DOI:
10.1039/D1RA06842C
(Paper)
RSC Adv., 2021,
11, 40120-40135
GC-MS analysis of phytoconstituents from Ruellia prostrata and Senna tora and identification of potential anti-viral activity against SARS-CoV-2†
Received
13th September 2021
, Accepted 7th December 2021
First published on 17th December 2021
Abstract
SARS-CoV-2 is an etiologic agent responsible for the coronavirus disease 2019 (COVID-19) pandemic. The virus has rapidly extended globally and taken millions of lives due to the unavailability of therapeutics candidates against the virus. Till now, no specific drug candidates have been developed that can prevent or treat infections caused by the pathogen. The main protease (Mpro) of the SARS-CoV-2 plays a pivotal role in mediating viral replication and mechanistically inhibition of the protein can hinder the replication and infection process of the virus. Therefore, the study aimed to identify the natural bioactive compounds against the virus that can block the activity of the Mpro and subsequently block viral infections. Initially, a total of 96 phytochemicals from Ruellia prostrata Poir. and Senna tora (L.) Roxb. plants were identified through the gas chromatography-mass spectrometry (GC-MS) analytical method. Subsequently, the compounds were screened through molecular docking, absorption, distribution, metabolism, excretion (ADME), toxicity (T), and molecular dynamics (MD) simulation approach. The molecular docking method initially identified four molecules having a PubChem CID: 70825, CID: 25247358, CID: 54685836 and, CID: 1983 with a binding affinity ranging between −6.067 to −6.53 kcal mol−1 to the active site of the target protein. All the selected compounds exhibit good pharmacokinetics and toxicity properties. Finally, the four compounds were further evaluated based on the MD simulation methods that confirmed the binding stability of the compounds to the targeted protein. The computational approaches identified the best four compounds CID: 70825, CID: 25247358, CID: 54685836 and, CID: 1983 that can be developed as a treatment option of SARS-CoV-2 disease-related complications. Although, experimental validation is suggested for further evaluation of the work.
Introduction
Severe acute respiratory syndrome coronavirus 2 (SARS-CoV-2) has been recognized as the etiologic agent of the ailment termed coronavirus disease 2019 (COVID-19).1 The virus created a life-threatening circumstance to the worldwide public community with surge mortality and sickness.2–4 The etiologic agent of COVID-19 was first identified in patients with pneumonia during the outbreak of this virus infection in Wuhan, China on 29 December 2019, and is still enduring a radical challenge to global public health security.5,6 Patients infected with the pathogen experience common cold to more rare and serious respiratory diseases that can be led to death due to the lack of appropriate treatment options.7 To date, more than 263.1 million (December 01, 2021, 06:57 GMT) confirmed COVID-19 cases were recorded with more than 5.23 million deaths in 222 countries and territories around the world (https://www.worldometers.info/coronavirus). Nevertheless, available therapeutics have not been developed and suggesting the emergency of developing specific drug candidates against the virus.8
The SARS-CoV-2 is an enveloped single-stranded RNA beta-coronavirus that contain a genome ranging from 26 to 32 kb in length.9 The virus belongs to the Coronaviridae family that has sequence identity 82% and >90% with SARS-CoV, and Middle East respiratory syndrome coronavirus (MERS-CoV), respectively.10 Infections of this virus may cause lower respiratory tract disease, gastrointestinal, liver, and central nervous system damage in humans and animals, but SARS-CoV-2 infection is much more severe than the other two coronaviruses.11 Although the virus uses the same receptor angiotensin-converting enzyme 2 (ACE2) of SARS-CoV to enter into the host, SARS-CoV-2 can be transmitted from human to human more frequently.12,13 Initially, the structural spike (S) glycoproteins known as the main antigen component in all proteins of the SARS-CoV-2 plays an important role in the host receptor recognition and fusion mediate entry process (Fig. 1). The S protein of the virus includes two subunits known as S1 and S2.14 The S1 subunit recognizes and attaches to the host receptor ACE2, and another cellular serine protease is known as TM protease serine 2 (TMPRSS2) help in S protein priming by cleavage at the S1/S2 and the S2′ site and promotes fusion mediate entry into host the cell.15 The fusion mediates entry releases the viral polycistronic RNA in the cytoplasm and is translated into replicase polyprotein pp1a or pp1ab.16 The polyprotein is latterly cleaved by the main protease Mpro (also called 3CLpro) and papain-like protease (PLpro) that produce 16 non-structural proteins.15,17,18 Later, the proteins of the virus make a replicase–transcriptase complex (RTC) also known as a multiprotein complex, and further utilized for the synthesis of the full-length genome of the virus.19 The multiprotein complex also consists of RNA-dependent RNA polymerase (RdRp) plays an important role in the transcription of the viral genomic RNA.20 The structural and nonstructural proteins undergo the translation process and assemble into the lumen of the endoplasmic reticulum (ER). Finally, the assemble immature viruses are mature from the Golgi intermediate compartment and subsequently release into the cells.21 As, the virus Mpro plays an important role in virus translation and replication, therefore blocking the protein will be able to hinder the replication process of the virus.22
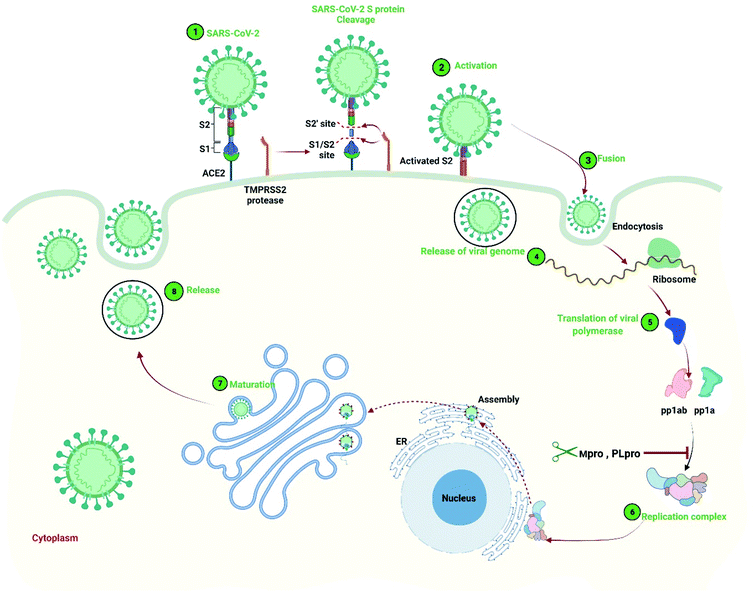 |
| Fig. 1 SARS-CoV-2 entry mechanism and replication process in the host cell. Initially, (1). The S protein of the virus recognizes the host receptor ACE2 and binds with the protein. The S protein cleavage at the S1/S2 and the S2′ site with the help of serine protease TMPRSS2, (2). Activation of the S2 subunits of the S protein, (3). Allows fusion of viral and cellular membranes resulting entry of the virus, (4). Release of the viral genome into the cytoplasm of the host cell, (5). Translation of viral polymerase and formation of replicase polyprotein pp1a and pp1ab, (6). Polyprotein cleavage by the main protease Mpro (also called 3CLpro) and papain-like protease (PLpro) and production of replicase–transcriptase complex that translated into the transcript and assemble into the lumen of the endoplasmic reticulum (ER), (7). Immature virus maturation by the help of Golgi intermediate compartment, (8). Release of the virus. | |
Medicinal plants and isolated phytochemicals encompass a diverse chemical space are widely used in the treatment of various viral diseases and drug discovery.23 Natural bioactive compounds isolated from different medicinal plants has gained the focus of modern world healthcare researchers due to optimum side effect and better synthesis accessibility.24 Over the last few decades, phytochemicals-dependent drug discovery has been accelerated because of their antiviral, proinflammatory, and antioxidant activities.25 The natural compounds isolated from different medicinal plants interact with diverse host targets and can regulate the signaling mechanisms of the host. The phytochemicals also help in reducing pro-inflammatory,26 as well as immune-boosting27 such as Tumor necrosis factor-alpha (TNF-α), Interleukin (IL-1, 6, 8, 1β), Nuclear factor-kappa B (NF-κB), and Reactive oxygen species (ROS)28 and help to minimize respiratory disorders.29 Since infection with the SARS-CoV-2, can motive critical lung damage and breathing problems, the phytochemicals or secondary metabolites of the plants can help to lessen the severity of those pulmonary (airways, lungs, and blood vessels) systems along with antiviral (especially anti-CoVs) effects. Previously different medicinal plants include Allium sativum, Camellia sinensis, Zingiber officinale, Nigella sativa, Echinacea spp., Hypericum perforatum, and Glycyrrhiza glabra, Scutellaria baicalensis, and the secondary metabolites of the plant show anti-viral properties against different coronavirus.30,31 The natural bioactive compounds originated from different medicinal plants show potential benefits for COVID-19 prevention. Therefore, plant-based potential bioactive compounds can be utilized as preventive and therapeutic option against SARS-CoV-2.
R. prostrata Poir is an annual perennial herb that grows up to 25–40 cm belongs to the Acanthaceae family and is native to the Central African Republic to Eritrea and South Africa, Arabian Peninsula, and the Indian Subcontinent include Bangladesh.32 The plant extract has been used as herbal medicine for treating humans due to its analgesic, antitussive, hypotensive and anti-inflammatory effects.32–39 Where, S. tora (L.) Roxb is another herbaceous annual fetid medicinal herb that grows to a height of 1 m in the wastelands and roadsides of tropical Asia includes Bangladesh.38 The extract of the plant was lethal for chloroquine-resistant and chloroquine-sensitive Plasmodium falciparum and frequently used for medicinal purposes, including as components of laxatives, anti-inflammatory agents, cardioprotective, antibacterial, antifungal, anti-arthritic, anticancer, and hepatoprotection.40–45 The previous study found that the extract of this medicinal plant is assumed to have the capacity to modulate the immune response, but the activity of the plant against SARS-CoV-2 remains unknown. Therefore, the study examined the antiviral properties of the two plants against SARS-CoV-2.
