Considering the hexad of learning domains in the laboratory to address the overlooked aspects of chemistry education and fragmentary approach to assessment of student learning
Received
30th September 2021
, Accepted 3rd April 2022
First published on 4th April 2022
Abstract
This article seeks to provide researchers and practitioners in laboratory education, particularly those involved in the curriculum design and implementation of teaching laboratories at university level, with a conceptual framework and a working model for an integrated assessment of learning domains, by attending to a more holistic approach to learning in the laboratory. Prevailing learning theories suggest that the triad of cognitive, psychomotor, and affective domains should be addressed in order to warrant meaningful learning. In the research tradition of psychology and philosophy of mind, this triad also manifests as a concert of cognitive, conative, and affective domains. The paper argues that at least in the context of chemistry laboratory education, this is insufficient. The social and epistemic domains are often overlooked or dismissed altogether. Research in science studies may provide insight into the urgency and usefulness of integrating these domains into chemistry teaching and learning. Firstly, laboratory work is conceptualised here as an epistemic practice, in which students generate data, propose knowledge derived from the data, evaluate, and legitimise it. Secondly, the operationalisation of the hexad of learning domains is proposed, in terms of curriculum design, instruction, and assessment.
Introduction
Ever since Justus von Liebig introduced individual laboratory work in the chemistry laboratory during his professorship at the University of Giessen in Germany back in the 19th century, the laboratory has been developed and viewed as an essential part of chemistry education at university level as we know today. Teaching laboratories are also common in health and medical sciences. They comprise various practical activities involving teaching staff and laboratory technicians, requiring materials and equipment which often amount to substantial educational expenses. Notwithstanding the perpetual presence and seemingly self-evident importance of laboratory work, scholars have been critical of its justification in chemistry education, especially considering the high cost to run a teaching laboratory (c.f., Hofstein and Lunetta, 1982, 2003; Kirschner, 1992; Hodson, 1993; Reid and Shah, 2007; Bretz, 2019). Chiefly for this reason, chemistry educators need to provide more evidence of student learning in the laboratory, which goes beyond unidimensional marking of laboratory reports. In her editorial piece on the importance of laboratory courses, Bretz (2019) succinctly reasserts the need for substantiating learning in the undergraduate laboratory. She contends that evidence of student learning in the teaching laboratory is paramount because higher education is influenced by diverse stakeholders who do not necessarily share the same value proposition.
In an attempt to substantiate the effect of laboratory instructions on student learning process and outcomes, chemistry education researchers have conducted empirical studies focused on various elements of learning in the laboratory at tertiary level (Agustian et al., unpublished work). To date, some of these studies have been situated within particular theoretical frameworks, in terms of how learning is conceptualised and how the concept is operationalised into research instruments. For instance, student learning in the laboratory has been conceptualised in terms of cognitive load theory (e.g., Winberg and Berg, 2007; Limniou et al., 2009; Agustian, 2020a). Other researchers refer to the theory of meaningful learning, in which not only is the cognitive domain of learning investigated, but also in relation to the affective and psychomotor domains (e.g., Rubin and Tamir, 1988; Bledsoe and Flick, 2012; Galloway and Bretz, 2015a, 2015b, 2015c).
Although research endeavours in this field have, to some extent, demonstrated the pedagogical value of teaching laboratories, I argue that the manner in which various aspects of learning have been conceptualised, operationalised, and substantiated is somewhat fragmentary or treated in isolation, with an exception of a few studies such as those published by Bretz's research group. But notably, attention to the social and epistemic domains of learning in the laboratory is rather scant (see also, Nakhleh et al., 2002; Duschl and Grandy, 2013). The argument for more integration between these different domains stems from the longstanding conundrum of connecting experimental work to the underlying theory (Agustian, 2020a). While this problem may indicate a lack of correspondence between ‘doing’ and ‘thinking’, it also signifies a need for more elucidation of how epistemological aspects of laboratory work could help students construct meanings of procedural and declarative knowledge involved in their exercise. Likewise, various interactions in the laboratory, both among people and with instruments and equipment, necessitate more investigation.
With that in mind, this article seeks to achieve the following:
• critique the scope of research aimed at substantiating learning in the context of post-secondary chemistry laboratory;
• propose a working model for integrated learning domains in the laboratory; and
• suggest how they could be operationalised in research and practice.
Learning in the laboratory
The importance of the laboratory in chemistry courses used to lie primarily in the need to prepare students for a career in chemistry after they graduate, be it in industry or academia. As students shift their career pursuits elsewhere, however, this stance needs to be revisited (Reid and Shah, 2007). The nature of work and the career options available for chemistry graduates currently require them to possess a degree of flexibility, by which they are expected to be able to navigate in an increasingly more complex society (Taber, 2000). As members of such society, they also need to participate in and be well informed about various decision making processes pertaining to science (Agustian, 2020b). From a career orientation perspective, the aforementioned flexibility can be afforded with the acquisition and development of transferable skills (Stephenson and Sadler-Mcknight, 2016; van Merriënboer and Kirschner, 2017; George-Williams et al., 2018). To this end, more efforts should be made towards a wider and deeper learning process (DeKorver and Towns, 2015; Reid and Shah, 2007), such that students also learn about and through chemistry.
Research development in university chemistry education demonstrates that students’ learning experience in the laboratory is multidimensional (Agustian et al., unpublished work) and distinctive in comparison to other environments (Dalgety et al., 2003; Hofstein and Lunetta, 2003; Lastusaari et al., 2016). Despite ongoing debates on the efficacy and efficiency of the laboratory in chemistry education, several arguments for its purpose are still considerably valid. The laboratory is a place where students could get a sense of how scientists conduct experimental work. Ideally, it should invoke interest in chemistry and motivation to learn more about it, as well as to enhance conceptual understanding and develop problem-solving skills (Russell and Weaver, 2011). Others, like Hodson (1993) and Kirschner (1992), argue that the laboratory should focus more on improving practical skills than learning about scientific concepts.
Having compared and critiqued various chemistry educators’ and researchers’ positions with regards to laboratory education, six distinctive learning goals of the laboratory in university chemistry education beyond content-specific goals can be summarised, as shown in Table 1. These goals are pertaining to university chemistry education at the degree and course levels, as opposed to that of individual laboratory experiments. Albeit not an exhaustive list, it can be used as a point of departure from which the purpose of laboratory work is conceptualised in this article.
Learning goals |
Description |
Specific practical skills |
Planning and execution, manipulation, observation, investigation, and reporting skills |
Scientific reasoning |
Ability to recognise problems, understand experimental methods, organise and interpret data, test hypotheses, and make generalisations |
Creativity and problem solving |
Creativity with experimental designs, revision of methods and enacted procedures, data interpretation, and drawing conclusions |
Social relationships |
Constructive social relationships, team working, peer teaching, and positive learning environment |
Affective domain |
Attitudes to science (e.g. interest, enjoyment, satisfaction) and scientific attitudes (e.g. open- and critical-mindedness, scepticism, curiosity, intellectual honesty) |
Understanding of the nature of science |
Empirical and tentative nature of science, role of theories and laws, theory-ladenness and philosophical subjectivity, etc. |
The aforementioned goals represent the potential value of laboratory education gathered from the literature. From a perspective of curriculum development, they can be regarded as an intended curricular form, which entails rationale or philosophy underlying the curriculum (Thijs and van den Akker, 2009). However, they still need to be transposed into implemented and attained forms of curriculum, whereby discrepancy is resolved, and coherence maintained. This also means that typology of laboratory curricula matters, whether it is expository, problem-based, more open inquiry, or research-based (Domin, 1999b; Weaver et al., 2008).
