Investigating the role of multiple categorization tasks in a curriculum designed around mechanistic patterns and principles†
Received
28th September 2021
, Accepted 5th February 2022
First published on 7th February 2022
Abstract
The goal of the present work is to extend an online reaction categorization task as a research instrument to a formative assessment tool of students’ knowledge organization for organic chemistry reactions. Herein, we report our findings from administering the task with undergraduate students in Organic Chemistry II, at a large, research intensive Canadian university, including the relationship between instrument and exam scores. The online categorization task uses 25 reaction cards that participants were asked to sort first into categories of their choosing (i.e., an open sort) then into the mechanistic categories defined in a Patterns of Mechanisms curriculum (i.e., a closed sort). We observed a small, significant correlation between how learners chose to organize their knowledge (i.e., open sort) and their cued ability (i.e., match with expert sort) at the beginning of the Organic Chemistry II course (N = 65, r = 0.28, p = 0.026). We conducted a correlation analysis between students’ scores on the open and closed sort tasks and academic achievement. We found a strong relationship between the scores in the online categorization tasks and Organic Chemistry II exams, especially from the closed sort tasks (N = 43, r = 0.70, p = 0.000). To date, no other discipline specific card-sort tasks have shown such a strong correlation with final assessment grades. We also found an increasing relationship between students’ choice and ability over time as students developed their expertise in the domain. This work also added evidence to the validity and reliability of the organic card-sort instrument, through multiple measures. Educators and students could use the card sort task as a self-assessment measure and as part of classroom activities related to mechanistic analysis. Future work is needed to investigate how card sort tasks of this type are connected with expertise in other settings.
Introduction
As efforts towards curricular reforms continue, so does the increased need for the development of effective instruments for educators to evaluate the efficacy of curricular changes. The University of Ottawa implemented a transformed “Patterns of Mechanism” Organic chemistry curriculum that emphasises the underlying mechanistic patterns (Fig. 1) that govern reactions to promote meaningful learning, and the progression of expertise in Organic Chemistry (Flynn and Ogilvie, 2015). The role of pattern recognition as a fundamental skill in science has been highlighted in The Next Generation Science Standards framework (National Research Council, 2012). This framework places the importance not only on identifying patterns but using these patterns as a tool to seek the underlying cause of a phenomenon and formulate questions about this phenomenon. The ability to create meaningful patterns in a domain has long been attributed as a key feature of expertise, where an expert will often notice deep features that novices will overlook (Bransford et al., 2010). Previous work evaluating the “Patterns of Mechanism” curriculum implemented an online categorization task to evaluate changes in expertise with respect to how students choose to see connections between reactions as well as their ability to see the underlying mechanistic patterns (Lapierre and Flynn, 2020). That work illustrated a potential lack of alignment between the expertise demonstrated in how a participant chooses to sort and their ability when cued, proposing the question of what relationship, if any, exists between various sorting contexts. This study aims to expand on that work, investigating the connections of multiple different categorization tasks including open and closed sorts, varied stakes in a “Mechanistic patterns and principles” curriculum, as well as the relationship to academic performance. In doing so, this work aims to increase the validity and reproducibility of the online categorization task as a tool for instructors to measure the formation of expertise as students’ progress through the Organic Chemistry curriculum, and potentially to identify students who may have lower achievement in the course and benefit from additional support.
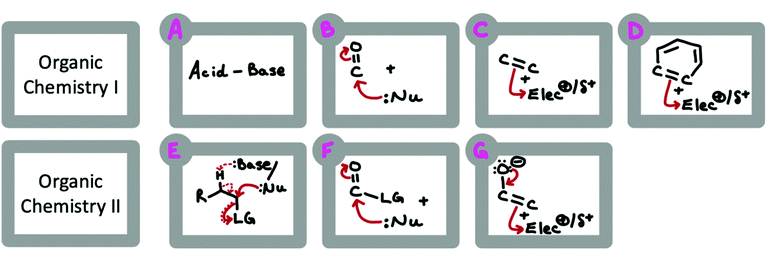 |
| Fig. 1 Reactivity portion of the curriculum, with two examples. | |
Transformed organic chemistry curriculum: patterns of mechanisms
Organic chemistry curricula are traditionally organized using a functional group approach. This approach is thought to emphasize the structural features of a reaction rather than the mechanistic patterns and principles of reactivity that govern the reactions (Graulich and Bhattacharyya, 2017). With an organization by structural features, there are limited opportunities for learners to identify underlying concepts and patterns, although these are the skills needed to think like experts, who organize their thinking by patterns and principles (Chi et al., 1981; Galloway et al., 2018). Organic chemistry is described as difficult and confusing for students (Bradley et al., 2002; Anderson and Bodner, 2008), with students relying on rote memorization demonstrating little understanding of the underlying mechanisms (Cruz-Ramírez De Arellano et al., 2014). These challenges are further exacerbated by students possessing challenges relating to understanding the mechanistic language of organic chemistry (Bhattacharyya and Bodner, 2005; Strickland et al., 2010; Grove and Bretz, 2012; Bodé and Flynn, 2016; Flynn and Featherstone, 2017; Webber and Flynn, 2018) and concepts of reactivity in organic chemistry (Anderson and Bodner, 2008; Grove and Bretz, 2012; Anzovino and Bretz, 2015; Bodé and Flynn, 2016; Webber and Flynn, 2018).
The University of Ottawa implemented a new Patterns of mechanisms organic chemistry curriculum (Flynn and Ogilvie, 2015) structured around the underlying mechanistic patterns that govern reactions (Fig. 1). This curricular organization intends to highlight the principles of reactivity and explicitly outlines the meaningful patterns between reactions that experts describe (Galloway et al., 2018). This two-term curriculum begins with a section on structure and the electron-pushing formalism (not shown) (Flynn and Featherstone, 2017; Galloway et al., 2017), then organizes reactions in a gradient of difficulty, where simpler acid–base reactions are used to help students learn the core principles of reactivity before moving to more complex reactions. This progression provides students with the tools to better understand the patterns that connect these reactions while attempting to promote meaningful learning and transferability to other disciplines.
Since its implementation, a design-based research approach (Cobb et al., 2003) to curricular evaluation has been underway to investigate the impacts of the curriculum on how students place meaning to the language of organic chemistry (Flynn and Featherstone, 2017; Galloway et al., 2017), problem solving skills (Webber and Flynn, 2018), how students organize their knowledge (Galloway et al., 2018, 2019), and the overall curriculum design (Raycroft and Flynn, 2019). While the previous studies are an essential part of the curricular evaluation, using the instruments from these studies in courses is not very practical for educators as many include lengthy qualitative interviews or detailed exam analyses. The goal of the present work is to extend work establishing the online categorization task as an instrument that can easily allow educators to capture students’ knowledge organization around organic chemistry reactions, reflective of their expertise as they progress through the curriculum.
Theoretical framework
Differences between novices and experts
In this study, we use a relative research approach to expertise (Chi, 2006). In that approach, individuals who are considered experts within a domain are more knowledgeable, such as a professor, and can be compared with someone who is considered a novice and has less knowledge, such as a student. This approach is contrasted against the absolute research approach to expertise that assumes that expertise arises from innate talent. A relative approach to expertise places expertise on a continuum and emphasizes the goal of understanding how a novice can become more proficient in a domain, with the underlying assumption that anyone can become an expert (Chi, 2006). Because we are interested in student learning and development of expertise, the relative approach is most suitable.
