DOI:
10.1039/D1RA04318H
(Paper)
RSC Adv., 2021,
11, 25933-25942
Optimisation of a gold nanoparticle-based aptasensor integrated with image processing for the colorimetric detection of acephate using response surface methodology†
Received
4th June 2021
, Accepted 7th July 2021
First published on 28th July 2021
Abstract
Acephate (Ac) is an organophosphate (OP) compound, which is able to inhibit the activity of acetylcholinesterase. Thus, the aim of this study was to optimize the detection of Ac using a thiolated acephate binding aptamer-citrate capped gold nanoparticle (TABA–Cit-AuNP) sensor that also incorporated an image processing technique. The effects of independent variables, such as the incubation period of TABA–Cit-AuNPs (3–24 h) for binding TABA to Cit-AuNPs, the concentration of phosphate buffer saline (PBS) (0.001–0.01 M), the concentration of thiolated acephate binding aptamer (TABA) (50–200 nM), and the concentration of magnesium sulphate (MgSO4) (1–300 mM) were investigated. A quadratic model was developed using a central composite design (CCD) from response surface methodology (RSM) to predict the sensing response to Ac. The optimum conditions such as the concentration of PBS (0.01 M), the concentration of TABA (200 nM), the incubation period of TABA–Cit-AuNPs (3 h), and the concentration of MgSO4 (1 mM) were used to produce a TABA–Cit-AuNPs sensor for the detection of Ac. Under optimal conditions, this sensor showed a detection ranging from 0.01 to 2.73 μM and a limit of detection (LOD) of 0.06 μM. Real sample analysis demonstrated this aptasensor as a good analytical method to detect Ac.
Introduction
Acephate (Ac) is widely used as an organophosphate (OP) insecticide for the crop protection.1 It is a highly water-soluble chemical; therefore, it can easily contaminate groundwater and soil, in addition to being easily absorbed by plants, which subsequently accumulate in their edible parts.2–4 High application levels of Ac have resulted in health problems and created environmental pollution issues.1 OPs are highly neurotoxic synthetic compounds5 that inhibit cholinesterase and regulate acetylcholine needed for the functioning of the nervous system.6,7 It is important to detect the presence of Ac even at low levels. In line with this matter, numerous detection techniques have been developed for the determination of Ops, including Ac.
Gas chromatography-mass spectrometry (GCMS),8–11 liquid chromatography-mass spectrometry (LCMS),12–14 high performance liquid chromatography (HPLC),15–17 and surface-enhanced Raman spectroscopy (SERS)18–22 are common techniques used for the detection of OPs with high accuracy and precision. However, their bulky size, complicated operating procedures, long operation time, and expensive analytical procedures justify the need for a simple, easy to operate, and affordable method that can be employed on site. Many sensors have been developed based on colorimetric23–25 and electrochemical26–28 detection methods for the detection of OPs. However, a rapid, sensitive, and selective method for an accurate detection of Ac remains highly desired.
Sensitive and selective colorimetric detection methods for OPs have been rapidly developed due to their reliability and cost-effectiveness.29,30 Noble metal nanoparticles have been extensively utilised for the development of colorimetric sensors due to their strong localized surface plasmon resonance (LSPR) extinction in the visible light region.31,32 Gold nanoparticles (AuNPs) have also been known to be an effective sensor array owing to their inherent stability and distinct color changes as the detection takes place.33–36 This colour change can be easily distinguished using the naked eye without needing precise instruments.37 In addition, Wang et al. (2010) suggested that AuNPs are ideal candidates for colorimetric assays as they can be prepared in suitable sizes and possess distance-dependent optical properties.38 The color of AuNP suspension was sensitive to interparticle aggregation and/or dispersion due to changing interparticle plasmon coupling and surface plasmon band shift. Moreover, the extinction coefficient of AuNPs was higher than that of organic dyes,39 which thus enhanced the sensitivity of AuNP-based colorimetric recognition.35,40 Therefore, AuNP-based colorimetric assays have been developed as useful analytical tools for the detection of various analytes (pesticides, anions, metal ions, and biomolecules) in real samples.41
One of the best approaches to improve the selectivity and sensitivity of a sensor array is to incorporate molecular recognition elements such as aptamers to AuNPs.33,42,43 Compared to antibodies, aptamers exhibit certain outstanding merits, such as excellent stability, in vitro synthesis, ease of modification and fixing, small size, low toxicity, and cost efficiency.44 Aptamers are single-stranded, synthetic oligonucleotides (DNA or RNA), which fold into 3-dimensional shapes45,46 and are capable of binding non-covalently and with high affinity to a target molecule.47,48 They can bind with such specificity that they can differentiate enantiomers and molecules that differ by as little as one functional group.49 Moreover, molecular interactions between aptamers and their target molecules are affected by physicochemical conditions of the binding environment, including both ionic concentration and pH.45,50–55 Li et al. (2021) developed a label-free target binding induced aptamer structure switch colorimetric sensor for ultrasensitive detection of kanamycin based on Exo I-assisted signal amplification and polycationic protamine mediated aggregation of negatively charged AuNPs with a low detection limit of 2.8 × 10−14 M under optimal conditions.44 Besides that, many studies have used AuNPs based aptasensors for the detection of OPs, which resulted in notable low limits of detection (LOD).23,33,36,56–60
The detection of an analyte is simple and easy using a colorimetric method; however, there is a concern about detection accuracy, which may arise when the determination of color change is only based on naked eyes. Still, enhanced reproducibility can be achieved by applying an image processing technique to convert the captured color images into RGB (red, green, and blue) values. Image processing techniques have been reported as being an excellent technique to replace naked eyes for the determination of color change of a sensor for the detection of OPs.61,62 Using image processing, one can eliminate the subjectivity found when performing manual readings, thereby promoting accuracy in recognising the color change caused by the detection of OPs.63 Detection accuracy of Ac was improved using a colorimetric sensor array consisting of citrate-capped gold nanoparticles (Cit-AuNPs), which was coupled with an applied image processing.64 Ma et al. (2021) developed an AuNPs based colorimetric sensor for the detection of pesticides, in which the color changes of the aggregated AuNPs were captured using a smartphone camera, and the images were processed to obtain average RGB (red, green, and blue) values using self-developed software.65
Recently, Qu et al. (2020) evaluated the effect of pH and ionic strength (through the addition of common salt (NaCl)) on the sensitivity and selectivity of colorimetric detection towards ametryn. They noted that pH played a major role during the detection of ametryn by changing the influence of aggregation behavior of AuNPs, subsequently affecting its absorption profiles. Changing the ionic strength of the sensing solutions might result in improved aggregation behaviour of AuNPs towards ametryn but might also result in unstable AuNPs.66 Another study by Hwang et al. (2019) investigated the effect of the concentration of aptamer on colorimetric capability towards bisphenol A. The concentration of aptamer played an important role as it could result in false-positive and negative signals.67 To the best of our knowledge, however, literature examples using AuNPs based aptasensors, which are enhanced by adding salt and integrated with image processing techniques, are still lacking for the sensing of Ac.