One of the most salient features of the laboratory that has been scrutinised is the extent to which students learn from laboratory instruction. The problems associated with learning in the laboratory can be analysed through various lenses. For instance, in terms of conceptual understanding and cognitive engagement, empirical findings demonstrate that students often conduct experiments absent-mindedly, without really understanding what they are actually doing (Ausubel, 2000; Rudd II et al., 2001; Reid and Shah, 2007; Winberg and Berg, 2007; Teo et al., 2014; Barrie et al., 2015). Apart from the predominantly expository approach to laboratory instruction, much of this problem is attributed to the overwhelming burden on students’ cognition within the limited hours of their laboratory period. With so much information to process and new skills to practise, they are left with little space to think about the underpinning theories and the nature of scientific work they are dealing with. They may be able to manipulate equipment and materials, but not ideas (Fraser et al., 2012). This explains why other goals such as a deeper understanding of the nature of science and the investigation of scientific phenomena, are not even part of the learning trajectories that most students envision (DeKorver and Towns, 2015). All of these problems pertain mainly to the cognitive domain of learning.
Learning can be cognitive, affective, psychomotor, social, or any combination of these, but for any learning to occur, some mental or physical activity is required (Rennie, 2001). Accordingly, laboratory activities often seem to be written with the idea of addressing as many goals as possible, such as illustration of chemical principles (cognitive domain) and simultaneous training in technical skills (psychomotor domain). My notion of attending to various domains in assessing student learning in this context stems from theoretical development in the learning sciences. Relevant to the discussion here is the work of Illeris (2018), in which he posits a comprehensive conceptualisation of learning, encompassing basic processes and interrelated dimensions. Central to his notion of learning is an integration between functionality (which encompasses knowledge, skills, and understanding) and sensitivity (which is associated with mental and bodily balance). The latter also manifests in constructs such as motivation, emotion, and volition. The model and framework proposed in the present article mirror such conceptualisation of learning.
An attempt to integrate various domains in the investigation into learning in the laboratory can be discerned from the work of Bretz and their collaborators (Bretz et al., 2013; Galloway and Bretz, 2015b, 2015c; Galloway et al., 2016), using meaningful learning theory. According to this framework, three criteria must be met for meaningful learning to occur: (i) relevant prior knowledge of the student; (ii) meaningful material organised by the teacher to connect to this prior knowledge; and (iii) the conscious choice of the student to make connections between the prior knowledge and the new meaningful material. I aim to extend this framework to encompass more aspects of learning. Firstly, by proposing a working model to integrate all six domains into an interconnected framework. Secondly, by operationalising the model in the context of research and assessment of student learning.
Proposed model for integrated learning domains
The intricate relationships between various domains of learning in the laboratory are supposedly harnessed as a source of pedagogical capital, whereby all six domains (cognitive, conative, affective, psychomotor, social, and epistemic) work in synergy to enhance learning, and overlaps between two or more domains sophisticate the resulting constructs. In cognitive science, human cognition is mapped into a spectrum from low to high order, which is often represented in a taxonomy of thinking skills and performances (Leighton and Gierl, 2011). I would argue that such stratification of low-order and high-order could also be applied to the extent to which the integration takes place, i.e. the more integrated they are, the more sophisticated learning outcomes will be. While this can be applied to the existing quandary of experimental-conceptual connection in the laboratory mentioned previously, it also propagates to other combinations, such as cognitive-social-epistemic (Duschl and Grandy, 2013) and cognitive-affective-psychomotor (Galloway et al., 2016).
The idea of integrating various elements of learning in enhancing educational outcomes is not new in science education (e.g., Gollub et al., 2002; Duschl, 2008; Littledyke, 2008). Particularly in chemistry laboratory education, the integration of learning domains in both curriculum design and assessment is relevant because the chemistry laboratory is a complex learning environment that lends itself to diverse learning outcomes, if assessed wholly and adequately. In the past, critique of laboratory education maintained that apart from the psychomotor domain, instruction in this educational context did not lead to significantly higher learning gains when compared to, for instance, classroom instruction. However, this has been responded to in recent years by studies aimed at substantiating learning outcomes associated with laboratory work (Agustian et al., unpublished work), including several experimental designs and longitudinal studies conducted in laboratory vs. lecture settings (Oliver-Hoyo et al., 2004; House et al., 2016; Matz et al., 2012).
Mere focus on the narrowly defined goals pertaining to the cognitive domain renders the assessment unidimensional and ignores the possibility of assessing other forms of learning. In many cases, even the psychomotor domain is not assessed adequately (Agustian, 2020a). When more than one domain is assessed, there is a tendency that different domains are treated in isolation (DeKorver and Towns, 2015). Accordingly, the notion of multidimensional learning assessment in lieu of unidimensional learning has been substantiated in the larger context of chemistry education (Pazicni et al., 2021), which can be traced back to the work of Cooper and their collaborators (Cooper and Stowe, 2018; Underwood et al., 2018; Stephenson et al., 2020). It is noteworthy that the conceptualisation of ‘multidimensional’ in their works refers to ideas, concepts, and practices.
Lazarowitz (2007) intimates that in relation to laboratory work, learning theories, instructional strategies, as well as methods of learning and assessment need to be transformed so as to enable teachers to not only assess students’ academic achievement but also aspects belonging to other domains. Therefore, curriculum designers should be cognizant of how learning environments could foster co-construction of meaning and mutually-shared sense-making (Sawyer, 2014). The notion of distributed cognition is also relevant here, which marks the social domain of learning in the laboratory. Taking into account all of these arguments and corresponding empirical insights, a model for integrating all six domains of learning in the laboratory is proposed here.
At the heart of the model is a view of the learner as both an individual and a part of a group. As an individual, they are expected to continually activate their mind and maintain coordination with their body to carry out various elements of experimental work. In his autobiographical note, Liebig himself argues, ‘[t]he faculty of thinking in phenomena can only be cultivated if the mind is constantly trained’ (Liebig, 1892, p. 3). The activation and coordination between mind and body are conceptualised here in terms of cognitive, conative, affective, and psychomotor domains of learning, which will be briefly described in the following paragraphs.
Cognition is one of the faculties of mind devoted to thinking and reasoning. There is a wealth of literature in cognitive science that can inform laboratory education, but a useful taxonomy of cognitive learning can be discerned from the work of Biggs and Tangs (2007, 2011) on improving the quality of learning at university level, in which they conceptualise the hierarchy in terms of observable learning outcomes, namely:
• Unistructural, focusing primarily on one part of the task, such as identifying and carrying out simple procedure
• Multistructural, covering more than one part but not necessarily establishing connections, such as discussing and reporting
• Relational, integrating concepts and approaches to learning, such as reviewing and arguing
• Extended abstract, conceptualising the coherent whole at a higher level of abstraction, such as hypothesising and reflecting
They argue that learning at university level requires student to operate at higher cognitive level, so it is important to design learning activities that afford them more opportunities to accomplish relational and extended abstract learning outcomes. Relevant to the discussion here is the field of research in epistemic cognition, which signifies a shift towards more focus on learning trajectories that lead to expert-like conceptualisations, skills, and performance (Greene et al., 2016).
The conative domain focuses on conation, which refers to mental processes that drive us to act and strive to perform at the highest levels. It entails human needs, desires, intentionality, and will. The concept of conation appears in the writing of early philosophers such as Plato and Aristotle and continues to appear in discussions of philosophy, psychology, and education today. However, the literature on teaching, learning, and assessment is generally not informed by consideration of the conative domain, especially in science education (Snow and Jackson III, 1997; Reeves, 2006). Goldin (2019) concedes that empirical research tends to present this domain in a tacit rather than an explicit manner. Researchers usually refer to this domain in terms of motivation, volition, and related constructs such as self-efficacy and self-regulation (Kupermintz, 2002; Pintrich, 2004; Bartels et al., 2011). The essential role of the conative domain in relation to the other learning domains is succinctly asserted by Reeves (2006):
“While an individual may possess the cognitive capacity, affective values, and physical skills to perform a given task, [they may not] possess… the will, desire, drive, level of effort, mental energy, intention, striving, and self-determination to actually perform at the highest standards possible” (Reeves, 2006, p. 297).