Experts within a domain are characterized by several principles (Fig. 2). Their high level of expertise is seen (1) in the patterns that they deem meaningful and (2) in the way they organize their knowledge (Bransford et al., 2010). Foundational work by Chi et al. (1981) in physics education illustrated the utility of a categorization task in eliciting an individual's organization of knowledge. When experts in physics were confronted with a problem, experts were more likely to organize their knowledge around the underlying features of the problem, whereas novices tended to organize their knowledge around surface features. These differences between novices and experts were attributed to experts possessing a more interconnected knowledge structure, while novices possessed a developing knowledge structure that can become more interconnected as expertise grows (Acton et al., 1994). Additionally, (3) research in expertise has demonstrated that experts in a domain possess conditionalized knowledge. Conditionalized knowledge is described as an expert's ability to identify the specific subset of knowledge required to answer a problem, from their larger body of knowledge. (4) Experts possess effortless retrieval of relevant information when compared to novices and (5) are fluently able to adapt their knowledge to new situations (Bransford et al., 2000; Chi et al., 2014).
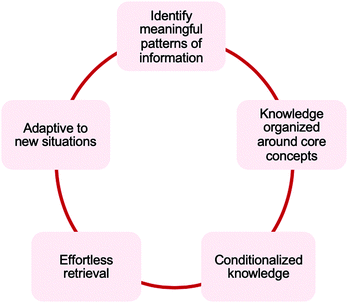 |
| Fig. 2 Key features of an expert. | |
Categorization (or card sorting) tasks serve as one of many available approaches used to investigate the differences in expertise in multiple areas of discipline-based education research (Chi et al., 1981; Smith, 1992; McCauley et al., 2005; Domin et al., 2008; Lin and Singh, 2010; Mason and Singh, 2011; Smith et al., 2013; Irby et al., 2016; Krieter et al., 2016; Graulich and Bhattacharyya, 2017; Galloway et al., 2019). When doing an open sort task, participants create categories of their own choosing (Spencer, 2009). An open categorization is often used to investigate the patterns or similarities an individual naturally determines as meaningful between various items (e.g., reaction cards, problems, or images). In contrast, a closed sort task provides participants with pre-determined categories that asks participants to place cards/items within these categories (Spencer, 2009). Closed sort tasks can be a useful tool to evaluate a student's expertise when provided with the hypothesized deep features connecting these items (Lapierre and Flynn, 2020). Using pre-determined categories (closed sort tasks) has resulted in increased probability of participants sorting by the deep features compared to open sort tasks, based on previous work in both chemistry (Krieter et al., 2016; Lapierre and Flynn, 2020) and biology (Smith et al., 2013; Bissonnette et al., 2017) education research.
The present study builds on previous research conducted by our group that investigated the differences between novices and experts in organic chemistry using a categorization task with organic chemistry reactions on cards in an interview setting (Galloway et al., 2018, 2019; Lapierre and Flynn, 2020). That work identified four main ways in which organic chemistry reactions were categorized (Fig. 3), organized by two lower static levels and two upper process-oriented levels (Galloway et al., 2019). This work found that novices used many levels of interpretation but had a general tendency to categorize reactions by static features (i.e., Identical structural features and Similar properties of structures). In contrast, experts in organic chemistry categorized reactions primarily by process-oriented features (i.e., similar reaction type and similar mechanism) (Galloway et al., 2018).
A subsequent study used an online version of the card sort task to identify students’ interpretations of organic reactions with a larger sample size and measured changes over time (Lapierre and Flynn, 2020). That study found that students’ ability to sort according to the underlying mechanisms improved over time, moving to a more process-oriented interpretation of reactions. While this study demonstrated the utility of the categorization tool for evaluating the students’ choices and abilities separately, it did not probe how these varied measures of expertise are related, if at all. Additionally, no work was done to investigate the validity of these measures against other potential indicators of expertise or the reproducibility of the results.
Assessing the validity and reliability of an assessment
When evaluating an educational intervention, such as a curriculum, the quality of the assessment or instruments needs to be considered. Low-stakes assessments, such as the online categorization task, are frequently used to capture invaluable information for both practitioners and the university about the academic performance of learners (Wise and DeMars, 2005). Low-stakes assessments are assessments having no meaningful consequences to the students (e.g., low or no grade value), whereas a high-stakes possesses some meaningful consequences (e.g., medium to high grade value) (Cole and Osterlind, 2008).
There is a concern with low-stakes assessments that the lack of consequences leads to low student effort and may not provide an accurate reflection of what a student knows (Wise and DeMars, 2005). Analogously, the online categorization tasks are of low stakes, so low effort could be a limiting factor of the identified demonstrated expertise, thereby limiting the validity and reliability of the instrument. To make evidence-based decisions regarding the curriculum, the findings from the online categorization task need to be valid and reliable.
Validity is analogous to the accuracy of the instrument; various forms of validity evidence are used to demonstrate that the instrument is measuring the intended construct (Barbera and VandenPlas, 2011; Creswell, 2011; American Educational Research Association (AERA) et al., 2014) The validity of the instrument refers to the validity of the data collected by the instrument within a specific population, rather than the instrument itself. While an instrument may have proved valid in one context, that same instrument can produce different results in a new context and group. In previous work, the reaction cards used within the online categorization task had been evaluated for evidence based on test-content by a panel of experts in organic chemistry (Galloway et al., 2019). The physical and online categorization tasks were evaluated for response process validity through qualitative interviews. Other methods to establish validity include evidence based on the internal structure and relationship to other variables. When assessing validity based on relations to other scores, the intent is to relate the score of the instrument against similar (convergent validity) or dissimilar (divergent validity) tests to see if the relationship is positive or negative. This analysis is often performed and investigated but is seldom described as an aspect of validity (Arjoon et al., 2013).
In this work, we implemented categorization tasks to elicit students' organization of knowledge around organic chemistry reactions. To our knowledge, no work has attempted to assess the validity of the expertise demonstrated in a categorization task in organic chemistry against academic performance. The following work will look specifically at assessing the validity of expertise demonstrated in various categorization tasks in relation to other variables intended to measure the expertise of students, specifically academic performance on examinations.
Network analysis can be used to investigate students’ knowledge organization and its relation to academic performance (Acton et al., 1994). Quantitative measures to accompany network analysis can include coherence, path length correlation, and neighbourhood similarity. Neighbourhood similarities are a measure of the degree a concept has a similar neighbourhood between two networks (Neiles, 2014). Structural knowledge networks of individual instructors, individual non-instructor experts, average experts, and average “good” students have been investigated in comparison to novice networks as predictors of academic performance (Acton et al., 1994). This work demonstrated the utility of comparing expert networks to novices as a metric for predicting achievement on examinations and supports the potential relationship between the online categorization task and academic achievement.
Concept mapping is a commonly implemented tool for investigating and measuring a student's knowledge structure. Concept maps are graphical representations typically constructed by the participant, containing nodes and lines. In a concept map, these nodes represent a concept or important term, and lines represent a relationship between concepts. A more expert-like concept map is thought to be more interconnected than that of a novice, analogously to their knowledge structure. One of the key features of a concept map is the ability for it to be scored accurately and consistently (Ruiz-Primo et al., 1997), similarly to work described in Lapierre and Flynn (2020) with respect to analysing the online categorization task. Concept maps can be used to monitor and track changes in knowledge structure throughout the semester as the online categorization task was (Cook, 2017), but unlike the online categorization tasks require more training to prepare students to use the task.
The relationship between knowledge structure established through concept maps and academic performance determined by final grade in organic chemistry has been investigated (Szu et al., 2011). Two areas were strong predictors of the final grade: introduction to reactions and substitution and elimination reactions. While this work suggested the role of conceptual knowledge as a predictor of academic performance, the study suffered from a small sample size (N = 20) leaving little room for generalizability.
Reliability is analogous to the precision of the instrument and is attributed to the consistency of a measure. Reliability evidence aims to demonstrate that the scores of an instrument are not due to chance and are reproducible (Barbera and VandenPlas, 2011; Arjoon et al., 2013). Reliability of an instrument is often demonstrated through evidence based on replicate administrations or through internal consistency. When gathering evidence based on replicate administration, individuals from the same group will be administered the assessment twice. Following the repeated administration, the correlation coefficient between the two scores provides evidence of test-retest reliability (Association et al., 2014). While the correlation coefficient helps identify if students are achieving the same scores on tests, it provides no context at the item level. That limitation can be addressed using a chi-squared test (Brandriet and Bretz, 2014) and investigates the consistency of student responses at an item level during test–retest reliability. Transitioning these concepts to the present study's context, we aim to further improve on the validity of the online categorization task as an instrument for measuring expertise in organic chemistry. Additionally, we investigate the reproducibility of the findings from the instrument in a near-identical context.