Therefore, in this study, we demonstrated using AuNPs, modified DNA aptamer (modified with thiol group), and magnesium sulphate salt to enhance the selectivity and sensitivity of a colorimetric aptasensor for the detection of Ac. Moreover, image processing was employed to improve the accuracy of the detection. To obtain the best results for the detection, the following parameters were optimised using response surface methodology (RSM) based central composite design (CCD): the incubation period of thiolated acephate binding aptamer-based citrate capped AuNPs (TABA–Cit-AuNPs) (3–24 h) for binding TABA to Cit-AuNPs, a specific concentration of phosphate buffer saline (PBS) (0.001–0.01 M), and concentration of magnesium sulphate (MgSO4) (1–300 mM). Images of the color solution before and after detection were captured using a portable digital microscope. Then the colorimetric images were converted to R, G, B values using ImageJ software, where later R value was chosen as the response parameter for enhancement of detection accuracy.
Materials and methodology
Materials
Thiolated acephate binding aptamer (TABA) with a sequence of 5′-thiol-AAG CTT GCT TTA TAG CCT GCA GCG ATT CTT GAT CGG AAA AGG CTG AGA GCT ACG C-3′ was purchased from Integrated DNA Technologies, Singapore. The DNA sequence used in this research was similar to that used by previous researchers,56,68 with a modification through the addition of a thiol group at the beginning of the TABA sequence (C5). Gold(III) chloride trihydrate (HAuCl4·3H2O; >49% Au basis), acephate (Ac) (C4H10NO3PS) Pestanal analytical grade, and phosphate buffered saline powder (PBS) (pH: 7.4) were purchased from Sigma Aldrich, USA. Tri-sodium citrate dihydrate (C6H5Na3O7·2H2O) (Na-Cit) and magnesium sulphate (MgSO4) were purchased from Merck KGaA, Germany. All other chemicals were of analytical grade. All preparation and dilution of solutions were carried out using Milli-Q water with a resistivity of 18.2 MΩ cm.
Synthesis of citrate capped gold nanoparticles
Citrate capped gold nanoparticles (Cit-AuNPs) were synthesised by mixing 100 mL of 0.25 mM gold(III) chloride trihydrate and 2 mL of 34 mM Na-Cit in a beaker and heated in a microwave oven chamber (NN-CS599S, Panasonic) for 10 min at 300 W. The prepared Cit-AuNPs suspension was then stored in a dark amber glass bottle at 5.0 ± 0.5 °C.
Preparation of thiolated acephate binding aptamer-based citrate capped gold nanoparticles (TABA–Cit-AuNPs)
Thiolated acephate binding aptamer-based citrate capped gold nanoparticles (TABA–Cit-AuNPs) suspensions were freshly prepared for each detection. One hundred μL of TABA was incubated with the synthesised AuNPs (800 μL) at 25 °C for a chosen period (between 3.0 to 24.0 h). The suspension was then centrifuged at 12
000 rpm for 10.0 min to remove excess TABA and then re-dispersed using Milli-Q water.
Experimental design and statistical analysis
The experiments were designed at three different levels based on a central composite design (CCD) of response surface methodology (RSM) using Design Expert 11 software (USA). The parameters investigated in this study were concentration of PBS (A) (0.001–0.01 M), concentration of TABA (B) (50–200 nM), incubation period of TABA–Cit-AuNPs (C) (3.0–24.0 h), and concentration of MgSO4 (D) (1–300 mM). The experimental design of a total of 30 experimental runs for optimisation of Ac detection is presented in Table 1.
Table 1 Experimental factor in coded and actual units
Standard run number |
A |
B |
C |
D |
1 |
0.010 |
200 |
3.0 |
1.0 |
2 |
0.005 |
125 |
3.0 |
150.5 |
3 |
0.005 |
125 |
13.5 |
150.5 |
4 |
0.010 |
50 |
3.0 |
300.0 |
5 |
0.005 |
125 |
13.5 |
1.0 |
6 |
0.005 |
125 |
13.5 |
300.0 |
7 |
0.001 |
200 |
3.0 |
1.0 |
8 |
0.001 |
50 |
3.0 |
300.0 |
9 |
0.010 |
200 |
3.0 |
300.0 |
10 |
0.010 |
200 |
24.0 |
1.0 |
11 |
0.001 |
50 |
24.0 |
300.0 |
12 |
0.005 |
200 |
13.5 |
150.5 |
13 |
0.010 |
50 |
24.0 |
300.0 |
14 |
0.001 |
50 |
3.0 |
1.0 |
15 |
0.005 |
125 |
13.5 |
150.5 |
16 |
0.005 |
125 |
24.0 |
150.5 |
17 |
0.010 |
200 |
24.0 |
300.0 |
18 |
0.010 |
50 |
3.0 |
1.0 |
19 |
0.010 |
50 |
24.0 |
1.0 |
20 |
0.001 |
50 |
24.0 |
1.0 |
21 |
0.001 |
200 |
24.0 |
1.0 |
22 |
0.005 |
125 |
13.5 |
150.5 |
23 |
0.005 |
125 |
13.5 |
150.5 |
24 |
0.001 |
200 |
3.0 |
300.0 |
25 |
0.005 |
125 |
13.5 |
150.5 |
26 |
0.005 |
125 |
13.5 |
150.5 |
27 |
0.010 |
125 |
13.5 |
150.5 |
28 |
0.005 |
50 |
13.5 |
150.5 |
29 |
0.001 |
200 |
24.0 |
300.0 |
30 |
0.001 |
125 |
13.5 |
150.5 |
The response was used to generate a mathematical model, which relates to the independent variables using a second-degree polynomial equation as shown in eqn (1).
|
Y = X0 + X1A + X2B + X3C + X4D + X5A2 + X5B2 + X7C2 + X8D2 + X9AB + X10AC + X11AD + X12BC + X13BD + X14CD
| (1) |
where
Y,
X0,
X1–
X4,
X5–
X8, and
X9–
X14 are the predicted responses, the constant coefficient, linear coefficients, quadratic coefficients, and interaction coefficients.
Analysis of variance (ANOVA) was used for graphical analyses of the data to identify the interactions between the variables and responses. The accuracy of the fitted model was determined by the R2 of the coefficient. The significant model terms were evaluated by their probability values (p-value) at a 95% confidence interval.
Optimisation of acephate detection conditions
Detection of 1.09 mM of Ac for each experimental run was performed in triplicate under the conditions shown in Table 1. At first, 100 μL of PBS was added into 100 μL of the prepared TABA–Cit-AuNPs suspension in a glass vial. Then, 100 μL of Ac was mixed into the glass vial before adding 100 μL of magnesium salt. Finally, the glass vial was placed in an image capturing box for image capturing of the mixture using a portable digital microscope at a fixed distance of 10.5 cm (Fig. 1).
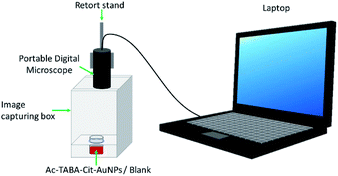 |
| Fig. 1 Schematic of experimental set-up for detection of Ac assisted by image processing technique. | |
Image processing of captured images
The captured color images were processed using ImageJ software (Fig. S1†). First, each image was cropped to a constant area carefully, as displayed in Fig. S1(a).† Then, the undesirable region of the image was cleared by the software (Fig. S1(b)†). Eventually, the mean RGB values were extracted from the image (Fig. S1(c) and (d)†), and red value (RV) was used as the response of the colorimetric aptasensor.
