DOI:
10.1039/D2RA04333E
(Paper)
RSC Adv., 2022,
12, 24178-24186
Identification of 4-acrylamido-N-(pyridazin-3-yl)benzamide as anti-COVID-19 compound: a DFTB, molecular docking, and molecular dynamics study†
Received
13th July 2022
, Accepted 16th August 2022
First published on 25th August 2022
Abstract
Omicron is one of the variants of COVID-19 and continuing member of a pandemic. There are several types of vaccines that were developed around the globe to fight against the virus. However, the world is suffering to find suitable drug candidates for the virus. The main protease (Mpro) enzyme of the virus is the best target for finding drug molecules because of its involvement in viral infection and protein synthesis. ZINC-15 is a database of 750 million commercially available compounds. We find 125 compounds having two aromatic rings and amide groups for non-covalent interactions with active site amino acids and functional groups with the capability to bind –SH group of C145 of Mpro through covalent bonding by a nucleophilic addition reaction. The lead compound (Z144) was identified using molecular docking. The non-covalent interactions (NCI) calculations show the interactions between amino acids present in the active site of the protein and the lead molecules are attractive in nature. The density functional-based tight-binding (DFTB) study of the lead compound with amino acids in the active site indicates that Q190 and Q193 play a very critical role in stabilization. The Michael addition of the acrylamide group of the lead molecule at β-position is facile because the low energy lowest unoccupied molecular orbital (LUMO) is concentrated on the group. From molecular dynamics during 100 ns, it has come to light that strong non-covalent interactions are key for the stability of the lead inside the protein and such binding can fold the protein. The free energy for this interaction is −42.72 kcal mol−1 which was obtained from MM-GB/SA calculations.
Introduction
In the last three years, the most rapidly growing scenario in the field of structural biology is the search for SARS-CoV-2 Mpro inhibitor complexes after the coronavirus and omicron outbreak.1 Although vaccination at a large scale is going on worldwide, still, the disease is far from being completely eliminated because of poor public compliance, and the emergence of new variants.2 It is well known that the viral proteases have long been presented to be effective targets for antiviral therapies, which represent potential targets for the inhibition of mainly viral replication.3,4 Most of the successful antiviral drugs including coronavirus targets are the main protease (Mpro, also called 3CLpro).1,5 Many of the protease inhibitors are being used in the treatment of COVID-19, owing to the well-designed role of Mpro in the viral life cycle, and hence, it becomes the most demanding protein target for the design of COVID-19 drugs.6,7
Recently, much effort has been paid to developing new vaccines improving the immune response to SARS-CoV-2 and its select strains.8,9 These consist of encapsulated RNA-based vaccine (mRNA-1273, Moderna), non-replicating viral vector (Sputnik V, Gamaleya; AZD1222, Oxford/Astra Zeneca), and inactivated viral vaccine (Covaxin, Bharat Biotech).8,9 However, the progress on new strains of SARS-CoV-2 (e.g., delta variant) facing a pronounced obstacle in attaining immunization comeback to treat this deadly disease. Instead, drug expansion campaigns can be stimulated to target intact structural proteins that may not undergo massive alterations in their structure and their associated functions.
In the way of drug discovery for COVID-19 and their variants through the development of SARS-CoV-2 Mpro inhibitors, the scientific community is working immensely from the very beginning. The first approach was attempted by Jin et al. and they have used a peptidomimetic α-ketoamide N3 which can inhibit the protein by the formation of a covalent bond with the C145 residue in the active site of the protein.10 Similarly, Hilgenfeld et al. designed a series of α-ketoamide as potential inhibitors of SARS-CoV-2 Mpro, which is crucial for blocking viral replication.11 In this case, lead molecules to exhibit potential inhibitory activity against SARS CoV-2 with IC50 = 0.67 ± 0.18 μM. While some of the Mpro inhibitors are in the preclinical or early clinical stages of a trial, there have been no approved treatments yet.
In silico methods were more successfully used not only to find new inhibitor molecules for the SARS-CoV-2 Mpro protein12 but also to understand the mechanism of action.13 Halazonetis and co-workers screened five molecules that can bind through non-covalent interactions.14 Sekijima was used in silico and in vitro methods to find lead compounds from 180 peptidyl secondary amides (from the ChEMBL database).15 Das Mohapatra and co-workers screened a series of natural products against the protein using molecular docking and dynamics.16 Same methodology was utilized by Sepay et al. for testing natural flavonoids, terpenoids, and alkaloids against the protein.17,18 Our group has also found the required structure of the lead molecules and interaction mechanism (for both non-covalent and covalent interactions) with the help of crystallographic information.13
ZINC-15 is a database of commercially available compounds, that can be accessed for virtual screening and contains 750 million compounds.19 In this study, we select 125 compounds for virtual screening to the target protein SARS-CoV-2 Mpro. We use molecular docking, NCIplots, DFTB, molecular dynamics, and MM-GB/SA studies to get lead molecules to find the nature of the interaction, to get interaction energy between the lead molecule and each amino acid, to understand their binding behaviour, and to evaluate the change of free energy for binding of a lead molecule to the protein.
