectiveness for measuring student knowledge of green chemistry. The 24-item true–false instrument was given to a total of 448 students to gather data on the reliability, validity, and sensitivity. The instrument proved to be sensitive for distinguishing known groups with various levels of green chemistry knowledge and instructional exposure. The instrument was able to detect gains in green chemistry knowledge in pre- and post- conditions. Psychometric analysis revealed that the item difficulty range matches the sample ability range. The findings verified that the ASK-GCP is an efficient and accurate instrument to measure student knowledge of green chemistry principles.
Introduction
Following a recent call to implement more green chemistry into instruction, chemistry instructors can find many examples for how to introduce green chemistry within their curriculum or outreach activities (Andraos and Dicks, 2012; Zuin et al., 2021). This integration of green chemistry into the curriculum is in alignment with the Anchoring Concepts Content Map, which has been adapted to incorporate green chemistry concepts (Holme et al., 2020). Within chemistry courses, green chemistry has been implemented in lectures (Heaton et al., 2006), laboratories (Galgano et al., 2012), outreach activities (Cannon et al., 2020), and service-learning courses (Lasker, 2019). For current reviews of green chemistry teaching and education, refer to Savec and Mlinarec (2021), Zuin et al. (2021), Marques et al. (2020), and Chen, Jeronen and Wang (2020). Additionally, members of the Green & Sustainable Chemistry Education Module Development Project are currently developing green chemistry modules for undergraduate courses (Green & Sustainable Chemistry Education Module Development Project, 2021). Regardless of the method of implementation, educators need an effective and rapid strategy to assess student knowledge of green chemistry principles. Unfortunately, reports of instruments to assess student knowledge of green chemistry and subsequent evaluations of the reliability and validity of the data obtained from those instruments are missing in the literature.
Since green chemistry principles are the most integrated aspect of green chemistry in current chemistry instruction, we sought to develop an instrument that can be used to measure student knowledge of green chemistry principles. Educating students about green chemistry principles has been shown to increase the depth of student analysis and discussion, promote student innovation, and increase student memory retention (Płotka-Wasylka et al., 2018). These principles have been integrated into the curriculum by focusing on them, either as individual principles or as a cumulative framework to assess a compound or process (Armstrong et al., 2018). The twelve green chemistry principles and their descriptions as outlined by the ACS Green Chemistry Institute (2021) are presented in Box 1.
1. Prevention: It is better to prevent waste than to treat or clean up waste after it has been created. 2. Atom economy: Synthetic methods should be designed to maximize incorporation of all materials used in the process into the final product. 3. Less hazardous chemical syntheses: Wherever practicable, synthetic methods should be designed to use and generate substances that possess little or no toxicity to human health and the environment. 4. Designing safer chemicals: Chemical products should be designed to preserve efficacy of function while reducing toxicity. 5. Safer solvents and auxiliaries: The use of auxiliary substances (e.g., solvents, separation agents, etc.) should be made unnecessary wherever possible and, innocuous when used. 6. Design for energy efficiency: Energy requirements should be recognized for their environmental and economic impacts and should be minimized. Synthetic methods should be conducted at ambient temperature and pressure. 7. Use renewable feedstocks: A raw material or feedstock should be renewable rather than depleting whenever technically and economically practicable. 8. Reduce derivatives: Unnecessary derivatization (use of blocking groups, protection/deprotection, temporary modification of physical/chemical processes) should be minimized or avoided if possible, because such steps require additional reagents and can generate waste. 9. Catalysis: Catalytic reagents (as selective as possible) are superior to stoichiometric reagents. 10. Design for degradation: Chemical products should be designed so that at the end of their function they break down into innocuous degradation products and do not persist in the environment. 11. Real-time analysis for pollution prevention: Analytical methodologies need to be further developed to allow for real-time, in-process monitoring and control prior to the formation of hazardous substances. 12. Inherently safer chemistry for accident prevention: Substances and the form of a substance used in a chemical process should be chosen to minimize the potential for chemical accidents, including releases, explosions, and fires. |
.
True–false instruments for assessment of student knowledge in STEM
Historically, the ACS examinations utilized the multiple true–false format to assess student knowledge (Brandriet et al., 2015). More recently, true–false instruments such as the Nano-Knowledge Instrument (Schönborn et al., 2015) and the Genetic Drift Inventory (Price et al., 2014) have been used to rapidly assess respondent knowledge. Furthermore, modifications to the traditional true–false format include the use of multiple true–false questions (Couch et al., 2018) and the use of systemic true–false questions (Fahmy and Lagowski, 2012).
True–false instruments provide a variety of advantages over other testing formats because they promote quick and accurate scoring, provide reliable data, and require less reading than a comparable multiple choice question (Frisbie and Becker, 1991). Additionally, true–false instruments can be rapidly administered and allow for at least 50% more questions than multiple choice instruments during the same time period (Frisbie, 1974; Oosterhof and Glasnapp, 1974; Frisbie and Becker, 1991). Although true–false items have been shown to exhibit lower discrimination values than multiple choice items, the ability to utilize more items in the same time period compensates for this limitation (Frisbie and Becker, 1991). Further, the reliability and validity of the data collected from true–false items have been shown to be higher when students are instructed not to guess on the questions (Kinney and Eurich, 1933) providing evidence for incorporating an option for students who do not know the answer in formative assessments. Recently, it has been reported that the multiple true–false format that required students to independently evaluate each item in a multiple choice format provided a more accurate view of student knowledge of the topic than a traditional multiple choice question (Couch et al., 2018).
While true–false instruments provide many advantages, there are limitations that need to be considered. One limitation that has been widely discussed is the ability for student guessing to influence the final score. However, this impact greatly decreases as the length of the test increases (Ebel, 1970). Additionally, this impact can be reduced through requiring students to correct false statements so that they are true (McCullough, 1993) or through the use of confidence-weighted true–false tests (Dutke and Barenberg, 2015).
Project goal and research question
The purpose of this study was to develop an instrument that can be rapidly administered and assessed for measuring student knowledge of green chemistry principles (GCP). This paper addresses the development of the Assessment of Student Knowledge of Green Chemistry Principles (ASK-GCP), a 24-item true–false instrument. This study was guided by the following research question: what evidence exists for the validity and reliability of the data produced by the ASK-GCP instrument? The framework utilized to answer this question was adapted from Arjoon et al. (2013) and is illustrated in Fig. 1.
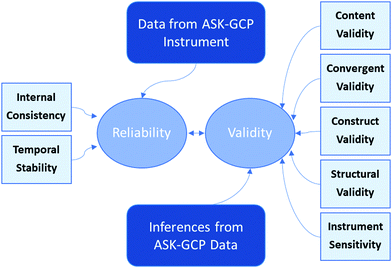 |
| Fig. 1 Framework used in the development of the ASK-GCP instrument. | |
While this assessment can be utilized in any course, it was particularly designed for students in organic chemistry because 53% of the green chemistry instructional activities have been implemented within organic chemistry (Marques et al., 2020). However, despite the frequency of reports on how green chemistry activities were perceived by students, very few report the assessment of student cognitive outcomes. An in-house designed assessment task remains the only way to assess an impact on student learning, weakening an overall inference about the effectiveness of numerous reported curriculum implementations. Therefore, this paper presents the development and evaluation of an assessment measure of green chemistry knowledge for students enrolled in organic chemistry courses. This instrument addressed an important, long-existing void of an easy to administer, score, and make inferences from assessment tool for numerous reported instructional innovations in green chemistry education. It was developed to be suitable for large enrollment classes, effective for all courses, and rapidly implemented and assessed so instructors can easily monitor student progress.
Methods
Instrument development
The instrument was developed over two years with approval from the Institutional Review Board (Protocols # IRB0003546 and SM20271). Students enrolled in the G-OC, GC, and OC courses were informed of the study and provided their consent when completing the instrument. Students enrolled in the major's organic laboratory course were informed of the study at the end of each semester via an e-mail and announcement on the course learning management system after the final grades were posted. Students were asked to e-mail the researchers to opt out of the study if they did not want their submitted course materials used for research purposes.
The initial set of 24 items were chosen from a list of 72 true–false items written by five individuals with experience in green and organic chemistry. Items were developed and formatted using recommendations for true–false items outlined by Thorndike and Thorndike-Christ (2010). The selected items were chosen so that each green chemistry principle is assessed by one item whose correct response was true and one item whose correct response was false.