Validation of the detection optimum conditions
Validation of the detection optimum conditions was conducted by detecting 1.09 mM of Ac (1.09 mM) at the following proposed optimum conditions by CCD-RSM: concentration of PBS (0.01 M), concentration of TABA (200 nM), incubation period of TABA–Cit-AuNPs (3 h), and concentration of MgSO4 (1 mM) in five replications. In brief, 100 μL of PBS was added to 100 μL of the prepared TABA–Cit-AuNPs suspension in a glass vial. Then, 100 μL of Ac was mixed into the glass vial before adding 100 μL of MgSO4. Eventually, the mean of experimental response (RVs) was determined using the standard curve of AC and compared with the mean of predicted response (RVs).
Development of acephate standard curve
The detection of various concentrations (0.01, 0.03, 0.05, 0.14, 0.27, 0.55, 1.36, and 2.73 μM) of Ac was conducted under optimum conditions in 3 replications for the construction of the Ac standard curve.
Real sample analysis
To assess the applicability of the colorimetric aptasensor, real sample analysis was studied by spiking real samples (tap water and lake water) with Ac at chosen concentrations (0.05, 0.27, 0.55, and 2.73 mM) under optimum conditions. All collected water samples were filtered through filter paper and kept at 5 °C in a dark capped bottle. Next, in a glass vial, 100 μL of the Ac spiked water sample was added to the prepared TABA–Cit-AuNPs. Then, 100 μL of 300 mM MgSO4 containing Mg2+ ion was added to the mixture. The images of the Ac–TABA–Cit-AuNPs complex formed were photographed and digitised. Eqn (2) was used to calculate the recovery of Ac in various water samples, where the calculated concentration of Ac was determined from the standard curve of Ac. |
 | (2) |
Independent t-test
All independent t-test analyses were conducted using Minitab 18 software at a 95% confidence level.
Results and discussion
In this study, a total number of 30 experiments of four different combinations of tested parameters involving PBS concentration, TABA concentration, incubation period of TABA–Cit-AuNPs, and MgSO4 concentration were evaluated. The results of mean RVs according to the central composite design are shown in Table 2. In this design, the experimental data were analysed using multiple regression analysis and then generated through a second-order polynomial equation, where mean RVs were a function of the four tested independent parameters.
Table 2 Experimental factors in coded and actual units and experimental responses
Experimental run |
A |
B |
C |
D |
Response (RVs) |
Experimental data |
Predicted data |
1 |
0.010 |
200 |
3.0 |
1.0 |
33.97 ± 0.68 |
33.09 |
2 |
0.005 |
125 |
3.0 |
150.5 |
38.13 ± 0.32 |
38.32 |
3 |
0.005 |
125 |
13.5 |
150.5 |
43.10 ± 1.45 |
43.14 |
4 |
0.010 |
50 |
3.0 |
300.0 |
44.25 ± 0.11 |
44.29 |
5 |
0.005 |
125 |
13.5 |
1.0 |
36.99 ± 0.41 |
37.55 |
6 |
0.005 |
125 |
13.5 |
300.0 |
43.65 ± 1.04 |
43.13 |
7 |
0.001 |
200 |
3.0 |
1.0 |
37.91 ± 0.67 |
38.37 |
8 |
0.001 |
50 |
3.0 |
300.0 |
43.11 ± 1.38 |
43.34 |
9 |
0.010 |
200 |
3.0 |
300.0 |
43.32 ± 0.41 |
43.69 |
10 |
0.010 |
200 |
24.0 |
1.0 |
36.10 ± 2.07 |
36.20 |
11 |
0.001 |
50 |
24.0 |
300.0 |
37.64 ± 2.12 |
38.17 |
12 |
0.005 |
200 |
13.5 |
150.5 |
46.89 ± 1.84 |
47.73 |
13 |
0.010 |
50 |
24.0 |
300.0 |
41.18 ± 0.33 |
41.06 |
14 |
0.001 |
50 |
3.0 |
1.0 |
38.07 ± 1.91 |
37.84 |
15 |
0.005 |
125 |
13.5 |
150.5 |
43.57 ± 1.73 |
43.14 |
16 |
0.005 |
125 |
24.0 |
150.5 |
37.45 ± 0.07 |
37.29 |
17 |
0.010 |
200 |
24.0 |
300.0 |
41.95 ± 0.52 |
41.84 |
18 |
0.010 |
50 |
3.0 |
1.0 |
35.07 ± 2.33 |
35.35 |
19 |
0.010 |
50 |
24.0 |
1.0 |
36.95 ± 0.49 |
37.07 |
20 |
0.001 |
50 |
24.0 |
1.0 |
37.66 ± 1.11 |
37.62 |
21 |
0.001 |
200 |
24.0 |
1.0 |
39.92 ± 1.27 |
39.54 |
22 |
0.005 |
125 |
13.5 |
150.5 |
42.48 ± 1.17 |
43.14 |
23 |
0.005 |
125 |
13.5 |
150.5 |
43.66 ± 1.43 |
43.14 |
24 |
0.001 |
200 |
3.0 |
300.0 |
45.98 ± 2.40 |
45.53 |
25 |
0.005 |
125 |
13.5 |
150.5 |
42.70 ± 2.37 |
43.14 |
26 |
0.005 |
125 |
13.5 |
150.5 |
43.43 ± 0.12 |
43.14 |
27 |
0.010 |
125 |
13.5 |
150.5 |
42.72 ± 0.33 |
42.93 |
28 |
0.005 |
50 |
13.5 |
150.5 |
47.87 ± 1.44 |
47.07 |
29 |
0.001 |
200 |
24.0 |
300.0 |
41.69 ± 0.60 |
41.75 |
30 |
0.001 |
125 |
13.5 |
150.5 |
44.31 ± 2.11 |
44.13 |
The following second-order polynomial is shown in eqn (3):
|
Y = 43.14 − 0.60A + 0.33B − 0.52C + 2.79D − 0.70AB + 0.48AC + 0.86AD + 0.35BC + 0.41BD − 1.24CD + 0.40A2 + 4.26B2 − 5.33C2 − 2.80D2
| (3) |
where
Y = mean RV;
A,
B,
C, and
D are coded values of PBS concentration, TABA concentration, the incubation period of TABA–Cit-AuNPs, and MgSO
4 concentration, respectively. Based on the above equation, the quadratic model was generated and evaluated by the determination of
F-value and
p-value, and it was found to be 77.39 and <0.0001, respectively. This indicated that our developed model was highly significant.
69–71 The model fitness was assessed through a non-significant
p-value lack of fit test, which was found to be 0.2809, thus revealing that there was a 28.09% chance that the lack of fit could be largely due to noise, confirming its validity.
72 The applicability of the developed model was determined using the values of
R2, adjusted
R, and coefficient variance (CV).
69 The
R2 value was 0.986, revealing that only 1.4% of the model's variability could not be explained.