Research questions
Using the research questions below, this study probes the relationship between various categorization tasks, compares categorization tasks with other indicators of expertise, and investigates the reproducibility of the findings.
RQ1: What relationship can be observed between categorization tasks in varied contexts (i.e., open vs. closed sort and low vs. high-stakes), if any?
RQ2: How do measures of expertise determined through a categorization task relate to academic performance?
RQ3: To what extent are findings from various categorization tasks stable and reproducible?
Methods
Instructional context and participants
This study was performed at a large research-intensive university in Canada in the context of an Organic Chemistry II course. In this institution, Organic Chemistry I (one semester) is offered in the students’ first year of studies and Organic Chemistry II (one semester) is offered in the students’ second year. The courses include both chemistry major and chemistry non-major students, with the Organic Chemistry I and Organic Chemistry II courses being a course requirement for many STEM related programs. Organic Chemistry II course was offered in a flipped format where students watch lectures online before the class (as short videos), with in-class time being dedicated to interactive learning activities. The Organic Chemistry II course was offered in the fall semester of the academic year (Flynn, 2015, 2017) and comprises a series of weekly pre-class tests and assignments, two midterms, and a final exam. Institutional research ethics board approval was received for secondary use of data. Students entering Organic Chemistry II had been previously taught the symbolism of organic chemistry (i.e., the electron-pushing formalism) and had been taught reactions governed by the following mechanistic patterns: acid–base reactions, π-electrophiles + nucleophiles, π nucleophiles + electrophiles, and aromatic nucleophiles + electrophiles (Fig. 2). All students enrolled in Organic Chemistry II were invited to participate in optional, consequence free, low-stakes online categorization tasks, a convenience sampling method (Cohen, 2010). Students who participated in a minimum of one of the two online categorization tasks (low-stakes) received a 1% bonus on a participation mark that accounted for 5% of their total grade.
Data collection
Throughout the Organic Chemistry II course, during the 2017 and 2018 fall terms, categorization tasks were incorporated using three components: online card-sort tasks, guided in-class activities, and high-stakes assessments—midterm and final exams (Fig. 4). Throughout the course, students were first asked to complete an online categorization task (low-stakes) delivered through the Optimal Sort software (Optimal Workshop, 2015). The online card-sort was administered twice in 2017 (early administration N = 65, late administration N = 43) and once in 2018 (N = 20). In the online categorization tasks, participants were asked to categorize a set of 25 organic chemistry reaction cards in both an open and closed format; the cards are the same as used in previous studies (Galloway et al., 2019); see Appendix I (ESI†). In the open sort, participants were prompted to (i) generate categories of their choosing, (ii) provide both a name for each category, and (iii) give an overall explanation for how they decided to make their categories. The open sort allows for insight into how the students’ chose to organize their knowledge, illustrating the connections between reactions cards that participants viewed as meaningful at that time. In the closed categorization task, participants were tasked with sorting the same 25 reaction cards into eight pre-determined categories aligned with, and presented as, the key mechanistic patterns in the Patterns of Mechanisms curriculum (Fig. 1), as well as an Other category. In doing so, the closed sort aimed to investigate students’ cued ability to identify the mechanistic patterns between reaction cards. Participants were asked to complete the open and the closed sort in sequential order; participants who completed the tasks in reverse order were excluded from the analysis. If participants completed a sort multiple times within a single administration, the final sort created was used.
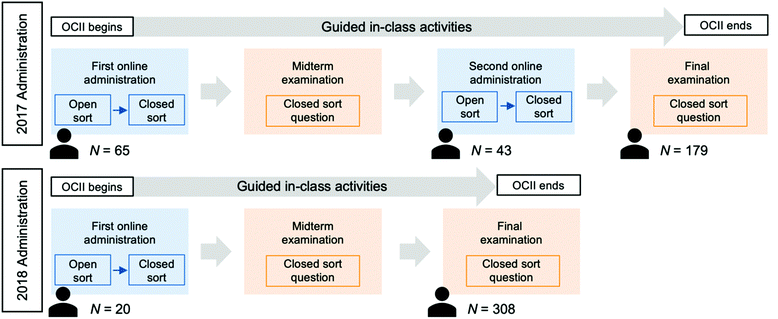 |
| Fig. 4 Study's timeline and key activities. | |
The two additional components asked students to complete a closed sort-like categorization task during guided in-class activities and major assessments. These tasks asked students to sort up to eight reaction cards into the pre-determined categories, again aligned with the curriculum's categories (Fig. 1). These reactions aimed to be of similar difficulty to reactions asked within the low-stakes task. While only the closed-sort tasks provided on the final examination are included in this study, the guided in-class activities were used to emphasize the importance of the underlying mechanistic patterns discussed throughout the curriculum and provide practice opportunities. All closed sort-like tasks required students to be knowledgeable on mechanistic patterns are previously seen in Organic Chemistry I as well those learned in Organic Chemistry II. The midterm and final examinations that include the closed sort-like task were given approximately two weeks after completing the low-stakes card sorts; these major assessments contributed 10–20% and 40–60% towards students’ final course grade, respectively. Students’ academic performance was captured as their overall grade on the major assessments, including the closed sort-like problem. The closed sort task was included in this measure as it comprised less than 5% of the overall assessment weight. All assessments included in the courses were designed by an expert in chemistry education to reflect the content of the Patterns of Mechanisms Curriculum, with a strong emphasis on student understanding and ability to reason through principles of reactivity.
Level of interpretation: quantifying the expertise demonstrated in how a participant chooses to sort
In the open sort, the names that participants provided as well as the explanations of the categories were used to assign one of four levels of interpretation (Fig. 3) using the previously established coding (Galloway et al., 2019; Lapierre and Flynn, 2020). These four levels of interpretations reflect increasing levels of expertise with respect to the features of a reaction that a participant identified as being meaningful. In addition to the levels of interpretations, groups with no similarity or unknown similarity observed by the participant were assigned the title of “unknown”. Participants who provided no chemical rationale or insufficient evidence to make a claim on the level of expertise demonstrated of their groups were assigned the title of “no data”. This “no data” group was observed in the following example: “I chose categories based on my skill level of how well I can do them.” Participants’ open sorts were initially coded by the first author and areas of concerns were addressed with the principal investigator until consensus was reached. Following this process, all data were recoded to encompass any changes in the coding scheme.
Once all participants’ groups were assigned a level of interpretation, the sorts were quantified for statistical analysis. To effectively capture the distribution of levels used by each participant, levels of interpretation were quantified per card. Each card was allocated a numeric value increasing from 1–4 based on the level of interpretations in the features attended to, where 1 was assigned if a card was at the most novice level of “Similar Structural Features” increasing to 4 if a card was at the highest level of “Similar Mechanism”. This scheme was applied to all reaction cards to give a total possible demonstrated level of interpretation out of 100 (4 points × 25 cards total). Participant groupings that contained no chemical rational (i.e., “no data” group) or were assigned to an “Unknown” category were given no weight towards their overall level, i.e., assigned a value of 0. This method aimed to mimic the scoring procedure used in concept mapping, but rather than capture accuracy and correctness, scores were an indication of the expertise captured through the level of interpretation used (Ruiz-Primo et al., 1997). Again, this method was designed to place weight on the way in which students organize their knowledge of reactions, not their specific content knowledge. For example, a participant could incorrectly identify a reaction, but still demonstrate a process-oriented level of interpretation.
