DOI:
10.1039/D2RA06125B
(Paper)
RSC Adv., 2022,
12, 35151-35157
Discriminating sensing of explosive molecules using graphene–boron nitride–graphene heteronanosheets†
Received
28th September 2022
, Accepted 16th November 2022
First published on 7th December 2022
Abstract
Since the synthesis of graphene–boron nitride heterostructures, their interesting electronic properties have attracted huge attention for real-world nanodevice applications. In this work, we combined density functional theory (DFT) with a Green's function approach to examine the potential of graphene–boron nitride–graphene heteronanosheets (h-NSHs) for discriminating single molecule sensing. Our result demonstrates that the graphene–boron nitride–graphene (h-NSHs) can be used for discriminate sensing of the 2,4-dinitrotoluene (DNT), octahydro-1,3,5,7-tetranitro-1,3,5,7-tetrazocine (HMX), pentaerythritol tetranitrate (PENT), and 2,4,6-trinitrotoluene (TNT) molecules. We demonstrate that as the length of the BN region increases, the sensitivity of the heteronanosheets to the presence of these explosive substances increases.
1. Introduction
The ability to detect explosive materials and their compounds is a major challenge, which has recently become an important priority for security applications.1–5 A variety of laboratory-based approaches have been employed for discriminated sensing of explosives,4–7 for instance, non-portable devices for detecting such as gas chromatography,6,8,9 ion-mobility spectrometer, electronic noses,3,10–12 mass spectrometer,3,4,6,13 high pressure liquid chromatography-diode array detection,14,15 radiation,2–4,6,8 and Raman spectroscopy.16,17
To meet the challenge of designing effective, portable and less expensive discriminating sensing nanodevices, there is a need to develop novel nanostructure materials and concepts, with devising new strategies for managing and developing on sensor chips. Nanomaterials might be promising nominees to design chemical sensors with high sensitivity.18–23 To avoid the high costs and preclude the need for chemical amendment or separation of the analytes, the label free techniques for discriminating sensing of molecules are coveted targets in technology.24 Graphene–boron nitride heterostructures have attracted the attention of scientists and research groups due to their unique electrical, mechanical, morphological, thermal properties and optoelectronics.25–29 In-plane junctions between single layer boron nitride and graphene are already synthesised,30–33 but the heterostructure materials are challenging to recognise experimentally. Doping graphene derivatives with heteroatoms are promising materials for high-performance sensing,34 for example gas and toxic gas sensors.35–39 The detection of target analytes using graphene materials is mostly depend on the changes of the conductance after the adsorption of analytes to be sensed.34 Graphene–boron nitride heterostructures have been utilised as gas sensor.40–43 For example, it has been reported that graphene–boron nitride heterostructure is stable structure, and also the Fermi level, work function, and conductivity are remarkably changed upon adsorption of the analytes on them compared to the pristine graphene–boron nitride heterostructures. Other studies44–46 demonstrated the potential of graphene–boron nitride heterostructures for biosensors. For example, it was shown that the nanopore formed from graphene–boron nitride heterosheet can be used for discriminating sensing of the four nucleotides of DNA.
The 2,4-dinitrotoluene (DNT), octahydro-1,3,5,7-tetranitro-1,3,5,7-tetrazocine (HMX), pentaerythritol tetranitrate (PENT), and 2,4,6-trinitrotoluene (TNT) molecules (shown in Fig. 1, middle sub-figure) are common explosives. The development of suitable detector to sense this materials has received attention from research groups.5,7,47–54 In this paper, we demonstrate that graphene–boron nitride–graphene heteronanosheet (h-NSHs) can be a promising candidate for discriminating sensing of these explosive materials. h-NSHs are formed from two graphene nanosheets as left/right leads connecting via equivalent boron nitride nanosheet as scattering region as shown in Fig. 1a–c. Electrical current flows from left lead (G, graphene) to the right lead (G, graphene) through the scattering region (BN, boron nitride). To understand the change in conductance of the h-NSHs when single explosive molecule binds to the h-NSHs, we perform first principal computations. In what follows, by analysis the results of the response of the h-NSHs junctions to different BN-region with varied lengths, a notable fingerprint of each analyte is obtained which can be used for sensing.