70,73 Furthermore, the expected
R2 value was 0.931, which was close to the
R2 value (0.986), thus exhibiting a good correlation between the experimental and predicted data.
74,75 The developed model thus shows good reliability and high precision based on the low coefficient of variance (C.V.) obtained (1.44).
70,73
All the tested independent parameters, such as the concentration of PBS (A), the concentration of TABA (B), the incubation period of TABA–Cit-AuNPs (C), and concentration of MgSO4 (D) had significant effects on the reduction in RV values, as shown in Table 3, which reflects the degree of aggregation of Ac–TABA–Cit-AuNPs complexation. Besides that, all the linear coefficients (A, B, C, and D), cross-product coefficients (AB, AC, AD, BC, BD, and CD), and quadratic coefficients (B2, C2, D2) were significant with their p-values being <0.05. Among the tested parameters, MgSO4 concentration was found to have a major influence on the aggregation of Ac–TABA–Cit-AuNPs based on the high F-value of 401.65 obtained compared to other parameters. Meanwhile, the concentration of TABA was found to have the least significant effect on the aggregation of Ac–TABA–Cit-AuNPs.
Table 3 Analysis of variance (ANOVA) for response surface quadratic model for RVs
Source |
Sum of squares |
df |
Mean square |
F-Value |
p-Value |
Significant. Not significant. |
Model |
377.00 |
14 |
26.93 |
77.39 |
<0.0001a |
A |
6.44 |
1 |
6.44 |
18.52 |
0.0006a |
B |
1.95 |
1 |
1.95 |
5.61 |
0.0317a |
C |
4.79 |
1 |
4.79 |
13.76 |
0.0021a |
D |
139.75 |
1 |
139.75 |
401.65 |
<0.0001a |
AB |
7.77 |
1 |
7.77 |
22.33 |
0.0003a |
AC |
3.76 |
1 |
3.76 |
10.79 |
0.0050a |
AD |
11.80 |
1 |
11.80 |
33.92 |
<0.0001a |
BC |
1.93 |
1 |
1.93 |
5.54 |
0.0326a |
BD |
2.74 |
1 |
2.74 |
7.87 |
0.0133a |
CD |
24.52 |
1 |
24.52 |
70.48 |
<0.0001a |
A2 |
0.4059 |
1 |
0.4059 |
1.17 |
0.2972b |
B2 |
47.10 |
1 |
47.10 |
135.38 |
<0.0001a |
C2 |
73.58 |
1 |
73.58 |
211.47 |
<0.0001a |
D2 |
20.25 |
1 |
20.25 |
58.21 |
<0.0001a |
Residual |
5.22 |
15 |
0.3479 |
— |
— |
Lack of fit |
4.05 |
10 |
0.4055 |
1.74 |
0.2809b |
Pure error |
1.16 |
5 |
0.2329 |
— |
— |
Cor total |
382.22 |
29 |
— |
— |
— |
R2 = 0.986 |
R2 adjusted = 0.974 |
R2 predicted = 0.931 |
C.V. = 1.44% |
Three-dimensional response surface (3D) and contour plots were plotted through the second-order polynomial equation (eqn (3)) to better understand the four tested parameters' behaviour towards the RVs.
In these graphical plots, the RVs were obtained by varying the values of two different tested parameters, while the other two parameters were fixed at constant values. The shape of the contour plots, whether circular or elliptical, indicates the significance of interactions between variables.76 A circular contour represents a less significant effect; meanwhile, elliptical contours represent a high significance effect on the interaction between variables.76,77
The combined effect of independent parameters on the sensitivity of the sensor towards the detection of 1.09 mM Ac is depicted in Fig. 2. It was observed that the lowest RVs, which represented the highest degree of aggregation of Ac–TABA–Cit-AuNPs complex, was 39.343. Fig. 2a illustrates the effect of TABA concentration and PBS concentration on RVs value of the developed sensor. It appears that lower RVs values were found when the range of TABA concentrations was 110–140 nM, and there was no notable change in RVs when decreasing or increasing the PBS concentration. Fig. 2b shows the effect of the incubation period of TABA–Cit-AuNPs and PBS concentration. Similar to the observations in Fig. 2a, the PBS concentration had less impact on RVs values. A rise in RVs value was observed with increasing the incubation period of TABA–Cit-AuNPs from 3 h to 13 h, and RVs slightly declined after 13 h. Fig. 2 c demonstrates the effect of MgSO4 concentration and PBS concentration. At a fixed concentration of PBS, as MgSO4 concentration decreased from 300 to 1 mM, RVs values significantly decreased. The effect of the incubation period of TABA–Cit-AuNPs and TABA concentration is depicted in Fig. 2d. Obviously, the highest RVs could be obtained at the incubation period of 12–15 h either at 50 nM or 200 nM TABA concentration. The effect of MgSO4 concentration, TABA concentration, and incubation period of TABA–Cit-AuNPs is shown in Fig. 2e and f. It is clearly seen that a lower concentration of MgSO4 resulted in lower RVs. Meanwhile, RVs decreased with a decline in TABA concentration from 110–140 nM and slightly increased beyond 140 nM. Based on the contour plot (Fig. 2f), the highest and lowest RVs were observed at 240.20 nM MgSO4 concentration at 13.50 h and 1 nM MgSO4 concentration at 1 h, respectively.
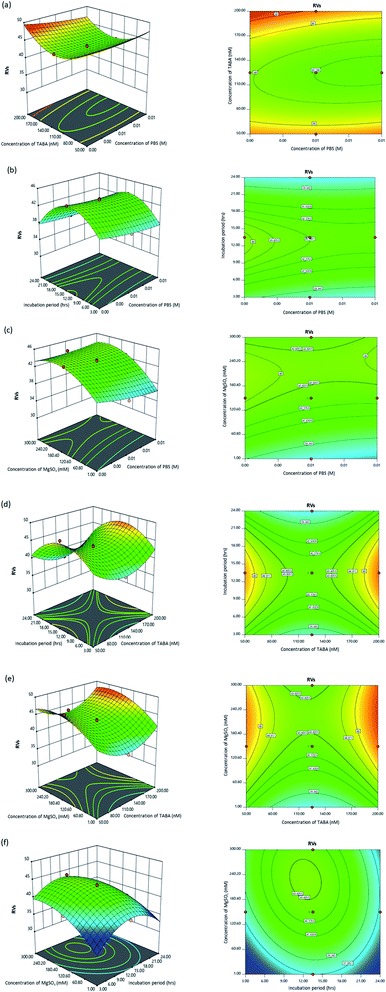 |
| Fig. 2 Response surface plot showing the effect of (a) concentration of PBS and concentration of TABA, (b) concentration of PBS and incubation period of TABA–Cit-AuNPs, (c) concentration of PBS and concentration of MgSO4, (d) concentration of TABA and incubation period of TABA–Cit-AuNPs, (e) concentration of PBS and concentration of MgSO4 and (f) incubation period of TABA–Cit-AuNPs and concentration of MgSO4. | |
The detection of Ac was improved when PBS (0.01 M) (pH: 7.4) was used, as it provided a neutral condition for the detection (Fig. 2b and c). Similar optimum working pH (nearly neutral) of TABA were reported by previous researchers.78,79 The detection was also enhanced as the TABA concentration increased due to a higher number of binding sites available for Ac, as shown in Fig. 2d and e. In addition, the formation of covalent bonding between thiol-modified TABA and Cit-AuNPs causes the formation of a strong suspension of TABA–Cit-AuNPs.35,36,80,81 A sufficient incubation period for the formation of TABA–Cit AuNPs complex is crucial to ensure Cit-AuNPs surfaces are fully immobilised with TABA, providing more binding sites for Ac and reduce non-specific binding82,83 towards Ac. A certain amount of MgSO4 was required because of its specific binding to the Ac–TABA–Cit-AuNPs complex, which weakens the repulsive forces between them and facilitates aggregation of the Ac–TABA–Cit-AuNPs complex.84,85 Thus, the colour change is improved from red to blue with and without the presence of Ac. The maximum aggregation for the Ac–TABA–Cit-AuNPs complex was influenced by aptamer folding, which can be seen in Fig. 2c.86,87
Optimisation of acephate detection conditions
The most important part of this study was the determination of optimum sensing conditions for the detection of 1.09 mM Ac, which would be demonstrated by the lowest RVs due to maximum aggregation. Optimisation was carried out using a Design Expert numerical optimisation method. The optimum sensor perimeter to achieve RVs = 33.10 with composite desirability of 1.000 was suggested with the following conditions (Fig. 3).
