DOI:
10.1039/D2RA02092K
(Paper)
RSC Adv., 2022,
12, 17570-17584
Molecular investigations on Candida glabrata clinical isolates for pharmacological targeting†
Received
31st March 2022
, Accepted 3rd June 2022
First published on 14th June 2022
Abstract
Prevalence of drug resistant C. glabrata strains in hospitalized immune-compromised patients with invasive fungal infections has increased at an unexpected pace. This has greatly pushed researchers in identification of mutations/variations in clinical isolates for better assessment of the prevailing drug resistance trends and also for updating of antifungal therapy regime. In the present investigation, the clinical isolates of C. glabrata were comprehensively characterized at a molecular level using metabolic profiling and transcriptional expression analysis approaches in combination with biochemical, morphological and chemical profiling methods. Biochemically, significant variations in azole susceptibility, surface hydrophobicity, and oxidative stress generation were observed among the isolates as compared to wild-type. The 1H NMR profiling identified 18 differential metabolites in clinical strains compared to wild-type and were classified into five categories, that include: sugars (7), amino acids and their derivatives (7), nitrogen bases (3) and coenzymes (1). Transcriptional analysis of selective metabolic and regulatory enzymes established that the major differences were found in cell membrane stress, carbohydrate metabolism, amino acid biosynthesis, ergosterol pathway and turnover of nitrogen bases. This detailed molecular level/metabolic fingerprint study is a useful approach for differentiating pathogenic/clinical isolates to that of wild-type. This study comprehensively delineated the differential cellular pathways at a molecular level that have been re-wired by the pathogenic clinical isolates for enhanced pathogenicity and virulence traits.
1. Introduction
Fungal infections are among the leading cause of mortalities across the world contributing to nearly 1.4 million deaths every year in hospitalized patients.1 Among different fungal genus, the incidences of Candida species related infections in immune-suppressed patients are highest in the clinical setup. The distribution frequency of Candida species varies immensely with geographical area.2 Candida albicans is the most prevalent causative agent of candidiasis, followed by other non-albicans Candida species (NACs), C. glabrata, C. tropicalis, C. krusei and C. parapsilosis. Out of all the NAC species, the infections caused by C. glabrata dominate the list of drug-resistant NACs.3–5 C. glabrata accounts for 15–25% invasive candidiasis cases with 40 to 60% mortalities and/or morbidities.2 In U.S. and North-western Europe, C. glabrata accounts for the second highest number of blood-stream infections (BSIs) in ICU patients.6 Furthermore, in women with vulvovaginal candidiasis the prevalence of C. glabrata is exceptionally high, with nearly 40% chance of recurrence.7,8
Phylogenetically, C. glabrata is closely related to Saccharomyces cerevisiae and distantly to all Candida species. Such an evolutionary divergence bestowed C. glabrata with different morphogenetic and pathogenic traits, thus favoring its escape from the host immune response.6,9 Additionally, C. glabrata expresses cell surface adhesins belonging mainly to the EPA (epithelial adhesin) family. These surface proteins enable C. glabrata cells to adhere and colonize the host epithelial and endothelial tissue surfaces.4 After colonization, C. glabrata initiates the formation of a multi-cellular architecture of adhered cells enclosed in a self-secreted polymeric matrix, known as a biofilm. During biofilm development in C. glabrata, the expression of stress response proteins gets upregulated while that of glycolytic proteins becomes downregulated, and imparts higher antifungal resistance.10,11
Inherently, C. glabrata is less susceptible to azole drugs, and with time they are becoming resistant to other antifungals classes such as echinocandins and polyenes.1 The prime resistance mechanisms behind antifungal therapy failure include an excessive expression of efflux pumps, alteration in drug targets, stress responses, and mutations at the genomic level.12–15 The key factors responsible for their increased pathogenicity, and evolution of antifungal resistance mechanism are the environmental stresses (antifungal exposure) and growth conditions. These mutations accumulated at genetic level are difficult to identify and revert, and become evident only when pathogens depict physiological and metabolic variations.16 Such changes effectively facilitate survival, growth and propagation of fungal pathogens under unfavorable conditions. The strains isolated from diseased patients are far different from laboratory wild-type strain in several aspects related to virulence, drug susceptibility and genetic makeup.17 Henceforth, comprehensive identification and characterization of these clinical isolates at the molecular level is essential for pharmacological target identification.
For dissecting the molecular level variations in clinical isolates, comprehensive studies comprising of integrated omics technologies such as metabolomics, and transcriptomics supplemented with chemical profiling, morphological and biochemical studies are quintessential.18–20 Among omics approaches, metabolic profiling details the occurrence/availability of low-molecular weight metabolites that are intermediates of various biochemical processes within the cell. These intracellular metabolites present in the cell at a particular time reflect the cellular response with respect to genetic and environmental changes.21,22 Metabolomics has been used for biomarker identification, assessment of environmental stress, comparison among mutants, toxicology, drug discovery etc.23,24
The current study attempts to characterize molecular level differences in clinical isolates from that of wild-type strain of C. glabrata. For this, a comprehensive approach of chemical profiling, metabolomics and gene expression studies combined with morphological, physical/topological and biochemical techniques have been applied to unravel the differences in their molecular makeup. The azole susceptibility and hydrophobicity data suggested extreme variations between wild-type and clinical isolates of C. glabrata. Further chemical and metabolic profiling investigations highlighted that the clinical isolates differ from wild-type C. glabrata in their glucose metabolism, fatty acid and sterol biosynthesis, and amino acids biosynthesis. Hence, these identified pathways/biomarkers identified through the metabolic fingerprints could be potentially considered as a target for formulating next-generation antifungal agents.
2. Experimental section
2.1 Strains and growth conditions
Candida glabrata wild-type strain (C0, MTCC 3019) and four clinical isolates C1, C2, C3 and C4 used in this study were obtained as a gift from Dr. Navin Kumar, Graphic Era University, Dehradun, India.25 All the strains were maintained in YPD media (yeast extract 1%, dextrose, peptone and agar, 2% each) in laboratory under aseptic conditions at 37 °C. For the azole susceptibility determination, RPMI 1640 media MOPS buffered containing 0.2% sodium bicarbonate was used. Unless otherwise specified, all the chemicals used in this study were purchased from Sigma-Aldrich, HiMedia and SRL, India.
2.2 Susceptibility against azoles
The susceptibility of C. glabrata to azoles (fluconazole and miconazole) was determined by following the both micro-dilution, CLSI guidelines.26 Briefly, overnight culture of C. glabrata in YPD broth was obtained by incubation for 18 hours at 37 °C and 180 rpm. For log phase, overnight culture was diluted with fresh YPD and incubated for 2–3 h at 37 °C and 180 rpm. The log phase cells were washed and resuspended in RPMI 1640 medium to a final concentration of 2.5 × 103 cells per mL. In each well of microtiter plate (MTP), two-fold dilution of drug in the concentration range of 0.125 to 250 μg mL−1 was prepared in RPMI 1640, and to this 100 μL of cell suspension was added to make a final volume of 200 μL. The plates were incubated at 37 °C for 48 h, and after the incubation period, the cells from plate were spotted on the surface of YPD agar media plates. After 18 h of incubation at 37 °C, the growth on agar media plates was recorded by photography.27
2.3 Growth kinetics
Growth kinetics of all the strains was studied by method described by Tan et al. with some modifications.28 Fifty millilitres of YPD broth was inoculated with log phase cells obtained as mentioned above at 0.4 OD600 and incubated a 37 °C at 180 rpm. After the time intervals of 3, 6, 9, 12, 18 and 24 h, 200 μL of culture was collected and cell density was measured at 600 nm using UV-visible spectrophotometer (Biospectrophotometer, Eppendorf). At the end of 24 h, the wet biomass was collected and weighed by centrifuging the 100 mL culture at 6000 rpm.
2.4 Cell morphology analysis
2.4.1 Light microscopy. The overnight grown C. glabrata cells were re-inoculated into fresh YPD in the morning for 2 h at 37 °C, 180 rpm to attain log phase. The cells thus obtained were harvested in phosphate buffered saline (PBS, pH 7.0) by centrifugation at 6000 rpm for 5 min. Cells were re-suspended in PBS at a density of 1 × 103 cells per mL, and visualized under light microscope (EVOS-FL, Advanced Microscopy Group, Mill Creek, WA) at 60× magnification. The images from different fields were captured and cell size along the major ellipsoid axis was determined using ImageJ software.
2.4.2 Scanning electron microscopy. Magnified structure of C. glabrata cells were observed by field emission scanning electron microscopy (FE-SEM). The log phase cells were washed with PBS, and cell suspension of approximately 1 × 103 cells per mL was placed on a piece of glass slide (1 cm2), air dried, and fixed with 2.5% glutaraldehyde at 4 °C for overnight.29 The fixed specimen was washed with PBS and dehydrated using gradient of ethanol. After dehydration, the specimen was gold sputtered and visualized under scanning electron microscope (Carl Zeiss, Germany) at 15 kV, and magnification of 5000× and 25
000×.
