DOI:
10.1039/D1RA03481B
(Paper)
RSC Adv., 2021,
11, 25788-25794
Detection of SARS-CoV-2 and its S and N proteins using surface enhanced Raman spectroscopy†
Received
4th May 2021
, Accepted 2nd July 2021
First published on 26th July 2021
Abstract
The COVID-19 pandemic demonstrated the critical need for accurate and rapid testing for virus detection. This need has generated a high number of new testing methods aimed at replacing RT-PCR, which is the golden standard for testing. Most of the testing techniques are based on biochemistry methods and require chemicals that are often expensive and the supply might become scarce in a large crisis. In the present paper we suggest the use of methods based on physics that leverage novel nanomaterials. We demonstrate that using Surface Enhanced Raman Spectroscopy (SERS) of virion particles a very distinct spectroscopic signature of the SARS-CoV-2 virus can be obtained. We demonstrate that the spectra are mainly composed by signals from the spike (S) and nucleocapsid (N) proteins. It is believed that a clinical test using SERS can be developed. The test will be fast, inexpensive, and reliable. It is also clear that SERS can be used for analysis of structural changes on the S and N proteins. This will be an example of application of nanotechnology and properties of nanoparticles for health and social related matters.
Introduction
The number of cases of COVID-19 and its associated mortality have raised serious concerns worldwide. Early diagnosis of viral infection allows rapid intervention, disease management, and substantial control of the rapid spread of the disease.1 The most common test used to detect the virus is the real time reverse transcriptase-polymerase chain reaction RT-qPCR utilizing nasal or pharyngeal swabs or saliva.2 This technique detects viral RNA regions, and it is highly sensitive and very specific. However, it has a relatively long turnaround time, requires expensive equipment, and involves highly trained scientists. Additionally, this technique requires the use of DNA-binding dyes, which sometimes are difficult to obtain.
Recent improvements have cut down the response time significantly and there has been a very rapid advance in new testing methods. Developments include immunological methods, such as Enzyme Linked Immunosorbent Assays (ELISAs), chemiluminescence immunoassays (CLIAs), lateral flow immunoassays (LFIAs), and multiple others.3–7 Recently, the development of clustered regularly interspaced short palindromic repeats (CRISPR)-based diagnostics has been used to detect the SARS-CoV-2 virus.8,9 This method is very promising but is still in an early stage. Several other methods based on nanotechnology and 2-D materials have also been reported.10–14 A comprehensive review of the detection methods can be found in ref. 15–17. A general conclusion is that the methods, other than RT-PCR detection assays, have some limitations including low detection sensitivity and, thus, have not replaced RT-PCR as the gold standard.
In this paper we explore the development of a new test, which, if successful, can be broadly implemented with higher sensitivity and reliability. Whereas most of the currently available testing is based on biochemical methods, we present the possibility of expanding our capabilities using the advances in techniques based on physics and, more specifically, the properties of condensed matter and its interactions with light.
Raman spectroscopy is a well-established technique for the study of inorganic and organic materials.18–21 Raman spectroscopy has been used for the detection of bacteria in food22 in the detection of bacterial infections23–25 and HIV-1 virus detection.26–29 Recently it has been applied to the study of SARS-CoVid-2 using gold nanoparticles30 and conventional Raman spectroscopy.31 The Raman technique uses the inelastic fraction of light interacting with a molecule, that due to this interaction, the wavelength of the light is shifted, called Raman shift, which is directly related to the vibrational modes of the molecules analysed and therefore represents a fingerprint of the molecule. Raman spectroscopy is intrinsically highly specific to chemicals compared to other spectroscopies, such as fluorescence or visible/near-infrared (NIR) absorption, and hence offers many advantages.
The Raman signal intensity is very low, about 10−6 times the initial laser light intensity and, thus, a signal amplification is required. This can be achieved using the plasmonic effects of metallic nanoparticles. Depending on the shape (aspect ratio) or chemical composition, the plasmonic field enhances the signal up to ∼108–109 times.32,33 In addition, other materials such as transition metal dichalcogenides and other 2-D materials34,35 can produce an additional signal enhancement of ∼102 due to electronic transfer. This method is known as Surface Enhanced Raman Spectroscopy (SERS) and can be a powerful method to detect viruses and other pathogens.36 Raman based detection is expected to have high sensitivity and specificity as demonstrated by the detection of breast cancer using SERS.37,38
In this paper we report preliminary studies of SARS-CoV-2 virion particles and its spike (S) and nucleocapsid proteins (N). We developed a highly sensitive SERS substrate based on plasmonic and excitonic phenomena which allows a very complete characterization of the virion SARS-CoV-2 and its S and N proteins. We show that SERS can provide a solid platform for viral testing.