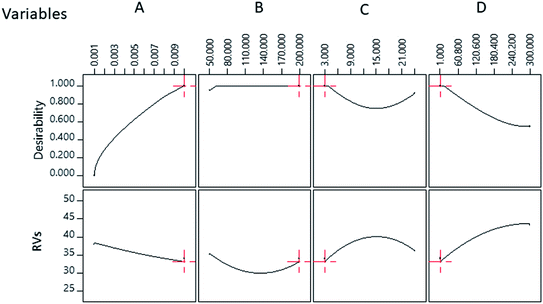 |
| Fig. 3 Numerical optimisation parameter of RVs. | |
The predicted and experimental values of RVs were 33.10 and 33.96, respectively, for the detection of 1.09 mM Ac under optimal conditions. A comparison between the predicted and experiment results indicated that the percentage error was 2.6%. The optimized values for the concentration of PBS, concentration of TABA, concentration of MgSO4 and incubation period of TABA–Cit-AuNPs were found to be 0.01 M, 200 nM, 1 mM, and 3 h, respectively, as shown in Fig. 3.
Standard curve of acephate
Using the optimised sensor, detection at different concentrations (0.01, 0.03, 0.05, 0.14, 0.27, 0.55, 1.36, and 2.73 μM) of Ac was conducted in 3 replications under optimal conditions. A statistical tool (independent t-test) was used to identify any significant differences between the RVs of blank and Ac for all cropped images at a 95% confidence level. The results of the detection and the analysis are summarised in Table 4.
Table 4 Results for detection of various concentrations of Ac at the optimum conditions
Concentration of Ac (μM) |
Blank |
0.01 |
0.03 |
0.05 |
0.14 |
0.27 |
0.55 |
1.36 |
2.73 |
Cropped images |
 |
 |
 |
 |
 |
 |
 |
 |
 |
Mean RVs |
145.5 |
143.1 |
140.2 |
138.7 |
135.4 |
127.1 |
115.6 |
82.3 |
36.2 |
Standard deviation |
0.80 |
0.14 |
0.19 |
0.06 |
0.44 |
0.27 |
0.15 |
0.80 |
0.15 |
Relative standard variation (%) |
0.55 |
0.10 |
0.13 |
0.04 |
0.33 |
0.21 |
0.13 |
0.98 |
0.42 |
p-Value |
— |
0.037 |
0.008 |
0.005 |
0.000 |
0.000 |
0.000 |
0.000 |
0.000 |
The significant difference between the two RVs was determined from the p-value of the t-test. The results revealed that all mean RVs values of Ac (0.01–2.73 μM) had a significant difference compared to that of the blank as indicated by p-values of <0.05. Therefore, the results indicated the promising potential of the colorimetric sensor for the detection of Ac. Standard deviations (RSDs) were applied to evaluate the reproducibility of the results in this study. In addition, the study by Hongsibsong et al. (2020) also used RSDs as the coefficient of variation to evaluate the reproducibility of their results.88 According to Gao et al. (2013), the coefficient of variation is usually termed as RSD,89 whereas Cozma et al. (2015) concluded that there was no significant intra-assay and inter-assay variation within the run and between the runs for aliquots of the sample with an RSD% < 15%.90 Based on the results (Table 4), detection of Ac (0.01–2.73 μM) under optimal conditions demonstrated good reproducibility as indicated by the low RSDs values (<1%). Thus, the developed colorimetric aptasensor can be used for the detection of Ac.
The mean values of RVs were used in developing a linear regression model to detect Ac in a linear range of 0.01–2.73 μM (Fig. 4). The calibration curve displays a high correlation of coefficient value (0.9921). The limit of detection (LOD) of Ac was found to be 0.06 μM, which was calculated using the formula reported by Bala et al. (2016).91
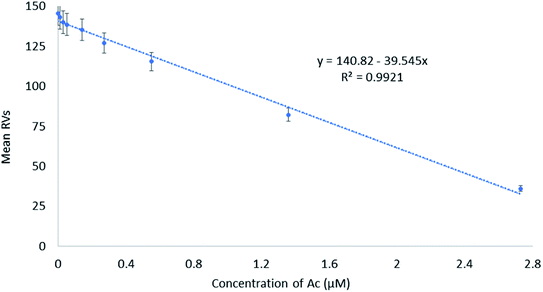 |
| Fig. 4 Standard curve of Ac by optimised colorimetric aptasensor coupled with image processing. | |
Fig. 5 illustrates the proposed detection mechanism using the colorimetric aptasensor. After AuNPs are synthesized using the citrate reduction method, TABA is immobilised on the surface of AuNPs through the formation of covalent bonding between Au and S (from the thiol group of the aptamer), which is stronger than the electrostatic attraction of Au–citrate forming the TABA–Cit-AuNPs complex. In the absence of Ac, the TABA–Cit-AuNPs complex is stabilised by a repulsive electrostatic interaction between negatively charged phosphate group ions of TABA with the counter-ions in the medium, which prevents the AuNPs aggregation and maintains the red colloidal AuNPs solution against high concentrations of magnesium sulfate.92 Upon the introduction of Ac, the interaction between Ac and aptamer sequences induces a conformal change in TABA and hinders its electrostatic repulsion between the negatively charged phosphate group of TABA with the counter-ions in the medium. As a result, AuNPs aggregate with the addition of magnesium sulfate, which then leads to a color change of the colloidal AuNPs solution from red to purple.93 A similar explanation of the effect of adding NaCl was reported by Bala et al. (2016).91 The results also showed that the sensitivity of detection could be enhanced with the addition of magnesium sulfate.
