DOI:
10.1039/D1GC03651C
(Paper)
Green Chem., 2022,
24, 285-294
New insight into lignin aggregation guiding efficient synthesis and functionalization of a lignin nanosphere with excellent performance†
Received
5th October 2021
, Accepted 3rd November 2021
First published on 9th December 2021
Abstract
Understanding the molecular mechanism of lignin nanoparticle (LNP) formation will precisely instruct its functionalization, which is of importance for biomass valorization. Trial-and-error approaches for mechanistic research at the molecular level are extremely difficult and resource intensive. Here we have revealed that the intra-molecular interactions of lignin aromatic rings were the internal force driving the aggregation of lignin into LNPs by combining molecular simulation with experimental methods. A quantitative aromatic interaction correlated with the configuration distribution of lignin dimer and LNP yields was also developed for guiding the synthesis and functionalization of LNPs. A cellulose-derived solvent, γ-valerolactone (GVL), was then screened out to sustain lignin molecules’ intramolecular stacking (−5.54 kJ mol−1) into a spherical nucleus for the growth of a uniform and stable lignin nanosphere during solvent shifting. With the assistance of the LNP formation mechanism, lignin could successfully stabilize in situ generated gold nanoparticles with excellent photothermal performance during the production of LNPs in a GVL/water solution. The newly proposed mechanism will provide an important theoretical basis for upgrading lignin to value-added biomaterials in a green and facile pathway.
Introduction
Lignin is the most abundant renewable phenolic biopolymer on the Earth, and will likely replace petroleum-based aromatic polymers. The valorization of lignin, should reduce the carbon footprint to fulfill a sustainable economy.1,2 Lignin nanoparticles (LNPs), a self-assembly colloid of lignin,3 with biodegradable and biocompatible merits show immense potential as building blocks.4–7 In recent years, LNPs were mainly used as a green tool to carry drugs, genes and proteins via strong non-bonding interactions between them for anti-microbial or disease treatments.5,8,9 Except as the desired nano-vehicles in biomedicine, LNPs certainly have huge potential in many fields.10 For example, LNPs could spatially confine enzymes for efficient biocatalytic ester synthesis in water.11 LNPs, natural reinforcers, were also incorporated into materials to provide superior dynamic sacrificial bonds for high performance artificial muscles.12 No matter what field nanolignin is applied in, its aggregated form plays an important role. Without the aggregation of lignin, it is impossible to modify nano-lignins with a specific function, or even form uniform nano-lignin. However, the driving force behind the aggregation of lignins into nanospheres remains unclear.
Nowadays, most studies believe that the π–π stacking and hydrogen bonding between lignin molecules lead to the formation of nanospheres.13–15 For instance, in the process of preparing a nano-lignin using the dominant solvent-exchange method, lignin molecules will aggregate into nanospheres due to the inter-molecular interactions between a good solvent and one that is not good.3 However, no one can explain why a lignin dissolved in some solvents cannot form uniform nanospheres through the solvent exchange method. For example, when a nano-lignin is prepared using an aqueous hydrotropic or ethylene glycol solution of the lignin, regular nano-spheres cannot be obtained.16,17 It could not even form a nano-lignin, when we used DMSO as the solvent for lignin dissolution in this work.
Furthermore, LNPs usually need to be functionalized to empower them for more applications.9,18 However, the functionalization of LNPs mostly requires multiple processes, which not only augment the risks of generating toxic wastes, but also consume huge amounts of manpower and resources.9,19 For example, some researchers used the produced LNPs to synthesize and stabilize gold or silver nanoparticles, where an abundance of water and time violated the principles of green chemistry.19,20 Besides, the water-insoluble drugs were hard to encapsulate once they formed functional LNP colloids for the synergistic therapy of diseases.9 At present, rare studies are reported on integrating multifunctions into LNPs for biomedical applications, not to mention reaching the destination by a one-step process.21 The cause of this situation may also be ascribed to the unclear formation mechanism of LNPs produced by the predominant solvent exchange method, inhibiting the tailoring of LNP functions.22 In such a case, the study needs to devote great efforts toward optimizing the LNP-based nano-agents by attempting burdensome trial-and-error experiments. The conventional explanation of the mechanism followed the classical nucleation theory (CNT) in a general way at the macroscopic level.23 Nevertheless, it cannot clarify the effect of a specific solvent on the formation of LNPs at the molecular level, which is crucial for precise functionalization.
Although there have been some researchers focusing on the mechanism of LNP formation via molecular simulations or experimental approaches, little progress has been made.15,22,24 The question of whether all these lignin solvents can be introduced for producing uniform lignin colloids is still unanswered. Especially, to the best of our knowledge, no study reported how the intra-molecular interactions of lignin affected the final formation of LNPs. The limited studies about the mechanisms via solvent exchange then impeded the precise functionalization and application of LNPs.
To deeply understand the formation mechanism of LNPs by a solvent exchange approach, we carried out wet experiments with the assistance of computational methods. Several common solvents with different solvating capacities were chosen for the solubilization of lignin to prepare pure and functional LNPs. The morphologies and properties of the resulting particles were investigated and compared to gain insights into the underlying mechanism. Their formation mechanism was investigated using multiscale methods by combing density functional theory (DFT), semiempirical quantum chemistry (SQC) and molecular dynamics (MD). After integrating with the computational and experimental methods, γ-valerolactone (GVL), a green and non-toxic solvent,14 was screened out to show its excellent capacity in the formation and functionalization of LNPs. GVL can interact with the lignin molecule in a suitable way to make the lignin stack into stable and uniform spherical LNPs. Meanwhile, GVL can be hydrolyzed to 4-hydroxyvaleric acid (HVA) under an acidic or heated environment to synergistically stabilize the LNP supported noble metal nanoparticles (e.g., AuNPs, etc.) with excellent photothermal performance in one-pot by one-step.