Experimental
SARS-CoV-2 proteins and virions
Purified SARS-CoV-2 spike (S) protein expressed in baculovirus insect cells was purchased from Sino Biological (40589-V08B1). This in vitro expressed protein, containing the S1 and S2 subunits, was purified by nickel chromatography using affinity his-6 tag and which is capable of binding to the ACE2 receptor. Similarly, we cloned the SARS-CoV-2 nucleocapsid (N) protein into an E. coli expression vector and then purified it using its his-6 tag and Ni chromatography. SARS-CoV-2 WA1 and HCoV-NL63 (BEI Resources) virions were grown on VeroE6 (SARS-CoV-2) or LLC-MK2 (HCoV-NL63) cells. Overlying media, containing virus, was harvested, and tittered to determine the number of infective particles prior to Raman analysis. Ethanol inactivation (70%) of the virus was performed prior to the Raman analysis. To examine the samples, we used Au/Cu nanostars prepared using the methods reported previously by José-Yacamán and colleagues.39 Those authors report the controlled selective growth and high-yield production of AurichCu concave pentagonal nanoparticles (CPNPs) and their evolution into branched nanostructures, concave “nanostars” (CNSs), via a seed-mediated growth method by using oleylamine (OLA) as a capping and self-assembly promoter agent. The adding of Cu is necessary to produce 5-arm structures (see Experimental section in ESI Fig. S1(a–c)†) with concave facets and sharp tip surface charge distributions showed significant induced polarization along the arms of the CNSs, resulting in a highly localized plasmon field at the tips (785 nm), as well as a surface polarization due to the formation of image charges. Additionally, the Au–Cu nanostars have concave facets which also enhance the signal by non-plasmonic mechanisms as discussed by Lee and co-workers.40 Therefore, by controlling the shape, we can obtain enhanced plasmon fields and make them ideal for SERS of proteins. An image of the Au–Cu nanostars is shown in Fig. 1. It has been demonstrated that every arm of the nanostars acts as an antenna for the enhancement of the Raman signal. The length and width of the nanostars arms were modified until a light absorption peak around 800 nm was obtained on the UV-Vis spectra, indicating that the nanoparticles were responding in the 785 nm wavelength of the laser excitation source.
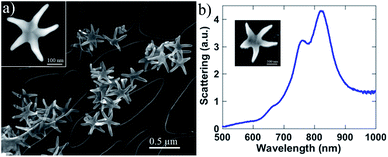 |
| Fig. 1 (a) SEM image of the Au–Cu nanostars used as SERS substrate. The image shows very sharp peaks which act as plasmonic antennas. (b) Optical absorption spectra of the nanostars revealed a strong peak on the near IR. | |
The SERS amplification requires that the plasmonic nanoparticles be in contact with the analyte. The electric field polarization along the nanostar arms promotes that they attach to proteins or virions. However, it is necessary to promote the attachment by agitation of the nanoparticles–protein solution. The Au–Cu nanostars were mixed with the buffer used on the virion particles or the S and N proteins. The particle concentration on the solution was 1.0% weight. The mixed solution was centrifugated/shaken for several minutes to increase the attachment of the nanoparticles to the proteins. We also used a MoS2 of few monolayers thickness on a microscope slide, the solution of nanoparticles with proteins was dropped on the substrate. The Raman spectra were obtained using a Horiba XploRA Plus 100 spectrometer 785 nm laser – 200 mW power (100% filter) – 20 μm laser beam diameter, with 5 s acquisition time and 5 spectral accumulations.
Results and discussion
Spectra of SARS-CoV-2 virion particles and the S and N proteins
In Fig. 2 we show the typical Raman-SERS spectra of inactivated SARS-CoV-2 virion particles and the purified S and N proteins. Those spectra are the result of averaging 30–50 different spectra. The number of averaging spectra varies according to the surface of the sample area, and it is difficult to keep a constant number of spectra through all the analysis for all the samples, however we have kept a higher number of spectrums for reproducibility analysis purposes. The fluorescence contribution and noise were eliminated using LabSpec software from Horiba. In the case of the virion spectra, several peaks that were not present in all spectra were eliminated. Most of those peaks appear in only one spectrum so we consider them not being representative. We attribute those peaks to the inactivation procedure. In general, the spectra of the SARS-CoV-2 virus and the S and N proteins demonstrated broad peaks, which are the result of many overlapping smaller peaks related to several molecular vibrations. To obtain an accurate analysis of the virus and proteins it is necessary to deconvolute the peaks into individual components. This can be demonstrated by simulation of the Raman-SERS spectra. Vibrational frequencies simulations were calculated using the Gaussian code based on the Hartree–Fock approximation41 for an unrestricted isolated molecule, which is part of the N protein to obtain the Raman spectra. The N and S protein contain a very large number of diverse molecules and, thus, its full structure will be very difficult to simulate. For instance, a protein containing 25
000 atoms will be prohibitively expensive in computer time (3D structures of the N, S proteins, and the virion, as well as the region of the calculations can be found in ESI Fig. S2–S4†).
