DOI:
10.1039/D2RA01500E
(Paper)
RSC Adv., 2022,
12, 9121-9129
Process optimization for the synthesis of functionalized Au@AgNPs for specific detection of Hg2+ based on quality by design (QbD)†
Received
7th March 2022
, Accepted 16th March 2022
First published on 23rd March 2022
Abstract
The current study highlights the advantages of using the quality by design (QbD) approach to synthesise and optimize SERS substrates for the detection of Hg2+. Considering that the performance of Au@AgNPs is affected by many factors, Plackett–Burman (PB) experimental design was used to determine the critical process parameters (CPPs) for evaluating the performance of Au@AgNPs. The quantitative relationships between the CPPs and the critical quality attributes (CQAs) were assessed by Box-Behnken Design (BBD). The optimal design space for Au@AgNPs was calculated via a Monte Carlo algorithm. Finally, detection of Hg2+ in the range of 1 ∼ 100 ng mL−1 (R2 = 0.9891) was achieved by SERS in combination with 4,4-bipyridine (Dpy) as signal molecules. The recoveries for licorice ranged from 83.53% to 92.96%. Specificity and practicality studies indicated that the method based on the QbD concept and design space not only met the optimal performance of Au@AgNPs but also improved the rapid detection of Hg2+ in Chinese medicine samples.
1 Introduction
Traditional Chinese Medicine (TCM) plays an important role in curing diseases and keeping the body in good condition, it also has been greatly contributing to the treatment of the COVID-19. However, its further development worldwide has been seriously hampered by contamination with trace harmful substances during cultivation and production.1,2 Heavy metal ions, one of the most important factors currently polluting the environment, have caused widespread concern as they cannot be degraded by microorganisms and accumulate in the human body through various forms of interconversion.3,4 In particular, heavy metal ions such as mercury (Hg2+), cadmium (Cd2+), lead (Pb2+), arsenic (As3+) and copper (Cu2+), which are not essential for life activities, damaging the brain and eyesight if they enter the body, even at trace levels. It has been reported that these heavy metals exert dangerous effects by affecting protein folding, cell signalling, DNA functions.5–8 Hg2+, as a highly toxic pollutant, is rich in stability and water solubility making it prone to accumulation. Once it entered the human body, it not only disrupted human metabolism, but also damaged the immune system and central nervous system, posing in a serious threat to human health and life.9–11 Therefore, it is necessary to strictly control the amount of Hg2+ in food, herbs, water and other substances. A lot of methods have been used to detect Hg2+, including Atomic Absorption Apectrometry (AAS),4,12,13 inductively coupled plasma mass spectrometry (ICP-MS),14–16 Fluorescence17,18 and Electrochemistry.19,20 Although most of these methods are convincing and accurate enough, they generally require expensive instrumentation and sophisticated equipment, which makes them unavailable for rapid and on-line detection in situ analysis. In recent years, novel techniques such as spectroscopy,21 spectral imaging22 and computer imaging23 have received widespread attention in the areas of drug quality and food safety. Among these, surface-enhanced Raman spectroscopy (SERS), is a powerful molecular vibration spectroscopy technique that has obtained great progress in the detection of harmful chemical residues, such as heavy metal ions and pesticide residues due to its simplicity of sampling, non-destructive and rapid data collection nature.24–27
Most scholars believe that SERS consists mainly of both physical and chemical enhancement. Physical enhancement, which is the dominant factor, is thought to be the enhancement of the electromagnetic field on the surface of the metal nanostructure excited by incident light. Chemical enhancement is based on charge transfer between molecules.28–30 Currently, AuNPs and AgNPs are the two commonly used SERS substrates, whose surface plasmon resonances in the visible and near-infrared (NIR) range are widely reported.31,32 Compared to the instability and aggregation of monometallic nanoparticles, Au@AgNPs exhibits not only the high enhancement properties of AgNPs, but also the advantages of long stability and high homogeneity of AuNPs.25,33 More importantly, Au@AgNPs are the best alternative to achieve high performance SERS substrates compared to expensive and cumbersome self-assembled nanomaterials such as nanopores and nanoarrays.34,35
Au@AgNPs were achieved via gold seed solutions and the process was optimized by employing Quality by Design (QbD). QbD is considered an important method to improve product quality by designing scientific, rational and reliable processes.36,37 It has been widely used in chemical synthesis,38 herbal extraction,39 separation and quantification processes.40 In this work, the enhanced performance of Au@AgNPs was considered as a critical quality attribute (CQAs), and the critical process parameters (CPPs) were screened by Plackett–Burman (PB) design. Then, a mathematical model between CPPs and CQAs of the Au@AgNPs synthesis process was developed through Box-Behnken (BBD) design, and the design space was calculated and validated using a Monte Carlo algorithm.41
Here, we presented a highly sensitive and selective Dpy-functionalized Au@AgNPs as a label-free probe for the detection of Hg2+ (Scheme 1). The indirect determination of Hg2+ in homogeneous solutions was achieved with the use of Au@AgNPs as SERS substrate, mainly based on the original Raman signal of Dpy and the strong coordination with metal ions. In the range of 1–100 ng mL−1, there was a clear correlation between the reduced Raman intensity of Dpy and the concentration of Hg2+. In fact, there have been several reports on Hg2+ detection based on Dpy-conjugated noble metal NPs.42 The pyridine group on Dpy acted as adsorption sites of Hg2+. The N atoms on the pyridine ring of Dpy were adsorbed onto the silver shell of Au@AgNPs, subsequently generating a Raman signal. When Hg2+ was present in the system, the strong coordination of Hg2+ to Dpy exceeded the binding capacity between Dpy and Au@AgNPs. Therefore, Hg2+ could quench the Raman signal of Dpy in Au@AgNPs, thus providing an ultra-sensitive analytical method for indirect detection of Hg2+.