Computer-aided drug design (CADD) has become a popular method for over three decades that are being utilized in the screening, development, and design of therapeutically important molecular candidates.46 These methods are a combination of different approaches includes molecular docking, ADME (“absorption, distribution, metabolism, and excretion”), toxicity, and molecular dynamics (MD) simulation that help to optimize and screen compounds more effectively. Nowadays, molecular docking is another popular method that helps to determine the interaction between a target protein and small molecules candidates.47 The methods can initially identify the potential molecules that can be further utilized for drug design, where MD simulation can confirm the stability of the desired molecules to the target protein. The ADME indicates pharmacokinetics and pharmacology properties that are vital steps in the drug design process and allow us to understand the safety and efficacy of a small molecular candidate as a drug. Toxicity analysis is another step of the drug design process that can also analysis through computational methods and able to determine an adverse biological effect chemical substance or a small molecular candidate. Previously many small molecular candidates have been identified through the CADD approaches that have passed clinical trials and become novel therapeutics in the treatment of different viral diseases.48,49 Therefore, the study aimed to identify bioactive natural compounds from R. prostrata aerial parts and S. tora leaves by Gas chromatography-mass spectrometry (GC-MS) and computational approaches that can be utilized as a treatment option for COVID-19.
Materials and methods
Collection and preparation of plant
The aerial parts of R. prostrata Poir. were collected in March 2019 from Churamonkathi, Jashore, Bangladesh. The leaves of Senna tora (L.) Roxb. were collected in July 2019 from Newmarket, Noapara, and Jashore, Bangladesh. Botanical identification and authentication were done by the taxonomist Dr Sardar Nasiruddin, Chief Scientific Officer, National Herbarium, Dhaka, Bangladesh. The deposited voucher number was DACB 65141 for R. prostrata Poir and DACB 65146 for S. tora (L.) Roxb. Individual plant parts were washed under tap water and rewashed under distilled water. Initially, plant parts were air-dried in the shade at room temperature (25 °C) and then crushed into fine powder by using a mechanical grinder and passing through a 50-mesh size. The fine powder was stored in an air-tight container.
Crude extraction of plant
The plant product extraction was conducted through the method defined previously by Rahman et al., with some minor modifications.50 Briefly, five hundred grams of powdered plant material from each plant was distributed separately into a five-1000 mL conical flask. Each conical flask was filled with 400 mL of ethyl acetate and shaken at 250 rpm for 72 h at 37 °C. The mixture was filtered first by cleaned cotton gauze and then by Whatman no. 1 filter paper. To evaporate the solvent, the filtrates were concentrated under a vacuum on a rotary evaporator (Stuart, UK) at room temperature to obtain concentrated extracts of R. prostrata (25.55 g dry weight, 5.11% w/w) and S. tora (25.55 g dry weight, 6.03% w/w). Each extract was subjected to GC-MS analysis to determine the compounds.
GS-MS based phytochemicals identification
The phytochemicals of R. prostrata and S. tora were analyzed by Gas Chromatography-Mass Spectroscopy (Shimadzu triple-quad GCMS-TQ8040) with an Rtx-5MS capillary column (30 m × 0.25 mm id, film thickness of 0.25 μm).51 Initially, 1 μL of the sample was injected in splitless mode into the injection port of the GC column at a constant flow rate of 1 mL per minute. The injection temperature was kept at 250 °C and helium gas was used as a carrier during the process. The initial column oven temperature was set at 50 °C for 1 minute, then 200 °C for 2 minutes, and finally 300 °C for 7 minutes, by using a temperature control system, where the total run time was set for 40 min. The following detector parameters were used for GC-MS analyses: interface temperature of 250 °C, ion source temperature of 230 °C, mass spectrometry with Q3 scan, m/z 50–600 scanning range, scan speed mode 2000, and scan time of 0.3 s per scan. The peak area denotes the relative percentage of extracted constituents. After comparing the spectral configurations obtained with those of the available mass spectral National Institute of Standards and Technology (NIST) library was used to identify the compounds.
Retrieval and preparation of protein and ligands
The 3D crystal structure of the SARS-CoV-2 main protease (Mpro) was retrieved from the RCSB Protein Data Bank having a PDB ID: 6LU7. The atomic and molecular structure of the crystal was resolved by the X-ray diffraction method that has a resolution value of 2.16 Å.52 Initially, the protein structure was prepared by using the Protein preparation wizard available at Maestro v11.4.53 The default parameter of preparation wizard has been utilized to prepare the protein and the bond orders of the protein have been assigned, hydrogens were added, missing side chains were added, and subsequently, the water of the protein was removed. The PubChem database (https://pubchem.ncbi.nlm.nih.gov/) was used to retrieve the 3D structure of bioactive compounds and further processed by the LigPrep option of maestro v11.4. Finally, the protein and chemical structures were optimized by using the OPLS3e force field.54
Protein–ligand binding score analysis by molecular docking
Molecular docking studies can reveal the binding interactions between specific proteins and desire ligands. Natural compounds identified through GS-MS have been docked with the desired macromolecules through the Glide package of the Schrödinger Suite.55 The docking process utilized OPLS3e as a force field in standard precision mode.54 The native inhibitor (N3) in a complex with the desired protein has been analyzed and the binding position was chosen for receptor grid generation. The binding site residues-based receptor grid identified a box range X = −13.334, Y = 14.058, Z = 69.482. From this, target protein and ligand binding energy were obtained. Ligand binding residues and different kinds of chemical bonds were visualized by the Maestro viewer.
Assessment of pharmacokinetics properties
Pharmacokinetics is the mathematical observation of the ADME properties related to the intensity and time course of a drug. The computational drug design and development process relay on the early-stage assessment of pharmacokinetics properties that help to optimize a molecular candidate to become an effective drug.56 Therefore, the pharmacokinetic properties of the selected compounds were identified by using a freely accessible web tool known as Swiss-ADME (http://www.swissadme.ch/index.php) server.57 The server helps to observe and predict various pharmacokinetic and pharmacodynamic properties of the selected compounds.
Assessment of toxicity
An early assessment of the compound's toxicity is very crucial in the field of drug discovery and development.58 The toxicity of a chemical compound can be assessed in terms of toxicity endpoints like mutagenicity, carcinogenicity, and other features, and these endpoints can be measured both quantitatively and qualitatively.59 The selected compound's toxicity (mutagenicity, carcinogenicity, acute toxicity) was accessed through the ProTox-II (https://tox-new.charite.de/protox_II/) server.60
Protein–ligand stability analysis by molecular dynamics simulation
Molecular dynamics (MD) simulation was carried out to observe the structural stability of the protein–ligand complex in a specific physiological environment. A 150 ns MD simulation was carried out by using the Desmond package available at Schrödinger suit.61 Initially, the protein–ligand complexes structure was generated from molecular docking studies and the complex structure was pre-processed by using the protein preparation wizard.18 For each complex simple point-charge (SPC) water model was used to solve the system and an orthorhombic periodic boundary box shape with distance (10 × 10 × 10 Å3) has been assigned to maintain a specific volume of the systems. Na+ and Cl− ions were chosen and placed randomly in the solvated system to maintain a salt concentration of 0.15 M. The system was minimized and relaxed by using the OPLS3e force field.62 Finally, the constant pressure-constant temperature (NPT) ensemble was performed at 300.0 K temperature and 1.01325 bar pressure.63 The system for each complex was relaxed initially followed by 150 picoseconds recording intervals with an energy of 1.2 was used to final production run.64 Furthermore, RMSD, RMSF, rGyr, and SASA values were calculated to evaluate the stability and dynamic properties of these complexes.65 The entire molecular docking and molecular dynamics simulations were executed in the Linux (Ubuntu-20.04.1 LTS) environment with an Intel Core i7-10700K processor CPU, 3200 MHz DDR4 RAM, and RTX 3080 DDR6 8704 CUDA core GPU.
Results
GS-MS based phytochemicals identification
The GC-MS analysis of R. prostrata and S. tora plant identified 43 and 53 peaks, respectively. Observation of retention time (RT), peak area (percent), and molecular formula identified the presence of unique compounds for each peak.
During the 50 min retention time, the ethyl acetate extracts of R. prostrata isolated a total of 43 compounds shown in Fig. 2A and listed in Table 1, where S. tora determined 53 compounds shown in Fig. 2B and listed in Tables 1 and S1.† Most of the compounds were eluted between 4.0–39.0 min analysis time and a total of 96 compounds were identified in this study.