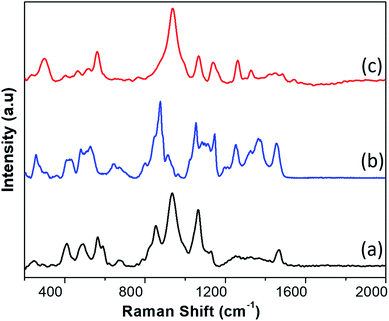 |
| Fig. 2 SERS spectra of (a) the N protein, (b) the S protein, and (c) the inactivated SARS-CoV-2 virion particles. | |
Therefore, we only calculated the spectra of some components of the N protein. We limit our calculation to two partial molecules histidine and tryptophan. Calculations were made using the Gaussian program, and Raman frequencies were resolved by using the default solvent model using water as the solvent medium. In the present work we only considered two amino acids. The results are shown in Fig. 3, which shows the spectra of 10 atoms of the molecule histidine and 50 atoms of the molecule tryptophan. The resulting peaks (around 1000 cm−1) are compared to the SARS-CoV-2 virus. As can be seen, there are many peaks that overlap and fall on the observed positions for the SARS-CoV-2 spectra. All peaks for the different molecules represented in the spectra overlap, resulting in a large broad peak which is the important result of the calculation. The virion particle is even more complex because contains the S and N proteins and many other minority components. Thus, to analyse the Raman spectra of the virion particles more accurately, we need to deconvolute the peaks. The deconvolution process was performed using the Fityk program version 1.3,42 which offers an alternative of bell-shaped functions to fit the Raman bands. The choice of fitting function does not alter the wavenumber position of a given band but results in slightly different full widths at half maximum (FWHM), or line broadenings. Before the fitting was performed, careful consideration must be paid to determine as best as possible the base line of the Raman spectra. To do this, the fluorescence background was determined by fitting a fifth-degree polynomial curve to the measured spectrum from the points where no Raman signal is expected to be present, and this is subtracted from the original data. Judging from the results, only reliable identifications may be assigned to the spectral details above 400 cm−1. The fit was covered to include all spectral details below 1800 cm−1 as all spectral details, originating of the side-chains of the amino-acids, as well as from the peptide bonds (amide fingerprints) constitutive of the virion proteins are expected to be found in this spectral region. The red line illustrates the result of the fits using Lorentzian functions to deconvolute the presence of the contributing Raman bands to the spectra. The result of the fitting is presented in Tables 1–3 for the SARS-CoV-2 virion, and the S and N proteins, respectively. Fig. 4 shows an example of the peak deconvolution for the N protein and illustrates its necessity (additional deconvolution data is shown in Fig. S5(a–c)† for the N, S protein and virion Raman spectra (NL-63), respectively).
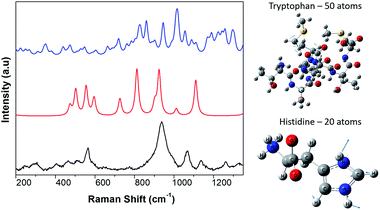 |
| Fig. 3 Theoretical simulation of portions of the Raman spectra of components of the protein N. We use a portion of 50 atoms from the tryptophan molecule (blue), a portion of 10 atoms of the histidine molecule (red), and a portion of the experimental spectra of the SARS-CoV-2 virion particle (black). The overlap of peaks close to the 1000 cm−1 Raman shift can be observed. | |
Table 1 Raman shifts for the SARS-CoV-2 virion particle
Raman shift (cm−1) |
Assignment |
501.5 |
S–S bond (β-sheet) |
531.65 |
S–S bond (α-helix) |
577.0 |
Trp, Cys |
656.2 |
Tyr (α-helix) |
716.7 |
Phospholipids |
782.5 |
Histidine |
846.5 |
Tyr doublet |
902.3 |
C–O–C skeletal modes |
951.5 |
Trp |
983.7 |
Trp–Val |
1060.3 |
Phe |
1121 |
Trp, Phe |
1150 |
C–N, glycogen |
1207 |