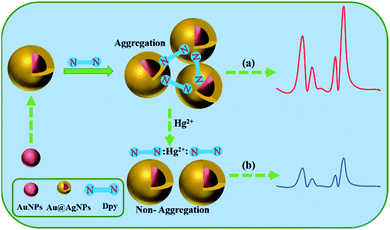 |
| Scheme 1 Schematic illustration of the fabrication of Au@Ag–Dpy SERS probe and a sensing protocol for Hg2+. (a) Indicates the SERS signal without the addition of mercury ions; (b) indicates the SERS signal after the addition of mercury ions. | |
2 Experimental
2.1 Chemicals and reagents
Licorice herbs were supplied by the laboratory. Nitric acid (HNO3, 65%) and hydrochloric acid (HCl, 35–38%) were purchased from Sinopharm Chemical Reagent Co., Ltd (Hangzhou, China). Ascorbic acid (AA, 99%), silver nitrate (AgNO3, ≥99.0%) and trisodium citrate (C6H5Na3O7·2H2O, ≥99.0%) were brought from Chengdu Kolon Chemicals Co., Ltd. 4,4-bipyridine (Dpy) and chloroauric acid (HAuCl4·4H2O, 99.9%) were purchased from Macklin. The standard solutions of Hg, Cd, As, Cu, Pb (1000 μg mL−1) were brought from national institutes for food and drug control. All glassware used in the work was washed with aqua regia (HCl/HNO3 = 3
:
1, v/v) and then rinsed with ultrapure water (18.2 MΩ cm).
2.2 Apparatus and instruments
The Raman spectra were collected by Prott-EZRamanB2 (SB5) analyser (Enwave Optronics Inc, USA) with excitation wavelength of 785 nm and 1 cm quartz cell. An intelligent double-controlled thermostatic magnetic stirrer (Model DF101S, China) and a digital shaker (TYZD-II, China) were used for the synthesis of Au@AgNPs. UV-Vis spectrophotometer (UV-2450, Japan) with a scanning range of 200 to 800 nm was used to record absorption spectra. 300 kV high resolution transmission electron microscope (Tecnai G2 F30, Netherlands) was selected for characterisation of nanomaterials. Ultrasonic detector (KQ-250DB, Kunshan, China) with a frequency of 35 kHz. Electronic balance (FA2204N, Shanghai, China). Ultrapure water purification system (Milli Q, Thermo Fisher Scientific, China).
2.3 Preparation of AuNPs and Au@AgNPs
The gold nanoparticles (AuNPs) were synthesized according to the previously reported method.32 Typically, 950 μL of HAuCl4·4H2O (5 g L−1) was added to a three-necked flask containing 60 mL of ultrapure water. The solution was heated to boiling and held at 120 °C for 1 min, then 600 μL of 1% trisodium citrate was rapidly added and stirred continuously under strong magnetic field stirring for 5 min. A change from a colourless solution to a burgundy colour could be observed, the heating was switched off and the solution was cooled to room temperature.
The core–shell Au@AgNPs were synthesized based on the above AuNPs as seed solution. Firstly, 3 mL of AuNPs seed solution was added to a 10 mL EP tube, and ultrasounded for 10 minutes in order to avoid aggregation. Then, 150 μL of 10 mM ascorbic acid was added to the test tube and mixed with the AuNPs seed colloid under shaking on a digital shaker for 2 min. Finally, 160 μL of freshly prepared aqueous AgNO3 solution at a concentration of 10 mM was added drop by drop at 15 second intervals with continuous shaking. After 15 min of continuous shaking, the core–shell Au@AgNPs were transferred to brown bottles and stored at 4 °C. All experiments were carried out at room temperature. The morphology and particle size of the prepared Au@AgNPs were characterised by UV-visible absorption spectroscopy (UV-vis) and transmission electron microscopy (TEM).
2.4 Optimization of the synthesis process of Au@AgNPs by QbD
2.4.1 Determination of critical process parameters (CPPs). Based on previous experiments, it was found that the environments, reaction systems, instrumentation and analytical parameters were the main factors affecting the capability of Au@AgNPs. The different risks for the synthesis of Au@AgNPs were described in a fishbone diagram (Fig. 1). Six factors that have a more critical influence on the performance of Au@AgNPs were selected for investigation through risk assessment and single-factor experiments. The Raman enhancement effect (R) of Au@AgNPs was identified as the CQAs. Six factors, including trisodium citrate dosage (X1), gold nanosynthesis time (X2), ascorbic acid dosage (X3), silver nitrate dosage (X4), oscillation time (X5) and incubation time (X6), were used as potential CPPs. The factors and corresponding levels of the PB experimental design were shown in ESI 1.† According to the Placket–Burman (PB) design, the CPPs affecting the performance of Au@AgNPs will be further determined.