Students were administered the pilot version of the true–false instrument and subsequently asked to provide feedback using the prompt, “Were any of the statements unclear or confusing to you? If yes, provide examples and explain in the space below.” The use of student feedback to remove linguistically complicated wording is in accordance with findings by Lee and Orgill (2021), which indicated that reducing linguistic complexity of assessment prompts can benefit all students, especially English language learners. Due to student feedback received and analysis of the results, the instrument was revised to remove the technical wording from items 3 and 24 to increase clarity in the statements. Item 3 originally stated, “reactions at elevated temperatures should be prioritized over reactions at ambient temperature,” but was revised to change the term “ambient temperature” to “room temperature.” The resulting item 3 was “reactions at elevated temperatures should be prioritized over reactions at room temperature.” Additionally, item 24 originally stated, “halogenated molecules are structural features that promote facile biodegradation.” It was revised to remove the phrases “facile biodegradation” and “halogenated molecules;” the revised version stated, “organic compounds containing chlorine are easily broken down.” Additionally, to limit the impact of student guessing due to only true and false options, the option “don’t know” was added for the revised version (Schönborn et al., 2015).
Participants, setting and data collection
Study participants were students at a midwestern university who were enrolled in one of three chemistry courses with varying levels of green chemistry integrated into the course curriculum (N = 448). The initial version of the instrument was piloted in an organic chemistry course that integrated green chemistry instruction through green chemistry moments, hereafter referred to as G-OC (N = 85). The green chemistry moments included applications of green chemistry principles to core curriculum concepts of the organic chemistry course. A green chemistry moment was provided via lecture for each chapter of the textbook. An example of a green chemistry moment is a discussion on the replacement of diethyl ether with 2-methyltetrahydrofuran to illustrate an application of green chemistry principles #5 (safer solvents and auxiliaries) and #7 (use of renewable feedstocks).
Subsequently, the revised version of the instrument was administered the following year to students enrolled in either a general chemistry, hereafter referred to as GC (N = 302), or an organic chemistry course, hereafter referred to as OC (N = 61), which were taught by other faculty who were not members of the research team. To ease administration of the instrument, it was administered to students in the corresponding laboratory sections with a typical class size of 24 students. Neither the GC nor OC course explicitly integrated green chemistry instruction into the curriculum which allowed for comparisons between groups. Only students who responded to all questions of the ASK-GCP instrument were included in the analysis. Additionally, it was administered to students enrolled in two semesters of majors’ organic chemistry laboratory (N = 14) to assess reliability and validity of repeated measures of the ASK-GCP data.
The instrument was administered electronically using Qualtrics. Students who completed the instrument were rewarded with nominal extra credit points. For both the initial and revised versions, student responses were scored as correct (1) or incorrect which included the option don’t know (0).
Data analysis
Descriptive statistics included Kuder–Richardson Formula 20 (KR-20), Ferguson's delta, mean, standard deviation and range of scores. Furthermore, analysis of the normality of the distribution of the data, including kurtosis and skewness, was conducted. These analyses were performed for each of the groups using StataIC 16.
Confirmatory factor analysis was conducted using combined data from all three chemistry courses using StataIC 16. Due to the dichotomous nature of the data, response scores for the two questions for each green chemistry principle were summed and used in the analysis. Therefore, the model consisted of one factor, green chemistry knowledge, and 12 indicators representing each of the twelve principles. The model for CFA was identified using unit variance identification.
Item analysis was performed using jMetrik and StataIC 16 to determine item difficulty and discrimination indices. A detailed account describing item analysis in jMetrik is available (Leontyev et al., 2017). After confirming the unidimensionality of the data, Rasch analysis was performed on the combined data collected from the OC and GC course which were combined since neither explicitly taught about green chemistry. A Wright Map was generated in RStudio using the TAM and WrightMap packages. Rasch analysis was conducted using StataIC 16.
Results & discussion
Descriptive statistics
Descriptive statistics were calculated for each group and are shown in Table 1. The possible range of scores was from 0 to 24. The average score for G-OC was 18.51, whereas that for OC was 12.84 and for GC was 10.90. This difference in averages was expected since G-OC was explicitly taught about green chemistry principles in lecture whereas OC was not explicitly taught. Likewise, students in GC chemistry scored the lowest among the respondents since they were not explicitly taught about the principles and had the least amount of chemistry content knowledge.
Table 1 Descriptive statistics from the results of the individual courses and the combined courses
Group |
N
|
M
|
SD |
Range |
KR-20 |
Ferguson's δ |
Students completed the initial version of the survey.
Students completed the revised version of the survey.
|
G-OCa |
85 |
18.5 |
2.4 |
0–24 |
0.496 |
0.904 |
OCb |
61 |
12.8 |
4.2 |
0–19 |
0.786 |
0.948 |
GCb |
302 |
10.9 |
4.4 |
0–21 |
0.789 |
0.972 |
OC & GCb |
363 |
11.0 |
4.5 |
0–21 |
0.792 |
0.972 |
Analysis of the distribution of student scores from all three cohorts (Fig. 2) indicated that neither a ceiling effect nor floor effect was observed since few students scored either a 24 or 0, respectively. Additionally, the almost symmetrical distribution indicated that the data followed an approximately normal distribution. To further analyze the normality of the data, the kurtosis and skewness of the data were calculated. Kurtosis represents whether the data is heavy-tailed or light-tailed in relation to a normal distribution, whereas skewness represents any asymmetry in the distribution of the data. While a normal distribution has a skewness of 0, analysis indicated the data had a skewness of −0.407, indicating that it was slightly skewed to the left. Thus, the mean (M = 12.61) was slightly less than the median (med = 13). Furthermore, a normal distribution would have a kurtosis value of 3, whereas our data indicated a kurtosis of 2.89, suggesting that it had a slightly lighter-tailed distribution than a normal distribution. However, overall, it followed an approximately normal distribution. Therefore, the mean and other descriptive statistics were representative of the data (Mishra et al., 2019). Similarly, Ferguson's δ was calculated for each group and is reported in Table 1 and was determined to be 0.981 overall. Ferguson's δ measures the discriminatory power of a test by comparing the range of observed student scores with the total possible range (Ferguson, 1949; Ding and Beichner, 2009). Ferguson's δ ranges from 0 to 1 and instruments with values greater than 0.9 are generally considered to have good discriminatory power (Ding and Beichner, 2009). The overall Ferguson's δ for all three groups was 0.981, indicating that the sample was distributed over 98.1% of the possible range of total scores (Bretz and Linenberger, 2012).
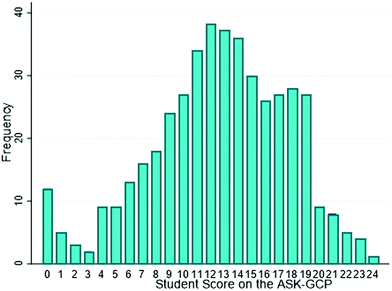 |
| Fig. 2 Frequency of students’ scores on the ASK-GCP from the combined G-OC, OC, and GC courses [n = 448]. | |
Reliability
According to the American Educational Research Association Standards for Educational and Psychological Testing, reliability represents the “consistency of the scores across instances of the testing procedure” (AERA, APA, and NCME, 2014). While a variety of aspects of reliability can be assessed, the ASK-GCP was evaluated for internal consistency and test-retest reliability because they are the most pertinent for this instrument since the internal consistency indicates the degree to which the items measure a common characteristic, in this case green chemistry, and test-retest reliability indicates that any variation in scores is due to learned content; thus indicating the utility of the instrument for assessing student knowledge.
Internal consistency.
Because the ASK-GCP items produced dichotomous data, the Kuder–Richardson formula 20 (KR-20) was utilized to assess the internal consistency reliability. The KR-20 provides an estimate of the internal consistency of a set of items scored dichotomously (correct = 1; incorrect = 0) and therefore indicates the degree to which the items measure a common characteristic and are free from measurement error (Thorndike and Thorndike-Christ, 2010). The KR-20 values for each of the groups are provided in Table 1. Due to homogeneity of the sample and thus lower variance due to all students of the G-OC course correctly answering two of the items, the KR-20 for the G-OC course (0.496) was lower than that for OC (0.786) and GC (0.789) courses. However, overall, the KR-20 values indicate that the instrument exhibits internal consistency. To provide a fuller picture of the evidence supporting reliability, we also calculated its test-retest reliability.
Test–retest reliability.
The test–retest reliability was measured using data collected from students enrolled in both a majors’ organic chemistry I and II laboratory (N = 14); the same students took the ASK-GCP before (M = 17.4, SD = 2.3) and after winter break (M = 17.3, SD = 3.3) as illustrated in Table 4. The average change in an individual's score after the break was −0.43 with a standard deviation of 2.17 which was calculated by taking the average of the differences between individual pre-test 3 and post-test 2 scores. Since instruction on green chemistry was not provided over winter break, we expected the ASK-GCP scores to remain stable. This minimal decrease in scores provides evidence for the temporal stability. Furthermore, Pearson's correlation, r(14) = 0.77, p = 0.0013 indicated that the scores were strongly correlated, thus providing evidence for stability of relative student score ranking.