Experimental and computational sections
Materials
Chloroauric acid and Alkaline lignin (AL) (CAS number: 8068-05-1 and product number: 370959-100G) were purchased from Sigma-Aldrich Corp. The average molecular weight of lignin determined by SEC is 3980 Da, and its phenolic hydroxyl content is 3.43 mmol per gram of lignin.14 Methanol, acetone, gamma-valerolactone (GVL), tetrahydrofuran (THF), dimethyl sulfoxide (DMSO), and dimethylformamide (DMF) were of A.R. grade and were bought from Aladdin Co., Ltd (Shanghai, China).
Preparation of lignin nanoparticles (LNPS)
Lignin nanoparticles from different solvent systems were prepared using a dropping nanoprecipitation method according to our previous report.23 To improve the solubility of lignin, unless otherwise specified, all the organic solvents were mixed with water with a volume ratio of 4
:
1 in this study. In a specific experiment, 40 mg of alkaline lignin (AL) was dissolved in 1 mL of a GVL/water binary solvent, where the volume ratio of GVL and water was set as 4
:
1 to achieve a suitable dissolving capacity for lignin. 0.2 mL of lignin solution was added dropwise into 10 mL of deionized water under a gentle stir at 30 °C for 2 h, to generate LNPs. The formed LNPs were precipitated out by centrifuging at 10
000g for 10 min, and re-dispersed with freshly DI water. The centrifuging and re-dispersing processes were repeated in triplicate to remove free GVL. The procedure of LNP production from other organic solvent/water binary solvents was the same as that from the GVL/water solvent. The yield of LNPs was calculated by the weighing method.
One-pot synthesis of gold nanoparticle doped LNPs (Au-LNPs)
The preparation of Au-LNPs from different solvent systems was similar to that of the LNPs. The main difference between them was that the lignin solution was dropped into an aqueous solution of chloroauric acid (1 mmol L−1) instead of DI water. The preparation of Au-LNPs in a GVL/water binary solvent system with different GVL contents was also carried out. During this process, the aqueous solution of chloroauric acid in the above method was replaced by the GVL/water solution of chloroauric acid (1 mmol L−1).
Characterization of nanoparticles
The hydrodynamic sizes of the prepared nanoparticles, including LNPs and Au-LNPs, were determined using a Zetasizer nano ZS particle analyzer (Malvern instruments Limited). All data are based on the average value of three independent experiments. The morphologies of all nanoparticles were observed using a transmission electron microscope (TEM) (TECNAI G2, FEI, Maastricht, The Netherlands). The gold surface plasmon resonance (SPR) peaks of Au-LNPs prepared under different conditions were analyzed using a UV-Vis spectrophotometer, Shimadzu UV-2550. The valence of gold in nanoparticles was detected by X-ray photoelectron spectroscopy (XPS), Thermo Scientific K-Alpha+. The content of gold in the nanoparticles was determined via inductively coupled plasma optical emission spectroscopy (ICP-OES) at Agilent 720.
Computational section
The ability (pKbh) of solvent molecules for hydrogen binding was calculated by combining the change of the Gibbs free energy and the thermodynamic cycle (Scheme 1). The calculation of pKbh refers to eqn (1), in which was similar to pKa calculation.25 All of the configurations of the molecules including S and SH+ were optimized with density functional theory (DFT) at the B3LYP-D3/6-311G* level of the Gaussian 16 software package, and frequency analysis was then conducted at the same level to confirm them without virtual frequency.26 The solvation free energies including ΔGsolv(S) and ΔGsolv(SH+) of optimized molecules in water were computed at the M062x/6-31G* level under the SMD model, a universal continuum solvation model.26 A composite wavefunction theoretical method, CBS-QB3, was then used to compute their single point energies (gas-phase free energy) including Ggas(S) and Ggas(SH+).25 The solvation free energy of ΔGsolv(H+) and single point energy of Ggas(H+) were −264.61 and −6.28 kcal mol−1, respectively, derived from the experiment.25 The pKbh was then calculated by eqn (4). |  | (1) |
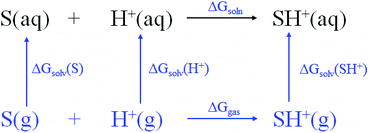 |
| Scheme 1 Thermodynamic cycle of solvent molecules associated with hydrogen ion. | |
For thermodynamic cycle,
| ΔGsoln = ΔGgas + ΔGsolv(SH+) − ΔGsolv(H+) − ΔGsolv(S) | (2) |
and
|  | (3) |
| pKbh = [Ggas(SH+) − Ggas(S) + ΔGsolv(SH+) − ΔGsolv(S) + 272.78]/1.3644 | (4) |
A lignin dimer, guaiacylglycerol-beta-guaiacyl ether (GGE), was chosen as the representative of lignin. To obtain a configuration with the lowest energy in diverse solvents, thousands of GGE configurations were first generated using a molecular dynamics (MD) method in the xTB (an extended tight-binding semi-empirical program package).27 The generated GGE configurations were pre-optimized with the semi-empirical quantum chemical method at the GFN0-xTB level, following at the GFN2-xTB level under different implicit solvent models.28 The resulting configurations were then re-optimized with the density functional theory (DFT) at the B97-3c level29 of the ORCA 4.2 software packages, and frequency analysis was then conducted at the same level to confirm them without virtual frequency. The single point energies of all of the optimized isomers were calculated at the PWPB95-D3/def2-QZVPP level under the SMD model.30 Their Boltzmann distributions and relative energies for the configuration with the lowest energy were summarized by the Molclus software package.31 The reduced density gradient (RDG) method32 was implemented for optimized GGE in the Multiwfn 3.7 program33 to investigate the non-covalent interactions (NCIs) between the aromatic rings of GGE. The NCIs were visualized via the VMD 1.9.3 program.34