Phe, Tyr |
1263 |
Amide III |
1297.4 |
Amide III |
1340.4 |
Trp, Cα–H deformation |
1378 |
L-Alanine, N-acetyl-glucosamine |
1445.1 |
C–H deformation |
1492.1 |
L-Histidine |
1554.0 |
Indole ring Trp |
1600.7 |
Tyr, Phe, Trp |
Table 2 Raman shifts for the spike (S) protein
Raman shift (cm−1) |
Assignation |
Indicates the peaks are also present on the Covid spectrum. |
410.5 |
Tryptophan or histidine |
432 |
L-Tyr |
442.6 |
Glucose |
478.0 |
N-Acetyl-glucosamine |
498.85 |
S–S bond (α/β) |
510.96a |
Phosphorylated protein and lipids |
528.7 |
S–S bond (α-helix) |
545.5 |
S–S bond (β-sheet) |
601.0 |
Phe |
633.8 |
Trp |
651.7 |
Tyr (α-helix) |
678.44 |
Trp |
716.5a |
Phospholipids |
786.04 |
Histidine |
801.1 |
Trp |
849.0 |
Tyr doublet |
875.8 |
Tryptophan |
891.9a |
Mono and disaccharides C–O–C skeletal modes |
920.3a |
Glucose/glycogen |
1033.1 |
Phe |
1050.2a |
C–N and C–C protein stretching |
1081.0 |
Histidine |
1100.2 |
Trp, galactosamine |
1115.6 |
ν-C–C aliphitic |
1128.0a |
Trp, Phe |
1150.2a |
Glycogen |
1220.1 |
Phe |
1250.7a |
Amide III |
1270.7 |
α-Helix amide III |
1305.9 |
Phe |
1320.0a |
Amide III (α-helix structures) |
1356.7 |
Trp, Cα–H deformation |
1383.9 |
C–H rocking in lipids |
1449.8a |
Fatty acids, C–H stretching of glycoproteins |
1463.7 |
C–H deformation |
Table 3 Raman shifts for the nucleocapsid (N) protein
Raman shift (cm−1) |
Assignation |
Indicates the peaks are also present on the SARS-CoV-2 Raman-SERS spectrum. |
373.8 |
δ C–C aliphatic chains |
419.6 |
Tryptophan or histidine |
495.2 |
S–S bond (β-sheet) |
602.1 |
Phe |
654.5 |
Tyr (α-helix) |
678.4 |
Trp |
729.77 |
ν-C–C aliphatic chains |
783.46 |
Histidine |
823.8 |
Tyr |
852.4 |
Tyr doublet |
862 |
β-Sheet Tyr |
922.3 |
Val–N–Cα–C |
975.8 |
Trp, Val |
1050a |
C–N and C–C stretching |
1076.7 |
ν-C–C aliphatic chains |
1107.8 |
Histidine, Trp |
1219.3 |
Phe, ν-C–C aliphatic chains |
1248.6a |
Secondary amide bands |
1266.9 |
Amide III |
1320.65a |
Amide III (α-helix structures) |
1390.0a |
C–H rocking in lipids |
1458.1a |
General fatty acids, C–H stretching of glycoproteins |
1517.4 |
Galactosamine, aromatic rings |
1554.9 |
Indole ring Trp |
1608.0 |
Trp, Tyr, Phe |
1671.7 |
Amide I |
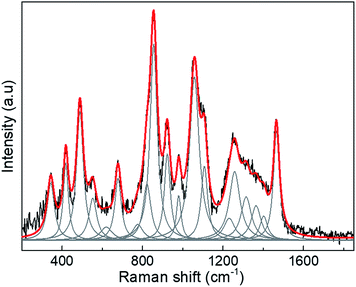 |
| Fig. 4 Example of the deconvolution (red line) of the SERS peaks for the N protein. | |
To obtain the peaks reported in Tables 1–3, we deconvolute 50 spectra of each type and calculated the average. Our results produce a significant number of peaks that can be used as a very complete fingerprint for identification. We found that 15 of the observed peaks on the virion particle correspond to the S and N proteins. This the result of the virion particle structure. As summarized on the ESI† the SARS-CoV-2 virion particles contain several other proteins such as the membrane and envelop proteins plus RNA. The membrane protein has a concentration three times more than the N protein. In addition, the virion particles S protein might have combined with some compounds. So, we do not expect the full virion particle have only peaks of S and N proteins.
Raman spectra of inactivated HCoV-NL63 virion particles
To demonstrate the capability of the Raman method for virus testing, we studied the human coronavirus HCoV-NL63. This virus causes mostly upper and at times lower respiratory tract infections, community acquired pneumonia, and croup in children.43 This virus can be considered as a surrogate of the SARS-CoV-2 virus. Fig. 5 shows a typical Raman-SERS spectrum of the HCoV-NL63 virus. We followed the same fitting procedure described above and the peaks are identified in Table 4.
