DOI:
10.1039/D0MO00067A
(Research Article)
Mol. Omics, 2021,
17, 153-159
NMR-based plasma metabolomics of adult B-cell acute lymphoblastic leukemia†
Received
5th June 2020
, Accepted 12th November 2020
First published on 13th November 2020
Abstract
Acute lymphoblastic leukemia (ALL) is one of the common malignant tumors. Compared with childhood ALL, the treatment effect of adult B-cell ALL is less effective and remains a big challenge. In order to explore the pathogenesis of adult B-cell ALL and find new diagnostic biomarkers to develop sensitive diagnostic tools, we investigated the plasma metabolites of adult B-cell ALL by using 1H NMR (nuclear magnetic resonance) metabolomics. Relative to healthy controls, adult B-cell ALL patients showed abnormal metabolism, including glycolysis, gluconeogenesis, amino acid metabolism, fatty acid metabolism and choline phospholipid metabolism. What's more important, we also found that the optimal combination of choline, tyrosine and unsaturated lipids has the potential to diagnose and prognose adult B-cell ALL in the clinic.
Introduction
ALL is one of the common malignant tumors in children and adults in developed and developing countries, and the incidence of ALL is increasing year by year. With the advancement of science and technology, the cure rate of children's ALL is as high as 80%.1,2 However, the therapeutic effect of adult ALL is still very poor and the cure rate is only about 30–40%.3,4 The long-term survival rate of elderly ALL patients over 60 years old is less than 10%.5,6 Adult ALL is a multi-factor complex disease, and the pathogenesis of adult ALL disease remains to be revealed. At present, the clinical diagnosis of ALL patients still mainly depends on karyotype, immunophenotype and other clinical and laboratory indicators. All these make it impossible for people to have a deeper understanding of adult ALL disease, resulting in a low cure rate. Therefore, finding new diagnostic biomarkers to develop sensitive diagnostic pathways is the main way to improve the cure rate of adult ALL.
Metabolomics is an emerging discipline that quantitatively describes endogenous metabolites of the whole body and their response to internal and external changes as an important aspect of systems biology.7,8 It has been proved to be a powerful approach for investigating the pathogenesis and discovering new biomarkers for disease diagnosis and prognosis.9–13 NMR is one of the most commonly used detection methods in metabolomics and has many advantages that other detection methods do not have, such as being non-invasive, unbiased, flexible and simple sample processing. NMR-metabolomics has already been applied to investigate various diseases such as diabetes,14 kidney,15 lung,16 and liver tumors,17 chronic inflammatory rheumatic disease,18 breast cancer,19 melanoma,20 chronic lymphocytic leukemia21 and acute myeloid leukemia (AML).22–26
There are currently several studies using metabolomics techniques to study childhood ALL. Tiziani et al. used NMR and gas chromatography-mass spectrometry (GC-MS) to study fatty acids in childhood ALL.27 Bai et al. studied the biomarkers of childhood ALL metabolomics by liquid chromatography mass spectrometry (LC-MS).28 Musharraf et al. used serum metabolomics to differentiate the metabolic alterations associated with ALL and AML.29 Liu et al. used blood metabolomics by LC-MS to study the amino acids in blood and developed a mathematical model for assisting the diagnosis of pediatric acute leukemia (AL).30 Petrick, L. M. et al. used metabolomics of neonatal blood spots to reveal the distinct phenotypes of pediatric ALL and the potential effects of early-life nutrition.31 So far, no one has used metabolomics to study adult ALL specifically. Compared with childhood ALL, adult ALL has a lower cure rate and higher mortality rate. Revealing the comprehensive pathogenesis of adult ALL through metabolomics is very important for the diagnosis and treatment of adult ALL.
In this study, we investigated the plasma metabolites of adult B-cell ALL through NMR-based metabolomics, and showed the different metabolites between adult B-cell ALL patients and healthy controls. An abnormality in the metabolism of adult B-cell ALL patients was discovered. These results are likely to provide key clues for investigating the pathogenesis and discovering new biomarkers for adult B-cell ALL diagnosis and prognosis.
Materials and methods
Collection and preparation of clinical samples
The bone marrow plasma samples of the ALL patients were collected from 19 patients (male/female, 10/9) who ranged from 23 to 58 years. The 19 ALL patients were diagnosed without treatment before. In order to avoid age and gender differences, 19 plasma samples as healthy controls were collected from 19 healthy donors (male/female, 10/9) who ranged from 18 to 58 years. The above 38 plasma samples were obtained from the Peking University People's Hospital. Both healthy volunteers and ALL patients provided informed consent or parental permission prior to collection of any data.
The study was approved by the Ethics Committee of Peking University People's Hospital and was in accordance with the national research committee and the 1964 Declaration of Helsinki and its subsequent amendments or comparable ethical standards. The plasma samples with clinical records were collected with informed consent.
The donors had not undergone any treatment and also fasted for 12 h before sampling, which may eliminate possible interferences. Owing to the negligible interference of heparin on metabolites,32–34 the blood samples were collected in vacuum tubes containing heparin anticoagulant and shaken slowly. The samples were then placed at room temperature for 1 h and centrifuged at 1000g for 10 min at 4 °C. The plasma supernatant samples were collected and stored immediately at −80 °C until analysis.
