DOI:
10.1039/D1NR05630A
(Paper)
Nanoscale, 2022,
14, 99-107
Enhanced thermal conductivity of nanofluids by introducing Janus particles
Received
26th August 2021
, Accepted 7th December 2021
First published on 7th December 2021
Abstract
The addition of nanoparticles to a base fluid (i.e., nanofluids) is an effective strategy to achieve a higher thermal conductivity of a fluid. In a common nanofluid, the suspended nanoparticles are mostly symmetrical spheres. In the present paper, we propose to add Janus nanoparticles into a fluid (termed as Janus nanofluids), to further enhance the thermal conductivity of nanofluids. By using molecular dynamics simulations, it is found that the thermal conductivity can be distinctly improved by introducing Janus particles into the nanofluids in contrast with common nanofluids. Based on the calculation results of the molecular radial distribution function around the nanoparticle, and the diffusion coefficient of the base fluid and the Janus nanoparticle, the enhancement in the thermal conductivity of Janus nanofluids is attributed to the enhanced Brownian motion of Janus nanoparticles, which increases the probability of inter-molecular collisions and leads to enhanced energy transfer in nanofluids. The Janus nanofluids proposed in this work provide insights for the design of nanofluids with high thermal conductivity.
I. Introduction
Nanofluids are fluids which contain suspended nanometer sized nonmetallic or metallic nanoparticles, including inactive metal powders, ceramic nanoparticles, and metal carbide nanoparticles.1–5 An increase of 9%–108% in the thermal conductivity of nanofluids has been reported for only a small volume fraction (0.05%–4%) of solid particles in base fluid, depending on the temperature and nanoparticles’ size.6–8 Nanofluids can be used as a working fluid with higher heat transfer coefficient in many industrial applications, including microchannel cooling, thermal energy storage, oil recovery, cooling techniques and thermal management.9–16
In the past decades, there have been considerable theoretical, experimental and numerical studies to investigate the thermal transport mechanism of nanofluids.17–27 However, the mechanism of the increase in thermal conductivity of nanofluids is far from clear. The classical continuum models, such as Maxwell model17 and Hamilton-Crosser (HC)18 model, have been employed to predict the thermal conductivities of nanofluids. However, it is found that the prediction by the classical model is inconsistent with the numerical and experimental results, because the classical model cannot take the movement of solid and liquid atoms and possible collisions between them into account, which might contribute to the heat transfer.19,20 Several possible mechanisms for the enhancement of the thermal conductivity of nanofluids have been proposed, including transport of thermal energy by Brownian motion of nanoparticles, the nature of heat transport through nanoparticles, the layer between the base fluid and nanoparticle, and the effects of nanoparticles clustering.3,21–23 The local micro-convection of base fluid caused by nanoparticles also can be responsible for the increase in thermal conductivity of nanofluids.24 In other literature, it is believed that the agglomeration and interaction of nanoparticles is the main reason for the improvement of the thermal transport in nanofluids.25,26 It is believed that the enhanced heat transfer in nanofluids is the result of a combined effect of the above mechanisms.23,27
The transport properties of the nanoparticles and the fluid molecules at the molecular level can play important roles in the thermal transport through nanofluids. The nanoparticles in a base fluid are expected to undergo random Brownian motion. Due to the interaction between the nanoparticles and the fluid molecules, the particle motion can result in the stirring action on the fluid molecules around the particle and the micro-convection of the base fluid, which might contribute to the increase in thermal conductivity of nanofluids. It is reported that the thermal diffusion is much faster than the Brownian diffusion of nanoparticles, so the particle motion is too slow to transport significant amount of heat through nanofluids.3 Based on the kinetic theory analysis and molecular dynamics (MD) simulations, it is claimed that the Brownian motion of nanoparticles have only a minor effect on the thermal conductivity of nanofluids.28–30 However, these exists theoretical analysis which led to the conclusion that the Brownian motion of nanoparticles is an important mechanism governing the thermal behavior of nanofluids.31,32 Some recent MD simulations suggest that the strong diffusion of nanoparticles can lead to high thermal conductivity of nanofluids.33–36 Therefore, the disputes about the effect of the Brownian motion of nanoparticles on the thermal conductivity of nanofluids have not been settled yet.37–40
In a common nanofluid, the suspended nanoparticles are mostly symmetrical spheres. Actually, the surface properties of the particle can be manipulated to be asymmetric, which is termed as Janus particle. Here, the Janus particle is named after the two-faced Roman god.41–44 In recent decades, the fabrication methods have been developed successfully.45,46 The surface selective modification method can be employed to fabricate Janus particles with one hydrophilic side and one hydrophobic side. Usually, Janus nanoparticles have good diffusion characteristics in a fluid environment due to their asymmetrical surface properties.47–50 So, adding Janus nanoparticles into a base fluid (termed as Janus nanofluids in the present paper) is supposed to enhance the diffusion effect of nanoparticles, by which the effect of the nanoparticle Brownian motion on the thermal conductivity of nanofluids can be examined.
