DOI:
10.1039/D1RA09339H
(Paper)
RSC Adv., 2022,
12, 7872-7882
Bioactive phytoconstituents as potent inhibitors of casein kinase-2: dual implications in cancer and COVID-19 therapeutics
Received
28th December 2021
, Accepted 19th February 2022
First published on 10th March 2022
Abstract
Casein kinase 2 (CK2) is a conserved serine/threonine-protein kinase involved in hematopoietic cell survival, cell cycle control, DNA repair, and other cellular processes. It plays a significant role in cancer progression and viral infection. CK2 is considered a potential drug target in cancers and COVID-19 therapy. In this study, we have performed a virtual screening of phytoconstituents from the IMPPAT database to identify some potential inhibitors of CK2. The initial filter was the physicochemical properties of the molecules following the Lipinski rule of five. Then binding affinity calculation, PAINS filter, ADMET, and PASS analyses followed by interaction analysis were carried out to discover nontoxic and better hits. Finally, two compounds, stylopine and dehydroevodiamines with appreciable affinity and specific interaction towards CK2, were identified. Their time-evolution analyses were carried out using all-atom molecular dynamics simulation, principal component analysis and free energy landscape. Altogether, we propose that stylopine and dehydroevodiamines can be further explored in in vitro and in vivo settings to develop anticancer and antiviral therapeutics.
Introduction
Cancer is one of the most common diseases found in almost every part of the globe.1 DNA damage is said to be the fundamental cause of sporadic cancers.2 It is also caused due to genomic instability, and a few are caused due to inherited genetic mutations.3–7 One of the extreme routes to cancer development and progression is the altered expression of the various genes, including kinases. The altered expression of kinases cause different cellular anomalies, leading to cancer progression. Casein kinase 2 (CK2) is a conserved serine/threonine protein kinases that attunes the many signaling pathways involved in hematopoietic cell survival and functions. Thus, it is considered as a promising drug target.8–11
CK2 is constitutively expressed in various cell types.10–12 It exists in mammalian cells, usually as a tetrameric complex consisting of two alpha subunits, CK2α (CSNK2A1) and CK2α' (CSNK2A2) and two regulatory subunits CK2β.13 CK2 regulates necessary cellular processes, which are typically deregulated in cancer cells. It mainly increases cell proliferation, growth, and survival. It also changes cell morphology, builds up the cellular transformation and stimulates angiogenesis.14,15 CK2 transcripts and proteins are both upregulated in various forms of cancer, as suggested by several studies.12 In studies of the most common five cancer types (breast, lung, prostate, colon, and ovarian), CK2 transcript expression was upregulated.16,17
Studies suggest that transcriptional mechanisms can enhance CK2 in human tumors.18,19 In animal models, CK2's ability to promote tumors mainly because it regulates signal transduction pathways, which vary in different cancers. CK2 regulates various signal transduction cascades like Wnt signaling,20 Hedgehog signaling,21 NF-kB,22 JAK/STAT,23 and PTEN/PI3K/Akt-PKB.24 Tumorigenesis caused by the modulation of various signaling pathways, cascades and protein kinases. CK2 is associated with cancer development and thus its selective targeting can be helpful in therapeutic development of anticancer drugs.
In a recent study, CK2 is reported as a most strongly regulated kinases in SARS-CoV-2 infection.25 During the infection, CK2 regulates actin tail formation, which enables the efficiency of the virus to spread through cell-to-cell.25 One of the CK2 inhibitors under clinical trials named silmitasertib (CX-4945) showed potent antiviral activity, which suggests the role of CK2 to regulate the life cycle of SARS-CoV-2.25 The inhibitor of CK2 promotes the formation of street granules which ultimately can prevent viruses from gaining nucleic acids to make a new virus. Altogether, CK2 is a positive regulator of cancer progression and is involved in SARS-CoV-2 infection. Utilizing CK2 as an attractive target for developing anticancer and antiviral therapeutics, exploring natural compounds against CK2 is an attractive strategy.
Plant-based natural products are the sources of new drugs which play an essential role in various pharmaceutical industries.26–33 India is one of those countries which practices traditional medicines and ethnopharmacology. The phytochemicals of medicinal plants encircle a manifold of chemical space for drug design and discovery. IMPPAT (Indian medicinal plant, phytochemistry and therapeutics) is a manually curated online database of plant-based natural compounds. It consists of more than 1700 Indian medicinal plants, approximately 10
000 phytochemicals of around 1100 therapeutic uses.34
This study used a structure-based virtual screening approach to screen the plant-based natural compounds obtained from the IMPPAT database. First, the database was filtered out based on the physicochemical properties of the compounds, and then a molecular docking-based screen was performed to get high-affinity binding partners of CK2. ADMET analysis followed by PASS analysis of the selected compounds was then performed. From the top hits generated, we have further screened the compounds based on their specific interactions towards the CK2 binding pocket, followed by molecular dynamics (MD) simulations for 100 ns to know the conformational dynamics and stability of identified compounds with CK2.
Materials and methods
Computational resources
Bioinformatics software, including, InstaDock,35 Discovery Studio Visualizer,36 GROMACS37 and PyMOL,38 were used for a systematic drug design and discovery approach based on molecular docking and simulation studies. SwissADME,39 pkCSM server,40 RCSB-Protein Data Bank (PDB), QtGrace,41 etc., were used for data retrieval and evaluation. The three-dimensional structural coordinates of CK2 protein were retrieved from PDB (PDB ID: 6QY9). All co-crystallized ligand and co-crystallized hetero atoms, including water molecules, were removed from the original coordinates. The CK2 structure was prepared by remodeling missing residues, adding hydrogen atoms to polar groups and assigning suitable atom types for virtual screening in InstaDock. A library of ∼6000 compounds was taken from the IMPPAT database following the Lipinski rule of five.
Molecular docking-based screening
Molecular docking is used to predict the suitable orientation and binding affinity of small molecules to a receptor, typically proteins.42–44 A virtual screening process employing the molecular docking approach was used to find the best conformational pose and binding affinity of selected compounds towards CK2.45–49 InstaDock with blind search space with a grid size of 70 Å, 80 Å, and 80 Å for X, Y, Z coordinates, respectively, were used in this study for protein–ligand docking. The center of the grid was set to X: 13.29, Y: −0.40, Z: 0.90 axes. The grid spacing was set to 1 Å, and the other docking parameters were set to their default values. InstaDock software was used to calculate and analyze the binding affinities and other docking parameters of the phyto-compounds towards CK2. The docking output was filtered based on the energy values and ligand efficiency. The docking poses of each phytocompounds were generated for the interaction analysis with the help of Discovery Studio Visualizer and PyMOL.