Validity
According to the American Educational Research Association Standards for Educational and Psychological Testing, validity represents “the degree to which evidence and theory support the interpretations of test scores for proposed uses of tests” (AERA, APA, and NCME, 2014). While a variety of aspects of validity can be assessed, the ASK-GCP was evaluated for content, convergent, construct, and structural validity and sensitivity because they are the most pertinent for this instrument.
Content validity.
Content validity refers to the ability of the instrument's questions to represent the variables of the construct being measured (Zamanzadeh et al., 2015). The content validity was evaluated by organic chemistry instructors who are independent from the instrument development team using the method reported by Polit and Beck (2006). The panel consisted of 10 experts teaching at different institutions with the highest degree in chemistry as associate, bachelors, masters, or doctorate. On average, panel members had 16 years of teaching experience and indicated various levels of integration of green chemistry into organic chemistry instruction. Each item was presented to experts who were asked to rate an item by its relevance to their organic chemistry instruction. Scale content validity index (0.67) was computed as an average of proportions of experts indicating that an item was either quite or highly relevant. Scale relevancy index (0.97) is an average of proportions indicating that an item was either somewhat, quite, or highly relevant. Thus, it was concluded that the instrument was relevant to organic instruction in a variety of instructional settings thus warranting its use to assess green chemistry knowledge.
Convergent validity.
Convergent validity refers to how well an instrument relates with other measures of the same construct (Krabbe, 2017). To evaluate the convergent validity, we calculated the Pearson correlation for the scores obtained by the chemistry majors for the pre- and post-test ASK-GCP with their scores on an open-ended prompt by Armstrong et al. (2019) that asked students to identify up to five factors they would consider when deciding which of two reactions was “greener.” Responses received one point for each correct factor identified. Because some responses included more than one correct factor per provided space, the maximum points assigned was 6 instead of the expected 5. Descriptive statistics from the instrument and the prompt by Armstrong et al. (2019) are illustrated in Table 2. The Pearson correlation indicated that there was a statistically significant moderate correlation between the two scores, r(38) = 0.41, p = 0.0097.
Table 2 Descriptive statistics for the ASK-GCP and Greener Reactions prompts on the pre- and post-tests
Assessment |
N
|
M
|
SD |
Range |
ASK-GCP |
38 |
17.6 |
4.2 |
1–24 |
“Greener” reaction |
38 |
3.31 |
1.3 |
1–6 |
Construct validity.
Construct validity refers to an instrument's ability to collect accurate information and particularly how well the instrument's scores represent a theoretical construct such as green chemistry (Hays and Reeve, 2008). One method of assessing construct validity is to use the known-groups method, which utilizes groups with known differences. The G-OC course actively integrated green chemistry, whereas the OC course did not actively teach green chemistry. However, both courses covered the same chemistry content. Therefore, to assess the construct validity, the scores of the G-OC course (M = 18.51, SD = 2.40) and the OC course (M = 12.84, SD = 4.2) were compared using an independent samples t-test. The results indicated that knowledge of green chemistry and green chemistry principles resulted in a significant increase to the assessment score, t(86) = 9.257, p < 0.001. Furthermore, Cohen's d, the standardized mean difference between pre- and post-test, for this analysis (d = 1.696) indicated that on average student scores were higher by 1.7 standard deviations with green chemistry instruction.
Furthermore, the percent of correct responses provided by students in G-OC and OC for each GCP was compared. As shown in Fig. 3, G-OC outperformed OC on each of the GCP prompts, providing further evidence for the instrument's ability to distinguish student knowledge on green chemistry, not just on the overall score, but also on the individual scores for each of the GCP, again providing further evidence for its construct validity.
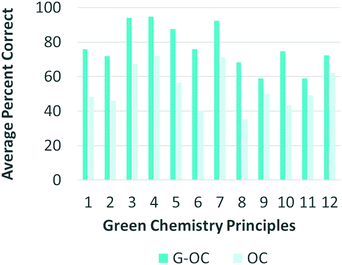 |
| Fig. 3 Percent of students providing correct responses for each GCP. | |
Structural validity.
Structural validity refers to the extent that the instrument's structure correlates with the designed theoretical structure according to the construct being measured (Loevinger, 1957; Wren and Barbera, 2014). Since all the questions were designed to measure green chemistry, the instrument should be unidimensional. Therefore, to assess this we conducted confirmatory factor analysis using data collected from all three courses. Due to the dichotomous nature of the data, scores for each of the two items for each principle were added and the combined score of 0, 1, or 2 for each of the twelve principles was assessed using CFA.
First, the goodness of fit for the model was established using model fit indices and their respective criteria. The comparative fit index (CFI), root mean square error of approximation (RMSEA), and standardized root mean squared residual (SRMR) were calculated. Overall, the one-factor CFA model was found to exhibit a good fit: χ2 (54, N = 448) = 103.09 (p < 0.001), χ2/df = 1.91, CFI = 0.961, RMSEA = 0.045, SRMR = 0.037, therefore, establishing the construct validity of the scores obtained from the ASK-GCP.
After confirming a good model fit, the statistical significance and salience of each of the GCP was assessed and are illustrated in Table 3. All items were found to exhibit statistical significance (p < 0.001) with nine of the twelve GCP exhibiting salience and the remaining three GCP are near salience, thus indicating that most are sufficiently measuring the construct of interest. The three GCP (#9, 11, 12) that approached salience warrant future investigation.
Table 3 Analysis of the significance and salience for each of the GCP indicators. All GCP were found to be significant (p < 0.001) and bolded GCP salient due to standardized loadings greater than 0.30
Indicator |
ASK-GCP item numbers |
Standardized loading |
GCP1
|
13, 17
|
0.354
|
GCP2
|
9, 2
|
0.418
|
GCP3
|
5, 4
|
0.510
|
GCP4
|
1, 20
|
0.417
|
GCP5
|
6, 15
|
0.481
|
GCP6
|
7, 3
|
0.388
|
GCP7
|
14, 8
|
0.401
|
GCP8
|
10, 21
|
0.377
|
GCP9 |
22, 12 |
0.298 |
GCP10
|
23, 24
|
0.459
|
GCP11 |
19, 16 |
0.282 |
GCP12 |
11, 18 |
0.255 |
Instrument sensitivity.
To assess the sensitivity of the instrument, it was administered four times to evaluate two green chemistry instructional interventions, twice as a pre-assessment and twice as a post-assessment, in organic chemistry I and II laboratories for chemistry majors because many of the same students were enrolled in both semesters. While both semesters incorporated student-generated instructional materials, the first semester (Intervention 1) prompted students to create an infographic about a topic that was relevant to both green and organic chemistry, whereas the second semester (Intervention 2) expanded on student knowledge by asking them to create an open educational resource for which they selected one of the key reactions from second semester organic chemistry, found an example of its use in the literature, and evaluated the greenness of the selected reaction in the literature. In both semesters, our goal was to teach students about the green chemistry principles and how green and organic chemistry relate to each other, with an introduction to it in the first semester via creating an infographic and a deeper perspective by applying green chemistry, planetary boundaries, and sustainable development goals to specific examples in the second semester. The implementations of these two interventions are described in detail elsewhere (Grieger and Leontyev, 2021, in press). The ASK-GCP instrument was used each semester to evaluate the effectiveness of the incorporated green chemistry intervention and subsequently evaluated across two semesters for its sensitivity to detect the change. The resultant average scores and learning gains from students enrolled in both semesters are illustrated in Table 4. The average learning gain for each intervention was calculated by taking the average of the differences between individual post- and pre-test scores.