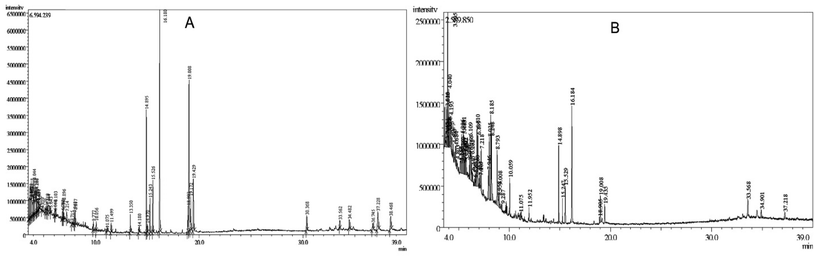 |
| Fig. 2 GC-MS chromatogram of ethyl acetate extract of (A) R. prostrata aerial parts and (B) Senna tora (L.) Roxb. leaves. | |
Table 1 List of compounds identified from ethyl acetate extract of R. prostrata aerial parts and Senna tora (L.) Roxb. leaves by GC-MS analysis
Plant name |
Peak |
R. time |
Area% |
Compound name |
CID |
R. Prostrata Poir |
1 |
3.52 |
2.38 |
Z,Z,Z-1,4,6,9-Nonadecatetraene |
5362676 |
2 |
3.595 |
2.53 |
6-Heptynoic acid, methyl ester |
557075 |
3 |
3.76 |
2.46 |
Cyclopentanol,1-(1-methylene-2-propenyl)- |
549059 |
4 |
3.82 |
3.11 |
1,5,9,9-Tetramethyl-2-oxatricyclo[6.4.0.0(4,8)]dodecane |
586811 |
5 |
3.905 |
2.22 |
1-Oxaspiro[2.2]pentane,5-isopropylidene-2,2,4,4-tetramethyl- |
549662 |
6 |
4.005 |
1.25 |
1,3,3-Trimethoxybutane |
81084 |
7 |
4.045 |
2.4 |
3-Methylbenzyl alcohol, TBDMS derivative |
22967275 |
8 |
4.12 |
0.75 |
Cycloheptanone, 4-methoxy- |
551377 |
9 |
4.28 |
2.15 |
o-Xylene |
7237 |
10 |
4.36 |
0.88 |
2-Methylpiperidine-1-thiocarboxylic acid 2-[1-[2-pyridyl 1-oxide] |
249948356 |
11 |
4.425 |
1.16 |
Glutaric acid, monochloride, 2-ethylbutyl ester |
91714556 |
12 |
4.495 |
0.84 |
Styrene |
7501 |
13 |
4.81 |
0.26 |
Pentanoic acid, 4-methyl-, methyl ester |
17008 |
14 |
5.12 |
0.48 |
Ethyl(1-adamantylamino)carbothioylcarbamate |
2728763 |
15 |
5.645 |
0.46 |
Decane |
15600 |
16 |
6.06 |
0.26 |
4-Methyl-2-hexanol |
123156 |
17 |
6.79 |
0.57 |
Benzaldehyde, 4-methyl- |
7725 |
18 |
6.86 |
0.74 |
Cyclopentanone, 2-methyl-3-(1-methylethyl)- |
41124 |
19 |
6.895 |
1 |
3-(Hydroxy-phenyl-methyl)-2,3-dimethyl-octan-4-one |
559104 |
20 |
7.715 |
0.33 |
5-Methyl-2-benzoylimino-1,3-thiazolidine |
569357 |
21 |
7.95 |
0.73 |
4,5-Dimethoxy-2-[(4-methylphenyl)carbonyl]benzonitrile |
25247358 |
22 |
8.015 |
1.03 |
Dodecane |
8182 |
23 |
9.775 |
0.5 |
(E)-1-(2,3,6-Trimethylphenyl)buta-1,3-diene (TPB, 1) |
20585933 |
24 |
10.055 |
0.37 |
Tetradecane |
12389 |
25 |
11.075 |
0.28 |
Phenol, 3,5-bis(1,1-dimethylethyl)- |
70825 |
26 |
11.5 |
0.64 |
2(4H)-Benzofuranone, 5,6,7,7a-tetrahydro-4,4,7a-trimethyl-, (R)- |
6432173 |
27 |
13.35 |
1.14 |
Methyl tetradecanoate |
31284 |
28 |
14.18 |
0.36 |
6-Hydroxy-4,4,7a-trimethyl-5,6,7,7a-tetrahydrobenzofuran-2(4H)-one |
14334 |
29 |
14.895 |
7.34 |
Neophytadiene |
10446 |
30 |
14.97 |
0.59 |
2-Pentadecanone, 6,10,14-trimethyl- |
10408 |
31 |
15.245 |
2.34 |
3,7,11,15-Tetramethyl-2-hexadecen-1-ol |
5366244 |
32 |
15.525 |
3.44 |
3,7,11,15-Tetramethyl-2-hexadecen-1-ol |
5366244 |
33 |
16.18 |
15.37 |
Hexadecanoic acid, methyl ester |
8181 |
34 |
18.9 |
2.11 |
9,12-Octadecadienoic acid, methyl ester |
5284421 |
35 |
19.01 |
12.93 |
7-Hexadecenoic acid, methyl ester, (Z)- |
5364431 |
36 |
19.17 |
3.11 |
3,7,11,15-Tetramethyl-2-hexadecen-1-ol |
5366244 |
37 |
19.43 |
4.47 |
Methyl stearate |
8201 |
38 |
30.37 |
1.31 |
trans-Geranylgeraniol |
5281365 |
39 |
33.56 |
0.6 |
Stigmastan-6,22-dien, 3,5-dedihydro- |
5364573 |
40 |
34.48 |
1 |
Cholest-5-en-3-ol (3.beta.)-, carbonochloridate |
111262 |
41 |
36.745 |
0.95 |
Cholest-5-en-3-ol (3.beta.)-, carbonochloridate |
111262 |
42 |
37.23 |
2.78 |
Stigmasta-5, 22-dien-3-ol, acetate, (3.beta.)- |
6432445 |
43 |
38.47 |
2.01 |
Cholest-5-en-3-ol (3.beta.)-, carbonochloridate |
111262 |
Senna tora (L.) Roxb |
1 |
3.62 |
2.17 |
2-Piperidinecarboxylic acid |
849 |
2 |
3.74 |
0.7 |
1,1-Cyclohexanedimethanol |
250594 |
3 |
3.815 |
2.11 |
Phenol, 4-(methoxymethyl)- |
79310 |
4 |
3.905 |
4.82 |
3-Furaldehyde |
10351 |
5 |
3.95 |
0.67 |
4-Cyclopentene-1,3-dione |
70258 |
6 |
4 |
0.51 |
2-Ethoxyethyl 3-methylbutanoate |
91698641 |
7 |
4.04 |
1.97 |
3-Methylbenzyl alcohol, TBDMS derivative |
22967275 |
8 |
4.075 |
0.88 |
2-Decenal, (E)- |
5283345 |
9 |
4.275 |
0.68 |
o-Xylene |
7237 |
10 |
4.375 |
0.66 |
Cyclopent-4-ene-1,3-dione |
70258 |
11 |
4.49 |
0.72 |
1,3,5,7-Cyclooctatetraene |
637866 |
12 |
4.685 |
0.75 |
2(5H)-Furanone |
10341 |
13 |
4.805 |
0.57 |
Bicyclo[3.1.1]heptan-3-ol, 2,6,6-trimethyl-, (1.alpha., 2.beta., 3.alpha., 5.alpha.)- |
99038 |
14 |
5.15 |
1.33 |
Trimethylsilyl 3-methyl-4-[(trimethylsilyl)oxy]benzoate |
91740684 |
15 |
5.215 |
1.15 |
4,2,7-Ethanylylidenecyclopenta[b]pyran, octahydro-7a-methyl- |
565150 |
16 |
5.305 |
2.76 |
2-Furancarboxaldehyde,5-methyl- |
12097 |
17 |
5.36 |
1.25 |
Hydroperoxide, 1-ethylbutyl |
141085 |
18 |
5.44 |
2.44 |
Carbamic acid, phenyl ester |
69322 |
19 |
5.59 |
0.97 |
Carbonic acid, ethyl 2-propenyl ester |
137020 |
20 |
5.62 |
1.03 |
Fumaric acid, decyl 2-methylpentyl ester |
91737497 |
21 |
5.65 |
0.99 |
1-Propanol, 3-methoxy-2-(methoxymethyl)-2-methyl- |
542357 |
22 |
5.725 |
1.39 |
2-Bromononane |
98219 |
23 |
5.755 |
0.58 |
l-Ascorbic acid, 5,6-O-ethylboranediyl- |
54685836 |
24 |
6.075 |
0.8 |
Octane, 4-chloro- |
33574 |
25 |
6.11 |
1.94 |
Methyl 6,6,8,8,10,10-hexamethyl-3-oxo-2,5,7,9,11-pentaoxa-6,8,10-trisilatridecan-13-oate |
91738767 |
26 |
6.165 |
2.78 |
Acetaminophen |
1983 |
27 |
6.28 |
1.47 |
2-Methyl-3-(methylthio)furan |
526618 |
28 |
6.34 |
0.5 |
Ent-3a-acetoxy-2b-hydroxy-13-iodomethyl-16-oxo-8,13-epi-17,20-dinorgibberell-1(10)-en-7,19-dioic acid,19,2-lactone,7-methyl est |
51136328 |
29 |
6.57 |
0.53 |
7-Hexadecenal, (Z)- |
5364438 |
30 |
6.635 |
0.89 |
1,5-Diazabicyclo[4.3.0]non-5-ene |
76349 |
31 |
6.76 |
1.13 |
Bicyclo[2.2.1]heptane-2-carboxylic acid isobutyl-amide |
565668 |
32 |
6.81 |
3.6 |
1-Butanol, 3-methyl-, acetate |
31276 |
33 |
6.9 |
3.35 |
3-(Hydroxy-phenyl-methyl)-2,3-dimethyl-octan-4-one |
559104 |
34 |
7.075 |
0.49 |
Levoglucosenone |
699486 |
35 |
7.105 |
0.63 |
Phenylethyl alcohol |
6054 |
36 |
8.025 |
3.65 |
2-(2-(2-Butoxyethoxy)ethoxy)ethyl 2-methylbutanoate |
91693497 |
37 |
8.185 |
3.9 |
Benzofuran, 2,3-dihydro- |
10329 |
38 |
8.25 |
4.03 |
5-Hydroxymethylfurfural |
237332 |
39 |
8.795 |
3.58 |
Resorcinol |
5054 |
40 |
9.285 |
0.52 |
2-Propyl-1-heptanol |
24847 |
41 |
10.06 |
1.44 |
Tetradecane |
12389 |
42 |
11.075 |
0.48 |
Phenol, 3,5-bis(1,1-dimethylethyl)- |
70825 |
43 |
11.95 |
0.52 |
Pentadecane |
12391 |
44 |
14.9 |
4.17 |
Neophytadiene |
10446 |
45 |
15.245 |
1.46 |
3,7,11,15-Tetramethyl-2-hexadecen-1-ol |
5366244 |
46 |
15.53 |
2.05 |
3,7,11,15-Tetramethyl-2-hexadecen-1-ol |
5366244 |
47 |
16.185 |
7.28 |
Hexadecanoic acid, methyl ester |
8181 |
48 |
18.905 |
0.48 |
Cyclopropaneoctanoic acid, 2-[[2-[(2-ethylcyclopropyl)methyl]cyclopropyl]methyl]-, methyl ester |
534619 |
49 |
19.01 |
1.88 |
8,11,14-Docosatrienoic acid, methyl ester |
5364473 |
50 |
19.435 |
1.34 |
Methyl stearate |
8201 |
51 |
33.57 |
1.34 |
Stigmasta-5,22-dien-3-ol, acetate, (3.beta.)- |
6432445 |
52 |
34.9 |
0.65 |
DL-Alpha.-Tocopherol |
2116 |
53 |
37.22 |
0.58 |
3,4,3′,4′-Tetrahydrospirilloxanthin |
5366 411 |
Protein–ligand binding score analysis by molecular docking study
A molecular docking study was performed to understand molecular interactions and binding affinity between the target protein and phytochemicals. The Mpro of SARS-CoV-2 (PDB ID: 6LU7) and the phytochemicals were docked to identify the binding score between protein and the compounds and the binding score has listed in Tables S2 and S3.† Based on the docking score the best two compounds (CID: 25247358, CID: 70825) from R. prostrata and two compounds (CID: 54685836, CID: 1983) from S. tora have been selected, where the cut-off energy value was −6.00 kcal mol−1 (Fig. 3). Interestingly, compound CID: 70825 was found for both plants, which has a binding score >−6.0 kcal mol−1. As the compounds were the same for both plants that showed the highest score and instead of the compound CID: 70 825 the compound CID: 1983 has been taken for further evaluation. The final four compounds (CID: 25247358, CID: 70825, CID: 54685836, CID: 1983) were considered for further evaluation in this study.