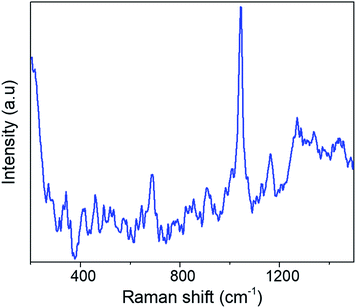 |
| Fig. 5 SERS spectra of inactivated CoV-NL63 virions. | |
Table 4 Raman shifts of the HCoV-NL63 virion particle
Raman shift (cm−1) |
Assignation |
557.92 |
S–S bond (β-sheet) |
626.86 |
Try |
644.00 |
Tyr (α-helix) |
656.50 |
Try (β-sheet) |
684.82 |
ν-C–C aliphatic chains |
740.93 |
Try (β-sheet) |
759.64 |
Trp (β-sheet) |
784.18 |
Histidine |
825.18 |
Tyr (α-helix) |
854.34 |
Tyr (β-sheet) |
920.42 |
Glucose/glycogen |
962.20 |
Trp, Val (α-helix) |
1005.04 |
Phe ring modes |
1026.20 |
Phe |
1071.08 |
ν-C–C aliphatic chains |
1101.00 |
Trp, Val |
1129.43 |
Phe, Trp |
1156.12 |
C–N, glycogen |
1198.06 |
ν-C–C aliphatic chains, Tyr |
1231.14 |
Amide III (β-sheet) |
1268.94 |
Amide III (α-helix) |
1313.23 |
Trp, Cα–H deformation |
1323.71 |
Amide III (α-helix structures) |
1340.92 |
α/β Trp, Cα–H deformation |
1368.81 |
N-Acetyl-glucosamine |
1395.94 |
C–H rocking in lipids |
1446.42 |
General fatty acids, C–H stretching of glycoproteins |
1454.92 |
General fatty acids, C–H stretching of glycoproteins |
1520.56 |
Galactosamine, aromatic rings |
1549.18 |
Indole ring Trp |
Discussion
A protein Raman spectrum is typically composed of contributions from three major types of vibrational modes originating from the polypeptide backbone (amide bands) and aromatic and non-aromatic amino acid side chain residues. The Raman-SERS spectra of virion particles is complex and produces broad peaks that are the result of the many closely spaced peaks resulting from multiple chemical species.44 A similar example is the spectra of HIV-1 virus reported by Lee et al.45 A very interesting fact is that the virion spectra and the S protein are lacking peaks corresponding to the amide I bands (1640–1678 cm−1). This has been a common observation.36 This has been explained by Kuroski et al.46 with SERS studies of different homo-peptides consisting of Gly-, Ala-, Tyr- and Trp-rich chains under a variety of experimental conditions. There, it was demonstrated that the absence of amide I bands in the spectra depended on the size of the amino acid side chain. The side chain increases the distance between the peptide bond and the metal nanoparticle preventing their immediate contact. However, it is also possible the lack of the amide peak on the virion might also be related to the virus a deactivation procedure which might break amino acids. Recently Carlomagno et al.31 reported Raman studies (not using SERS) of SARS-CoV-2 in saliva samples they also do not report the amide I peaks. In other recent paper Zhang et al.47 used silver-nanorods SERS array functionalized with binding protein and the viral receptor of the cellular receptor angiotensin-converting enzyme 2 (ACE2). There, they reported a very weak amide I peak, which is very close to the noise. These authors used a Raman peak of 1189 cm−1 in the ACE-2 with a shift to 1182 cm−1 when the spike protein was attached, and then used the ratio of those two peaks to predict the virus presence; their SARS-CoV-2 spectra showed very few weak peaks. Another study, Desai et al.,48 reported Raman study of an RNA virus in saliva, using a lentivirus vector system (based on HIV-1) and their reported Raman spectra also showed only a few peaks and no amide I peak. However, the N protein shows the amide I peak. Our N protein was complete and purified whereas the S protein was commercially available and broken in two subunits S1 and S2. This further suggest the invisibility of the amide I peak is related to the size of the amino acid chains.
On the other hand, it is well known that SERS amplification effect requires that the molecule to be in contact with the metal nanoparticle. Our approach using a SERS with a substrate that combines plasmonic and excitonic amplification and the mathematical analysis allow us to obtain a large number of peaks. In the case of the virion particles we have obtained very consistent spectrum. At least 15 of those peaks can be associated with the presence of N and S proteins. Some of the variations on the peaks that are observed can be attributed to the virion particles being deactivated. It is likely that during the process of deactivation some parts of the virus were destroyed. To reinforce the valuable information that could be extracted from the SERS spectra it was calculated the limit of detection for a calibration curve for the S protein. The SERS spectra of the InBios-Spike-Protein was collected for concentrations ranging from 744 μM to 7 nM. Then, using PLSR (Partial Least Squares Regression) with two principal components to the calibration curve the variance for the two components was calculated to be 99.9%. Thus, a detection limit was found to be 8.89 × 10−9 M with a Root Mean Square Error (RMSE) ≈2.27 × 10−9, which is a good approximation for concentrations as low as nM range (see ESI Fig. S6(a-c)†).
Conclusions
We have shown that using SERS produced by gold nanostars nanoparticles and MoS2 thin layers we can obtain a very consistent signature for SARS-CoV-2 virion particles. In addition, the main proteins, S and N, produce well-defined spectra. This suggests that Raman-SERS spectroscopy can be used not only for virus detection but also to study the structure of the proteins and follow in situ changes produced by different conditions.
Our study is preliminary, clinical testing and a direct comparison to other tests will be necessary to assess the sensitivity and specificity of Raman. Nevertheless, our research shows great promise for developing the technique. If developed, a Raman-SERS test on saliva will produce instant results and will only require the use of nanoparticles, which can be massively fabricated at low cost.