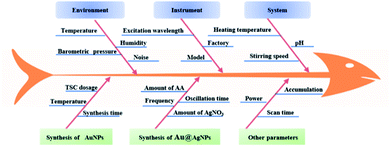 |
| Fig. 1 Fishbone diagram of CPPs affecting the performance of Au@AgNPs. | |
2.4.2 Establishment of regression modelling between CPPs and CQAs. A Box-Behnken experimental design was used to investigate the mathematical model between the CPPs and CQAs of the Au@AgNPs synthesis process (shown in ESI 2†). The amount of ascorbic acid was fixed at 150 μL, the shaking time was fixed at 15 min and the incubation time was fixed at 5 min.
2.4.3 Development of the method operable design region (MODR). MODR was calculated based on the results of the regression model (BBD) and based on the Monte Carlo algorithm in the environment of MATLAB software. In the optimization procedures, MODR was established with (R) as the evaluation index and X1, X2 and X4 as CPPs. The number of calculations was set as 1000, the pass rate was 0.8, and the step sizes was set as 0.5, 0.2 and 0.5 P-values < 0.05 was considered significant. Averaged by comparing the data from the three experiments, and then the SERS spectra were plotted using Origin 2019b.
2.5 Procedure for the detection of Hg2+
In brief, 200 μL Au@AgNPs, 200 μL Dpy and 200 μL at different concentrations of Hg2+ were added sequentially to a 3 mL cuvette, mixed thoroughly and reaction for 5 min. The SERS signal was acquired by Raman spectroscopy. The signal change of Dpy at 1296 cm−1 was recorded as a basis for quantification. All samples were collected using a portable Raman spectrometer equipped with a semiconductor laser of 300 mW power and a Peltier-cooled CCD detector at −70 °C. The spectra were scanned from 250 to 2350 cm−1, the acquisition time for each spectrum was set to 10 s, each spectrum was accumulated for five times, and all experiments were performed three times in parallel. Cosmic ray interference was removed using a self-contained algorithm, averaged by comparing the data from the three experiments, and then the SERS spectra were plotted using Origin 2019b.
2.6 Detection of Chinese medicine samples
To verify the practicality of this protocol, our strategy was tested using liquorice extraction as a spiked sample. Firstly, the liquorice was powdered and sieved, 5 g of the powder was added to 50 mL of water, ultrasound for 30 min, centrifuged and passed through a 0.22 μm filter membrane to remove insoluble impurities. Then, a series of Hg2+ standard solutions were added to the mixture. Finally, the spiked samples were tested by SERS method.
2.7 Data processing
Experimental design and data analysis were performed by Design Expert V8.0 software (Stat-Ease, USA). Method operable design region was calculated by MATLAB (Mathematical Works, USA). Raman spectral data processing was implemented by Origin 2019b, and SERS spectra were acquired for five times of each sample.
3 Results and discussion
3.1 Discrimination of CPPs and CQAs
In the present study, the effect of some potential CPPs on the enhancement performance of Au@AgNPs was investigated by means of a Plackeet–Burman (PB) experimental design, and the results were shown in Table 1.
Table 1 Plackeet–Burman design experiment
Number |
Potential CPPs |
CQAs |
X1 (μL) |
X2 (min) |
X3 (μL) |
X4 (μL) |
X5 (min) |
X6 (min) |
R (a.u.) |
1 |
400 |
5 |
150 |
200 |
10 |
5.00 |
6204 |
2 |
400 |
5 |
150 |
150 |
10 |
3.00 |
6332 |
3 |
400 |
5 |
200 |
150 |
15 |
5.00 |
9457 |
4 |
400 |
10 |
150 |
200 |
15 |
3.00 |
16 932 |
5 |
400 |
10 |
200 |
200 |
10 |
3.00 |
13 233 |
6 |
400 |
10 |
200 |
150 |
15 |
5.00 |
11 873 |
7 |
600 |
5 |
200 |
150 |
15 |
3.00 |
31 676 |
8 |
600 |
5 |
200 |
200 |
10 |
5.00 |
34 252 |
9 |
600 |
5 |
150 |
150 |
15 |
3.00 |
32 521 |
10 |
600 |
10 |
200 |
200 |
10 |
3.00 |
39 497 |
11 |
600 |
10 |
150 |
200 |
15 |
5.00 |
41 505 |
12 |
600 |
10 |
150 |
150 |
10 |
5.00 |
34 111 |
As shown in Table 2, the CPPs affecting the performance of Au@AgNPs according to P-value were X1 > X2 > X4 > X5> X6 > X3. The results showed that X1 had an extremely significant effect on the performance of Au@AgNPs with P-value < 0.0001, X2 and X4 reached a significant level with P-value < 0.05, while X3, X5 and X6 had no significant effects on the performance of Au@AgNPs. A multiple regression analysis of the CPPs was carried out by Design Expert 8.0 software. Fig. 2 showed the Pareto analysis plots of six CPPs and CQAs. In summary, X1, X2 and X4, were selected for further Box-Behnken experimental design and analysis. X3, X5 and X6 were fixed at 150 μL, 15 min and 5 min, respectively.