To investigate the interactions between GGE and different solvent molecules under the implicit water model, thousands of configurations of clusters containing three solvent molecules and GGE with the lowest energy were first produced using the MD method. The generated clusters were pre-optimized at the GFN0-xTB level, following at the GFN2-xTB level under implicit water models.33 The resulting configurations were then re-optimized with the density functional theory (DFT) at the B97-3c level of the ORCA 4.2 software packages, and frequency analysis was then conducted at the same level to confirm them without virtual frequency. The single point energies of all of the optimized clusters were calculated at the PWPB95-D3/def2-TZVP level under the SMD model with water as the solvent. Their Boltzmann distributions and relative energies for the configuration with the lowest energy were also summarized by the Molclus software package.31 The independent gradient model (IGM) method35 was implemented for the optimized GGE in the Multiwfn 3.7 program33 to investigate the non-covalent interactions (NCIs) between GGE and different organic solvent molecules. The NCIs were visualized via the VMD 1.9.3 program.34
To investigate the interactions between four GGE molecules in different solvents, over one hundred configurations of four GGE associated solvent molecules with the lowest energy were randomly generated by the genmer program in the Molclus software packages.31 The generated clusters were pre-optimized at the GFN0-xTB level, then re-optimized at the GFN2-xTB level under implicit water models.28 Their configurations with the lowest energy were then visualized using the VMD 1.9.3 program.34
Results and discussion
Experimental study on the effect of organic solvents on the formation of LNPs
Six common water-miscible organic solvents covering a wide range of Hansen solubility parameters36 (Fig. S1†) including methanol, acetone, gamma-valerolactone (GVL), tetrahydrofuran (THF), dimethyl sulfoxide (DMSO), and dimethylformamide (DMF), respectively, were selected for producing LNPs. Apparently, a large amount of spherical LNPs can be produced from GVL and THF solvents, but less were produced from acetone and methanol. Contrarily, no lignin nanospheres were observed from DMSO and DMF (Fig. 1). When a tiny droplet of lignin solution with a high concentration of these solvents were, respectively, added to DI water, the obtained suspension showed significantly different Tyndall effects (Fig. 1a). Suspension from DMSO and DMF displayed almost no light scattering. Meanwhile, after dialyzing the suspension against water to remove the free organic solvent molecules, and applying centrifugal force (10000g, 10 min) to the dialyzed suspension, no precipitate was collected from the samples containing DMSO and DMF solution (inserted tubes in Fig. 1b and c). When the solvent-free suspension was air-dried and exposed to TEM, fibrous and lamellar lignin bulk particles (Fig. 1b and c) were detected for the DMF and DMSO cases, respectively. All of these phenomena indicate that no uniform nanoparticles were generated while using DMF and DMSO as solvents for lignin. In contrast, the light was strongly scattered by suspension from GVL and THF lignin solution, and weakly scattered by suspension from methanol and acetone lignin solution. Over 50% of lignin was precipitated from GVL and THF lignin solution, but less than 5% was obtained from the other two (inserted images in Fig. 1d–g). TEM images show that these precipitates contained uniform and separate nanoparticles (Fig. 1h–k), nevertheless nanoparticles trapped in the network frameworks (Fig. 1d–g) were observed after air-drying their solvent-free suspension on the sample grids.
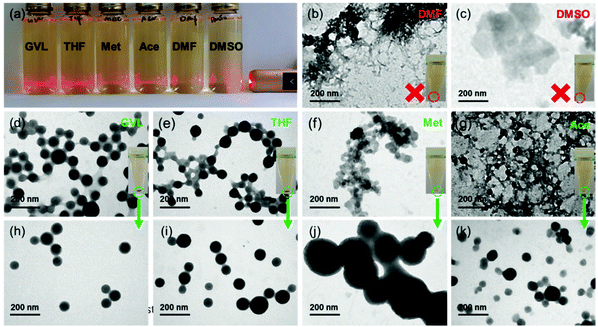 |
| Fig. 1 Characterization of LNPs produced from distinct solvent systems. (a) Photo images of lignin suspensions from different solvents, (b–g) TEM images of air-dried solvent-free suspensions from DMF, DMSO, GVL, THF, methanol and acetone samples, respectively, and (h–k) TEM images of precipitates from GVL, THF, methanol and acetone samples, respectively. | |
Some studies have reported that intermolecular π–π stacking interactions are the driving force of lignin self-assembling into nanoparticles from the ‘good’ solvent to the ‘bad’ solvent.3 However, limited reports investigated the effect of solvent diversities on the LNP formation,37 and the underlying molecular mechanism was paid no attention. Intuitively, lignin molecules in DMSO or DMF might lose the hydrogen of the phenol hydroxyl to become anionic, and in turn hinder the stacking interaction due to the Lewis basic properties of DMSO and DMF. Nevertheless, the pH value of the lignin solution from DMSO and DMF was only slightly higher than 7 (Fig. S2†), but much lower than the pKa (about 10) of phenol hydroxyl. Actually, such basic strength was not enough to ionize the lignin molecules. The small pH differences (Fig. S2†) among GVL, THF, methanol and acetone also cannot explain the great distinction between LNP yields. Subsequently, we also calculated the ability (pKbh) of solvent molecules for hydrogen binding (Fig. S2†) using the thermodynamic cycle with the density functional theory (DFT) method. Unfortunately, exactly as seen with the pH parameter, the pKbh of solvents did not show a strong correlation with the LNP formation.