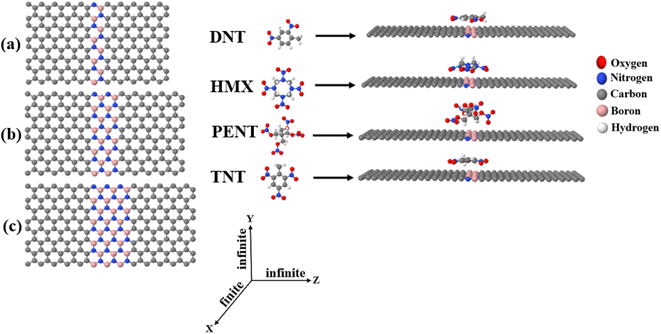 |
| Fig. 1 Shows (a–c) the h-NSH junctions constructed from graphene joined by a perfect insulator BN, ranging from 1BN strip to 3BN strips. (Right) An example of h-NSH with 1BN junction with four explosive molecules (DNT, HMX, PENT and TNT) on 1BN scatterer. | |
2. Characterising of the junctions
Fig. 1 shows the three h-NSHs (labelled a–c) which are composed of graphene (G) nanosheet as left/right leads and connected by boron nitride (BN) nanosheet scatterer of length varying from one strip to three strips (1BN to 3BN, respectively).
To find the ground state geometry of the h-NSHs, we employed the SIESTA55 implementation of density functional theory (DFT). For all structures, we used the local density approximation (LDA)56 with Ceperley–Alder (CA) parameterisation, the double zeta polarized (DZP) basis sets of pseudoatomic orbitals with norm-conserving pseudopotentials. The initial h-NSHs were relaxed until the forces below 0.01 eV Å−1. For lead calculations, a k-point grid of 1 × 30 × 30 is sampled in the Brillouin zone. All the h-NSHs are infinite in Y and Z directions, whereas a vacuum space of 50 Å has been chosen along the X direction to avoid the interaction between neighbouring h-NSHs.
After obtaining the relaxed geometry of each analyte, the analytes were placed on 1BN, 2BN and 3BN scatters, as shown in Fig. S1,† which shows the relaxed h-NSHs with 1BN, 2BN and 3BN scatterer after placing the explosive molecules on BNs regions. To investigate electrical conductance and current through the junctions, we employ Landauer approach,57 and calculate transmission coefficients T(E) for electrons of energy (E) passing from left lead to the right lead through the boron nitride (BN) scatterer using the Green's function based quantum transport code GOLLUM58 combined with the mean field Hamiltonian (MFH) that obtained from SIESTA.
3. Discriminating single-molecule sensing of the h-NSH junctions
In this section, we investigate the electron transmission coefficients T(E) of the bare h-NSH junctions with varied BN length as shown in Fig. 2a.
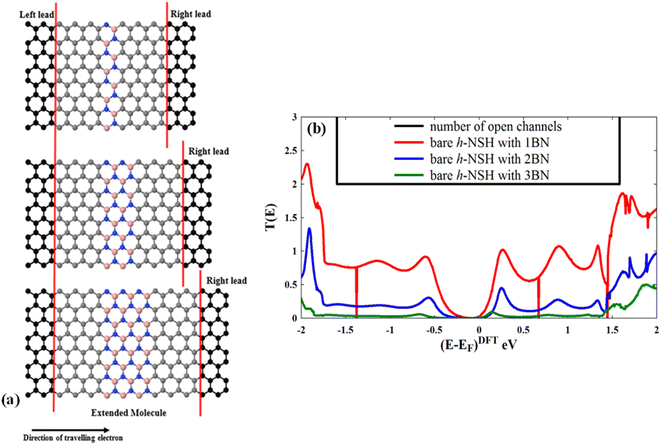 |
| Fig. 2 Shows (a) the characterisation of the bare h-NSH junctions and (b) their electronic transmission coefficients T(E). | |
Fig. 2b shows the corresponding T(E). We note that the energy gap progressively increases as the BN region length increases, and also the T(E) drops exponentially with the length of the BN region when the energy (E) lies within the energy gap of the BN scatterer.24,59
We also calculate the maximum current (I) at room temperature for small finite voltage of the three bare h-NSH junctions, which carried by the system from the left lead to the right lead using the following equation:57
|
 | (1) |
where
e = |
e| is the electron's charge,
h is Planck's constant,
T(
E) is the electronic transmission coefficient,
f is Fermi–Dirac distribution function
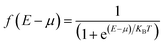
, which associated with the electrochemical potential
μ,
kB is Boltzmann's constant, and
T is temperature. The obtained current shown in
Fig. 3.