Match with expert: quantifying a participant's ability to the mechanistic pattern governing a reaction
To quantify changes in a participant cued ability in the closed sort task, groups generated by participants were compared against an expert sort, to provide a “Match with expert” score (%). This expert sort designed by the primary author to be representative of the most mechanistically specific category presented within the transformed curriculum. This hypothesized sort was then validated with two experts in the field of organic chemistry (see complete expert sort in ESI†). Additionally, a second expert sort was developed for the high-stakes’ final examination categorization task (see complete expert sort in ESI†). This approach for quantifying the high-stakes task allowed for a similar comparison of a participant's ability to categorize reaction cards like an expert would, giving participants a “Match with Expert” Score out of 6. A “Match with Expert” score of six indicates that participants correctly categorized all reaction cards included in the problem, including the reactions in part A of the task that are assigned by participants (four reactions) and those in part B that required participants to correctly identify the two reaction cards belonging to a category 7 – activated π nucleophiles + electrophiles. These participant specific scores allowed for us to readily make comparisons against the level of interpretation score determined from the open sort, and other metrics such as academic achievement.
Data analysis
Characterizing the relationships between multiple categorization tasks (RQ1).
To investigate the relationship between the expertise demonstrated in the online categorization tasks (i.e., level of interpretation and match with expert sort), as well as demonstrated in closed-sort like task (match with expert sort) in a higher stake setting, a correlation analysis was performed using SPSS (IBM SPSS Statistics, 2019). Pearson's R was implemented in all correlation analysis, the strength of the correlation (r value) are qualitatively described as small = 0.1, medium = 0.3, and large = 0.5 (Cohen, 1988). Two assumptions must be met to calculate Pearson's R: linearity and normality. Since the samples here are considered large (N > 30) it can be assumed that they are normally distributed due to the central limit theorem (Lumley et al., 2002; Field, 2013). In addition to the correlation analysis, trends in how participants sorted similar reaction cards were investigated between low-stakes, online closed sort, and higher stakes major assessment closed sort-like problems. Participants included in this section of this analysis were those who had completed the late administration of the online categorization task (N = 43), and the final examination closed sort (N = 179). Each card in Question 1 of the high-stake categorization task possessed a matched card in the low-stakes online task. Reaction cards between the two categorization tasks were matched based on similarities in the underlying mechanistic patterns. Trends were first visually inspected, followed by the quantitative investigation using a chi-squared test of independence to identify any differences in frequency of use between the pre-determined mechanistic categories (Franke et al., 2012). By evaluating the relationship between the low-stakes online categorization task and high-stakes major assessment tasks, we aimed to increase the validity of findings and support its ability to assess a participant's expertise without fear of losing efficacy due to lack of motivation as common in low-stakes assessments.
Evaluating the relationship to academic performance (RQ2) and reproducibility (RQ3).
All measures of expertise were investigated in relation to the participants' academic performance on major assessments throughout the course. In the early (N = 65) and late (N = 43) administration, the relationship between participants’ level of interpretation score (/100) and match with expert (%) were correlated against major assessment scores, including the first midterm and final examination score, respectively. This correlation was calculated in order to investigate the link between the expertise observed in each sort with respect to the external variable of the students’ overall expertise in the courses in a concurrent fashion (i.e., at approximately the same time) or a predictive fashion. Additionally, the relationship between the scores on sorting questions and assessment score (N = 179) was investigated. Throughout this investigation, we acknowledge that while students’ performance on a major assessment is not an omnibus assessment of expertise, the ability to use a simple tool such as a categorization task to anticipate how a student may perform can provide students and educators with important information.
To ensure the reliability and reproducibility of the previously established findings, both the early administration of online categorization task and the final examination question were implemented in Organic Chemistry II of the following year. Students in this reproduction group were in a near-identical context to those of the main group i.e., used the same curriculum, flipped course organization, and course instructor. Like the original group, students in the reproduction group were given explicit instructions about the mechanistic patterns and a final examination that possessed a similar structure, and many identical problems. Due to the similarity of the final examination, participants in the reproduction group who completed the online categorization task (N = 20) were subsequently matched with a participant from the main study according to standardized z-scores from the final examination, with an average difference in z-scores of 0.026. While this method of matching does not allow for us to capture the changes that participants might have incurred in expertise from the early to late time points in the course, investigating the similarities on the examination act as the most objective measure of expertise available. The online categorization tasks and final examination categorization task were quantified using the aforementioned procedures. Using the two population samples, we were able to determine the reproducibility of the findings with respect to the expertise demonstrated in choice and ability. Spearman's rho (rs) was used as a correlation coefficient here as opposed to Pearson's R because the samples were small and not normally distributed. Additionally, the stability of results relating to the final examination categorization task of the main group (N = 179) and reproduction group (N = 308) were compared using a chi-squared analysis to compare the use frequency of mechanistic categories used. Lastly, the matched sample was used to illustrate the test–retest reliability of the categorization task for probing expertise related to how participants chose to sort and their ability when cued to do so.
Results and discussion
RQ1. What relationship, if any, can be observed between categorization tasks in varied contexts?
The following aims to investigate the relationships between categorization tasks in varied context, including the relationship between choice and ability, as well as ability in various stakes, in two ways: (I) broadly with a correlation analysis exploring the relationships and using (II) an item-based approach to inspecting trends between different categorization contexts.
(i) Investigating the relationship between multiple categorization tasks.
We investigated the relationship between the open and the closed sort to establish the relationship between how students may choose to organize their knowledge (via the open sort) and their ability to apply this knowledge at the highest level of interpretation (via the closed sort). We hypothesized that participants who sorted by features requiring higher expertise (open sort) would also be able to identify the underlying mechanistic patterns (closed sort). We observed a small, significant correlation (N = 65, r = 0.28, p = 0.026) between how learners chose to organize their knowledge (i.e., level of interpretation, /100) and their cued ability (i.e., match with expert sort, %) at the beginning of the Organic Chemistry II course (Fig. 5). This small correlation provides weak evidence to the relationship between the two online sorts at the early administration. At the late administration, the correlation was large and significant between participants’ demonstrated choice and cued ability (N = 43, r = 0.53, p = 0.000) (Fig. 5). Together, these findings suggest an evolving relationship between the multiple facets of learners’ expertise. The way participants organized the reactions in the open sort did not immediately connect with an ability to recognize the mechanistic patterns in the closed sort. While the content in Organic I was organized by the governing mechanism, the course did not necessarily teach students explicitly how to recognize the patterns. This lack of explicit instruction may be a factor contributing to the reason that the students were less able to identify mechanistic patterns when cued during the task. As students progressed through the curriculum, students demonstrated more expertise with respect to how they chose to sort and were more likely to recognize patterns in the underlying mechanisms of reactions.
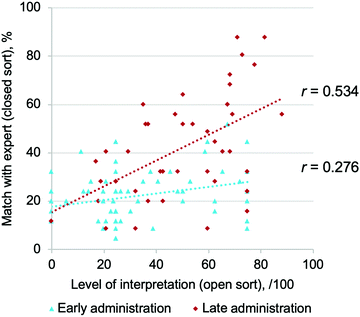 |
| Fig. 5 Relationship between how participants chose to sort (Level of interpretation, open sort) and their ability to sort according to the deep features (match with expert, closed sort) at both early (N = 65) and late (N = 43) administration. | |
We investigated the relationship between participants’ cued ability in various stakes, specifically the low-stakes online categorization tasks and the high-stakes major assessment categorization task (exam question with eight reactions to categorize) at the late administration. We found a small, non-significant correlation (N = 43, r = −0.29, p = 0.061) (Fig. 6). The lack of relationship between cued ability in tasks with varied stakes may be a result of changes in participants’ ability between the two-time points. Alternatively, this result may be an indicator that the smaller eight card instrument is not able to capture the high levels of diversity in participants’ abilities compared to the larger, 25 card instrument. While card sorting tasks are unavoidably variant from administration to administration (Harper et al., 2003), this lack of continuity between the tasks of varied stakes requires further investigation. Specifically, one could investigate the factors that influenced the change in participants’ measured expertise and how can we verify that participants who could categorize by the deep underlying features in the low-stakes setting were learning the concepts meaningfully. To further investigate the relationship between the varied contexts, an item-based approach was used to examine trends between specific reaction cards (Fig. 7).