2.4.3 Surface charge analysis (zeta potential). For surface charge determination, the overnight C. glabrata cells were grown for 2 hours to attain log phase and harvested in PBS as described in light microscopy studies. Cell suspension was diluted to approximately 103 cells per mL in PBS. One millilitre of the suspension was used to determine zeta potential by Zetasizer instrument (Malvern, UK).30
2.5 Biochemical characterization
2.5.1 Cell surface hydrophobicity. Cell surface hydrophobicity (CSH) was estimated using log phase C. glabrata cells that were grown in YPD media for 2 h at 37 °C.29 After incubation, cells were washed with PBS and resuspended in 3 mL sodium phosphate buffer (pH 7.2) at an OD600 of 0.4–0.5 (A1), followed by addition of 400 μL of hexadecane and mixed vigorously. Finally, the cell density in the aqueous phase was determined by measuring the absorbance at 600 nm (A2). The hydrophobicity index (HI) was calculated by the following eqn (I). |
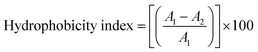 | (I) |
2.5.2 Ergosterol content. Ergosterol content was analysed by growing C. glabrata cells in YPD broth for 24 h.31 After incubation, cells were harvested, washed with distilled water and biomass was quantified. Cells were treated with 3 mL of lysing agent (25% alcoholic KOH) and incubated for 1 h at 85 °C in water bath. For extraction of sterols, the 1
:
3 mixture of water and n-heptane was added to the lysed cell suspension followed by vortexing for 3 min. The n-heptane layer was collected in glass bottles and kept at −20 °C. Before scanning, 100 μL of absolute ethanol was added to 20 μL of n-heptane layer and scanned from 230 to 400 nm. The ergosterol concentration was estimated by following eqn (II): |
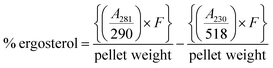 | (II) |
where, 290 and 518 are the molar absorptivity of crystalline ergosterol and 24(28) dehydroergosterol respectively; and F is dilution factor.
2.5.3 Intracellular reactive oxygen species (iROS) generation. The ROS generation in C. glabrata cells was studied by dichlorofluorescine diacetate (DCFDA) assay.32 The log phase cells were added in the wells of black well MTP in triplicates with 10 μM DCFDA. The plate was incubated in dark at 37 °C for 30 min. For ROS estimation, the absorbance of cells in MTP wells was recorded at an excitation of 485 nm and an emission of 520 nm using multimode microtiter plate reader (BIOTEK, Agilent).
2.6 Chemical profiling
Chemical profiling of C. glabrata cells was performed using different cellular pathway stressors by plate spot assay.18 Briefly, the cells were grown to log phase and diluted in PBS to make dilutions of 105, 104, 103, 102 and 101 cells per mL. The YPD agar media plates containing different stressors at sub-inhibitory concentrations were prepared. Ascorbic acid (AA), sodium chloride (NaCl), calcium chloride (CaCl2), cobalt chloride (CoCl2), congo red (CR), miconazole (MCZ), sodium nitroprusside (NaNO), nystatin (Nys), sodium dodecyl sulphate (SDS) and tert-butyl-hydroperoxide (TBO) were used as stressors. On the surface of each plate 4 μL of cell suspension was spotted, air dried and incubated in aerobic conditions for about 18 h at 37 °C. After incubation, the plates were photographed against black background.
2.7 Metabolic profiling
2.7.1 Extraction of metabolites and sample preparation for NMR spectroscopy. Metabolite extraction was done with moderate alterations in the method as described by Arora et al.33 About 100 μL of the log phase cells (six replicates) were inoculated in 100 mL YPD media and allowed to grow for 24 h at 37 °C 180 rpm. The cells were harvested by centrifugation (6000 rpm) at 4 °C. Pellets were resuspended in distilled water to remove excess media components. The cells were re-pelleted down and lyophilized. Fifty mg of the dry biomass of each replicate was then crushed in the presence of methanol and liquid nitrogen for three cycles to extract metabolites. The methanolic extract was centrifuged at 10
000 rpm to remove cell debris and allowed to freeze dry. For NMR spectra recording, sample was prepared by dissolving the freeze-dried extract in 550 μL deuterium oxide (D2O) containing 5 μL of trimethylsilyl-propionic acid (TSP) as a reference standard.
2.7.2 NMR spectral recording and processing. One dimensional proton NMR spectra were recorded on Bruker Avance 500 MHz instrument at 298 K with triple resonance probe (TXI). The Carr–Purcell–Meiboom–Gill pulse sequence (cpmgpr1d) was used with pre-saturation of water peak. The acquisition parameters were set as follows: spectral width 20 ppm, number of scans 832, total relaxation time of 4 seconds and free induction decay (FID) size of 65
536.After recording, the spectra were processed for Fourier transformation and manual correction for phase and baseline by employing Bruker's Topspin 4.0.7 software, and calibrated to reference standard TSP (0.0003 mM). Peak assignment was done by using Chenomx NMR Suite 8.4. The peaks were assigned by referring the Chenomx 500 MHz compound library and yeast metabolome database (YMDB 2.0).34–36
For binning, the water suppression region δ (4.68 to 4.88 ppm) was removed from the spectra, and the binning was done from δ (0.6 to 9.4 ppm) with δ 0.01 ppm as bin size using Chenomx processor tool. Bin file so obtained was employed for statistical analysis on MetaboAnalyst 4.0.37 The binned data were analyzed by two discrimination analysis tools, principal component analysis (PCA) and partial least squares-discriminant analysis (PLS-DA).38
The metabolites of discriminatory significance were identified by variable importance on projection (VIP) score derived from PLS-DA model. The variables identified from VIP scores were also assessed for statistical significance by one-way analysis of variance (ANOVA) followed by Tukey's post HSD test with p < 0.001. For comparison between groups, fold change analysis was performed. The fold change of two or more was considered for analysis.39 The distribution of metabolites among all the groups responsible for group discrimination was visualized by box plots. The values represented are normalized concentrations of individual metabolites with discriminatory significance. The pattern of significantly altered level of metabolites with VIP score more than 2.0 among all groups was considered for constructing the heatmap.40
2.8 Transcription analysis
Transcription analysis of specific genes was performed for C. glabrata wild-type (C0), C3 and C4 strains. RNA was extracted from log phase cells using RNA extraction kit (RNeasy Kit, Qiagen). Further, 100 ng of RNA was used for synthesis of cDNA using Verso cDNA synthesis kit (Applied Biosystems). The primers required for qRT-PCR were customized from Macrogen Inc. (South Korea) (Table S1†). The transcription analysis was performed using SYBR green method (Applied Biosystems, Vilnius, Lithuania). The obtained cycle threshold (CT) values of target genes were normalized with that of housekeeping gene (ACT1) and fold change was calculated by ΔΔCT method using formula 2−ΔΔCT.31
2.9 Statistical significance
All experiments were performed in triplicates, and the values presented were the average values with standard deviation, obtained from three different observations. For NMR studies, six replicates of each group were considered. Student's t-test was used for the statistical analysis, and a value of P < 0.05 was considered statistically significant (*), P < 0.01 as highly significant (**) and P < 0.001 as extremely significant (***).
3. Results
Investigations from geographically diverse areas on drug susceptibility of clinical isolates of Candida are required for understanding the variations and formulating an effective antifungal therapy for health practitioners. In the present study, the susceptibility of the clinical strains obtained from Uttarakhand, India were comprehensively characterized for their drug resistance mechanisms at the molecular level by using a multitude of methods to dissect the drug susceptibility characteristics, morphological and biochemical features and metabolite/gene expression profiles.
3.1 Analysing the differences in drug susceptibility, morphological and biochemical characteristics of C. glabrata and its clinical strains
For characterization of clinical isolates, azole susceptibility study was performed by following CLSI micro-broth dilution guidelines. The lowest MIC90 value of fluconazole and miconazole was recorded for C0; 25 μg mL−1 and 0.78 μg mL−1, respectively (Table 1). The MIC90 value of fluconazole was 225 μg mL−1 for both C1 and C4 while it is more than 250 μg mL−1 for C2 and C3. Likewise, clinical isolates showed variations in susceptibility to miconazole; miconazole MIC90 value for C1, C2 and C3 was 3.12 μg mL−1, and 1.56 μg mL−1 for C4. The MIC90 values of azoles depicted the differential susceptibilities of clinical strains to that of C. glabrata wild-type. In order to understand the differences in drug susceptibilities of C1–C4 to that of C0, the growth rate of all the strains was studied in YPD broth by taking absorbance at 600 nm. After 24 h of growth study, it has been observed that all the clinical isolates have overlapping growth rate with each other (Fig. S1†). All studied strains of C. glabrata showed no differences in the growth pattern till the first 12 h of incubation. The differences in growth rate between C0 and C1–C4 became significant during 18 to 24 h of incubation. The wet biomass in 100 mL of cultures at 24 h was estimated to be C0 = 855 ± 35 mg, clinical isolates = 514 ± 35, 639 ± 4, 558 ± 9 and 610 ± 11 mg for C1–C4 respectively. Thus, a significant reduction of biomass was observed in the clinical isolates as compared to C0 (Fig. 1A).