Results and discussion
Choice of organic compounds
To choose a compound against a micro-organism, a target like protein, DNA, RNA, or other is required. In the case of coronavirus and other variants, it contains the largest viral positive-stranded RNA as genetic material, four structural and sixteen non-structural proteins.20 Among them, the main protease enzyme of severe acute respiratory syndrome virus (SARS-CoV-2 Mpro), a non-structural protein, is our target because it plays a key role in the viral infection mechanism and replication.10,11 Another advantage of its choice is the structural and functional dissimilarity with the human protease enzyme. Therefore, compounds targeting this enzyme will not hamper the normal function of human protease.10,11
Structurally, the SARS-CoV-2 Mpro enzyme is a cysteine protease. It contains C145 and H41 amino acids as a catalytic dyad in the S1′ domain of the active site (having S1, S1′, S2, and S4 domains) of the protein.13 Here, –SH of C145 acts as a nucleophile in the hydrolysis of the peptide bond, and H41 acts as a base to deprotonate and protonate the –SH in the course of the catalytic process.13 Therefore, this nucleophilic nature of the C145 can be utilized for inhibition of the protein activity with the help of more electrophilic compounds with respect to the peptide (Fig. 1a). An intense literature investigation revealed that there are only a few functional groups are used for targeting cysteine in the active site through a covalent bond.21–27 These functional groups are acrylamides, acrylonitriles, activated acetylenes, α,β-unsaturated ketones, α-chloroacetamides, primary haloalkanes, epoxides, methyl vinylsulfones, phenylsulphonate esters, and aminomethyl methyl acrylates (Fig. 1b). First four are most popular choice.
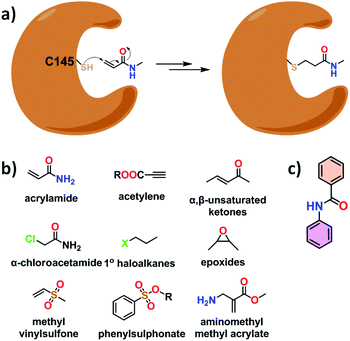 |
| Fig. 1 (a) Inhibition of SARS-CoV-2 Mpro enzyme through covalent bond formation, (b) cysteine targeting functional groups, and (c) essential functionality for SARS-CoV-2 Mpro protein binding. | |
A detailed crystallographic investigation of the active site of the SARS-CoV-2 Mpro enzyme shows that the N–H bond of C145 and G143 creates a hydrophilic pocket that can recognize carbonyl group and for better binding of a compound in the active site two aromatic rings connected with amide group are essential (Fig. 1c).13 On the basis of these observations, we set the rule for choosing compounds from the ZINC15 database. The compound having acrylamide groups, or two aromatic rings connected with an amide group, or both were searched in the database and found 125 compounds out of 750 million purchasable compounds available in it. In this case, compounds with 40% structural similarity were considered. These selected compounds were used in a molecular docking study which was validated with the help of molecular dynamics.
Protein structure preparation
At present time, there are 492 crystal structures of SARS-CoV-2 Mpro enzymes are present in the protein data bank (PDB). For this study, the crystal structure having the highest resolution (1.25 Å, PDB id 7JKV28) has been chosen and fixed the missing residues of protein were through homology modeling. The GMQE and QMEANDisCo Global scores29 of the obtained model are 0.96 and 0.90 ± 0.05, respectively, which are very good scores for the model (Fig. S1, see the ESI†). The correctness of the model was checked by finding missing atoms and/or residues of it. The modeled structure of the protein was energy minimized to use in further studies. The energy of the model before and after minimization were −3.285 × 103 and −4.915 × 103 kcal mol−1, respectively, and the change in structure can be visualized through their overly (Fig. S2, see ESI†).
The structure of the model was validated for the structure annotation and stereochemistry of the protein structures especially the secondary structures after energy minimization. z-Score is the indication of the overall quality of the model and a low score shows its good quality. In the case of energy minimized modeled protein, the z-score value is −7.26 which demonstrates it is a very good model and has a native protein-like structure (Fig. 2a). The local model quality can be shown in terms of energies versus amino acid sequence position i plotting. The plot shows that most of the amino acids have negative scores which is evidence of good quality structure (Fig. 2b). The amino acid residues in the protein may be stabilized or destabilized after energy minimization which is demonstrated in Fig. 2c. In the 3D structure of the protein, they are indicated by deep blue and red color coding, respectively (Fig. 2c).
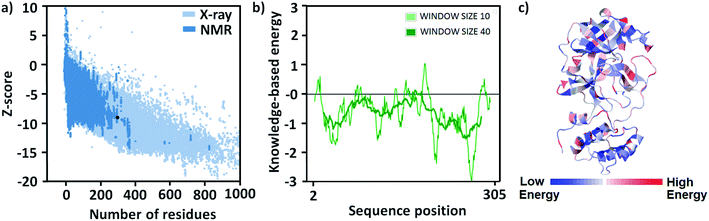 |
| Fig. 2 (a) A plot of z-score with respect to number of residues of different proteins, (b) energy versus amino acid sequence position i plot, and (c) 3D structure of the protein with notation of stable and unstable residues in it. | |
Molecular docking and NCI plot
Like quantitative structure–activity relationship (QSAR), molecular docking is one of the popular methods used to find a new pharmacophore lead molecule.30–32 All the 125 selected molecules are docked to the SARS-CoV-2 Mpro enzyme and calculated the values of the change of free energy for their interaction with the protein are tabulated in Table S1 (see ESI†). Based on these results, the molecule having a highest negative value of the free energy change is the lead compound.
Most of the compounds are showing the ΔGo in the range of −4.7 to −6.5 kcal mol−1. Only two compounds interact with −7.3 and 7.0 kcal mol−1 ΔGo values and their zinc id are ZINC736024144 and ZINC137137124, respectively. Their chemical structures are represented in Fig. S3.† Therefore, the lead compound is ZINC736024144 (Z144). It was observed that the molecule (pink) bound at the active site of the protein (Fig. 3a) by covering S1′, S1, and S2 domes at the position where the ligand (cyan) covalently attached (found in X-ray crystallography study). It is very interesting to note that, in the docking pose, the β position of the acrylamide group is closer to the SH group of C145. They are only 4.17 Å separated from each other (Fig. 3b). It indicates that the SH group has the potential to attack at the aforesaid position through the Michael reaction.