 |
| Fig. 6 Relationship between cued ability in a high-stakes final examination question and low-stakes online categorization task (closed sorts) (N = 43). | |
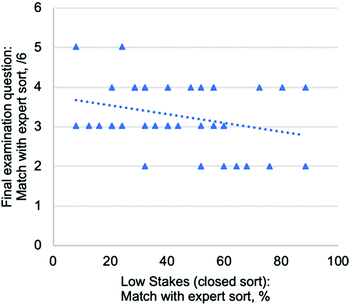 |
| Fig. 7 Major trends observed between participant responses of a high and low stakes categorization task. Star in the figure denotes categories with significant differences, p < 0.05 based on chi squared test. Categories possessing <5% distribution, and “other” category from the low stakes sort were omitted from the figure for simplicity. | |
(ii) An item-based approach to inspect trends between different categorization contexts.
We identified several key similarities when we compared the sorting decisions for the four reaction cards in the closed sort question on the final exam (cards 1–4) and their associated cards in the online categorization task (cards A, E, F, and X), (Fig. 7). Reaction pairs F/1 and X/4 both follow the mechanistic pattern of a π electrophile with a leaving group reacting with a nucleophile (leaving groups are initially “hidden” in the cards X and 4). We observed similarities in how participants sorted the paired reaction cards F/1 and X/4, demonstrating no significant differences between the categories that participants chose to use. In contrast, the reaction pairs of A/2 and E/3 demonstrated a significant difference in the distribution of the primary category used between the two different categorization tasks. Although, there was a significant difference in both categorization tasks of ∼20%, the shift demonstrated an increased likelihood in the high-stakes to sort correct. From these two sets (A/2 and E/3), we additionally observed similarities in the common errors that were made during categorization. For example, in the reaction pair of A/2, ∼20% of participants in each categorization task identified the reaction as an aromatic nucleophile reacting with an electrophile although no reactants have an aromatic ring. From a chemistry learning perspective, areas of concern include students’ ability to identify aromatic rings (in cards A/2) and the inability to recognize a hidden leaving group as (in cards X/4). The similarities between the two sorts help demonstrate the reproducibility of the results, the generalizability of the low-stakes assessment, and provide greater evidence that the trends observed may not be limited by the lack of consequences (i.e., the low stakes).
RQ2. How do measures of expertise determined through a categorization task relate to academic performance?
Through the comparison of the various measures of expertise determined through the categorization tasks, we aimed to evaluate the criterion validity of these measures against a more tangible measure of expertise for students, major assessment grades. These measures of expertise were first compared to assessments given at approximately the same time point (i.e., within two weeks). This approach aimed to capture the concurrent relationship between the measures of expertise capture on the low-stakes categorization tasks and an external but related measure. With respect to the measure of how a participant chooses to sort (i.e., level of interpretation), we observed a small correlation at the early administration (N = 65, r = 0.28, p = 0.023) and a medium correlation at the late administration (N = 43, r = 0.38, p = 0.012), Fig. 8. With respect to the measure of a participant's ability, we observed a large correlation at the early administration (N = 65, r = 0.53, p = 0.000) and an even larger correlation at the final administration (N = 43, r = 0.70, p = 0.000), Fig. 8.
 |
| Fig. 8 Concurrent relationship between major assessment score and (a) level of interpretation in the online open sort and (b) match with expert sort in the online closed sort at both the early administration (N = 65) and late administration (N = 43). | |
Understanding how a participant's choice and ability to categorize reactions are related to academic performance on the major assessment allows us to understand which tools provide a stronger insight in students’ expertise. The open sort offers a unique insight into the connections that participants naturally view as meaningful and allow for educators to observe any potential errors or weaknesses in the connections students are naturally making, such as a tendency to sort by solvent (Lapierre and Flynn, 2020). The expertise demonstrated in the open sort lacked a strong relationship with academic performance, which may be due to the high variability in how participants chose to sort acting as a limiting factor. The closed sort tasks provided a consistent and strongly correlated measure with students’ academic performance and may be a more accessible instrument for instructors to assess expertise in the course. In comparison to the open sort, the closed sort is a quicker and easier instrument for instructors to use as it does not require interpretation of qualitative data. While previous research had demonstrated the closed sort's ability to cue novices to sort by deeper features (Lapierre and Flynn, 2020), this finding establishes the ability to use these tools to measure academic performance in a concurrent fashion, with relevancy for instructors who wish to identify potentially low achieving students (or for students to self-assess their ability) before the major assessment, who may benefit from additional support. This result parallels findings from other card-sort task studies done in general chemistry (Irby et al., 2016; Krieter et al., 2016), biology (Smith et al., 2013), and computer science (McCauley et al., 2005), all of which found that discipline specific card-sort tasks were capable of differentiating students’ levels of expertise in the given subject. However, no other card-sort instruments to date have shown such a strong correlation with exam results.
In addition to assessing the concurrent alignment of the open and closed online categorization task with students’ academic performance, the viability of the online categorization task to predict future academic performance was investigated. Students who participated in the early administration demonstrated a moderate correlation between how they chose to sort (i.e., level of interpretation, open sort) and final examination grade (N = 65, r = 0.36, p = 0.003) and a larger correlation between their demonstrated ability (i.e., match with expert, closed sort) and final examination grade (N = 65, r = 0.48, p = 0.000) (Fig. 9). These findings demonstrate the predictive capacity of both the open and closed categorization tasks for detecting academic performance early in the course. The early administration of the open sort had a stronger correlation to the final major assessment than to the early major assessment.
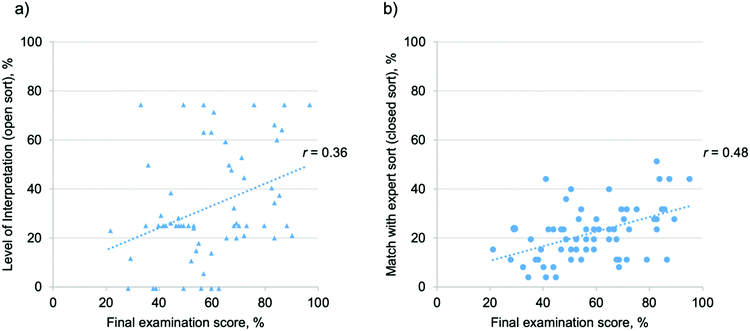 |
| Fig. 9 Predictive relationship between major assessment score and (a) level of interpretation in the online open sort and (b) match with expert sort in the online closed sort at both the early administration (N = 65). | |
This finding may be attributed to the variable nature of the expertise an individual may choose to use in the open sort. Alternatively, this can be an indicator of the varied expertise that was required and measured by the major assessment; meaning the final examination may have had a stronger alignment with the expertise captured in the open sort's levels of interpretation than the midterm examination. Regardless, this finding aids in establishing the validity of the assessment for the overall expertise of an individual, and further promotes the utility of the closed sort for predicting academic performance and potentially low achieving students (Lapierre and Flynn, 2020) who may benefit from additional support. We observed no significant correlation between the expertise demonstrated on the categorization task of the final examination and the overall assessment grade. This finding can indicate multiple possibilities. First, it can indicate there was an issue with the examination categorization questions, in which the questions may have been beyond the scope of knowledge expected of OCII students (unlikely as these were reactions that had been directly taught in the course); alternatively, this result could indicate changes in expertise that may have occurred between the final examination and the online closed sort. This latter idea is supported by the previously discussed lack of relationship between the measure from the online closed sort and final examination closed sort. The inconsistency of the relationship of the closed sort categorization tasks on exams with the indicators of expertise such as academic achievement is troubling for the transferability of the skill, which we believe we are assessing with the online instrument.