Pretreatment of plasma samples
The plasma samples were thawed at room temperature and centrifuged at 12
000g for 10 min at 4 °C. Each plasma sample (100 μL) was mixed with phosphate buffer (100 μL, pH 7.4) which was prepared by mixing a solution of 1 M K2HPO4 (100% D2O) and a solution of 0.25 M NaH2PO4 (100% D2O) in a 1
:
1 ratio by volume. The mixture solution was shaken for 1 min and centrifuged at 12
000g for 10 min at 4 °C. Finally, the supernatant (150 μL) was added to the 3 mm NMR tube.
NMR data collection of plasma samples
The 1H NMR spectra of the plasma samples were recorded at 600.13 MHz using a Bruker 600 Ultra Shield TM spectrometer with a BBI probe at 300 K. The standard 1H NMR spectra (NOESY), the T2 edited 1H NMR spectra (Car-Purcell-Meiboom-Gill (CPMG)) and the diffusion-edited 1H NMR spectra (bipolar pulse pair-longitudinal eddy current delay pulse sequence (BPP-LED)) were obtained for all plasma samples. We focused on the analysis of low weight molecule metabolites in plasma through the relaxation edited CPMG.21 The pre-saturation sequence was used to eliminate the interference of water. The relaxation delay was 2 s. The data points were collected at 64 K over a spectral width of 30 ppm and the number of scans was set to 256. The line exponential function was set to 1.0 Hz line broadening factor before Fourier transformation. The 1H NMR spectra of CPMG were calibrated to the proton signal of lactate at the chemical shift (δ) = 1.336. The BPP-LED with water suppression was also carried out to select the signals of macromolecules.35 The spectra were acquired with a gradient amplitude of 50.0 G cm−1, a diffusion delay of 50 ms, a data point of 30 ppm spectral width and a line-broadening factor of 1.0 Hz. The 1H NMR of BPP-LED spectra were calibrated to the proton signal of phosphatidylcholine at a chemical shift (δ) = 3.220.
Pretreatment of NMR spectra
The pretreatment of all NMR spectra was done using the software of MestReNova (6.1.1-6384-win), including adjusting the phase and baseline, calibrating chemical shift, removing the interference of water peak, dividing into buckets and normalizing. The algorithms of automatic phase correction and baseline correction were set to Metabolomics and Polynomial fitting, respectively. The proton signal of lactate (δ = 1.336) was used to calibrate the chemical shift to eliminate the systematic error of chemical shift. The spectra over the range from δ 0.5 to δ 9.5 were divided into buckets at an equal width of 0.005 ppm with the regions at δ 4.200–δ 5.100 being removed as the water peak. In order to minimize the effect of concentration differences between different samples, all buckets of spectra were normalized to a constant sum (100).21,36,37
Multivariate data analysis
The data from the NMR spectra were imported into the software of SIMCA-P (version 14.0, Umetrics) for multivariate analysis. Principal components analysis (PCA) and orthogonal projection to latent structure with discriminant analysis (OPLS-DA) were carried out to screen the differential metabolites between healthy controls and ALL patients.35,37,38 The scaling of PCA was set to the mean-center and the scaling of OPLS-DA was set to Pareto before analysis in CPMG spectra. The two components were retained in all multivariate models and the quality of the models was ensured by their parameters, such as R2X, R2Y and Q2. The CV-ANOVA method35,39,40 and permutation testing (n = 200) were conducted to further evaluate the model which was ultimately used to screen differential metabolites. We performed a multivariate statistical analysis of BPP-LED spectra.
Differential metabolites analysis
The different plots of the VIP > 1 between healthy controls and ALL patients were processed using the Mann–Whitney test (GraphPad prism 5). The significantly different plots were screened between groups (P-value). In order to study the trend of differential metabolites, box-plot (Metaboanalyst: http://www.metaboanalyst.ca/) and hierarchical cluster analysis (HCA, Ri386 3.2.1) were conducted. Logistic regression and receiver operating characteristic curve (ROC) were used to screen potential diagnostic biomarkers and test the diagnostic efficiency of potential diagnostic biomarkers, respectively (SPSS 17.0).
Results
Characteristics of plasma samples
In this study, the aspects of all plasma samples were shown in Table S1 (ESI†). The stratification of ALL patients complies with the World Health Organization criteria and the National Comprehensive Cancer Network clinical practice guidelines.41 There was no statistically significant difference in age and gender between the controls and the ALL patients (Age, P = 0.9245 > 0.05 (Wilcoxon test); gender, the ratio of male to female is exactly the same). This indicates that any possible metabolic differences in subjects of age and gender will not affect the majority of variance in the data.