Table 1 Susceptibility of C. glabrata strains for azoles. C0 = C. glabrata wild-type strain; C1–C4 = clinical isolates
Strain |
MIC90 (μg mL−1) |
Fluconazole |
Miconazole |
C0 |
25 |
0.78 |
C1 |
225 |
3.12 |
C2 |
>250 |
3.12 |
C3 |
>250 |
3.12 |
C4 |
225 |
1.56 |
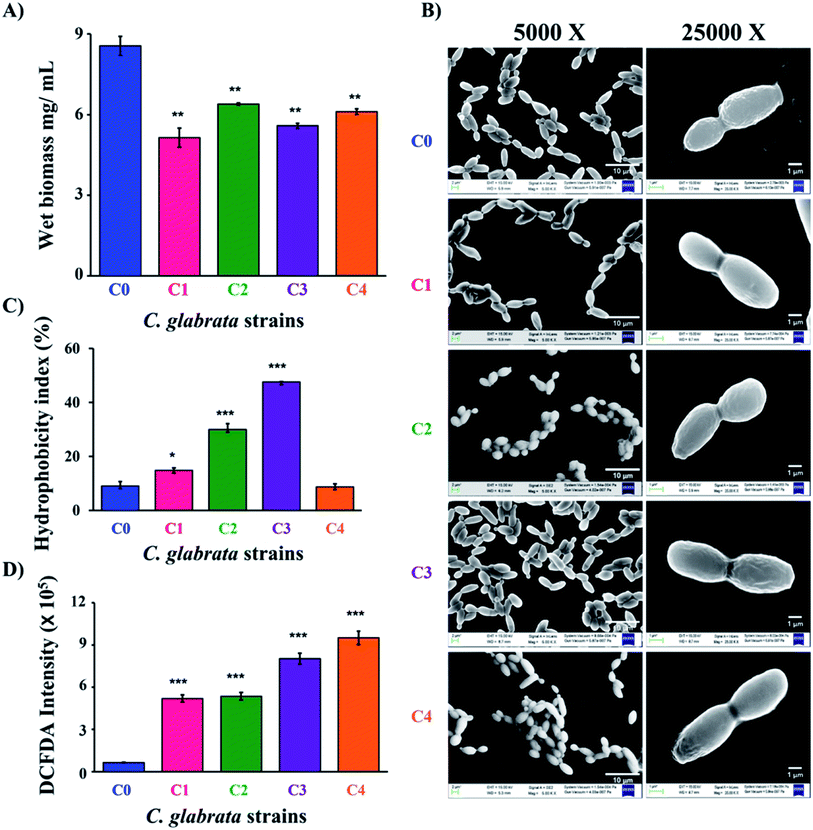 |
| Fig. 1 Morphological and biochemical studies of C. glabrata strains. (A) The wet biomass of C0 to C4 strains was estimated after 24 h of incubation at 37 °C in YPD media (mg mL−1 ± SD, n = 3). (B) Field emission scanning electron microscope (FE-SEM) images of C. glabrata cells (C0–C4) at a magnification of 5000× and 25 000×. (C) Cell surface hydrophobicity of C. glabrata strains measured by two-phase system in terms of hydrophobicity index (%). (D) intracellular reactive oxygen species (iROS) generation in C. glabrata strains was spectrophotometrically estimated using DCFDA. C. glabrata wild-type strain (C0), and clinical isolates (C1–C4). | |
To further differentiate the C. glabrata strains, the morphological and topological parameters (cell size, morphology) were investigated. Initially, the difference in cell size of clinical isolates was studied by light microscopy, where 40 different fields of the slide were clicked at 40× and then the size of 100 cells were randomly measured using ImageJ software along long axis. The mean of 100 cell size value was calculated and the values found for C0, C1, C2, C3 and C4 cells were 6.07 ± 0.74, 7.37 ± 0.73, 6.60 ± 0.63, 6.85 ± 0.77 and 5.41 ± 0.65 μm, respectively (Fig. S2 and S3A†). The data evidenced for no significant difference in the obtained mean size (5–7 μm) for all the measured strains. Further, FESEM was performed to identify any distinct surface morphological features, if exist on the outer surface of C. glabrata and its clinical isolates (Fig. 1B). In coherence to this, the FESEM images of cells have not shown any considerable variation in cell shape and surface of clinical isolates (C1–C4) as compared to C. glabrata wild-type (C0).
Further, to characterize the C. glabrata strains biochemically, their cell surface characteristics in terms of cell charge (zeta potential) and cell surface hydrophobicity (CSH), ergosterol content and intracellular reactive oxygen species (iROS) generation was studied. In fungal pathogens, cell surface charge and hydrophobicity are very critical factor in deciding their virulence and colonizing ability.41 CSH is a macroscopic feature and is conferred by surface proteins and level of glycosylation.42,43 The hydrophobicity index (HI) of all clinical strains except C4 was found to be significantly higher than wild-type strain C0 (Fig. 1C). C3 have the highest HI value followed by C2 and C1. The difference in HI value of C4 and C0 was insignificant. The high HI value of clinical strains reflects their more pathogenic trait over C0. Another way of identifying cell surface variations among cells is the cell surface charge that is measured in terms of zeta potential. The zeta potential analysis revealed the mean outer surface charge of C2 was lowest negative (−13.6 ± 4.83 mV) and that of C0 was highest negative (−22.4 ± 5.97 mV). Rest of the variants had similar charges (−17.0 ± 6.17, −20.0 ± 8.08, −21.9 ± 7.67 mV respectively for C1, C3 and C4), but the associated high standard deviation made the differences insignificant (Fig. S3B†).
Ergosterol content in fungal cell membrane is also an important determinant of pathogenicity due to its prime role in maintaining cellular integrity.44 The spectrophotometric estimation of ergosterol in C. glabrata strains revealed no considerable differences in their ergosterol content (Fig. S3C†). Other than cell surface and membrane components, intracellular oxidative stress generation is also responsible for the altered pathogenic trait of C. glabrata. Therefore, intracellular reactive oxygen species (iROS) generation of all chosen C. glabrata strains (C0–C4) was estimated. The highest amount of iROS has been recorded in C4 and C3 followed by C1 and C2 (Fig. 1D). The measured iROS was 5 to 7-fold more in clinical isolates as compared to C0. Such extremely significant enhancement of iROS is a potential indicator of their clinical and virulent nature, as increase in iROS aids in morphological transitions that ultimately leads to pathogenicity.45 Collectively, the physical and the biochemical data evidenced that these clinical isolates could be significantly different from wild-type C. glabrata in molecular level, which is indeed reflected in their drug susceptibility and biochemical phenomenon, without any change in their phenotypic features.
3.2 Chemical profiling of C. glabrata strains
Chemical profiling was performed to discriminate C. glabrata strains on the basis of alterations in their stress responsive regulatory pathways. The different stressors used in the study target specific cellular pathway like, osmotic stress (NaCl and CaCl2), hypoxia (CoCl2), cell wall (CR), ergosterol biosynthesis pathway (MCZ), cytotoxicity (NaNO), cell membrane (Nys and SDS) and oxidative stress (TBO). In the unstressed plate, the colony size of clinical isolates was bigger than C0, and colony texture was rough as compared to C0 (Fig. 2). The increased colony size is indicative of better adaptability of cells to the environment, and thus the growth. The growth of C0 to C4 was found unaffected in presence of AA, CoCl2, NaCl, and TBO as compared to their growth on control YPD plate suggesting for no significant alterations in these pathways. However, the growth gets compromised for all C0 to C4 in the presence of CaCl2, CR, NaNO, and SDS as compared to untreated (YPD) control indicating that the cellular pathways corresponding to these stressors have similar functioning in all the chosen strains. Interestingly, the varying level of growth defect was observed in all strains against the cell membrane (Nys) and ergosterol biosynthesis (MCZ) pathway stressors that were actually responsible for increased virulence of clinical isolates over wild-type C. glabrata. In presence of MCZ, the highest growth was observed in C3 while in Nys, C2, C3 and C4 exhibited similar growth. C0 and C1 were unable to grow in presence of Nys. These observations highlighted that the clinical isolates differ from wild-type in cell membrane and ergosterol biosynthesis. Henceforth, to decipher the variations in clinical isolates over wild-type strain at molecular level, detailed metabolic profiling and transcription/gene expression studies were carried out as discussed below.
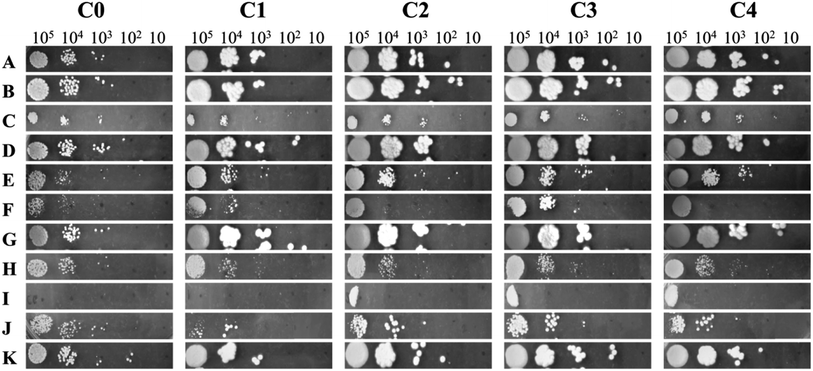 |
| Fig. 2 Chemical profiling of C. glabrata strains to different stressors added into YPD agar media by plate spot assay. The growth sensitivity of the cell towards different stressors is compared to the growth in absence of stressor. The stressors used are known to activate different stress-related physiological pathways in the cells. (A) Yeast peptone dextrose media; (B) ascorbic acid; (C) calcium chloride; (D) cobalt chloride; (E) Congo red; (F) miconazole; (G) sodium chloride; (H) sodium nitroprusside; (I) nystatin; (J) sodium dodecyl sulphate; (K) tert-butyl-hydroperoxide. C. glabrata wild-type strain (C0), and clinical isolates (C1–C4). | |
3.3 NMR based metabolic profiling of C. glabrata strains
Currently, mass spectroscopy (MS) and nuclear magnetic resonance (NMR) spectroscopy are being used for metabolomics. Out of these, NMR offers additional advantages of no specific sample preparation is needed, and the lyophilized extracts are directly used for analysis.46,47 Moreover, the technique being non-invasive, the samples can be safeguarded for other complementary analysis. To establish the variations in clinical strains as compared to C. glabrata wild-type at molecular level, NMR based metabolomic profiling was performed. The 1D proton NMR spectra (1H NMR) of intracellular metabolites for all C. glabrata strains (C0–C4) were recorded, and the obtained resonance assignments were identified and cross verified with the Yeast Metabolome Database (YMDB). A total of 83 metabolites were identified in the samples C0–C4 (Fig. 3A). Broadly, these compounds were categorized based on their biochemical role; nitrogen bases (15), amino acids (24), organic acids (22), sugars (13), vitamins and coenzymes (4), choline derivatives (2), alcohols (2), and amides (1). The resonance assignments of all the obtained metabolites/compounds were presented in Table S2†.