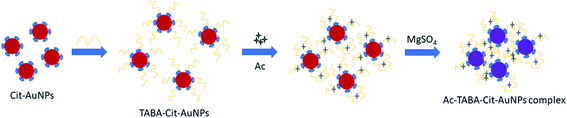 |
| Fig. 5 Schematic illustration of the mechanism detection of acephate using AuNPs based-aptasensor. | |
Real sample analysis
Fig. 6 shows the results of mean RVs obtained from the detection of Ac in different water samples. Water samples from two different sources (tap water and lake water) were taken and spiked with different Ac concentrations (2.73, 0.55, 0.27, and 0.05 μM). The concentrations of Ac in the real samples were then calculated against the standard curve and presented in μM. Good and notable recovery was found and recorded, namely between 100% to 122% for all the samples tested (Table 5). Furthermore, the value was comparable with an acceptable RSD, thus validating the employability of the optimized sensor for the detection of Ac. The same conclusion was made in a previous study.94
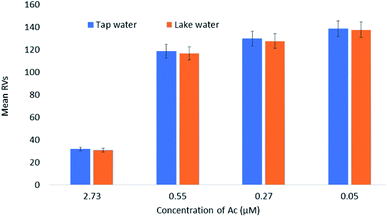 |
| Fig. 6 Detection of Ac in different spiked water samples. | |
Table 5 Detection results of tap water and lake water spiked with Ac
Water sample |
Tap water |
Lake water |
Spiked concentration (μM) |
2.73 |
0.55 |
0.27 |
0.05 |
2.73 |
0.55 |
0.27 |
0.05 |
Cropped images |
 |
 |
 |
 |
 |
 |
 |
 |
Calculated mean concentration (μM) |
2.744 |
0.553 |
0.273 |
0.054 |
2.765 |
0.597 |
0.329 |
0.053 |
Standard deviation |
0.029 |
0.027 |
0.004 |
0.002 |
0.031 |
0.012 |
0.012 |
0.002 |
Relative standard deviation (%) |
1.043 |
4.833 |
1.416 |
3.277 |
1.137 |
1.970 |
3.666 |
4.056 |
Percentage recovery (%) |
100.5 |
100.5 |
101.3 |
108.4 |
101.3 |
108.6 |
121.8 |
106.0 |
Conclusions
CCD-RSM was successfully used for the optimisation of colorimetric detection of Ac. CCD-RSM gave a better understanding of the relationship of all parameters on the digitised RVs values of the complex formed after exposure to Ac. Based on the ANOVA analysis, a small lack of fit value and the C.V. value proved that the developed model has a low level of pure error and showed good reliability and high precision. The TABA–CIt-AuNPs aptasensor was optimised as suggested by the results of the CCD-RSM based on the parameters that produced the lowest RVs values. The optimised parameters suggested by numerical optimisation (0.01 M concentration of 100 μL PBS, 200 nM concentration of 100 μL TABA, 3 h incubation period of TABA–Cit-AuNPs, and 1 mM concentration of 100 μL MgSO4) demonstrated the good potential for Ac detection by the aptasensor with the LOD being 0.06 μM. Good recovery was recorded for both tap water and lake water samples, which indicated that the optimized sensor could be a good candidate for on-site detection of Ac.
Conflicts of interest
There are no conflicts to declare.
Acknowledgements
We would like to thank Ministry of Higher Education Malaysia for funding this project (UPNM/2018/CHEMDEF/ST/2) and Universiti Pertahanan Nasional Malaysia for providing the workplace and facilities to conduct the project.
References
- V. Kumar, N. Upadhyay, V. Kumar and S. Sharma, Arabian J. Chem., 2015, 8, 624–631 CrossRef CAS.
- Z. Lin, S. Pang, W. Zhang, S. Mishra, P. Bhatt and S. Chen, Front. Microbiol., 2020, 11, 1–18 CrossRef PubMed.
- S. Mohapatra, A. K. Ahuja, M. Deepa and D. Sharma, Bull. Environ. Contam. Toxicol., 2011, 86, 101–104 CrossRef CAS PubMed.
- J. H. Syed, A. Alamdar, A. Mohammad, K. Ahad, Z. Shabir, H. Ahmed, S. M. Ali, S. G. A. S. Sani, H. Bokhari, K. D. Gallagher, I. Ahmad and S. A. M. A. S. Eqani, Environ. Sci. Pollut. Res., 2014, 21, 13367–13393 CrossRef CAS PubMed.
- W. Latip, V. F. Knight, N. Abdul Halim, K. K. Ong, N. A. Mohd Kassim, W. M. Z. Wan Yunus, S. A. Mohd Noor and M. S. Mohamad Ali, Catalysts, 2019, 9, 1–11 CrossRef.
- G. Liu and Y. Lin, Anal. Chem., 2005, 77, 5894–5901 CrossRef CAS PubMed.
- F. N. Diauudin, J. I. A. Rashid, V. F. Knight, W. M. Z. Wan Yunus, K. K. Ong, N. A. M. Kasim, N. Abdul Halim and S. A. M. Noor, Sens. Bio-Sens. Res., 2019, 26, 100305 CrossRef.
- T. H. Sukirtha and M. V. Usharani, Int. J. Curr. Microbiol. Appl. Sci., 2013, 2, 321–329 Search PubMed.
- F. Habedank, M. Abraham, H. Tardel, F. Feldhusen and D. E. Schulz-bull, Int. J. Environ. Anal. Chem., 2017, 1–12 Search PubMed.
- A. D. S. Pinheiro, G. Olimpio and J. B. De Andrade, Microchem. J., 2011, 99, 303–308 CrossRef CAS.
- J. Ma, R. Xiao, J. Li, X. Zhao, B. Shi and S. Li, J. Chromatogr. Sci., 2009, 47, 110–115 CAS.
- A. Cappiello, G. Famiglini, P. Palma, F. Mangani and S. Chimiche, Anal. Chem., 2002, 74, 3547–3554 CrossRef CAS PubMed.
- S. Inoue, T. Saito, H. Mase and Y. Suzuki, J. Pharm. Biomed. Anal., 2007, 44, 258–264 CrossRef CAS PubMed.
- J. L. Armstrong, R. L. Dills, J. Yu, M. G. Yost and R. A. Fenske, J. Environ. Sci. Health, Part B, 2014, 49, 102–108 CrossRef CAS PubMed.
- R. Akoijam, A. Ningombam, A. Beemrote and R. S. Telem, Int. J. Curr. Microbiol. Appl. Sci., 2018, 7, 3036–3041 CrossRef CAS.
- R. Mahajan and S. Chatterjee, Environ. Monit. Assess., 2018, 190, 327–335 CrossRef PubMed.
- P. K. Behniwal and J. She, Int. J. Environ. Anal. Chem., 2017, 1–15 Search PubMed.
- C. Zhu, X. Hu and X. Wang, Appl. Surf. Sci., 2019, 470, 423–429 CrossRef CAS.
- L. Yande, Z. Yuxiang, W. Haiyang and Y. Bing, Int. J. Agric. Biol. Eng., 2016, 9, 179–185 Search PubMed.
- T. A. Benitta, S. Kapoor, S. Christy R, I. S. Raj C and T. T. Kumaran J, Orient. J. Chem., 2017, 33, 760–767 CrossRef CAS.
- T. Yaseen, H. Pu and D. Sun, Talanta, 2018, 196, 537–545 CrossRef PubMed.