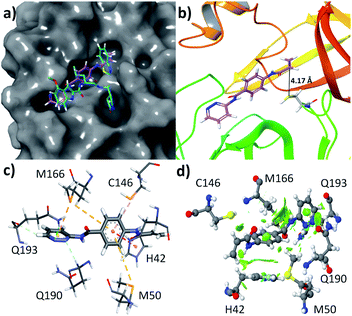 |
| Fig. 3 (a) Docking pose of Z144 in the active site of SARS-CoV-2 Mpro protein, (b) the distance between the β position of acrylamide group and the SH group of C145, and (c) different non-covalent interactions between Z144 and active site amino acid residues of SARS-CoV-2 Mpro protein and their (d) NCI plot of the interactions. | |
The compound Z144 contains one pyridazin-3-amine moiety, p-aminobenzoic acid (PABA) part, and one acrylamide group. All these groups are assembled through an amide linkage. In the active site of the protein, the molecule interacts with the amino acids through hydrophobic interactions, and aromatic rings of Z144 play an important role (Fig. 3c). The pyridazine ring interacts with Q190 by conventional hydrogen bonding, with Q193 through both conventional as well as non-conventional hydrogen bonding, and with M166 via π⋯S interaction. The aromatic ring of the PABA unit is involved in C–H⋯π interactions with M166, π⋯S with contacts M50 and C146, and π⋯π interactions with H42.
To understand, whether these interactions are favorable or not, an NCI plot was calculated over 0.35 iso surface. It was found that the green iso surface in the contact region of the above-mentioned interactions indicates the attractive forces between them. Therefore, all the interactions between Z144 and active site amino acid residues are attractive in nature and can be stabilized the molecule inside the active site of the protein. To validate the results we performed molecular dynamics with the Z144 – SARS-CoV-2 Mpro complex.
Electron localization function (ELF) diagram
ELF assists in finding out an electron in an adjacent region of the mentioned electron with the identical spin situated at a specific point.33–35 The areas with the finding possibility of an electron having an opposite spin-pair function or where the sole electron is the most intense have the highest Pauling repulsion. The ELF value of this region is maximum i.e. 1. Oppositely, the minimum Pauli repulsion region shows a minimum ELF value i.e. 0. Hence, the region with well-localized electrons like lone pairs, chemical bonds, atomic shells, etc. will show the strongest Pauli repulsion. Therefore, this knowledge can give crucial information on bonding, chemical structure, and reactivity. ELF information provides the picture of delocalized and localized electrons in the molecules. The localized electrons are more available for chemical reactivity and interactions. The 2D and 3D representation of ELF is similar to those used in geographical maps. The color coding in ELF is from red to blue color where red indicates the highest ELF (14 × 101), from orange, yellow to light green, and sky blue demonstrates middle values of ELF (ca. 0.7), and any lower values are expressed by dark blue.36–38
It is to be noted that for Z144, a very high value takes place at two ends and in a small patch in between them (Fig. 4). The bright red patches (top and bottom) are appeared due to the pyridazine ring and acrylonitrile unit where electrons are localized. However, the region between these two patches is of PABA unit where the ELF values are low. Therefore, in this molecule PABA unit is in electron delocalization. The thin red spot in this region is due to the oxygen lone pair of C
O. The ELF analysis indicates that the electron-rich region (red spots) can interact with the amino acids present in its vicinity through non-covalent interactions.
 |
| Fig. 4 Shaded surface map with projection effect of Z144. | |
DFTB and DFT studies
To explore the interactions between Z144 and amino acid, the DFTB technique was also utilized for the calculation of energy involved in each interaction. All the Z144–amino acid interactions obtained from the molecular docking study were optimized through this method. Non-covalent interactions play a very important role in the stability of the aforesaid complexes. Therefore, the DFTB methodology can be helped to analyze the interactions in a thermodynamic manner. Ganji and co-workers showed that optimization of each interaction can be used to optimize the entire system.39 In our study, the same approach has been used to explore the Z144–active site amino acid interactions. The model systems were relaxed initially to estimate the interaction energies.
The DFTB calculations indicate that all the interactions obtained from the docking study are favourable. All the results obtained from the calculations are summarized in Table 1. The S⋯π interaction between –SH of C146 and the aromatic ring of PABA of Z144 produces (Fig. 3c) −9.174 kcal mol−1 energy. However, the other two S⋯π contacts by M166 and M50 with the different parts of Z144 are not so strong with respect to the former one and their interaction energies are −4.440 and −1.250, respectively. In this context, it may be mentioned here that the contact distances of the last are sufficiently higher than that of the former (Table 1). The interaction energy for Q193 and Z144 have contributed the highest which may be due to the conventional hydrogen bonding between pyridazine nitrogen and the NH2 group of the amino acid. The second pyridazine nitrogen showed non-conventional hydrogen bonding with Q190 residue which gave −12.002 kcal mol−1 interaction energy. The interaction energy value for parallel-displaced π⋯π interaction of imidazole ring of H41 residue with benzene ring of PABA is −7.174 kcal mol−1. Therefore, above mentioned hydrogen bonding interactions of Z144 molecule have a critical role in its stability in the active site. Again, the energy values of C146 indicate its interaction strength is moderate which makes it flexible to nucleophilic attack on the acrylamide group situated very close to its interaction site. Similarly, moderate energy value in the case of H41, makes it available for acid–base reaction with C145 during its nucleophilic attack.