RQ3. To what extent are findings from various categorization tasks stable and reproducible?
To ensure the reliability and reproducibility of the previously mentioned findings, the online categorization task was administered at the beginning of the subsequent years OCII course, plus the final examination included a closed sort question. Through the term, the explicit use of mechanistic patterns was incorporated into the curriculum to encourage mechanistic thinking. In doing this, we investigated the stability of the levels used from year to year (e.g., is the reliance on surface features consistent at the beginning of the course), the stability of any trends determined within the final examination problem, as well as the stability of the predictive power.
To draw comparisons between a year to year, we first aim to look broadly at the overall measures of expertise used at the beginning of the year, both demonstrated level of interpretation and demonstrated ability (Fig. 10). In the open categorization tasks, there were no significant differences observed between students entering OCII in 2017 (Mdn2017= 25) and 2018 (Mdn2018 = 35), U = 758, z = 1.13, p = 0.259, r = 0.12 (a Mann–Whitney U test was used for this comparison as the 2018 group was a small, non-normal sample). As students entered OCII, they primarily used static levels of interpretation. In the closed categorization, we again observed a non-statistically significant difference from year to year between students entering OCII in 2017 (N = 65, M2017 = 22 SD2017 = 11%, and 2018 (M2018 = 23% SD2018 = 11%), (F(1, 32) = 0.045, p = 0.834. Through an independent t-test, with Welch's correction to account for unbalanced samples, we determined that students entering OCII showed consistency in the measure of expertise with the categorization task. This additionally supports the reproducibility of previous findings from Lapierre and Flynn (2020), improving the generalizability within the context of the curriculum.
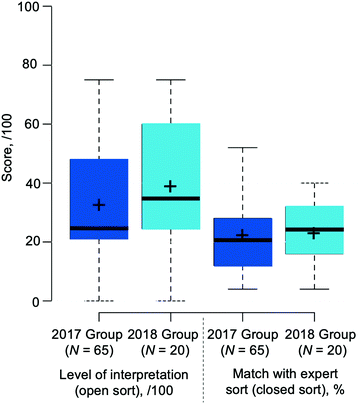 |
| Fig. 10 Reproducibility of distribution of data from the online categorization task. Median values are represented by a bold line; mean values are indicated by plus symbols (+). | |
To further probe the stability of the categorization task, participants from the reproduction group were given a matched pair from the main sample using corrected z-scores of final examination grades (Fig. 11). With respect to the open sort and how a participant chooses to see connections, no significant relationship was observed between the expertise demonstrated in the open sort between the paired participants (Fig. 12) (N = 20, rs = −0.14, 95% BCa CI [−0.523, 0.283], p = 0.544). This lack of continuity when paired on academic performance supports the high level of variability found at the early administration in how participants chose to sort. In contrast, the relationship between the ability of participants demonstrated between the two groups (closed sorts) was highly correlated (N = 20, rs = 0.62, 95% Bca [0.190, 0.916], p = 0.004). These findings emphasize the stability of the card sort task for measuring students’ cued ability to identify deep features, as well as emphasis again the relationship between the students’ measured ability and academic performance.
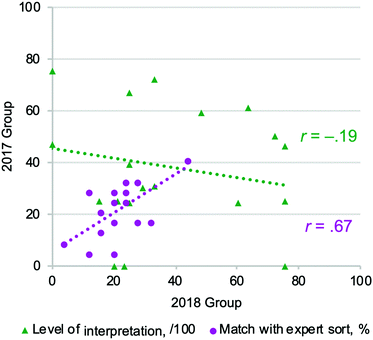 |
| Fig. 11 Test–retest reliability of 2017 and 2018 groups, using pairs of students, matched through final examination grade Z-scores. | |
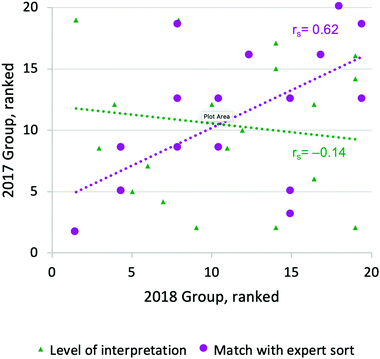 |
| Fig. 12 Test–retest reliability of 2017 and 2018 groups, using pairs matched through final examination. Data are of ranked percentages. | |
Lastly, we investigated the stability of any trends determined in the final examination using an item-based analysis. We use a similar method to the one described above (Fig. 7), this time comparing the same item from year to year (Fig. 12). Each item elicited the same trend from one administration to another, again demonstrating the stability of the examination categorization task, with no significant difference (p > 0.05) observed between the main 2017 group and the 2018 group. These findings also demonstrate the stability of trends previously discussed, i.e., students incorrectly categorizing reaction card B as an aromatic nucleophile and students struggling to see beyond the surface features to the deep underlying mechanism as seen in reaction cards A and D.
Limitations
The goal of this study was to investigate the relationship between how students categorize organic chemistry reactions in a transformed curriculum, using this relationship to aid in the validity and reliability of these online instruments. While strong evidence of a relationship between the online closed categorization task at the end of the term and final examination performance was observed, we are unable to determine if achieving a high score is directly related with a student understanding these underlying mechanistic patterns and principles of reactivity.
While the transition of the categorization task to the online platform possessed several key advantages, it consequently brought new limitations to the instrument related to how participants engaged with the tool. Participants were found to be limited by their prior knowledge of symbolism in organic chemistry found in the closed sort, such as LG to represent leaving group (Lapierre and Flynn, 2020). While in an interview setting, the researcher would be able to note if this issue arises, the remote nature of the online task prevents us from understanding how knowledge of organic symbolism impacts how individual students are sorting. While we would hypothesize that students, who possess a more static or novice-like organization of knowledge, would be the students who are limited by the symbolism, we are unable to verify this hypothesis using the online sort. Lastly, while the remote nature aimed to promote a natural response from the participants, we are unable to monitor if students followed the instructions of working independently without outside resources. While similarities within sorts were found at the surface, no two participants were found to possess identical reasoning for sorts. Using multiple administrations, we aimed to enhance the reproducibility, and generalizability of the findings, but due to the small sample size in the 2018 administration we are unable to see the full breadth of patterns which may have emerged from the new sample and can only provide a limited context of students within the patterns of mechanism curriculum. Finally, the sampled student population came from only a single institution that teaches the Patterns of Mechanisms curriculum. Due to this limited sample, the findings from this study cannot be applied outside of the current context (Fig. 13).
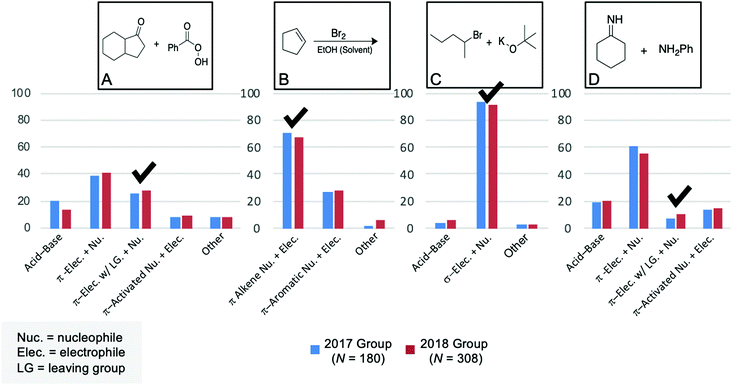 |
| Fig. 13 Stability of trends observed in a final examination categorization question. A checkmark in the figure denotes correct mechanistic category. No significant differences, p < 0.05 based on chi-squared analysis. | |
Conclusions
Findings from this work increase the validity of the online categorization tasks, illustrating a strong relationship between the expertise demonstrated in the online categorization and academic performance in Organic Chemistry II. By using the low-stakes online categorization task in conjunction with high-stakes categorization problems, and examining academic performance, we aimed to capture multiple measurements of a students’ expertise in organic chemistry. A correlation analysis of the relationship between the participants’ level of interpretation (/100) and match with expert (%) provide a clearer understanding of the relationship between the connections that students chose to identify and their ability to categorize reactions in a more expert-like fashion (i.e., by mechanism). These findings demonstrated a growing relationship between choice and ability as they developed their expertise in the domain.