Computational study on the effect of implicit solvent on the formation of LNPs
To better understand the reasons behind why the solvents had utterly different influences on the formation of LNPs, computational simulation was executed to dig into the underlying mechanism at the molecular level. The guaiacylglycerol-beta-guaiacyl ether (GGE) (Fig. 2a), a lignin dimer,38 was utilized to investigate the inter/intra-molecular non-covalent interactions (NCIs) of the lignin molecule. NCIs were mainly based on the peaks which appeared in the reduced density gradient (RDG) at low densities.35 When the RDG (Fig. 2b and e) as a function of the density across the GGE molecules in the implicit GVL model was plotted, the appearance of a peak at low density (dashed rectangle region in Fig. 2b) was observed for the GGE configuration with the lowest energy. This peak with the green color indicated strong van der Waals (VDW) interactions present in the GGE molecule. Moreover, the isosurface with an RDG value of 0.5 exhibited a big green region between the two aromatic rings of GGE (Fig. 2d), unveiling that π–π stacking interactions (one type of strong van der Waals interactions) are present in the most stable GGE molecule (the lowest energy state), in which the aromatic rings were folded as the stacked configuration of GGE by the intramolecular π–π stacking interactions. In contrast, the GGE configuration from GVL with the second lowest energy was stretching due to the weak NCIs between its aromatic rings (Fig. 2c and e). In other implicit solvent models, GGE stacked and stretched configurations also existed simultaneously (Fig. S3†). As shown in Fig. 2f, their Boltzmann distribution for stacked and stretched GGE with different energies was counted in the implicit solvent model at 298.15 K. Generally, the GGE stacked configuration dominated over all of the configurations in the implicit solvent models. Of special note, in water, appropriately 90% of the configurations of GGE displayed intramolecular π–π stacking interactions, which further contributed to the following effective clustering into LNPs. The percentages of stacked configurations in organic solvents relatively decreased in the following order: GVL > THF > methanol > DMF > acetone > DMSO. Lower percentages of stacked configurations were observed in organic solvents than that in water meant that stretched lignin molecules would tend to fold into stacked ones when the lignin solution was added into water.
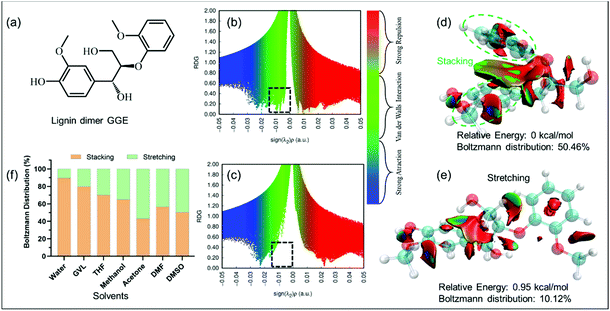 |
| Fig. 2 Theoretical configuration of lignin dimer model in distinct solvent systems. (a) chemical structure of lignin dimer model GGE, (b and c) Scatter graph and (d and e) isosurface (value = 0.5) of reduced density gradient (RDG) of GGE configurations with the two lowest energies, respectively, in the implicit GVL model (GGE: CPK model, C-cyan, H-white, O-red), and (f) Boltzmann distribution of GGE stacking and stretching configuration in the implicit solvent models. | |
Computational study on the effect of explicit solvent on the formation of LNPs
After attempting the stacked GGE interactions with the solvent molecules under the implicit water model, we found that varying solvent molecules had significantly different impacts on the stacking of GGE according to the structures and RDG isosurfaces shown in Fig. 3a–f. The GGE could be well solvated by all of the used solvents based on the big green RDG isosurface region between them. The two aromatic rings of GGE still remained in offset stacking (over 90% of total configurations) when the GGE interacted with GVL or THF molecules, resulting from the strong π–π interactions between the two rings with a big green isosurface region (Fig. 3a and b). It is worth noting that GVL had a weaker interaction with GGE, compared to that of THF (Fig. S4†), indicating that GVL can be more easily and thoroughly removed compared to THF when the lignin suspension was dialyzed against water. Solvation of GGE by methanol could laterally shift about 75% of two offset stacked aromatic rings, exhibiting an aromatic CH-π interaction. Acetone and DMF molecules also forced 31% and 100% of stacked GGE to laterally shift, respectively, where the methoxy group on the benzene ring interacted with another aromatic ring via aliphatic CH-π interactions. For DMSO, it can almost make two aromatic rings utterly stretch, suggesting that the stacking of GGE was completely destroyed due to the solvation of DMSO. Hence, there were mainly three categories of configurations including offset stacking, lateral shifting and stretching for GGE owing to the three types of intramolecular interactions including π–π, CH–π, and no interaction, respectively.