The term ‘affect’ refers to positive and negative valence of transactional experiences with certain situations, encompassing emotions and attitudes (Dai and Sternberg, 2004). From a neuroscience perspective, Dolcos et al. (2020) posit that it consists of multiple subcomponents including physiological, appraisals, expressions, and behaviours that define the relation between the individual and environment. In an educational context, affect is a necessary condition for learning to occur (Koballa Jr. and Glynn, 2007) and a catalyst for learners to achieve higher (Hwang et al., 2017). This interplay is reflected in the present model, both at individual and group levels. The latter reference to ‘catalyst to achieve higher’ denotes the other faculty of mind, i.e., conation, as previously described.
Physical skills in the quote from Reeves (2006) above are naturally a distinctive aspect of learning in the laboratory, which is conceptualised here as psychomotor domain. It concerns manipulation of equipment and instruments, performance of particular laboratory techniques, as well as attending to sensory awareness during a learning task and following safety procedures (Zaghloul, 2001; Feisel and Rosa, 2005; Hofstein and Kind, 2012). As the etymology of ‘psychomotor’ implies, it also denotes coordination between mental and motoric actions. Hence, the model visualises this domain as an extension of the three faculties of mind (see Fig. 1).
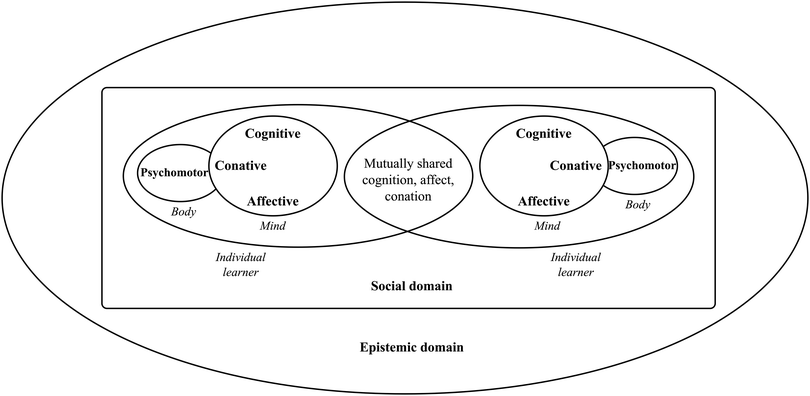 |
| Fig. 1 A working model for integrated learning domains in the laboratory. | |
The model highlights the importance of looking at the laboratory as an educational premise that brings together learners in a complex but intellectually stimulating environment, in which conceptual and technical goals are set and learning activities are designed upon an underlying epistemic domain. Making a clear reference to the research tradition in the learning sciences, educational psychology, science studies, and philosophy of mind, it views learners as agents who have to continually navigate between their cognitive system, their emotional states and traits, their motivation and volition, and the learning task at hand. Accordingly, they have to activate their mind and perform required technical skills at the same time. To make it more complex, it is also very likely that they have to work with peers on how to conduct the inquiry and presumably co-interpret the data that they will analyse individually.
The epistemic domain constitutes the foundational domain that supposedly underpins all those endeavours. Matthews (2018) contends that engaging students in the epistemic practices of science and engineering is pivotal to the deep understanding about the nature of their disciplines through participation. However, merely going through the motions of laboratory procedures does not necessarily lend itself to developing such knowledge and understanding, especially where the curriculum and instruction are of expository nature. He underscores that students can be afforded meaningful insights into scientific knowledge construction through the processes of inquiry, reasoning, and design, but only if properly organised and reflected upon.
The foundational role of epistemic domain of learning in the laboratory can be traced back to how epistemic culture permeates knowledge construction in science. An extended work informed by sociology of science relevant to this argument can be discerned from the work of Knorr-Cetina (1999), as she asserts that the laboratory and experimental work are central to the processes of science and legitimisation of scientific knowledge. Although teaching laboratories are typically not expected to generate new knowledge, I argue that laboratory education particularly at university level should nevertheless reflect this underlying epistemic role, in order to establish the laboratory as a place to learn to do chemistry, as predicated by Seery (2020).
In the present model, the social domain is embedded in the epistemic domain, which reflects how science itself has been practised. For example, the social system of certification and dissemination of scientific knowledge involves collective and collaborative efforts of the community, which ensures a social quality control and an epistemic control (Erduran and Dagher, 2014). Correspondingly, laboratory experiments are no longer viewed as a way of portraying scientific methods to verify concepts, but are valued for their role in providing evidence for knowledge claims open to public scrutiny (Hofstein and Kind, 2012). The present model regards the laboratory as a ‘social plane’ (Scott et al., 2006), both in the sense of social-epistemic context as well as a physical space. In this plane, individual learners interact with each other, with the instructors, and often also with laboratory instruments. Dialogue and discourse are important, and they may provide a rich description of what students actually learn and how their learning unfolds.
Taken together, the six domains of learning in this model represent the complexity of student learning in the laboratory, where activation, coordination, and integration are key to successful teaching and learning. In the following sections, laboratory work will be framed as an epistemic practice, in which the hexad of learning domains are considered. Recommendations for researchers and eventually practitioners in the field regarding the operationalisation of this framework will also be proposed. Whenever relevant, assumptions about learning will be explicated and aligned with contemporary scholarship in science education.
Laboratory work as an epistemic practice
In the working model presented in this paper, the epistemic domain underlies the other five. This domain encapsulates the kind of practice inherent in experimental work, where students typically generate their own data, propose some form of knowledge derived from those data, evaluate it, and to some extent, ‘legitimise’ the knowledge, i.e., the kind situated and contextualised in the experiment they have conducted. In the longstanding tradition of philosophical discourse, such process can be represented by ‘epistemic practice’. This concept is relevant and useful for the framing of integrated learning domains in the laboratory because it considers the practitioner of such practice as an agent who deploys their mind and body in coordination with their social context (Annis, 1982; Andersson et al., 2018). The element of body and the corresponding embodied learning are represented by the psychomotor domain in the model, whereas the element of mind is represented by the three faculties (cognition, affect, conation). With the overlap representing the social domain, it is important to clarify that this model applies primarily to the type of laboratory exercise in which students work in pairs or small groups, as opposed to entirely individual experimental work that typifies open inquiry projects in the final year of undergraduate or postgraduate levels. As a whole, the goal of learning how to do chemistry (Seery, 2020) is central to the characterisation of epistemic practice in this educational premise, and it mirrors the notion of learning the syntactical structure of science, as argued elsewhere (Schwab, 1967; Kirschner, 1992; Agustian and Seery, 2017). Indeed, it can be viewed as an enculturation of the students into the epistemic cultures (Knorr-Cetina, 1999) of the natural science.
Inclusion of social features of science in the chemistry laboratory, which reflects the manner in which science is socially organised, has been argued to facilitate learning (Erduran and Dagher, 2014; Erduran and Kaya, 2019). Accordingly, the situational aspects of collaboration pertaining to laboratory work are the mechanism that leads to learning, in which social epistemology – like beliefs about interpersonal context and social cohesion – plays a substantial role (Sawyer, 2014). Previous empirical research has shown positive results of collaborative learning in laboratory education research (e.g., Shibley and Zimmaro, 2002; Wilson and Wilson, 2017). Apart from more efficient task and resource distribution, the rationale for the wide use of collaborative learning approach in laboratory education is that groups of people are increasingly acknowledged as a major source of knowledge construction, particularly when they collectively solve problems or carry out tasks (Sawyer, 2014). However, this is not without challenges:
“When solving problems or completing tasks, teams of people with different experiences, values, and knowledge are often more effective than are individuals. However, to be able to adequately solve problems or carry out tasks, they face the challenge of integrating their different perspectives and developing a shared understanding of the problem at hand. This can be established through rich interaction, interactive discussion, and negotiation” (Miyake and Kirschner, 2014, p. 419).