Table 4 Average class score for each of the four assessments
|
Intervention 1 (N = 15) |
Intervention 2 (N = 14) |
Pre-test 1 |
Post-test 2 |
Gain 1a |
Pre-test 3 |
Post-test 4 |
Gain 2a |
While Gain 1 was statistically significant (p = 0.019), Gain 2 was not statistically significant (p = 0.916).
|
Average |
13.9 |
17.4 |
3.8 |
17.3 |
18.4 |
1.0 |
SD |
3.9 |
2.3 |
4.4 |
3.3 |
2.7 |
3.0 |
A one-way repeated measures ANOVA was used to assess the statistical significance of the impact of the two interventions and the ability of the instrument to detect the change. While a statistically significant difference was observed, F(3, 40) = 8.62, p = 0.0016, the post hoc analysis indicated significant differences between pre-test 1 and post-test 2, pre-test 1 and pre-test 3, and pre-test 1 and post-test 4. There was no significant difference measured between pre-test 3 and post-test 4. Due to the small sample size consisting of less than 20 participants, Hedges g, which is the unbiased standardized mean difference between pre- and post-test, was used to calculate the effect size for each of the learning gains (Lakens, 2013). The effect size of the learning gain for Intervention 1 (g = 1.06) indicated that on average student scores increased by one standard deviation upon completion of the activity. However, the effect size of the learning gain for Intervention 2 (g = 0.35) indicated that on average student scores only increased by approximately one-third of a standard deviation. Thus, the non-significance of the learning gain for Intervention 2 can be attributed to the smaller effect size which is likely due to the students having prior exposure to the green chemistry principles in the first semester.
Psychometric analysis using classical test theory
Classical test theory (CTT) is often referred to as the true score theory. It asserts that the observed test score is a composite of the true score and the error score (Magno, 2009). CTT has been widely used over the last century to improve exam items due to its simplicity, convenience, and accessibility (Fan, 1998; Kline, 2005; Progar and Sočan, 2008). Within CTT, the item parameters, including item difficulty and discrimination, are specific to a given examinee population (Bichi et al., 2019), therefore the difficulty and discrimination values for each course are listed in Table 5.
Table 5 Item difficulty and discrimination values generated from the results of each course with indicated items classified as extremely easy or hard
Item |
Difficulty |
Discrimination |
G-OC |
OC |
GC |
OC&GC |
G-OC |
OC |
GC |
OC&GC |
Items classified as extremely easy due to item difficulty indices greater than 0.91.
Items classified as extremely difficult due to item difficulty indices less than 0.24.
These discrimination indices could not be calculated since all students correctly answered the prompt for the item.
|
1 |
0.98a |
0.80 |
0.74 |
0.75 |
0.13 |
0.48 |
0.33 |
0.37 |
2 |
0.49 |
0.26 |
0.20b |
0.21b |
0.11 |
0.23 |
0.28 |
0.27 |
3 |
0.76 |
0.34 |
0.31 |
0.32 |
0.21 |
0.20 |
0.23 |
0.22 |
4 |
0.92a |
0.52 |
0.36 |
0.39 |
0.03 |
0.35 |
0.41 |
0.41 |
5 |
0.96a |
0.82 |
0.69 |
0.71 |
0.24 |
0.54 |
0.43 |
0.45 |
6 |
1.00a |
0.87 |
0.64 |
0.67 |
NAc |
0.47 |
0.43 |
0.45 |
7 |
0.75 |
0.46 |
0.52 |
0.51 |
0.17 |
0.33 |
0.31 |
0.30 |
8 |
0.89 |
0.79 |
0.72 |
0.73 |
0.33 |
0.51 |
0.32 |
0.35 |
9 |
0.94a |
0.66 |
0.35 |
0.40 |
−0.09 |
0.29 |
0.31 |
0.33 |
10 |
0.73 |
0.30 |
0.27 |
0.28 |
0.06 |
0.29 |
0.24 |
0.23 |
11 |
0.91a |
0.75 |
0.78 |
0.78 |
−0.19 |
0.19 |
0.30 |
0.27 |
12 |
0.28 |
0.21b |
0.19b |
0.20b |
0.38 |
0.10 |
0.27 |
0.24 |
13 |
1.00a |
0.77 |
0.71 |
0.72 |
NAc |
0.25 |
0.43 |
0.40 |
14 |
0.95a |
0.64 |
0.65 |
0.65 |
0.12 |
0.48 |
0.43 |
0.43 |
15 |
0.75 |
0.26 |
0.22b |
0.23b |
0.07 |
0.35 |
0.31 |
0.32 |
16 |
0.26 |
0.16b |
0.15b |
0.15b |
0.09 |
0.13 |
0.20 |
0.17 |
17 |
0.52 |
0.20b |
0.23b |
0.22b |
0.14 |
0.00 |
0.21 |
0.19 |
18 |
0.54 |
0.49 |
0.28 |
0.32 |
0.21 |
0.13 |
0.20 |
0.20 |
19 |
0.92a |
0.82 |
0.73 |
0.75 |
0.17 |
0.52 |
0.44 |
0.46 |
20 |
0.92a |
0.64 |
0.60 |
0.61 |
0.22 |
0.46 |
0.37 |
0.39 |
21 |
0.64 |
0.41 |
0.18b |
0.22b |
0.89 |
0.33 |
0.21 |
0.26 |
22 |
0.89 |
0.79 |
0.61 |
0.64 |
0.14 |
0.37 |
0.30 |
0.31 |
23 |
0.99a |
0.66 |
0.52 |
0.55 |
0.21 |
0.53 |
0.46 |
0.48 |
24 |
0.51 |
0.21b |
0.25b |
0.25b |
0.23 |
0.30 |
0.30 |
0.27 |
Item difficulty refers to the proportion of respondents who correctly answered the item. Thus, within CTT, items with high item difficulty values indicate an easy item since a larger proportion of respondents correctly answered the item, whereas items with low difficulty values indicate more difficult items. In terms of item difficulty, items with a difficulty of 0 or 1 exhibit no discrimination because all students either correctly or incorrectly answered the item (Kline, 2005). This was observed for items 6 and 13 in the G-OC course because all students correctly answered those items, indicating a ceiling effect of those items after green chemistry instruction. Although a ceiling effect was observed, it indicated the ability of the instrument to measure student competencies upon completion of green chemistry instruction. Item difficulty indices under 0.24 can be considered as extremely difficult and item difficulty indices greater than 0.91 can be considered extremely easy (Downing and Yudkowsky, 2009; Lahner et al., 2018). While 11 items were classified as extremely easy for the G-OC course, no items were classified as extremely easy for the OC or GC courses. Similarly, no items were classified as extremely difficult for the students in the G-OC course; however, four items were classified as extremely difficult for students in the OC course and seven items were classified as extremely difficult for students in the GC course. Thus, the results of this psychometric analysis provided additional evidence for the instrument's construct validity.
The discrimination index refers to an item's ability to distinguish between individuals without reference to an external criterion (Hankins, 2008). Item discrimination was traditionally calculated by comparing the item difficulty of the highest achieving students on the test to that of the lowest achieving students for each of the items. However, it is now typically calculated as a point-biserial correlation, which is the correlation between the item score and total test score (Meyer, 2014). Items with higher discrimination indexes have a greater percentage of high achieving students than lower achieving students answering the question correctly. Likewise, items with a negative value for their discrimination value indicate a greater portion of lower achieving students than high achieving students correctly answering the item (Kline, 2005). Values close to zero indicate no discrimination (Meyer, 2014). A slight inversion was observed for items 9 and 11 for the G-OC course. However, while they were inverted both exhibited discrimination values close to zero indicating minimal discrimination and had a difficulty of greater than 0.9, indicating most students in the class answered the items correctly. Point-biserial correlations less than 0.20 indicate the item can benefit from revision, between 0.20 and 0.30 indicate the item is fair, and those between 0.40 and 0.70 are considered good (McGahee and Ball, 2009). As shown in Table 5, most of the discrimination indexes indicated fair to good items for the OC and GC courses. However, lower values were observed in the G-OC course due to the ceiling effect which limited their ability to psychometrically perform well.
Comparing difficulty for true–false items.
Through an analysis of item difficulty, it was observed that students appeared to score higher on true statements in which the correct response is to indicate true than on false statements in which the correct response is false. Thus, to determine if a statistically significant difference was observed, an unpaired sample t-test was conducted to compare the scores of the true statements (M = 0.64, SD = 0.15) to that of the false statements (M = 0.41, SD = 0.14). The results indicated that students were significantly more likely to identify true statements as true than false statements as false, t(22) = 3.96, p = 0.0007. Furthermore, Cohen's d, the standardized mean difference between pre- and post-test, for this analysis (d = 1.55) indicated that on average students scored about one and a half standard deviations higher on the true statements than on the false statements. This tendency to mark a statement as true is consistent with findings by Fritz (1927). More recently, this effect was also observed on the Nano-Knowledge Instrument (Schönborn et al., 2015). Using data provided in their paper, we performed an unpaired sample t-test to compare the scores between the true (M = 0.59, SD = 0.17) and false (M = 0.50, SD = 0.21) statements. The results indicated the difference was not statistically significant, t(26) = 1.31, p = 0.20. However, Cohen's d of 0.50 indicated that, on average, students scored about one-half standard deviations higher on the true statements than on the false statements. Therefore, our findings that the false items were more difficult correlates with results from other papers. Assessing the psychometrical functioning of true versus false statements is an area for further research.