In this paper, the thermal conductivity and diffusion property of Janus nanofluids is investigated using MD simulation method, wherein the Janus nanoparticle is constructed by two semispheres with different solid–liquid interaction coupling strength. It is found that both the nanoparticle diffusion coefficient and the thermal conductivity of Janus nanofluids can be enhanced compared to nanofluids with symmetrical nanoparticle. The enhancement of nanoparticle diffusion coefficient in Janus nanofluids is employed to explain the enhancement in thermal conductivity of Janus nanofluids. The rest of the present paper is organized as follows: section II gives the MD simulation details and the calculation method for the thermal conductivity and of nanofluids and mean square displacement (MSD) of fluid molecules and the nanoparticle. In section III, the fluid adsorption layer, micro-convection, and Brownian motion of Janus nanofluids are discussed in detail to elucidate the underlying mechanisms for the thermal conductivity enhancement. The conclusions are given in section IV.
II. Simulation methods
A. Simulation model
In our MD simulations, argon is used as the base fluid, and three different Janus particles (copper, silver and gold nanoparticles) suspend in the base fluid. The MD simulation model is shown in Fig. 1. The interatomic potential of both substances is described by the Lennard-Jones (LJ) 12-6 potential,38 | 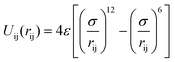 | (1) |
where ε and σ are the binding energy and equilibrium distance, respectively, and rij is the interatomic distance between atoms i and j.
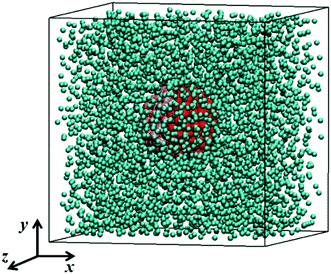 |
| Fig. 1 Geometric configuration of Janus nanofluid system. Outer (green) atoms are fluid atoms and inner (red) atoms are solid atoms. | |
The LJ potential parameters between argon atoms are given by εAr = 1.67 × 10−21 J and σAr = 0.3405 nm. For copper atoms, εCu = 65.625 × 10−21 J and σCu = 0.2338 nm. For silver atoms, εAg = 55.241 × 10−21 J and σAg = 0.2644 nm. For gold atoms, εAu = 70.749 × 10−21 J and σAu = 0.2637 nm. For the coupling parameters between liquid and solid atoms, the following Lorentz-Berthelot mixing rule was used:38,51
|  | (2) |
|  | (3) |
The density of argon is 1418 kg m−3. The atoms in the solid have a face-centered cubic structure with a lattice constant of a = 3.615 Å.52
The Janus nanoparticle is constructed by two semispheres with different solid–liquid interaction coupling strength. The left and right interfacial binding parameters are denoted by εsl,left = cleftεsl and εsl,right = crightεsl, respectively. In the present paper, five types of Janus particles are considered, as shown in Table 1. Note that the averaged solid–liquid coupling parameters over the particle surface are the same to each other for these 5 types of nanofluids. For type I, cleft = cright, namely, the nanoparticle is nothing but a normal symmetrical sphere. A parameter δ is introduced to denote the difference between the left and right interfacial coupling strength, which is given by δ = (cright − cleft)/(cright + cleft). With increasing δ, the discrepancy between two semispheres is more pronounced.
Table 1 The parameters for Janus nanofluids
Type |
I |
II |
III |
IV |
V |
c
left
|
1 |
0.8 |
0.6 |
0.4 |
0.2 |
c
right
|
1 |
1.2 |
1.4 |
1.6 |
1.8 |
δ
|
0 |
0.2 |
0.4 |
0.6 |
0.8 |
All MD simulations are performed by using the large-scale atomic/molecular massively parallel simulator (LAMMPS) packages. In our MD simulations, a Janus particle (Cu, Ag or Au nanoparticle) with a radius of about 1 nm and base fluid of argon atoms was performed. The volume fraction of the nanofluid can be varied by changing the volume of the liquid part. For a volume fraction of 1%, the length of simulation box is set as Lb = 7.212 nm in all x, y and z directions, and there are 8021 Ar atoms. For volume fractions of 0.5% and 2%, there are 15
508 and 4010 Ar atoms, respectively. The Newton's equations of motion are integrated by the Velocity-Verlet algorithm with a time step is 4 fs. The cutoff radius is set to 0.9534 nm. Periodic boundary conditions are employed in the three-dimensional simulation box. The NVT ensemble, by which the total number of atoms, the system volume, and temperature are constant in the simulation, is initially employed to relax for 0.4 ns under the Nosè–Hoover thermostat with temperature 86 K. Then, the data collection is performed for 4 ns under NVE ensemble. Based on the ergodic theory, the physical quantity in the present paper is obtained by long-term average in the collection time. Our MD simulation results are the averaged value of 100 independent runs under different initial conditions.