ADMET properties
After getting the high-affinity binding partners of CK2 from the molecular docking approach, ADMET properties of the filtered compounds were calculated with the help of SwissADME and pkCSM servers. Secondly, to avoid the compounds with specific patterns that show a higher tendency to bind with multiple targets, we applied the PAINS (pan-assay interference compounds) filter.50 Compounds showing thriving ADMET and drug-like properties without toxic patterns were selected for further analysis.
Biological activity prediction
Biological activity prediction of compounds is one of the main components in drug discovery. The PASS webserver51 was used to predict the biological properties of the selected compounds from the ADMET filter. PASS web server predicts the biological properties based on the compound's structure–activity-relationship. It gives a brief record of potential biological properties on account of Pa (probability of being active) and Pi (probability of being inactive) ratio by comparing the query structure with the inbuilt data set of known compounds having different biological properties. It indicates that the higher the Pa value, the higher the possibility of a biological property of a compound being studied.
Interaction analysis
The interaction analysis between CK2 and the selected ligands from the PASS analysis was carried out to explore different bonds and forces involved in binding. For the possible conformations of the filtered compounds, the out-files of docked compounds were generated and then explored with the help of Discovery Studio Visualizer and PyMOL. In PyMOL, the closed interactions described within 3.5 Å were recorded as polar contacts between the compounds and CK2. The detailed interactions between the compounds with the CK2 binding pocket were explored using Discovery Studio Visualizer. Only those compounds found to interact with the critical residues of the CK2 binding pocket were selected. Moreover, the compounds showing the close interaction with the binding site residues of CK2 were selected for further analysis. To compare our docking results, the binding site and docking pose of a co-crystallized known CK2 inhibitor pyrrolo[2,3-d]pyrimidinyl was taken as the reference (PDB ID: 6QY9).
MD simulations
MD simulation is used to study the atomic motions of protein and protein–ligand systems.48,52–57 The results obtained from docking of interaction between CK2 and the selected compounds (stylopine and dehydroevodiamines) were corroborated through MD simulation studies. To simulate the structural coordinates of CK2, its complexes with stylopine and dehydroevodiamines, we used GROMACS v5.5.1. It is an open-source MD simulation program widely utilized in drug discovery. The receptor–ligand complex topologies were created by parametrizing the compounds through the PRODRG server.58 The PRODRG server is a reliable tool for generating topology coordinates of small molecules. Each system was placed with a 10 Å distance in the cubic box center to the edges for solvation in the simple point charge (SPC216) water model.
Furthermore, the simulation systems were neutralized by adding an appropriate amount of counterions (Na+ and Cl−). The possible steric hindrances between the atoms were removed by energy minimization in the solvated system with the 1500 steepest descent followed by conjugate gradient methods. At constant volume with gradual heating from (0–300 K) temperature and at a pressure of 1 atm, the two-step equilibration under the periodic boundary setting was carried out for 100 ps. Simulation for all the systems was done for 100 ns. The protein–ligand stability was checked by analyzing the resultant data with the help of the GROMACS tools as described in our previous reports.42,44,59,60
Principal component analysis and essential dynamics
Data dimensionality can be minimized while retaining most of the variation in the data set by using a widely used mathematical algorithm known as principal component analysis (PCA).61 This reduction is accomplished by identifying directions, called principal components (PCs), along which the data variation is maximal. It uses only a few components to represent each sample using relatively few numbers instead of using values for thousands of variables. Then the sample can be plotted, making it possible to vividly assess similarities and differences between samples and determine whether the sample can be grouped. Moreover, PCA uncovers the motion of high amplitude in MD trajectories.61 We have explored the MD trajectories of CK2, CK2-stylopine and CK2-dehydroevodiamines complexes for conformational sampling, atomic motions and stability through PCA and free energy landscape (FEL) analyses.62
Result and discussion
Molecular docking-based screening
Virtual screening is a commonly used computational method to identify potential compounds against pre-defined biological targets.44,63 It is an important technique that also reduces experimental efforts and time consumption. We used molecular docking-based virtual screening to identify small molecules having appreciable binding scores with CK2. From the docking analysis, we have initially selected the top 10 compounds based on their significant binding affinities by using InstaDock software. The top 10 hits from ∼6000 compounds had a binding affinity score ≤−12.3 with CK2 (Table 1). The known CK2 inhibitor, silmitasertib was found to possess an appreciable binding affinity and ligand efficiency (LE) towards CK2.
Table 1 List of selected hits and their docking score with CK2
S. no. |
Compound ID |
Compound |
Source |
Affinity (kcal mol−1) |
pKi |
LEa |
LE, ligand efficiency in values in kcal per mol per non-H atom. |
1 |
5315739 |
N-Acetyldehydroanonaine |
Zanthoxylum nitidum |
−13.4 |
9.83 |
0.52 |
2 |
443716 |
Hydroxysanguinarine |
Fumaria indica |
−13.3 |
9.75 |
0.46 |
3 |
440583 |
Stylopine |
Fumaria indica |
−13.0 |
9.53 |
0.48 |
4 |
147329 |
Corysamine |
Meconopsis aculeata |
−12.6 |
9.24 |
0.45 |
5 |
2754650 |
Irenolone |
Musa paradisiaca |
−12.6 |
9.24 |
0.50 |
6 |
161899 |
Dehydroroemerine |
Nelumbo nucifera |
−12.5 |
9.17 |
0.52 |
7 |
6453733 |
L-N-Acetylanonaine |
Zanthoxylum nitidum |
−12.5 |
9.17 |
0.48 |
8 |
9817839 |
Dehydroevodiamines |
Agrimonia pilosa |
−12.5 |
9.17 |
0.48 |
9 |
146680 |
Elliptinone |
Plumbago zeylanica |
−12.3 |
9.02 |
0.40 |
10 |
633072 |
Chitranone |
Plumbago zeylanica |
−12.3 |
9.02 |
0.40 |
11 |
CX-4945 |
Silmitasertib |
— |
−10.2 |
7.48 |
0.36 |
12 |
SL-2052 |
Wortmannin |
Penicillium funiculosum |
−7.2 |
5.28 |
0.23 |
Interestingly, the elucidated compounds from our study are showing better docking scores than the silmitasertib. Wortmannin, an inhibitor of PI 3-kinase that does not inhibit CK2, was selected as a negative control to compare with the elucidated compounds.64 Wortmannin doesn't show decent binding affinity and LE towards CK2 compared to elucidated compounds and positive control silmitasertib (Table 1). This comparison supports the validity of the docking output for the identified compounds towards CK2. The results show that all the selected hits have appreciable binding affinity and LE values.