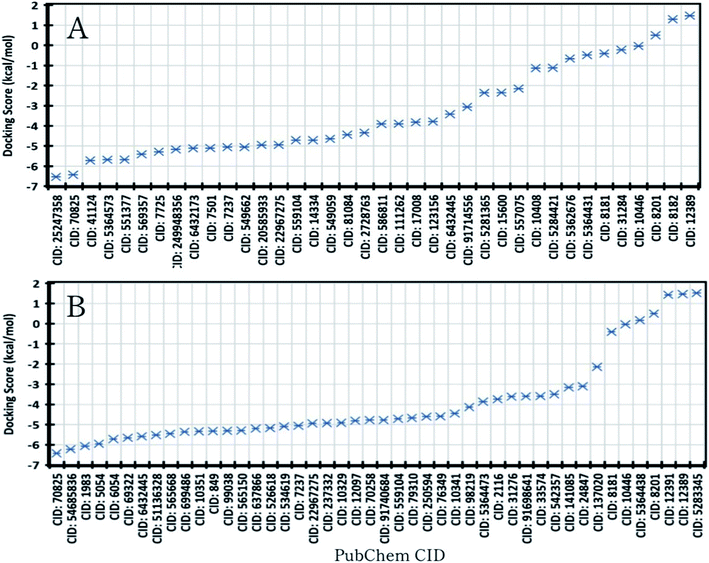 |
| Fig. 3 Molecular docking score of SARS-CoV-2 Mpro protein and natural compounds of (A). R. prostrata and (B). S. tora was identified through the GS-MS analysis approach. | |
Protein–ligand interaction analysis
The best docking score containing selected four compounds has been retrieved for further analysis and molecular interactions have been visualized through the Maestro module of the schrödinger suite shown in Fig. 4. Different types of non-bonded interactions between receptors and ligands, like hydrogen bonds, electrostatic bonds, and hydrophobic bonds, have been identified. The compounds of R. prostrata namely 4,5-dimethoxy-2-[(4-methylphenyl) carbonyl]benzonitrile (CID: 25247358), 4,5-dimethoxy-2-[(4-methylphenyl)carbonyl]benzonitrile (CID: 70825) showed an energy score −6.53 kcal mol−1 and −6.427 kcal mol−1, respectively (Table 2). Another plant S. tora compounds namely L-ascorbic acid, 5,6-O-ethylboranediyl-(CID: 54685836), and acetaminophen (CID: 1983) showed −6.222 kcal mol−1 and, −6.067 kcal mol−1 binding energies respectively. All 4 compounds showed interaction with a different amino acid that is illustrated and represented in Fig. 4 and listed in Table 2. The cocrystal N3 inhibitor has a polar bond with THR24, THR25, THR26, HID41, ASN142, SER144, HID163, HID164, HID172, and Hydrophobic Bond with VAL3, LEU4, LEU27, MET49, PHE140, LEU141, GLY143, CYS145, MET165 amino acid residues of the 6LU7 protein listed in Table 2 and shown in Fig. S1.† The THR25, HIS41, HIS163, HIS164, HIE172, PHE140, LEU141, GLY143, CYS145, MET165 residues are common interacting residues among 4 ligands and N3 inhibitors.
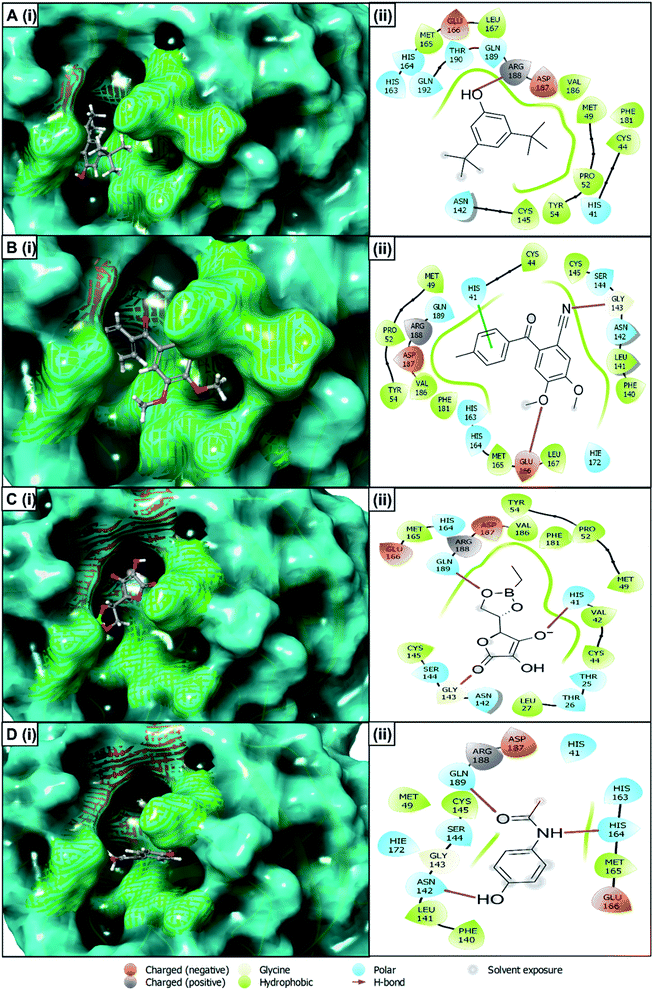 |
| Fig. 4 Interaction between the SARS-CoV-2 Mpro and selected four compounds representing in 3D (left) and 2D (right) format. Representing the compounds, A (i, ii)) CID: 70825, B (i, ii) CID: 25247358, C (i, ii) CID: 54685836 and, D (i, ii) CID: 1983 in the active pocket of the protein. | |
Table 2 Molecular docking score and amino acid-binding residues among SARS-CoV-2 Mpro and selected four compounds along with the native N3 inhibitor of the protein
Plant name |
CID |
Docking score (kcal mol−1) |
H-bond |
Polar bond |
Hydrophobic bond |
R. prostrata |
25247358 |
−6.53 |
GLY143, GLU 166 |
HIS41, SER144, ASN142, HIE172, HIS163, HIS164, GLN189 |
CYS44, MET49, PRO52, TYR54, PHE140, LEU141, CYS145, MET165, LEU167, PHE181, VAL186 |
70825 |
−6.427 |
ARG188 |
HIS41, ASN142, HIS163, HIS164, GLN189, THR190, GLN192 |
CYS44, MET49, PRO52, CYS54, CYS145, MET165, LEU167, PHE181, VAL186 |
S. tora |
54685836 |
−6.222 |
HIS41, GLY143, GLN189 |
THR25, THR26, HIS41, ASN142, SER144, HIS164, GLN189 |
MET165, VAL186, PHE181, TYR54, PRO52, MET49, VAL42, CYS44, LEU27, CYS145 |
1983 |
−6.067 |
ASN142, HIS164, GLN189 |
HIS41, ASN142, SER144, HIS163, HIS164, HIE172, GLN189 |
MET49, PHE140, LEU141, GLY143, CYS145, MET165 |
N3 inhibitor |
The native ligand of 6LU7 |
|
|
THR24, THR25, THR26, HIS41, ASN142, SER144, HIS163, HIS164, HIE172 |
VAL3, LEU4, LEU27, MET49, PHE140, LEU141, GLY143, CYS145, MET165 |
Assessment of pharmacokinetics properties
Pharmacokinetics properties that comprise ADME properties are influenced by the route of administration and the functioning of body organs of a drug that depends on patient-related factors as well as on the drug's chemical properties. These properties can indicate that drugs will not fail during clinical trials for reasons that could have been determined and skipped. Therefore, the selected compounds Pharmacokinetics properties have been evaluated. The compounds (CID: 25247358, CID: 70825, CID: 54685836, CID: 1983) have been evaluated based on their physicochemical, lipophilicity, water-solubility, gastrointestinal absorption, drug-likeness (Lipinski's rule of five), and synthesis accessibility and listed in Table 3. All the compounds selected in the study show good pharmacokinetics properties indicate that drugs have low chances to fail during clinical trials.