B. Thermal conductivity and MSD calculation
For the equilibrium molecular dynamics simulations, the thermal conductivity of nanofluids can be obtained by integrating the equilibrium heat flux autocorrelation function through the Green–Kubo theory, | 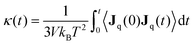 | (4) |
where κ is the thermal conductivity, kB is the Boltzmann constant, V is the system volume, T is the system temperature, Jq is the microscopic heat current vector, and the angular bracket denotes the ensemble average (in a MD simulation, the average over time). The heat current vector is given by | 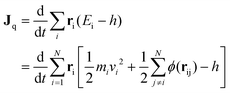 | (5) |
where ri is the position vector, Ei denotes the excess energy, vi is the molecular velocity, ϕ(rij) is the pair potential between atom i and j, and h is the enthalpy per nanoparticle. Here, the enthalpy represents the energy carried by the mediums but not transported between them. For a single component system, the enthalpy is zero due to the zero-average velocity. For a two-component system, the enthalpy cannot be ignored and the heat current can be expressed as the constitution of the potential part, the kinetic part, and the collision part.4,38 Then, an extended form of eqn (5) is employed to calculate the heat current vector for the two-component system53,54 | 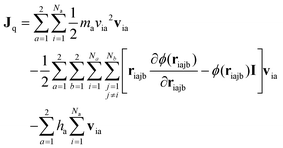 | (6) |
where the subscripts a and b denote two species in a nanofluid. Na and Nb are the number of atoms of species a and b, respectively. via is the velocity of an atom i of specie a, riajb is the interatomic distance between atom i of specie a and atom j of specie b. I denotes the unit tensor, and ha denotes the average enthalpy per nanoparticle of specie a. Here, the averaged enthalpy is calculated as the sum of the averaged kinetic energy, potential energy, and virial terms per nanoparticle of each species. According to eqn (6), the thermal conductivity can be calculated by |  | (7) |
where Ns is the number of MD time steps after equilibration and Ms is the number of steps over which the time average is calculated. To ensure good statistical averaging results, Ms should be much smaller than Ns.
The diffusion coefficient can be obtained based on the Einstein's diffusion theory. For three-dimensional isotropic system, the mean square displacement is defined as55
| MSD(t) = 〈Δri(t)2〉 = 〈(ri(t) − ri(0))2〉 | (8) |
where
ri(
t) −
ri(0) is the vector distance traveled by atom i over a time interval of
t. The limiting slope of MSD(
t) is related to the diffusion coefficient
D by
| 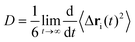 | (9) |
C. Verification of simulation method
It is necessary to verify the simulation method used in the present paper. It has been reported that the MD simulation results are in good agreement with the experimental results for pure argon when the number of atoms is larger than 500, and for nanofluids with the number of atoms larger than 1372.38 In a testing MD simulation, a pure argon fluid with 2048 atoms is considered. Using eqn (4), the heat flux autocorrelation function and running thermal conductivity are shown in Fig. 2a and b, wherein the time range from 100 to 200 ps is selected for the calculation of the thermal conductivity. The thermal conductivity of the pure liquid argon obtained in our simulation is 0.1302 W m−1 K−1, which agrees quite well with the experimental value (0.1320 W m−1 K−1 (ref. 56)) and previous MD simulation results (0.1270 W m−1 K−1 (ref. 38)). Fig. 2c and d plot the heat flux autocorrelation function and running thermal conductivity for a nanofluid with normal Cu nanoparticles. Then, it is obtained that the thermal conductivity is equal to 0.1417 W m−1 K−1 with a volume fraction of 1%, which agrees quite well with previous MD simulation results (0.1493 W m−1 K−1 (ref. 57), 0.1440 W m−1 K−1 (ref. 4) and 0.1486 W m−1 K−1 (ref. 58)). Hence, the simulation model can be used to investigate the thermal conductivity of Janus nanofluids.
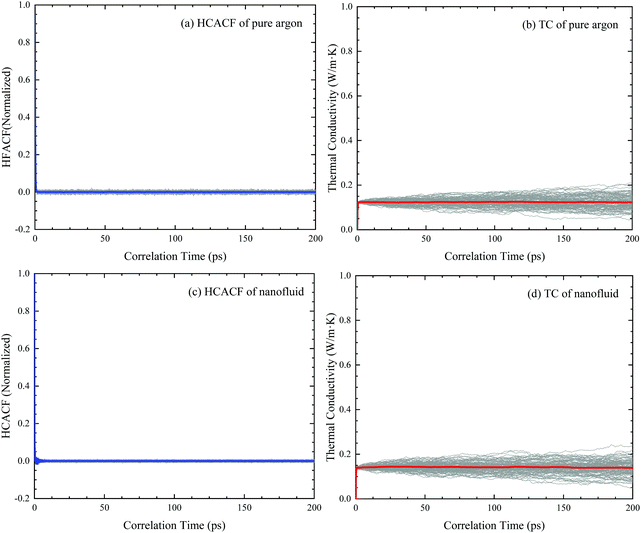 |
| Fig. 2 Heat flux autocorrelation function (normalized) of correlation time for (a) pure argon and (c) Janus nanofluids. Running thermal conductivity as a function of correlation time for (b) pure argon and (d) Janus nanofluids. The thinner and the thicker lines represent the results of independent simulations with different initial velocities and the ensemble average over the independent simulations, respectively. | |
III. Results and discussion
A. Calculation results for thermal conductivity of Janus nanofluids
Fig. 3 shows the calculation results for the thermal conductivity of Janus nanofluids versus the parameter δ. For Ag and Au Janus nanofluids, the volume fraction is set as 1%, while the Janus nanofluids with volume fractions of 0.5%, 1% and 2% are considered for Cu Janus nanofluids. For comparison, the thermal conductivity of the pure base fluid is also presented. As expected, the thermal conductivity of the nanofluids with δ = 0.0 (type I nanofluid with suspended symmetric spheres) is slightly greater than that of the pure argon. For Cu Janus nanofluids, it is found that its thermal conductivity increases with the volume fraction, which is consistent with the general results in open literature.38,57,58 For all the Janus nanofluids with suspended Cu, Ag and Au Janus nanoparticles, the thermal conductivities increase sequentially with increasing δ, which means that the thermal conductivity can be distinctly improved by introducing Janus particles into the nanofluids. Since the enhancement effect by the Janus particles can be observed for different nanoparticles, in the rest of the present paper, we focus on the mechanism of the enhanced thermal conductivity of the Cu Janus nanofluid with its volume fraction of 1%.
