Ambient characterisation of PurpleAir particulate matter monitors for measurements to be considered as indicative†
Received
11th July 2022
, Accepted 8th September 2022
First published on 14th September 2022
Abstract
Low-cost particulate matter systems output airborne particle mass concentrations based on optical particle counter sensors. Because the relationship between the particle number and mass is complex and varies with time and space, the research community has developed correction methodologies which imply co-locating a low-cost system and a reference-grade instrument. Such a requirement may not always be achievable. This work aims at supporting users unable to perform co-locations for calibration, providing characterization in terms of indicative measurements. Nine different PurpleAir units in total were deployed (up to 8 units at a time) in parallel with a GRIMM monitor during three different measurement periods in the cities of Berlin and Potsdam, Germany. The three measurement periods encompass over 80 days in total and span from December 2017 to July 2019. We compare the low cost systems' performances against the research-grade measurements and investigate the transferability of correction functions between systems and campaigns. Given the specificity of the correction functions (systems and campaigns), we explore the possibility of their use as indicative measurements under the European legislation specifications as a function of temperature and relative humidity. The results are compiled into a database, the PurpleAir bidimensional look-up matrix, BLUM-i, that is freely available and comes with software for the analysis of PurpleAir uncalibrated data. The BLUM-i is designed as an open-source database application to provide the probability that measurements meet the criteria for indicative measurements as defined in European legislation. The BLUM-i can be continually improved by including more data.
Environmental significance
The development of low-cost sensors for air quality measurements has made such measurements accessible to a broad set of users. Unfortunately, data quality is often still an issue, with robust measurements requiring substantial resources for calibration and data processing. Here we present a look-up matrix (BLUM-i1) for a widely used low-cost particulate matter sensor (PurpleAir) that would allow all users a straight-forward way to evaluate and understand the reliability of their measurements based on widely available environmental conditions (temperature and relative humidity data). The matrix has been developed using a series of co-location experiments under various environmental conditions where PurpleAir sensors were compared to reference instrumentation and provides the likelihood that a measurement meets the data quality criteria for being ‘indicative’ as defined by the EU Air Quality Directive. The BLUM-i matrix can easily be expanded and made more robust through the addition of further co-location data.
|
Introduction
In a world where the most prominent risks are all environmental,2 air pollution stands out as the main global health-impacting environmental issue.3 A global total of 8.8 million premature deaths due to outdoor air pollution by particulate matter (PM) and ozone were estimated for 2015,4 with most adverse effects attributed to PM.5 A meta-analysis of data from over 600 cities worldwide reviewed the concentration–response associations of PM with all-cause mortality and found them to be positively associated, with no discernible threshold and at levels below most global and regional air quality guidelines.6
The European Air Quality Directive sets out the limit and target values for air pollutants, as well as requirements for the measurements. For PM, the standard is a gravimetric measurement method to determine the mass concentration. In terms of data quality, the uncertainty associated with the PM measurements cannot exceed 25%. For indicative measurements, the uncertainty cannot exceed 50%.7 The existing monitoring networks have produced long term time series, useful to determine the effect of policies (large-scale in space and time) or evaluate targets.8 For certain applications (e.g. determining exposures or assessing the effect of a policy at a very localized scale), air pollution measurements at a higher density or in locations differing from those of traditional infrastructure can be desirable but often difficult or impossible.
Low-cost sensors are versatile on the temporal and the spatial scales when compared to traditional infrastructure.9,10 A synergy between traditional monitoring networks, low-cost sensors, modelling and satellite-based measurements could overcome the limitations of the present monitoring schemes.11–15 For example, current networks may increase their capacities through the deployment of distributed networks of sensors,11,16–19 static and mobile20–23 with high time resolution capacities to help identify hot spots and short-term emission spikes,24–29 of great relevance for, e.g., human exposure assessment,30,31 or to develop real-time strategies.32
By bringing air quality data closer to a broader set of stakeholders (citizens, industry, scientists and policy-makers), e.g. under the scope of citizen-science projects, low-cost sensors can be understood as a tool of transformative research33,34 towards behavioral change and effective policy-making.35,36
The literature characterizing low-cost sensor performance and evaluating the applicability of low-cost sensors for specific use cases is growing quickly.37–39 A low-cost system (LCS) is understood as a low-cost sensor within a platform comprising other devices (global positioning units, mobile telecommunication units, temperature and relative humidity sensors, etc…) and electronics.40,41 The evaluation of low-cost sensors and systems is often investigated in a context with limited variability in terms of environmental conditions.42 While such evaluations are both relevant and necessary to quantify performance, the results will not necessarily hold true for the same type of LCS used in a different context or across a set evaluated in parallel, leading to less precise measurements.43,44 This is largely owing to the difficulties in computing the PM mass from particle counts.45,46 In other words, the dimensions measured by using the LCS (six size bins, temperature, T, and relative humidity, RH, for the PurpleAir) are limited when providing the high-dimensionality of the complex relationship between the PM number and dry mass.
The transferability and applicability are therefore circumscribed to applications similar to those under which the LCS was calibrated. Units of a same LCS model may exhibit high reproducibility47 but may as well perform significantly disparately.29,48 This implies that, when using a LCS for applications which require reference-grade quality, frequent and rigorous calibrations under conditions which approximate the meteorological and emission conditions of the targeted area are needed.29,49–51 Among the types of LCS users (e.g. citizen-science actors, etc.), not all will have the possibility to calibrate frequently or may lack access to the detailed data needed to implement a correction.52,53 Despite the limitations faced by such users, this should not mean that the data lack usefulness.
In the present study, we acknowledge the limitations of the PM LCS, and the possible variations in data quality among LCSs that are identical in terms of design and components. In place of seeking to derive specific relationships to retrieve concentrations that are as accurate as possible, we propose general characterisation of the use of PurpleAir systems in terms of indicative measurements across a variety of conditions. We aim to address the cohort of users that do not have access to or the means with which to carry out regular calibrations and/or apply correction approaches. The characterisation is reported in the form of a probability that a measurement meets the requirements to be ‘indicative’, as a function of ambient meteorological variables also reported by the PurpleAir systems. This takes the form of a bidimensional look-up matrix (the PurpleAir BLUM-i). A machine-readable version of the PurpleAir BLUM-i is open access and easily applicable to the output of any PurpleAir system for the evaluation of indicative measurements.1 We believe that the product is thus useful for actors, e.g., those outside the research community, who use PurpleAir systems for PM monitoring that do not have access to further resources, such as reference instrumentation, for regular calibration. What we present here is an initial dataset to populate the PurpleAir BLUM-i; however, the product is open to new inputs of data, which would make it more accurate and robust.