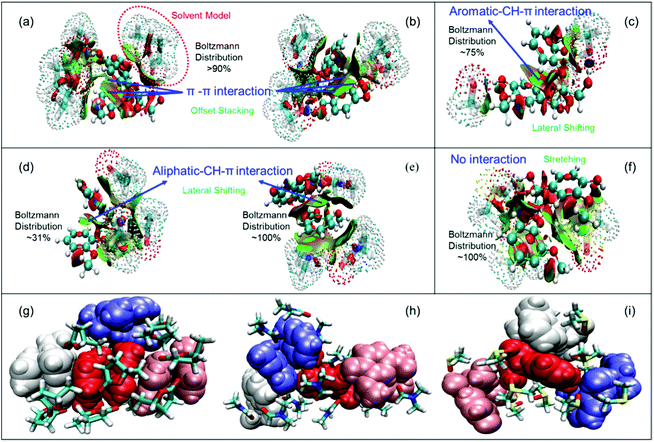 |
| Fig. 3 Intra/inter-molecular interactions of lignin models in distinct solvents. (a–f) Configurations and isosurfaces of GGE interacted with GVL, THF, methanol, acetone, DMF, and DMSO, respectively (GGE: CPK model; solvent molecules: solvent and licorice model, C-cyan, H-white, O-red, N-blue, S-yellow), and (g–i) cluster configurations of different GGEs including offset stacking, lateral shifting and stretching, respectively. | |
Fig. 3g–i further revealed the intermolecular interactions for these three kinds of GGEs. It showed that four offset-stacked GGEs can self-assemble via intermolecular π–π interactions, while the solvent molecules surrounded the stacked cluster. However, the laterally shifted and stretched GGEs could not aggregate tightly, which can be attributed to solvent molecules like DMF and DMSO filling in the spaces among GGEs. In other words, the strong solvation of DMF and DMSO for GGEs damaged the intramolecular stacking, leading to the separation of GGEs. Considering the Boltzmann distribution of GGE in the implicit solvent model in Fig. 2f and TEM images in Fig. 1, we can conclude that LNPs could not be formed from lignin DMF/DMSO solution mainly due to the disappearance of intramolecular π–π stacking configurations. Meanwhile, only a small volume of LNPs was obtained from lignin methanol/acetone solution due to partial destruction of the intramolecular stacking by methanol or acetone molecules. Nevertheless, GVL and THF can preserve intramolecular stacking, resulting in high yields of LNPs.
Quantification of intra/inter-molecular interactions of GGE
In order to better provide guidance for the synthesis of nano-lignin in the future, we quantified the π–π stacking effect between the intramolecular benzene rings of lignin caused by the solvent molecules. The energy decomposition analysis for GGE after optimization under various solvents shown in Fig. 3a–f based on the molecular forcefield (EDA-FF) was thereby executed in Multiwfn.39 The interaction of intramolecular aromatic rings of GGE is summarized in Table 1. We found that repulsion and dispersion effects simultaneously exist in intramolecular aromatic rings, while the dispersion played a dominant role, which resulted in the mutual attraction between them. The attraction (−5.54 kJ mol−1) between aromatic rings of GGE in GVL was as strong as that (−5.59 kJ mol−1) in water, meaning that the intramolecular π–π stacking was almost kept unchanged when lignin was dissolved in GVL/water. In such cases, four GGE molecules showed strong intermolecular interactions (−151.27 kJ mol−1), facilitating the aggregation of lignin molecules. In contrast, GGE in DMSO showed the smallest aromatic interaction (−3.13 kJ mol−1), resulting in the weakest intermolecular interactions. Both the repulsion and dispersion interactions were especially weak between the aromatic rings due to the stretched configuration as shown in Fig. 3f. Most importantly, the intramolecular aromatic interaction energy of GGE had a good correlation with the distribution of folding configurations and the yield of nano-lignin, indicating that this quantitative method can be used to predict the formation of nano-lignin.
Table 1 Energy decomposition analysis for GGE after optimization under various solvents
Solvent |
Repulsion (kJ mol−1) |
Dispersion (kJ mol−1) |
Aromatic inter-action (kJ mol−1) |
Inter-molecular inter-action (kJ mol−1) |
nc stands for no calculated data.
|
Water |
15.83 |
−21.42 |
−5.59 |
nca |
GVL |
15.05 |
−20.59 |
−5.54 |
−151.27 |
THF |
8.69 |
−14.09 |
−5.4 |
nc |
Methanol |
13.90 |
−18.78 |
−4.88 |
nc |
Acetone |
18.93 |
−23.11 |
−4.18 |
nc |
DMF |
14.75 |
−17.93 |
−3.18 |
−127.45 |
DMSO |
1.46 |
−4.59 |
−3.13 |
−92.42 |
Formation mechanism of LNPs
Additionally, uniform and clear spherical LNPs can be achieved by applying a centrifugal force with 10
000 g for 10 min to the lignin suspension from GVL or THF solvents (Fig. 4a). However, by increasing the centrifugal force up to 15
000 g, some tiny lignin nanoparticles with a diameter of about 3 nm can be observed on the surface of relatively large nanoparticles and in the background (Fig. 4b and c). When we performed the molecular simulation for a large softwood lignin model molecule with a molecular weight of 3675 g mol−1,40 which is approximately the size of a real lignin molecule, the stretched lignin molecule tended to curl up into a small spherical particle with a diameter of about 2.5 nm (Fig. 4d and e) under the implicit water model. The Gibbs free energy changing of this transformation was as low as −211 kcal mol−1, indicating that it could spontaneously occur in water. This result further confirmed the conclusion that intramolecular stacking interactions are a precursor to forming the final LNPs.