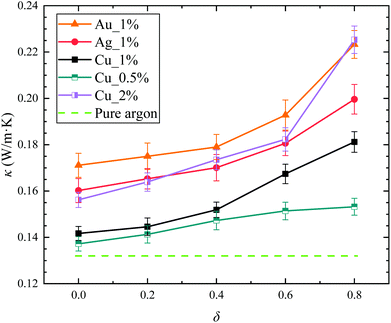 |
| Fig. 3 Thermal conductivities of pure argon and Janus nanofluids (with suspended Cu, Ag and Au Janus nanoparticles). For Cu nanoparticles, thermal conductivities of Janus nanofluids with volume fractions of 0.5%, 1% and 2% are presented. Error bars represent the standard deviation of one hundred independent MD simulations. | |
As mentioned above, the classical Maxwell model cannot predict the thermal conductivity of nanofluids (usually underestimate the thermal conductivity), because the classical model is based on the thermophysical properties of the nanoparticle material and base fluid. Some molecular effects induced by nanoparticle, such as Brownian motion of nanoparticle, micro-convection of base fluid and adsorption layer around nanoparticle, cannot be taken into account in the classical model. This may be the reason for the discrepancy between the results from the classical model and MD simulations for the calculation of the thermal conductivity of nanofluids.
B. Effect of fluid adsorption layer
Since the binding energy between copper atoms and argon atoms is much stronger than that between argon atoms, then the fluid atoms can be adsorbed on the nanoparticle surface to form a solid-like structure. This adsorption layer is related to the cutoff distance of the interfacial interaction (2.8σsl), which is roughly 2–3 layers of fluid atoms, depending on the solid–fluid coupling strength. The adsorption layer plays an important role in the thermal transport through the nanofluids. Usually, the fluid atoms are well ordered around the particle surface.59,60 The thermal transport through ordered atoms is much easier than that through the disordered atoms because of the reduced diffusion effect of the lattice vibrational modes.61,62 Correspondingly, the thermal conductivity of the adsorption layer is expected to be much larger than that of bulk liquid, which may help to improve the thermal conductivity of the nanofluids. This can partly explain the enhanced heat transfer in nanofluids.
For Janus nanofluids, the solid–liquid coupling strength of the left and right semispheres are asymmetric, so the Janus nanoparticles have different adsorption scenarios on the two semispheres, which may affect the adsorption layer. Fig. 4a depicts the radial distribution function (RDF) of liquid atoms for different types of Janus nanofluids. The RDF of argon atom pair has three curve peaks at 0.36 nm, 0.71 nm and 1.4 nm respectively. It is shown that the RDF curves of argon atoms for different types of Janus nanofluids are almost the same to each other. Fig. 4b depicts the radial distribution function of Cu–Ar atoms for different types of Janus nanofluids. It is found that the locations of the first peak of the RDF of solid–liquid atoms are the same (at 0.32 nm) for different types of Janus nanofluids. The value of the first RDF peak decreases slightly with increasing parameter δ owing to the weak solid–liquid interfacial interaction at the weak-coupling semisphere for very large δ. Thus, the effect of the asymmetric surface properties of the Janus particle on the RDF can be neglected or even has a very weak negative effect on the thermal conductivity of the Janus nanofluid, which cannot explain the thermal conductivity enhancement of Janus nanofluids. This is because the suspended nanoparticles usually undergo rapidly Brownian rotation, then the orientation distribution of the Janus particle is almost uniform. As shown in Table 1, the averaged solid–liquid coupling strength over the particle surface is almost the same for varying parameter δ. The density distribution pattern of the base fluid for different types of Janus nanofluids are given in Fig. 5. Fig. 5a plots the density distribution pattern of pure argon, which shows the disordered distribution of liquid atoms. Fig. 5b–f present the density distribution pattern of the base fluid in the Janus nanofluids with varying δ. The liquid atoms are well ordered around the nanoparticle, and there is no distinct difference between different types of Janus nanofluids, which confirms the above statement. Therefore, the effect of the fluid adsorption layer is related to the enhancement of the thermal conductivity of the nanofluids compared to the base fluid. However, the fluid adsorption layer cannot explain the increase in the thermal conductivity of Janus nanofluids compared to a nanofluid with symmetric nanoparticles.