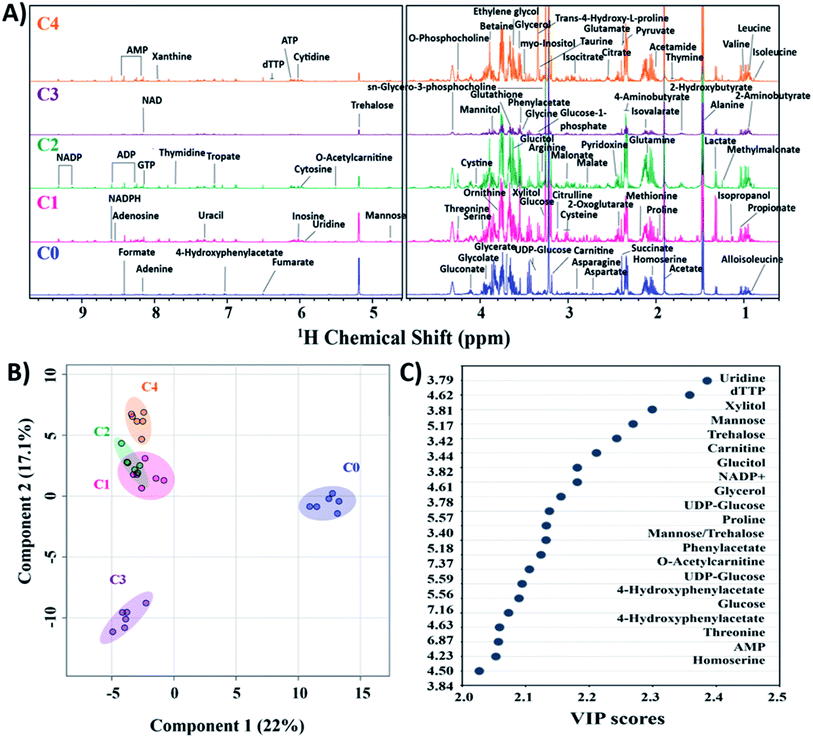 |
| Fig. 3 Metabolomics analysis of C. glabrata strains. (A) 1H NMR spectra of intracellular metabolites of C0–C4 in YPD media. All identified compounds are indicated with their respective peaks at particular chemical shift (ppm) as confirmed from yeast metabolome database. (B) Partial least squares discriminant analysis (PLS-DA) scores plot indicating group discrimination among C. glabrata strains. (C) VIP scores plot showing variables of importance in class discrimination by PLS-DA model. The identified compounds are mentioned at particular ppm values. C. glabrata wild-type strain (C0), and clinical isolates (C1–C4). | |
To confirm the authenticity of the observed differences and class discrimination across the studied strains (C0–C4), multivariate principal component analysis (PCA) and partial least squares discriminant analysis (PLS-DA) were performed. Both these statistical analyses provide an idea about general overview and pattern of clustering within the data. The clustering of six biological replicates of each clinical isolate (C1–C4) was statistically different from wild-type as observed from PCA and PLS-DA (Fig. 3B, and S4†). From both PCA and PLS-DA, it is evident that the C1–C4 groups besides showing significant difference from C0, these clinical isolates organised themselves into two cluster where C1, C2 and C4 constitute one cluster and C3 alone form second cluster. PCA scores plot determined the 39% contribution of first two principal components in defining variability among all five groups. The PLS-DA score plot also showed 22% and 17.1% contribution by first two components in class discrimination (Fig. 3B). As the PLS-DA model is prone to overfit the data, it is essential to strictly validate the model to verify the statistical significance. The performance of PLS-DA model was validated by 10-fold cross-validation method, which evaluates the classification accuracy by employing top five components. The cross-validation parameters, predictive ability (Q2), goodness of fit (R2) and accuracy for top five components was estimated to be 0.95, 0.98 and 0.86 respectively, thus validating the observed results (Fig. S5†).
The metabolites highly influencing the classification among groups were identified by special feature of PLS-DA model namely variable importance in projection (VIP). The model determines VIP score of metabolites based on their contribution to the variance among identified groups. From the VIP plot, a total of 18 assigned compounds were found to be the most critical variables for group discrimination of C0–C4 (Fig. 3C).
The relative distribution of these most significant variable metabolites in clinical isolates (C1–C4) to that of the wild-type (C0) has been depicted in box plots to elucidate the significant variances (Fig. 4). From the box plots, uridine, xylitol, O-acetylcarnitine, UDP-glucose, phenylacetate, 4-hydroxyphenylacetate and NADP+ followed the distribution representing the three clusters formed by PCA and PLS-DA scores plot, thus different concentrations in C0, C3 and rest of the clinical isolates in C1, C2 and C4 (where the difference in concentrations was insignificant). The concentrations of mannose, trehalose, carnitine, glucose, glycerol, threonine and homoserine in C0 were significantly different from all the clinical isolates. Glucitol concentration was highest in C0, followed by C3 and C4, and lowest in C2. Proline levels were higher in C0 and C1, followed by C2, and lowest in C3 and C4. In line to this, the heatmap analysis also revealed that the concentration of all the metabolites except phenylacetate, 4-hydroxyphenylacetate and threonine were higher in C0 than other clinical isolates (Fig. S6†). In addition, the heatmap highlighted variations among clinical isolates, where C1 contained higher levels of glucitol, carnitine, trehalose, and proline; C2 showed higher levels of 4-hydroxyphenylacetate, O-acetylcarnitine, UDP-glucose, glucose, and threonine; C3 contained higher levels of AMP, xylitol, and uridine; whereas the AMP level was lowest in C4, and all other metabolites in C4 were found to be moderately deviated from rest of the clinical isolates. Henceforth, the heat map analysis suggests that the metabolic profile of all the clinical strains could be significantly different from that of the lab strain.
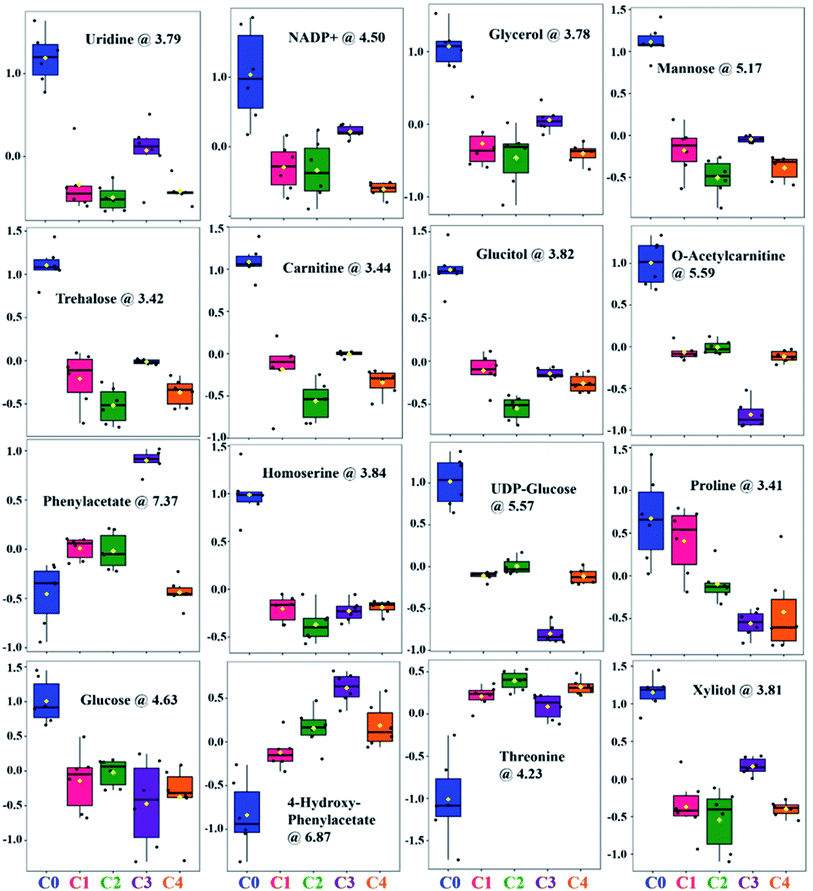 |
| Fig. 4 Box and whisker plots showing relative normalized concentrations of important metabolites selected on the basis of VIP score. C. glabrata wild-type strain (C0), and clinical isolates (C1–C4). | |
It is evident from all the obtained statistical analysis/box plots that the groups C1, C2 and C4 have similar traits. Henceforth, considering the overall changes observed in biochemical studies, chemical profiling and metabolite analysis of these three groups, C4 has been chosen as a representative member of the third cluster. Therefore, the fold change analysis was performed with three distinctly identified groups namely C0, C3 and C4 based on clustering pattern of PCA/PLS-DA analysis. The clinical strains C3 and C4 data was compared with C0 using one-way ANOVA for fold change (FC) analysis and has been represented as log
2 (FC). The fold change values of all the differentially identified 18 metabolites for C3 and C4 groups to that of C0 is given in Table 2. These metabolites were classified according to their chemical nature and biochemical role in metabolism into five categories, these include sugars (7), amino acids and their derivatives (7), nitrogen bases (3) and coenzymes (1). All of the seven sugar moieties involved in group differentiation were found to be downregulated in C3 and C4; four of which were simple six carbon (6C) sugars (glucose, UDP-glucose, glucitol and mannose) that are inter-convertible and function mainly as source of energy within the cell. Reduction in their intracellular sugars concentration in stationary growth phase indicates that clinical isolates consume energy sources faster, and growth reaches to saturation soon as compared to C0. Also, the quantity of xylitol (5C sugar), a substrate of purines and pyrimidines was found reduced in clinical isolates resulting in shortage of nucleotides for DNA replication, and thus cell division. On similar lines, reduction in intracellular fraction of four out of five amino acids (carnitine, O-acetylcarnitine, proline, and homoserine) in C3 and C4 along with increase in threonine and metabolic derivatives of phenylalanine (phenylacetate and 4-hydroxyphenylacetate) implies excessive utilization of these essential nutrients for cell growth and replication by the clinical isolates.