Table 1 Different parameters for the interactions of active site amino acids and Z144
Amino acid |
ΔE (kcal mol−1) |
Distance (Å) |
Angle (°) |
C146 |
−9.174 |
3.96 |
122.12 |
M166 |
−4.440 |
3.66 |
54.88 |
Q193 |
−19.413 |
2.33 |
164.03 |
Q190 |
−12.002 |
2.37 |
156.36 |
M50 |
−1.250 |
6.36 |
58.64 |
H41 |
−7.396 |
3.84 |
68.24 |
Molecular mechanics and DFT-based methods were used to understand the nature of Z144. The molecule has six single bonds where atoms/groups attached to the ends of the bonds are rotatable around the bond axis. All the favourable conformers were calculated and overlaid with their structures (Fig. 5a). The outcome of the experiment shows that all the molecular fragments (benzamide, acrylamide, and pyridazine) are almost coplanar to each other in all the obtained conformers which may be due to the resonance stabilization. The amide nitrogen-aromatic ring single bonds are more allowed to rotate which offers the equal distribution of pyridazine nitrogen atoms and acrylamide C
C bond across their bond axis. This study also shows that the degree of deviation from the co-planarity pyridazine ring is the highest. This feature was also found in a molecular docking study.
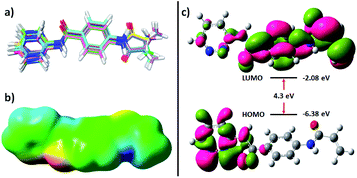 |
| Fig. 5 (a) Overlay of different favourable conformers of Z144, (b) molecular electrostatic potential, and (c) HOMO and LUMO of the molecule. | |
Molecular electrostatic potential map of the conformer found in docking study of the molecule two bright red patches in the iso surface which represents the amide oxygen atoms and nitrogen atoms of pyridazine ring (Fig. 5b). These atoms are available for hydrogen bonding through electron donation. Two dark blue regions can also be identified on this surface which corresponds to the acidic hydrogen atoms (amide N–H). A large green surface indicates neutral regions. These results show that the molecule can interact with other molecules through both hydrophilic and hydrophobic interactions.
The highest occupied molecular orbital (HOMO) and lowest unoccupied molecular orbital (LUMO) of a molecule provides knowledge of its chemical reactivity. The energy and orbitals of the electronic states of Z144 were calculated and represented in Fig. 5c. The energy of HOMO of Z144 is quite low and electrons are concentrated mainly in the pyridazine ring. The low LUMO energy of the molecule (−2.08 eV) makes it a very good electron acceptor. LUMO is concentrated over PABA and acrylamide units. The electron density at the beta position of the acrylamide C
C bond indicates its feasibility to undergo nucleophilic attack at this center in the active site of the protein by the –SH of C145. Therefore, considering all these electronic feature, it may be state that Z144 can be a very good inhibitor of the SARS-CoV-2 Mpro enzyme.
Molecular dynamics (MD)
The physical movements of atoms and molecules can be simulated and analysed by a computational method called MD. In drug discovery, the method plays a very important role to find the binding site, understanding the behaviour of small molecules in the binding site of the receptor (especially protein), enhancing the traditional virtual-screening methodologies, and predicting binding energies for protein–small molecule interactions. MD of SARS-CoV-2 Mpro protein and with Z144 were performed here for 100 ns.
This study shows the small molecule (orange) is stable at the active site where it (green) was found in the docking study (Fig. 6a). However, it was found to be little displaced toward the S1′ region of the active site. Root mean square deviation (RMSD) of the Z144 changed rapidly in the first 10 ns, then decreased for 14 ns and increase sharply which may be due to the interaction of the molecule with active site amino acids (Fig. 6b). RMSD of Z144 varies from 0.25 to 0.52 ns. After this interaction, the molecule became gradually unstable till 60 ns and then stabilized during 80 ns. It was found that the compound is stable in the last 20 ns. Therefore, the molecule can bind with the SARS-CoV-2 Mpro protein and form a stable complex with it.
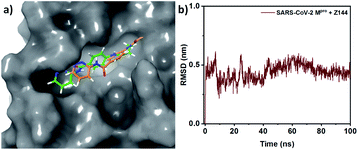 |
| Fig. 6 (a) Overlay of Z144 bound SARS-CoV-2 Mpro enzyme structures obtained from molecular docking (green) and dynamics (orange), (b) RMSD of Z144 in the 100 ns MD trajectory of its complex with SARS-CoV-2 Mpro. | |
MD helps to understand the effect of Z144 on the protein structure during their interaction in the active site. The RMSD of the SARS-CoV-2 Mpro protein is in the range of 0.25–0.34 nm (Fig. 7a). Initially, the RMSD of the protein–Z144 complex fluctuates immensely with respect to the native protein in the first 20% of the trajectory. It shows maxima of 50 ns and gradually decreased to the end of the trajectory. The fluctuation of RMSD of protein–Z144 complex is low in this part than that of the unbound protein.
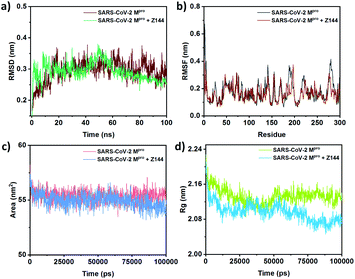 |
| Fig. 7 Comparison of (a) RMSD, (b) RMSF, (c) SASA, and (d) Rg between free SARS-CoV-2 Mpro and Z144 bound SARS-CoV-2 Mpro. | |
The root mean square fluctuation (RMSF) of the amino acids of the protein-bound and unbound state with Z144 were obtained from their 100 ns MD (Fig. 7b). The change of RMSF values of amino acids is almost similar in both systems. Amino acid residues 23, 42, 50, 81, 121, 136, 166, 187, 190, 193, 219, 280, and 285 were found to be stabilized may be due to the direct or indirect interactions with the compounds. This list contains all the amino acids found in molecular docking. Therefore, all these amino acid residues are in the short contact with the investigating compound. Hence, these amino acids can form a stable complex with the Z144 in the active site of the protein.