ADMET properties of compounds
The selected hits from the docking study were further screened to predict their ADMET properties. pkCSM and SwissADME web servers were used to calculate the ADMET properties of the top 10 selected compounds from the docking filter. PAINS patterns were also checked for all the compounds to avoid any hazardous pattern in the compound's molecular structure. ADMET prediction consists of a set of parameters and the threshold values on which the physicochemical and pharmacokinetic properties of chemical compounds are to be depicted. Here similar kinds of properties are shown by all the compounds. It was found that both stylopine and dehydroevodiamines have a set of desired physicochemical properties without any AMES toxicity and PAINS patterns (Table 2). The two compounds out of 10 having ADMET within the range of drug candidacy were selected. Stylopine and dehydroevodiamines share a similar class of ADMET properties without any toxic patterns thus were selected for further analysis. Both compounds share similar properties as the established leads silmitasertib and wortmannin (Table 2).
Table 2 ADMET properties of the top 10 hits
Compound |
Absorption |
Distribution |
Metabolism |
Excretion |
Toxicity |
GI absorption (%) |
Water solubility |
BBB/CNS permeation |
CYP2D6 inhibitor |
OCT2 substrate |
AMES |
Acetyldehydroanonaine |
98.82 |
−5.01 |
No |
No |
No |
Yes |
Hydroxysanguinarine |
100.00 |
−33.25 |
No |
No |
No |
Yes |
Stylopine |
94.37 |
−2.70 |
No |
Yes |
No |
No |
Corysamine |
85.01 |
−2.89 |
No |
No |
No |
Yes |
Irenolone |
91.52 |
−4.61 |
No |
No |
No |
Yes |
Dehydroroemerine |
98.22 |
−5.68 |
No |
No |
No |
Yes |
L-N-Acetylanonaine |
96.87 |
−3.80 |
No |
No |
No |
Yes |
Dehydroevodiamines |
99.69 |
−3.79 |
No |
No |
Yes |
No |
Elliptinone |
93.49 |
−3.99 |
No |
No |
No |
Yes |
Chitranone |
91.26 |
−4.38 |
No |
No |
No |
Yes |
Silmitasertib |
99.25 |
−3.30 |
No |
Yes |
No |
No |
Wortmannin |
100.00 |
−4.08 |
No |
No |
No |
No |
Biological activities of selected compounds
Biological activities should be adequately studied to design safe and effective therapeutic molecules. Such an estimation can be performed by using the PASS analysis approach with the help of machine learning based on the structure–activity relationship. We used the PASS webserver to explore the biological activities of the selected compounds. Based on multiple mechanisms of action, drug–drug interaction, and activity–activity, the compounds can be selected using the PASS analysis approach. The predictions for anticarcinogenic, antiviral and kinase inhibitory potential have been shown by the compound stylopine and dehydroevodiamines with the Pa values ranging from 0.291 to 0.598 when Pa is greater than Pi. The biological properties of stylopine and dehydroevodiamines are shown in Table 3. We also predicted the biological properties of silmitasertib and wortmannin for validation. Silmitasertib and wortmannin show HK2 inhibitory and phosphatidylinositol kinase inhibitory potential, respectively, for which they are known, validated the PASS prediction (Table 3). Finally, compounds stylopine and dehydroevodiamines were selected for MD simulation studies with CK2 based on binding studies, specific interactions toward CK2 binding site and PASS analysis.
Table 3 Biological activities of the selected compounds predicted through the PASS webserver
S. no. |
Compound ID |
Pa |
Pi |
Biological activity |
1 |
Stylopine |
0.598 |
0.004 |
Antineoplastic alkaloid |
0.529 |
0.017 |
Antihypertensive |
0.449 |
0.017 |
Antineoplastic (lung cancer) |
0.391 |
0.101 |
Antiviral (rhinovirus) |
0.334 |
0.064 |
Antiviral (adenovirus) |
2 |
Dehydroevodiamines |
0.478 |
0.008 |
Antineoplastic alkaloid |
0.406 |
0.037 |
Diabetic neuropathy treatment |
0.389 |
0.032 |
MAP3K5 inhibitor |
0.431 |
0.087 |
Antiviral (picornavirus) |
0.291 |
0.026 |
Antineoplastic (non-small cell lung cancer) |
3 |
Silmitasertib |
0.731 |
0.025 |
Taurine dehydrogenase inhibitor |
0.686 |
0.008 |
Protein kinase inhibitor |
0.533 |
0.010 |
Tyrosine kinase inhibitor |
0.498 |
0.072 |
Antineoplastic |
0.320 |
0.005 |
Protein kinase (CK2) inhibitor |
4 |
Wortmannin |
0.995 |
0.001 |
Phosphatidylinositol kinase inhibitor |
0.972 |
0.002 |
Lipid metabolism regulator |
0.890 |
0.003 |
Prostate cancer treatment |
0.870 |
0.005 |
Antineoplastic |
0.521 |
0.018 |
Antiviral (rhinovirus) |
Interaction analysis
The interaction analysis was performed by splitting all the possible docking conformations from the out files of the docked stylopine and dehydroevodiamines. The analysis found that stylopine and dehydroevodiamines interact with common residues, including the ATP binding site of CK2 Lys69. Also, it is the site where the co-crystallized available CK2 inhibitor pyrrolo[2,3-d]pyrimidinyl bound (PDB ID: 6QY9) is bound. An explained binding pattern of stylopine and dehydroevodiamines along with silmitasertib and wortmannin is illustrated in Fig. 1. The figure shows that stylopine and dehydroevodiamines interact with Lys69 (ATP binding site) of CK2, which is crucial for its activity (Fig. 1B). The structural representation shows that stylopine and dehydroevodiamines are bound into the deep binding pocket cavity of CK2 with a virtuous complementarity where silmitasertib also interacts (Fig. 1C). At the same time, wortmannin was interacting with the CK2 binding cavity but could not be accommodated into the inner core of the binding site. It is loosely bound to the pocket without any close interaction with the critical residues of the CK2 binding site.