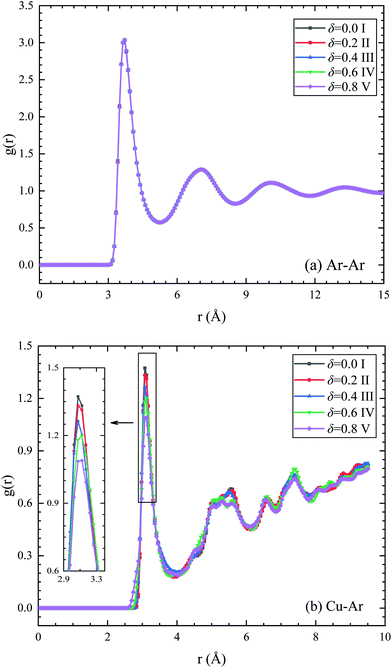 |
| Fig. 4 Radial distribution function of (a) argon–argon atoms and (b) copper–argon atoms for different types of Janus nanofluids. | |
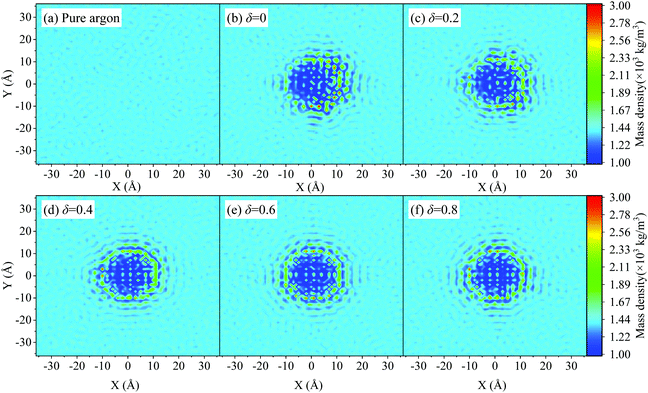 |
| Fig. 5 (a)–(f) Density distribution cloud map of base fluid for different types of Janus nanofluids. | |
C. Effects of micro-convection and Brownian motion of nanoparticles
a. Micro-convection.
The micro-convection (which is also called localized-convection24) represents the fluid convection or molecular flow around the nanoparticle due to the Brownian motion of nanoparticles.30,38,39 The micro-convection can be identified by the diffusion coefficient of the base fluid, since the mass transfer is associated with the convective heat transfer. The MSD and diffusion coefficient D of pure argon and base fluid are obtained according to eqn (8) and (9), and the calculation results are plotted in Fig. 6. In Fig. 6a, the diffusion coefficient of the base fluid is lower than that of pure argon, because the motion of the fluid atoms around the particle surface is restricted around the nanoparticle (i.e., the adsorption layer). On the other hand, there is almost no change in the diffusion coefficient D of the base fluid with varying δ.
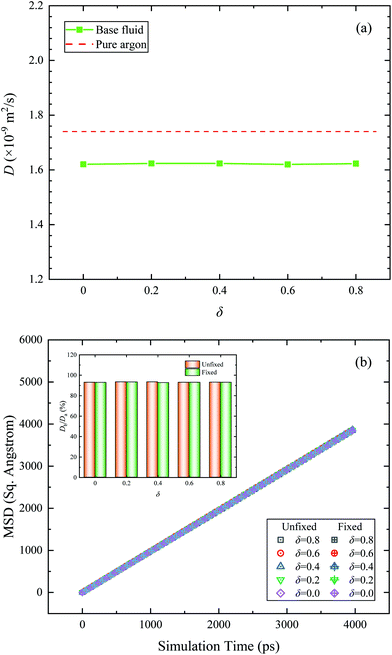 |
| Fig. 6 (a) Diffusion coefficient of the base fluid and pure argon. (b) MSD and diffusion coefficient of the base fluid under the fixed and unfixed conditions. | |
To eliminate the combined effect between the micro-convection and the Brownian motion of nanoparticles, two conditions for the Janus nanoparticle (unfixed and fixed conditions) are considered in the present paper. Under the fixed condition, the motion of Cu nanoparticle is restricted by subtracting the translational and rotational centroid velocities of nanoparticle. The comparison of MSD and D of base fluid between unfixed and fixed conditions is shown in Fig. 6b. It is clearly seen that there is no obvious difference in both MSD and D results under unfixed and fixed conditions. So, the micro-convection effect to the thermal conductivity of Janus nanofluids can be ignored.
b. Brownian motion of nanoparticles.
The Brownian motion is a random motion of nanoparticles resulting from their collision with the fast-moving molecules in the fluid.31 The Brownian motion of nanoparticles may induce the collision between the nanoparticles and fluid molecules, which can contribute to the energy transfer in nanofluids. For a Janus particle suspended in a fluid, the asymmetry can drive the particle because the two distinct “faces” have different surface properties (different coupling strength).42–44 So, the diffusion coefficient is expected to be enhanced for the Janus nanoparticles, which might in turn enhance the thermal conductivity of Janus nanofluids.