Rasch analysis
Rasch analysis is a statistical procedure and mathematical modeling technique within item response theory that is centered on the existence of a latent trait (Boone, 2016). Rasch analysis has been used extensively in the development and assessment of instruments (Wren and Barbera, 2014; Taskin et al., 2015; He et al., 2016; Lu and Bi, 2016; Nedungadi et al., 2019; Lu et al., 2020; Balabanoff et al., 2021). Rasch analysis is particularly beneficial in the analysis of instruments because it allows for generalization of the results to the greater population, whereas analysis using CTT is sample dependent and thus not generalizable between samples. Furthermore, Rasch modeling has been found to be more effective at detecting learning gains than CTT for instruments containing at least 20 items (Pentecost and Barbera, 2013; Jabrayilov et al., 2016; Sorenson and Hanson, 2021). Thus, to facilitate generalization of the item difficulties the instrument was further analyzed using Rasch analysis.
For Rasch analysis to be performed, the items of the instrument must exhibit unidimensionality, local independence, and monotinicity (Kean et al., 2018). As shown through the CFA analysis, the instrument's items were found to be unidimensional, thus representing the construct of green chemistry. Local independence implies that the items of a test should be unrelated, which means that performance on the item indicates only the respondent's ability and the characteristics of the item (Kean et al., 2018). Monotinicity refers to the correlation between item response and ability, such that greater responses correlate to greater ability (Kean et al., 2018). Since these criteria have been met, Rasch analysis was appropriate for analyzing the data.
The general Rasch model equation used for this analysis is illustrated in the following equation, which expresses the probability of correctly answering an item, Pni(x = 1), as a function of the difference between a person's ability, Bn, and the difficulty of the item, Di (Barbera, 2013). Person ability, Bn, refers to an odds-of-success for any task, whereas item difficulty, Di, refers to an odds-of-failure estimate for any respondent (Ludlow and Haley, 2016).
| 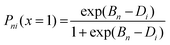 | (1) |
Rasch analysis creates a single linear scale, the logit, for both item difficulty and person ability. Based on the model, the logit is equal to Bn − Di and is defined as the natural log of the odds ratio (Ludlow and Haley, 2016). An item that has average difficulty will have a logit unit of zero since within the Rasch model the mean item difficulty is set to zero logit units (Bond and Fox, 2013; Lutter et al., 2019).
The ability of the model to predict student responses based on ability and difficulty estimates are described by the fit statistics. Through fit statistics, items that produce unexpected student responses or students who provide unexpected answers can be identified (Wren and Barbera, 2014). Therefore, psychometric estimates of the items, including infit mean-square (MNSQ), outfit MNSQ, and item difficulty measures were calculated and are reported in Table 6.
Table 6 Psychometric estimates of ASK-GCP items from Rasch Analysis, including infit and outfit statistics and item difficulty measures
Item |
Infit MNSQa |
Outfit MNSQa |
Item difficulty measureb |
Acceptable range for MNSQ values is 1.00 ± 0.5 (Bond and Fox, 2013; Meyer, 2014; Wren and Barbera, 2014).
The lower the negative number, the easier the item (Wren and Barbera, 2014).
|
1 |
1.02 |
1.01 |
−1.53 |
2 |
0.97 |
0.84 |
1.37 |
3 |
1.06 |
1.11 |
0.73 |
4 |
0.89 |
0.84 |
0.38 |
5 |
0.91 |
0.83 |
−1.26 |
6 |
0.92 |
0.88 |
−1.05 |
7 |
1.06 |
1.06 |
−0.22 |
8 |
1.04 |
1.04 |
−1.38 |
9 |
0.99 |
0.97 |
0.31 |
10 |
1.04 |
1.00 |
0.97 |
11 |
1.10 |
1.22 |
−1.71 |
12 |
0.96 |
1.07 |
1.46 |
13 |
0.97 |
0.95 |
−1.30 |
14 |
0.94 |
0.88 |
−0.91 |
15 |
0.90 |
0.86 |
1.26 |
16 |
0.99 |
1.13 |
1.79 |
17 |
1.04 |
1.03 |
1.29 |
18 |
1.10 |
1.06 |
0.73 |
19 |
0.91 |
0.81 |
−1.49 |
20 |
0.98 |
0.95 |
−0.68 |
21 |
0.98 |
0.88 |
1.31 |
22 |
1.05 |
1.09 |
−0.84 |
23 |
0.87 |
0.82 |
−0.38 |
24 |
0.96 |
0.98 |
1.14 |
Infit and outfit were calculated to measure how well the data fit the Rasch model. Infit and outfit statistics have an expected value of 1 and are always positive in value (Meyer, 2014). Values greater than one indicate underfit because the data contains more variation than expected by the Rasch model; likewise, items with infit and outfit less than one indicate that the responses are too consistent with what is expected from the model. Infit and outfit statistics between 0.5 and 1.5 are considered productive for measurement, whereas a range between 0.8 and 1.2 is recommended for high-stakes tests (Bond and Fox, 2013; Meyer, 2014; Wren and Barbera, 2014). All items exhibited acceptable infit and outfit statistics with ranges of 0.87–1.10 and 0.81–1.22, respectively, thus indicating the items functioned well for students within the ability range of the item.
Furthermore, the item difficulty measure in Rasch analysis indicates how difficult an item is; the lower the negative number, the easier the item (Wren and Barbera, 2014). Thus, the easiest three items were items 11 (GCP 12), 1 (GCP 4), and 19 (GCP 11), respectively, whereas the hardest three items were items 16 (GCP 11), 12 (GCP 9), and 2 (GCP 2), respectively. Surprisingly, GCP 11 was identified as having one of the easiest and hardest items.
Rasch analysis was also used to provide further validation of the ASK-GCP instrument through assessment of its item reliability and person reliability, which is illustrated in Table 7. Item reliability indicates the extent to which items represent a range of difficulty for a single variable; whereas person reliability indicates whether the instrument is able to discriminate across the ability range of the participants (Connor and Shultz, 2018). The item separation reliability (0.99) indicated that the instrument's items represented a single well-defined variable and also provided evidence for the reliability of the location of the items on the scale. Further, it indicated that the local independence assumption for the data was valid (Arias González et al., 2015). A lower person separation (separation index <2, reliability <0.8) was observed, indicating the instrument may not be sensitive enough to distinguish between high and low achievers. However, the reliability was greater than 0.5, indicating that it may be able to discriminate respondents into two levels. This differentiation between groups was observed in both the repeated measures evaluation and between known-groups validation. This lower person separation can be attributed to the selected sample of OC and GC students not being exposed to green chemistry and thus having a narrow range of abilities. However, a good item separation was observed (separation index >3, reliability >0.9), indicating that the person sample was large enough to evaluate the item difficulty hierarchy (Linacre, 2021).
Table 7 Scale quality statistics for the Rasch model
Statistic |
Items |
Persons |
Observed variance |
1.3199 |
1.0587 |
Observed SD |
1.1489 |
1.0289 |
Mean square error |
0.0169 |
0.2583 |
Root MSE |
0.1302 |
0.5082 |
Adjusted variance |
1.3029 |
0.8005 |
Adjusted SD |
1.1415 |
0.8947 |
Separation index |
8.7681 |
1.7606 |
Number of strata |
12.0241 |
2.6808 |
Reliability |
0.9872 |
0.7561 |
Within Rasch analysis, Wright maps are used to illustrate the relationship between student ability and item difficulty. A key feature of Wright maps is that item difficulty uses the same linear scale—logits—as the person measure of student ability (Boone, 2016). Fig. 4 illustrates the Wright map generated for this study. The right side of the map illustrates the distribution of student ability, whereas the left side of the map illustrates the item difficulties. The items are arranged by GCP so that comparisons between the true and false item for each principle can be observed. The difficulty of items ranged between −2 and 2 logits, whereas the ability of respondents ranged between −3 and 2 logits. Items with difficulty measures that are close to the average student ability will have high item reliability estimates. Thus, items close to the center of the scale such as items 7, 23, and 20 will exhibit the most reliable measures when compared to items such as 11 and 16, which are located at the extremes of the scale (Wren and Barbera, 2014). The Wright map provided a range of information about the data. First, it provided further evidence that the range of student ability was approximately normally distributed. Further, it provided evidence that item difficulty approximately matched student ability, thus indicating that the instrument is an appropriate tool for measuring student knowledge. Finally, it illustrated that the items with the correct answer of false are distributed in the higher ability region (upper region) whereas true items corresponded to lower ability (bottom region). This corresponded with the findings from the item difficulties calculated using CTT that indicated that the false items had greater difficulties.