Table 2 Effect evaluations of each factor under Plackett–Burman test designa
Potential CPPs |
P-value |
Significance |
Significant (P < 0.05); extremely significant (P < 0.01). |
Model |
<0.0001 |
Extremely significant |
X1 |
<0.0001 |
Extremely significant |
X2 |
0.0142 |
Significant |
X3 |
0.9588 |
|
X4 |
0.0480 |
Significant |
X5 |
0.1386 |
|
X6 |
0.5273 |
|
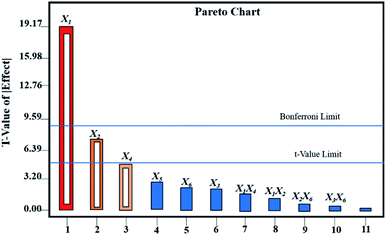 |
| Fig. 2 Pareto analysis charts of the CPPs and the CQAs. | |
3.2 Analysis of response surface experimental design
The response surface experiments were implemented by the Box-Behnken experimental design. The results and regression equations were presented in ESI 2 and 3.† Models were fitted to 17 sets of experimental data by the Desing-Expert software, and the ANOVA were presented in Table 3. The regression model had a p-value < 0.0001, indicating that the model was highly significant. The p-value for the lack of fit was 0.9555 > 0.05, and the experimental error was not significant, which proved that the model was acceptable. The P-value of the monomial coefficient X1 was <0.0001, which was a highly significant term (++), X2 (P = 0.0089 < 0.05) and X4 (P = 0.0032 < 0.05) were both significant terms. The P-values of the binomial coefficient X12 and X42 were <0.0001, indicating that the effect of X1 and X4 on the Raman enhancement of Au@AgNPs was very obviously, while the effect of the X2 was relatively weak. Based on the p-values of the independent variables X1, X2 and X4, the order of importance of each factor on the Raman enhancement effect of Au@AgNPs was determined as X1 > X4 > X2. This was consistent with the above description. The fitted model had a regression coefficient of determination R2 = 0.9897 and a corrected regression coefficient of determination R2 adj = 0.9765, indicating that the data model was well fitted (ESI 3†). The response surface interactions for each factor were shown in Fig. 3. The BBD results showed that the best experimental points for the three parameters were X1 = 550.70 μL; X2 = 6.55 min; X3 = 170.67 μL, respectively.
Table 3 ANOVA of Raman enhancement effect of Au@AgNPs
Source model |
Sum of squares |
df |
Mean square |
F-value |
P-value |
Significant level |
Model |
1.968E + 009 |
9 |
2.187E + 008 |
74.97 |
<0.0001 |
++ |
X1 |
1.463E + 009 |
1 |
1.463E + 009 |
501.74 |
<0.0001 |
++ |
X2 |
1.947E + 007 |
1 |
1.947E + 007 |
6.67 |
0.0089 |
+ |
X4 |
2.246E + 007 |
1 |
2.246E + 007 |
7.70 |
0.0032 |
+ |
X1X2 |
2.136E + 006 |
1 |
2.136E + 006 |
0.73 |
0.2733 |
|
X1X4 |
5.991E + 007 |
1 |
5.991E + 007 |
20.54 |
0.7420 |
|
X2X4 |
61 256.25 |
1 |
61 256.25 |
0.021 |
0.8462 |
|
X12 |
2.077E + 008 |
1 |
2.077E + 008 |
71.21 |
<0.0001 |
++ |
X22 |
9.936E + 005 |
1 |
9.936E + 005 |
0.34 |
0.0055 |
|
X42 |
2.401E + 008 |
1 |
2.401E + 008 |
82.32 |
<0.0001 |
++ |
Residual |
2.042E + 007 |
7 |
2.917E + 006 |
|
|
|
Lack of fit |
1.058E + 007 |
3 |
3.526E + 006 |
1.43 |
0.9555 |
Not significant |
Pure error |
9.838E + 006 |
4 |
2.460E + 006 |
74.97 |
|
|
Cor total |
1.988E + 009 |
16 |
|
|
|
|
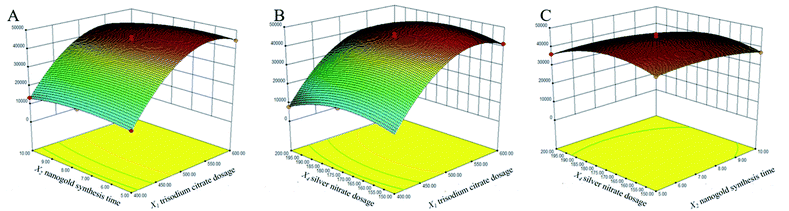 |
| Fig. 3 Three-dimensional response surface diagram of the interaction of three factors. (A) Interaction between X1 and X2; (B) interaction between X1 and X4; (C) interaction between X2 and X1. | |
3.3 Design space development and operation point selection
In order to improve the robustness of the Au@AgNPs synthesis method, a Monte Carlo algorithm was used to automatically obtain the design space of the method. As shown in Fig. 4, the shape of the design space was regular and the red area was the optimal design space. The results indicated that the three CPPs were 510 to 550 μL for X1, 150 to 190 μL for X4, and 5 to 9 min for X2, respectively. The design space was then experimentally validated, and the validation results were shown in Table 4. The results showed that the actual and predicted values of R were basically consistent, illustrating that the design space established in this study was relatively reliable.