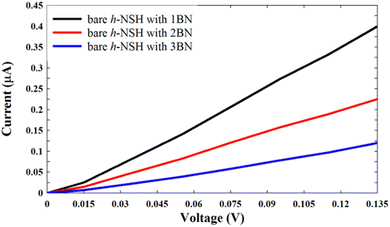 |
| Fig. 3 Shows the I–V curves for the junctions in Fig. 2a. | |
From Fig. 3, we can see that the current decreases as the BN length increases.
Next, we examine the h-NSH with 1BN junction for discriminating sensing. For this we first find the ground state geometry of the DNT, HMX, PENT and TNT molecules by calculating the binding energies (EBin) and performing geometry optimisation of molecules on 1BN, 2BN and 3BN as shown in Fig. S1.† To calculate the EBin, we use eqn (2) to minimise the basis set superposition error (BSSE):60,61
|
EBin = Eh-NSH+mol − (Eh-NSH + Emol)
| (2) |
where the total energy of the system (h-NSH with molecule) is
Eh-NSH+mol,
Eh-NSH (h-NSH) and
Emol (molecule) are the total energies of the isolated subsystems.
Table 1 shows the obtained binding energies of the h-NSH with 1BN/2BN/3BN after placing the DNT, HMX, PENT and TNT molecules on the 1BN/2BN/3BN regions.
Table 1 The binding energy (EBin) of the DNT, HMX, PENT and TNT molecules place on BN region of the h-NSH with 1BN/2BN/3BN junctions
Junction |
EBin (eV) |
+DNT |
+HMX |
+PENT |
+TNT |
h-NSH with 1BN |
−0.301 |
−0.387 |
−0.410 |
−0.312 |
h-NSH with 2BN |
−0.411 |
−0.460 |
−0.540 |
−0.480 |
h-NSH with 3BN |
−0.570 |
−0.490 |
−0.460 |
−0.510 |
From Table 1, it is clear that the obtained binding energies for the h-NSH with 1BN are from −0.410 eV to −0.301 eV, for the h-NSH with 2BN are ranging from −0.540 eV to −0.411 eV, and for the h-NSH with 3BN are from −0.570 eV to −0.460 eV. PENT has the highest binding energy to 1BN and 2BN regions, while DNT molecule shows highest binding energy to 3BN.
Now we calculate transmission coefficient T(E) of electrons with energy E traversing from one electrode to the other through h-NSH with 1BN junction in the absence and presence of analyst.
Form Fig. 4a–d, we can see that a notable fingerprint for each molecule around the EF comparing to the bare h-NSH with 1BN. Similarly, we calculate the T(E) of the h-NSHs with 1BN/2BN in the presence of analytes (DNT, HMX, PENT and TNT) on 2BN and 2BN scatterers as shown Fig. S2 and S3 in the ESI.† Once again, we obtain remarkable fingerprints for each molecule around EF. These new peaks around the EF due to the present of explosive molecules can lead to enhancement the Seebeck coefficient (S) as shown in Fig. 5, which can be used for sensing molecules using Seebeck effect (Seebeck sensing). For more clarity, see Table S1,† which shows the values of the Seebeck coefficient of the h-NSHs with 1BN/2BN/3BN junctions introducing the analytes.