Table 3 Pharmacokinetics properties include physicochemical properties, lipophilicity, water-solubility, gastrointestinal absorption, drug-likeness, and synthesis accessibility of selected 4 compounds
Properties |
CID: 25247358 |
CID: 70825 |
CID: 54685836 |
CID: 1983 |
Physico-chemical properties |
MW (g mol−1) |
281.31 |
206.32 |
213.98 |
151.16 |
Heavy atoms |
21 |
15 |
15 |
11 |
Arom. heavy atoms |
12 |
6 |
0 |
6 |
Rotatable bonds |
4 |
2 |
2 |
2 |
H-Bond acceptors |
4 |
1 |
6 |
2 |
H-Bond donors |
0 |
1 |
2 |
2 |
Lipophilicity |
log Po/w |
2.98 |
3.89 |
−0.55 |
0.93 |
Water solubility |
log S (ESOL) |
Soluble |
Soluble |
High |
High |
Pharmacokinetics |
GI absorption |
High |
High |
High |
High |
Drug likeness |
Lipinski |
Yes |
Yes |
Yes |
Yes |
Medi. chemistry |
Synth. accessibility |
2.51 |
1.37 |
3.78 |
1.0 |
Assessment of toxicity
Toxicity can be defined as the degree to which a chemical compound can harm a living organism. The toxicity can damage the formation of essential enzymes in an organism through a single or short-term exposure and can lead to the failure of a major organ. Sometimes the compounds designed as drug candidates can be toxin and harmful for another organ that can cause organ toxicity, toxicity immunotoxicity, mutagenicity, and cytotoxicity for human or animal body. Therefore, the toxicity of the selected four compounds has also been accessed in this study. All the selected four compounds CID: 25247358, CID: 70825, CID: 54685836, CID: 1983 good toxicity properties, which were in the toxicity class of V, IV, V, IV, respectively, and listed in Table 4.
Table 4 Toxicity properties like organ toxicity, toxicity endpoints include immunotoxicity, mutagenicity, cytotoxicity, oral rat LD50 value of selected 4 compounds
Endpoint |
Target |
CID: 25247358 |
CID: 70825 |
CID: 54685836 |
CID: 1983 |
Organ toxicity |
Hepatotoxicity |
Inactive |
Inactive |
Inactive |
Inactive |
Toxicity endpoints |
Carcinogenicity |
Inactive |
Inactive |
Inactive |
Inactive |
Immunotoxicity |
Low |
Low |
Low |
Low |
Mutagenicity |
Inactive |
Inactive |
Inactive |
Inactive |
Cytotoxicity |
Inactive |
Inactive |
Inactive |
Inactive |
LD50 (mg kg−1) |
2100 |
800 |
5000 |
338 |
Toxicity class |
5 |
4 |
5 |
4 |
Protein–ligand complex stability and conformational flexibility by MD simulation
MD simulation can be applied in computer-aided drug discovery to understand the protein–ligand complex's stability and intermolecular interaction in reference time. It can also determine the conformational change of the complex system in an artificial environment. Therefore, to understand conformational changes of the protein in complex with selected ligand a 150 ns MD simulation was performed in this study. Initially, the last snapshots were extracted from the respective 150 ns MD trajectories and analyzed for intermolecular behavior observation. The MD simulation result was analyzed based on root-mean-square deviation (RMSD), root means square fluctuation (RMSF), the radius of gyration (Rg), and Solvent Accessible Surface Area (SASA). Protein–ligand interaction mapping, ligand-protein contacts, and intramolecular hydrogen bond of protein–ligand during simulation time.
RMSD analysis
The root means square deviation (RMSD) value is used to calculate the mean variation in dislocation of selected atoms for a particular time compared to a reference time. The average change in RMSD of the protein–ligand complex with a range between 1–3 Å is completely acceptable. If the RMSD value is larger than 1–3 Å it means, there is a vast conformational change in the protein structure. Therefore, to evaluate the conformation change of the desired protein in the complex of the selected compound includes CID: 70825, CID: 25247358, CID: 54685836, and CID: 1983 a 150 ns MD simulation was performed and the correspondence RMSD value was observed. In the case of the compound CID: 70825 the average RMSD value was in a range between 2.1 Å to 2.3 Å. The fluctuation was a bit high at a range between 85 to 90 ns simulation time and the least of the time the compound shows good stability compared to the Mpro apoprotein shown in Fig. 5. The average RMSD value change from the Mpro Apo to another three compounds CID: 25247358, CID: 54685836, and CID: 1983 maintenance an average range of 1–2.5 Å. The value change for the compound shows very optimum fluctuation, which was lower than the desired range indicated the conformational stability of the protein–ligands complex structure shown in Fig. 5.
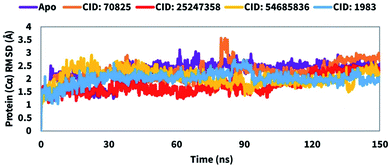 |
| Fig. 5 Showing the RMSD values of the SARS-CoV-2 Mpro in complex with the selected four compounds extracted from Cα atoms of the complex system. The RMSD of the native Mpro (PDB: 6LU7) is shown in purple color, where the selected four compounds CID: 70825, CID: 25247358, CID: 54685836, and CID: 1983 in complex with the desired protein were represented by orange, red, yellow, and blue color, respectively. | |
RMSF analysis
The Root Mean Square Fluctuation (RMSF) can help to characterize and determine the local changes within the protein chain during the interaction of compounds to the specific residues. Therefore, the RMSF value of the compounds CID: 25247358, CID: 70825, CID: 54685836, CID: 1983 in complex with the Mpro were calculated to observe the change in protein structural flexibility during the attachment of selected compounds to a specific residual position and shown in Fig. 6. In Fig. 6, all the selected compounds show a peak area of the protein at GLY711, ASP153, GLY193, and ASN277 residual positions that fluctuate the most during the simulation. The RMSF found minimal between 5 to 295 AA residue of the most rigid secondary structure elements includes alpha-helices and beta-strands. Compare to native structure elements (apo) to the complex structure the fluctuation of the residues is very low indicating the rigidity of the protein structure. Most of the fluctuation observe at the initial and last portion of the protein due to the presence of the N- and C-terminal domain. Therefore, it can be said that the displacement of a particular atom has a low fluctuation probability in a real-life environment for all the selected four compounds.
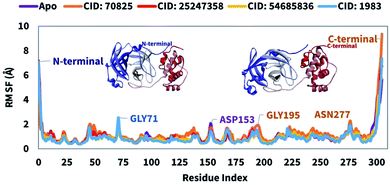 |
| Fig. 6 RMSF values were extracted from protein Cα atoms of the protein–ligand docked complex. The RMSF of the native Mpro is shown in purple color, where the selected four compounds CID: 70825, CID: 25247358, CID: 54685836, and CID: 1983 in complex with the desired protein were represented by orange, red, yellow, and blue color, respectively. Additionally, the N- and C-terminal domains of the SARS-CoV-2 Mpro has represented in 3D format. | |
Hydrogen bonds
Hydrogen bonds play a critical role in drug binding to the binding site of the desired protein. The number of hydrogen bonds can help to characterize a drug, that has a strong influence on drug specificity, metabolization, and adsorption. Therefore, the number of hydrogen bonds of the selected compounds CID: 25247358, CID: 70825, CID: 54685836, CID: 1983 in complex with the Mpro was computed for systems by investigating configurations every 150 ps represented in Fig. 7. Hydrogen bond numbers were calculated from initial to final times during the 150 ns simulation run to observe each hydrogen bond. All the compounds formed several hydrogen bonds ranging between 250 to 290 occur simultaneously until 150 ns simulation time. Therefore, all the compounds will increase the strength and stability of the ligands–receptor interaction substantially.
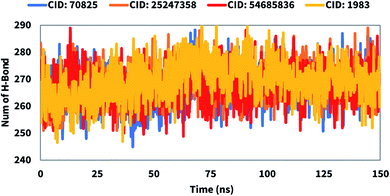 |
| Fig. 7 Representing the number of hydrogen bonds formed of the selected four compounds in a complex with the desire SARS-CoV-2 Mpro during the 150 ns molecular dynamics simulation. The Y-axis ordinate is the number of hydrogen bonds in the protein–ligands complex, and the X-axis ordinate is time (ns). The colors blue, orange, red, and yellow indicate CID: 70825, CID: 25247358, CID: 54685836, and CID: 1983, respectively. | |
Radius of gyration (Rg)
The radius of gyration (Rg) can be defined as the distribution of atoms of a protein–ligand complex system around its axis. The computation of Rg is one of the most important markers for forecasting a macromolecule's structural activity and indicates the changes in complex compactness. Therefore, the stability of CID: 70825, CID: 25247358, CID: 54685836, CID: 1983 in complex with the desired protein were also analyzed in terms of Rg over 150 ns simulation time shown in Fig. 8. The average Rg was found to be 2.9, 3.6, 3.01, and 2.6 for the compounds CID: 70825, CID: 25247358, CID: 54685836, CID: 1983, respectively indicating the active site of the protein does not induce major conformational changes after binding the selected compounds.
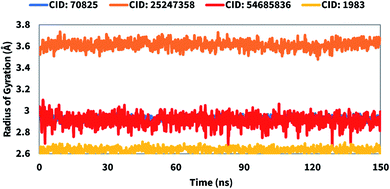 |
| Fig. 8 The radius of gyration (Rg) of the protein–ligand complex was calculated from the 150 ns simulation. The Rg value of the selected four compounds CID: 70825, CID: 25247358, CID: 54685836, and CID: 1983 in complex with the SARS-CoV-2 Mpro represented by a blue, orange, red, and yellow color, respectively. | |
Solvent accessible surface area
The structure and function of biological macromolecules are influenced by solvent-accessible surface area (SASA). In most cases, amino acid residues on a protein's surface operate as active sites and/or interact with other molecules and ligands that help to understand the solvent-like behavior (hydrophilic or hydrophobic) of a molecule as well as protein–ligand complexes. Therefore, the SASA value of the protein in complex with the compounds CID: 70825, CID: 25247358, CID: 54685836, CID: 1983 were calculated and presented in Fig. 9. The SASA value identified for the complex system was an average between 75 to 400 Å2 indicating the high level of exposure of an amino acid residue to the selected compound in the complex systems.