The superiority of collaborative learning in a laboratory context can be explained with the concept of distributed cognition (Hutchins, 2001; Zhang and Patel, 2006), whereby the dynamic and interactive aspects of knowledge are emphasised. In the laboratory, this concept encompasses cognitive distribution between and across people (students, instructors, technicians) as well as between them and laboratory instruments and equipment. In this regard, Miyake and Kirschner (2014) proposed a model in which distributed cognition plays a significant role, as shown in Fig. 2. In their model, learning is defined as processes of construction and co-construction of meaning driven by constructive conflict. The interaction among members of the group, the characteristics of their discourse, and negotiation of meanings are key to an effective collaboration, through which mutually shared, distributed cognition is reached.
The integrated learning domains in the present paper essentially resonates with Miyake and Kirschner's model, with a consideration of the affective and conative domains of learning in the process of co-construction of meaning and, indeed, the epistemic practice that encapsulates the entire process. Hence, students may also share some values related to laboratory work, as well as emotional, motivational, and volitional aspects.
Implications
The framing of laboratory work as an epistemic practice has implications on a predication that curriculum design, instruction, and learning assessment should be underpinned by this framework. In the following sections, I will elaborate this.
Implications for curriculum design and instruction
When designing a laboratory curriculum, it is important to formulate intended epistemic learning outcomes, which relates to how students know what they know, by understanding the criteria for evaluating knowledge claims, using theories or models, or the criteria for choosing one explanation over alternative ones (Jiménez-Aleixandre and Crujeiras, 2017). Conventional laboratory courses are designed with a range of conceptual and technical goals, but they rarely include epistemic goals. Likewise, there is scarcity of research focussing on faculty's epistemic goals for laboratory instruction. To illustrate, in a systematic review of university chemistry laboratory education, only about 11 out of 355 studies incorporate some extent of epistemic learning (Agustian et al., unpublished work). Of those, merely three studies (Russell and Weaver, 2008; Cessna et al., 2009; Seung et al., 2016) address a formulation of epistemic goals. As the model in Fig. 1 suggests, establishing these goals first is crucial to the framing of laboratory work beyond algorithmic, unistructural, and lower-order cognitive goals.
The formulation of intended epistemic learning outcomes is coherent with the move towards more focus on higher-order cognition in university chemistry (Domin, 1999a). In its common form, laboratory work rarely affords students an opportunity to hypothesise and reflect, as argued by Biggs and Tangs (2007, 2011) above. Accordingly, researchers in the field can play a crucial role in substantiating how abstraction in the lab unfolds. This may indeed close the gap between the concrete level of experimental activities and the theories informing the experiment, which also reflects the classic conundrum of connecting the three levels of representation in chemistry education (Johnstone, 2000, 2006). Chittleborough (2004) found that these representation levels can be a source of social-affective learning, and models can be helpful in identifying and relating one level to another. The ability to transfer among macroscopic, sub-microscopic, and symbolic levels is characteristic of chemistry learning, and laboratory work can be utilised to help students develop it.
Given the reality of modern laboratory education enrolling large population of students, it may be relevant to consider whether this model can still be applied to a traditional rotation system where the curriculum is predominantly expository (Domin, 1999b). I argue that there is a scope for its operationalisation, but it may need to be contextualised in a longer progression of increasing inquiry levels, such as described in Seery et al. (2019a, 2019b, 2019c). In their curriculum model for developing experimental design competence, each stage of this progression is marked with emphases on different elements of epistemic practice, with well-defined protocol experiments in the first year mainly focussing on measurement and skills acquisition. The important point here is that in the implementation of such curriculum model, laboratory instructors should provide ample time and more focus on dialogic, discursive feedback (Hickey et al., 2012; Seng and Hill, 2014). The purpose is to encourage students to reflect on the connection between what they observe and underlying theories; use theoretical concepts as epistemological resources to plan and perform actions related to the experiment; and eventually use these concepts to interpret the results. Such approach is exemplified by Jiménez-Aleixandre and Reigosa (2006) in the context of molarity-neutralisation experiment using epistemic engagement as an analytical lens.
It is worthwhile to also encourage students to verbalise their reflections and deploy them as a source of discourse in the laboratory. This accentuates the social aspect of the epistemic practice in the lab, and instructors play a substantial role as a ‘director’ (Scott et al., 2006) of how the discourse unfolds and can be geared towards a better understanding of knowledge evaluation. Indeed, the laboratory is not only a place for conducting scientific experiments, it also provides an opportunity for intellectual social interaction, in which discussions and argumentations should be encouraged (French and Russell, 2006). Incorporating student reflections has been shown to enhance metacognition and motivation (Cavilla, 2017) and promote meaningful learning (Bulte et al., 2006). Elevating these reflections from the individual to the group level, by using them as a source of laboratory discourse may also strengthen epistemic engagement, as the model illustrates.
As students develop in this progression of ever-increasing epistemic engagement, and as the curriculum allows for more scope for open inquiry, the understanding of epistemic practice associated with experimental work is supposedly more sophisticated. But even in the entirely open scientific inquiry where students design and implement experimental protocols for an unfamiliar topic (Seery et al., 2019b), it may only be accomplished if the elements of reflection, dialogue, and discourse are present in the laboratory. For the purpose of learning assessment, they can be documented in an artefact, such as reflective journals and laboratory reports. Thus, while the use of laboratory reports is already common in practice, there is a large scope for incorporating more reflective accounts of epistemic practice. Examples can be drawn from Xu and Talanquer (2013) using science writing heuristics, Lawrie et al. (2016) using wiki laboratory notebooks, Ho et al. (2021) using reflective essays, and Warren (2020), focussing on experimental and theoretical evaluation of hypothesis.
Implications for assessment
The proposed hexad model in the present paper implies that it is crucial to gear assessment towards richer accounts of various aspects of learning associated with the practice described above, with a consideration of the whole being more than the sum of its parts. Primarily due to the different ontological, methodological, and axiological assumptions tied to various ways in which the learning domains can be substantiated, a comprehensive assessment of learning in the laboratory is arguably relevant.
The notion of ‘comprehensive assessment of learning’ stems from an idea that student learning accounts for both process and outcomes, in which knowledge, skills, attitudes, and values contribute to their competency development (Milligan, 1998; Dai and Sternberg, 2004; Arcario et al., 2013; Illeris, 2018). In the literature, references to both ‘competency’ and ‘competence’ are present. Although they are often used interchangeably, nuance matters. As a concept, the concept ‘competency’ refers to cognitive abilities and skills that enable learners to solve particular problems, as well as motivational, volitional, and social capacity to utilise the solution successfully in various situations (OECD, 2007; DeBoer, 2011). In comparison, ‘competence’ in the context of the present framework and model is defined from an analytic view (Ropohl et al., 2018), as mastery of situational demands that require learners to relate latent traits (cognitive, conative, affective) to their performance and identify a common, underlying dimension. In a sense, both concepts reflect the integration of the hexad of learning domains argued in this paper, but the former is somewhat broader in scope than the latter. To get insights into the elements entailed in these definitions and the dynamic between them, multiple modalities of and approaches to eliciting student responses are required. A comprehensive assessment of learning in the laboratory provides information that indicates students’ development in all learning domains. This may manifest in a combination of direct and indirect measures, as well as formative and summative approaches (see also, Abrahams et al., 2013; Glazer, 2014; Talanquer, 2019; Agustian, 2020a). The main characteristic of such form of assessment, in the context of laboratory work as an epistemic practice, is consideration of the whole when designing a part-task. In the following, some guidelines for its design and implementation are proposed.
1. Experimental competences should be assessed in terms of underlying epistemic practice and with considerations of cognition, affect, and conation.