To predict academic performance, the closed sort had strong correlations with upcoming major assessments, while the open sort had small to medium correlations with major assessments. Additionally, the strong correlation with the academic performance on major assessments—i.e., a metric of students’ expertise in organic chemistry—not only increases the validity of the findings but suggests the closed sort may be a powerful educational resource for instructors and students alike.
The findings from the categorization tasks were shown to be reproducible broadly by investigating the levels of expertise used in the online categorization from year to year, showing no difference in the mean values used and a strong correlation in the ability demonstrated by participants matched on final exam performance (rs = 0.62). To date, no other card-sort tasks have shown such a strong correlation with academic achievement in a given subject. Analysis from an item-based perspective illustrated reproducible trends in the data with no significant difference from year to year. Collectively, these findings aid to the reliability of the low-stakes online categorization task for consistently assessing how different samples of students in the redesigned “Mechanistic pattern and principles” curriculum are interpreting these reactions.
Implications for teaching and learning
Findings from this work aid in highlighting the specific role of understanding both how a participant chooses to sort and their cued ability, providing instructors in organic chemistry courses with a simple instrument to evaluate the changes of levels of expertise as students progress throughout their curriculum. The closed sort was an effective formative assessment for students or educators to gauge students’ progress before major assessments. Educators and students could use the feedback to tailor their efforts. While the high-stakes categorization tasks were found to have no relationship with academic success, their implementation remains essential to demonstrate the value of being able to analyse these deep underlying features throughout the course. More research needs to be done on the relation between closed-sort tasks and academic achievement before we can recommend the use of these tasks in high-stakes assessments. Categorization tasks can be designed in a number of different ways, as we have previously reported (Galloway et al., 2018).
Implications for research
The current study explored the relationship between the open and closed sort, as well as the varied stakes within a limited context. The limited context of this work illustrates the need for a multi-institution design of the online categorization task. While the utility of these online categorization tasks was found to be effective in a curriculum that emphasizes these underlying patterns, the current study design provided no evidence of how students in the traditional curriculum would perform and whether these relationships would remain as strong. The high-stakes categorization tasks were found to have no relationship with academic success but trends within the high-stakes were found to be consistent. Further work is required to explore to how these high-stakes categorizations differ from the low-stakes, and to identify to what degree their inclusion throughout the course works as a scaffold for emphasizing these deep underlying features throughout the course. The next stage of this research is to extend the closed sort task into different contexts at different institutions. The findings will provide important information about the generalizability of the results, including for curricula that use a functional group approach.
Conflicts of interest
There are no conflicts to declare.
Acknowledgements
We thank group members for valuable discussions.
References
- Acton W. H., Johnson P. J. and Goldsmith T. E. (1994), Structural knowledge assessment: Comparison of referent structures, J. Educ. Psychol., 86(2), 303–311 DOI:10.1037/0022-0663.86.2.303.
- American Educational Research Association (AERA), American Psychological Association (APA) and National Council on Measurement in Education (NCME), (2014), The Standards for Educational and Psychological Testing, American Educational Research Association.
- Anderson T. L. and Bodner G. M., (2008), What can we do about ‘Parker’? A case study of a good student who didn’t ‘get’ organic chemistry, Chem. Educ.: Res. Pract., 9(2), 93–101.
- Anzovino M. E. and Bretz S. L., (2015), Organic chemistry students’ ideas about nucleophiles and electrophiles: The role of charges and mechanisms, Chem. Educ.: Res. Pract., 16(4), 797–810.
- Arjoon J. A., Xu X. and Lewis J. E., (2013), Understanding the state of the art for measurement in chemistry education research: Examining the psychometric evidence. J. Chem. Educ., 90(5), 536–545 DOI:10.1021/ed3002013.
- Association, A. E. R., Association. A. P. and Education. N. C. on M. in and (U.S.), J. C. on S. for E. and P. T., (2014), Standards for educational and psychological testing.
- Barbera J. and VandenPlas J. R., (2011), All assessment materials are not created equal: The myths about instrument development, validity, and reliability, in ACS Symposium Series, vol. 1074, American Chemical Society, pp. 177–193 DOI:10.1021/bk-2011-1074.ch011.
- Bhattacharyya G. and Bodner G. M., (2005), “It gets me to the product”: How students propose organic mechanisms, J. Chem. Educ., 82(9), 1402–1407.
- Bissonnette S. A., Combs E. D., Nagami P. H., Byers V., Fernandez J., Le D., Realin J., Woodham S., Smith J. I. and Tanner K. D., (2017), Using the biology card sorting task to measure changes in conceptual expertise during postsecondary biology education, CBE Life Sci. Educ., 16(1), 1–15 DOI:10.1187/cbe.16-09-0273.
- Bodé N. E. and Flynn A. B., (2016), Strategies of successful synthesis solutions: Mapping, mechanisms, and more, J. Chem. Educ., 93(4), 593–604 DOI:10.1021/acs.jchemed.5b00900.
- Bradley A. Z., Ulrich S. M., Jones M. and Jones S. M., (2002), Teaching the sophomore organic course without a lecture. Are you crazy? J. Chem. Educ., 79(4), 514 DOI:10.1021/ed079p514.
- Brandriet A. R. and Bretz S. L., (2014), The development of the redox concept inventory as a measure of students’ symbolic and particulate Redox understandings and confidence, J. Chem. Educ., 91(8), 1132–1144 DOI:10.1021/ed500051n.
- Bransford J. D., Brown A. L. and Cocking R. R. (ed.), (2000), How people learn: brain; mind; experience; school, in How People Learn: Brain; Mind; Experience; and School: Expanded Edition, National Academies Press.
- Bransford J. D, Brown A. L. and Cocking R. R. (ed.), (2010), How People Learn: Brain; Mind; Experience; School. In How People Learn: Brain; Mind; Experience; and School: Expanded Edition, National Academies Press DOI:10.17226/9853.
- Chi M. T. H., (2006), Two Approaches to the Study of Experts’ Characteristics, in The Cambridge Handbook of Expertise and Expert Performance, Cambridge University Press, pp. 21–30 DOI:10.1017/CBO9780511816796.002.
- Chi M. T. H., Feltovich P. J. and Glaser R., (1981), Categorization and representation of physics problems by experts and novices, Cogn. Sci., 5(2), 121–152 DOI:10.1207/s15516709cog0502_2.
- Chi M. T. H., Glaser R. and Farr M. J. (ed.), (2014), The Nature of Expertise, Psychology Press.
- Cobb P., Confrey J., DiSessa A., Lehrer R. and Schauble L., (2003), Design experiments in educational research, Educ. Res., 32(1), 9–13 DOI:10.3102/0013189X032001009.
- Cohen J., (1988), Set correlation and contingency tables, Appl. Psychol. Meas., 12(4), 425–434.
- Cohen L., (2010), Research methods in education, in Research Methods in Education DOI:10.4324/9780203224342.
- Cole J. S. and Osterlind S. J., (2008), Investigating differences between low- and high-stakes test performance on a general education exam, J. Gen. Educ., 57(2), 119–130 DOI:10.1353/jge.0.0018.
- Cook L. J., (2017), Using Concept Maps to Monitor Knowledge Structure Changes in a Science Classroom, ProQuest LLC.
- Creswell J., (2011), Educational Research: Planning, Conducting, and Evaluating Quantitative and Qualitative Research (Fourth), Pearson Education.