Instruments and data
PurpleAir low cost systems
The PurpleAir systems use laser particle counters (Plantower PMS5003 for all 9 units of this study) to quantify particulates. PMS5003 sensors come factory calibrated and count suspended particles in six size bins: >0.3, >0.5, >1.0, >2.5, >5.0, and >10 μm (https://www2.purpleair.com/pages/technology). The distribution of the 9 units through the 3 campaigns is given in Table 1.
Table 1 List of the PurpleAir systems used in the campaigns and coefficients of variation (average ± standard deviation) for PM2.5
System |
PurpleAir ID |
Winter |
Spring |
Summer |
A |
5C CF 7F 5C 9C 97 |
0.19 ± 0.21 |
|
|
B |
5C CF 7F 5C 9D CF |
0.10 ± 0.15 |
|
0.14 ± 0.17 |
C |
60 1 94 4B 29 C6 |
0.13 ± 0.14 |
|
|
D |
60 1 94 4B 2B 47 |
0.15 ± 0.15 |
|
0.10 ± 0.13 |
E |
60 1 94 58 A4 37 |
0.11 ± 0.13 |
|
|
F |
60 1 94 58 EC 95 |
0.09 ± 0.12 |
0.55 ± 0.62 |
|
G |
60 1 94 58 F1 47 |
0.11 ± 0.18 |
|
|
H |
60 1 94 59 AA E |
0.07 ± 0.11 |
|
0.15 ± 0.13 |
I |
84 F3 EB 45 42 31 |
|
0.08 ± 0.08 |
|
The PMS 5003 model has a fan to pull air through the sensor.54–57 The particle counts are based on the amount of light (680 nm) scattered at a 90° angle56,58 and processed by the system (PurpleAir firmware version 2.50i) to output the PM1, PM2.5 and PM10 mass concentrations (μg m−3) every minute. PM mass concentrations are reported uncorrected (“CF = 1”, suitable for indoor environments) or corrected using a proprietary algorithm (“ATM”, suitable for outdoor environments, used in the present study).
Each PurpleAir system has two channels (two PMS5003 sensors), which measure PM independently and thereby provide a precision check on the data. The system also outputs readings for T and RH.
Precision and inter-channel QA/QC
Precision was determined for each system and campaign-wise by means of the coefficient of variation (CV):43,44where σ is the standard deviation and μ is the average of the coincident concentrations reported by both sensors within a system.
The data underwent a quality control check to be included in the study. The PurpleAir system documentation states that the uncertainty associated with each channel is 15 μg m−3 (for concentrations below 100 μg m−3) or 15% (for concentrations above 100 μg m−3). We consider measurements where the difference between the two channels is within the combined uncertainty as passing the quality check. If the absolute difference between the two channels (A and B) is larger than the combination of the errors from both cells (following the law of error propagation), they do not pass the quality check.
GRIMM samplers
The PurpleAir systems were co-located with a GRIMM sampler, either an EDM 164, manufacturer-calibrated at the end of 2018 or an EDM 165, which had recently been calibrated at the Forschungszentrum Jülich. These report PM1, PM2.5 and PM10 every minute via the detection of the scattering by single particles (660 nm) in 31 size bins between 0.25 and 32 μm. Both GRIMM models also have a small meteorological station, from which the T and RH readings were used in this study. Although we acknowledge that they do not correspond to the actual reference methodology, the GRIMM samplers were used as the reference instrumentation and are referred to as research-grade hereafter.
Sampling locations
The PurpleAir systems were co-located with a research-grade instrument at two different locations in Germany (the campus of the Humboldt Universität zu Berlin (HU) and the Institute of Advanced Sustainability Studies (IASS), Potsdam) between 2018 and 2019 (Table 2). LCS availability at the time of the campaign dictated which LCS and how many of them were used, and is detailed in Table 1. The co-locations at the HU were part of a larger measurement campaign.59
Table 2 Dates, locations and meteorological conditions for the three sampling campaigns
Campaign |
Start date end date |
Sampling site |
Temperature °C |
Relative humidity (−) |
Winter |
8.3.2018 |
HU |
Min.: −5.9 |
Min.: 0.23 |
31.3.2018 |
Berlin |
Max.: 16 |
Max.: 0.99 |
Spring |
8.5.2019 |
IASS |
Min.: 3.1 |
Min.: 0.22 |
29.5.2019 |
Potsdam |
Max.: 27 |
Max.: 1.0 |
Summer |
30.5.2019 |
IASS |
Min.: 7.3 |
Min.: 0.23 |
9.7.2019 |
Potsdam |
Max.: 40 |
Max.: 1.0 |
The measurement site at the HU was located in between buildings of the Geographical Institute on a fenced-in green space (Alexander von Humboldt weather station, https://hu.berlin/wetter, on the outskirts of the city away from any high-traffic roads) at ground level. The instruments were attached to metal rods approximately 1.5 m above the ground. This location would be similar to a suburban background station.
The measurement site at the IASS was located on a balcony (first floor, European counting, approximately 15 m from the road kerbside) facing a main thoroughfare (Berlinerstrasse, two car lanes with tram tracks in the center) leading into central Potsdam. The location is otherwise largely residential with some commercial properties mixed in, including substantial green space and trees, and would have some elevated concentrations because of the proximity to the road, but would more generally be described as an urban background location.
PurpleAir bias and calibrations
The bias of the PurpleAir systems was evaluated against the co-located GRIMM using the following equation: | System bias = (system − GRIMM)/GRIMM | (2) |
where system is the uncalibrated concentration output by the PurpleAir system and GRIMM is the coincidental concentration from the co-located GRIMM monitor.
The use of a LCS is often framed by a calibration, empirically or based on theoretical considerations, to retrieve concentrations which are as accurate as possible.42,60 The calibration occurs by co-locating the LCS and a reference instrument for a certain amount of time, before, after or between measurement campaigns.51 Calibration approaches (for a single LCS or a network of LCS units), recently reviewed,61 comprise several techniques.19,21,47,62 Here, we explore ordinary least squares to build a linear relationship between the PurpleAir uncalibrated output and the research-grade instrument. The slope and the intercept of the linear functions are obtained using the LCS uncalibrated concentrations as independent variables and the coincident research-grade concentrations as dependent variables. The linear equation thus obtained has the form:
| Calibrated concentration = m × LCS concentration + b | (3) |
The necessity of such a correction derives from the complex relationships between the PM number and the dry mass and between the particle morphology and the light scattered. The factors influencing those relationships are both ambient (e.g. T, RH, local concentrations of organic and inorganic gaseous species which have the ability to partition between the gas and the particulate phases as PM ages and meteorology evolves) and source-specific (e.g. the aerosol size distribution and its chemical composition which control its hygroscopicity and chemical ageing),50,63 resulting in non-linear behaviours.55,56,62,64,66,67 Reference instruments have a dryer placed at the inlet to remove water and measure dry mass. LCSs do not generally heat the inlet and therefore RH affects the LCS light-scattering response and the mass concentration output due to deliquescent growth and droplet formation.66,68,69
Although some field studies did not consider environmental RH and T for calibration or correction,70 others found no influence,71 while still others successfully corrected PM readings when considering RH.48,53,58,65,72 Some studies discarded the influence of temperature,66 while others obtained the best results when including it in the correction model.47,53,72,73 In order to investigate the bias and the calibration of the deployed systems' PM readings against the co-located research-grade methodology, we use five-minute averages in order to retain the high temporal resolution characteristics of the LCS.