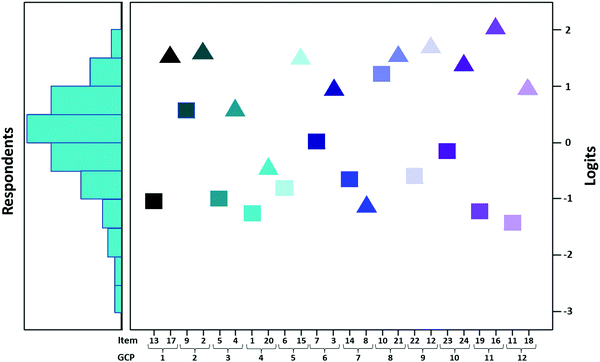 |
| Fig. 4 Wright map of item person ability and item difficulty plotted on a logit scale. The instrument item numbers are grouped according to their GCP, with two items for each GCP. Items indicated by a square had a correct response of true and items indicated by a triangle had a correct response of false. | |
Implications for practice
The ASK-GCP instrument quickly assesses student knowledge of green chemistry with most respondents from our study completing it in less than ten minutes. We found it useful to dissuade students from looking up the answers by including a statement about not looking up answers before the instrument and providing nominal credit for completion rather than accuracy. Thus, we recommend it be used as a low-stakes formative assessment rather than a graded assessment. Due to its ease of use, the student scores from this instrument can be evaluated using CTT by calculating the average score either overall or for each green chemistry principle. Additionally, it can be evaluated using Rasch analysis when assessing learning gains since analysis via Rasch has been shown to be more effective than CTT (Pentecost and Barbera, 2013; Jabrayilov et al., 2016; Sorenson and Hanson, 2021).
Because we have evaluated and provided difficulties for each item, instructors who are concerned with the ceiling effect can remove items 6 and 13 when utilizing the assessment or select items so that approximately two-thirds of the instrument have false as a correct response since the false items were found to be more difficult. This is supported by research that indicates that false items are more able to discriminate between students and should be included in a higher proportion of up to 67% of the items (Frisbie, 1974; Frisbie and Becker, 1991).
This instrument has also been shown to be sensitive to student learning gains, even in two-fold interventions. Therefore, it is well-suited for use in measuring learning gains as a pre-post assessment or for measuring differences between treatment and control groups, including multi-tiered studies with various treatments. It can also be used to initially screen for student knowledge of green chemistry for qualitative studies to ensure students of diverse knowledge levels are selected.
This instrument was administered online, so has been shown to provide valid and reliable data through remote instruction. It has been demonstrated by Nissen et al. (2018) and Lewis (2020) that the method of administration method of administration may not impact the results of the assessment. Therefore, as we transition back into in-person instruction future work examining whether the instrument works consistently in person would be beneficial.
When evaluating the results of this instrument, one must remember that due to the nature of true–false items, it cannot provide complete insight into student conceptions, misconceptions, or reasoning. Furthermore, it aims to test student knowledge of green chemistry rather than the application of that knowledge. Therefore, other more open-ended questions on green chemistry, such as asking students to compare the greenness of two reactions, should be used in tandem with this prompt to gain further insights into student knowledge and reasoning structures.
Limitations
This assessment was developed to measure student knowledge of the green chemistry principles; however, as with all instruments there are limitations which should be acknowledged. These limitations include the impact of student guessing due to the nature of the instrument, the unexplored potential existence of differential item functioning, the limited evidence for response process validity, the single mode of administration, and administering the instrument at only one institution.
Impact of student guessing
This assessment was designed as a true–false instrument to facilitate rapid completion and grading. However, since true–false instruments are dichotomous, the final score can be influenced by student guessing, although its impact greatly decreases as the length of the assessment increases. This impact of guessing can be modeled using the three-parameter logistic model (Meyer, 2014), but it would require a sample size of at least 750 respondents for a 20 item instrument (Şahin and Anıl, 2017). Thus, due to sample size it was not performed in this study. Instead, a “don’t know” option was added in the second iteration to limit the effect of student guessing; however, it does not provide an indicator of student confidence in their selected answer. Therefore, an alternative would be to utilize a confidence tier asking students to assess their confidence in their answer. For this study we chose not to do this as it would require more testing time and make the test more tedious for students. However, further work can involve studying its impact through follow-up self-assessment of confidence questions.
Differential item functioning
For this study, student demographics were not collected and therefore the items were not assessed for differential item functioning (DIF). DIF arises when different subgroups of a population with equal ability perform statistically different on an assessment item (Kendhammer and Murphy, 2014). Thus, it is important to check for DIF as it provides evidence for the internal structure of the instrument (Arjoon et al., 2013). Therefore, this assessment will benefit from future studies analyzing if DIF is observed for any of the items.
Limited evidence for response process validity
The initial version of the instrument included a clarity question which asked students to provide feedback and comment on the clarity of the items. Feedback received from this prompt led us to revising two of the statements for enhanced clarity. However, to assess response process validity, cognitive interviews with students completing the instrument based on Tourangeau's four stage cognitive model of response process should be conducted in future work (Deng et al., 2021).
Single mode of administration
This study reports the findings from only electronic administrations of the assessment which did not have a time limit. Therefore, further investigation is warranted to assess whether other forms of administration, such as paper and pencil or timed formats, impact the functionality of the assessment.
Instrument assessed at only one institution
Although used in courses taught by different instructors, at this stage of analysis the instrument was only administered to students at one institution. Additionally, students who completed the ASK-GCP as a pre-and post-test were instructed by the authors. Thus, the instrument's sensitivity to detect the impact of various green chemistry curriculum or instructional implementations on student learning may differ depending on their nature, intensity, duration, and population being studied. Future work will include administering it at various institutions and with various green chemistry curricula.
Conclusions
We sought to develop an instrument, the ASK-GCP, that provides valid and reliable data and efficiently assesses student knowledge of green chemistry. The reliability of the data obtained from this instrument was illustrated through assessing its internal consistency and temporal stability. Furthermore, the validity of the data obtained from this instrument was demonstrated through assessing its content, convergent, construct and structural validity along with its sensitivity. Analysis of the data using classical test theory supported the instrument's ability to function for groups of differing levels of green chemistry content knowledge. Since Rasch analysis requires the data to be unidimensional, confirmatory factor analysis was used to assess unidimensionality of the data. Once this was established, Rasch analysis was used to illustrate the appropriateness of the item difficulties for use in distinguishing student ability. Finally, the Wright map provided evidence that the item difficulties approximately matched the range of student abilities. Furthermore, it showed that, overall, the false items of the assessment were more difficult than the true items. Through each of these analyses, the ASK-GCP was found to be an effective tool for assessing student knowledge of green chemistry principles. The instrument and answer key have been provided in the appendix of this article to facilitate instructor adoption of this assessment tool. Thus, with this instrument we hope to bridge the gap in assessing student knowledge of green chemistry, which will allow for more robust analysis of the impacts of green chemistry instruction.
Conflicts of interest
There are no conflicts to declare.