Solvent-accessible surface area (SASA) of a bio-macromolecule is the surface area available for solvent interactions. For a protein molecule, this parameter is the decisive factor in its stability, folding, and unfolding with time. Generally, the driving force of protein folding in water is the hydrophobic effect of non-polar amino acid side chains which trends to bury in the protein core. The degrees to which such side-chains interact with the protein core and the solvent spontaneously correspond to the surface area exposed to the solvent. The SASA of the SARS-CoV-2 Mpro protein unbound and bound with Z144 varies within 54–57 nm2 (Fig. 7c). SASA of the second case is slightly lower from former in last 40 ns of the trajectory. The results indicate the binding of Z144 to the protein cause folding of it in the course of MD simulation.
The radius of gyration (Rg) is the root-mean-square average of the distance of all the atoms from the centre of the protein. Therefore, a decrease or increase of Rg values indicates compactness or diffuseness of a protein, respectively. The effect of binding a molecule to the protein site can be understood by Rg because it demonstrates the conformational change of the protein. The average Rg values of the native protein and its complex with Z144 were found to be approximately 2.12 nm and 2.09 nm, respectively (Fig. 7d). The compactness of the protein in both free and ligand-bound states is almost similar in the first 60% of the trajectory. However, the protein becomes slightly more compact in the remaining part of the trajectory. The result was also supported by the surface area of the protein which was 10
814.65 Å2 and 10
577.57 Å2 for the protein after molecular docking and MD, respectively. This result indicates the compactness of the protein-enhanced due to internal adjustment of Z144 inside the active site of the protein through optimization of the non-covalent interactions with the amino acids present in the active site.
From the docking study, it was found that Q193 is involved in hydrogen bonding interaction with the compound. However, the MD simulation shows that two hydrogen bonding are present on average during the trajectory (Fig. S4†). Therefore, this protein–Z144 binding is hydrophobic interaction driven. This result is supporting the observation obtained in the molecular docking study.
MM-GB/SA
The efficiency of binding of small molecules in the binding site of a protein can be calculated using molecular mechanics where amino acid residues and small molecules are considered flexible. In this process, the Gibbs free energy change (ΔGo) is dependent on the gas-phase energy (MM), electrostatic solvation energy (GB), and non-electrostatic contribution to solvation energy (SA). Using these components, the method of ΔGo calculation is well-known as MM-GB/SA. In this case, ΔGo values of binding of Z144 in the active site of SARS-CoV-2 Mpro protein in a flexible manner were calculated through MM-GB/SA method. The value was found to be −42.72 kcal mol−1. Considering all the information mentioned above, it may be concluded that the compound Z144 is able to bind at the active site of the SARS-CoV-2 Mpro protein spontaneously through hydrophobic interactions.
Experimental
Protein structure preparation
The coordinates of the SARS-CoV-2 Mpro protein was downloaded from RCSB website and the PDB ID is 7JKV. This structure may have some missing atom or residue problem which was fixed by homology modeling with Swiss Model web-server.29 The small molecules present in the active site of the protein was removed and its atomic coordinates in the active site were considered as the original binding site of the ligand. To check whether all the missing atoms and residues are fixed correctly in the model, ProCheck program40 was used. This structure of the modeled protein was energy-minimized using NAMD41 on CHARMM General Force Field.42 For visualization of the structures (both protein and small molecules), chimera 1.10.2 software,43 Discovery Studio 2017 R2 client44 and Pymol were utilized. For structural validation and to check the stereochemistry and structure annotation, especially the secondary structures of these energy-minimized structures, ProSA-webserver45 was used.
Molecular docking
The molecular docking was performed on the energy minimized modelled protein structure of SARS-CoV-2 Mpro protein. All the 125 molecules under investigation were docked in active site of the protein. For this docking, AutoDockTools46 was used to prepare all the structures small molecules and protein including. Protonation states of all the compounds and protomers, tautomers and ionization states for protein were set out using the same tools. Using noBondOrder method, the Gasteiger charge of the protein was found to be −4.012. Same method was used for all the small molecule preparation and they are used to determine the number of rotatable bonds in it. Molecular docking between the protein and each small molecule were performed using AutoDock 4.2 (ref. 46) with the help of Lamarckian algorithm inside a 26 × 26 × 26 Å3 grid box at (at X = 3.259, Y = 5.064 and Z = 15.837 coordinates with a grid spacing of 1.00 Å).
The docking poses of the compounds in the protein was predicted with the help of binding energy, ligand efficiency, inhibition constant, intermolecular energy, van der Waals, hydrogen bond dissolve energy, total internal energy, torsional energy, and unbound energy using following scoring function.