Statistical analysis
In order to test the equivalence of the calibration functions, three statistical tests were used: (1) a t-test on the slopes, (2) a t-test on the intercepts and (3) a Kolmogorov–Smirnov test comparing the calibrated values. Hypothesis tests are interpreted in terms of p-values after a Bonferroni correction: the threshold for significance is set at a p-value of 0.05/12. In the first test, each calibration's slope is compared to that of the remaining calibrations using a t-test on the slope's pair. If the slopes are identical, the t-test will show that the means are equal (null hypothesis). The procedure for the intercepts is similar. Finally, each calibration is compared to the remaining ones with a Kolmogorov–Smirnov test on the calibrated values. The PurpleAir output for a given sensor/campaign pair is calibrated with both the linear models derived from its data and from each one of the other LCS/campaign pairs. The concentrations yielded by both models are tested with the Kolmogorov–Smirnov test. If the calibration functions are equivalent, the Kolmogorov–Smirnov test is expected to show that the yielded calibrated concentrations come from the same distribution (null hypothesis).
Indicative measurements
Indicative measurements are required to have, at most, an uncertainty of 50%
7 and have a similar status, in terms of requirements and applicability, as modelling data. In the following, we refer to an indicative measurement as one deviating from the GRIMM (reference) measurement by 50% or less.
The bidimensional look-up matrix, BLUM-i
The BLUM-i is a matrix that provides the probability that a given measurement meets the criteria of an indicative measurement as defined by European air quality legislation. As outlined in the introduction, we are addressing the user base that will not be able to (frequently) calibrate their PurpleAir sensors. To assist such users in extracting valuable information from their data, we compiled the data on performance in terms of indicative measurements as a function of T and RH in a bidimensional matrix where T and the RH are binned into 10 intervals. For each pair of (T, RH) bins a corresponding average performance is provided. This performance statistic is the fraction of the measurements from the co-locations that correspond to the (T, RH) pairs that meet the criteria for indicative measurements. The standard deviation of the reported average is given in a similar matrix. The same information (average and standard deviation) is also made available in a machine-readable format (the bidimensional look-up matrix, BLUM-i) for ease-of-use.1
Performance analysis
Precision and inter-channel QA/QC
The coefficient of variation is given in Table 1 for each system and campaign for PM2.5.
The results of the quality check are shown in Fig. 1. The data in the unshaded areas in Fig. 1 meet the quality check criteria as outlined in the methods section. The measurement pairs in the shaded areas (which do not pass the inner-sensor, intra-channel, QA/QC) reach a maximum of 4% for a given campaign, sensor and fraction. Fig. 1 shows that large swaths of points in the shaded areas occur for a single unit at a time, and not for several of the co-located units. This excludes environmental effects and evidences the unit-specificity of such episodes.
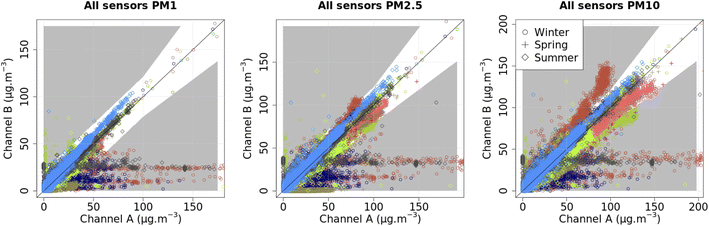 |
| Fig. 1 Inter-channel performance of the PurpleAir systems for PM1, PM2.5 and PM10 (1 minute readings). Each color represents an individual system/campaign pair. The shaded areas represent measurements that do not meet the QA/QC criteria. | |
Inter-unit performance
The time series (Fig. 2, for PM2.5, similar plots for PM1 and PM10 in the ESI) show that co-located units generally capture coincident low and high concentration episodes, although possibly with different intensities. This is an indication that correction functions may be unit-specific, invalidating their transferability between units, even if co-located for calibration (Table 3).
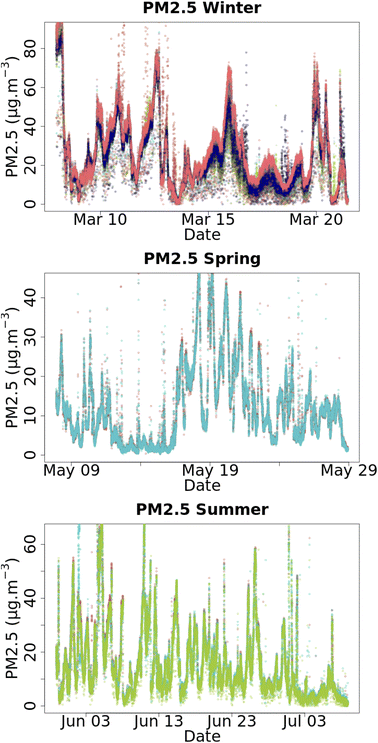 |
| Fig. 2 PM2.5 time series for the 3 campaigns. The different PurpleAir systems are differentiated by color. | |
Table 3 Bias (average ± standard deviation, 10th and 90th percentiles) of the PurpleAir systems for PM2.5
System |
PurpleAir ID |
Winter |
Spring |
Summer |
A |
5C CF 7F 5C 9C 97 |
0.59 ± 0.39, 0.13, 1.1 |
|
|
B |
5C CF 7F 5C 9D CF |
0.74 ± 0.31, 0.32, 1.1 |
|
0.52 ± 0.71, 0.08, 0.97 |
C |
60 1 94 4B 29 C6 |
0.63 ± 0.30, 0.23, 1.0 |
|
|
D |
60 1 94 4B 2B 47 |
0.67 ± 0.39, 0.12, 1.2 |
|
0.57 ± 0.77, 0.09, 1.1 |
E |
60 1 94 58 A4 37 |
0.66 ± 0. 34, 0.19, 1.1 |
|
|
F |
60 1 94 58 EC 95 |
0.49 ± 0.31, 0.10, 0.95 |
0.50 ± 0.60, 0.08, 0.85 |
|
G |
60 1 94 58 F1 47 |
0.54 ± 0.34, 0.10, 1.0 |
|
|
H |
60 1 94 59 AA E |
0.78 ± 0.34, 0.29, 1.2 |
|
0.50 ± 0.70, 0.07, 0.90 |
I |
84 F3 EB 45 42 31 |
|
0.51 ± 0.60, 0.09, 0.86 |
|
PurpleAir bias and calibrations
Fig. 3 shows the LCS and campaign-specific linear calibrations for each PM fraction. The calibration lines are not identical. Thus, given the same LCS response (independent data), a different calibrated concentration will be output from the different calibrations. This indicates that calibrations are possibly system and/or campaign specific. In particular, when a LCS is deployed and no calibration is available, one should not use the calibration from another, yet identical, system. The differences in bias (Table 3) within a same campaign or for a same LCS also indicates the specificity of the calibrations.