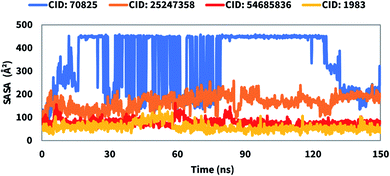 |
| Fig. 9 The by solvent accessible surface area (SASA) of the protein–ligand complex was calculated from 150 ns simulation. The SASA value of the selected four compounds CID: 70825, CID: 25247358, CID: 54685836, and CID: 1983 in complex with the SARS-CoV-2 Mpro represented by a blue, orange, red, and yellow color, respectively. | |
Protein–ligand contact analysis
The complex structure of a protein with the selected ligands and their intermolecular interactions has been evaluated for 150 ns simulation time via the simulation interactions diagram (SID). Depending on some parameters, including hydrogen bond, non-covalent bond (hydrophobic bond), ionic bond, and water bridge bond, the contact between protein and the selected compounds CID: 70825, CID: 25247358, CID: 54685836, CID: 1983 have been analyzed and represented in Fig. 10. During the 150 ns simulation time, all compounds were found to form different multiple interactions through hydrogen, hydrophobic, ionic, and water bridge bonding and maintenance until the end of the simulation and helped to make a stable binding with the desired protein.
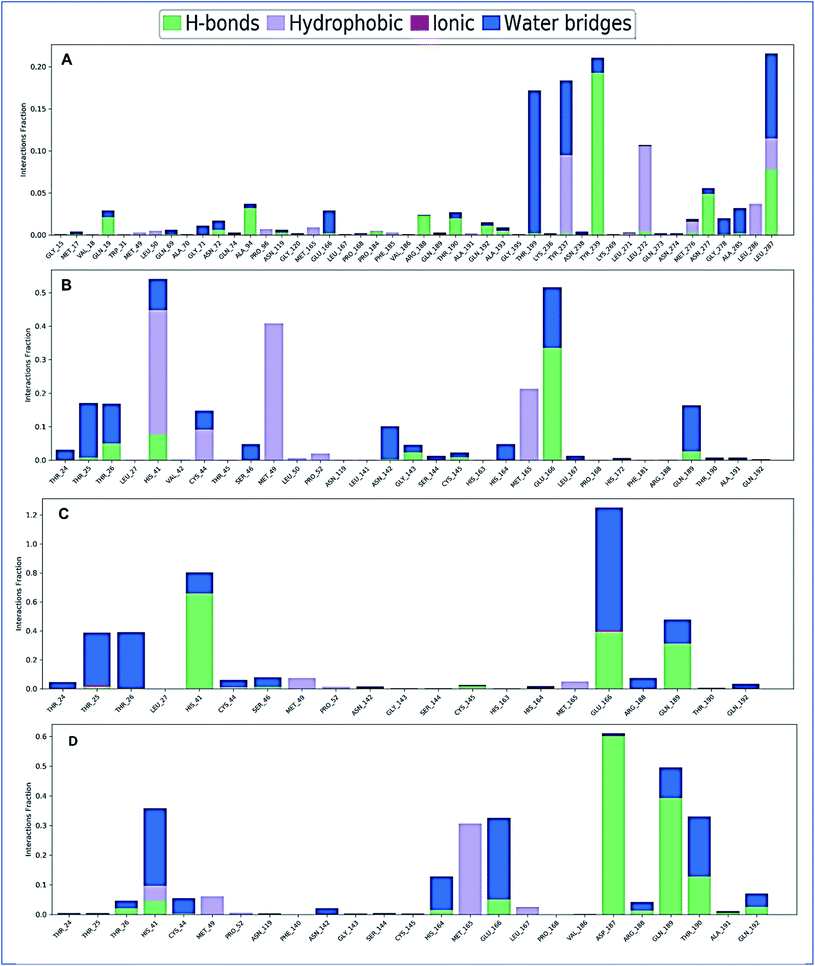 |
| Fig. 10 The stacked bar charts represent the protein–ligands interactions found during the 150 ns simulation. Herein, showing the interaction of selected four compounds A) CID: 70825, (B) CID: 25247358, (C) CID: 54685836, and (D) CID: 1983 in complex with the SARS-CoV-2 Mpro. | |
Discussion
SARS-CoV-2 appoints a devastating situation all over the world and various medication strategies are trying to be developed.66,67 For thousands of years, natural products and their derivatives isolated from various sources have been demonstrated to be effective therapeutic agents, and thus play an important role in treating diverse infectious diseases.68 The chemical structure and extensive biological activities of these compounds vary comprehensively. That's why natural compounds incessantly offer inspiration for innovations in drug discovery and medical sciences.69 Recently, researchers from different parts of the world have been working extensively to find certain potential lead compounds from medicinal plants that are active against several enzymes and other proteins responsible for the SARS-CoV-2 replication and transcription.70,71 Till now, no specific drug candidates of the virus have been determined. The plant R. prostrata previously showed anti-inflammatory and antioxidant properties,34 and the leaf extract of the S. tora plant is reported to have antiviral, antibacterial, antifungal, and anti-arthritic activity.40 But the activity of the plant as a medication option of SARS-CoV-2 remains unknown. Therefore, the study aims to identify potential natural Mpro inhibitors through computational approaches to overcome the current devastating situation that originated from SARS-CoV-2.
In this study, we have identified potential drug candidates against SARS-CoV-2 by targeting the Mpro of the virus. Initially, the compounds of R. prostrata and S. tora plant were identified through the GS-MS analysis approach. Subsequently, the identified compounds were screened through the molecular docking process that primarily selected four compounds CID: 70825, CID: 25247358, CID: 54685836, CID: 1983 having a lower binding affinity. The compounds fitted with the active pockets of the SARS-CoV-2 Mpro that interacted with HIE41 and Cys145 residues. Interestingly, the HIE41 and Cys145 residues belong to the catalytic dyads of the SARS-CoV-2 Mpro.72 The RO5 demonstrated the drug-like properties of the selected compounds.73,74
The pharmacokinetics include the ADME properties of all the selected four compounds were evaluated and the Lipinski's rules five (RO5) of molecules were justified. All the selected compounds show good pharmacokinetics properties as well as maintenance of the RO5. The compound with good ADME properties has been further evaluated through the toxicity properties to measure the harmful effect on humans or animals.75 Analysis of toxicity found no or less toxicity of the selected four compounds.
Molecular dynamics simulation is used to confirm the stability of a protein in a complex with ligands.75,76 Also, it can determine the stability and rigidity of protein–ligand complexes in a specific artificial environment like the body.76 The RMSD values of the complex systems indicate the best stability of the compounds and RMSF values measure mean fluctuation that determines the compactness of the protein–ligand complex.77 The Cα atoms of the protein–ligand complexes were used to calculate the RMSD of the system that confirm low fluctuation of the protein. The fluctuation of the protein was also determined based on the RMSF value that also confirms low fluctuation of the complex system indicated the stability of the compounds to the desired protein. The center of mass from the protein C and N terminals tests the stability of the protein structure and gives a broader understanding of protein folding characteristics for Rg calculated.78 The lower Rg value means that high compactness and the larger value display the disassociation of the compounds from the protein and all the compounds show better Rg value.79 The larger SASA value indicates the less stable structure, whereas the lower value means the tightly contracted complex of water molecules and amino acid residues.80,81 The study found optimum Rg and SASA values of the selected four compounds. The evaluation of selected four compounds based on different parameters supported diverse results, therefore the compound has been chosen for further assessment through various wet lab-based experimental methods.
Conclusions
Drug design is becoming an essential, efficient, and external approach to identifying inhibitory compounds against a specific target protein. In this study, we describe the quick and successful identification of novel natural Mpro inhibitors using a computer-aided drug design approach (CADD). The CADD include the molecular docking, ADMET, and MD simulation approaches that identified four natural compounds, CID: 70825, CID: 25247358, CID: 54685836, and CID: 1983 that can potentially inhibit the activity of Mpro and result in blocking the replication of SARS-CoV-2 into the human host cell.
Funding
This research received no external funding.
Author contributions
MMR and FA designed the project; RA contributed to generate and analyze the data. RA, FA, RRI, MMR and SA wrote the manuscript; RRI and MEKT performed data analysis; RA, SA, MAH, FA, and MMR critically reviewed the manuscript. All authors read and approved the final manuscript.
Conflicts of interest
The authors declare no conflict of interest.
Acknowledgements
The authors are grateful to the Laboratory of Computational Biology, Biological Solution Centre (BioSol Centre; http://www.biosolcentre.org), Jashore 7408, Bangladesh for providing technical support.
References
- C.-C. Lai, T.-P. Shih, W.-C. Ko, H.-J. Tang and P.-R. Hsueh, Severe acute respiratory syndrome coronavirus 2 (SARS-CoV-2) and coronavirus disease-2019 (COVID-19): The epidemic and the challenges, Int. J. Antimicrob. Agents, 2020, 55(3), 105924 CrossRef CAS PubMed.
- X. Li, et al., Risk factors for severity and mortality in adult COVID-19 inpatients in Wuhan, J. Allergy Clin. Immunol., 2020, 146(1), 110–118 CrossRef CAS PubMed.
- P. Bastard, L. B. Rosen, Q. Zhang, E. Michailidis, H. H. Hoffmann, Y. Zhang and J. L. Casanova, Autoantibodies against type I IFNs in patients with life-threatening COVID-19, Science, 2020, 370(6515), eabd4585 CrossRef CAS PubMed.
- Q. Zhang, P. Bastard, A. Bolze, E. Jouanguy, S. Y. Zhang, A. Cobat and J. L. Casanova, Life-Threatening COVID-19: Defective Interferons Unleash Excessive Inflammation, Med, 2020, 1(1), 14–20 CrossRef PubMed.
- Y. Shi, G. Wang, X. P. Cai, J. W. Deng, L. Zheng, H. H. Zhu, M. Zheng, B. Yang and Z. Chen, An overview of COVID-19, J. Zhejiang Univ. Sci. B, 2020, 21(5), 343–360 CrossRef CAS PubMed.
- H. Zhang, Challenges and Approaches of the Global Governance of Public Health Under COVID-19, Frontiers in Public Health, 2021, 9, 727214 CrossRef PubMed.