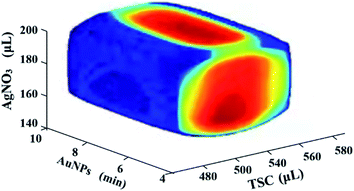 |
| Fig. 4 Analytical design space and verification points. | |
Table 4 Results of validation experiments
Number |
X1 (μL) |
X2 (min) |
X4 (μL) |
R (a.u.) |
Prediction |
Qualified rate |
01 |
520 |
5 |
150 |
45 063 |
44 466 |
98.67% |
02 |
540 |
8 |
180 |
46 345 |
45 009 |
97.11% |
03 |
560 |
9 |
160 |
43 815 |
44 032 |
100.49% |
04 |
450 |
10 |
200 |
20 098 |
21 777 |
108.35% |
05 |
620 |
5 |
100 |
35 077 |
37 091 |
105.74% |
3.4 Characterization of AuNPs and Au@AgNPs
The Au@AgNPs were optimized and synthesised according to the method determined by QbD above. UV-Vis absorption spectroscopy and TEM were used to characterize the properties of the Au@AgNPs. As shown in Fig. 5A, the UV-Vis spectrum with an obvious absorption band at 526 nm, which belonged to the characteristic peak of Au. The UV-Vis spectrum of Au@AgNPs had distinct absorption bands at 495 nm and 417 nm, which were the surface plasmon resonance peak of the Ag shell and the surface plasmon resonance peak of the Au, respectively (Fig. 5B). A blue-shifted was observed on the peak about 417 nm after the Ag shell was coated, which was caused by the hole coupling between the AuNPs and the Ag shell.33 The TEM results showed that the AuNPs prepared by this method were spherical and relatively uniform in size, with an average particle size of 23 nm (Fig. 5C). The morphology of the core–shell Au@AgNPs was shown in Fig. 5D, the thickness of the core–shell obtained by adding different volume of AgNO3 into Au seeds. The thickness of the core–shell of Au@AgNPs was 8.5 nm and showed excellent SERS activity.
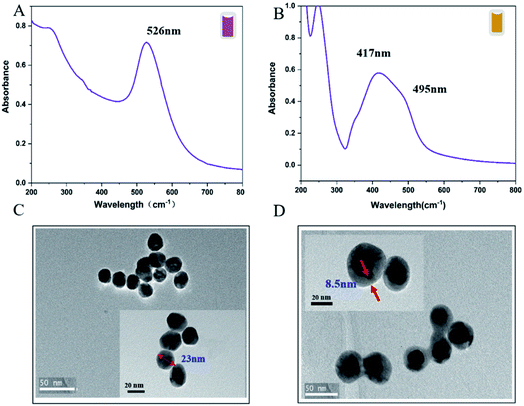 |
| Fig. 5 The characterization of AuNPs and core–shell Au@AgNPs. The UV-Vis spectra of (A) AuNPs and (B) Au@AgNPs; TEM images of (C) AuNPs and (D) core–shell Au@AgNPs. The colours of AuNPs and Au@AgNPs are indicated in the upper right corner of (A) and (B), respectively. | |
3.5 Optimize the reaction system of Dpy and Au@AgNPs
In order to obtain the best probe performance for Hg2+ detection, the necessary experimental parameters were systematically optimized. The concentration of Dpy and the incubation time of Au@AgNPs–Dpy were taken into. As shown in Fig. 6A, the Raman signal showed a trend of enhancing and then decreasing with increasing Dpy concentration. In particular, the Raman signal of Dpy was suppressed when the concentration exceeded 10−4M. Due to that the more Dpy was introduced into the system, the more Au@AgNPs–Dpy binding exceeded the optimum level, masking the enhancement of Au@AgNPs. Therefore, 10−4 M of Dpy was chose as the optimal concentration.
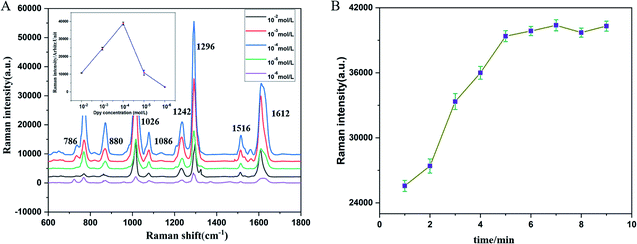 |
| Fig. 6 (A) Raman intensity at different concentrations (Dpy). (B) Raman intensity of Dpy with Au@AgNPs at different incubation times. | |
According to previous study, diazide NPs were formed when Dpy was combined with AgNPs. Therefore, the variation of Raman signal intensity for different incubation times of Dpy and Au@AgNPs was further tested. As shown in Fig. 6B, the Raman intensity (R) gradually increased over 1–5 minutes. This indicated that Dpy was continuously binding to Au@AgNPs. It was not until after 5 minutes that the system reached saturation with Au@AgNPs. With further increases in incubation time, R will not change much. Therefore, the best time for incubation was chosen to be 5 minutes.
3.6 Detection of Hg2+ with Dpy-functionalized SERS substrates
The SERS spectra of Au@AgNPs–Dpy with different Hg2+ concentrations (1–100 ng mL−1) were shown in Fig. 7. The detection of Hg2+ was achieved by observing the decrease in the SERS intensity of Dpy. The Raman intensity at 1296 cm−1 represented its characteristic Raman peak, which decreased with the increasing of Hg2+ concentration. As the Hg2+ concentration increased, the Dpy was stripped off the surface of the Ag shell, which caused a gradual decrease in the Raman intensity of Dpy. As shown in Fig. 7B, the relative Raman intensity of Dpy showed a good linear relationship with the concentration of Hg2+. (I − I0, I and I0 represented the SERS intensity of Dpy at 1296 cm−1 when Hg2+ presented or absented). A regression equation was further obtained: y = 90.221x + 1687.4 (R2 = 0.9891). Compared to the absence of Hg2+, there was a significant reduction in the Raman intensity of the mixture when the Hg2+ concentration was over 1 ng mL−1. Therefore, the limit of detection was 1 ng mL−1, which was below the level allowed in drinking water and pharmacopoeias 2020. On the other hand, 10 SERS spectra of Au@AgNPs incubated with 50 ng mL−1 Hg2+ were randomly selected to assess the reproducibility of our tests. All spectra showed highly consistent characteristic peaks and signal intensities for Dpy. As shown in Fig. 7C, the RSD of the characteristic Raman peak of Dpy at 1296 cm−1 was 6.81%, indicating the good reproducibility of these experiments.