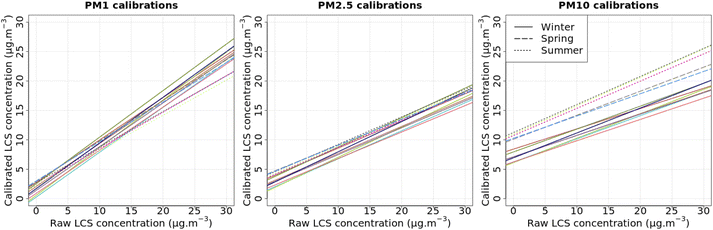 |
| Fig. 3 Linear calibration of the LCS PM measurements. Each color represents an individual LCS/campaign pair. Line styles distinguish the campaigns: winter (solid lines), spring (dashed lines) and summer (dotted lines). | |
Statistical analysis
We further investigate the possible specificity of the calibrations by comparing each calibration within a PM fraction (PM1, PM2.5 and PM10) to the remaining ones using three statistical tests: (1) a t-test on the slopes, (2) a t-test on the intercepts and (3) a Kolmogorov–Smirnov test comparing the calibrated values. Hypothesis tests are interpreted in terms of p-values after a Bonferroni correction: the threshold for significance is set at a p-value of 0.05/12. Similar plots for PM1 and PM10 are provided in the ESI. Fig. 4 shows the results of the comparison of each calibration's slope to that of the remaining calibrations using a t-test on the slope's pair. Fig. 5 shows the results of the t-test comparing the intercepts from the calibration. Fig. 6 shows the results of the Kolmogorov–Smirnov test comparing the calibrated values. Each calibration is compared to the remaining ones with a Kolmogorov–Smirnov test on the calibrated values. Both the X and Y axes represent a single system/campaign pair. The PurpleAir output for the system/campaign pair on the X axis is calibrated with both the linear models from the system/campaign pairs on the X and Y axes. The concentrations yielded by both models are tested with the Kolmogorov–Smirnov test. If the calibration functions are equivalent, the Kolmogorov–Smirnov test is expected to show that the yielded calibrated concentrations come from the same distribution.
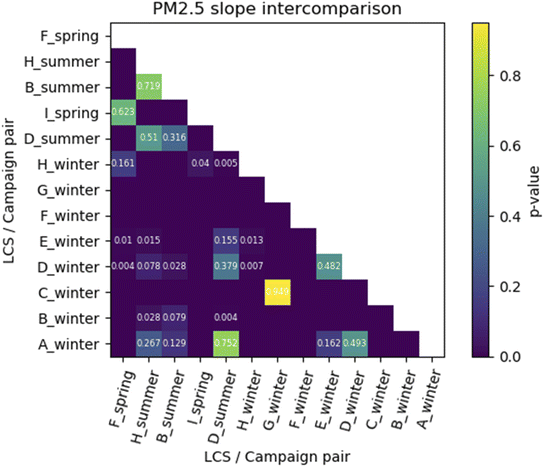 |
| Fig. 4 Results of the statistical t-tests for the PM2.5 data. Only the p-values which indicate a null difference in means (p-value > 0.05, with a subsequent Bonferroni correction) are written on the plot. | |
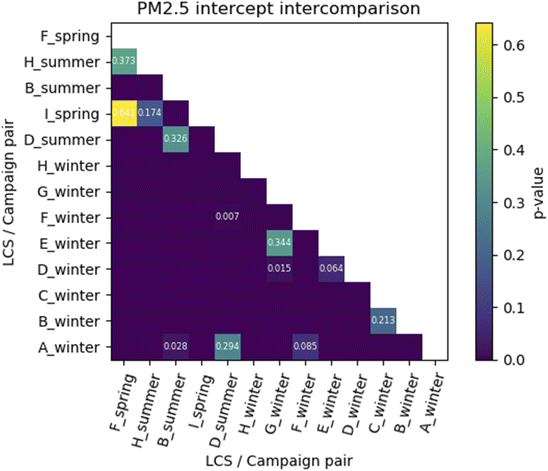 |
| Fig. 5 Results of the t-test on the intercept pair for the PM2.5 calibration. Only the p-values which indicate a null difference in means (p-value > 0.05, with a subsequent Bonferroni correction) are written on the plot. | |
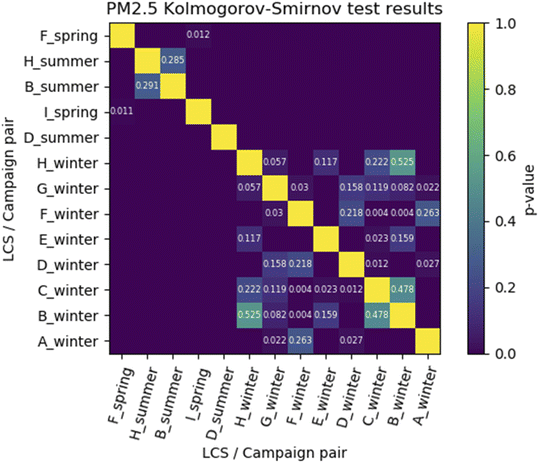 |
| Fig. 6 Results of the Kolmogorov–Smirnov test for the PM2.5 calibrations. The color scale in the plots indicates the p-values from the tests. Only the p-values which indicate an origin from the same distribution (p-value > 0.05, with a subsequent Bonferroni correction) are written on the plot. | |
The intercomparison of the calibration slopes and intercepts, by means of the pairwise Student's t-tests, shows that, regardless of the location or season, they are statistically different from one another for a large majority of pairs: 52 and 66 out of 78 for the slopes and the intercepts, respectively. The results of the Kolmogorov–Smirnov tests show that the distributions of concentration outputs by pairs of linear calibrations are different for a majority of pairs (116 out of 156). Within the same campaign, the pairs considered from the same distribution are 2 out of 6 (Summer), 2 out of 2 (Spring) and 36 out of 56 (Winter). Between different campaigns, distributions are always different. Thus, there is some transferability within the same campaign, but none between campaigns.