Author contributions
MJY, PK: conceptualization, investigation, funding acquisition, project administration, data curation, formal analysis, writing original draft, supervision. ES, SJ, MH, MR, JVS, JES: investigation, methodology, resources, validation. JES, AL, JG, CRA, HRNC: data curation, data analysis, formal analysis. DMW, AK, RK: writing review and editing.
Conflicts of interest
The authors have no conflicts to declare.
Acknowledgements
The authors would like to acknowledge the financial support from the NSF Rapid Grant # 2030488. We also acknowledge the NSF-NCI-SW center for financial support in using ASU cores and the MIRA-NAU Institute for grant on metallic nanoparticles. We acknowledge the access to Laboratorio Nacional de Análisis Físicos, Químicos y Biológicos-UASLP (LANAFQB), during the course of this research. This project benefited from the financial support granted by Consejo Nacional de Ciencia y Tecnología (CONACyT) México for Mantenimiento de Infraestructura Científica en Laboratorios Nacionales 2020-314931, as well as from FAI-UASLP. Flinn Foundation and NAU TRIF funds were used to support the virus work.
References
- M. Jayaweera, H. Perera, B. Gunawardana and J. Manatunge, Environ. Res., 2020, 188, 109819, DOI:10.1016/j.envres.2020.109819.
- L. Dong, J. Zhou, C. Niu, Q. Wang, Y. Pan, S. Sheng, X. Wang, Y. Zhang, J. Yang, M. Liu, Y. Zhao, X. Zhang, T. Zhu, T. Peng, J. Xie, Y. Gao, D. Wang, X. Dai and X. Fang, Talanta, 2021, 224, 121726, DOI:10.1016/j.talanta.2020.121726.
- J. A. Lieberman, G. Pepper, S. N. Naccache, M.-L. Huang, K. R. Jerome and A. L. Greninger, J. Clin. Microbiol., 2020, 58(8), e00821, DOI:10.1128/JCM.00821-20.
- N. Veyrenche, K. Bolloré, A. Pisoni, A. S. Bedin, A. M. Mondain, J. Ducos, M. Segondy, B. Montes, P. Pastor, D. Morquin, A. Makinson, V. Le Moing, P. Van de Perre, V. Foulongne and E. Tuaillon, J. Med. Virol., 2021, 93(5), 3069–3076, DOI:10.1002/jmv.26855.
- C. Zhang, T. Zheng, H. Wang, W. Chen, X. Huang, J. Liang, L. Qiu, D. Han and W. Tan, Anal. Chem., 2021, 93(7), 3325–3330, DOI:10.1021/acs.analchem.0c05059.
- K. Taki, I. Yokota, T. Fukumoto, S. Iwasaki, S. Fujisawa, M. Takahashi, S. Negishi, K. Hayasaka, K. Sato, S. Oguri, M. Nishida, J. Sugita, S. Konno, T. Saito and T. Teshima, J. Infect. Chemother., 2021, 27(2), 410–412, DOI:10.1016/j.jiac.2020.10.029.
- K. Zwirglmaier, M. Weyh, C. Krüger, R. Ehmann, K. Müller, R. Wölfel and K. Stoecker, J. Virol. Methods, 2021, 290, 114083, DOI:10.1016/j.jviromet.2021.114083.
- P. Nimsamer, O. Mayuramart, S. Rattanaburi, N. Chantaravisoot, S. Saengchoowong, J. Puenpa, Y. Poovorawan and S. Payungporn, J. Virol. Methods, 2021, 290, 114092, DOI:10.1016/j.jviromet.2021.114092.
- H. Rahimi, M. Salehiabar, M. Barsbay, M. Ghaffarlou, T. Kavetskyy, A. Sharafi, S. Davaran, S. C. Chauhan, H. Danafar, S. Kaboli, H. Nosrati, M. M. Yallapu and J. Conde, CRISPR, ACS Sens., 2021, 27, 0c02312, DOI:10.1021/acssensors.0c02312.
- M. Alafeef, K. Dighe, P. Moitra and D. Pan, ACS Nano, 2020, 14(12), 17028–17045, DOI:10.1021/acsnano.0c06392.
- I. S. Donskyi, C. Nie, K. Ludwig, J. Trimpert, R. Ahmed, E. Quaas, K. Achazi, J. Radnik, M. Adeli, R. Haag and K. Osterrieder, Small, 2021, 17(11), e2007091, DOI:10.1002/smll.202007091.
- B. Mojsoska, S. Larsen, D. A. Olsen, J. S. Madsen, I. Brandslund and F. A. Alatraktchi, Sensors, 2021, 21(2), 390, DOI:10.3390/s21020390.
- M. Srivastava, N. Srivastava, P. K. Mishra and B. D. Malhotra, Sci. Total Environ., 2021, 754, 142363, DOI:10.1016/j.scitotenv.2020.142363.