Table 2 Significantly altered intracellular metabolites identified at particular ppm value in the 1D 1H NMR spectra of C. glabrata wild-type C0, and clinical isolates (C3 and C4). The fold change is expressed as Log
2 (FC) which denotes metabolites varied at least 2-fold as compared to C0. Peak types: s-singlet, d-doublet, t-triplet, q-quartet and m-multiplet
S. no. |
Compound |
Assignment |
ppm (δ) |
Peak type |
Log 2(FC) C3 |
Log 2(FC) C4 |
1 |
Uridine |
C14H2 |
3.79 |
q |
−2.52 |
−3.77 |
2 |
dTTP |
C5H |
4.62 |
d |
−3.01 |
−3.46 |
3 |
Xylitol |
C7H/C3H |
3.81 |
m |
−2.27 |
−3.57 |
4 |
Mannose |
C2H |
5.17 |
d |
−2.42 |
−3.09 |
5 |
Trehalose |
C5H/C11H |
3.42 |
q |
−2.34 |
−3.04 |
6 |
Carnitine |
γ-CH2 |
3.44 |
m |
−2.28 |
−2.95 |
7 |
Glucitol |
C9H |
3.82 |
m |
−2.39 |
−2.60 |
8 |
NADP+ |
C30H |
4.61 |
m |
−2.90 |
−1.87 |
9 |
Glycerol |
C2H |
3.78 |
m |
−2.18 |
−3.17 |
10 |
UDP-glucose |
C2H |
5.57 |
q |
−3.78 |
−2.39 |
11 |
Proline |
C2H2 |
3.40 |
m |
−2.73 |
−2.16 |
12 |
Phenylacetate |
C3H/C5H |
7.37 |
m |
2.57 |
2.14 |
13 |
O-Acetylcarnitine |
C5H |
5.59 |
m |
−3.87 |
−2.27 |
14 |
4-Hydroxypenylacetate |
C2H/C6H |
6.87 |
m |
2.51 |
1.93 |
15 |
Glucose |
C2H |
4.63 |
d |
−2.97 |
−3.00 |
16 |
Threonine |
C6H |
4.23 |
m |
1.84 |
2.28 |
17 |
AMP |
C4H |
4.50 |
q |
−2.54 |
−4.46 |
18 |
Homoserine |
C4H2 |
3.84 |
q |
−2.19 |
−2.12 |
Among nitrogen bases, the cellular levels of uridine and dTTP, the components of RNA and DNA respectively, were declined in both C3 and C4. Additionally, adenosine monophosphate (AMP), a regulator of intracellular signalling and a component of nucleic acid was also decreased in the clinical isolates. Nicotinamide adenine dinucleotide phosphate (NADP+) an important coenzyme involved in regulation of pentose phosphate pathway, nucleic acid and lipid synthesis was also observed to be depleted. Furthermore, the disaccharide trehalose, an essential component of cell wall was also found to be reduced indicating the differential cell wall characteristics of clinical isolates, C3 and C4.
The interplay between these differential metabolites observed in C3–C4 to that of C0, which can potentially regulate certain cellular pathways has been depicted using metabolic pathway diagram (Fig. 5). From the schematic, it can be observed that how the central glycolysis cycle can consume various interconvertible hexose sugars (glucose, mannose, glucitol, UDP-glucose) and convert them into pentose sugars, disaccharides, nitrogen bases, amino acids and their derivatives. Considering the variations in these metabolite concentrations, the corresponding genes that regulate these cellular pathways have been selected and investigated using gene expression studies.
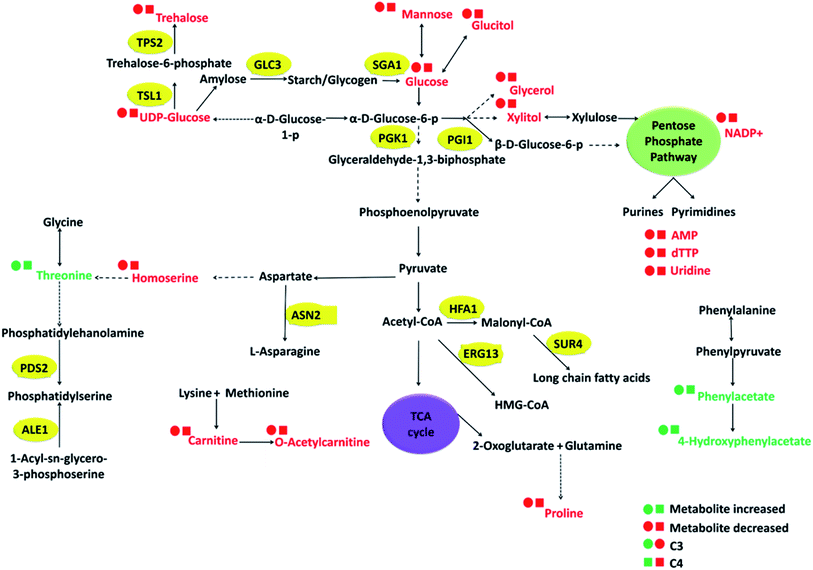 |
| Fig. 5 Flux analysis of highly influential metabolites in group discrimination in C. glabrata clinical isolates C3 and C4 as compared to the wild type strain (C0). The metabolites identified from NMR spectra are displayed in coloured text. Circular and square dots represent concentration in C3 and C4 respectively with colour codes green and red for up and down-regulation respectively. The genes selected for transcriptional analysis are displayed in yellow ovals. | |
3.4 Transcription analysis of C. glabrata strains
Based on metabolic and chemical profiling observations, 16 genes belonging to cell fitness, fatty acid/sterol biosynthesis, carbohydrate metabolism, cell wall integrity, and ABC transporters along with housekeeping gene (ACT1) were studied in C3 and C4 with respect to C0 (Fig. 6). Several gene names and their functions mentioned here were taken from Saccharomyces genome database (SGD) as majority of C. glabrata ORFs are uncharacterized according to available data on Candida genome database (CGD). For cell fitness, the contribution of two genes (PDS2, ALE1) was studied, and found that PDS2 (phosphatidylserine decarboxylase activity) was down-regulated in both C3 and C4; while ALE1 (O-acyltransferase activity) was selectively up-regulated in C3 and down-regulated in C4 (Fig. 6). Fatty acid/sterol biosynthesis was another crucial pathway that was found different in clinical isolates as compared to C0. Three representative genes HFA1 (mitochondrial acetyl-coenzyme A carboxylase), SUR4 (fatty acid elongase) and ERG13 (3-hydroxy-3-methylglutaryl coenzyme A synthase) of this pathway were studied, and their expression has found to be reduced in both the clinical isolates, C3 and C4.