- Cruz-Ramírez De Arellano D., Towns M. H., Cruz D. and Towns M. H., (2014), Students’ understanding of alkyl halide reactions in undergraduate organic chemistry, Chem. Educ. Res. Pract., 15(4), 501–515 10.1039/c3rp00089c.
- Domin D. S., Al-Masum M. and Mensah J., (2008), Students’ categorizations of organic compounds, Chem. Educ. Res. Pract., 9(2), 114–121 10.1039/b806226a.
- Field A. P., (2013), Discovering statistics using IBM SPSS statistics (4th edition), Sage.
- Flynn A. B., (2015), Structure and evaluation of flipped chemistry courses: Organic & spectroscopy, large and small, first to third year, English And French, Chem. Educ.: Res. Pract., 16, 198–211.
- Flynn A. B., (2017), Flipped chemistry courses: Structure, aligning learning outcomes, and evaluation, in Online Approaches to Chemical Education, American Chemical Society, pp. 151–164 DOI:10.1021/bk-2017-1261.ch012.
- Flynn A. B. and Featherstone R. B., (2017), Language of mechanisms: exam analysis reveals students’ strengths, strategies, and errors when using the electron-pushing formalism (curved arrows) in new reactions. Chem. Educ.: Res. Pract., 18(1), 64–77 10.1039/C6RP00126B.
- Flynn A. B. and Ogilvie W. W., (2015), Mechanisms before reactions: A mechanistic approach to the organic chemistry curriculum based on patterns of electron flow, J. Chem. Educ., 92(5), 803–810 DOI:10.1021/ed500284d.
- Franke T. M., Ho T. and Christie C. A., (2012), The chi-square test: Often used and more often misinterpreted, Am. J. Eval., 33(3), 448–458 DOI:10.1177/1098214011426594.
- Galloway K. R., Stoyanovich C. and Flynn A. B., (2017), Students’ interpretations of mechanistic language in organic chemistry before learning reactions, Chem. Educ. Res. Pract., 18(2), 353–374 10.1039/C6RP00231E.
- Galloway K. R., Leung M. W. and Flynn A. B., (2018), A comparison of how undergraduates, graduate students, and professors organize organic chemistry reactions, J. Chem. Educ., 95(3), 355–365 DOI:10.1021/acs.jchemed.7b00743.
- Galloway K. R., Leung M. W. and Flynn A. B., (2019), Patterns of Reactions: A card sort task to investigate students’ organization of organic chemistry reactions, Chem. Educ. Res. Pract., 20(1), 30–52 10.1039/C8RP00120K.
- Graulich N. and Bhattacharyya G., (2017),
Investigating students’ similarity judgments in organic chemistry, Chem. Educ. Res. Pract., 18(4), 774–784 10.1039/c7rp00055c.
- Grove N. P. and Bretz S. L., (2012), A continuum of learning: From rote memorization to meaningful learning in organic chemistry, Chem. Educ.: Res. Pract., 13(3), 201–208.
- Harper M. E., Jentsch F. G., Berry D., Lau H. C., Bowers C. and Salas E., (2003), TPL—KATS-card sort: A tool for assessing structural knowledge, Behav. Res. Meth., Instrum., Comput., 35(4), 577–584 DOI:10.3758/BF03195536.
- IBM SPSS Statistics (Version 25), (2019).
- Irby S. M., Phu A. L., Borda E. J., Haskell T. R., Steed N. and Meyer Z., (2016), Use of a card sort task to assess students’ ability to coordinate three levels of representation in chemistry, Chem. Educ. Res. Pract., 17(2), 337–352 10.1039/C5RP00150A.
- Krieter F. E., Julius R. W., Tanner K. D., Bush S. D. and Scott G. E., (2016), Thinking like a chemist: Development of a chemistry card-sorting task to probe conceptual expertise, J. Chem. Educ., 93(5), 811–820 DOI:10.1021/acs.jchemed.5b00992.
- Lapierre K. R. and Flynn A. B., (2020), An online categorization task to investigate changes in students’ interpretations of organic chemistry reactions, J. Res. Sci. Teach., 57(1), 87–111 DOI:10.1002/tea.21586.
- Lin S. Y. and Singh C., (2010), Categorization of quantum mechanics problems by professors and students, Eur. J. Phys., 31(1), 57–68 DOI:10.1088/0143-0807/31/1/006.
- Lumley T., Diehr P., Emerson S. and Chen L., (2002), The importance of the normality assumption in large public health data sets. Annu. Rev. Public Health, 23, 151–169 DOI:10.1146/annurev.publhealth.23.100901.140546.
- Mason A. and Singh C., (2011), Assessing expertise in introductory physics using categorization task, Phys. Rev. ST – Phys. Educ. Res., 7(2), 1–17 DOI:10.1103/PhysRevSTPER.7.020110.
- McCauley R., Murphy L., Westbrook S., Haller S., Zander C., Fossum T., Sanders K., Morrison B., Richards B. and Anderson R., (2005), What do successful computer science students know? An integrative analysis using card sort measures and content analysis to evaluate graduating students’ knowledge of programming concepts, Expert Syst., 22(3), 147–159 DOI:10.1111/j.1468-0394.2005.00306.x.
- National Research Council, (2012), A Framework for K-12 Science Education.
- Neiles K. Y., (2014), Measuring knowledge: Tools to measure students’ mental organization of chemistry information, in Bunce D. M. and Cole R. S. (ed.), Tools of Chemistry Education Research, American Chemical Society, pp. 169–189 DOI:10.1021/bk-2014-1166.ch010.
- Optimal Workshop, (2015), OptimalSort Online Card Sorting Software.
- Raycroft M. A. R. and Flynn A. B., (2019), What works? What's missing? An evaluation model for science curricula with five lenses of learning outcomes, Submitted.
- Ruiz-Primo M. A., Shavelson R. J. and Shultz S. E., (1997), On the validity of concept map-base assessment interpretations: An experiment testing the assumption of hierarchical concept maps in science, CSE Technical Report 455, 6511(310) DOI:10.1017/CBO9781107415324.004.
- Smith M. U., (1992), Expertise and the organization of knowledge: Unexpected differences among genetic counselors, faculty, and students on problem categorization tasks, J. Res. Sci. Teach., 29(2), 179–205 DOI:10.1002/tea.3660290207.
- Smith J. I., Combs E. D., Nagami P. H., Alto V. M., Goh H. G., Gourdet M. A. A., Hough C. M., Nickell A. E., Peer A. G., Coley J. D. and Tanner K. D., (2013), Development of the biology card sorting task to measure conceptual expertise in biology, CBE—Life Sci. Educ., 12(4), 628–644 DOI:10.1187/cbe.13-05-0096.
- Spencer D., (2009), Card sorting: Designing usable categories, in Card Sorting: Designing Usable Categories, Rosenfeld Media.
- Strickland A. M., Kraft A. and Bhattacharyya G., (2010), What happens when representations fail to represent? Graduate students’ mental models of organic chemistry diagrams, Chem. Educ.: Res. Pract., 11, 293–301.
- Szu E., Nandagopal K., Shavelson R. J., Lopez E. J., Penn J. H., Scharberg M. and Hill G. W., (2011), Understanding academic performance in organic chemistry, J. Chem. Educ., 88(9), 1238–1242 DOI:10.1021/ed900067m.
- Webber D. M. and Flynn A. B., (2018), How are students solving familiar and unfamiliar organic chemistry mechanism questions in a new curriculum? J. Chem. Educ., 98(9), 1451–1467 DOI:10.1021/acs.jchemed.8b00158.
- Wise S. L. and DeMars C. E., (2005), Low examinee effort in low- stakes assessment: Problems and potential solutions, Educ. Assess., 10(1), 1–17 DOI:10.1207/s15326977ea1001.
Footnote |
† Electronic Supplementary Information (ESI) available. See DOI: 10.1039/d1rp00267h |
|
This journal is © The Royal Society of Chemistry 2022 |