- M. J. Christie, A. T. Irving, S. C. Forster, B. J. Marsland, P. M. Hansbro, P. J. Hertzog and M. F. Nold, Of bats and men: Immunomodulatory treatment options for COVID-19 guided by the immunopathology of SARS-CoV-2 infection, Sci. Immunol., 2021, 6(63), eabd0205 CrossRef CAS PubMed.
- M. Goyal, N. Tewatia, H. Vashisht, R. Jain and S. Kumar, Novel corona virus (COVID-19); Global efforts and effective investigational medicines: A review, J. Infect. Public Health, 2021, 14(7), 910–921 CrossRef PubMed.
- C. Yin, Genotyping coronavirus SARS-CoV-2: methods and implications, Genomics, 2020, 112(5), 3588–3596 CrossRef CAS PubMed.
- A. Banerjee, et al., Predicting the recombination potential of severe acute respiratory syndrome coronavirus 2 and Middle East respiratory syndrome coronavirus, J. Gen. Virol., 2020, 101(12), 1251–1260 CrossRef CAS PubMed.
- J. Xu, S. Zhao, T. Teng, A. E. Abdalla, W. Zhu, L. Xie, Y. Wang and X. Guo, Systematic Comparison of Two Animal-to-Human Transmitted Human Coronaviruses: SARS-CoV-2 and SARS-CoV, Viruses, 2020, 12(2) DOI:10.3390/v12020244.
- A. Samad, T. Jafar and J. H. Rafi, Identification of angiotensin-converting enzyme 2 (ACE2) protein as the potential biomarker in SARS-CoV-2 infection-related lung cancer using computational analyses, Genomics, 2020, 112(6), 4912–4923 CrossRef CAS PubMed.
- S. Pokhrel, et al., Spike protein recognizer receptor ACE2 targeted identification of potential natural antiviral drug candidates against SARS-CoV-2," (in eng), Int. J. Biol. Macromol., 2021, 191, 1114–1125 CrossRef CAS PubMed.
- A. Samad, et al., Designing a multi-epitope vaccine against SARS-CoV-2: an immunoinformatics approach, 2020, pp. 1–17 Search PubMed.
- Q. Zhang, et al., Molecular mechanism of interaction between SARS-CoV-2 and host cells and interventional therapy, Signal Transduction and Targeted Therapy, 2021, 6(1), 233 CrossRef CAS PubMed.
- M. Romano, A. Ruggiero, F. Squeglia, G. Maga and R. Berisio, A Structural View of SARS-CoV-2 RNA Replication Machinery: RNA Synthesis, Proofreading and Final Capping, Cells, 2020, 9(5) DOI:10.3390/cells9051267.
- T. M. Karpiński, M. Kwaśniewski, M. Ozarowski and R. Alam, In silico studies of selected xanthophylls as potential candidates against SARS-CoV-2 targeting main protease (Mpro) and papain-like protease (PLpro), Herba Pol., 2021, 1–8 Search PubMed.
- B. Goyal and D. Goyal, Targeting the Dimerization of the Main Protease of Coronaviruses: A Potential Broad-Spectrum Therapeutic Strategy, ACS Comb. Sci., 2020, 22(6), 297–305 CrossRef CAS PubMed.
- R. Ulferts, I. Imbert, B. Canard, and J. Ziebuhr, Expression and Functions of SARS Coronavirus Replicative Proteins, in Molecular Biology of the SARS-Coronavirus, ed. S. K. Lal, Berlin, Heidelberg, Springer Berlin Heidelberg, 2010, pp. 75–98 Search PubMed.
- K. Naydenova, K. W. Muir, L. F. Wu, Z. Zhang, F. Coscia, M. J. Peet and C. J. Russo, Structure of the SARS-CoV-2 RNA-dependent RNA polymerase in the presence of favipiravir-RTP, Proc. Natl. Acad. Sci. U. S. A., 2021, 118(7) DOI:10.1073/pnas.2021946118.
- A. R. Fehr and S. Perlman, Coronaviruses: an overview of their replication and pathogenesis, Methods in molecular biology, Clifton, N.J., vol. 1282, pp. 1–23, 2015 Search PubMed.
- C. Wu, et al., Analysis of therapeutic targets for SARS-CoV-2 and discovery of potential drugs by computational methods, Acta Pharm. Sin. B, 2020, 10(5), 766–788 CrossRef CAS PubMed.
- M. Mukhtar, M. Arshad, M. Ahmad, R. J. Pomerantz, B. Wigdahl and Z. Parveen, Antiviral potentials of medicinal plants, Virus Res., 2008, 131(2), 111–120 CrossRef CAS PubMed.
- S. Gouda, G. Das, S. K. Sen, H.-S. Shin and J. K. Patra, Endophytes: A Treasure House of Bioactive Compounds of Medicinal Importance, Mini Review, 2016, 7(1538), 431–441 Search PubMed.
- M. J. Balunas and A. D. Kinghorn, Drug discovery from medicinal plants, Life Sci., 2005, 78(5), 431–441 CrossRef CAS PubMed.
- Y. J. Zhang, R. Y. Gan, S. Li, Y. Zhou, A. N. Li, D. P. Xu and H. B. Li, Antioxidant Phytochemicals for the Prevention and Treatment of Chronic Diseases, Molecules, 2015, 20(12), 21138–21156 CrossRef CAS PubMed.
- A. Parveen, L. Subedi, H. W. Kim, Z. Khan, Z. Zahra, M. Q. Farooqi and S. Y. Kim, Phytochemicals Targeting VEGF and VEGF-Related Multifactors as Anticancer Therapy, J. Clin. Med., 2019, 8(3), 350 CrossRef CAS PubMed.
- A. T. Lau, Y. Wang and J. F. Chiu, Reactive oxygen species: current knowledge and applications in cancer research and therapeutic, J. Cell. Biochem., 2008, 104(2), 657–667 CrossRef CAS PubMed.
- P. Smruti, A review on natural remedies used for the treatment of respiratory disorders, Int. J. Pharm., 2021, 8, 104–111 CAS.
- M. Boozari and H. Hosseinzadeh, Natural products for COVID-19 prevention and treatment regarding to previous coronavirus infections and novel studies, Phytother. Res., 2021, 35(2), 864–876 CrossRef CAS PubMed.
- M. T. Sultan, M. S. Buttxs, M. M. N. Qayyum and H. A. R. Suleria, Immunity: plants as effective mediators, Crit. Rev. Food Sci. Nutr., 2014, 54(10), 1298–1308 CrossRef CAS PubMed.
- N. M. Atre and D. D. Khedkar, A Review on Herbal Remedies for Sexually Transmitted Infections (STIs) from Melghat Region of Maharashtra State, India, Eur. J. Med. Plants, 2020, 1–17 Search PubMed.
- S. B. Roopa, J. Thakral and A. N. Kalia, Antioxidant potential fractionation from methanol extract of aerial parts of Ruellia prostrata poir (Acanthaceae), Int. J. Pharm. Sci. Res., 2011, 2(4), 1015–1022 Search PubMed.
- C. O. Wangia, J. A. Orwa, F. W. Muregi, P. G. Kareru, K. Cheruiyot and E. Guantai, Quantitative and Fourier Transform Infrared Analysis of Saponins from Three Kenyan Ruellia Species: Ruellia prostrata, Ruellia lineari-bracteolata and Ruellia bignoniiflora, Int. J. Biol. Ecol. Eng., 2018, 12(6), 155–159 Search PubMed.
- K. Maneenoon, C. Khuniad, Y. Teanuan, N. Saedan, S. Prom-In, N. Rukleng, W. Kongpool, P. Pinsook and W. Wongwiwat, Ethnomedicinal plants used by traditional healers in Phatthalung Province, Peninsular Thailand, J. Ethnobiol. Ethnomed., 2015, 11, 43 CrossRef PubMed.
- K. Kirtikar and B. J. D. BasuIndian medicinal plants International book distributors, 1995, India, pp. 1–456 Search PubMed.
- S. Jain and U. Patil, Phytochemical and pharmacological profile of Cassia tora Linn. -An Overview, 2010 Search PubMed.
- H. A. Pawar and K. J. I. J. o. B. Lalitha, Extraction, characterization, and molecular weight determination of Senna tora (L.) seed polysaccharide, vol. 2015, 2015 Search PubMed.
- M. L. A. San San Maw, H. H. Lwin and M. Z. Z. Thet, Qualitative Analysis and Antimicrobial Activities on Leaves of Senna tora (L.) Roxb, 3rd Myanmar Korea, Conf. Res. J., 2020, 3(2), 2020 Search PubMed.
- R. Gaykhe, S. Khan and V. Kadam, Biochemical Profile of Senna tora Linn, Int. J. Biomed. Invest., 2018, 1(3) DOI:10.31531/2581-4745.1000116.
- M. Samuni-Blank, Intraspecific directed deterrence by the mustard oil bomb in a desert plant, Curr. Biol., 2012, 22(13), 1218–1220 CrossRef CAS PubMed.
- N. Chankana, A. Prapaiwong and L. Charoenchai, Antifungal effect of Senna tora (L.) Roxb. Seed extract against Microsporum gypseum, Proceedings in the RSU International Research Conference, 2017, 76, 81 Search PubMed.
- V. R. Tripathi, S. Kumar and S. K. Garg, A study on trypsin, Aspergillus flavus and Bacillus sp. protease inhibitory activity in Cassia tora (L.) syn Senna tora (L.) Roxb. seed extract, BMC Complementary Altern. Med., 2011, 11(1), 56 CrossRef PubMed.
- S. Shukla, R. Pandey, B. Kumar, S. K. Tewari and M. Pal, Phenolic Compounds and Radical Scavenging Activity of Senna tora (L.) Roxb. Herbs Collected from Various Geographical Locations of India, Pharm. Chem. J., 2021, 55(7), 684–690 CrossRef CAS.