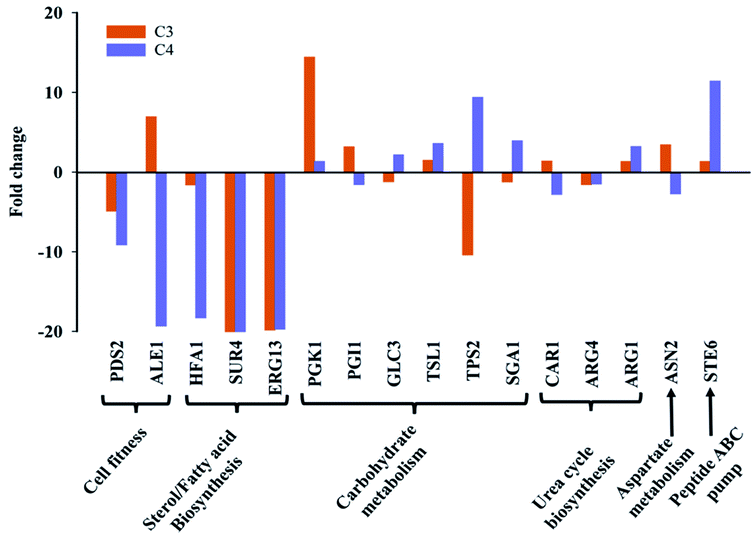 |
| Fig. 6 Transcription pattern of selected genes involved in biosynthesis of differential metabolites expressed as fold change in clinical isolates C3 and C4 as compared to the wild-type (C0). The fold change in comparative Ct was estimated by RT-PCR with SYBR green fluorescence assay. | |
Carbohydrate metabolism is the utmost essential pathway for cell survival and growth, as it is primarily responsible for fulfilling cell's energy demand as well as cellular and structural macromolecular synthesis. A total of six genes (PGK1, TSL1, PGI1, GLC3, TPS2, SGA1) corresponding to different carbohydrate metabolic pathways (glycolysis, trehalose pathway etc.) were undertaken. PGK1 (3-phosphoglycerate kinase) and TSL1 (alpha-trehalose-phosphate synthase) were up-regulated in both C3 and C4. The expression of PGI1 gene was selectively up-regulated in C3 and remain unchanged in C4, whereas SGA1 expression was increased in C4 and remain unchanged in C3. TPS2 (trehalose-phosphatase activity) gene expression was observed to be considerably down-regulated in C3 and up-regulated in C4 and the GLC3 (1,4-alpha-glucan branching activity) gene expression was unaltered in both strains (Fig. 6).
The expression level of three urea cycle genes; CAR1, ARG4, ARG1, and one aspartate metabolism gene ASN2 was studied, and no significant change in their expression was observed in C3 and C4 in comparison to C0. Interestingly, the transcript level of STE6 (ABC-type peptide transporter), was up-regulated in both clinical isolates, more intensely in C4 as compared to wild-type (Fig. 6). The major metabolic/molecular pathways found to be differentially regulated in C3 and C4 in comparison to wild-type are carbohydrate metabolism, sterol/fatty acid biogenesis, trehalose biosynthesis, peptide transporter, cell division and cell fitness. Majority of the differential gene expression results observed also affirms the metabolic and chemical profiling results described in the previous sections.
4. Discussion
4.1 Mechanistic insights into differential cellular pathway regulation of C. glabrata clinical isolates
In recent years, researchers have extensively investigated the clinical versions of pathogenic fungi at gene level and highlighted the variations in growth pattern, virulence, antifungal susceptibility, morphology and physiology of clinical isolates in comparison to wild-type counterpart.48–52 The phenomenon of natural selection plays key role in helping microbes to evolve themselves in order to survive and tolerate stress present inside the host.53 Therefore, time to time updation in our understanding about the fungal clinical strains is the most logical way of rationalizing the antifungal treatment practice. The present investigation attempts to trace the variation in C. glabrata clinical strain as compared to wild-type using a comprehensive approach of NMR-based metabolic profiling, transcriptional analysis and chemical profiling together with biochemical and morphological studies.
The very first step towards clinical isolate characterization is antifungal susceptibility testing in order to establish their response towards conventional drugs. As expected from their origin, the clinical isolates showed resistance or low susceptibility towards fluconazole and miconazole, and this is obvious that they have accumulated mutations as a result of prolonged antifungal exposure54 (Table 1). The lower susceptibility of clinical isolates to antifungals was also supported by observations of chemical profiling, where the clinical isolates exhibited enhanced grown in presence of miconazole and nystatin (Fig. 2). Such a varying susceptibility profile can be attributed to the prolong antifungal exposure in host niche, which altered their cellular responses in contrast to wild-type under azole stress. Indeed, the azole stress related mutation in ERG2 gene of clinical isolates of C. glabrata has been reported, and considered to be responsible for decreased antifungal susceptibility.55 Moreover, the fluconazole susceptibility of C. glabrata is pH, carbon source and loss of mitochondrial function dependent, which are essential and interesting to decipher in future studies.56
In the present investigation, despite the lower azole susceptibility and growth variations in clinical isolates, no considerable morphological and phenotypic variation was recorded. Therefore, it is imperative to dissect the molecular aspect of how these mutations regulates the cellular pathways for their role in virulence and survival. Henceforth, the study was aimed to present a comprehensive picture of altered signaling pathways. The current study evidenced variations in (A) cell growth and cell division; (B) cell wall and membrane components (ergosterol and trehalose biosynthesis, CSH), and (C) oxidative stress pathways of C3 and C4 that are possibly responsible for their increased virulence and has depicted in Fig. 7. The detailed information of all the identified metabolic pathways is presented in the following paragraphs.
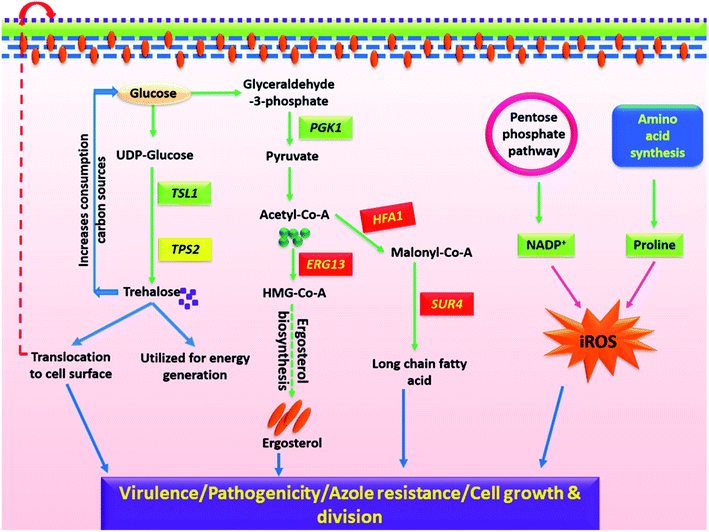 |
| Fig. 7 A plausible schematic representation of differential interplay between metabolites in clinical isolates of C. glabrata. Glucose playing a key role in biosynthesis of trehalose, ergosterol and long chain fatty acids which get translocated to cell wall and are involved in morphogenesis. Glucose provides precursor to pentose phosphate pathway and aids in regulation of NADP+/NADPH that along with proline boosts iROS generation. All these metabolic processes within the clinical isolate work in harmony to empower it with improved growth, viability, virulence and azole resistance. | |
The cell growth and division are the critical parameters to define the mortality rate of a Candida species. Growth rate, cell biomass and lag time together define cell growth.57 As rapidly as cells grow, the more intense is their infection rate. Several significantly altered metabolites of carbohydrate pathway in C3 and C4 are responsible for their enhanced cell growth and cell division, thus contributing to the virulence trait. Reduction in their intracellular sugar concentration in stationary growth phase (24 h) indicates that clinical isolates consume energy sources faster, and growth reaches to saturation soon as compared to C0. Also, the quantity of xylitol (5C sugar), a substrate of purines and pyrimidines was found reduced in clinical isolates resulting in shortage of nucleotides for DNA replication, and thus the cell division. On similar lines, reduction in intracellular fraction of five amino acids (carnitine, O-acetylcarnitine, proline, threonine and homoserine), nitrogen bases (TTP, uridine) in C3 and C4 highlights excessive utilization of these essential nutrients for cell growth and replication by the clinical isolates as also evident from their cell biomass (Fig. 1A).
Fungal cell wall and cell membrane is composed of several components where trehalose, ergosterol and level of protein glycosylation are crucial in determining the virulence and pathogenicity.58–61 Alterations in cell wall and membrane composition was firstly evident from increased CSH value of clinical isolates over wild-type (Fig. 1C). The clinical isolates also exhibited improved growth in presence of cell membrane stressor (Nyst) and ergosterol biosynthesis (MCZ) as compared to C0 (Fig. 2). Such differential features between the wild-type and C3/C4 were also noted in sterol/fatty acid pathway analysis, where variations in the transcript level of three genes (HFA1, SUR4, ERG13) involved in their biosynthesis was significantly modulated (Fig. 6), although the overall ergosterol content remain unaltered at the chosen time point. The gene expression pattern and chemical profiling observations (MCZ, Nyst) have strengthened the stress resistant trait in C3 and C4, and regulated ergosterol biosynthesis in harmony with other unidentified molecules. The alteration in ergosterol biosynthesis of C3 and C4 might have forced the cell to neutralize the changes by increasing sterol import and increasing ergosterol turnover via feedback mechanism.62 The participation of transcriptional regulator of azole drug efflux pumps (PDR1) in mediating drug resistance in C. glabrata was previously established using NGS technique, where a gain of 12 functional mutations in CgPDR1 facilitated the over-expression of drug efflux pumps.63
Trehalose is a non-reducing glucose disaccharide that serves as an alternative energy source and also has an important role in fungal stress response to temperature and oxidative stress.60,64,65 Trehalose was observed to be down regulated in C3 and C4 as compared to C0. This might be due to quick utilization of trehalose by C3 and C4 as carbon source for improving cell wall stress tolerance, as it provides resistance to Candida sp. upon macrophage attack.66,67 In line with the current observations, alterations in intracellular trehalose amount were also observed by Oliver et al., in C. glabrata isolates, suggesting that trehalose might possess some prominent role in clinical traits of these fungal pathogens.68 Interestingly, transcriptional data also evidenced for considerable modulation in TSL1 and TPS2, the significant players of trehalose biosynthetic pathway possessing the alpha-trehalose-phosphate synthase activity and trehalose-6-phosphate phosphatase activity respectively.69 Furthermore, the enzymes of trehalose pathway are long-known to be the contributors of fungal virulence and pathogenesis.70,71 As C. glabrata is the only yeast species that utilizes trehalose instead of sucrose and such a specific characteristic feature difference can act as a molecular marker for rapid identification of C. glabrata from other Candida species.72
Oxidative stress generation and its contributing pathways were one of the most altered processes identified in C. glabrata clinical isolates as compared to wild-type in biochemical assays. The observations were further supported by the reduced level of proline and NADP+ in metabolic profiling data. NADP+ has oxidoreductase property, and is associated with resisting oxidative stress and reactive oxygen species generation within cell.73,74 Further, proline is an amino acid that is known for reducing oxidative stress generation in the mitochondria by modulating cellular reducing potential.75 Reduced NADP+ and proline level facilitates increased oxidative stress generation that contributes toward pathogenic and virulent nature of clinical isolates. All these evidences threw light on the molecular level details of differential cellular pathways elucidating how these clinical isolates have adapted themselves to therapeutic formulations to modulate their responses by re-wiring the cellular pathways.