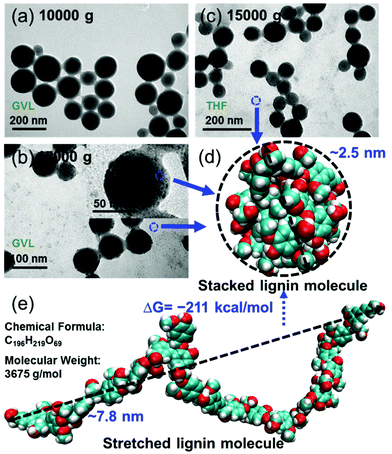 |
| Fig. 4 Experimental and theoretical morphologies of LNPs. (a–c) TEM images of LNPs from different centrifuging conditions, and (d–e) stacked and stretched configurations of a large softwood lignin molecule. | |
Combining all of the results and discussions above, we can propose a mechanism (Fig. 5) for LNP formation via the solvent exchange method. In the solvents with a relatively gentle solvating ability like GVL or THF, lignin molecules kept intramolecular stacking, where the intramolecular aromatic interaction of GGE is approximate to that (−5.59 kJ mol−1) in water, to form a spherical seed during the heterogeneous nucleation process. These nuclei formed a cluster when the lignin solution with a high concentration was dropped into the water. The formed clusters would further aggregate into LNPs based on diffusion-limited cluster−cluster aggregation (DLCA), a classical nucleation theory. With the improvement of solvating ability, aromatic rings of lignin molecules would be pulled away from intramolecular stacking by solvent molecules like DMSO, preventing lignin molecules from forming spherical cores and aggregating to form LNPs. Therefore, to produce uniform and stable LNPs with high yields via the solvent exchange method, solvents which not only dissolve lignin, but also preserve its spherical cores should be desirable.
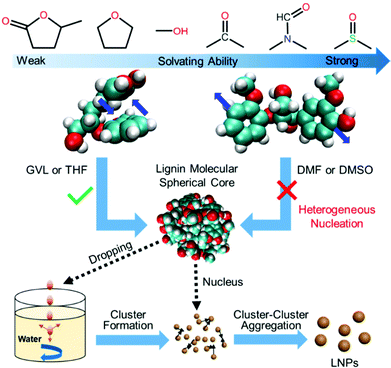 |
| Fig. 5 Formation mechanism of LNPs from different solvents. | |
Effect of solvents on the one-step synthesis of LNP supported AuNPs
After understanding the formation mechanism of LNPs from different solvents, the feasibility of its functionalization was further assessed. Gold nanoparticles (AuNPs), as an efficient near-infrared (NIR) photothermal conversion agent, have been widely used for eradicating tumors by photothermal therapy (PTT).41 Similar to the preparation of LNPs, lignin in different solvents was, respectively injected into an aqueous chloroauric acid solution to produce AuNPs at room temperature. A surface plasmon resonance (SPR) peak (at ∼540 nm) of AuNPs was observed in the absorption spectra of all of the samples (Fig. 6a), indicating that lignin in all kinds of solvents can reduce Au3+ to form AuNPs. Their XPS spectra also demonstrated the generation of Au0 as shown in Fig. S5.† However, the SPR strength (directly proportional to the yield) of AuNPs from different solvents was quite different, following the order: GVL > THF > methanol > acetone > DMF > DMSO. Interestingly, the yield of AuNPs increased with the decrease of solvating ability of solvents which dissolved the lignin. This result was consistent with the formation of LNPs. Besides the yields, we found that the products from methanol, acetone, DMF and DMSO lignin solutions were particularly unstable. Their hydrodynamic diameters could reach a few micrometers within 2 hours of reaction time. It can also be seen from Fig. 6e–h that a few AuNPs (particles with a dark color in TEM images) scattered on the large lignin networks. Besides, this instability was also proved by the obvious phenomenon that particles completely precipitated in the bottoms of centrifuge tubes after keeping these suspensions standing for 1 day (Fig. S6†). In contrast, the particles from GVL and THF lignin solution had relatively stable and small sizes in the range of the nanometer scale (Fig. 6b). From the TEM images (Fig. 6c and d), it can be seen that AuNPs were stacked onto the surface of spherical LNPs as the Au-LNPs. When adding the GVL-lignin solution to a chloroauric acid solution containing a different volume of GVL solvent, we can observe that increasing the GVL volume would impair the yield and stabilities of AuNPs due to a decrease in the LNP yields (Fig. S7†). Meanwhile, ferulic acid, a natural building block of lignin, was dissolved in the same set of solvents as the contrast reducing agent for the synthesis of AuNPs. Ferulic acid can also reduce Au3+ to Au0 in 10 minutes, which was confirmed by the SPR peak in the UV-Vis absorption spectra (Fig. S8†). However, in comparison with lignin, all the ferulic acid-reducing AuNPs from the set of solvents quickly aggregate into bulk particles beyond the micro-meter scale (Fig. S9†), indicating that ferulic acid and solvent alone cannot work as a good stabilizer for AuNPs. These results suggest that spherical LNPs should be formed prior to the generation of AuNPs to gain stable AuNPs. In addition, the Au-LNPs from the GVL lignin solution almost maintain an unchangeable nature with a diameter of ∼120 nm for one day (Fig. 6b and c), much smaller and more stable than the case from THF. Given the similar solvating abilities between GVL and THF, we further investigated the reactivity between GVL and chloroauric acid. Interestingly, we found that GVL can be hydrolyzed to 4-hydroxyvaleric acid (HVA) in the presence of chloroauric acid, which functioned as a chelating agent synergistically with LNPs to stabilize the AuNPs, as shown in Fig. S10† using COSY-NMR analysis. Both of the obtained nanoparticles including LNPs and Au-LNPs produced from GVL-lignin solution kept stable with a diameter in the range of 120 to 150 nm for several days as shown in Fig. S11.† The heating curves (Fig. S12†) proved that the obtained LNP supported AuNPs also have an excellent photothermal effect. Moreover, it can simultaneously and efficiently load drugs during this one-pot synthesis of LNP-supported AuNPs to integrate more functions into lignin (Data were not shown in this work).