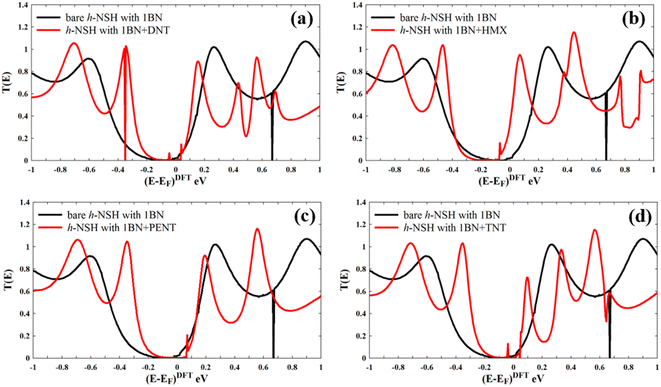 |
| Fig. 4 Shows the T(E) of the relaxed junctions (a) h-NSH with 1BN + DNT, (b) h-NSH with 1BN + HMX, (c) h-NSH with 1BN + PENT, (d) h-NSH with 1BN + TNT. In all figures, the Fermi energy (EF) is shifted at zero. | |
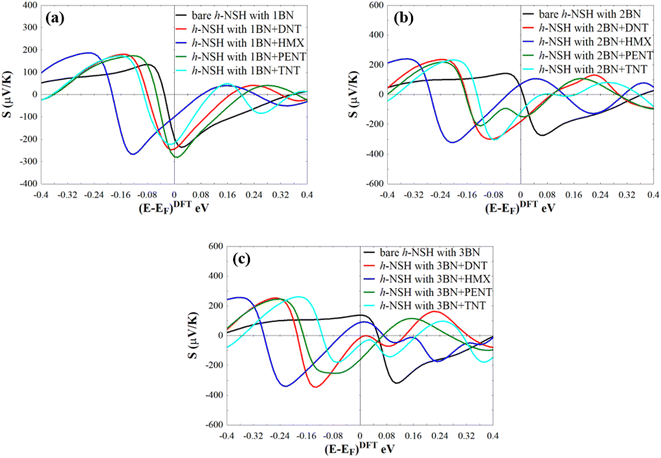 |
| Fig. 5 Shows the room-temperature thermopower (S) of the junctions with/without molecules, (a) h-NSH with 1BN, (b) h-NSH with 2BN, and (c) h-NSH with 3BN. In all figures, bare junctions are in black line and the Fermi energy (EF) is shifted at zero. | |
To confirm the ability of the h-NSH with 1BN junction for discriminating sensing of the analytes (shown in Fig. S1a†), we used eqn (1) to calculate the current (I) as shown in Fig. 6.
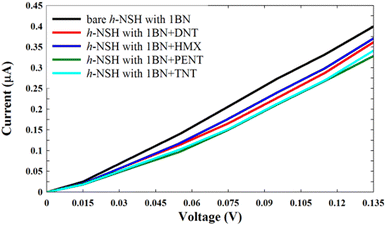 |
| Fig. 6 Shows the current of the h-NSH with 1BN junction after place the DNT, HMX, PENT and TNT on the 1BN scatterer. | |
From Fig. 6, we can see that each molecule has a specific value of current comparing with the current through the bare h-NSH with 1BN junction (black line). These results reinforce that the h-NSH with 1BN system can be used for discriminating sensing of the explosive molecules.
We repeated the same strategy with the h-NSHs with 1BN/2BN junctions shown in Fig. S1b and c,† the resulting I shown in Fig. 7.