- Y. Jiang, D. Sun, H. Pu and Q. Wei, Trends Food Sci. Technol., 2018, 75, 10–22 CrossRef CAS.
- D.-L. Liu, Y. Li, R. Sun, J.-Y. Xu, Y. Chen and C.-Y. Sun, J. Nanosci. Nanotechnol., 2019, 20, 2114–2121 CrossRef PubMed.
- Y. Li, C. Hou, J. LEI, B. Deng, J. Huang and M. Yang, Anal. Sci., 2016, 32, 719–724 CrossRef CAS PubMed.
- G. Rebollar-Pérez, F. Lima-Zambrano, G. Bairán, A. Rodríguez-Enríquez, N. Ornelas-Soto, E. Méndez and E. Torres, Environ. Eng. Sci., 2016, 33, 951–961 CrossRef.
- L. Qiu, P. Lv, C. Zhao, X. Feng, G. Fang, J. Liu and S. Wang, Sens. Actuators, B, 2019, 286, 386–393 CrossRef CAS.
- A. Gothwal, P. Beniwal, V. Dhull and V. Hooda, Int. J. Anal. Chem., 2014, 2014, 1–9 CrossRef PubMed.
- G. Selvolini, I. Bajan, O. Hosu, C. Cristea, R. Sandulescu and G. Marrazza, in Conference: IV Convegno Nazionale Sensori – CNS 2018, Catania, 2019, pp. 135–140 Search PubMed.
- R. Singh, P. Thakur, A. Thakur, H. Kumar, P. Chawla, J. V. Rohit, R. Kaushik and N. Kumar, Int. J. Environ. Anal. Chem., 2020, 1–17 Search PubMed.
- G. Alberti, C. Zanoni and L. R. Magnaghi, Int. J. Environ. Res. Public Health, 2020, 17, 1–23 Search PubMed.
- W. Zhou, X. Gao, D. Liu and X. Chen, Chem. Rev., 2015, 115, 10575–10636 CrossRef CAS PubMed.
- Z. Zhang, H. Wang, Z. Chen, X. Wang, J. Choo and L. Chen, Biosens. Bioelectron., 2018, 114, 52–65 CrossRef CAS PubMed.
- I. S. C. Sulaiman, B. W. Chieng, M. J. Osman, K. K. Ong, J. I. A. Rashid, W. M. Z. W. Yunus and S. A. M. Noor, Microchim. Acta, 2020, 187, 1–22 CrossRef PubMed.
- S. Wu, D. Li, Y. Zhao, S. Dong and X. Wang, Sens. Actuators, B, 2016, 238, 427–433 CrossRef.
- C. C. Chang, C. P. Chen, T. H. Wu, C. H. Yang, C. W. Lin and C. Y. Chen, Nanomaterials, 2019, 9, 1–24 Search PubMed.
- R. Bala, R. K. Sharma and N. Wangoo, Anal. Bioanal. Chem., 2015, 408, 333–338 CrossRef PubMed.
- N. Liang, X. Hu, W. Li, A. W. Mwakosya, Z. Guo, Y. Xu, X. Huang, Z. Li, X. Zhang, X. Zou and J. Shi, Food Chem., 2021, 343, 1–7 CrossRef PubMed.
- G. Wang, Y. Wang, L. Chen and J. Choo, Biosens. Bioelectron., 2010, 25, 1859–1868 CrossRef CAS PubMed.
- S. K. Ghosh and T. Pal, Chem. Rev., 2007, 107, 4797–4862 CrossRef CAS PubMed.
- M. Rex, F. E. Hernandez, A. D. Campiglia, P. O. Box and C. Florida, Anal. Chem., 2006, 78, 445–451 CrossRef CAS PubMed.
- K. Rana, J. R. Bhamore, J. V. Rohit, T. J. Park and S.
K. Kailasa, New J. Chem., 2018, 42, 9080–9090 RSC.
- M. J. Osman, W. M. Z. Wan Yunus, K. K. Ong and J. Abdul Rashid, J. Def. Sci. Eng. Technol., 2019, 2, 49–70 Search PubMed.
- R. Bala, S. Mittal, R. K. Sharma and N. Wangoo, Spectrochim. Acta, Part A, 2018, 196, 268–273 CrossRef CAS PubMed.
- J. Li, Y. Liu, H. Lin, Y. Chen, Z. Liu, X. Zhuang, C. Tian, X. Fu and L. Chen, Food Chem., 2021, 347, 128988 CrossRef CAS PubMed.
- A. Ruscito, M. C. DeRosa, I. Jeddi and L. Saiz, Front. Chem., 2016, 4, 1–14 CAS.
- I. Jeddi and L. Saiz, Sci. Rep., 2017, 7, 1178–1191 CrossRef PubMed.
- J. P. Elskens, J. M. Elskens and A. Madder, Int. J. Mol. Sci., 2020, 21, 4522–4553 CrossRef CAS PubMed.
- M. McKeague and M. C. Derosa, J. Nucleic Acids, 2012, 2012, 1–20 CrossRef PubMed.
- F. Odeh, H. Nsairat, W. Alshaer, M. A. Ismail, E. Esawi, B. Qaqish, A. Al Bawab and S. I. Ismail, Molecules, 2019, 25, 1–51 CrossRef PubMed.
- C. Acquah, Y. W. Chan, S. Pan, L. S. Yon, C. M. Ongkudon, H. Guo and M. K. Danquah, Sci. Rep., 2019, 9, 1–11 CAS.
- H. Hasegawa, N. Savory, K. Abe and K. Ikebukuro, Molecules, 2016, 21, 421–436 CrossRef PubMed.
- P. J. J. Huang, R. Kempaiah and J. Liu, J. Mater. Chem., 2011, 21, 8991–8993 RSC.
- C. K. L. Gordon, M. Eisenstein and H. T. Soh, ACS Sens., 2018, 3, 2574–2580 CrossRef CAS PubMed.
- M. Saberian, D. Asgari, Y. Omidi and H. Hamzeiy, Iran. J. Pharm. Sci., 2012, 8, 119–126 Search PubMed.
- S. Cai, J. Yan, H. Xiong, Y. Liu, D. Peng and Z. Liu, Analyst, 2018, 143, 5317–5338 RSC.
- W. Bai, C. Zhu, J. Liu, M. Yan, S. Yang and A. Chen, Environ. Toxicol. Chem., 2015, 34, 2244–2249 CrossRef CAS PubMed.
- Y. Tian, Y. Wang, Z. Sheng, T. Li and X. Li, Anal. Biochem., 2016, 513, 87–92 CrossRef CAS PubMed.
- G. Yue, S. Su, N. Li, M. Shuai, X. Lai, D. Astruc and P. Zhao, Coord. Chem. Rev., 2016, 311, 75–84 CrossRef CAS.
- R. Bala, S. Dhingra, M. Kumar, K. Bansal, S. Mittal, R. K. Sharma and N. Wangoo, Chem. Eng. J., 2017, 311, 111–116 CrossRef CAS.
- P. Wang, Y. Wan, A. Ali, S. Deng, Y. Su, C. Fan and S. Yang, Sci. China: Chem., 2016, 59, 237–242 CrossRef CAS.