Fig. 7 shows thermal conductivity of Janus nanofluids under unfixed and fixed conditions. In the case of fixed nanoparticles, the Brownian motion of nanoparticles is artificially eliminated. As a result, the thermal conductivities under fixed condition are smaller than those under unfixed condition, and independent on the parameter δ. In the case of unfixed Janus nanoparticles, the thermal conductivity increases evidently with increasing δ. Therefore, the Brownian motion of Janus nanoparticles has a significant impact on the thermal conductivity of Janus nanofluids. Probably, the particle Brownian motion should be responsible for the thermal conductivity increase of Janus nanofluids. Fig. 8a shows the MSD of the Janus nanoparticles for different types of Janus nanofluids, and Fig. 8b presents the diffusion coefficient Dp. It is found that the diffusion coefficient of the Janus nanoparticle increases gradually with increasing δ. For type V nanofluid (δ = 0.8), the diffusion coefficient was found to increase by 40% compared to the diffusion coefficient of type I (δ = 0.0, symmetric nanoparticle), which is very close to the enhancement ratio of the thermal conductivity (39%). Here, the enhancement ratio of the thermal conductivity of the Janus nanofluids (κ) and the diffusion coefficient of the Janus particles (Dp) are calculated by κ(δ = 0.8)/κ(δ = 0.0) and Dp(δ = 0.8)/Dp(δ = 0.0), respectively. According to Fig. 3 and 8, the enhancement ratio of thermal conductivity and diffusion coefficient has a similar trend with increasing δ. The enhanced diffusion effect of the Janus nanoparticle increases the probability of collisions with surrounding liquid atoms, which leads to the enhanced energy transfer in the nanofluid and a higher thermal conductivity of the Janus nanofluids. Consequently, the Brownian motion of nanoparticles should be responsible for the improved thermal conductivity of the Janus nanofluids.
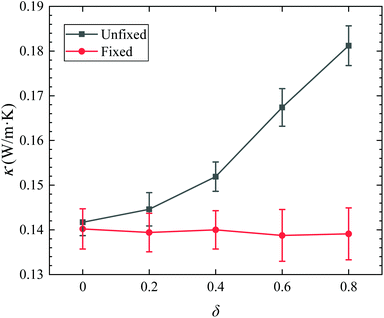 |
| Fig. 7 Comparison of thermal conductivity for Cu Janus nanofluid under unfixed and fixed conditions. | |
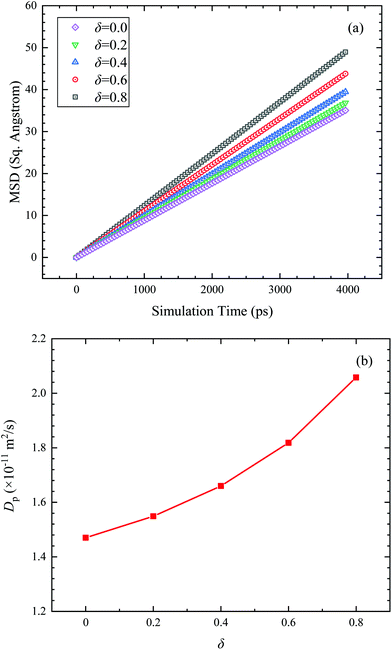 |
| Fig. 8 (a) Mean square displacement (MSD) of Janus nanoparticle with simulation time in different types. (b) Diffusion coefficient of Janus nanoparticle (Dp) in different types. | |
In the present paper, only one nanoparticle is considered in the Janus nanofluid. As mentioned in Introduction, the agglomeration and interaction of nanoparticles can also contribute to the thermal transport of nanofluids. For Janus particles, due to the anisotropic interaction, the Janus particles can assemble into clusters with diverse morphologies, including micelles, vesicles, or lamellar phases.63–67 In this case, the enhanced conduction due to larger aggregation of highly conductive nanoparticle can play a significant role which enhances the thermal conductivity of nanofluids.25,26,68 This effect will be considered in our future studies.
IV. Conclusions
In the present paper, the molecular dynamics simulations have been conducted to study the thermal conductivity of Janus nanofluids. It is found that the thermal conductivity of nanofluids can be enhanced by the addition of the Janus nanoparticles in contrast with common nanofluids, and the thermal conductivity of Janus nanofluids increases with gradually the parameter δ (which denotes the difference between the left and right interfacial coupling strength). By analyzing the mean square displacement and radial distribution function of base fluid, it has been revealed that the fluid adsorption layer at the solid–liquid interface and the micro-convection of base fluid are probably not the dominate mechanisms for the enhancement in the thermal conductivity of Janus nanofluids. According to the calculation results of the thermal conductivity of Janus nanofluids under fixed and unfixed conditions, and the diffusion coefficient of the Janus nanoparticles, it is concluded that the Brownian motion of Janus nanoparticle should be responsible for the improved thermal conductivity of the Janus nanofluids. The findings in the present paper offer possible avenues for optimizing the material selection and provide insights for the design of nanofluids.
Author contributions
Xin Cui, Jun Wang and Guodong Xia designed the research, Xin Cui conducted the numerical simulations, Xin Cui and Jun Wang conducted the theoretical analysis. All authors reviewed and approved the manuscript.
Conflicts of interest
There are no conflicts to declare.
Acknowledgements
This work is supported by the National Natural Science Foundation of China (grants no. 51776007). The simulations are carried out at National Supercomputer Center in Tianjin, and the calculations were performed on TianHe-1 (A).
Notes and references
-
S. U. S. Choi and J. A. Eastman, ASME International Mechanical Engineering Congress & Exposition, San Francisco, 1995 Search PubMed.
- S. U. S. Choi, Z. G. Zhang, W. Yu, F. E. Lockwood and E. A. Grulke, Appl. Phys. Lett., 2001, 79, 2252–2254 CrossRef CAS.