ΔGbinding = ΔGvdW + ΔGelec + ΔGhbond + ΔGdesolv + ΔGtors |
All required docking results are recorded in the dlg file which was analyzed using AutoDockTools to find the docking poses and it was visualized by PyMol. In our study, the interactions between ligands and amino acids were defined as follows: hydrogen bond: 3.40 Å; D–H–A angle: 90–180°; CH–π interaction: 5.0 Å; angle: 40°; π–π interaction: 6.0 Å; angle: 50°; van der Waals interaction: 5.5 Å.47
NCIPlot
The NCIPlot of interactions between Z144 and amino acids present in the vicinity of the molecule was calculated using NCIPLOT Version 4.0 with standard procedure.48
Electron localization function (ELF) diagram
The electron structure characteristic (using ELF)35 of the molecule was calculated using 6-311G* basis set.49 Multiwfn program was used for the calculation and presentation.49
DFTB and DFT study
The compound that showed the best binding energy in molecular docking was investigated using DFTB and DFT methods.32,50 The docking pose of the molecule and amino acids interacting with it was chosen as starting point geometry. All the other amino acids were removed with the help of the Discovery Studio 2017 R2 client. GaussView 5 (ref. 51) was utilized to fix all the amino acids manually. The geometry of the model was optimized with this method. The SMART algorithm has been used. During the calculation, convergence tolerance energy and force were 0.05 kcal mol−1 and 0.5 kcal mol−1 Å−1, respectively. Here, the motion of groups was allowed. For parameters, the 3ob element interactions set from the Slater–Koster library were utilized.
The geometry of the docking conformation of the molecule was optimized with the help of Gaussian 09W D1 revision52 using the B3LYP functional and 6-311g basis set. The same level of theory has been employed for the molecular electrostatic potential map (MEP) generation. HOMO, LUMO, and MEP were visualized using GaussView 5 software.51
Molecular dynamics (MD)
For the MD simulations of SARS-CoV-2 Mpro protein and its complex with Z144 was performed using GROMACS.53 Here, the force field GROMOS96 43a1 was utilized. In this calculation, a cubic box of water with 0.15 M NaCl were used for the neutralization of charge on the protein surface. To minimize the energy of the system, Steepest Descent integrator was used. For getting the equilibrium at 300 K and 1.0 bar temperature and pressure, respectively, the NVT and NPT methods were utilized simultaneously. The molecular dynamics simulation was performed for 100 ns. In this case, 1000 frames is used for per simulation. For the short-range and electrostatic interaction calculations, the cut-off value and the PME were 10 Å and 1.0 Å, respectively.
MM-GB/SA
The binding free energy for the interaction of SARS-CoV-2 Mpro protein with Z144 was calculated by the MM-GB/SA method through the surface area-based generalized Born model with a hydrophobic solvent.54 All the steric clashes of amino acid residues present within 5.0 Å of Z144 was minimized using Prime MM-GB/SA. Here, these residues was allowed to be flexible during the minimization of the system under exploration.
Conclusions
In the summary of the work, we designed a workflow to find an effective molecule that can inhibit all the variants of COVID-19. Here, the target is to find a molecule that can bind with SARS-CoV-2 Mpro protein. The ZINC-15 database of commercially available molecules has been searched to build a set of small molecules which contain two aromatic rings connected by an amide group for good binding through non-covalent interactions and one such functional group where –SH of C146 amino acid (present in the active site of SARS-CoV-2 Mpro protein) can attach by a covalent bond. A total of 125 molecules were selected for this study and Z144 was found to be the best in the docking study. From both molecular docking and NCI plot, it was found that hydrophobic and similar types of non-covalent interactions are the key attraction forces for its protein binding. The energy involved due to the interaction between Z144 and each amino acid in the protein active site was calculated with the help of the DFTB method and found that Q190 and Q193 residues strongly interacted. The LUMO energy is sufficiently low and the orbital is concentrated over acrylamide and adjacent part which may facilitate the Michael addition at β-position of the group by –SH of C146. The protein–Z144 complex is stable after 100 ns molecular dynamics simulation. The protein folded after binding the compound during the trajectory.
Author contributions
NS conceptualise, supervise, some data collection, data analysis, and write the manuscript with the help of other author. The set of molecules was built from ZINC-15 and performed molecular docking by SC, MA and AA. DFTB calculation was performed by DM.
Conflicts of interest
There are no conflicts to declare.
Acknowledgements
NS gratefully acknowledged The Department of Chemistry, Lady Brabourne College for all the research facilities. Researchers Supporting Project number (RSP-2021/288), King Saud University, Riyadh, Saudi Arabia are gratefully acknowledged. DM acknowledge Department of Science and Technology, Government of West Bengal for research Grant (Award Number: 28(sanc)-ST/P/S&T/15G-4/2018) and Foundation for Science and Technology; Department of Science & Technology and Biotechnology, Government of West Bengal.
Notes and references
- C. Wu, W. Yin, Y. Jiang and H. E. Xu, Acta Pharmacol. Sin., 2022, 1–13 Search PubMed.
- C. M. C. Rodrigues and S. A. Plotkin, Front. Microbiol., 2020, 11, 1526 CrossRef PubMed.
- J. Magden, L. Kääriäinen and T. Ahola, Appl. Microbiol. Biotechnol., 2005, 66, 612–621 CrossRef CAS PubMed.
- I. A. El-Shimy, M. M. A. Mohamed, S. S. Hasan and M. A. Hadi, Pharmacol. Res. Perspect., 2021, 9, e00698 CAS.
- W. Cui, K. Yang and H. Yang, Front. Mol. Biosci., 2020, 7, 616341 CrossRef CAS PubMed.
- M. Hasan, M. S. A. Parvez, K. F. Azim, M. A. S. Imran, T. Raihan, A. Gulshan, S. Muhit, R. N. Akhand, S. S. U. Ahmed and M. B. Uddin, Biomed. Pharmacother., 2021, 140, 111742 CrossRef CAS PubMed.