Calibration functions which change with time imply frequent calibrations to produce high quality outputs. This represents a possible limitation for the operator. It should not mean, however, that in the case of the impossibility of regular calibrations, the deployment of the LCS does not yield valuable information.
Indicative measurements
Fig. 7 shows the fraction of measurements qualifying as indicative for individual sensor/campaign pairs. The fraction is computed as follows: for each system/campaign pair both the LCS uncalibrated concentrations and the GRIMM concentrations are averaged over the intervals considered. Averaged uncalibrated LCS concentrations qualify as indicative if they are within ±50% of the averaged GRIMM measurement. The fraction of measurements qualifying as indicative is the number of averaged uncalibrated LCS concentration values which qualify as indicative over the total number of uncalibrated LCS concentration values.
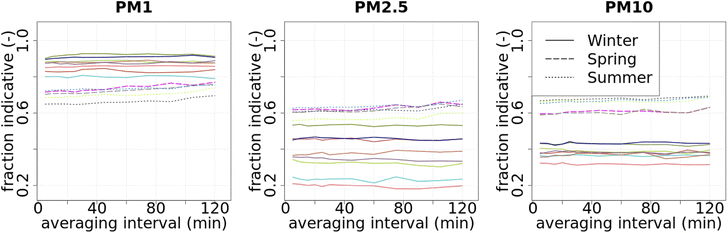 |
| Fig. 7 Fraction of indicative LCS uncalibrated measurements as a function of the averaging time (minutes). Each color represents an individual system/campaign pair. The line style indicates the campaign: winter (solid lines), spring (dashed lines) and summer (dotted lines). | |
The fraction of LCS measurements which qualify as indicative does not vary with the averaging time. This indicates that, for the purpose of deriving indicative measurements, the characteristic high time resolution of the LCS can be maintained. The lines appear clustered by the campaign, indicating seasonality, and thus likely dependence on meteorological variables.
The bidimensional look-up matrix, BLUM-i
The analysis and discussion above shows that (1) correction functions to attain reference-grade performance are sensor specific; (2) correction functions to attain reference-grade performance are time- and/or settings-specific; (3) the performance in terms of indicative measurements is independent from the time resolution; and (4) the performance in terms of indicative measurements appears to be meteorology dependent. The implication is that there is a strong requirement for frequent calibrations, via co-locations, if reference-grade measurements are to be obtained. Fig. 8 presents the visualization of the BLUM-i. Similar plots for PM1 and PM10 are in the ESI. The machine-readable version is freely available.1
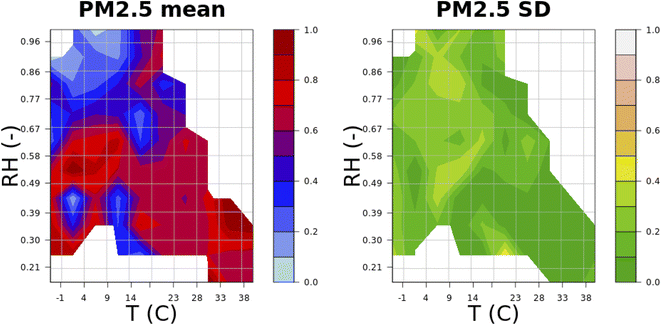 |
| Fig. 8 Visualization of the BLUM-i. Fraction of the PurpleAir PM2.5 measurements meeting the criteria to be considered indicative (5 minute time resolution) as a function of temperature and relative humidity. Left panel: average and right panel: standard deviation. | |
Example and instructions for use
For a single PM concentration from a PurpleAir unit, the PurpleAir BLUM-i can be used by applying the following algorithm: (1) from the temperature measurement associated with the PM concentration, determine the respective temperature bin in the PurpleAir BLUM-i; (2) from the relative humidity measurement associated with the PM concentration, determine the respective relative humidity bin in the PurpleAir BLUM-i; (3) the value in the PurpleAir BLUM-i cell relative to the two bins is the likelihood (taken here as the fraction) of the PM concentration given by the PurpleAir to meet the criteria of an indicative measurement.
Associated with the PurpleAir BLUM-i is a function written in the R scripting language reproducing the algorithm.1 The function takes the PurpleAir BLUM-i (provided) and a PurpleAir output file (user provided) as the input and outputs a table which is the PurpleAir output file with six supplementary fields: the likelihood of the PM1, PM2.5 and PM10 measurements to be indicative and the respective uncertainty (taken here as the standard deviation).
For example, based on the data currently populating the BLUM-i, if a PurpleAir PM2.5 measurement is taken at 5 °C and 50% RH, the likelihood of the measurement meeting the criteria to be considered indicative (i.e., an uncertainty of 50% or less) is 0.75 (as per Fig. 8 left plot) with an associated uncertainty of 0.3 (Fig. 8 right plot). In contrast, a PM2.5 measurement at 5 °C and 80% RH, has a much lower likelihood of meeting the criteria for indicative – only 0.25 (0.25 uncertainty). These two cases demonstrate the potential utility of such a look-up matrix for users who are unable to perform calibrations themselves. Provided they can access T and RH data, which is often part of the LCS output, they now have additional information that indicates that the measurements that they make at e.g., higher RH will be less reliable than those at lower RH for the same temperature.
We believe that the information is valuable for actors that do not have the resources to conduct their own calibrations. For example, if a user were aiming to use such measurements to inform decision-making, they could then decide whether and how to limit the data they include (or exclude) from their analysis. Another example of use is when prospecting for sampling locations for a monitoring project: the use of the BLUM-i reduces uncertainties when compared to uncalibrated LCS concentrations.
The PurpleAir BLUM-i can easily be expanded with data from other campaigns, and a similar concept can be used for other LCSs. As we and other users add more data to the BLUM-i that includes reference and PurpleAir data, the BLUM-i will become more robust and more complete in terms of coverage of greater temperature and relative humidity ranges.