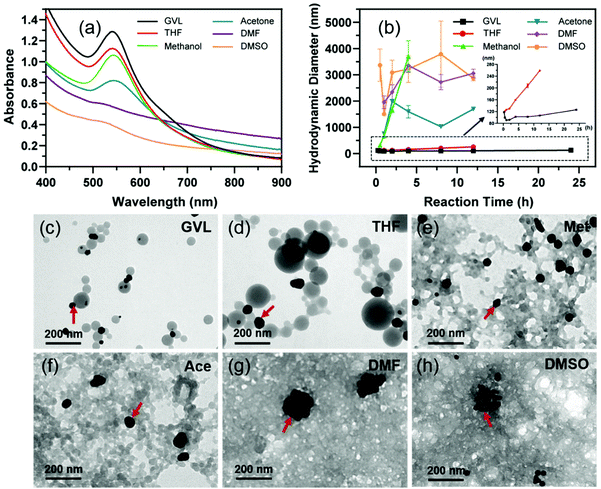 |
| Fig. 6 Characterization of Au-LNPs produced from distinct solvent systems. (a) UV-Visual spectra, (b) hydrodynamic diameters, and (c–h) TEM images of Au-LNPs from different solvents. | |
Conclusions
In summary, we initiated a new formation mechanism of LNPs at the molecular level by combining experimental and computational methods to guide the one-step multifunctionalization of lignin. We found that relatively moderate solvents like GVL preserved the intramolecular stacking of lignin molecules to form a spherical core as the nucleus for the growth of uniform and stable LNPs. GVL also assisted LNPs in stabilizing the functionalization due to its hydrolysis product with great chelating ability. It is worth noting that their abilities should not be limited to the functions presented in this study. For example, lignin can also assist in the synthesis and stabilization of diverse inorganic nanoparticles, and absorb many other cargoes with versatile properties. It will pave a broad avenue for lignin valorization for sustainable economic development. Meanwhile, we provided a quantitative method to characterize the intramolecular interaction of GGE associated with solvent molecules for reducing lots of unnecessary attempts on the LNP production and functionalization in the future.
Author contributions
The manuscript was written with contributions from all of the authors. All of the authors have given approval to the final version of the manuscript.
Conflicts of interest
The authors declare no competing financial interest.
Acknowledgements
This work was financially supported by the Fundamental Research Funds for the Central Universities (no. 21619301), the National Natural Science Foundation of China (nos. 51903104, 21808081, 22038004 and 31870943), the Natural Science Foundation of Guangdong Province (no. 2019A1515011279), the Science and Technology Project of Guangdong Province (no. 2018A050506019), the Guangdong Province Key Field R&D Program Project (no. 2020B1111380002) and the International Science and Technology Cooperation Project of Huangpu District/Guangzhou Development District (nos. 2018GH16 and 2019GH11).
Notes and references
- H. Zhou, Z. Li, S.-M. Xu, L. Lu, M. Xu, K. Ji, R. Ge, Y. Yan, L. Ma, X. Kong, L. Zheng and H. Duan, Angew. Chem., Int. Ed., 2021, 60, 8976–8982 CrossRef CAS PubMed
.
- M. Y. Balakshin, E. A. Capanema, I. Sulaeva, P. Schlee, Z. Huang, M. Feng, M. Borghei, O. J. Rojas, A. Potthast and T. Rosenau, ChemSusChem, 2021, 14, 1016–1036 CrossRef CAS PubMed
.
- M. Ago, B. L. Tardy, L. Wang, J. Guo, A. Khakalo and O. J. Rojas, MRS Bull., 2017, 42, 371–378 CrossRef CAS
.
- W. D. H. Schneider, A. J. P. Dillon and M. Camassola, Biotechnol. Adv., 2021, 47, 107685 CrossRef CAS PubMed
.
- M. H. Sipponen, H. Lange, C. Crestini, A. Henn and M. Osterberg, ChemSusChem, 2019, 12, 2039–2054 CrossRef CAS
.
- L. Chen, X. Zhou, Y. Shi, B. Gao, J. Wu, T. B. Kirk, J. Xu and W. Xue, Chem. Eng. J., 2018, 346, 217–225 CrossRef CAS
.
- L. Siddiqui, J. Bag, D. Mittal, A. Leekha, H. Mishra, M. Mishra, A. K. Verma, P. K. Mishra, A. Ekielski, Z. Iqbal and S. Talegaonkar, Int. J. Biol. Macromol., 2020, 152, 786–802 CrossRef CAS
.
- A. P. Richter, J. S. Brown, B. Bharti, A. Wang, S. Gangwal, K. Houck, E. A. C. Hubal, V. N. Paunov, S. D. Stoyanov and O. D. Velev, Nat. Nanotechnol., 2015, 10, 817–823 CrossRef CAS PubMed
.
- P. Figueiredo, K. Lintinen, A. Kiriazis, V. Hynninen, Z. Liu, T. Bauleth-Ramos, A. Rahikkala, A. Correia, T. Kohout, B. Sarmento, J. Yli-Kauhaluoma, J. Hirvonen, O. Ikkala, M. A. Kostiainen and H. A. Santos, Biomaterials, 2017, 121, 97–108 CrossRef CAS
.
- E. Lizundia, M. H. Sipponen, L. G. Greca, M. Balakshin, B. L. Tardy, O. J. Rojas and D. Puglia, Green Chem., 2021, 23, 6698–6760 RSC
.
- M. H. Sipponen, M. Farooq, J. Koivisto, A. Pellis, J. Seitsonen and M. Österberg, Nat. Commun., 2018, 9, 2300 CrossRef PubMed
.
- Z. Tu, W. Liu, J. Wang, X. Qiu, J. Huang, J. Li and H. Lou, Nat. Commun., 2021, 12, 2916 CrossRef CAS PubMed
.