- L. Charoenchai, N. Chankana, O. Theanphong, S. Jongrungruangchok, D. Meksuriyen and V. Lipipun, Formulation Development of Canine Antifungal Shampoo Containing Senna tora (L.) Roxb. Seed Extract, in Key Engineering Materials, Trans Tech Publ, 2020, vol. 859, pp. 181–187 Search PubMed.
- V. T. Sabe, T. Ntombela, L. A. Jhamba, G. Maguire, T. Govender, T. Naicker and H. G. Kruger, Current trends in computer aided drug design and a highlight of drugs discovered via computational techniques: A review, Eur. J. Med. Chem., 2021, 224, 113705 CrossRef CAS PubMed.
- T. A. Bouback, S. Pokhrel, A. Albeshri, A. M. Aljohani, A. Samad, R. Alam, M. S. Hossen, K. Al-Ghamdi, M. Talukder, F. Ahammad, I. Qadri and J. Simal-Gandara, Pharmacophore-Based Virtual Screening, Quantum Mechanics Calculations, and Molecular Dynamics Simulation Approaches Identified Potential Natural Antiviral Drug Candidates against MERS-CoV S1-NTD, Molecules, 2021, 26(16), 4961 CrossRef CAS PubMed.
- T. Talele, S. A. Khedkar and A. C. Rigby, Successful applications of computer aided drug discovery: moving drugs from concept to the clinic, Curr. Top. Med. Chem., 2010, 10(1), 127–141 CrossRef CAS PubMed.
- S. J. Y. Macalino, V. Gosu, S. Hong and S. Choi, Role of computer-aided drug design in modern drug discovery, Arch. Pharmacal Res., 2015, 38(9), 1686–1701 CrossRef CAS PubMed.
- M. M. Rahman, et al., Mikania cordata leaves extract promotes activity against pathogenic bacteria and anticancer activity in EAC cell-bearing swiss albino mice, J. Appl. Pharm. Sci., 2020, 10, 112–122 CrossRef CAS.
- Y. He, Y. Jia and F. Lu, New Products Generated from the Transformations of Ferulic Acid Dilactone, Biomolecules, 2020, 10(2), 175 CrossRef CAS PubMed.
- S. K. Burley, et al., RCSB Protein Data Bank: powerful new tools for exploring 3D structures of biological macromolecules for basic and applied research and education in fundamental biology, biomedicine, biotechnology, bioengineering and energy sciences, Nucleic Acids Res., Jan 8 2021, 49(D1), D437–d451 Search PubMed.
- G. M. Sastry, M. Adzhigirey, T. Day, R. Annabhimoju and W. Sherman, Protein and ligand preparation: parameters, protocols, and influence on virtual screening enrichments, J. Comput.-Aided Mol. Des., 2013, 27(3), 221–234 CrossRef PubMed.
- E. Harder, et al., OPLS3: A Force Field Providing Broad Coverage of Drug-like Small Molecules and Proteins, J. Chem. Theory Comput., 2016, 12(1), 281–296 CrossRef CAS PubMed.
- R. A. Friesner, et al., Extra precision glide: docking and scoring incorporating a model of hydrophobic enclosure for protein–ligand complexes, J. Med. Chem., 2006, 49(21), 6177–6196 CrossRef CAS PubMed.
- W. Zhou, Y. Wang, A. Lu and G. Zhang, Systems Pharmacology in Small Molecular Drug Discovery, Int. J. Mol. Sci., 2016, 17(2), 246 CrossRef PubMed.
- A. Daina, O. Michielin and V. Zoete, SwissADME: a free web tool to evaluate pharmacokinetics, drug-likeness and medicinal chemistry friendliness of small molecules, Sci. Rep., 2017, 7(1), 42717 CrossRef PubMed.
- C. Li, et al., Recent progress in drug delivery, Acta Pharm. Sin. B, 2019, 9(6), 1145–1162 CrossRef PubMed.
- M. D. Segall and C. Barber, Addressing toxicity risk when designing and selecting compounds in early drug discovery, Drug Discovery Today, 2014, 19(5), 688–693 CrossRef CAS PubMed.
- P. Banerjee, A. O. Eckert, A. K. Schrey and R. Preissner, ProTox-II: a webserver for the prediction of toxicity of chemicals, Nucleic Acids Res., 2018, 46(W1), W257–W263 CrossRef CAS PubMed.
- K. J. Bowers et al., Scalable Algorithms for Molecular Dynamics Simulations on Commodity Clusters," in SC '06: Proceedings of the 2006 ACM/IEEE Conference on Supercomputing, 2006, pp. 43–43 Search PubMed.
- K. Roos, et al., OPLS3e: Extending Force Field Coverage for Drug-Like Small Molecules, J. Chem. Theory Comput., 2019, 15(3), 1863–1874 CrossRef CAS PubMed.
- F. Ahammad, R. Alam, R. Mahmud, S. Akhter, E. K. Talukder, A. M. Tonmoy, S. Fahim, K. Al-Ghamdi, A. Samad and I. Qadri, Pharmacoinformatics and molecular dynamics simulation-based phytochemical screening of neem plant (Azadiractha indica) against human cancer by targeting MCM7 protein, Briefings Bioinf., 2021, 22(5), bbab098 CrossRef PubMed.
- T. A. Bouback, et al., Pharmacophore-Based Virtual Screening, Quantum Mechanics Calculations, and Molecular Dynamics Simulation Approaches Identified Potential Natural Antiviral Drug Candidates against MERS-CoV S1-NTD, Molecules, 2021, 26(16), 4961 CrossRef CAS PubMed.
- A. El-Demerdash, A. A. Al-Karmalawy, T. M. Abdel-Aziz, S. S. Elhady, K. M. Darwish and A. H. E. Hassan, Investigating the structure–activity relationship of marine natural polyketides as promising SARS-CoV-2 main protease inhibitors, RSC Adv., 2021, 11(50), 31339–31363, 10.1039/d1ra05817g.
- A. Zeidler and T. M. Karpinski, SARS-CoV, MERS-CoV, SARS-CoV-2 Comparison of Three Emerging Coronaviruses, Jundishapur J. Microbiol., 2020, 13(6), e103744 CrossRef CAS.
- T. M. Karpiński, M. Ożarowski, A. Seremak-Mrozikiewicz, H. Wolski and D. Wlodkowic, The 2020 race towards SARS-CoV-2 specific vaccines, Theranostics, 2021, 11(4), 1690–1702 CrossRef PubMed.
- S. M. Rahman, Anti-inflammatory, antinociceptive and antidiarrhoeal activities of methanol and ethyl acetate extract of Hemigraphis alternata leaves in mice, Clin. Phytosci., 2019, 5(1), 1–13 CrossRef.
- B. B. Mishra and V. K. Tiwari, Natural products: an evolving role in future drug discovery, Eur. J. Med. Chem., 2011, 46(10), 4769–4807 CrossRef CAS PubMed.
- M. Tahir Ul Qamar, S. M. Alqahtani, M. A. Alamri and L. L. Chen, Structural basis of SARS-CoV-2 3CL(pro) and anti-COVID-19 drug discovery from medicinal plants, J. Pharm. Anal., 2020, 10(4), 313–319 CrossRef PubMed.
- A. Rakib, et al., Biochemical and Computational Approach of Selected Phytocompounds from Tinospora crispa in the Management of COVID-19, Molecules, 2020, 25(17) CrossRef PubMed.
- B. Goyal and D. Goyal, Targeting the Dimerization of the Main Protease of Coronaviruses: A Potential Broad-Spectrum Therapeutic Strategy, ACS Comb. Sci., 2020, 22(6), 297–305 CrossRef CAS PubMed.
- C. A. Lipinski, Lead- and drug-like compounds: the rule-of-five revolution, Drug Discovery Today: Technol., 2004, 1(4), 337–341 CrossRef CAS PubMed.
- M. P. Pollastri, Overview on the Rule of Five, Curr. Protoc. Pharmacol., 2010 Search PubMed.
- M. O. Aljahdali, M. H. R. Molla and F. Ahammad, Compounds Identified from Marine Mangrove Plant (Avicennia alba) as Potential Antiviral Drug Candidates Against WDSV, an In-Silico Approach, Mar. Drugs, 2021, 19(5) CrossRef CAS PubMed.
- S. Bharadwaj, A. Dubey, U. Yadava, S. K. Mishra, S. G. Kang and V. D. Dwivedi, Exploration of natural compounds with anti-SARS-CoV-2 activity via inhibition of SARS-CoV-2 Mpro, Briefings Bioinf., 2021, 22(2), 1361–1377 CrossRef CAS PubMed.
- S. Krupanidhi, et al., Screening of phytochemical compounds of Tinospora cordifolia for their inhibitory activity on SARS-CoV-2: an in silico study, J. Biomol. Struct. Dyn., 2020, 1–5 Search PubMed.
- N. Baildya, A. A. Khan, N. N. Ghosh, T. Dutta and A. P. Chattopadhyay, Screening of potential drug from Azadirachta Indica (Neem) extracts for SARS-CoV-2: An insight from molecular docking and MD-simulation studies, J. Mol. Struct., 2021, 1227, 129390 CrossRef CAS PubMed.
- D. Elebeedy, et al., Anti-SARS-CoV-2 activities of tanshinone IIA, carnosic acid, rosmarinic acid, salvianolic acid, baicalein, and glycyrrhetinic acid between computational and in vitro insights, RSC Adv., 2021, 11(47), 29267–29286, 10.1039/d1ra05268c.
- S. Mahmud, et al., Computational discovery of plant-based inhibitors against human carbonic anhydrase IX and molecular dynamics simulation, J. Biomol. Struct. Dyn., 2021, 39(8), 2754–2770 CrossRef CAS PubMed.
- A. Mahmoud, et al., Telaprevir is a potential drug for repurposing against SARS-CoV-2: computational and in vitro studies, Heliyon, 2021, 7(9), e07962 CrossRef PubMed.
Footnote |
† Electronic supplementary information (ESI) available. See DOI: 10.1039/d1ra06842c |
|
This journal is © The Royal Society of Chemistry 2021 |