Discussion and conclusions
LCSs have the potential to complete traditional monitoring networks increasing both spatial coverage and temporal resolution. Our research is based on the deployment of PurpleAir systems (output PM1, PM2.5 and PM10 mass concentrations based on optical particle counts) at two locations within the cities of Berlin and Potsdam, Germany, and in the scope of three campaigns covering distinct meteorological conditions, in parallel alongside a research-grade instrument. The statistical analysis showed that (1) intra-system discrepancies are seldom but their screening is important prior to further use of the data, (2) intra-campaign differences between systems can be strong and (3) inter-campaign differences have such a magnitude that they prevent the transfer of calibration functions. The seasonal and the spatial specificity of LCS calibrations has been evidenced before.48,56,72,74 The importance of source specific factors, besides the relevance of ambient factors, is further supported by the low transferability or disparate performances of laboratory corrections against field conditions (lower vs. higher variability in composition).15,55,58,65
System- time- and/or setting-specificity of the calibration functions imposes frequent calibrations which may not be possible to conform to. Notwithstanding, the information provided by the deployment of such systems may still be valuable. Providing indicative measurements (not deviating from reference measurements by more than 50%, having a status similar to that of data derived from modelling) is an alternative to providing reference-grade measurements for the LCS when the time, hardware and labour-intensive process of frequent calibrations cannot be conducted.
The performance of the PurpleAir systems in terms of measurements which can be considered as indicative is independent from the averaging time and therefore the characteristic high-time resolution can be maintained, which is not always guaranteed when providing reference-grade measurements. The performance of the PurpleAir systems in terms of measurements which can be considered as indicative was characterised as a function of ambient conditions and summarized in a bidimensional matrix, the PurpleAir bidimensional look-up matrix PurpleAir BLUM-i, machine-readable format available from Caseiro,1 which can be readily used by PurpleAir operators to assess the performance, in terms of indicative measurement, of any PurpleAir particulate matter mass concentration output. The BLUM-i is a resource for PurpleAir users without the resources for calibration to evaluate if measurements qualify as indicative measurements and provide valuable data.
The BLUM-i is not yet universal. By its design principle, as it is complemented with data from further co-locations, possibly under more diverse conditions in terms of ambient and source conditions, it will be possible to understand how robust and universal it may become. The inclusion of data from other co-locations is planned to be performed by repeating the procedure carried out to populate this first version of the BLUM-i. The same principle can be easily applied to other systems and pollutants.
Conflicts of interest
The authors declare no competing interests.
Acknowledgements
The research of EvS, SS, AC and GV was supported by IASS Potsdam with financial support provided by the Federal Ministry of Education and Research of Germany (BMBF) and the Ministry for Science, Research and Culture of the State of Brandenburg (MWFK). Some of the research was carried out as part of the research program Urban Climate Under Change [UC]2, contributing to Research for Sustainable Development (FONA, https://www.fona.de), within the joint research project Three-dimensional observation of atmospheric processes in cities (3DO) funded by the German Federal Ministry of Education and Research (BMBF) under grant 01LP1602E. The authors wish to acknowledge Christoph Schneider from the Humboldt-Universität zu Berlin, Germany, for his support during the campaign.
References
-
A. Caseiro, Zenodo, DOI:10.5281/zenodo.5549675.
-
World Economic Forum, Global Risks Report 2022, 2022, ISBN: 978-2-940631-09-4 Search PubMed.
-
Health Effects Institute, State of global air, 2021, ISSN 2578-6873 Search PubMed.
- J. Lelieveld, A. Pozzer, U. Pöschl, M. Fnais, A. Haines and T. Münzel, Cardiovasc. Res., 2020, 116, 1910–1917 CrossRef CAS PubMed.
- P. J. Landrigan,
et al.
, Lancet, 2018, 391, 462–512 CrossRef.
- C. Liu,
et al.
, N. Engl. J. Med., 2019, 381, 705–715 CrossRef CAS PubMed.
-
Directive 2008/50/EC of the European Parliament and of the Council of 21 May 2008 on Ambient Air Quality and Cleaner Air for Europe, European Parliament and Council of the European Union, 2008 Search PubMed.
-
EC, “Commission Staff Working Documment Fitness Check of the Ambient Air Quality Directives, (2008/50/EC, 2004/107/EC)”, European Commission, 2019 Search PubMed.
- European Environment Agency, Assessing air quality through citizen science, 2020, DOI:10.2800/619.
-
A. C. Lewis, E. von Schneidemesser and R. E. Peltier, Low-cost sensors for the measurement of atmospheric composition: overview of topic and future applications, 2018, ISBN 978-92-63-11215-6 Search PubMed.
- M. I. Mead, O. A. M. Popoola, G. B. Stewart, P. Landshoff, M. Calleja, M. Hayes, J. J. Baldovi, M. W. McLeod, T. F. Hodgson, J. Dicks, A. Lewis, J. Cohen, R. Baron, J. R. Saffell and R. L. Jones, Atmos. Environ., 2013, 70, 186–203 CrossRef CAS.
- S. Munir, M. Mayfield, D. Coca and S. A. Jubb, Atmos. Environ.: X, 2019, 2, 100027 CAS.
- H. Z. Li, P. Gu, Q. Ye, N. Zimmerman, E. S. Robinson, R. Subramanian, J. S. Apte, A. L. Robinson and A. A. Presto, Atmos. Environ.: X, 2019, 2, 100012 CAS.
- J. Li, H. Zhang, C.-Y. Chao, C.-H. Chien, C.-Y. Wu, C. H. Luo, L.-J. Chen and P. Biswas, Atmos. Environ., 2020, 223, 117293 CrossRef CAS.
- A. Datta, A. Saha, M. Levy Zamora, C. Buehler, L. Hao, F. Xiong, D. R. Gentner and K. Koehler, Atmos. Environ., 2020, 242, 117761 CrossRef CAS PubMed.
- M. Gao, J. Cao and E. Seto, Environ. Pollut., 2015, 199, 56–65 CrossRef CAS PubMed.
- J. J. Caubel, T. E. Cados, C. V. Preble and T. W. Kirchstetter, Environ. Sci. Technol., 2019, 53, 7564–7573 CrossRef CAS PubMed.
- J. Kim, A. A. Shusterman, K. J. Lieschke, C. Newman and R. C. Cohen, Atmos. Meas. Tech., 2018, 11, 1937–1946 CrossRef CAS.
- Y. Lu, G. Giuliano and R. Habre, Environ. Res., 2021, 195, 110653 CrossRef CAS PubMed.
- A. de Nazelle, E. Seto, D. Donaire-Gonzalez, M. Mendez, J. Matamala, M. J. Nieuwenhuijsen and M. Jerrett, Environ. Pollut., 2013, 176, 92–99 CrossRef CAS PubMed.