Appendix
Table 8 ASK-GCP instrument and key
Item |
Statement |
True |
False |
Don’t know |
Q1 |
An understanding of toxicology and environmental chemistry assists in designing safer chemicals |
● |
○ |
○ |
Q2 |
A reaction that has 100% yield will result in a 100% atom economical reaction |
○ |
● |
○ |
Q3 |
Reactions at elevated temperatures should be prioritized over reactions at room temperature |
○ |
● |
○ |
Q4 |
Highly toxic chemicals are the most effective for synthetic purposes |
○ |
● |
○ |
Q5 |
The relative toxicity of starting materials should be considered when synthesizing molecules |
● |
○ |
○ |
Q6 |
Solvents are chosen based on energy requirements, toxicity profile, safety, and environmental impact |
● |
○ |
○ |
Q7 |
Removal of the solvent from a reaction is an energy consuming process |
● |
○ |
○ |
Q8 |
Fossil fuels are a renewable feedstock |
○ |
● |
○ |
Q9 |
Percent atom economy is calculated using the molecular weight of the desired product and the molecular weights of all the starting reagents |
● |
○ |
○ |
Q10 |
Protecting groups add extra steps to a synthesis, which in turn produces more waste |
● |
○ |
○ |
Q11 |
Reduction of exposure to hazards is the best way to minimize accidents |
● |
○ |
○ |
Q12 |
The most optimal catalytic reagent is used in a 1 : 1 mole ratio with the reactant |
○ |
● |
○ |
Q13 |
Designing reactions with fewer byproducts is a good method of waste prevention |
● |
○ |
○ |
Q14 |
Ethanol derived from corn is an example of a biomass chemical |
● |
○ |
○ |
Q15 |
Amongst the different components of a reaction mixture, solvents have the lowest environmental impact in industrial synthesis |
○ |
● |
○ |
Q16 |
Monitoring the progress of a reaction by analytical methods is used to measure the yield |
○ |
● |
○ |
Q17 |
The environmental factor equals the mass of waste produced in a chemical process |
○ |
● |
○ |
Q18 |
When designing a synthesis, the use of personal protective equipment is sufficient for controlling exposure to hazards |
○ |
● |
○ |
Q19 |
Real-time monitoring of the process helps to avoid incidents caused by side reactions |
● |
○ |
○ |
Q20 |
Highly reactive chemicals will selectively react with intended targets and do not affect other biological and ecological targets |
○ |
● |
○ |
Q21 |
A disadvantage of enzymes is that they suffer from poor selectivity, thus producing more derivatives |
○ |
● |
○ |
Q22 |
A catalyst lowers the activation energy, which allows for reduced reaction times |
● |
○ |
○ |
Q23 |
Understanding the mechanism of degradation assists in the design of biodegradable compounds |
● |
○ |
○ |
Q24 |
Organic compounds containing chlorine are easily broken down |
○ |
● |
○ |
Acknowledgements
This work was supported by NSF DUE-1852045, DUE-2021285, and CHE-2050802, and under EPSCoR Track-1 Cooperative Agreement OIA #1946202. Any opinions, findings, and conclusions or recommendations expressed in this material are those of the authors and do not necessarily reflect the views of the National Science Foundation. In part, this research was also supported by NDSU's NDUS ECOR Award FAR0035075. The authors wish to thank the students who participated in this project. The authors would like to thank Michael Chhoun, Kushal Das, Spencer Gilman and Quinlyn Waulters-Kline for contributing true–false statements for incorporation into the instrument. The authors would also like to thank Ariana McDarby for her help administering the instrument to the students and Nicolette Maggiore for her help with the analysis of the data obtained from the preliminary draft of the instrument. The authors also wish to express their gratitude to Brent Hill for his help with the statistical analysis of the data. Finally, the authors would like to thank Kristina Caton at the NDSU Center for Writers for her help with revising the manuscript.
References
- ACS Green Chemistry Institute, (2021), 12 Principles of Green Chemistry.
- AERA, APA, and NCME, (2014), Standards for Educational and Psychological Testing, American Educational Research Association.
- Andraos J. and Dicks A. P., (2012), Green chemistry teaching in higher education: A review of effective practices, Chem. Educ. Res. Pract., 13(2), 69–79.
- Arias González V. B., Crespo Sierra M. T., Arias Martínez B., Martínez-Molina A., and Ponce F. P., (2015), An in-depth psychometric analysis of the Connor–Davidson resilience scale: Calibration with Rasch–Andrich model, Health Qual. Life Outcomes, 13(1).
- Arjoon J. A., Xu X. and Lewis J. E., (2013), Understanding the state of the art for measurement in chemistry education research: Examining the psychometric evidence, J. Chem. Educ., 90(5), 536–545.
- Armstrong L. B., Rivas M. C., Douskey M. C. and Baranger A. M., (2018), Teaching students the complexity of green chemistry and assessing growth in attitudes and understanding, Curr. Opin. Green Sustain. Chem., 13, 61–67.
- Armstrong L. B., Rivas M. C., Zhou Z., Irie L. M., Kerstiens G. A., Robak M. A. T., et al., (2019), Developing a green chemistry focused general chemistry laboratory curriculum: What do students understand and value about green chemistry? J. Chem. Educ., 96(11), 2410–2419.
- Balabanoff M., Fulaiti H. Al, DeKorver B. K., Mack M. and Moon A., (2021), Development of the water instrument: A comprehensive measure of students’ knowledge of fundamental concepts in general chemistry, Chem. Educ. Res. Pract.
- Barbera J., (2013), A psychometric analysis of the chemical concepts inventory, J. Chem. Educ., 90(5), 546–553.
- Bichi A. A., Embong R., Talib R., Salleh S. and Ibrahim A. B., (2019), Comparative analysis of classical test theory and item response theory using chemistry test data, Int. J. Eng. Adv. Technol., 8(5), 2249–8958.
- Bond T. G. and Fox C. M., (2013), Applying the Rasch Model: Fundamental Measurement in the Human Sciences, Routledge.
- Boone W. J., (2016), Rasch analysis for instrument development: Why, when, and how? CBE Life Sci. Educ., 15(4).
- Brandriet A., Reed J. J. and Holme T., (2015), A historical investigation into item formats of acs exams and their relationships to science practices, J. Chem. Educ., 92(11), 1798–1806.
- Bretz S. L. and Linenberger K. J., (2012), Development of the enzyme–substrate interactions concept inventory, Biochem. Mol. Biol. Educ., 40(4), 229–233.
- Cannon A. S., Keirstead A. E., Hudson R., Levy I. J., MacKellar J., Enright M., et al., (2020), Safe and sustainable chemistry activities: Fostering a culture of safety in K-12 and community outreach programs, J. Chem. Educ., 98(1), 71–77.
- Chen M., Jeronen E. and Wang A., (2020), What lies behind teaching and learning green chemistry to promote sustainability education? A literature review, Int. J. Environ. Res. Public Health, 17(21), 1–24.
- Connor M. C. and Shultz G. V., (2018), Teaching assistants’ topic-specific pedagogical content knowledge in 1H NMR spectroscopy, Chem. Educ. Res. Pract., 19(3), 653–669.
- Couch B. A., Hubbard J. K. and Brassil C. E., (2018), Multiple-true–false questions reveal the limits of the multiple-choice format for detecting students with incomplete understandings, Bioscience, 68(6), 455–463.
- Deng J. M., Streja N. and Flynn A. B., (2021), Response process validity evidence in chemistry education research, J. Chem. Educ., 98(12), 3656–3666.
- Ding L. and Beichner R., (2009), Approaches to data analysis of multiple-choice questions, Phys. Rev. Spec. Top.: Phys. Educ. Res., 5(2), 020103.
- Downing S. M. and Yudkowsky R., (2009), Assessment in health professions education, Routledge.
- Dutke S. and Barenberg J., (2015), Easy and informative: Using confidence-weighted true–false items for knowledge tests in psychology courses, Psychol. Learn. Teach., 14(3), 250–259.
- Ebel R. L., (1970), The case for true–false test items, Am. J. Educ., 78(3), 373–389.
- Fahmy A. and Lagowski J., (2012), Systemic assessment as a new tool for assessing students learning in chemistry using SATL methods: Systemic true false [STFQs] and systemic sequencing [SSQs] question types, Afr. J. Chem. Educ., 2(2), 66–78.
- Fan X., (1998), Item response theory and classical test theory: An empirical comparison of their item/person statistics, Educ. Psychol. Meas., 58(3), 357–381.
- Ferguson G. A., (1949), On the theory of test discrimination, Psychometrika, 14(1), 61–68.
- Frisbie D. A., (1974), The effect of item format on reliability and validity: A study of multiple choice and true–false achievement tests, Educ. Psychol. Meas., 34(4), 885–892.
- Frisbie D. A. and Becker D. F., (1991), An analysis of textbook advice about true–false tests, Appl. Meas. Educ., 4(1), 67–83.
- Fritz M. F., (1927), Guessing in a true–false test, J. Educ. Psychol., 18(8), 558–561.
- Galgano P. D., Loffredo C., Sato B. M., Reichardt C. and Seoud O. A. E., (2012), Introducing education for sustainable development in the undergraduate laboratory: quantitative analysis of bioethanol fuel and its blends with gasoline by using solvatochromic dyes, Chem. Educ. Res. Pract., 13(2), 147–153.
-
Green & Sustainable Chemistry Education Module Development Project, (2021).
- Grieger K. and Leontyev A., (2021), Student-generated infographics for learning green chemistry and developing professional skills, J. Chem. Educ., 98(9), 2881–2891.
- Grieger K. and Leontyev A., (n.d.), Teaching green chemistry though student-generated open educational resources, J. Coll. Sci. Teach., in press.
- Hankins M., (2008), How discriminating are discriminative instruments? Health Qual. Life Outcomes, 6, 36.
- Hays R. D. and Reeve B. B., (2008), Measurement and modeling of health-related quality of life, Int. Encycl. Public Heal., 241–252.