- Q. Fu, C. Zhang, J. Xie, Z. Li, L. Qu, X. Cai, H. Ouyang, Y. Song, D. Du, Y. Lin and Y. Tang, Anal. Chim. Acta, 2019, 1092, 126–131 CrossRef CAS PubMed.
- R. Jin, D. Kong, X. Yan, X. Zhao, H. Li, F. Liu, P. Sun, Y. Lin and G. Lu, ACS Appl. Mater. Interfaces, 2019, 11, 1–10 CrossRef PubMed.
- K. Sankar, D. Lenisha, G. Janaki, J. Juliana, R. S. Kumar, M. C. Selvi and G. Srinivasan, Talanta, 2020, 208, 120408 CrossRef CAS PubMed.
- M. J. Osman, W. M. Z. Yunus Wan, K. K. Ong, B. W. Chieng, N. A. Mohd Kassim, S. A. Mohd Noor, V. F. Knight, J. I. Abdul Rashid and C. C. Teoh, J. Chem., 2020, 2020, 1–10 CrossRef.
- G. Ma, J. Cao, G. Hu, L. Zhu, H. Chen, X. Zhang, J. Liu, J. Ji, Z. Liu and C. Lu, Analyst, 2021, 146(14), 4576–4584 RSC.
- Y. Qu, H. Qian, Y. Mi, J. He, H. Gao, R. Lu, S. Zhang and W. Zhou, Anal. Methods, 2020, 12, 1919–1925 RSC.
- S. H. Hwang, S. Jeong, H. J. Choi, H. Eun, M. G. Jo, W. Y. Kwon, S. Kim, Y. Kim, M. Lee and K. S. Park, J. Nanomater., 2019, 2019, 1–7 CrossRef.
- L. Wang, X. Liu, Q. Zhang, C. Zhang, Y. Liu, K. Tu and J. Tu, Biotechnol. Lett., 2012, 34, 869–874 CrossRef CAS PubMed.
- S. K. Behera, H. Meena, S. Chakraborty and B. C. Meikap, Int. J. Min. Sci. Technol., 2018, 28, 621–629 CrossRef CAS.
- S. Greenland, S. J. Senn, K. J. Rothman, J. B. Carlin, C. Poole, S. N. Goodman and D. G. Altman, Eur. J. Epidemiol., 2016, 31, 337–350 CrossRef PubMed.
- S. Yi, Y. Su, B. Qi, Z. Su and Y. Wan, Sep. Purif. Technol., 2010, 71, 252–262 CrossRef CAS.
- R. E. Kass, B. S. Caffo, M. Davidian, X. L. Meng, B. Yu and N. Reid, PLoS Comput. Biol., 2016, 12, 1–8 CrossRef PubMed.
- N. Mansouriieh, M. R. Sohrabi and M. Khosravi, Arabian J. Chem., 2019, 12, 2524–2532 CrossRef CAS.
- R. Davarnejad, M. Mohammadi and A. Fauzi, J. Water Process. Eng., 2014, 3, 18–25 CrossRef.
- M. Moradi, F. Ghanbari, M. Manshouri and K. A. Angali, Korean J. Chem. Eng., 2015, 32, 1–8 CrossRef.
- H. Amiri, R. Nabizadeh, S. Silva Martinez, S. Jamaleddin Shahtaheri, K. Yaghmaeian, A. Badiei, S. Nazmara and K. Naddafi, Ecotoxicol. Environ. Saf., 2018, 147, 919–925 CrossRef CAS PubMed.
- Q. Shao, Y. Deng, H. shen, H. Fang and X. Zhao, Int. J. Biol. Macromol., 2011, 49, 958–962 CrossRef CAS PubMed.
- T. Hianik, V. Ostatná, M. Sonlajtnerova and I. Grman, Bioelectrochemistry, 2007, 70, 127–133 CrossRef CAS PubMed.
- L. Li, Y. Jiang, C. Cui, Y. Yang, P. Zhang, K. Stewart, X. Pan, X. Li, L. Yang, L. Qiu and W. Tan, J. Am. Chem. Soc., 2018, 140, 13335–13339 CrossRef CAS PubMed.
- V. S. R. Aaryasomayajula, J. Nanomed. Nanotechnol., 2014, 5, 210–216 Search PubMed.
- Y. Peng, L. Li, X. Mu and L. Guo, Sens. Actuators, B, 2013, 177, 818–825 CrossRef CAS.
- J. E. Smith, J. L. Chávez, J. A. Hagen and N. Kelley-Loughnane, J. Visualized Exp., 2016, 2016, 1–10 Search PubMed.
- J. Deka, R. Měch, L. Ianeselli, H. Amenitsch, F. Cacho-Nerin, P. Parisse and L. Casalis, ACS Appl. Mater. Interfaces, 2015, 7, 7033–7040 CrossRef CAS PubMed.
- D. Bhattacharyya, G. M. Arachchilage and S. Basu, Front. Chem., 2016, 4, 1–14 Search PubMed.
- E. Zavyalova, G. Tagiltsev, R. Reshetnikov, A. Arutyunyan and A. Kopylov, Nucleic Acid Ther., 2016, 26, 299–308 CrossRef CAS PubMed.
- A. Rafati, A. Zarrabi, S. Abediankenari, M. Aarabi and P. Gill, R. Soc. Open Sci., 2018, 5, 171835–171845 CrossRef CAS PubMed.
- M. Belleperche and M. C. DeRosa, Pharmaceuticals, 2018, 11, 80–93 CrossRef CAS PubMed.
- S. Hongsibsong, T. Prapamontol, T. Xu, B. D. Hammock, H. Wang, Z.-J. Chen and Z.-L. Xu, Int. J. Environ. Res. Public Health, 2020, 17, 4723–4737 CrossRef CAS PubMed.
- Y. Gao, M. G. Ierapetritou and F. J. Muzzio, J. Pharm. Innov., 2013, 8, 72–82 CrossRef.
- C. Cozma, S. Eichler, G. Wittmann, A. F. Bonet, G. J. Kramp, A.-K. Giese and A. Rolfs, PLoS One, 2015, 10, 1–14 CrossRef CAS PubMed.
- R. Bala, M. Kumar, K. Bansal, R. K. Sharma and N. Wangoo, Biosens. Bioelectron., 2016, 85, 445–449 CrossRef CAS PubMed.
- D. J. Javier, N. Nitin, M. Levy, A. Ellington and R. Richards-Kortum, Bioconjugate Chem., 2008, 19, 1309–1312 CrossRef CAS PubMed.
- B. Mondal, S. Ramlal, P. S. Lavu, N. Bhavanashri and J. Kingston, Front. Microbiol., 2018, 9, 1–8 CrossRef PubMed.
- R. Bala, S. Mittal, R. K. Sharma and N. Wangoo, Spectrochim. Acta, Part A, 2018, 196, 268–273 CrossRef CAS PubMed.
Footnote |
† Electronic supplementary information (ESI) available. See DOI: 10.1039/d1ra04318h |
|
This journal is © The Royal Society of Chemistry 2021 |
Click here to see how this site uses Cookies. View our privacy policy here.