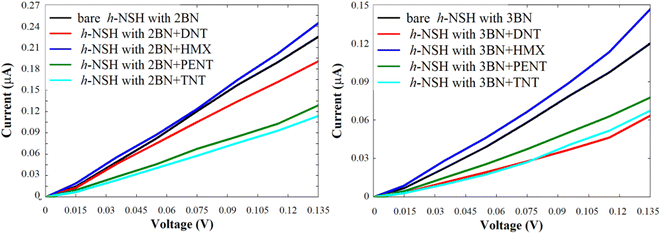 |
| Fig. 7 Shows the current of the h-NSH junctions (a) with 2BN and (b) with 3BN junctions after place the DNT, HMX, PENT and TNT on the BN scatterer. | |
Again, from Fig. 7, we can see obviously that each molecule has a particular value of the current comparing with the bare h-NSH with 2BN/3BN. The result show that the h-NSH with 1BN/2BN/3BN junctions are potential materials for discriminating sensing nanodevice of the explosive molecules, and the most preferable junctions for selective sensing are the h-NSH with 2BN/3BN junctions comparing with the h-NSH with 1BN junction.
When analyte physiosorbed on the membrane, it acts like a locale gate and modifies the potential energy of the membrane locally. Due to the charge transfer between the analyte and the membrane, the charge density on the membrane can also be affected. These can lead to the changes on the electrical conductance. Our calculations show that unlike graphene, these effects significantly influence the electrical conductance of membranes formed by graphene–hBN heterostructures.
4. Conclusions
We demonstrate that the h-NSH with BN (ranging from 1BN strip to 3BN strips) junctions are potential material for discriminating sensing of four explosive molecules (DNT, HMX, PENT, and TNT). We also show that an energy gap is formed by introducing BNs in graphene as scatterers, and can be turned by changing the length of the BN region. Our result demonstrates that the T(E) decay exponentially with increasing the length of the BN scatterer. Furthermore, our result show that the new peaks appear around the EF in the T(E) due to the presence of the explosive molecules lead to enhancement the Seebeck coefficient, which can be used for discriminating Seebeck sensing of molecules. Since both electrical current, and the sign and amplitude of Seebeck coefficient are changing upon translocation of analytes on the heterostructures, a combination of these factors can be used to reduce the error in the detection process. This provides a powerful tool for selective sensing of explosive materials with similar electronic finger print.
Author contributions
All authors were involved in interpreting the results, wrote and commented on the manuscript.
Conflicts of interest
The authors declare no conflict of interest.
Data availability
All requred information to reproduced the simulation data is given in the manuscript and the ESI.†
Acknowledgements
Laith A. Algharagholy, Qusiy H. Al-Galiby, Amaal A. Al-Backri, and Ahmed A. Wabdan acknowledge the Iraqi Ministry of Higher Education and Scientific Research, and Also University of Sumer, University of Al-Qadisiyah, and University of Baghdad for their support. H. S. acknowledges the UKRI for Future Leaders Fellowship number MR/S015329/2 and MR/X015181/1.
References
- N. R. Council, Existing and potential standoff explosives detection techniques, National Academies Press, 2004 Search PubMed.
- L. A. Algharagholy, H. Sadeghi and A. A. Al-Backri, Selective Sensing of 2, 4, 6-Trinitrotoluene and Triacetone Triperoxide using Carbon/Boron Nitride Heteronanotubes, Mater. Today Commun., 2021, 102739 CrossRef CAS.
- K. A. Singh, Technology Sector–Security. 2009 Search PubMed.
- J. Yinon, Detection of hidden explosives: An overview, American Laboratory, 2006. vol. 38, issue 12 Search PubMed.
- H. Vovusha and B. Sanyal, DFT and TD-DFT studies on the electronic and optical properties of explosive molecules adsorbed on boron nitride and graphene nano flakes, RSC Adv., 2015, 5(6), 4599–4608 RSC.
- R. E. Hummel, et al., Detection of explosive materials by differential reflection spectroscopy, Appl. Phys. Lett., 2006, 88(23), 231903 CrossRef.