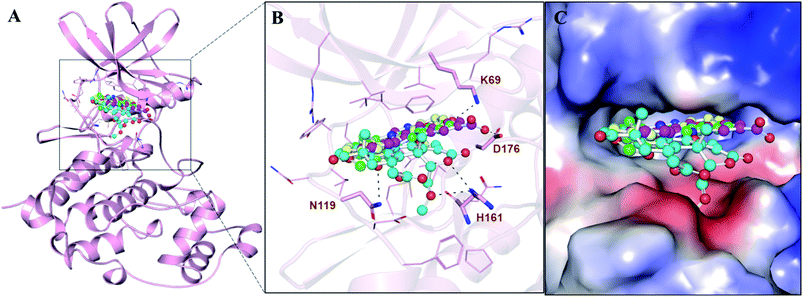 |
| Fig. 1 Molecular interactions of (A) casein kinase 2 with stylopine (green), dehydroevodiamines (yellow), silmitasertib (magenta), and wortmannin (cyan). (B) cartoon view of protein–ligands interactions. (C) Electrostatic potential of casein kinase 1 bound the selected compounds and controls. | |
The detailed analysis of both compounds was further explored for their interactions with the active/critical site residue of CK2. It is clear from Fig. 2 that the stylopine, dehydroevodiamines and silmitasertib share common interactions while interacting with the ATP-binding site residue of CK2. The ATP-binding site is essential for the functional activities of any kinase. The ATP-binding site is located at the main catalytic center of CK2, where stylopine, dehydroevodiamines and silmitasertib are binding (Fig. 2A–C), suggesting stylopine and dehydroevodiamines could act as the potential ATP competitive inhibitor of CK2. While wortmannin does not shows any close interaction with the critical residues of the CK2 binding site, rejecting its candidacy to be a potential binding partner of CK2 (Fig. 2D).
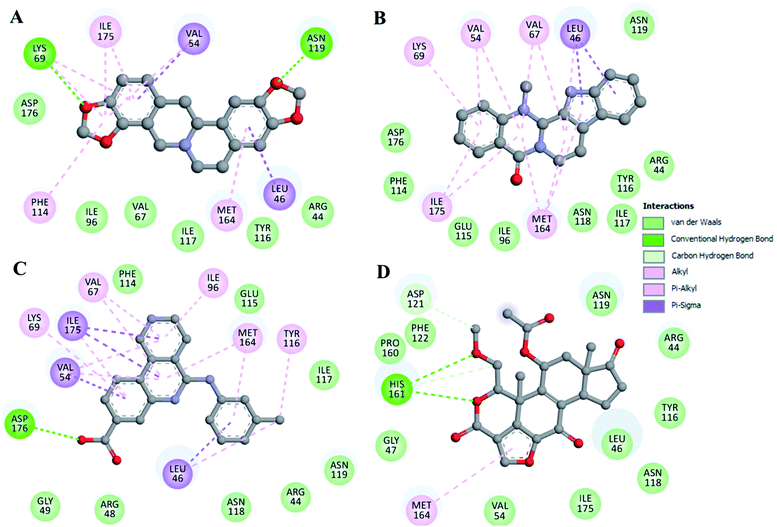 |
| Fig. 2 Representation of molecular interaction and 2D plots showing detailed interactions of (A) stylopine, (B) dehydroevodiamines, (C) silmitasertib, and (D) wortmannin. | |
MD simulation
MD simulation is one of the widely used methods to study structural details and dynamic behavior of protein–ligand complexes. CK2-stylopine, CK2-dehydroevodiamines and the apo CK2 are the three systems studied in MD simulations for 100 ns. The stability and dynamics of CK2 in complexes with stylopine and dehydroevodiamines were analyzed by exploring various systematic and structural parameters discussed below.
Structural dynamics and compactness
The structural dynamics in proteins can be altered after binding with the ligands. Root-mean-square deviation (RMSD) parameter is used to see the structural deviation in proteins over a period. It is one of the most valuable methods to investigate a protein structure's structural changes and dynamics.65,66 We have found the average value of RSMDs as 0.34 nm, 0.36 nm and 0.35 nm for CK2 alone, CK2-stylopine and CK2-dehydroevodiamines, respectively. The binding of stylopine and dehydroevodiamines with CK2 equilibrated throughout the simulation as indicated by RMSD, suggesting fair stability of docked complexes. From the initial simulation stage to the 100 ns trajectory, all three systems are significantly stable, as shown by the RMSD plot (Fig. 3A). A slight fluctuation was seen in RMSD, especially for the CK2-stylopine complex, but without any shift. It was seen that the RMSD of all the systems has stabilized and balanced during the entire simulation period of 100 ns. The distribution of RMSD values was plotted as probability distribution function (PDF), which illustrates the stabilization in CK2 dynamics with higher probability over compounds binding (Fig. 3A, lower panel).
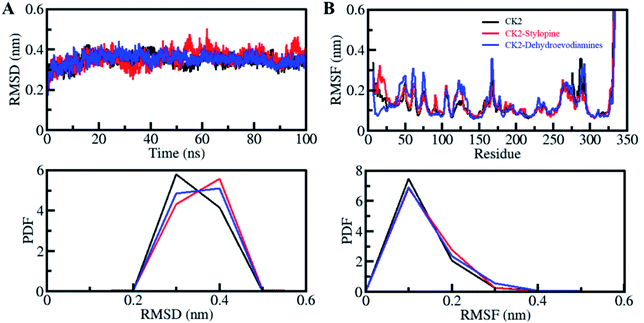 |
| Fig. 3 Structural dynamics of CK2 upon stylopine and dehydroevodiamines binding. (A) RMSD plot of CK2 in complexed with stylopine and dehydroevodiamines. (B) RMSF plot of CK2 and its complex with stylopine and dehydroevodiamines. Lower panels show the probability distribution function of values as PDF. | |
Root-mean-square fluctuation (RMSF) is helpful in measuring residual vibrations in the protein structures during MD simulation. The residual dynamics of a CK2 can be seen before and after ligands binding by plotting the RMSF of each residue (Fig. 3B). Minimization and stabilization in RMSF fluctuations upon dehydroevodiamines binding suggest incredible constancy of the protein–ligand system. While after stylopine, the binding marginally increased in residual vibrations at some places, which signifies higher dynamics local to the loop region. Comparatively, CK2-dehydroevodiamines complex is more stable than CK2-stylopine, as predicted from RMSD and RMSFs. A decreased residual fluctuation in CK2 over compounds binding was also shown by PDF (Fig. 3B, lower panel).