- He P., Liu X., Zheng C. and Jia M., (2016), Using Rasch measurement to validate an instrument for measuring the quality of classroom teaching in secondary chemistry lessons, Chem. Educ. Res. Pract., 17(2), 381–393.
- Heaton A., Hodgson S., Overton T. and Powell R., (2006), The challenge to develop CFC (chlorofluorocarbon) replacements: A problem based learning case study in green chemistry, Chem. Educ. Res. Pract., 7(4), 280–287.
- Holme T. A., MacKellar J., Constable D. J. C., Michels O. R., Trate J. M., Raker J. R. and Murphy K. L., (2020), Adapting the anchoring concepts content map (ACCM) of ACS exams by incorporating a theme: Merging green chemistry and organic chemistry, J. Chem. Educ., 97(2), 374–382.
- Jabrayilov R., Emons W. H. M. and Sijtsma K., (2016), Comparison of classical test theory and item response theory in individual change assessment, Appl.
Psychol. Meas., 40(8), 559–572.
- Kean J., Bisson E. F., Brodke D. S., Biber J. and Gross P. H., (2018), An introduction to item response theory and Rasch analysis: Application using the eating assessment tool (EAT-10), Brain Impair., 19(1), 91–102.
- Kendhammer L. K. and Murphy K. L., (2014), General statistical techniques for detecting differential item functioning based on gender subgroups: A comparison of the Mantel-Haenszel procedure, IRT, and logistic regression, ACS Symp. Ser., 1182, 47–64.
- Kinney L. B. and Eurich A. C., (1933), Studies of the true–false examination, Psychol. Bull., 30(7), 505–517.
- Kline T. J. B., (2005), Classical test theory: Assumptions, equations, limitations, and item analysis, in psychological testing: A practical approach to design and evaluation, Shaw L. C., Crouppen M., Hoffman C. A. and Weight B. (ed.), SAGE Publications, Inc.
- Krabbe P. F. M., (2017), Chapter 7 – Validity, in The Measurement of Health and Health Status, Academic Press, pp. 113–134.
- Lahner F.-M., Lörwald A. C., Bauer D., Nouns Z. M., Krebs R., Guttormsen S., et al., (2018), Multiple true–false items: A comparison of scoring algorithms, Adv. Heal. Sci. Educ., 23(3), 455–463.
- Lakens D., (2013), Calculating and reporting effect sizes to facilitate cumulative science: A practical primer for t-tests and ANOVAs, Front. Psychol., 4(NOV), 863.
- Lasker G. A., (2019), Connecting systems thinking and service learning in the chemistry classroom, J. Chem. Educ., 96(12), 2710–2714.
- Lee E. N. and Orgill M., (2021), Toward equitable assessment of english language learners in general chemistry: Identifying supportive features in assessment items, J. Chem. Educ.
- Leontyev A., Pulos S. and Hyslop R., (2017), Making the most of your assessment: Analysis of test data in jMetrik, ACS Symp. Ser., 1260, 49–64.
- Lewis S. E., (2020), Chemistry assessments through the sudden implementation of online instruction, J. Chem. Educ., 97(9), 3418–3422.
- Linacre J. M., (2021), Reliability and separation of measures, Winsteps.
- Loevinger J., (1957), Objective tests as instruments of psychological theory: Monograph supplement 9, Psychol. Rep., 3(7), 694.
- Lu S. and Bi H., (2016), Development of a measurement instrument to assess students’ electrolyte conceptual understanding, Chem. Educ. Res. Pract., 17(4), 1030–1040.
- Lu H., Jiang Y. and Bi H., (2020), Development of a measurement instrument to assess students’ proficiency levels regarding galvanic cells, Chem. Educ. Res. Pract., 21(2), 655–667.
- Ludlow L. H. and Haley S. M., (2016), Rasch model logits: Interpretation, use, and transformation, Educ. Psychol. Meas., 55(6), 967–975.
- Lutter J. C., Hale L. V. A. and Shultz G. V., (2019), Unpacking graduate students’ knowledge for teaching solution chemistry concepts, Chem. Educ. Res. Pract., 20(1), 258–269.
- Magno C., (2009), Demonstrating the difference between classical test theory and item response theory using derived test data, Int. J. Educ. Psychol. Assess., 1(1), 1–11.
- Marques C. A., Marcelino L. V., Dias É. D. S., Rüntzel P. L., Souza L. C. A. B. and Machado A., (2020), Green chemistry teaching for sustainability in papers published by the Journal of Chemical Education, Quim. Nova, 43(10), 1510–1521.
- McCullough T., (1993), A second look at true–false questions, J. Chem. Educ., 70(10), 829.
- McGahee T. W. and Ball J., (2009), How to read and really use an item analysis, Nurse Educ., 34(4), 166–171.
- Meyer J. P., (2014), Applied Measurement with jMetrik, Routledge.
- Mishra P., Pandey C. M., Singh U., Gupta A., Sahu C. and Keshri A., (2019), Descriptive statistics and normality tests for statistical data, Ann. Card. Anaesth., 22(1), 72.
- Nedungadi S., Paek S. H. and Brown C. E., (2019), Utilizing Rasch analysis to establish the psychometric properties of a concept inventory on concepts important for developing proficiency in organic reaction mechanisms, Chem. Teach. Int., 2(2).
- Nissen J. M., Jariwala M., Close E. W. and Dusen B. V., (2018), Participation and performance on paper- and computer-based low-stakes assessments, Int. J. STEM Educ., 5(1), 1–17.
- Oosterhof A. C. and Glasnapp D. R., (1974), Comparative reliabilities and difficulties of the multiple-choice and true—false formats, J. Exp. Educ., 42(3), 62–64.
- Pentecost T. C. and Barbera J., (2013), Measuring learning gains in chemical education: A comparison of two methods, J. Chem. Educ., 90(7), 839–845.
- Płotka-Wasylka J., Kurowska-Susdorf A., Sajid M., de la Guardia M., Namieśnik J. and Tobiszewski M., (2018), Green chemistry in higher education: State of the art, challenges, and future trends, ChemSusChem, 11(17), 2845–2858.
- Polit D. F. and Beck C. T., (2006), The content validity index: Are you sure you know what's being reported? Critique and recommendations. Res. Nurs. Health, 29(5), 489–97.
- Price R. M., Andrews T. C., McElhinny T. L., Mead L. S., Abraham J. K., Thanukos A. and Perez K. E., (2014), The Genetic Drift Inventory: A Tool for Measuring What Advanced Undergraduates Have Mastered about Genetic Drift, CBE Life Sci. Educ., 13(1), 65.
- Progar Š. and Sočan G., (2008), An empirical comparison of item response theory and classical test theory – PsycNET, Psihol. Obz./Horiz. Psychol., 17(3), 5–24.
- Şahin A. and Anıl D., (2017), The effects of test length and sample size on item parameters in item response theory, Educ. Sci. Theory Pract., 17(1), 321–335.
- Savec V. F. and Mlinarec K., (2021), Experimental work in science education from green chemistry perspectives: A systematic literature review using PRISMA, Sustain. 2021, 13, 12977.
- Schönborn K. J., Höst G. E. and Palmerius K. E. L., (2015), Measuring understanding of nanoscience and nanotechnology: development and validation of the nano-knowledge instrument (NanoKI). Chem. Educ. Res. Pract., 16(2), 346–354.
- Sorenson B. and Hanson K., (2021), Using classical test theory and rasch modeling to improve general chemistry exams on a per instructor basis, J. Chem. Educ., 98(5), 1529–1538.
- Taskin V., Bernholt S. and Parchmann I., (2015), An inventory for measuring student teachers’ knowledge of chemical representations: Design, validation, and psychometric analysis, Chem. Educ. Res. Pract., 16(3), 460–477.
- Thorndike R. M. and Thorndike-Christ T., (2010), Qualities Desired in Any Measurement Procedure: Reliability, in Measurement and Evaluation in Psychology and Education, Pearson Education, Inc., pp. 118–153.
- Wren D. and Barbera J., (2014), Psychometric analysis of the thermochemistry concept inventory, Chem. Educ. Res. Pract., 15(3), 380–390.
- Zamanzadeh V., Ghahramanian A., Rassouli M., Abbaszadeh A., Alavi-Majd H. and Nikanfar A.-R., (2015), Design and implementation content validity study: Development of an instrument for measuring patient-centered communication, J. Caring Sci., 4(2), 165.
- Zuin V. G., Eilks I., Elschami M. and Kümmerer K., (2021), Education in green chemistry and in sustainable chemistry: Perspectives towards sustainability. Green Chem., 23(4), 1594–1608.
|
This journal is © The Royal Society of Chemistry 2022 |