- H. Zhao, F. Liu, W. Xie, T. C. Zhou, J. OuYang, L. Jin, H. Li, C. Y. Zhao, L. Zhang, J. Wei, Y. P. Zhang and C. P. Li, Sens. Actuators, B, 2021, 327, 128899, DOI:10.1016/j.snb.2020.128899.
- M. Datta, D. Deepak Singh and A. R. Naqvi, Int. Rev. Immunol., 2021, 40, 143–156, DOI:10.1080/08830185.2020.1871477.
- P. Rai, B. K. Kumar, V. K. Deekshit, I. Karunasagar and I. Karunasagar, Appl. Microbiol. Biotechnol., 2021, 105(2), 441–455, DOI:10.1007/s00253-020-11061-5.
- M. Yüce, E. Filiztekin and K. G. Özkaya, Biosens. Bioelectron., 2021, 15, 112752, DOI:10.1016/j.bios.2020.112752.
- P. Gao, B. Han, Y. Du, G. Zhao, Z. Yu, W. Xu, C. Zheng and Z. Fan, J. Spectrosc., 2017, 5 DOI:10.1155/2017/5383948.
- H. Ma, X. X. Han and B. Zhao, TrAC, Trends Anal. Chem., 2020, 131, 116019, DOI:10.1016/j.trac.2020.116019.
- A. V. Vlasov, N. L. Maliar, S. V. Bazhenov, E. I. Nikelshparg, N. A. Brazhe, A. D. Vlasova, S. D. Osipov, V. V. Sudarev, Y. L. Ryzhykau, A. O. Bogorodskiy, E. V. Zinovev, A. V. Rogachev, I. V. Manukhov, V. I. Borshchevskiy, A. I. Kuklin, J. Pokorný, O. Sosnovtseva, G. V. Maksimov and V. I. Gordeliy, Crystals, 2020, 10(1) DOI:10.3390/cryst10010038.
- R. Tuma, J. Raman Spectrosc., 2005, 36, 307–319, DOI:10.1002/jrs.1323.
- Y. Guo, M. Girmatsion, H.-W. Li, Y. Xie, W. Yao, Q. He, B. Abraha and A. Mahmud, Crit. Rev. Food Sci. Nutr., 2020, 15, 10, DOI:10.1080/10408398.2020.1803197.
- D. Nicoleta Elena, C. Alia, S.-N. Tiberiu and P. A. Sebastian, Crit. Rev. Anal. Chem., 2017, 47(6), 499–512, DOI:10.1080/10408347.2017.1332974.
- M. Prochazka, Bioanalytical, Biomolecular and Medical Applications, 2016, pp. 127–148 Search PubMed.
- W. E. Huang, M. Li, R. M. Jarvis, R. Goodacre and S. A. Banwart, Advances in Applied Microbiology, Academic Press, 2010, vol. 70, pp. 153–186, DOI:10.1016/S0065-2164(10)70005-8.
- J. H. Lee, B. K. Oh and J. W. Choi, Sensors, 2015, 15(5), 9915–9927, DOI:10.3390/s150509915.
- L. Farzin, M. Shamsipur, L. Samandari and S. Sheibani, HIV biosensors for early diagnosis of infection: the intertwine of nanotechnology with sensing strategies, Talanta, 2020, 206, 120201, DOI:10.1016/j.talanta.2019.120201.
- Z. Li, L. Leustean, F. Inci, M. Zheng, U. Demirci and S. Wang, Biotechnol. Adv., 2019, 37(8), 107440, DOI:10.1016/j.biotechadv.2019.107440.
- S. Yadav, S. Senapati, D. Desai, S. Gahlaut, S. Kulkarni and J. P. Singh, Colloids Surf., B, 2021, 198, 111477, DOI:10.1016/j.colsurfb.2020.111477.
- T. A. Tabish, R. J. Narayan and M. Edirisinghe, MRS Commun., 2020, 10(4), 566–572, DOI:10.1557/mrc.2020.81.
- C. Carlomagno, D. Bertazioli, A. Gualerzi, S. Picciolini, P. I. Banfi, A. Lax, E. Messina, J. Navarro, L. Bianchi, A. Caronni, F. Marenco, S. Monteleone, C. Arienti and M. Bedoni, Sci. Rep., 2021, 11(1), 4943 CrossRef CAS PubMed.
- J. Reguera, J. Langer, D. Jiménez de Aberasturi and L. M. Liz-Marzán, Chem. Soc. Rev., 2017, 46, 3866, 10.1039/C7CS00158D.
- J. Lee, K. Takemura and E. Y. Park, Sensors, 2017, 17(10), 2332, DOI:10.3390/s17102332.
- A. Pramanik, Y. Gao, K. Gates, S. Begum and P. Chandra Ray, ACS Omega, 2019, 4(6), 11112–11118, DOI:10.1021/acsomega.9b00866.