The radius of gyration (Rg) is directly proportional to the alterations in protein structure. Rg is defined as the RMS distance from the set of atoms from their collective center of mass. Rg parameters is used to study the compactness of a protein structure.65 Here, Rg values were calculated to evaluate the compactness of CK2 before and after binding with stylopine and dehydroevodiamines (Fig. 4A). A small increment in the Rg value of CK2 was observed after the binding of stylopine, which agrees with the RMSD and RMSF values. As suggested by the Rg plot, CK2 was stable with both compounds. In the PDF analysis, there is no change in the average Rg value of CK2 upon dehydroevodiamines binding (Fig. 4A, lower panel).
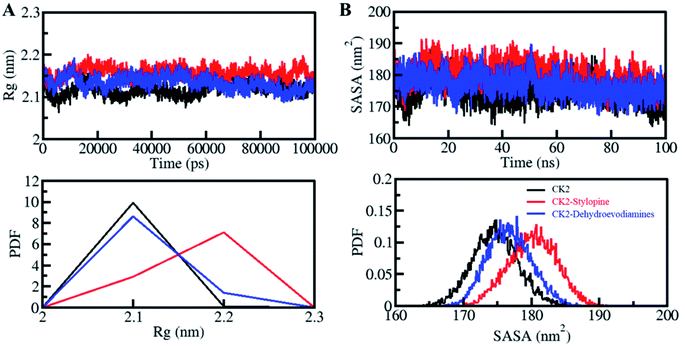 |
| Fig. 4 Structural compactness and folding of CK2 upon stylopine and dehydroevodiamines binding. (A) Rg plot and (B) SASA plot of CK2 with stylopine and dehydroevodiamines. Lower panels show the probability distribution function values as PDF. | |
The solvent-accessible surface area (SASA) values calculated to see the structural changes in protein while binding to a ligand.67,68 SASA analysis can be done to examine the stability and folding behavior of protein and its complexes.69 While studying the SASA plot, we noticed a minimal change in SASA values throughout the simulation time, which suggests that the complexes of CK2 with stylopine and dehydroevodiamines are stable (Fig. 4B). Lose packing of CK2, primarily upon stylopine binding, is the significance of a slight increase of the average value of SASA and without any disturbance of the overall protein folding. Moreover, after the compound binding, especially after stylopine binding, a minor increment in average SASA of CK2 was suggested by PDF analysis (Fig. 4B, lower panel).
Dynamics of hydrogen bonds
The formation of hydrogen bonds (H-bonds) is essential for formation of a stable protein-ligand complex. The time evolution of H-bonds was explored to evaluate the constancy of intramolecular bonding in CK2 before and after stylopine and dehydroevodiamines binding. As advocated by the plot generated, there was no significant change in the number of H-bonds observed within CK2 when complexed with stylopine and dehydroevodiamines. In CK2, intramolecularly, the average H-bonds formed before and after stylopine and dehydroevodiamines binding were evaluated to be 260, 255, and 259, respectively (Fig. 5, left panel). A slight decrease in H-bonds was noticed owing to the ligands' occupation of some intramolecular space in CK2. A fair constancy has shown by the PDF calculated for intramolecular H-bonds in all three systems (Fig. 5, right panel). The intramolecular H-bonds in CK2 show stability throughout the simulation, even after the binding of compounds.
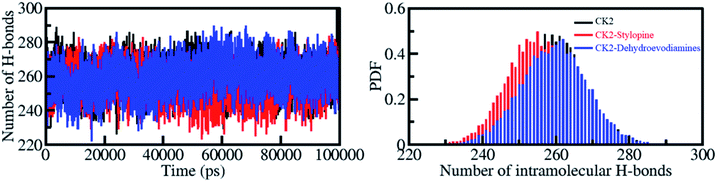 |
| Fig. 5 Hydrogen bond analysis. Time evolution of intramolecular H-bonds (left panel). The right panel shows the PDF of the hydrogen bonds distribution. | |
Moreover, the time evaluation of intramolecular H-bonds was further explored to determine the constancy of H-bonding in between the stylopine and dehydroevodiamines with CK2. Within the stylopine-CK2 and dehydroevodiamines-CK2, the average H-bonds formed were estimated to be 2 in each complex (Fig. 6, upper panel). With the higher PDF value and the average number of H-bonding as 2, the PDF suggested a fair constancy for intramolecular H-bonds in both systems (Fig. 6, lower panel). The stylopine and dehydroevodiamines haven't moved from their initial docking position on CK2 as predicted from the intermolecular H-bonding, which stabilizes the complex structures.
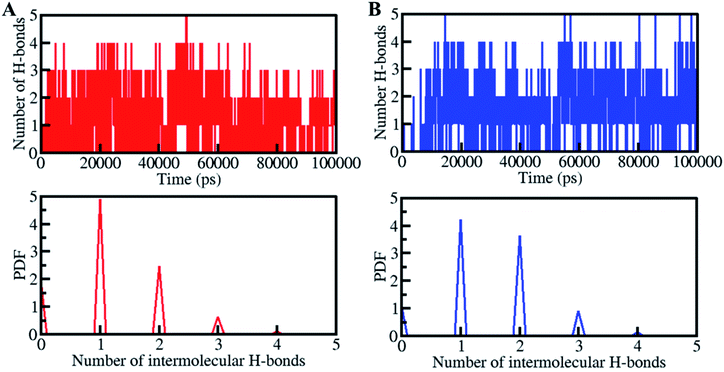 |
| Fig. 6 Time evolution of intermolecular H-bonds formed within 0.35 nm between CK2 and (A) stylopine and (B) dehydroevodiamines. The lower panels show the PDF of the hydrogen bonds distribution. | |
PCA and FELs analysis
PCA is a valuable method to examine the collective motions and conformational sampling in proteins. We have employed the essential dynamics approach to exploring the collective motions and conformational sampling of CK2, CK2-stylopine and CK2-dehydroevodiamines complexes. The conformational sampling of CK2, CK2-stylopine and CK2-dehydroevodiamines in the subspace is illustrated in Fig. 7A. The conformational sampling of CK2 and two different EVs projected by the protein Cα atoms, as displayed by the projection. The clusters of free CK2 were covered by the projection of conformational sampling by determining the phase space performance of CK2-stylopine and CK2-dehydroevodiamines projected by C-alpha atoms. The analysis shows that the CK2-stylopine and CK2-dehydroevodiamines complexes occupied the same conformational space as the free CK2. It has also been noticed that the lesser flexibility, conformational space covered by the CK2-dehydroevodiamines complex make it more stable than the CK2-stylopine complex Fig. 7B.