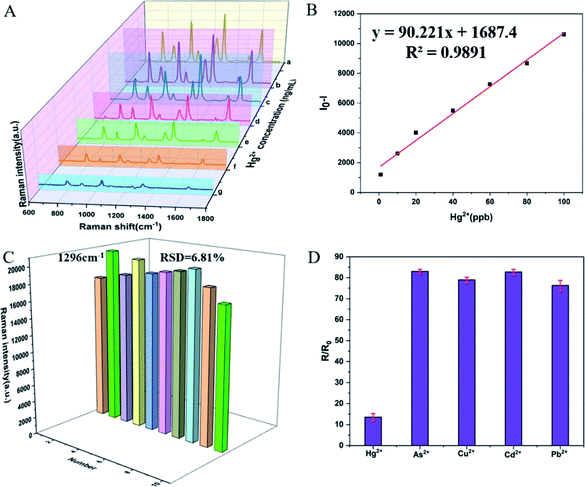 |
| Fig. 7 (A) The Raman spectra of the Au@AgNPs incubated with different amounts of Hg2+ (a–g: 1, 10, 20, 40, 60, 80, 100 ppb). (B) Linearity of the relative Raman intensity of Dpy (10−4M) at 1296 cm−1 with respect to Hg2+ concentration. (C) 10 randomly selected SERS spectra acquired from Au@Ag NPs incubated with Hg2+. (D) The relative SERS intensity of Dpy at 1296 cm−1 (R–R0) after added with Hg2+ or other cations. The error bars in each data showed the scattering in the signal from five repeated measurements. | |
Considering the specificity of the Au@AgNPs–Dpy probe in the determination of Hg2+, we tested the Raman response of the Dpy probe after the addition of different ions, including Pb2+, Cd2+, As3+, Cu2+ at a concentration of 10 μg mL−1 and Hg2+ at a concentration of 1 μg mL−1. As shown in Fig. 7D, the Raman intensity decreased significantly when Hg2+ was added, whereas the addition of other heavy metal ions only resulted in a slight decrease in the SERS intensity of the Dpy at 1296 cm−1. Therefore, these results indicated that the method established in this paper was highly selective for the detection of Hg2+. The main reason for this was that Hg2+ could coordinate with the N atom on Dpy to form a coordination structure, and the covalent bond formed by the coordination of the N atom with Hg was much more stable than that of the other metal ions. The addition of Hg2+ inhibited the binding of Dpy to Au@Ag NPs, so the Raman intensity of Dpy was significantly reduced.
3.7 Detection of Hg2+ in liquorice extract
To investigate the practical application of the constructed Au@AgNPs–Dpy approach for the determination of Hg2+, recovery experiments were carried out in liquorice extract. Notably, the actual sample assay was carried out by injecting Hg2+ into liquorice extract. After filtration through a 0.22 μm microporous filter to remove any particulate suspension, the liquorice extract was treated with different concentrations of Hg2+. The prepared samples were then measured by Raman spectrometry. As shown in Table 5, the recoveries ranged from 83.53% to 92.96%. The above results indicated that the SERS method was reliable, accurate, and applicable, which had a good potential for practical assays.
Table 5 Detect the Hg2+ in Licorice based on SERS analysis
Samples |
Spiked |
Detected |
Recovery (%) |
RSD (%) |
Licorice |
10 ng |
8.11 |
88.11 |
3.76 |
Licorice |
20 ng |
17.569 |
88.78 |
4.12 |
Licorice |
40 ng |
34.1161 |
83.53 |
3.61 |
Licorice |
60 ng |
57.7547 |
92.96 |
4.43 |
3.8 Compare the sensitivity of different analytical methods
In addition, to compare the sensitivity of this study with other detection techniques. We collected and summarised recent work on the detection of Hg2+. As shown in Table 6, ICP-MS, AAS, colorimetric, fluorescence, electrochemical and UV-visible methods were listed. It was clear that Au@AgNPs–Dpy had low detection limits compared to most of the above analytical strategies. It was worth mentioning that no specialist operator or complex sample pre-treatment was required. Therefore, it offers clear advantages in practical sample detection.