5. Conclusions
The present study has successfully identified some of the essential compositional, metabolic and transcriptional adaptations acquired by C. glabrata clinical isolates over wild-type that are attributed to their prolonged exposure to conventional drugs. NMR-based metabolomics has been successfully employed to distinguish clinical isolates from their wild-type counterpart, which although appear similar in physical trait. The present investigation highlights the modulated metabolic pathways in clinical isolates which are responsible for their virulent phenotype. Some important alterations identified in clinical isolates include trehalose pathway, ergosterol and fatty acid biosynthesis, oxidative stress generation, and cell membrane integrity related components. Among these identified metabolic pathways in clinical isolates, trehalose could be further explored for development of a novel therapeutic regime against antifungal resistant Candida clinical isolates, as it is most suitable target for antifungal development due to the absence of its complement in humans. Further, it is worth noting that the drug resistant mutations are not necessarily detectable at metabolite/transcript level as loss of heterogeneity, SNPs and aneuploidies are also responsible for drug resistance behaviours of clinical isolates.48,49 These possibilities also need to be taken into consideration while locating a point mutation in genetic material for better identification of drug resistant phenotype of clinical isolates.
Author contributions
KMP: conceptualization, project administration, supervision, writing—review and editing; HG and PG: investigation, formal analysis, visualization, writing—original draft; MK: formal analysis and visualization.
Conflicts of interest
There are no conflicts to declare.
Acknowledgements
HG acknowledges the All India Council for Technical Education (AICTE), New Delhi, for financial support under QIP scheme. PG acknowledges financial support from the Department of Biotechnology (DBT), Government of India, under DBT-RAship scheme. KMP acknowledges the receipt of support CRG/2018/001329 from SERB-DST from the Government of India. Authors are also thankful to the Institute Instrumentation Centre, IIT Roorkee, for microscopy analysis.
References
- K. R. Healey and D. S. Perlin, J. Fungi, 2018, 4, 4030105 CrossRef PubMed.
- T. P. McCarty, S. R. Lockhart, S. A. Moser, J. Whiddon, J. Zurko, C. D. Pham en and P. G. Pappas, J. Antimicrob. Chemother., 2018, 73, 1677–1680 CrossRef CAS.
- M. Galocha, P. Pais, M. Cavalheiro, D. Pereira, R. Viana en and M. C. Teixeira, Int. J. Mol. Sci., 2019, 20, 20092345 Search PubMed.
- A. Glockner en and O. A. Cornely, Mycoses, 2015, 58, 445–450 CrossRef PubMed.
- K. Kumar, F. Askari, M. S. Sahu en and R. Kaur, Microorganisms, 2019, 7, 1–22 CAS.
- Y. Hassan, S. Y. Chew en and L. T. L. Than, J. Fungi, 2021, 7, 7080667 Search PubMed.
- B. Goncalves, C. Ferreira, C. T. Alves, M. Henriques, J. Azeredo en and S. Silva, Crit. Rev. Microbiol., 2016, 42, 905–927 CrossRef CAS PubMed.
- C. Rodriguez-Cerdeira, E. Martinez-Herrera, M. Carnero-Gregorio, A. Lopez-Barcenas, G. Fabbrocini, M. Fida, M. El-Samahyen and J. L. Gonzalez-Cespon, Front. Microbiol., 2020, 11, 23 CrossRef.
- M. Rasheed, A. Battu en and R. Kaur, J. Biol. Chem., 2018, 293, 6410–6433 CrossRef CAS.
- C. J. Seneviratne, Y. Wang, L. J. Jin, Y. Abiko en and L. P. Samaranayake, Proteomics, 2010, 10, 1444–1454 CrossRef CAS PubMed.
- C.-J. N. Shantal, C.-C. Juan, B.-U. S. Lizbeth, H.-G. J. Carlos en and G.-P. B. Estela, Microbiol. Res., 2022, 260, 127038 CrossRef CAS PubMed.
- L. E. Cowen, D. Sanglard, S. J. Howard, P. D. Rogers en and D. S. Perlin, Cold Spring Harbor Perspect. Med., 2015, 5, a019752 CrossRef PubMed.
- Y. Lee, E. Puumala, N. Robbins en and L. E. Cowen, Chem. Rev., 2021, 121, 3390–3411 CrossRef CAS PubMed.
- P. Vandeputte, G. Tronchin, T. Berges, C. Hennequin, D. Chabasse en and J. P. Bouchara, Antimicrob. Agents Chemother., 2007, 51, 982–990 CrossRef CAS.
- S. G. Whaley en and P. D. Rogers, Curr. Infect. Dis. Rep., 2016, 18, 41 CrossRef.
- N. M. Revie, K. R. Lyer, N. Robbins en and L. E. Cowen, Curr. Opin. Microbiol., 2018, 45, 70–76 CrossRef CAS PubMed.
- E. A. Abi-Chacra, L. O. P. Souza, L. P. Cruz, L. A. Braga-Silva, D. S. Goncalves, C. L. Sodre, M. D. Ribeiro, S. H. Seabra, M. H. G. Figueiredo-Carvalho, L. S. Barbedo, R. M. Zancope-Oliveira, M. Ziccardi en and A. L. S. Santos, FEMS Yeast Res., 2013, 13, 831–848 CrossRef CAS PubMed.
- P. Gupta, H. Gupta en and K. M. Poluri, Appl. Microbiol. Biotechnol., 2021, 105, 5589–5605 CrossRef CAS PubMed.
- N. K. Khandelwal, M. Wasi, R. Nair, M. Gupta, M. Kumar, A. K. Mondal, N. A. Gaur en and R. Prasad, Antimicrob. Agents Chemother., 2019, 63, e01347-18 CrossRef PubMed.
- L. Vale-Silva, E. Beaudoing, V. D. T. Tran en and D. Sanglard, G3: Genes, Genomes, Genet., 2017, 7, 2413–2426 CrossRef CAS PubMed.
- O. Fiehn, Plant Mol. Biol., 2002, 48, 155–171 CrossRef CAS.
- B. Warth, A. Parich, C. Bueschl, D. Schoefbeck, N. K. N. Neumann, B. Kluger, K. Schuster, R. Krska, G. Adam, M. Lemmens en and R. Schuhmacher, Metabolomics, 2015, 11, 722–738 CrossRef CAS.
- A. H. Zhang, H. Sun, P. Wang, Y. Han en and X. J. Wang, Analyst, 2012, 137, 293–300 RSC.
- P. Brandt, E. Garbe en and S. Vylkova, PLoS Pathog., 2020, 16, e1008757 CrossRef CAS PubMed.
- P. Gupta, R. Chanda, N. Rai, V. K. Kataria en and N. Kumar, Assay Drug Dev. Technol., 2016, 14, 291–297 CrossRef CAS PubMed.
- A. Espinel-Ingroff, F. Barchiesi, M. Cuenca-Estrella, M. A. Pfaller, M. Rinaldi, J. L. Rodriguez-Tudela en and P. E. Verweij, J. Clin. Microbiol., 2005, 43, 3884–3889 CrossRef CAS PubMed.
- P. Gupta, R. C. Meena en and N. Kumar, Microb. Pathog., 2020, 149, 104469 CrossRef CAS PubMed.
- J. W. Tan, Z. Y. Liu, Y. Sun, L. J. Yang en and L. J. Gao, Mycopathologia, 2019, 184, 525–531 CrossRef CAS PubMed.
- P. Gupta, S. Gupta, M. Sharma, N. Kumar, V. Pruthi en and K. M. Poluri, ACS Omega, 2018, 3, 12201–12214 CrossRef CAS PubMed.
- Y. Miyake, T. Tsunoda, S. Minagi, Y. Akagawa, H. Tsuru en and H. Suginaka, FEMS Microbiol. Lett., 1990, 69, 211–214 CrossRef CAS.
- P. Gupta, V. Pruthi en and K. M. Poluri, J. Appl. Microbiol., 2021, 131, 105–123 CrossRef CAS.
- P. Gupta, A. Goel, K. R. Singh, M. K. Meher, K. Gulati en and K. M. Poluri, Int. J. Biol. Macromol., 2021, 172, 30–40 CrossRef CAS.
- N. Arora, D. Dubey, M. Sharma, A. Patel, A. Guleria, P. A. Pruthi, D. Kumar, V. Pruthi en and K. M. Poluri, ACS Omega, 2018, 3, 11847–11856 CrossRef CAS PubMed.