- P. Keblinski, S. R. Phillpot, S. U. S. Choi and J. A. Eastman, Int. J. Heat Mass Transfer, 2002, 45(4), 855–863 CrossRef CAS.
- L. Li, Y. W. Zhang and H. B. Ma, J. Nanopart. Res., 2010, 12(3), 811–821 CrossRef CAS.
- F. Jabbari, A. Rajabpour and S. Saedodin, Chem. Eng. Sci., 2017, 174(31), 67–81 CrossRef CAS.
- M. S. Liu, M. C. Lin, C. Y. Tsai and C. C. Wang, Int. J. Heat Mass Transfer, 2006, 49(17–18), 3028–3033 CrossRef CAS.
- S. K. Das, N. Putra, P. Thiesen and W. Roetzel, J. Heat Transfer, 2003, 125(4), 567–574 CrossRef CAS.
- S. Lee, S. U. S. Choi, S. Li and J. A. Eastman, J. Heat Transfer, 1999, 121(2), 280–289 CrossRef CAS.
- Y. Yang, Z. G. Zhang, E. A. Grulke, W. B. Anderson and G. Wu, Int. J. Heat Mass Transfer, 2005, 48(6), 1107–1116 CrossRef CAS.
- F. S. Moghanlou, A. S. Khorrami, E. Esmaeilzadeh and M. Vajdi, J. Braz. Soc. Mech. Sci. Eng., 2021, 43(3), 149 CrossRef.
- M. H. Zhang, S. Y. Chen, N. Sheng, B. X. Wang, Z. T. Wu, Q. Q. Liang and H. P. Wang, Nanoscale, 2021, 13(17), 8126–8136 RSC.
- S. Y. Mei, C. Qi, M. Liu, F. Fan and L. Liang, Int. J. Heat Mass Transfer, 2019, 134, 707–721 CrossRef CAS.
- Z. Y. Ou and M. Muthukumar, J. Chem. Phys., 2005, 123(7), 074905 CrossRef.
- S. L. Zhu, M. T. Nguyen and T. Yonezawa, Nanoscale Adv., 2021, 3(16), 4626–4645 RSC.
- X. Y. Liang, F. J. Zhou, T. B. Liang, R. Wang, H. Su and S. Yuan, J. Mol. Liq., 2021, 324, 114682 CrossRef CAS.
- M. Bahiraei, Int. J. Therm. Sci., 2016, 109, 90–113 CrossRef CAS.
-
J. C. Maxwell, A treatise on electricity and magnetism, Clarendon Press, Oxford, 1873 Search PubMed.
- R. L. Hamilton and O. K. Crosser, Ind. Eng. Chem. Res., 1962, 1(3), 27–40 Search PubMed.
- J. Eapen, L. Ju and S. Yip, Phys. Rev. Lett., 2007, 98(2), 028302 CrossRef PubMed.
- R. H. Davis, Int. J. Thermophys., 1986, 7(3), 609–620 CrossRef CAS.
- Y. Kobayashi and N. Arai, J. Electrochem. Soc., 2019, 166(9), B3223–B3227 CrossRef CAS.
- A. Mosavi, M. Hekmatifar, A. Alizadeh, D. Toghraie, R. Sabetvand and A. Karimipour, J. Mol. Liq., 2020, 319, 114183 CrossRef CAS.
- M. R. H. Roni, M. R. B. Shahadat and A. M. M. Morshed, Micro Nano Lett., 2021, 16(3), 221–226 CrossRef CAS.
- R. Prasher, P. Bhattacharya and P. E. Phelan, J. Heat Transfer., 2006, 128(6), 588–595 CrossRef CAS.
- B. X. Wang, L. P. Zhou and X. F. Peng, Int. J. Heat Mass Transfer, 2003, 46, 2665–2672 CrossRef CAS.
- R. Prasher, P. E. Phelan and P. Bhattacharya, Nano Lett., 2006, 6(7), 1529–1534 CrossRef CAS PubMed.
- W. Z. Cui, Z. J. Shen, J. G. Yang and S. H. Wu, Int. Commun. Heat Mass Transfer, 2016, 71, 75–85 CrossRef CAS.
- W. Evans, J. Fish and P. Keblinski, Appl. Phys. Lett., 2006, 88(9), 093116 CrossRef.
- H. Babaei, P. Keblinski and J. M. Khodadadi, J. Appl. Phys., 2013, 113(8), 084302 CrossRef.
- S. Lee, R. Saidur, M. F. M. Sabri and T. K. Min, Numer. Heat Transfer, Part A, 2016, 69(6), 643–658 CrossRef.
- S. P. Jang and S. U. S. Choi, Appl. Phys. Lett., 2004, 84(21), 4316–4318 CrossRef CAS.
- R. Prasher, P. Bhattacharya and P. E. Phelan, Phys. Rev. Lett., 2005, 94(2), 025901 CrossRef PubMed.
- W. Z. Cui, Z. J. Shen, J. G. Yang and S. H. Wu, Appl. Therm. Eng., 2015, 76, 261–271 CrossRef CAS.
- R. Essajai, A. Mzerd, N. Hassanain and M. Qjani, J. Mol. Liq., 2019, 293, 111494 CrossRef CAS.
- E. V. Timofeeva, J. L. Routbort and D. Singh, J. Appl. Phys., 2009, 106(1), 014304 CrossRef.