- B. Luan, T. Huynh, X. Cheng, G. Lan and H.-R. Wang, J. Proteome Res., 2020, 19, 4316–4326 CrossRef CAS PubMed.
- N. C. Kyriakidis, A. López-Cortés, E. V. González, A. B. Grimaldos and E. O. Prado, npj Vaccines, 2021, 6, 28 CrossRef CAS PubMed.
- G. Forni and A. Mantovani, Cell Death Differ., 2021, 28, 626–639 CrossRef CAS PubMed.
- Z. Jin, X. Du, Y. Xu, Y. Deng, M. Liu, Y. Zhao, B. Zhang, X. Li, L. Zhang, C. Peng, Y. Duan, J. Yu, L. Wang, K. Yang, F. Liu, R. Jiang, X. Yang, T. You, X. Liu, X. Yang, F. Bai, H. Liu, X. Liu, L. W. Guddat, W. Xu, G. Xiao, C. Qin, Z. Shi, H. Jiang, Z. Rao and H. Yang, Nature, 2020, 582, 289–293 CrossRef CAS PubMed.
- L. Zhang, D. Lin, X. Sun, U. Curth, C. Drosten, L. Sauerhering, S. Becker, K. Rox and R. Hilgenfeld, Science, 2020, 368, 409–412 CrossRef CAS PubMed.
- H. M. Mengist, T. Dilnessa and T. Jin, Front. Chem., 2021, 9, 622898 CrossRef CAS PubMed.
- N. Sepay, P. C. Saha, Z. Shahzadi, A. Chakraborty and U. C. Halder, Phys. Chem. Chem. Phys., 2021, 23, 7261–7270 RSC.
- G. G. Rossetti, M. A. Ossorio, S. Rempel, A. Kratzel, V. S. Dionellis, S. Barriot, L. Tropia, C. Gorgulla, H. Arthanari, V. Thiel, P. Mohr, R. Gamboni and T. D. Halazonetis, Sci. Rep., 2022, 12, 2505 CrossRef CAS PubMed.
- K. Z. Yamamoto, N. Yasuo and M. Sekijima, J. Chem. Inf. Model., 2022, 62, 350–358 CrossRef CAS PubMed.
- D. Verma, D. Mitra, M. Paul, P. Chaudhary, A. Kamboj, H. Thatoi, P. Janmeda, D. Jain, P. Panneerselvam, R. Shrivastav, K. Pant and P. K. Das Mohapatra, Curr. Res. Pharmacol. Drug Discov., 2021, 2, 100038 CrossRef PubMed.
- N. Sepay, N. Sepay, A. Al
Hoque, R. Mondal, U. C. Halder and M. Muddassir, Struct. Chem., 2020, 31, 1831–1840 CrossRef CAS PubMed.
- N. Sepay, A. Sekar, U. C. Halder, A. Alarifi and M. Afzal, J. Mol. Struct., 2021, 1228, 129433 CrossRef CAS PubMed.
- J. J. Irwin, T. Sterling, M. M. Mysinger, E. S. Bolstad and R. G. Coleman, J. Chem. Inf. Model., 2012, 52, 1757–1768 CrossRef CAS PubMed.
- R. Lu, X. Zhao, J. Li, P. Niu, B. Yang, H. Wu, W. Wang, H. Song, B. Huang, N. Zhu, Y. Bi, X. Ma, F. Zhan, L. Wang, T. Hu, H. Zhou, Z. Hu, W. Zhou, L. Zhao, J. Chen, Y. Meng, J. Wang, Y. Lin, J. Yuan, Z. Xie, J. Ma, W. J. Liu, D. Wang, W. Xu, E. C. Holmes, G. F. Gao, G. Wu, W. Chen, W. Shi and W. Tan, Lancet, 2020, 395, 565–574 CrossRef CAS.
- S. G. Kathman, Z. Xu and A. V. Statsyuk, J. Med. Chem., 2014, 57, 4969–4974 CrossRef CAS PubMed.
- Q. Liu, Y. Sabnis, Z. Zhao, T. Zhang, S. J. Buhrlage, L. H. Jones and N. S. Gray, Chem. Biol., 2013, 20, 146–159 CrossRef CAS PubMed.
- E. Weerapana, G. M. Simon and B. F. Cravatt, Nat. Chem. Biol., 2008, 4, 405–407 CrossRef CAS PubMed.
- R. Mah, J. R. Thomas and C. M. Shafer, Bioorg. Med. Chem. Lett., 2014, 24, 33–39 CrossRef CAS PubMed.
- D. S. Johnson, E. Weerapana and B. F. Cravatt, Future Med. Chem., 2010, 2, 949–964 CrossRef CAS PubMed.
- M. S. Cohen, C. Zhang, K. M. Shokat and J. Taunton, Science, 2005, 308, 1318–1321 CrossRef CAS PubMed.
- D. Toledo Warshaviak, G. Golan, K. W. Borrelli, K. Zhu and O. Kalid, J. Chem. Inf. Model., 2014, 54, 1941–1950 CrossRef CAS PubMed.
- S. Hattori, N. Higashi-Kuwata, H. Hayashi, S. R. Allu, J. Raghavaiah, H. Bulut, D. Das, B. J. Anson, E. K. Lendy, Y. Takamatsu, N. Takamune, N. Kishimoto, K. Murayama, K. Hasegawa, M. Li, D. A. Davis, E. N. Kodama, R. Yarchoan, A. Wlodawer, S. Misumi, A. D. Mesecar, A. K. Ghosh and H. Mitsuya, Nat. Commun., 2021, 12, 668 CrossRef CAS PubMed.