- K. Gulati, S. Sarkar en and K. M. Poluri, in Metabolomics, Springer, 2021, pp. 155–171 Search PubMed.
- T. Jewison, C. Knox, V. Neveu, Y. Djoumbou, A. C. Guo, J. Lee, P. Liu, R. Mandal, R. Krishnamurthy, I. Sinelnikov, M. Wilson en and D. S. Wishart, Nucleic Acids Res., 2012, 40, D815–D820 CrossRef CAS PubMed.
- M. Ramirez-Gaona, A. Marcu, A. Pon, A. C. Guo, T. Sajed, N. A. Wishart, N. Karu, Y. D. Feunang, D. Arndt en and D. S. Wishart, Nucleic Acids Res., 2017, 45, D440–D445 CrossRef CAS PubMed.
- J. Chong, O. Soufan, C. Li, I. Caraus, S. Li, G. Bourque, D. S. Wishart en and J. Xia, Nucleic Acids Res., 2018, 46, W486–W494 CrossRef CAS PubMed.
- L. Gupta, A. Guleria, A. Rawat, D. Kumar en and A. Aggarwal, Magn. Reson. Chem., 2021, 59, 85–98 CrossRef CAS PubMed.
- U. Kumar, A. Kumar, S. Singh, P. Arya, S. K. Singh, R. N. Chaurasia, A. Singh en and D. Kumar, Metab. Brain Dis., 2021, 36, 957–968 CrossRef CAS PubMed.
- A. Guleria, S. Phatak, D. Dubey, S. Kumar, A. Zanwar, S. Chaurasia, U. Kumar, R. Gupta, A. Aggarwal, D. Kumar en and R. Misra, J. Proteome Res., 2018, 17, 2440–2448 CrossRef CAS.
- R. Vij, C. Danchik, C. Crawford, Q. Dragotakes en and A. Casadevall, Msphere, 2020, 5, e00310-20 CrossRef PubMed.
- K. C. Hazen, J. G. Lay, B. W. Hazen, R. C. Fu en and S. Murthy, Infect. Immun., 1990, 58, 3469–3476 CrossRef CAS PubMed.
- T. M. J. Silva, P. M. Glee en and K. C. Hazen, J. Med. Vet. Mycol., 1995, 33, 117–122 CrossRef CAS.
- Q. Lv, L. Yan en and Y. Jiang, Virulence, 2016, 7, 649–659 CrossRef CAS PubMed.
- C. Schroter, U. C. Hipler, A. Wilmer, W. Kunkel en and U. Wollina, Arch. Dermatol. Res., 2000, 292, 260–264 CrossRef CAS.
- T. Hautbergue, E. L. Jamin, L. Debrauwer, O. Puel en and I. P. Oswald, Nat. Prod. Rep., 2018, 35, 147–173 RSC.
- A. Sevastos, I. F. Kalampokis, A. Panagiotopoulou, M. Pelecanou en and K. A. Aliferis, Data Brief, 2018, 19, 1162–1165 CrossRef CAS PubMed.
- C. B. Ford, J. M. Funt, D. Abbey, L. Issi, C. Guiducci, D. A. Martinez, T. Delorey, B. Y. Li, T. C. White, C. Cuomo, R. P. Rao, J. Berman, D. A. Thompson en and A. Regev, Elife, 2015, 4, e00662 CrossRef PubMed.
- M. P. Hirakawa, D. A. Martinez, S. Sakthikumar, M. Z. Anderson, A. Berlin, S. Gujja, Q. D. Zeng, E. Zisson, J. M. Wang, J. M. Greenberg, J. Berman, R. J. Bennett en and C. A. Cuomo, Genome Res., 2015, 25, 413–425 CrossRef CAS PubMed.
- J. Karkowska-Kuleta, D. Satala, O. Bochenska, M. Rapala-Kozik en and A. Kozik, BMC Microbiol., 2019, 19, 1–13 CrossRef CAS PubMed.
- M. M. Weerasekera, G. K. Wijesinghe, T. A. Jayarathna, C. P. Gunasekara, N. Fernando, N. Kottegoda en and L. P. Samaranayake, Mem. Inst. Oswaldo Cruz, 2016, 111, 697–702 CrossRef CAS PubMed.
- A. Ceballos-Garzon, L. Monteoliva, C. Gil, C. Alvarez-Moreno, N. E. Vega-Vela, D. M. Engelthaler, J. Bowers, P. Le Pape en and C. M. Parra-Giraldo, J. Antimicrob. Chemother., 2022, 77, 585–597 CrossRef CAS PubMed.
- H. Jafri, M. S. A. Khanen and I. Ahmad, Phytomedicine, 2019, 54, 206–213 CrossRef CAS PubMed.
- P.-C. Georgiou, M. C. Arendrup en and J. Meletiadis, J. Antimicrob. Chemother., 2022, dkac075 Search PubMed.
- C. M. Hull, O. Bader, J. E. Parker, M. Weig, U. Gross, A. G. S. Warrilow, D. E. Kelly en and S. L. Kelly, Antimicrob. Agents Chemother., 2012, 56, 6417–6421 CrossRef CAS.
- F. Alves, J. C. Carmello, E. G. D. Mima, C. A. D. Costa, V. S. Bagnato en and A. C. Pavarina, Med. Mycol., 2019, 57, 609–617 CrossRef CAS.
- S.-Y. M. Pang, S. Tristram en and S. Brown, Int. J. Med. Med. Sci., 2010, 1, 80–86 Search PubMed.
- R. Garcia-Rubio, H. C. de Oliveira, J. Rivera en and N. Trevijano-Contador, Front. Microbiol., 2020, 10, 02993 CrossRef PubMed.
- A. Thammahong, S. Puttikamonkul, J. R. Perfect, R. G. Brennan en and R. A. Cramer, Microbiol. Mol. Biol. Rev., 2017, 81, e00053-16 CrossRef.
- P. Van Dijck, L. De Rop, K. Szlufcik, E. Van Ael en and J. M. Thevelein, Infect. Immun., 2002, 70, 1772–1782 CrossRef CAS PubMed.
- B. G. Vu en and W. S. Moye-Rowley, Msphere, 2018, 3, e0009918 CrossRef PubMed.
- Q. Q. Li, H. F. Tsai, A. Mandal, B. A. Walker, J. A. Noble, Y. Fukuda en and J. E. Bennett, Mol. Med. Rep., 2018, 17, 6585–6597 CAS.
- K. Spettel, W. Barousch, A. Makristathis, I. Zeller, M. Nehr, B. Selitsch, M. Lackner, P. M. Rath, J. Steinmann en and B. Willinger, PLoS One, 2019, 14, e0210397 CrossRef CAS PubMed.
- N. Benaroudj, D. H. Lee en and A. L. Goldberg, J. Biol. Chem., 2001, 276, 24261–24267 CrossRef CAS PubMed.
- A. Thammahong, A. K. Caffrey-Card, S. Dhingra, J. J. Obar en and R. A. Cramer, mBio, 2017, 8, e00056-17 CrossRef PubMed.
- M. Martinez-Esparza, A. Aguinaga, P. Gonzalez-Parraga, P. Garcia-Penarrubia, T. Jouault en and J. C. Arguelles, Clin. Microbiol. Infect., 2007, 13, 384–394 CrossRef CAS PubMed.
- M. Martinez-Esparza, E. Martinez-Vicente, P. Gonzalez-Parraga, J. M. Ros, P. Garcia-Penarrubia en and J. C. Arguelles, Int. J. Med. Microbiol., 2009, 299, 453–464 CrossRef CAS PubMed.
- J. C. Oliver, L. Laghi, C. Parolin, C. Foschi, A. Marangoni, A. Liberatore, A. L. T. Dias, M. Cricca en and B. Vitali, Sci. Rep., 2020, 10, 16716 CrossRef CAS PubMed.
- W. Bell, W. N. Sun, S. Hohmann, S. Wera, A. Reinders, C. De Virgilio, A. Wiemken en and J. M. Thevelein, J. Biol. Chem., 1998, 273, 33311–33319 CrossRef CAS PubMed.
- J. R. Perfect, J. L. Tenor, Y. Miao en and R. G. Brennan, Virulence, 2017, 8, 143–149 CrossRef CAS PubMed.
- H. Tournu, A. Fiori en and P. Van Dijck, PLoS Pathog., 2013, 9, e1003447 CrossRef CAS PubMed.
- J. Lopez, F. Dalle, P. Mantelin, P. Moiroux, A. C. Nierlich, A. Pacot, B. Cuisenier, O. Vagner en and A. Bonnin, J. Clin. Microbiol., 2001, 39, 1172–1174 CrossRef CAS PubMed.
- W. L. Xia, Z. Wang, Q. Wang, J. Han, C. P. Zhao, Y. Y. Hong, L. L. Zeng, L. Tang en and W. H. Ying, Curr. Pharm. Des., 2009, 15, 12–19 CrossRef CAS PubMed.
- J. Li, Y. Sun, F. Liu, Y. Zhou, Y. Yan, Z. Zhou, P. Wang en and S. Zhou, Bioresour. Bioprocess., 2022, 9, 1 CrossRef.
- N. Krishnan, M. B. Dickman en and D. F. Becker, Free Radic. Biol. Med., 2008, 44, 671–681 CrossRef CAS PubMed.
|
This journal is © The Royal Society of Chemistry 2022 |
Click here to see how this site uses Cookies. View our privacy policy here.