Because experimental work lies at the heart of laboratory education, students’ development of related competences should be assessed first and foremost. These can be differentiated into basic and advanced experimental competences. The former refers to the performance of the experiment itself, understanding of laboratory procedures, data analysis and interpretation, and application of typical practical skills (glassware, instrumental, and various laboratory techniques), whereas the latter refers to experimental design. Some of these competencies have been assessed in practice and reported in the literature, either using direct measures to assess skill performance (e.g., Abrahams et al., 2013; Towns et al., 2015) or marking laboratory reports to assess data analysis and interpretation skills. However, the advanced competence of designing an experiment is often missing, particularly where the curriculum is mainly confirmatory with prescribed protocols. It is therefore essential to scaffold the curriculum and instruction such that students are able to design an experiment and that this is assessed (see, for example, van Riesen et al., 2018; Seery et al., 2019a, 2019b, 2019c). The assessment of experimental design competence, which is exemplified with a formative approach in Seery's work, provides a large scope for a focus on understanding of epistemic practice. As argued previously, this can be done with reflective accounts on how students propose and evaluate knowledge derived from the experiment. The use of group interviews and journals can be worthwhile. Few studies also demonstrate how assessment of experimental design skills is largely guided by the curriculum, such as the case of Undergraduate Research Experience (Harsh et al., 2011; Harsh, 2016; Pagano et al., 2018; Williams and Reddish, 2018).
In Fig. 1, the three faculties of mind are modelled both on individual and group levels. While assessment pertaining to the cognitive part is presumably in place, the affective and conative domains can be gauged using validated instruments designed with such integration in mind. An example is the Meaningful Learning in the Laboratory Inventory (Galloway and Bretz, 2015a). However, the present model seeks to extend this level of integration to also address the social and epistemic domains. Researchers in chemistry education are therefore encouraged to collaborate with practitioners to develop this element of the comprehensive assessment. The body of knowledge in self determination theory is relevant. Likewise, related concepts with epistemic orientation such as epistemic affect (Efklides, 2017) and epistemic curiosity (Schmidt and Rotgans, 2021) may provide theoretical grounding. The framework proposed in this perspective paper seeks to address the overlooked aspects of chemistry education, and there is a scope for more substantiation of the affective and conative domains in chemistry education, particularly in the laboratory (Agustian, 2020a; Flaherty, 2020). This is worthwhile, if the laboratory was to be upheld as pedagogically valuable in chemistry instruction (Bretz, 2019).
2. Assessment should be directed towards developing higher-order competences.
The integration of learning domains in the context of laboratory work as an epistemic practice can be fostered by focusing on higher-order competences. These are competences that typically encompass two or more learning domains, and the way they are constructed lends itself to the contextualisation in the epistemological aspects of experimental work. For instance, critical thinking, which can be defined as ‘a process that requires a skill set useful in decision-making, similar to the research process’ (Wisdom and Leavitt, 2015, p. xix). The cognitive part of critical thinking has been attributed to analysis, inference, evaluation, interpretation, and explanation, whereas the attitude part is characteristic of affective constructs such as open-mindedness, appreciation of evidence, as well as analytical, orderly, systematic, and inquisitive traits (Nakhleh et al., 2002). Self-regulation may also play a vital role here, which is typically conative. Other examples of higher-order competences that are highly relevant here are collaborative problem solving, argumentation, and metacognition, as well as experimental design, as argued before. The task for laboratory instructors and researchers is to design elements of the comprehensive assessment such that these constructs are contextualised in the pursuit of evaluating and legitimising knowledge. Specific literature in the chemistry laboratory is scarce, but inspirations can be drawn from an example such as Collegiate Learning Assessment (Klein et al., 2007; Shavelson, 2008), in which open-ended tasks are used to directly assess competences related to problem solving, critical thinking, analytical reasoning, and communication. In the laboratory context, this may be transposed into direct assessment rubric for higher-order competences, with items derived from previous works such as Zoller and Pushkin (2007), Sandi-Urena et al. (2012), and Yoon et al. (2014).
3. The part-task elements in the assessment should be formulated with the whole framework in mind.
Although this type of assessment seeks to be comprehensive, it may not be exhaustive, but that is not the intention in the first place. Particularly in higher education, comprehensive assessment affords both faculty and students opportunities to use insights from the assessment results to improve teaching and learning (McGourty et al., 1998). This is possible with its formative characteristics, in which instructors give and receive feedback on both outcomes and process. Development in laboratory education research also demonstrates how peer feedback is beneficial and can resolve the common problem of limited time and resources (Kind et al., 2011; Galloway and Bretz, 2016; Mathabathe and Potgieter, 2017; Seery et al., 2017; Seery et al., 2019a). The comprehensive assessment of learning in the laboratory also entails elements pertaining to pre- and post-laboratory work. Like the formulation of other part-task elements, they should also consider the whole framework. An example can be derived from the design considerations of pre-laboratory activities (Agustian and Seery, 2017), which is focused on the cognitive, affective, and psychomotor domains, operationalised within complex learning framework.
4. Social interactions in the laboratory should be assessed in elucidating educational processes.
The argument for more focus on the social domain of learning in the laboratory presented earlier in this paper implicates that assessment is no longer an individual prerogative. While other elements of the comprehensive assessment may be administered at individual level, it is also relevant to consider group level. The model provides a contextualisation of how this could be operationalised in terms of epistemic practice. A wealth of empirical literature in collaborative learning may prove useful, but especially in the context of chemistry education (Sadler et al., 2011; Yezierski, 2014), with the use of focus group interviews and observation protocols including epistemic engagement and laboratory discourse.
Conclusions
Research on student learning in the laboratory provides us with a multidimensional view of learning, which encompasses not only the cognitive domain but also affective, conative, psychomotor, social, and epistemic domains. Although references to these domains and related constructs have been identified, they are usually treated in isolation, or partly connected to one another. In the present article, I have argued for a more integrated approach to research on student learning in chemistry education, particularly in the laboratory, where the ever-present psychomotor domain distinguishes it from the mainstream context of classroom instructions. Particularly the social and epistemic domains are often overlooked in laboratory education research, but there is also a scope for empirical studies into the other domains. In an attempt to synthesise the hexad of learning domains, I have conceptualised laboratory work as an epistemic practice, in which the dynamic between various aspects of learning can be substantiated. Recommendations regarding the operationalisation of the model have also been presented, with regards to curriculum design, instruction in the laboratory, and comprehensive assessment of student learning.
Conflicts of interest
There are no conflicts to declare.
Acknowledgements
The author thanks Professor Bente Gammelgaard for the critical comments. The work presented in this article is supported by Novo Nordisk Foundation, grant number NNF 18SA0034990.
Notes and references
- Abrahams I., Reiss M. J. and Sharpe R. M., (2013). The assessment of practical work in school science, Stud. Sci. Educ., 49(2), 209–251.
- Agustian H. Y., (2020a), Students’ learning experience in the chemistry laboratory and their views of science: In defence of pedagogical and philosophical validation of undergraduate chemistry laboratory education, The University of Edinburgh, retrieved from https://era.ed.ac.uk/handle/1842/36802.
- Agustian H. Y., (2020b), Students’ understanding of the nature of science in the context of an undergraduate chemistry laboratory, Electron. J. Res. Sci. Math. Educ., 24(2), 56–85.
- Agustian H. Y. and Seery M. K., (2017), Reasserting the role of pre-laboratory activities in chemistry education: A proposed framework for their design, Chem. Educ. Res. Pract., 18(4), 518–532.
- Agustian H. Y., Finne L., Tarp J., Pedersen M. I., Christiansen F. V, Gammelgaard B. and Nielsen J. A., (n.d.), Learning outcomes of university chemistry teaching in laboratories: A systematic review of empirical literature, submitted.
- Andersson J., Garrison J. and Östman L., (2018), Empirical Philosophical Investigations in Education and Embodied Experience, Cham: Palgrave MacMillan.
- Annis D. B., (1982), Epistemology naturalized, Metaphilosophy, 13(3/4), 201–208.
- Arcario P., Eynon B., Klages M. and Polnariev B., (2013), Closing the loop: How we better serve our students through a comprehensive assessment process, Metropol. Univ., 24(2), 21–37.
- Ausubel D. P., (2000), The Acquisition and Retention of Knowledge: A Cognitive View, Dordrecht: Springer Science & Business Media.
- Barrie S. C., Bucat R. B., Buntine M. A., Burke da Silva, K., Crisp, G. T., George, A. V. and Yeung, A., (2015), Development, evaluation and use of a student experience survey in undergraduate science laboratories, Int. J. Sci. Educ., 37(11), 1795–1814.