- J. K. Cooper, C. D. Grant and J. Z. Zhang, Experimental and TD-DFT study of optical absorption of six explosive molecules: RDX, HMX, PETN, TNT, TATP, and HMTD, J. Phys. Chem. A, 2013, 117(29), 6043–6051 CrossRef CAS PubMed.
- J. Yinon, Field detection and monitoring of explosives, TrAC, Trends Anal. Chem., 2002, 21(4), 292–301 CrossRef CAS.
- S. D. Huang, L. Kolaitis and D. M. Lubman, Detection of explosives using laser desorption in ion mobility spectrometry/mass spectrometry, Appl. Spectrosc., 1987, 41(8), 1371–1376 CrossRef CAS.
- J. S. Caygill, F. Davis and S. P. Higson, Current trends in explosive detection techniques, Talanta, 2012, 88, 14–29 CrossRef CAS PubMed.
- J. Yinon, Peer reviewed: detection of explosives by electronic noses, ACS Publications, 2003 Search PubMed.
- L. A. Algharagholy, H. Sadeghi and A. A. Al-Backri, Selective sensing of 2,4,6-trinitrotoluene and triacetone triperoxide using carbon/boron nitride heteronanotubes, Mater. Today Commun., 2021, 28, 102739 CrossRef CAS.
- I. Buryakov, Coefficient of ion mobility versus electric field strength dependence in gases: Experimental determination, Tech. Phys., 2002, 47(11), 1453–1457 CrossRef CAS.
- T. Borch and R. Gerlach, Use of reversed-phase high-performance liquid chromatography–diode array detection for complete separation of 2, 4, 6-trinitrotoluene metabolites and EPA Method 8330 explosives: influence of temperature and an ion-pair reagent, J. Chromatogr. A, 2004, 1022(1–2), 83–94 CrossRef CAS PubMed.
- R. Schulte-Ladbeck, P. Kolla and U. Karst, Trace analysis of peroxide-based explosives, Anal. Chem., 2003, 75(4), 731–735 CrossRef CAS PubMed.
- D. D. Tuschel, et al., Deep ultraviolet resonance Raman excitation enables explosives detection, Appl. Spectrosc., 2010, 64(4), 425–432 CrossRef CAS PubMed.
- X. Fang and S. Ahmad, Detection of explosive vapour using surface-enhanced Raman spectroscopy, Appl. Phys. B: Lasers Opt., 2009, 97(3), 723–726 CrossRef CAS.
- D. Krepel and O. Hod, Selectivity of a graphene nanoribbon-based trinitrotoluene detector: a computational assessment, J. Phys. Chem. C, 2017, 121(39), 21546–21552 CrossRef CAS.
- F. Patolsky, G. Zheng and C. M. Lieber, Fabrication of silicon nanowire devices for ultrasensitive, label-free, real-time detection of biological and chemical species, Nat. Protoc., 2006, 1(4), 1711–1724 CrossRef CAS PubMed.
- M. Pumera, et al., Graphene for electrochemical sensing and biosensing, TrAC, Trends Anal. Chem., 2010, 29(9), 954–965 CrossRef CAS.
- S. Alwarappan, et al., Probing the electrochemical properties of graphene nanosheets for biosensing applications, J. Phys. Chem. C, 2009, 113(20), 8853–8857 CrossRef CAS.
- S. Alwarappan, et al., The effect of electrochemical pretreatment on the sensing performance of single walled carbon nanotubes, J. Nanosci. Nanotechnol., 2009, 9(5), 2991–2996 CrossRef CAS PubMed.
- S. Kesavan, et al., Real time detection of adenosine and theophylline in urine and blood samples using graphene modified electrode, Sens. Actuators, B, 2019, 278, 46–54 CrossRef CAS.
- L. Algharagholy, et al., Sensing single molecules with carbon–boron-nitride nanotubes, J. Mater. Chem. C, 2015, 3(39), 10273–10276 RSC.
- J. Wang, F. Ma and M. Sun, Graphene, hexagonal boron nitride, and their heterostructures: properties and applications, RSC Adv., 2017, 7(27), 16801–16822 RSC.