- M. H. Sipponen, H. Lange, M. Ago and C. Crestini, ACS Sustainable Chem. Eng., 2018, 6, 9342–9351 CrossRef CAS PubMed
.
- W. Zhao, L.-P. Xiao, G. Song, R.-C. Sun, L. He, S. Singh, B. A. Simmons and G. Cheng, Green Chem., 2017, 19, 3272–3281 RSC
.
- I. V. Pylypchuk, P. A. Linden, M. E. Lindstrom and O. Sevastyanova, ACS Sustainable Chem. Eng., 2020, 8, 13805–13812 CrossRef CAS
.
- L. Chen, J. Dou, Q. Ma, N. Li, R. Wu, H. Bian, D. J. Yelle, T. Vuorinen, S. Fu, X. Pan and J. Zhu, Sci. Adv., 2017, 3 Search PubMed
.
- C. Frangville, M. Rutkevicius, A. P. Richter, O. D. Velev, S. D. Stoyanov and V. N. Paunov, ChemPhysChem, 2012, 13, 4235–4243 CrossRef CAS PubMed
.
- P. Figueiredo, K. Lintinen, J. T. Hirvonen, M. A. Kostiainen and H. A. Santos, Prog. Mater. Sci., 2018, 93, 233–269 CrossRef CAS
.
- B. Wang, G. Yang, J. Chen and G. Fang, Nanomaterials, 2020, 10 CAS
.
- S. Chen, G. Wang, W. Sui, A. M. Parvez and C. Si, Green Chem., 2020, 22, 2879–2888 RSC
.
- M. S. Alqahtani, M. Kazi, M. Z. Ahmad, R. Syed, M. A. Alsenaidy and S. A. Albraiki, Int. J. Biol. Macromol., 2020, 163, 1314–1322 CrossRef CAS
.
- J. Wang, Y. Qian, L. Li and X. Qiu, ChemSusChem, 2020, 13, 4420–4427 CrossRef CAS
.
- L. Chen, Y. Shi, B. Gao, Y. Zhao, Y. Jiang, Z. Zha, W. Xue and L. Gong, ACS Sustainable Chem. Eng., 2020, 8, 714–722 CrossRef CAS
.
- J. Y. Zhu, U. P. Agarwal, P. N. Ciesielski, M. E. Himmel, R. Gao, Y. Deng, M. Morits and M. Österberg, Biotechnol. Biofuels, 2021, 14, 114 CrossRef CAS PubMed
.
- M. D. Liptak and G. C. Shields, J. Am. Chem. Soc., 2001, 123, 7314–7319 CrossRef CAS PubMed
.
- R. F. Ribeiro, A. V. Marenich, C. J. Cramer and D. G. Truhlar, J. Phys. Chem. B, 2011, 115, 14556–14562 CrossRef CAS PubMed
.
- S. Spicher and S. Grimme, Angew. Chem., Int. Ed., 2020, 59, 15665–15673 CrossRef CAS PubMed
.
- C. Bannwarth, S. Ehlert and S. Grimme, J. Chem. Theory Comput., 2019, 15, 1652–1671 CrossRef CAS PubMed
.
- J. G. Brandenburg, C. Bannwarth, A. Hansen and S. Grimme, J. Chem. Phys., 2018, 148, 064104 CrossRef PubMed
.
- S. Grimme, J. Antony, S. Ehrlich and H. Krieg, J. Chem. Phys., 2010, 132, 154104 CrossRef PubMed
.
-
T. Lu, Beijing Kein Research Center for Natural Science, China, 2016, DOI: http://www.keinsci.com/research/molclus.html Search PubMed
.
- E. R. Johnson, S. Keinan, P. Mori-Sánchez, J. Contreras-García, A. J. Cohen and W. Yang, J. Am. Chem. Soc., 2010, 132, 6498–6506 CrossRef CAS PubMed
.
- T. Lu and F. Chen, J. Comput. Chem., 2012, 33, 580–592 CrossRef CAS
.
- W. Humphrey, A. Dalke and K. Schulten, J. Mol. Graphics, 1996, 14, 33–38 CrossRef CAS
.
- C. Lefebvre, G. Rubez, H. Khartabil, J.-C. Boisson, J. Contreras-García and E. Hénon, Phys. Chem. Chem. Phys., 2017, 19, 17928–17936 RSC
.
- L. P. Novo and A. A. S. Curvelo, Ind. Eng. Chem. Res., 2019, 58, 14520–14527 CrossRef CAS
.
- K. Lintinen, Y. Xiao, R. Bangalore Ashok, T. Leskinen, E. Sakarinen, M. Sipponen, F. Muhammad, P. Oinas, M. Österberg and M. Kostiainen, Green Chem., 2018, 20, 843–850 RSC
.
- T.-f. Su, R. Huang, Y.-q. Su, G.-z. Zhao, D.-y. Wu, J.-a. Wang, C.-r. Gong and C.-l. Xu, Spectrochim. Acta, Part A, 2015, 139, 456–463 CrossRef CAS
.
- T. Lu, Z. Liu and Q. Chen, Mater. Sci. Eng. B, 2021, 273, 115425 CrossRef CAS
.
- J. Ralph, C. Lapierre and W. Boerjan, Curr. Opin. Biotechnol., 2019, 56, 240–249 CrossRef CAS PubMed
.
- M. R. K. Ali, Y. Wu and M. A. El-Sayed, J. Phys. Chem. C, 2019, 123, 15375–15393 CrossRef CAS
.
Footnotes |
† Electronic supplementary information (ESI) available. See DOI: 10.1039/d1gc03651c |
‡ These authors contributed equally. |
|
This journal is © The Royal Society of Chemistry 2022 |
Click here to see how this site uses Cookies. View our privacy policy here.