- J. Hofman, M. E. Nikolaou, T. H. Do, X. Qin, E. Rodrigo, W. Philips, N. Deligiannis and V. Panzica La Manna, IEEE Sens. J., 2020, 1–4 Search PubMed.
- P. Santana, A. Almeida, P. Mariano, C. Correia, V. Martins and S. M. Almeida, J. Cleaner Prod., 2021, 315, 128194 CrossRef.
- J. Van den Bossche, J. Theunis, B. Elen, J. Peters, D. Botteldooren and B. De Baets, Atmos. Environ., 2016, 141, 408–421 CrossRef CAS.
- R. Kumar, V.-H. Peuch, J. H. Crawford and G. Brasseur, Nature, 2018, 561, 27–29 CrossRef CAS PubMed.
- N. Zikova, P. K. Hopke and A. R. Ferro, J. Aerosol Sci., 2017, 105, 24–34 CrossRef CAS.
- H. Rickenbacker, F. Brown and M. Bilec, Sustain. Cities Soc., 2019, 47, 101473 CrossRef.
- J. S. Horsburgh, J. Caraballo, M. Ramírez, A. K. Aufdenkampe, D. B. Arscott and S. G. Damiano, Front. Earth Sci., 2019, 7, 00067 CrossRef.
- R. Subramanian, A. S. Kagabo, V. Baharane, S. Guhirwa, C. Sindayigaya, C. Malings, N. J. Williams, E. Kalisa, H. Li, P. Adams, A. L. Robinson, H. L. DeWitt, J. Gasore and P. Jaramillo, Clean Air J., 2020, 30, 1–15 Search PubMed.
- X. Qiao, Q. Zhang, D. Wang, J. Hao and J. Jiang, Sci. Total Environ., 2021, 779, 146381 CrossRef CAS PubMed.
- M. Jerrett, D. Donaire-Gonzalez, O. Popoola, R. Jones, R. C. Cohen, E. Almanza, A. de Nazelle, I. Mead, G. Carrasco-Turigas, T. Cole-Hunter, M. Triguero-Mas, E. Seto and M. Nieuwenhuijsen, Environ. Res., 2017, 158, 286–294 CrossRef CAS PubMed.
- C. Zuidema, C. S. Schumacher, E. Austin, G. Carvlin, T. V. Larson, E. W. Spalt, M. Zusman, A. J. Gassett, E. Seto, J. D. Kaufman and L. Sheppard, Sensors, 2021, 21(12), 4214 CrossRef CAS PubMed.
- P. Kumar, L. Morawska, C. Martani, G. Biskos, M. Neophytou, S. Di Sabatino, M. Bell, L. Norford and R. Britter, Environ. Int., 2015, 75, 199–205 CrossRef PubMed.
- C. Bacon, S. deVuono-Powell, M. L. Frampton, T. LoPresti and C. Pannu, Environ. Justice, 2013, 6, 1–8 CrossRef.
- S. Mahajan, P. Kumar, J. A. Pinto, A. Riccetti, K. Schaaf, G. Camprodon, V. Smári, A. Passani and G. Forino, Sustain. Cities Soc., 2020, 52, 101800 CrossRef.
- W. J. Ripple, C. Wolf, T. M. Newsome, P. Barnard and W. R. Moomaw, BioScience, 2019, 11, 8–12 Search PubMed.
- T. Schaefer, B. Kieslinger and C. M. Fabian, Citiz. Sci. Theory Pract., 2020, 5, 1–12 CrossRef PubMed.
- C. Borrego, J. Ginja, M. Coutinho, C. Ribeiro, K. Karatzas, T. Sioumis, N. Katsifarakis, K. Konstantinidis, S. De Vito, E. Esposito, M. Salvato, P. Smith, N. André, P. Gérard, L. A. Francis, N. Castell, P. Schneider, M. Viana, M. C. Minguillón, W. Reimringer, R. P. Otjes, O. von Sicard, R. Pohle, B. Elen, D. Suriano, V. Pfister, M. Prato, S. Dipinto and M. Penza, Atmos. Environ., 2018, 193, 127–142 CrossRef CAS.
- C. Borrego, J. Ginja, M. Coutinho, C. Ribeiro, K. Karatzas, T. Sioumis, N. Katsifarakis, K. Konstantinidis, S. De Vito, E. Esposito, M. Salvato, P. Smith, N. André, P. Gérard, L. A. Francis, N. Castell, P. Schneider, M. Viana, M. C. Minguillón, W. Reimringer, R. P. Otjes, O. von Sicard, R. Pohle, B. Elen, D. Suriano, V. Pfister, M. Prato, S. Dipinto and M. Penza, Atmos. Environ., 2016, 147, 246–263 CrossRef CAS.
- L. Morawska, P. K. Thai, X. Liu, A. Asumadu-Sakyi, G. Ayoko, A. Bartonova, A. Bedini, F. Chai, B. Christensen, M. Dunbabin, J. Gao, G. S. W. Hagler, R. Jayaratne, P. Kumar, A. K. H. Lau, P. K. K. Louie, M. Mazaheri, Z. Ning, N. Motta, B. Mullins, M. Mahmudur Rahman, Z. Ristovski, M. Shafiei, D. Tjondronegoro, D. Westerdahl and R. Williams, Environ. Int., 2018, 116, 286–299 CrossRef CAS PubMed.
- World Meteorological Organization, 2018. ISBN: 978-92-63-11215-6.
-
R. E. Peltier, N. Castell, A. L. Clements, T. Dye, C. Hüglin, J. H. Kroll, S.-C. C. Lung, Z. Ning, M. Parsons, M. Penza, F. Reisen and E. von Schneidemesser, An update on low-cost sensors for the measurement of atmospheric composition, 2020, ISBN: 978-92-63-11215-6 Search PubMed.
- Y. Jiang, X. Zhu, C. Chen, Y. Ge, W. Wang, Z. Zhao, J. Cai and H. Kann, Ecotoxicol. Environ. Saf., 2021, 211, 111958 CrossRef CAS PubMed.
- S. Sousan, S. Regmi and Y. M. Park, Sensors, 2021, 21(12), 4146 CrossRef CAS PubMed.
- D. Streuber, Y. M. Park and S. Sousan, Aerosol Air Qual. Res., 2022, 22, 220119 CrossRef.
- J. Kuula, T. Mäkelä, M. Aurela, K. Teinilä, S. Varjonen, Ó. González and H. Timonen, Atmos. Meas. Tech., 2020, 13, 2413–2423 CrossRef CAS.