- Bartels J. M., Magun-Jackson S. and Ryan J. J., (2011), Achievement goals, volitional regulation and help-seeking among college students: A multiple goal analysis, Individ. Differ. Res., 9(1), 41–51.
- Biggs J. and Tang C., (2007), Kinds of knowledge and levels of understanding, in Teaching for Quality Learning at University, Maidenhead: Open University Press.
- Biggs J. and Tang C., (2011), Teaching for Quality Learning at University, Maidenhead: Open University Press.
- Bledsoe K. E. and Flick L., (2012), Concept development and meaningful learning among electrical engineering students engaged in a problem-based laboratory experience, J. Sci. Educ. Technol., 21(2), 226–245.
- Bretz S. L., (2019), Evidence for the importance of laboratory courses [Editorial], J. Chem. Educ., 96(2), 193–195.
- Bretz S. L., Fay M., Bruck L. B. and Towns M. H., (2013), What faculty interviews reveal about meaningful learning in the undergraduate chemistry laboratory, J. Chem. Educ., 90(3), 281–288.
- Bulte A., Westbroek H., de Jong O. and Pilot A., (2006), A research approach to designing chemistry education using authentic practices as contexts, Int. J. Sci. Educ., 28(9), 1063–1086.
- Cavilla D., (2017), The effects of student reflection on academic performance and motivation, SAGE Open, 7(3), 1–13.
- Cessna S. G., Kishbaugh T. L. S., Neufeld D. G. and Cessna G. A., (2009), A multiweek, problem-based laboratory project using phytoremediation to remove copper from soil: General chemistry labs for teaching thermodynamics and equilibrium, J. Chem. Educ., 86(6), 726–729.
- Chittleborough G. D., (2004), The role of teaching models and chemical representations in developing students’ mental models of chemical phenomena, Curtin University of Technology, retrieved from https://espace.curtin.edu.au/handle/20.500.11937/763.
- Cooper M. M. and Stowe R. L., (2018), Chemistry education research – From personal empiricism to evidence, theory, and informed practice, Chem. Rev., 118(12), 6053–6087.
- Dai D. Y. and Sternberg R. J., (2004), Beyond cognitivism: Toward an integrated understanding of intellectual functioning and development, in Dai D. Y. and Sternberg R. J. (ed.), Motivation, Emotion, and Cognition: Integrative Perspectives on Intellectual Functioning and Development, Mahwah: Lawrence Erlbaum Associates, pp. 3–40.
- Dalgety J., Coll R. K. and Jones A., (2003), Development of chemistry attitudes and experiences questionnaire (CAEQ), J. Res. Sci. Teach., 40(7), 649–668.
- DeBoer G. E., (2011), The globalization of science education, J. Res. Sci. Teach., 48(6), 567–591.
- DeKorver B. K. and Towns M. H., (2015), General chemistry students’ goals for chemistry laboratory coursework, J. Chem. Educ., 92(12), 2031–2037.
- Dolcos F., Katsumi Y., Moore M., Berggren N., de Gelder B., Derakshan N., Dolcos S., (2020), Neural correlates of emotion-attention interactions: From perception, learning, and memory to social cognition, individual differences, and training interventions, Neurosci. Biobehav. Rev., 108, 559–601.
- Domin D. S., (1999a), A content analysis of general chemistry laboratory manuals for evidence of higher-order cognitive tasks, J. Chem. Educ., 76(1), 109–112.
- Domin D. S., (1999b), A review of laboratory instruction styles, J. Chem. Educ., 76(4), 543.
- Duschl R. A., (2008), Science education in three-part harmony: Balancing conceptual, epistemic, and social learning goals, Rev. Res. Educ., 32, 268–291.
- Duschl R. A. and Grandy R., (2013), Two views about explicitly teaching nature of science, Sci. Educ., 22(9), 2109–2139.
- Efklides A., (2017), Affect, epistemic emotions, metacognition, and self-regulated learning, Teach. Coll. Rec., 119(13), 1–22.
- Erduran S. and Dagher Z. R., (2014), Reconceptualizing the Nature of Science for Science Education, Dordrecht: Springer.
- Erduran S. and Kaya E., (2019), Transforming Teacher Education through the Epistemic Core of Chemistry: Empirical Evidence and Practical Strategies, Dordrecht: Springer.
- Feisel L. D. and Rosa A. J., (2005), The role of the laboratory in undergraduate engineering education, J. Eng. Educ., 94(1), 121–130.
- Flaherty A. A., (2020), A review of affective chemistry education research and its implications for future research, Chem. Educ. Res. Pract., 21(3), 698–713.
- Fraser B. J., Tobin K. G. and McRobbie C. J., (2012), Second International Handbook of Science Education, Dordrecht: Springer Science & Business Media.
- French D. P. and Russell C. P., (2006), Converting your lab from verification to inquiry, in Mintzes J. J. and Leonard W. H. (ed.), Handbook of College Science Teaching, Arlington: National Science Teacher Association Press.
- Galloway K. R. and Bretz S. L., (2015a), Development of an assessment tool to measure students’ meaningful learning in the undergraduate chemistry laboratory, J. Chem. Educ., 92(7), 1149–1158.
- Galloway K. R. and Bretz S. L., (2015b), Measuring meaningful learning in the undergraduate chemistry laboratory: A national, cross-sectional study. J. Chem. Educ., 92(12), 2006–2018.
- Galloway K. R. and Bretz S. L., (2015c), Using cluster analysis to characterize meaningful learning in a first-year university chemistry laboratory course, Chem. Educ. Res. Pract., 16(4), 879–892.
- Galloway K. R. and Bretz S. L., (2016), Video episodes and action cameras in the undergraduate chemistry laboratory: Eliciting student perceptions of meaningful learning, Chem. Educ. Res. Pract., 17(1), 139–155.
- Galloway K. R., Malakpa Z. and Bretz S. L., (2016), Investigating affective experiences in the undergraduate chemistry laboratory: Students’ perceptions of control and responsibility, J. Chem. Educ., 93(2), 227–238.
- George-Williams S. R., Soo J. T., Ziebell A. L., Thompson C. D. and Overton T. L., (2018), Inquiry and industry inspired laboratories: The impact on students’ perceptions of skill development and engagements, Chem. Educ. Res. Pract., 19(2), 583–596.
- Glazer N., (2014), Formative plus summative assessment in large undergraduate courses: Why both? Int. J. Teach. Learn. High. Educ., 26(2), 276–286.
- Goldin G. A., (2019), Exploring a conative perspective on mathematical engagement, in Chamberlin S. A. and Sriraman B. (ed.), Affect in Mathematical Modeling, Cham: Springer Nature Switzerland.
- Gollub J. P., Berthenthal M. W., Labov J. B. and Curtis P. C., (2002), Learning and Understanding: Improving Advanced Study of Mathematics and Science in US High Schools, Washington, DC: National Academies Press.
- Greene J. A., Sandoval W. A. and Bråten I., (2016), Handbook of epistemic cognition, in Handbook of Epistemic Cognition, New York: Routledge.
- Harsh J. A., (2016), Designing performance-based measures to assess the scientific thinking skills of chemistry undergraduate researchers, Chem. Educ. Res. Pract., 17(4), 808–817.
- Harsh J. A., Maltese A. V and Tai R. H., (2011), Undergraduate research experiences from a longitudinal perspective, J. Coll. Sci. Teach., 41(1), 84–91.
- Hickey D. T., Taasoobshirazi G. and Cross D., (2012), Assessment as learning: Enhancing discourse, understanding, and achievement in innovative science curricula, J. Res. Sci. Teach., 49(10), 1240–1270.
- Ho K., Smith S. R., Venter C. and Clark D. B., (2021), Case study analysis of reflective essays by chemistry post-secondary students within a lab-based community service learning water project, Chem. Educ. Res. Pract., 22(4), 973–984.
- Hodson D., (1993), Re-thinking old ways: Towards a more critical approach to practical work in school science, Stud. Sci. Educ., 22(1), 85–142.