- I. Zahmatkesh, M. Sheremet, L. Yang, S. Z. Heris, M. Sharifpur, J. P. Meyer, M. Ghalambaz, S. Wongwises, D. W. Jing and O. Mahian, J. Mol. Liq., 2021, 321, 114430 CrossRef CAS.
- J. Koo and C. Kleinstreuer, J. Nanopart. Res., 2004, 6, 577–588 CrossRef.
- S. Sarkar and R. P. Selvam, J. Appl. Phys., 2007, 102(7), 074302 CrossRef.
- X. P. Fang, Y. M. Xuan and Q. Li, Appl. Phys. Lett., 2009, 95(20), 203108 CrossRef.
- Z. Li, L. Cui, B. R. Li and X. Z. Du, Int. J. Heat Mass Transfer, 2020, 153, 119578 CrossRef CAS.
- A. Walther and A. H. E. Müller, Chem. Rev., 2013, 113(7), 5194–5261 CrossRef CAS PubMed.
- M. C. Yang and M. Ripoll, Phys. Rev. E: Stat., Nonlinear, Soft Matter Phys., 2011, 84, 061401 CrossRef PubMed.
- J. R. Gomez-Solano, A. Blokhuis and C. Bechinger, Phys. Rev. Lett., 2016, 116(13), 138301 CrossRef PubMed.
- T. Baier, S. Tiwari, S. Shrestha, A. Klar and S. Hardt, Phys. Rev. Fluids, 2018, 3(9), 094202 CrossRef.
- L. Hong, S. Jiang and S. Granick, Langmuir, 2006, 22(23), 9495–9499 CrossRef CAS PubMed.
- B. T. T. Pham, C. H. Such and B. S. Hawkett, Polym. Chem., 2015, 6, 426–435 RSC.
- A. Kharazmi and N. V. Priezjev, J. Chem. Phys., 2015, 142(23), 234503 CrossRef PubMed.
- A. Kharazmi and N. V. Priezjev, J. Phys. Chem. B, 2017, 121(29), 7133–7139 CrossRef CAS PubMed.
- J. D. Olarte-Plata and F. Bresme, J. Chem. Phys., 2020, 152(20), 204902 CrossRef CAS.
- H. R. Jiang, N. Yoshinaga and M. Sano, Phys. Rev. Lett., 2010, 105(26), 268302 CrossRef PubMed.
-
M. P. Allen and D. J. Tildesley, Computer imulations of liquid, Oxford University Press, Oxford, 1987 Search PubMed.
-
D. Gross, W. Hauger, J. Schröder and W. A. Wall, Engineering Mechanics, Springer, Berlin, 2014 Search PubMed.
- R. Vogelsang, C. Hoheisel and G. Ciccotti, J. Chem. Phys., 1987, 86(11), 6371–6375 CrossRef CAS.
- L. Xue, P. Keblinski and S. R. Phillpot, Int. J. Heat Mass Transfer, 2004, 47(19–20), 4277–4284 CrossRef.
-
D. T. Gillespie and E. Seitaridou, Simple Brownian diffusion, Oxford University Press, Oxford, 2013 Search PubMed.
- M. P. Florian, J. Chem. Phys., 1997, 106(14), 6082–6085 CrossRef.
- W. Z. Cui, Z. J. Shen, J. G. Yang, S. H. Wu and M. L. Bai, RSC Adv., 2014, 4, 55580–55589 RSC.
- H. Loulijat, H. Zerradi, A. Dezairi, S. Ouaskit, S. Mizani and F. Rhayt, Adv. Powder Technol., 2015, 26(1), 180–187 CrossRef CAS.
- H. Guo and N. Zhao, J. Mol. Liq., 2018, 259, 40–47 CrossRef CAS.
- S. Salassi, A. Cardellini, P. Asinari, R. Ferrando and G. Rossi, Nanoscale Adv., 2020, 2, 3181–3190 RSC.
- M. E. Caplan, A. Giri and P. E. Hopkins, J. Chem. Phys., 2014, 140(15), 154701 CrossRef.
- Y. Hu, T. L. Feng, X. K. Gu, Z. Y. Fan, X. F. Wang, M. Lundstrom, S. S. Shrestha and H. Bao, Phys. Rev. B, 2020, 101, 155308 CrossRef CAS.
- R. A. D. Araujo, D. J. B. Villegas, R. G. Larson and U. M. C. Figueroa, Soft Matter, 2016, 12, 4071–4081 RSC.
- G. I. Vega-Bellido, R. A. DeLaCruz-Araujo, I. Kretzschmar and U. M. C. Figueroa, Soft Matter, 2019, 15, 4078–4086 RSC.
- J. A. V. Camacho, R. A. D. Araujo, I. Kretzschmar and U. M. C. Figueroa, Soft Matter, 2020, 16, 2460–2472 RSC.
- Y. Kobayashi, N. Arai and A. Nikoubashman, Soft Matter, 2020, 16, 476–486 RSC.
- E. Bianchi, A. Z. Panagiotopoulos and A. Nikoubashman, Soft Matter, 2015, 11, 3767–3711 RSC.
- I. Topal and J. Servantie, Chem. Phys., 2019, 516, 147–151 CrossRef CAS.
|
This journal is © The Royal Society of Chemistry 2022 |