- Y. Zhang, et al., Design and Fabrication of Piezoresistive Graphene/HBN Heterostructure Pressure Sensor, in 2018 Prognostics and System Health Management Conference (PHM-Chongqing), IEEE, 2018 Search PubMed.
- Z.-G. Chen, et al., Observation of an intrinsic bandgap and Landau level renormalization in graphene/boron-nitride heterostructures, Nat. Commun., 2014, 5(1), 1–6 Search PubMed.
- Y. Gao, et al., High-speed electro-optic modulator integrated with graphene-boron nitride heterostructure and photonic crystal nanocavity, Nano Lett., 2015, 15(3), 2001–2005 CrossRef CAS PubMed.
- L. A. Algharagholy, et al., Tuning thermoelectric properties of graphene/boron nitride heterostructures, Nanotechnology, 2015, 26(47), 475401 CrossRef PubMed.
- P. Sutter, et al., Interface formation in monolayer graphene-boron nitride heterostructures, Nano Lett., 2012, 12(9), 4869–4874 CrossRef CAS PubMed.
- Y. Miyata, et al., Fabrication and characterization of graphene/hexagonal boron nitride hybrid sheets, Appl. Phys. Express, 2012, 5(8), 085102 CrossRef.
- J. Thomas, et al., Step-flow growth of graphene-boron nitride lateral heterostructures by molecular beam epitaxy, 2D Mater., 2020, 7(3), 035014 CrossRef CAS.
- Z. Liu, et al., In-plane heterostructures of graphene and hexagonal boron nitride with controlled domain sizes, Nat. Nanotechnol., 2013, 8(2), 119–124 CrossRef CAS PubMed.
- S. Kaushal, et al., Heteroatom-doped graphene as sensing materials: A mini review, RSC Adv., 2020, 10(48), 28608–28629 RSC.
- X. Deng, et al., The improvement of the adsorption abilities of some gas molecules on g-BN sheet by carbon doping, Phys. E, 2011, 44(2), 495–500 CrossRef CAS.
- S. Seyyedi, Z. M. Pour and E. Nadimi, Application of graphene and aluminum doped graphene as a CO sensor: An ab initio study, in 2014 22nd Iranian Conference on Electrical Engineering (ICEE), IEEE, 2014 Search PubMed.
- D. Singh, A. Kumar and D. Kumar, Adsorption of small gas molecules on pure and Al-doped graphene sheet: a quantum mechanical study, Bull. Mater. Sci., 2017, 40(6), 1263–1271 CrossRef CAS.
- H. Cruz-Martínez, et al., Recent developments in graphene-based toxic gas sensors: A theoretical overview, Sensors, 2021, 21(6), 1992 CrossRef PubMed.
- Q. Zhang, et al., Graphene Functionalized by Doping and Defects for Gas Sensor Application, Sens. Mater., 2021, 33(4), 1411–1429 CAS.
- N. H. Rad, E. Nadimi, and N. Manavizadeh, Sensing Properties of Lateral Graphene/h-BN Heterostructure towards NH3: A First Principles Study, in 2019 27th Iranian Conference on Electrical Engineering (ICEE), IEEE, 2019 Search PubMed.
- F. A. de Souza, et al., Hybrid 2D nanodevices (graphene/h-BN): selecting NO x gas through the device interface, J. Mater. Chem. A, 2019, 7(15), 8905–8911 RSC.
- N. H. Raad, et al., Gas sensing properties of a two-dimensional graphene/h-BN multi-heterostructure toward H2O, NH3 and NO2: A first principles study, Appl. Surf. Sci., 2021, 565, 150454 CrossRef.
- J. Mawwa, et al., In-plane graphene/boron nitride heterostructures and their potential application as toxic gas sensors, RSC Adv., 2021, 11(52), 32810–32823 RSC.
- A. Kiakojouri, I. Frank and E. Nadimi, In-plane graphene/h-BN/graphene heterostructures with nanopores for electrical detection of DNA nucleotides, Phys. Chem. Chem. Phys., 2021, 23(44), 25126–25135 RSC.