- Hofstein A. and Kind P. M., (2012), Learning in and from science laboratories, in Fraser B. J., Tobin K. G. and McRobbie C. J. (ed.), Second International Handbook of Science Education, Dordrecht: Springer Science & Business Media.
- Hofstein A. and Lunetta V. N., (1982), The role of the laboratory in science teaching: Neglected aspects of research, Rev. Educ. Res., 52(2), 201–217.
- Hofstein A. and Lunetta V. N., (2003), The laboratory in science education: Foundations for the twenty-first century, Sci. Educ., 88(1), 28–54.
- House C., Meades G. and Linenberger K. J., (2016), Approaching a conceptual understanding of enzyme kinetics and inhibition: Development of an active learning inquiry activity for prehealth and nonscience majors, J. Chem. Educ., 93(8), 1397–1400.
- Hutchins E., (2001), Distributed cognition, in International Encyclopedia of Social and Behavioral Sciences, pp. 2068–2072.
- Hwang W. Y., Kongcharoen C. and Ghinea G., (2017), Influence of students’ affective and conative factors on laboratory learning: Moderating effect of online social network attention, Eurasia J. Math., Sci. Technol. Educ., 13(3), 1013–1024.
- Illeris K., (2018), A comprehensive understanding of human learning, in Illeris K. (ed.), Contemporary Theories of Learning: … in Their Own Words, London: Routledge, pp. 1–14.
- Jiménez-Aleixandre M. P. and Crujeiras B., (2017), Epistemic practices and scientific practices in science education, in Taber K. S. and Akpan B. (ed.), Science Education: An International Course Companion, Rotterdam: Sense Publishers, pp. 69–80.
- Jiménez-Aleixandre M. P. and Reigosa C., (2006), Contextualizing practices across epistemic levels in the chemistry laboratory, Sci. Educ., 90(4), 707–733.
- Johnstone A. H., (2000), Teaching of chemistry-logical or psychological? Chem. Educ. Res. Pract., 1(1), 9–15.
- Johnstone A. H., (2006), Chemical education research in Glasgow in perspective, Chem. Educ. Res. Pract., 7(2), 49–63.
- Kind P. M., Kind V., Hofstein A. and Wilson J., (2011), Peer argumentation in the school science laboratory: Exploring effects of task features, Int. J. Sci. Educ., 33(18), 2527–2558.
- Kirschner P. A., (1992), Epistemology, practical work and academic skills in science education, Sci. Educ., 1(3), 273–299.
- Klein S., Benjamin R., Shavelson R. and Bolus R., (2007), The collegiate learning assessment: Facts and fantasies, Eval. Rev., 31(5), 415–439.
- Knorr-Cetina K. D., (1999), Epistemic Cultures: How the Sciences Make Knowledge, Cambridge: Harvard University Press.
- Koballa Jr. T. R. and Glynn S. M., (2007), Attitudinal and motivational constructs in science learning, in Abell S. K. and Lederman N. G. (ed.), Handbook of Research on Science Education, New York: Routledge.
- Kupermintz H., (2002), Affective and conative factors as aptitude resources in high school science achievement, Educ. Assess., 8(2), 123–137.
- Lastusaari M., Laakkonen E. and Murtonen M., (2016), ChemApproach: Validation of a questionnaire to assess the learning approaches of chemistry students, Chem. Educ. Res. Pract., 17(4), 723–730.
- Lawrie G. A., Grøndahl L., Boman S. and Andrews T., (2016), Wiki laboratory notebooks: Supporting student learning in collaborative inquiry-based laboratory experiments, J. Sci. Educ. Technol., 25(3), 394–409.
- Lazarowitz R., (2007), High school biology curricula development: Implementation, teaching, and evaluation from the twentieth to the twenty-first century, in Abell S. K. and Lederman N. G. (ed.), Handbook of Research on Science Education, New York: Routledge.
- Leighton J. P. and Gierl M. J., (2011), The Learning Sciences in Educational Assessment: The Role of Cognitive Models, Cambridge: Cambridge University Press.
- Liebig J. von., (1892), Justus von Liebig: An autobiographical sketch, Popul. Sci. Mon., 40(March), 1–15.
- Limniou M., Papadopoulos N. and Whitehead C., (2009), Integration of simulation into pre-laboratory chemical course: Computer cluster versus WebCT, Computers and Education, 52(1), 45–52.
- Littledyke M., (2008), Science education for environmental
awareness: Approaches to integrating cognitive and affective domains, Environ. Educ. Res., 14(1), 1–17.
- Mathabathe K. C. and Potgieter M., (2017), Manifestations of metacognitive activity during the collaborative planning of chemistry practical investigations, Int. J. Sci. Educ., 39(11), 1465–1484.
- Matthews M. R., (2018), History, Philosophy and Science Teaching – New Perspectives, Cham: Springer.
- Matz R. L., Rothman E. D., Krajcik J. S. and Banaszak Holl M. M., (2012), Concurrent enrollment in lecture and laboratory enhances student performance and retention, J. Res. Sci. Teach., 49(5), 659–682.
- McGourty J., Sebastian C. and Swart W., (1998), Developing a comprehensive assessment program for engineering education, J. Eng. Educ., 87(4), 355–361.
- Milligan F., (1998), Defining and assessing competence: The distraction of outcomes and the importance of educational process, Nurse Educ. Today, 18(4), 273–280.
- Miyake N. and Kirschner P. A., (2014), The social and interactive dimensions of collaborative learning, in Sawyer R. K. (ed.), The Cambridge Handbook of the Learning Sciences, Cambridge: Cambridge University Press.
- Nakhleh M. B., Polles J. and Malina E., (2002), Learning chemistry in a laboratory environment, in Gilbert J. K., de Jong O., Justi R., Treagust D. F. and van Driel J. H. (ed.), Chemical Education: Towards Research-Based Practice, Dordrecht: Springer, pp. 69–94.
- OECD, (2007), PISA 2006: Science Competencies for Tomorrow's World: Executive Summary, Paris: Organisation for Economic Co-operation and Development.
- Oliver-Hoyo M. T., Allen D., Hunt W. F., Hutson J. and Pitts A., (2004), Effects of an active learning environment: Teaching innovations at a research institution. J. Chem. Educ., 81(3), 441.
- Pagano J. K., Jaworski L., Lopatto D. and Waterman R., (2018), An inorganic chemistry laboratory course as research, J. Chem. Educ., 95(9), 1520–1525.
- Pazicni S., Wink D. J., Donovan A., Conrad J. A., Darr J. P., Theall R. A. M., Richter-Egger D. L., Villalta-Cerdas A. and D. R. Walker, (2021), The American Chemical Society general chemistry performance expectations project: From task force to distributed process for implementing multidimensional learning, J. Chem. Educ.
- Pintrich P. R., (2004), A conceptual framework for assessing motivation and self-regulated learning in college students, Educ. Psychol. Rev., 16(4), 385–407.
- Reeves T. C., (2006), How do you know they are learning? The importance of alignment in higher education, Int. J. Learn. Technol., 2(4), 294.
- Reid N. and Shah I., (2007), The role of laboratory work in university chemistry, Chem. Educ. Res. Pract., 8(2), 172–185.
- Rennie L. J., (2001), Communicating science through interactive science centres: A research perspective, in S. M. Stocklmayer, M. M. Gore and C. Bryant (ed.), Science Communication in Theory and Practice, Dordrecht: Springer Science & Business Media, pp. 107–121.
- Ropohl M., Nielsen J. A., Olley C., Rönnebeck S. and Stables K., (2018), The concept of competence and its relevance for science, technology and mathematics education, in Dolin J. and Evans R. (ed.), Transforming Assessment through an Interplay between Practice, Research and Policy, Dordrecht: Springer.
- Rubin A. and Tamir P., (1988), Meaningful learning in the school laboratory, Am. Biol. Teach., 50(8), 477–482.
- Rudd II J. A., Greenbowe T. J., Hand B. M. and Legg M. J., (2001), Using the science writing heuristic to move toward an inquiry-based laboratory curriculum: An example from physical equilibrium, J. Chem. Educ., 78(12), 1680.