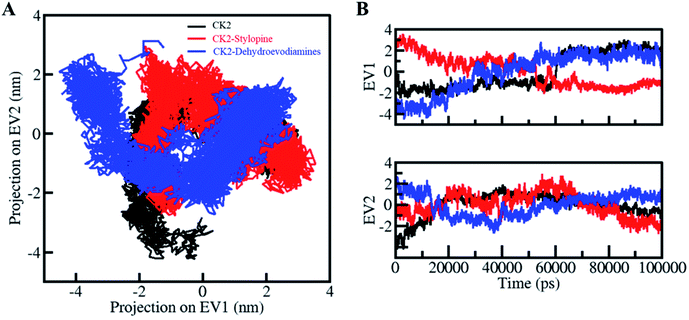 |
| Fig. 7 Principal component analysis. (A) 2D conformational projections of CK2, CK2-stylopine and CK2-dehydroevodiamines. (B) The time evolution of CK2 projections on both EVs. | |
By using the first two PCs, we have generated the FELs to visualize the energy minima and conformational landscape of the free CK2 and CK2-stylopine and CK2-dehydroevodiamines complexes. The outlined FELs of CK2, CK2-stylopine and CK2-dehydroevodiamines complexes are depicted in Fig. 8. The size and position of the phase confined within a single stable global minimum were slightly disturbed by the binding of CK2 with stylopine and dehydroevodiamines, as suggested by the FEL plots (Fig. 8).
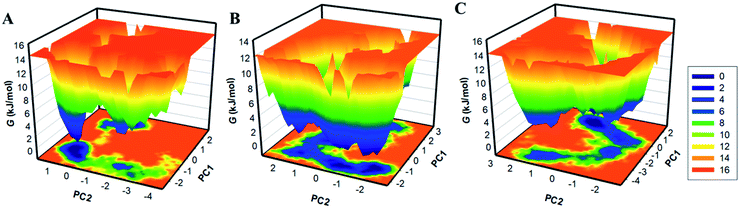 |
| Fig. 8 The free energy landscapes of (A) free CK2, (B) CK2-stylopine, and (C) CK2-dehydroevodiamines. | |
Deeper blue in FELs signifies the protein conformation with lower energy near-native states. We see that CK2 has a single global minimum overall confined within a single basin Fig. 8A. Likewise, while binding with stylopine and dehydroevodiamines, CK2 acquires different states with multiple basins but overall global minima (Fig. 8B and C). Overall, results suggested that the binding of stylopine and dehydroevodiamines with CK2 doesn't lead to the unfolding of CK2 during the simulation.
Conclusions
The pharmacologic inhibition of CK2 possesses anticancer and antiviral efficacy, representing potential cancer and COVID-19 therapies. This work aims to contribute to the therapeutic management of cancer and COVID-19 by using natural compounds targeting CK2. Utilizing CK2 as an attractive target for developing anticancer and antiviral therapeutics, we have carried out an in silico analysis using state-of-the-art computational approaches. Two phytoconstituents, stylopine and dehydroevodiamines, were identified by assessing their physicochemical and drug-like properties and stable binding towards CK2. We propose that stylopine and dehydroevodiamines be further explored in in vitro and in vivo settings to develop anticancer and antiviral therapeutics.
Funding
This work was supported by Taif University Researchers Supporting Project Number (TURSP-2020/131), Taif University, Taif, Saudi Arabia.
Author contributions
Conceptualization, A. S., F. A., and T. M.; methodology, F. A. A., S. A. A., T. M.; software, F. A., G. M. A., A. S. B., M. N. S., M. I. H.; validation, G. M. A., D. K. Y. and F. A.; formal analysis, A. S.; F. A. A., S. A. A., G. M. A. investigation, T. M., M. N. S.; resources, F. A., D. K. Y.; data curation, T. M., M. N. S.; writing—original draft preparation, A. S., M. N. S., D. K. Y., F. A., T. M.; writing—review and editing, F. A. A., S. A. A., G. M. A., M. I. H.; visualization, F. A., G. M. A., A. S. B.; supervision, A. S., D. K. Y., M. I. H.; project administration, D. K. Y., F. A.; funding acquisition, D. K. Y., A. S. All authors have read and agreed to the published version of the manuscript.
Conflicts of interest
The authors declare no conflict of interest.
Acknowledgements
This work was supported by Taif University Researchers Supporting Project Number (TURSP-2020/131), Taif University, Taif, Saudi Arabia. The Deanship of Scientific Research (DSR) at King Abdulaziz University, Jeddah, Saudi Arabia, has funded this project under grant no. KEP-36-130-42. The authors therefore, acknowledge with thanks DSR technical and financial support. MIH acknowledges the Council of Scientific and Industrial Research for financial support [Project No. 27(0368)/20/EMR-II] for financial support.
References
- H. Sung, J. Ferlay, R. L. Siegel, M. Laversanne, I. Soerjomataram, A. Jemal and F. Bray, Ca-Cancer J. Clin., 2021, 71, 209–249 CrossRef PubMed.
- N. Turner, A. Tutt and A. Ashworth, Nat. Rev. Cancer, 2004, 4, 814–819 CrossRef CAS PubMed.
- S. Negrini, V. G. Gorgoulis and T. D. Halazonetis, Nat. Rev. Mol. Cell Biol., 2010, 11, 220–228 CrossRef CAS PubMed.