- F. A. de Souza, et al., Electrically sensing Hachimoji DNA nucleotides through a hybrid graphene/h-BN nanopore, Nanoscale, 2020, 12(35), 18289–18295 RSC.
- F. A. de Souza, et al., Electrical detection of nucleotides via nanopores in a hybrid graphene/h-BN sheet, Nanoscale, 2017, 9(6), 2207–2212 RSC.
- F. Zapata, M. López-López and C. García-Ruiz, Detection and identification of explosives by surface enhanced Raman scattering, Appl. Spectrosc. Rev., 2016, 51(3), 227–262 CrossRef CAS.
- J. K. Cooper, C. D. Grant and J. Z. Zhang, Ab initio calculation of ionization potential and electron affinity of six common explosive compounds, Rep. Theor. Chem., 2012, 1, 11–19 CrossRef.
- L. C. Pacheco-Londono, O. M. Primera-Pedrozo and S. P. Hernandez-Rivera, Experimental and theoretical model of reactivity and vibrational detection modes of triacetone triperoxide (TATP) and homologues, in Optically Based Biological and Chemical Sensing for Defence, SPIE, 2004 Search PubMed.
- S. Parajuli and W. Miao, Sensitive determination of triacetone triperoxide explosives using electrogenerated chemiluminescence, Anal. Chem., 2013, 85(16), 8008–8015 CrossRef CAS PubMed.
- A. K. Jaini, et al., Halogen bonding interactions for aromatic and nonaromatic explosive detection, ACS Sens., 2019, 4(2), 389–397 CrossRef CAS PubMed.
- C. Wang, et al., Trace detection of RDX, HMX and PETN explosives using a fluorescence spot sensor, Sci. Rep., 2016, 6(1), 1–9 CrossRef PubMed.
- S. e. Sağlam, et al., Electrochemical determination of TNT, DNT, RDX, and HMX with gold nanoparticles/poly (carbazole-aniline) film-modified glassy carbon sensor electrodes imprinted for molecular recognition of nitroaromatics and nitramines, Anal. Chem., 2018, 90(12), 7364–7370 CrossRef PubMed.
- M. Maziejuk, et al., Detection of Triacetone Triperoxide (TATP) and Hexamethylene Triperoxide Diamine (HMTD) from the Gas Phase with Differential Ion Mobility Spectrometry (DMS), Sensors, 2021, 21(13), 4545 CrossRef CAS PubMed.
- J. M. Soler, et al., The SIESTA method for ab initio order-N materials simulation, J. Phys.: Condens. Matter, 2002, 14(11), 2745 CrossRef CAS.
- D. M. Ceperley and B. J. Alder, Ground state of the electron gas by a stochastic method, Phys. Rev. Lett., 1980, 45(7), 566 CrossRef CAS.
- H. Sadeghi, Theory of electron, phonon and spin transport in nanoscale quantum devices, Nanotechnology, 2018, 29(37), 373001 CrossRef PubMed.
- J. Ferrer, et al., GOLLUM: a next-generation simulation tool for electron, thermal and spin transport, New J. Phys., 2014, 16(9), 093029 CrossRef.
- P. Moreno-García, et al., Single-molecule conductance of functionalized oligoynes: length dependence and junction evolution, J. Am. Chem. Soc., 2013, 135(33), 12228–12240 CrossRef PubMed.
- M. L. Senent and S. Wilson, Intramolecular basis set superposition errors, Int. J. Quantum Chem., 2001, 82(6), 282–292 CrossRef CAS.
- M. C. Daza, et al., Basis set superposition error-counterpoise corrected potential energy surfaces. Application to hydrogen peroxide⋯X (X = F−, Cl−, Br−, Li+, Na+) complexes, J. Chem. Phys., 1999, 110(24), 11806–11813 CrossRef CAS.
|
This journal is © The Royal Society of Chemistry 2022 |