- A. Waterhouse, M. Bertoni, S. Bienert, G. Studer, G. Tauriello, R. Gumienny, F. T. Heer, T. A. P. de Beer, C. Rempfer, L. Bordoli, R. Lepore and T. Schwede, Nucleic Acids Res., 2018, 46, W296–W303 CrossRef CAS PubMed.
- G. Venkatesh, Y. Sixto-López, P. Vennila, Y. S. Mary, J. Correa-Basurto, Y. S. Mary and A. Manikandan, J. Mol. Struct., 2022, 1258, 132678 CrossRef CAS.
- G. Raja, G. Venkatesh, J. S. Al-Otaibi, P. Vennila, Y. S. Mary and Y. Sixto-López, J. Mol. Struct., 2022, 1269, 133785 CrossRef CAS.
- M. Muthukkumar, T. Bhuvaneswari, G. Venkatesh, C. Kamal, P. Vennila, S. Armaković, S. J. Armaković, Y. Sheena Mary and C. Yohannan Panicker, J. Mol. Liq., 2018, 272, 481–495 CrossRef CAS.
- J. Poater, M. Duran, M. Solà and B. Silvi, Chem. Rev., 2005, 105, 3911–3947 CrossRef CAS PubMed.
- A. Fatima, G. Khanum, S. Kumar Srivastava, I. Verma, N. Siddiqui and S. Javed, Chem. Phys. Lett., 2021, 784, 139103 CrossRef CAS.
- A. Fatima, M. Singh, N. Singh, S. Savita, I. Verma, N. Siddiqui and S. Javed, Chem. Phys. Lett., 2021, 783, 139049 CrossRef CAS.
- A. Fatima, M. Singh, N. Agarwal, I. Verma, R. J. Butcher, N. Siddiqui and S. Javed, J. Mol. Liq., 2021, 343, 117549 CrossRef CAS.
- S. Savita, A. Fatima, K. Garima, K. Pooja, I. Verma, N. Siddiqui and S. Javed, J. Mol. Struct., 2021, 1243, 130932 CrossRef CAS.
- G. Khanum, A. Fatima, N. Siddiqui, D. D. Agarwal, R. J. Butcher, S. K. Srivastava and S. Javed, J. Mol. Struct., 2022, 1250, 131890 CrossRef CAS.
- M. Rezvani, M. Astaraki, A. Rahmanzadeh and M. Darvish Ganji, Phys. Chem. Chem. Phys., 2021, 23, 17440–17452 RSC.
- R. A. Laskowski, M. W. MacArthur, D. S. Moss and J. M. Thornton, J. Appl. Crystallogr., 1993, 26, 283–291 CrossRef CAS.
- J. C. Phillips, D. J. Hardy, J. D. C. Maia, J. E. Stone, J. V. Ribeiro, R. C. Bernardi, R. Buch, G. Fiorin, J. Hénin, W. Jiang, R. McGreevy, M. C. R. Melo, B. K. Radak, R. D. Skeel, A. Singharoy, Y. Wang, B. Roux, A. Aksimentiev, Z. Luthey-Schulten, L. V. Kalé, K. Schulten, C. Chipot and E. Tajkhorshid, J. Chem. Phys., 2020, 153, 044130 CrossRef CAS PubMed.
- K. Vanommeslaeghe, E. Hatcher, C. Acharya, S. Kundu, S. Zhong, J. Shim, E. Darian, O. Guvench, P. Lopes, I. Vorobyov and A. D. Mackerell, J. Comput. Chem., 2009, 31, 671–690 Search PubMed.
- E. F. Pettersen, T. D. Goddard, C. C. Huang, G. S. Couch, D. M. Greenblatt, E. C. Meng and T. E. Ferrin, J. Comput. Chem., 2004, 25, 1605–1612 CrossRef CAS PubMed.
- Dassault Syst., BIOVIA, Discov. Stud. Client, R2, Dassault Syst., San Diego, 2017 Search PubMed.
- M. Wiederstein and M. J. Sippl, Nucleic Acids Res., 2007, 35, W407–W410 CrossRef PubMed.
- G. M. Morris, R. Huey, W. Lindstrom, M. F. Sanner, R. K. Belew, D. S. Goodsell and A. J. Olson, J. Comput. Chem., 2009, 30, 2785–2791 CrossRef CAS PubMed.
- N. Sepay, R. Mondal, M. K. Al-Muhanna and D. Saha, New J. Chem., 2022, 46, 9735–9744 RSC.
- J. Contreras-García, E. R. Johnson, S. Keinan, R. Chaudret, J.-P. Piquemal, D. N. Beratan and W. Yang, J. Chem. Theory Comput., 2011, 7, 625–632 CrossRef PubMed.
- T. Lu and F. Chen, J. Comput. Chem., 2012, 33, 580–592 CrossRef CAS PubMed.
- G. Venkatesh, M. Govindaraju, C. Kamal, P. Vennila and S. Kaya, RSC Adv., 2017, 7, 1401–1412 RSC.
- GaussView, Version 5, Gaussian, Inc., Wallingford, CT, 2009 Search PubMed.
- Gaussian 09, Revision D.01, Gaussian, Inc., Wallingford, CT, 2009 Search PubMed.
- M. J. Abraham, T. Murtola, R. Schulz, S. Páll, J. C. Smith, B. Hess and E. Lindahl, SoftwareX, 2015, 1–2, 19–25 CrossRef.
- N. Sepay, M. Banerjee, R. Islam, S. P. Dey and U. C. Halder, Phys. Chem. Chem. Phys., 2022, 24, 6605–6615 RSC.
|
This journal is © The Royal Society of Chemistry 2022 |
Click here to see how this site uses Cookies. View our privacy policy here.