- J. S. Lee, H. Hwang, S. Y. Kim, K. J. Kim, J. S. Choi, M. J. Woo, Y. M. Choi, J. K. Jun, B. C. Lim and J. H. Chae, Ann. Lab. Med., 2018, 38, 473–480 CrossRef PubMed.
- H. J. Baek, S. E. Kim, E. K. Choi, J. K. Kim, D. H. Shin, E. J. Park, T. H. Kim, J. Y. Kim, K. G. Kim, C. X. Deng and S. S. Kim, Int. J. Biol. Sci., 2018, 14, 1755–1768 CrossRef CAS PubMed.
- J. Bae, H. S. Choi, S. Y. Park, D. E. Lee and S. Lee, Endocrinol. Metab., 2018, 33, 252–259 CrossRef CAS PubMed.
- S. Lee, M. Mannstadt, J. Guo, S. M. Kim, H. S. Yi, A. Khatri, T. Dean, M. Okazaki, T. J. Gardella and H. Juppner, J. Bone Miner. Res., 2015, 30, 1803–1813 CrossRef CAS PubMed.
- J. K. Cheong and D. M. Virshup, Int. J. Biochem. Cell Biol., 2011, 43, 465–469 CrossRef CAS PubMed.
- S. Sarno, S. Moro, F. Meggio, G. Zagotto, D. Dal Ben, P. Ghisellini, R. Battistutta, G. Zanotti and L. A. Pinna, Pharmacol. Ther., 2002, 93, 159–168 CrossRef CAS.
- N. N. Q. Tran and K. H. Chun, Molecules, 2021, 26, 4747 CrossRef CAS PubMed.
- H. J. Kim, D. Y. Kim and H. G. Cheon, Eur. J. Pharmacol., 2019, 861, 172596 CrossRef CAS PubMed.
- M. Ruzzene, G. Di Maira, K. Tosoni and L. A. Pinna, Methods Enzymol., 2010, 484, 495–514 CAS.
- A. Baier, J. Nazaruk, A. Galicka and R. Szyszka, Mol. Cell. Biochem., 2018, 444, 35–42 CrossRef CAS PubMed.
- H. Lian, M. Su, Y. Zhu, Y. Zhou, S. H. Soomro and H. Fu, Asian Pac. J. Cancer Prev., 2019, 20, 23 CrossRef CAS PubMed.
- T. Nuñez de Villavicencio-Diaz, A. J. Rabalski and D. W. Litchfield, Pharmaceuticals, 2017, 10, 27 CrossRef PubMed.
- C. E. Ortega, Y. Seidner and I. Dominguez, PLoS One, 2014, 9, e115609 CrossRef PubMed.
- M. M. Chua, M. Lee and I. Dominguez, PLoS One, 2017, 12, e0188854 CrossRef PubMed.
- D. Mottet, S. P. D. Ruys, C. Demazy, M. Raes and C. Michiels, Int. J. Cancer, 2005, 117, 764–774 CrossRef CAS PubMed.
- K. Ahmed, D. A. Gerber and C. Cochet, Trends Cell Biol., 2002, 12, 226–230 CrossRef CAS PubMed.
- D. H. Song, D. J. Sussman and D. C. Seldin, J. Biol. Chem., 2000, 275, 23790–23797 CrossRef CAS PubMed.
- D. Wu, C. Sui, F. Meng, X. Tian, L. Fu, Y. Li, X. Qi, H. Cui, Y. Liu and Y. Jiang, Acta Histochem., 2014, 116, 1501–1508 CrossRef CAS PubMed.
- I. Dominguez, G. Sonenshein and D. Seldin, Cell. Mol. Life Sci., 2009, 66, 1850–1857 CrossRef CAS PubMed.
- Y. Zheng, H. Qin, S. J. Frank, L. Deng, D. W. Litchfield, A. Tefferi, A. Pardanani, F.-T. Lin, J. Li and B. Sha, Blood, 2011, 118, 156–166 CrossRef CAS PubMed.
- X. Chen, C. Li, D. Wang, Y. Chen and N. Zhang, Molecules, 2020, 25, 870 CrossRef CAS PubMed.
- M. Bouhaddou, D. Memon, B. Meyer, K. M. White, V. V. Rezelj, M. C. Marrero, B. J. Polacco, J. E. Melnyk, S. Ulferts and R. M. Kaake, Cell, 2020, 182, 685–712 CrossRef CAS PubMed.
- A. K. Shakya, Int. J. Tradit. Herb. Med., 2016, 4, 59–64 Search PubMed.
- A. K. Mukherjee, S. Basu, N. Sarkar and A. C. Ghosh, Curr. Med. Chem., 2001, 8, 1467–1486 CrossRef CAS PubMed.
- T. A. Trinh, J. Park, J. H. Oh, J. S. Park, D. Lee, C. E. Kim, H. S. Choi, S. B. Kim, G. S. Hwang, B. A. Koo and K. S. Kang, Biomolecules, 2020, 10, 424 CrossRef CAS PubMed.
- Y. B. Lee, J. A. Lee and H. L. Lee, J. Altern. Complementary Med., 2020, 26, 976–999 CrossRef PubMed.
- J. H. Hwang and J. Ku, Medicine, 2020, 99, e20779 CrossRef PubMed.
- Y. Ko, B. H. Jang, M. S. Oh, S. J. Kim, Y. Y. Cha, E. J. Lee, Y. K. Song and S. G. Ko, Medicine, 2019, 98, e16466 CrossRef PubMed.
- J. Kim, H. Y. Kim, S. Hong, S. Shin, Y. A. Kim, N. S. Kim and O. S. Bang, Biomed. Pharmacother., 2019, 116, 108987 CrossRef CAS PubMed.
- J. Y. Choi, E. Y. Kwon and M. S. Choi, J. Med. Food, 2019, 22, 928–936 CrossRef CAS PubMed.
- K. Mohanraj, B. S. Karthikeyan, R. Vivek-Ananth, R. B. Chand, S. Aparna, P. Mangalapandi and A. Samal, Sci. Rep., 2018, 8, 1–17 CAS.
- T. Mohammad, Y. Mathur and M. I. Hassan, Briefings Bioinf., 2021, 22, bbaa279 CrossRef PubMed.
- D. Studio, Accelrys [2.1], 2008 Search PubMed.
- D. Van Der Spoel, E. Lindahl, B. Hess, G. Groenhof, A. E. Mark and H. J. Berendsen, J. Comput. Chem., 2005, 26, 1701–1718 CrossRef CAS PubMed.