- Russell C. B. and Weaver G., (2008), Student perceptions of the purpose and function of the laboratory in science: A grounded theory study, Int. J. Scholar. Teach. Learn., 2(2).
- Russell C. B. and Weaver G. C., (2011), A comparative study of traditional, inquiry-based, and research-based laboratory curricula: Impacts on understanding of the nature of science, Chem. Educ. Res. Pract., 12(1), 57–67.
- Sadler T., Puig A. and Trutschel B., (2011), Laboratory instructional practices inventory: A tool for assessing the transformation of undergraduate laboratory instruction, J. Coll. Sci. Teach., 41(1), 25–32.
- Sandi-Urena S., Cooper M. and Stevens R., (2012), Effect of cooperative problem-based lab instruction on metacognition and problem-solving skills, J. Chem. Educ., 89(6), 700–706.
- Sawyer R. K., (2014), The Cambridge Handbook of the Learning Sciences (2nd ed.), New York: Cambridge University Press.
- Schmidt H. G. and Rotgans J. I., (2021), Epistemic curiosity and situational interest: Distant cousins or identical twins? Educ. Psychol. Rev., 33(1), 325–352.
- Schwab J. J., (1967), Problems, topics, and issues, Quest, 9(1), 2–27.
- Scott P. H., Mortimer E. F. and Aguiar O. G., (2006), The tension between authoritative and dialogic discourse: A fundamental characteristic of meaning making interactions in high school, Sci. Educ., 90(4), 605–631.
- Seery M. K., (2020), Establishing the laboratory as the place to learn how to do chemistry, J. Chem. Educ., 97(6), 1508–1511.
- Seery M. K., Agustian H. Y., Doidge E. D., Kucharski M. M., O’Connor H. M. and Price A., (2017), Developing laboratory skills by incorporating peer-review and digital badges, Chem. Educ. Res. Pract., 18(3).
- Seery M. K., Agustian H. Y. and Lambert T. O., (2019a), Teaching and assessing technical competency in the chemistry laboratory, in Seery M. K. and McDonnell C. (ed.), Teaching Chemistry in Higher Education: A Festschrift in Honour of Professor Tina Overton, Dublin: Creatach Press, pp. 353–365.
- Seery M. K., Agustian H. Y. and Zhang X., (2019b), A framework for learning in the chemistry laboratory, Isr. J. Chem., 59(6–7), 546–553.
- Seery M. K., Jones A. B., Kew W. and Mein T., (2019c), Unfinished recipes: Structuring upper-division laboratory work to scaffold experimental design skills, J. Chem. Educ., 96(1), 53–59.
- Seng M. G. J. and Hill M., (2014), Using a dialogical approach to examine peer feedback during chemistry investigative task discussion, Res. Sci. Educ., 44(5), 727–749.
- Seung E., Choi A. and Pestel B., (2016), University students’ understanding of chemistry processes and the quality of evidence in their written arguments, Eurasia J. Math., Sci. Technol. Educ., 12(4), 991–1008.
- Shavelson R., (2008), The Collegiate Learning Assessment. Forum for the Future of Higher Education, Cambridge: Ford Policy Forum.
- Shibley I. A. and Zimmaro D. M., (2002), The influence of collaborative learning on student attitudes and performance in an introductory chemistry laboratory. J. Chem. Educ., 79(6), 745.
- Snow R. E. and Jackson III D. N., (1997), Individual differences in conation: Selected constructs and measures, Motiv.: Theory Res., 1522(310), 71–99.
- Stephenson N. S. and Sadler-Mcknight N. P., (2016), Developing critical thinking skills using the Science Writing Heuristic in the
chemistry laboratory, Chem. Educ. Res. Pract., 17(1), 72–79.
- Stephenson N. S., Duffy E. M., Day E. L., Padilla K., Herrington D. G., Cooper M. M. and Carmel J. H., (2020), Development and validation of scientific practices assessment tasks for the general chemistry laboratory, J. Chem. Educ., 97(4), 884–893.
- Taber K. S., (2000), Chemistry lessons for universities? A review of constructivist ideas, Univ. Chem. Educ., 4(2), 26–35.
- Talanquer V., (2019), Assessing for chemical thinking, in M. Schultz, S. Schmid and G. A. Lawrie (ed.), Research and Practice in Chemistry Education, Dordrecht: Springer, pp. 123–133.
- Teo T. W., Tan K. C. D., Yan Y. K., Teo Y. C. and Yeo L. W., (2014), How flip teaching supports undergraduate chemistry laboratory learning, Chem. Educ. Res. Pract., 15, 550–567.
- Thijs A. and van den Akker J., (2009), Curriculum in Development, Retrieved from https://issuu.com/slocom2/docs/curriculum-in-development.
- Towns M. H., Harwood C. J., Robertshaw M. B., Fish J. and O’Shea K., (2015), The digital pipetting badge: A method to improve student hands-on laboratory skills, J. Chem. Educ., 92(12), 2038–2044.
- Underwood S. M., Posey L. A., Herrington D. G., Carmel J. H. and Cooper M. M., (2018), Adapting assessment tasks to support three-dimensional learning, J. Chem. Educ., 95(2), 207–217.
- van Merriënboer J. J. G. and Kirschner P. A., (2017), Ten Steps to Complex Learning: A Systematic Approach to Four-Component Instructional Design, London: Routledge.
- van Riesen S., Gijlers H., Anjewierden A. and de Jong T., (2018), Supporting learners’ experiment design, Educ. Technol. Res. Dev., 66(2), 475–491.
- Warren A. R., (2020), Impact of Bayesian updating activities on student epistemologies, Phys. Rev. Phys. Educ. Res., 16(1), 10101.
- Weaver G. C., Russell C. B. and Wink D. J., (2008), Inquiry-based and research-based laboratory pedagogies in undergraduate science, Nat. Chem. Biol., 4(10), 577–580.
- Williams L. C. and Reddish M. J., (2018), Integrating primary research into the teaching lab: Benefits and impacts of a one-semester CURE for physical chemistry, J. Chem. Educ., 95(6), 928–938.
- Wilson M. V. and Wilson E., (2017), Authentic performance in the instrumental analysis laboratory: Building a visible spectrophotometer prototype, J. Chem. Educ., 94(1), 44–51.
- Winberg T. M. and Berg C. A. R., (2007), Students’ cognitive focus during a chemistry laboratory exercise: Effects of a computer-simulated prelab, J. Res. Sci. Teach., 44(8), 1108–1133.
- Wisdom S. and Leavitt L., (2015), Handbook of Research on Advancing Critical Thinking in Higher Education, Hershey: Information Science Reference.
- Xu H. and Talanquer V., (2013), Effect of the level of inquiry of lab experiments on general chemistry students’ written reflections, J. Chem. Educ., 90(1), 21–28.
- Yezierski E. J., (2014), Observation as a tool for investigating chemistry teaching and learning, in Bunce D. M. and Cole R. S. (ed.), Tools of Chemistry Education Research, Washington, DC: American Chemical Society, pp. 11–29.
- Yoon H., Woo A. J., Treagust D. and Chandrasegaran A. L., (2014), The efficacy of problem-based learning in an analytical laboratory course for pre-service chemistry teachers, Int. J. Sci. Educ., 36(1), 79–102.
- Zaghloul A. R. M., (2001), Assessment of lab work: A three-domain model; Cognitive, affective, and psychomotor. ASEE Annual Conference Proceedings, pp. 2279–2285.
- Zhang J. and Patel V. L., (2006), Distributed cognition, representation, and affordance, Pragmat. Cogn., 14(2), 333–341.
- Zoller U. and Pushkin D., (2007), Matching higher-order cognitive skills (HOCS) promotion goals with problem-based laboratory practice in a freshman organic chemistry course, Chem. Educ. Res. Pract., 8(2), 153–171.
|
This journal is © The Royal Society of Chemistry 2022 |
Click here to see how this site uses Cookies. View our privacy policy here.