- X. Zhou, D. Wu, Z. Jin, X. Song, X. Wang and S. Suib, J. Mater. Sci., 2020, 55(34), 16374–16384 CrossRef CAS.
- J. H. Lee, B. K. Oh and J. W. Choi, Sensors, 2015, 15(5), 9915, DOI:10.3390/s150509915.
- A. Catalina Hernández-Arteaga, J. de Jesús Zermeño-Nava, M. Ulises Martínez-Martínez, A. Hernández-Cedillo, H. Joazet Ojeda-Galván, M. José-Yacamán and H. Ricardo Navarro-Contreras, Arch. Med. Res., 2019, 50, 105–110, DOI:10.1016/j.arcmed.2019.05.013.
- A. Hernández-Arteaga, J. de Jesús Zermeño Nava, E. Samuel Kolosovas-Machuca, J. Jesús Velázquez-Salazar, E. Vinogradova, M. José-Yacamán and H. R. Navarro-Contreras, Nano Res., 2017, 10, 3662–3670 CrossRef.
- J. Jesús Velázquez-Salazar, L. Bazán-Díaz, Q. Zhang, R. Mendoza-Cruz, L. Montaño-Priede, G. Guisbiers, N. Large, S. Link and M. José-Yacamán, ACS Nano, 2019, 13(9), 10113–10128, DOI:10.1021/acsnano.9b03084.
- J. H. Lee, B. K. Oh and J. W. Choi, Sensors, 2015, 15(5), 9915–9927, DOI:10.3390/s150509915.
- M. J. Frisch, G. W. Trucks, H. B. Schlegel, G. E. Scuseria, M. A. Robb, J. R. Cheeseman, J. A. Montgomery Jr, T. Vreven, K. N. Kudin, J. C. Burant, J. M. Millam, S. S. Iyengar, J. Tomasi, V. Barone, B. Mennucci, M. Cossi, G. Scalmani, N. Rega, G. A. Petersson, H. Nakatsuji, M. Hada, M. Ehara, K. Toyota, R. Fukuda, J. Hasegawa, M. Ishida, T. Nakajima, Y. Honda, O. Kitao, H. Nakai, M. Klene, X. Li, J. E. Knox, H. P. Hratchian, J. B. Cross, C. Adamo, J. Jaramillo, R. Gomperts, R. E. Stratmann, O. Yazyev, A. J. Austin, R. Cammi, C. Pomelli, J. W. Ochterski, P. Y. Ayala, K. Morokuma, G. A. Voth, P. Salvador, J. J. Dannenberg, V. G. Zakrzewski, S. Dapprich, A. D. Daniels, M. C. Strain, O. Farkas, D. K. Malick, A. D. Rabuck, K. Raghavachari, J. B. Foresman, J. V. Ortiz, Q. Cui, A. G. Baboul, S. Clifford, J. Cioslowski, B. B. Stefanov, G. Liu, A. Liashenko, P. Piskorz, I. Komaromi, R. L. Martin, D. J. Fox, T. Keith, M. A. Al-Laham, C. Y. Peng, A. Nanayakkara, M. Challacombe, P. M. W. Gill, B. Johnson, W. Chen, M. W. Wong, C. Gonzalez and J. A. Pople, Gaussian 03, Revision B.01, Gaussian, Inc., Pittsburgh PA, 2003 Search PubMed.
- M. Wojdyr, J. Appl. Crystallogr., 2010, 43, 1126–1128, DOI:10.1107/S0021889810030499.
- G. Chloé, V. Mihayl and E. Duval Raphaël, Viruses, 2012, 4, 3044–3068, DOI:10.3390/v4113044.
- G. D. Chumanov, R. G. Efremov and I. R. Nabiev, Raman Spectrosc., 1990, 21, 43–48, DOI:10.1002/jrs.1250210109.
- J. H. Lee, B. K. Oh and J. W. Choi, Sensors, 2015, 15(5), 9915–9927, DOI:10.3390/s150509915.
- K. Dmitry, T. Postiglione, T. Deckert-Gaudig, V. Deckert and I. K. Lednev, Analyst, 2013, 138, 1665–1673, 10.1039/C2AN36478F.
- C. Zhang, T. Zheng, H. Wang, W. Chen, X. Huang, J. Liang, L. Qiu, D. Han and W. Tan, Anal. Chem., 2021, 93(7), 3325–3330, DOI:10.1021/acs.analchem.0c05059.
- S. Desai, S. V. Mishra, A. Joshi, D. Sarkar, A. Hole, R. Mishra, S. Dutt, M. K. Chilakapati, S. Gupta and A. Dutt, J. Biophotonics, 2020, 13, e202000189, DOI:10.1002/jbio.202000189.
Footnote |
† Electronic supplementary information (ESI) available. See DOI: 10.1039/d1ra03481b |
|
This journal is © The Royal Society of Chemistry 2021 |
Click here to see how this site uses Cookies. View our privacy policy here.