Table 6 Comparison of previously reported method with the present method for the detection of Hg2+
Analytical technique |
Dynamic range |
LOD (ppb) |
Ref. |
AAS |
— |
30 |
4 |
Fluorescence |
0.01–10 × 10−6 M |
11 |
17 |
Electrochemical |
20–1600 μg L−1 |
3.0 |
20 |
UV-visible spectroscopy |
2.5–58 × 10−7M |
5.8 |
43 |
ICP-MS |
0.01–10 mg L−1 |
7.0 |
14 |
Colorimetry |
0.1–1.0 × 10−6 M |
15.6 |
44 |
SERS |
0.5–100 μg mL−1 |
100 |
45 |
SERS |
5–1000 μg L−1 |
5 |
46 |
SERS |
1–100 ng mL−1 |
1.0 |
This work |
4 Conclusions
In summary, a novel method based on QbD concept for the preparation of a core–shell nanomaterial (Au@AgNPs) was presented. The prepared Au@AgNPs combined the advantages of both AuNPs and AgNPs, with both good SERS performance and good stability. The design space conditions for the Au@AgNPs were obtained using Monte Carlo algorithm: (X1) 510–550 μL for trisodium citrate, (X4) 150–190 μL for silver nitrate, (X2) 5–9 min for nanogold synthesis. The Dpy-based functionalised Au@AgNPs were used for the determination of Hg2+ in Chinese medicine samples with good linearity in the range of 1–100 ng mL−1. The detection limit was 1 ppb, which was well below the requirements in EPA and Ch.P 2020. In addition, selectivity and practicality studies showed that this method had excellent specificity and sensitivity. Compared with conventional methods such as ICP-MS and AAS for the detection of Hg2+, the SERS method based on the Dpy probe molecule has the advantages of simple pre-treatment, low solvent consumption, low detection sample volume, green and non-polluting, and convenient and sensitive detection, which is expected to provide a series of inexpensive, simple, reliable and ultra-sensitive detection strategies for the future field analysis of heavy metals in TCM products.
Author contribution
Hui Zhang: Formal analysis, conceptualization, methodology, writing – reviewing and editing. Baoling Wang: investigation, data curation, writing – original draft preparation. Xiaoyi Liu and Hongxu Zhang: supervision, validation, investigation. Jizhong Yan: funding acquisition, project administration and supervision.
Conflicts of interest
The authors declare that they have no known competing financial interests or personal relationships that could have appeared to influence the work reported in this paper.
Acknowledgements
This research was supported by Zhejiang Provincial Natural Science Foundation of China (Grant No. LY20H280014), the Basic Public Welfare Research Project of Zhejiang Province (Grant No. LBY22H280003) and Zhejiang Provincial Department of Science and Technology Project (Grant No. 2022C03062).
References
- C. M. Yang, M. Y. Chien, P. C. Chao, C. M. Huang and C. H. Chen, J. Hazard. Mater., 2021, 412, 125142 CrossRef CAS PubMed.
- L. Zheng, H. Mao, L. Zhang, Y. Jin, Y. Zhou, Y. Peng and S. Du, Anal. Methods, 2014, 6, 5925 RSC.
- N. Abdu, A. A. Abdullahi and A. Abdulkadir, Environ. Chem. Lett., 2016, 15, 65–84 CrossRef.
- T. T. Zuo, H. Y. Jin, L. Zhang, Y. L. Liu, J. Nie, B. L. Chen, C. F. Fang, J. Xue, X. Y. Bi, L. Zhou, M. R. Shen, S. M. Shi and S. C. Ma, Pharmacol. Res., 2020, 159, 104987 CrossRef CAS PubMed.
- Z. Jiang, N. Xu, B. Liu, L. Zhou, J. Wang, C. Wang, B. Dai and W. Xiong, Ecotoxicol. Environ. Saf., 2018, 157, 1–8 CrossRef CAS PubMed.
- M. S. Frost, M. J. Dempsey and D. E. Whitehead, Sens. Actuators, B, 2015, 221, 1003–1008 CrossRef CAS.
- S. Thatai, P. Khurana, S. Prasad and D. Kumar, Talanta, 2015, 134, 568–575 CrossRef CAS PubMed.
- S. S. Dasary, Y. K. Zones, S. L. Barnes, P. C. Ray and A. K. Singh, Sens. Actuators, B, 2016, 224, 65–72 CrossRef CAS PubMed.
- C. Fan, X. Zhang, J. M. Lv, J. Bai, Z. Qiu, Y. M. Zhao, Z. L. Wu and H. J. Xu, Anal. Methods, 2021, 13, 3274–3281 RSC.
- T. W. Clarkson, L. Magos and G. J. Myers, N. Engl. J. Med., 2003, 349, 1731–1737 CrossRef CAS PubMed.
- G. Panthi and M. Park, J. Hazard. Mater., 2021, 424, 127565 CrossRef PubMed.
- D. J. Butcher, Appl. Spectrosc. Rev., 2017, 52, 755–773 CrossRef.
- H. Erxleben and J. Ruzicka, Anal. Chem., 2005, 77, 5124–5128 CrossRef CAS PubMed.
- A. L. H. Muller, E. I. Muller, J. S. Barin and E. M. M. Flores, Anal. Methods, 2015, 7, 5218–5225 RSC.
- T. Thabit, D. I. H. Elgeddawy and S. A. Shokr, J. AOAC Int., 2020, 103, 1282–1287 CrossRef PubMed.
- Y. Chen, X. Cheng, F. Mo, L. Huang, Z. Wu, Y. Wu, L. Xu and F. Fu, Electrophoresis, 2016, 37, 1055–1062 CrossRef CAS PubMed.
- S. Choi and Y. Kim, RSC Adv., 2015, 5, 95268–95272 RSC.
- S. Xu, S. Xu, Y. Zhu, W. Xu, P. Zhou, C. Zhou, B. Dong and H. Song, Nanoscale, 2014, 6, 12573–12579 RSC.