- R. Williams, R. Duvall, V. Kilaru, G. Hagler, L. Hassinger, K. Benedict, J. Rice, A. Kaufman, R. Judge, G. Pierce, G. Allen, M. Bergin, R. C. Cohen, P. Fransioli, M. Gerboles, R. Habre, M. Hannigan, D. Jack, P. Louie, N. A. Martin, M. Penza, A. Polidori, R. Subramanian, K. Ray, J. Schauer, E. Seto, G. Thurston, J. Turner, A. S. Wexler and Z. Ning, Atmos. Environ.: X, 2019, 2, 100031 CAS.
- M. Badura, P. Batog, A. Drzeniecka-Osiadacz and P. Modzel, SN Appl. Sci., 2019, 1, 622 CrossRef.
- B. Feenstra, V. Papapostolou, S. Hasheminassab, H. Zhang, B. D. Boghossian, D. Cocker and A. Polidori, Atmos. Environ., 2019, 216, 116946 CrossRef CAS.
- K. Chan, D. N. Schillereff, A. C. W. Baas, M. A. Chadwick, B. Main, M. Mulligan, F. T. O'Shea, R. Pearce, T. E. L. Smith, A. van Soesbergen, E. Tebbs and J. Thompson, Prog. Phys. Geogr., 2021, 45, 305–338 CrossRef.
- M. Levy Zamora, J. Rice and K. Koehler, Atmos. Environ., 2020, 235, 117615 CrossRef PubMed.
- S. Schmitz, S. Towers, G. Villena, A. Caseiro, R. Wegener, D. Klemp, I. Langer, F. Meier and E. von Schneidemesser, Atmos. Meas. Tech., 2021, 14, 7221–7241 CrossRef CAS.
- S. E. Gillooly, Y. Zhou, J. Vallarino, M. T. Chu, D. R. Michanowicz, J. I. Levy and G. Adamkiewicz, Environ. Pollut., 2019, 244, 440–450 CrossRef CAS PubMed.
- C. Malings, R. Tanzer, A. Hauryliuk, P. K. Saha, A. L. Robinson, A. A. Presto and R. Subramanian, Aerosol Sci. Technol., 2020, 54, 160–174 CrossRef CAS.
-
Z. Yong, Plantower, 2016 Search PubMed.
- K. E. Kelly, J. Whitaker, A. Petty, C. Widmer, A. Dybwad, D. Sleeth, R. Martin and A. Butterfield, Environ. Pollut., 2017, 221, 491–500 CrossRef CAS PubMed.
- T. Sayahi, A. Butterfield and K. E. Kelly, Environ. Pollut., 2019, 245, 932–940 CrossRef CAS PubMed.
- F. M. J. Bulot, S. J. Johnston, P. J. Basford, N. H. C. Easton, M. Apetroaie-Cristea, G. L. Foster, A. K. R. Morris, S. J. Cox and M. Loxham, Sci. Rep., 2019, 9, 7497 CrossRef PubMed.
- J. Tryner, C. L’Orange, J. Mehaffy, D. Miller-Lionberg, J. C. Hofstetter, A. Wilson and J. Volckens, Atmos. Environ., 2020, 220, 117067 CrossRef CAS.
- D. Scherer, F. Ament, S. Emeis, U. Fehrenbach, B. Leitl, K. Scherber, C. Schneider and U. Vogt, Meteorol. Z., 2019, 28, 121–138 CrossRef.
- J. Li, S. K. Mattewal, S. Patel and P. Biswas, Aerosol Air Qual. Res., 2020, 20, 254–270 CrossRef CAS.
- M. R. Giordano, C. Malings, S. N. Pandis, A. A. Presto, V. F. McNeill, D. M. Westervelt, M. Beekmann and R. Subramanian, J. Aerosol Sci., 2021, 158, 105933 CrossRef.
- M. A. Zaidan, N. H. Motlagh, P. L. Fung, D. Lu, H. Timonen, J. Kuula, J. V. Niemi, S. Tarkoma, T. Petäjä, M. Kulmala and T. Hussein, IEEE Sensors J., 2020, 20(22), 13638–13652 CAS.
- A. Masic, D. Bibic, B. Pikula, A. Blazevic, J. Huremovic and S. Zero, Atmos. Meas. Tech., 2020, 13, 6427–6443 CrossRef.
- E. Austin, I. Novosselov, E. Seto and M. G. Yost, PLoS One, 2015, 10, e0137789 CrossRef PubMed.
- M. Levy Zamora, F. Xiong, D. Gentner, B. Kerkez, J. Kohrman-Glaser and K. Koehler, Environ. Sci. Technol., 2019, 53, 838–849 CrossRef CAS PubMed.
- Y. Wang, J. Li, H. Jing, Q. Zhang, J. Jiang and P. Biswas, Aerosol Sci. Technol., 2015, 49, 1063–1077 CrossRef CAS.
- T. Zheng, M. Bergin, K. Johnson, S. Tripathi, S. Shirodkar, M. Landis, R. Sutaria and D. Carlson, Atmos. Meas. Tech., 2018, 11, 4823–4846 CrossRef CAS.
- R. Jayaratne, X. Liu, P. Thai, M. Dunbabin and L. Morawska, Atmos. Meas. Tech., 2018, 11, 4883–4890 CrossRef CAS.
- A. Samad, F. E. M. Mimiaga, B. Laquai and U. Vogt, Sensors, 2021, 21(3), 804 CrossRef CAS PubMed.
- S. Commodore, A. Metcalf, C. Post, K. Watts, S. Reynolds and J. Pearce, Atmosphere, 2020, 11(8), 807 CrossRef.
- D. M. Holstius, A. Pillarisetti, K. R. Smith and E. Seto, Atmos. Meas. Tech., 2014, 7, 1121–1131 CrossRef.
- X. Liu, R. Jayaratne, P. Thai, T. Kuhn, I. Zing, B. Christensen, R. Lamont, M. Dunbabin, S. Zhu, J. Gao, D. Wainwright, D. Neale, R. Kan, J. Kirkwood and L. Morawska, Environ. Res., 2020, 185, 109438 CrossRef CAS PubMed.
- C. Buehler, F. Xiong, M. Levy Zamora, K. M. Skog, J. Kohrman-Glaser, S. Colton, M. McNamara, K. Ryan, C. Redlich, M. Bartos, B. Wong, B. Kerkez, K. Koehler and D. R. Gentner, Atmos. Meas. Tech., 2021, 14, 995–1013 CrossRef CAS PubMed.
- K. K. Barkjohn, B. Gantt and A. L. Clements, Atmos. Meas. Tech., 2021, 14, 4617–4637 CrossRef PubMed.
|
This journal is © The Royal Society of Chemistry 2022 |