- W. L. DeLano, CCP4 Newsletter on protein crystallography, 2002, vol. 40, pp. 82–92 Search PubMed.
- A. Daina, O. Michielin and V. Zoete, Sci. Rep., 2017, 7, 1–13 CrossRef PubMed.
- D. E. Pires, T. L. Blundell and D. B. Ascher, J. Med. Chem., 2015, 58, 4066–4072 CrossRef CAS PubMed.
- P. Turner, Center for Coastal and Land-Margin Research, Oregon Graduate Institute of Science and Technology, Beaverton, OR, 2005 Search PubMed.
- T. Mohammad, A. Shamsi, S. Anwar, M. Umair, A. Hussain, M. T. Rehman, M. F. AlAjmi, A. Islam and M. I. Hassan, Virus Res., 2020, 288, 198102 CrossRef CAS PubMed.
- M. Amir, T. Mohammad, K. Prasad, G. M. Hasan, V. Kumar, R. Dohare, A. Islam, F. Ahmad and M. Imtaiyaz Hassan, J. Biomol. Struct. Dyn., 2020, 38, 4625–4634 CrossRef CAS PubMed.
- T. Mohammad, S. Siddiqui, A. Shamsi, M. F. Alajmi, A. Hussain, A. Islam, F. Ahmad and M. Hassan, Molecules, 2020, 25, 823 CrossRef CAS PubMed.
- D. D. Singh, I. Han, E. H. Choi and D. K. Yadav, Front. Cell Dev. Biol., 2020, 8, 580202 CrossRef PubMed.
- H. Na, C. Mok and J. Lee, Food Chem., 2020, 302, 125306 CrossRef CAS.
- W. Jin, Cells, 2020, 9, 217 CrossRef CAS.
- D. K. Yadav, S. Kumar, E. H. Choi, P. Sharma, S. Misra and M. H. Kim, Front. Pharmacol., 2018, 9, 644 CrossRef PubMed.
- V. Sharma, P. K. Jaiswal, S. Kumar, M. Mathur, A. K. Swami, D. K. Yadav and S. Chaudhary, ChemMedChem, 2018, 13, 1817–1832 CrossRef CAS PubMed.
- D. K. Yadav, S. Kumar, Saloni, H. Singh, M. H. Kim, P. Sharma, S. Misra and F. Khan, Drug Des., Dev. Ther., 2017, 11, 1859–1870 CrossRef CAS PubMed.
- A. Lagunin, A. Stepanchikova, D. Filimonov and V. Poroikov, Bioinformatics, 2000, 16, 747–748 CrossRef CAS PubMed.
- A. A. Naqvi, T. Mohammad, G. M. Hasan and M. Hassan, Curr. Top. Med. Chem., 2018, 18, 1755–1768 CrossRef PubMed.
- M. K. Teli, S. Kumar, D. K. Yadav and M. H. Kim, J. Biomol. Struct. Dyn., 2021, 39, 703–717 CrossRef CAS.
- M. K. Teli, S. Kumar, D. K. Yadav and M. H. Kim, J. Cell. Biochem., 2021 DOI:10.1002/jcb.29933.
- K. Y. Lee, H. S. Choi, K. Y. Chung, B. J. Lee, H. J. Maeng and M. D. Seo, Biomol. Ther., 2016, 24, 191–198 CrossRef CAS PubMed.
- C. G. Gadhe and M. H. Kim, Mol. BioSyst., 2015, 11, 618–634 RSC.
- C. G. Gadhe, A. Balupuri and S. J. Cho, J. Biomol. Struct. Dyn., 2015, 33, 2491–2510 CrossRef CAS PubMed.
- A. W. Schüttelkopf and D. M. Van Aalten, Acta Crystallogr., Sect. D: Biol. Crystallogr., 2004, 60, 1355–1363 CrossRef.
- S. Fatima, T. Mohammad, D. S. Jairajpuri, M. T. Rehman, A. Hussain, M. Samim, F. J. Ahmad, M. F. Alajmi and M. I. Hassan, J. Biomol. Struct. Dyn., 2020, 38, 3610–3620 CrossRef CAS PubMed.
- S. Ali, F. I. Khan, T. Mohammad, D. Lan, M. Hassan and Y. Wang, Int. J. Mol. Sci., 2019, 20, 884 CrossRef CAS PubMed.
- G. G. Maisuradze, A. Liwo and H. A. Scheraga, J. Mol. Biol., 2009, 385, 312–329 CrossRef CAS PubMed.
- A. Altis, M. Otten, P. H. Nguyen, R. Hegger and G. Stock, J. Chem. Phys., 2008, 128, 06B620 CrossRef PubMed.
- D. K. Yadav, S. Kumar, E.-H. Choi, S. Chaudhary and M.-H. Kim, Front. Chem., 2020, 8, 250 CrossRef CAS PubMed.
- S. P. Davies, H. Reddy, M. Caivano and P. Cohen, Biochem. J., 2000, 351, 95–105 CrossRef CAS.
- R. Dahiya, T. Mohammad, S. Roy, S. Anwar, P. Gupta, A. Haque, P. Khan, S. N. Kazim, A. Islam and F. Ahmad, Int. J. Biol. Macromol., 2019, 136, 1076–1085 CrossRef CAS PubMed.
- F. Naz, F. I. Khan, T. Mohammad, P. Khan, S. Manzoor, G. M. Hasan, K. A. Lobb, S. Luqman, A. Islam and F. Ahmad, Int. J. Biol. Macromol., 2018, 107, 2580–2589 CrossRef CAS PubMed.
- M. Amir, S. Ahmad, S. Ahamad, V. Kumar, T. Mohammad, R. Dohare, M. F. Alajmi, T. Rehman, A. Hussain and A. Islam, J. Biomol. Struct. Dyn., 2020, 38, 1514–1524 CrossRef CAS PubMed.
- S. A. Ali, M. Imtaiyaz Hassan, A. Islam and F. Ahmad, Curr. Protein Pept. Sci., 2014, 15, 456–476 CrossRef CAS PubMed.
- T. J. Richmond, J. Mol. Biol., 1984, 178, 63–89 CrossRef CAS.
|
This journal is © The Royal Society of Chemistry 2022 |
Click here to see how this site uses Cookies. View our privacy policy here.