- D. Han, Y.-R. Kim, J.-W. Oh, T. H. Kim, R. K. Mahajan, J. S. Kim and H. Kim, The Analyst, 2009, 134 Search PubMed.
- D. Martin-Yerga, M. B. Gonzalez-Garcia and A. Costa-Garcia, Talanta, 2013, 116, 1091–1104 CrossRef CAS PubMed.
- T. Wang, J. C. Wei, Y. T. Wang and P. Li, China J. Chin. Mater. Med., 2021, 46, 62–71 Search PubMed.
- C. Ru, Z. Li and R. Tang, Sensors, 2019, 19, 2045 CrossRef CAS PubMed.
- L. Liu and H. Qu, J. Innovative Opt. Health Sci., 2019, 13, 1930014 CrossRef.
- D. K. Sarfo, A. Sivanesan, E. L. Izake and G. A. Ayoko, RSC Adv., 2017, 7, 21567–21575 RSC.
- I. J. Jahn, O. Zukovskaja, X. S. Zheng, K. Weber, T. W. Bocklitz, D. Cialla-May and J. Popp, Analyst, 2017, 142, 1022–1047 RSC.
- Y. Jiang, D.-W. Sun, H. Pu and Q. Wei, Trends Food Sci. Technol., 2018, 75, 10–22 CrossRef CAS.
- C. Zong, M. Xu, L. J. Xu, T. Wei, X. Ma, X. S. Zheng, R. Hu and B. Ren, Chem. Rev., 2018, 118, 4946–4980 CrossRef CAS PubMed.
- C. Song, B. Yang, Y. Yang and L. Wang, Sci. China: Chem., 2015, 59, 16–29 CrossRef.
- M. Fan, G. F. Andrade and A. G. Brolo, Anal. Chim. Acta, 2011, 693, 7–25 CrossRef CAS PubMed.
- L. E. Jamieson, S. M. Asiala, K. Gracie, K. Faulds and D. Graham, Annu. Rev. Anal. Chem., 2017, 10, 415–437 CrossRef CAS PubMed.
- Y. Y. Ma, W. Y. Li, E. C. Cho, Z. Y. Li, T. Yu, J. Zeng, Z. X. Xie and Y. N. Xia, ACS Nano, 2010, 4, 6725–6734 CrossRef CAS PubMed.
- K. Wang, D. W. Sun, H. Pu and Q. Wei, Talanta, 2019, 195, 506–515 CrossRef CAS PubMed.
- T. Wang, S. Wang, Z. Cheng, J. Wei, L. Yang, Z. Zhong, H. Hu, Y. Wang, B. Zhou and P. Li, Chem. Eng. J., 2021, 424 Search PubMed.
- H. Pu, Z. Huang, F. Xu and D. W. Sun, Food Chem., 2021, 343, 128548 CrossRef CAS PubMed.
- J. Huang, Y. Wen, J. Li, Y. Li, T. Gou, Y. Ma, Y. Qu, Z. Zhang, W. Ren, Z. Zhang, T. Liu and R. Sun, ACS Appl. Mater. Interfaces, 2020, 12, 37499–37505 CrossRef CAS PubMed.
- K. Lasić, A. Mornar and B. Nigović, Anal. Methods, 2020, 12, 2082–2092 RSC.
- T. J. N. Watson, R. Nosal, J. Lepore and F. Montgomery, J. Pharm. Innov., 2018, 13, 283–285 CrossRef.
- D. S. Metil, S. P. Sonawane, S. S. Pachore, A. Mohammad, V. H. Dahanukar, P. J. McCormack, C. V. Reddy and R. Bandichhor, Org. Process Res. Dev., 2017, 22, 27–39 CrossRef.
- L. Cai, B. Chen, F. Yi and S. Zou, Int. J. Biol. Macromol., 2019, 140, 907–919 CrossRef CAS PubMed.
- H. Zhang, J. Wang, Y. Chen, X. Shen, H. Jiang, X. Gong and J. Yan, J. Sep. Sci., 2019, 42, 1144–1154 CrossRef CAS PubMed.
- C. Y. Wang, X. C. Gong, Z. F. Fu and S. Q. Tong, China J. Chin. Mater. Med., 2021, 46, 2061–2066 Search PubMed.
- Y. Zhao, Y. Yamaguchi, Y. Ni, M. Li and X. Dou, Spectrochim. Acta, Part A, 2020, 233, 118193–118198 CrossRef CAS PubMed.
- Z. Q. Yan, L. Hu, L. Nie and H. Lv, Spectrochim. Acta, Part A, 2011, 79, 661–665 CrossRef CAS PubMed.
- Q. Da, Y. Y. Gu, X. F. Peng, L. Y. Zhang and S. H. Du, Microchim. Acta, 2018, 185, 357–365 CrossRef PubMed.
- X. Tan, X. B. Liu, H. J. Bai, X. D. Cui and T. Liu, J. Sichuan Univ., Nat. Sci. Ed., 2021, 58, 148–154 Search PubMed.
- T. Senapati, D. Senapati, A. K. Singh, Z. Fan, K. Rajashekhar and P. C. Ray, Chem. Comm., 2011, 47, 10326–10328 RSC.
Footnote |
† Electronic supplementary information (ESI) available. See DOI: 10.1039/d2ra01500e |
|
This journal is © The Royal Society of Chemistry 2022 |
Click here to see how this site uses Cookies. View our privacy policy here.