Metabolic profile analysis of plasma
The 1H NMR experiments were conducted for each plasma sample for a comprehensive analysis of metabolites in plasma. The 1H NMR spectra of plasma are shown in Fig. S1 (ESI†). The major assignments of plasma metabolites present were low weight molecular compounds, such as amino acids and glucose. The resonances of macromolecules were also shown in the CPMG spectra, such as lipids and glycoproteins. Therefore, we focused on the analysis of CPMG spectra. The assignments of plasma metabolites in typical CPMG 1H NMR spectra for healthy controls and ALL patients are shown in Fig. 1. The identification of metabolites includes amino acids, choline, N-acetyl-glycoproteins, saturated and unsaturated fatty acids, etc. With reference to the Human Metabolome Database, the relevant literature35,36,42,43 and the software AMIX (v 3.9.12, BrukerBioSpin), we identified a total of 35 metabolites in CPMG. In addition, we identified 9 metabolites in the BPP-LED spectra and the results are shown in Fig. S2 (ESI†). The 1H chemical shift and multiplicity of assignments are shown in ESI,† Table S2.
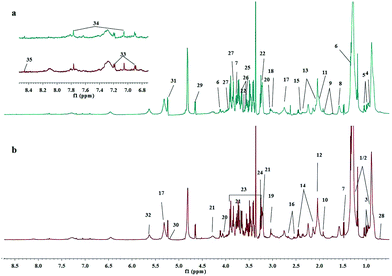 |
| Fig. 1 Typical CPMG 1H NMR spectra of plasma for healthy control (a) and ALL patient (b). Assignments of plasma metabolites: (1) lipids (mainly LDL); (2) lipids (mainly VLDL); (3) isoleucine; (4) leucine; (5) valine; (6) lactate; (7) alanine; (8) citrulline; (9) arginine; (10) acetate; (11) proline; (12) N-acetyl-glycoproteins(NAc); (13) glutamate; (14) glutamine; (15) pyruvate; (16) citrate; (17) unsaturated lipid; (18) α-ketoglutarate; (19) creatine; (20) creatinine; (21) choline; (22) phosphorylcholine(PC)/glycerophosphocholine(GPC); (23) glucose; (24) trimethylamine-N-oxide (TMAO); (25) glycine; 26. glycerol; (27) serine; (28) cholesterol; (29) β-glucose; (30) glycerol of lipids; (31) α-glucose; (32) urea; (33) tyrosine; (34) histidine; (35) formate. | |
Multivariate analysis
Multivariate analysis is often used to identify differences between two groups. In this paper, unsupervised PCA was used to identify the differences between healthy controls and ALL patients. However, the first two principal components of PCA failed to show a clear separation between healthy controls and ALL patients (ESI,† Fig. S3). This result may be due to the complexity of clinical samples affected by geography, diet and other external factors. We continued to analyze the data of healthy controls and ALL patients with supervised OPLS-DA. Finally, we used the model of OPLS-DA to separate healthy controls and ALL patients along the t1 direction with R2X = 52.2%, R2Y = 70.9% and Q2 = 51.5%. The result is shown in Fig. 2(a). We validated the OPLS-DA model using a permutation test (Fig. 2(b)) and CV-ANOVA. In the permutation test, the intercept value of the R2 truncation line is not more than 0.4 (0.222), the intercept value of the Q2 truncation line is not more than 0.05 (−0.415) and the leftmost data of the R2 and Q2 are lower than the rightmost values. All of these results demonstrate that the OPLS-DA model is not over-fitting and effective. On the other hand, the P value (6.2818 × 10−5) of the CV-ANOVA is less than 0.05, which also proves that the OPLS-DA model is effective. The multivariate analysis results of the BPP-LED spectra are shown in Fig. S4 (ESI†). We used the model of OPLS-DA to separate the healthy controls and the ALL patients with R2 = 53.1%, R2Y = 94.4% and Q2 = 46.3%.
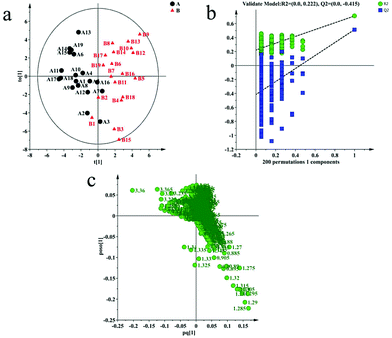 |
| Fig. 2 Multivariate analyses of healthy controls and ALL patients. (a) The scores of the OPLS-DA for healthy controls (A, black circle) and ALL patients (B, red triangle). (b) The permutation test result for the OPLS-DA model (R2 = (0.0, 0.222), Q2 = (0.0, −0.415)). (c) The corresponding loading plot of the OPLS-DA model. | |
Screening of differential metabolites
In order to screen the differential metabolites associated with the disease, we analyzed the corresponding loading plot of the OPLS-DA model (Fig. 2(c)), which indicates the metabolites primarily responsible for the separation and their tendency to change. The VIP > 1 plots of the OPLS-DA model were considered to be greater contribution plots for the clustering between healthy controls and ALL patients. Statistically significant difference is also a necessary condition for screening. So we screened the differential metabolites between controls and ALL patients using VIP > 1 and Mann–Whitney test < 0.05. 15 differential metabolites with statistically significant differences were screened out by us. The result was shown in Table 1. According to the corresponding loading plot of OPLS-DA, we can see that the levels of LDL, VLDL, NAc, glutamate, valine, isoleucine, alanine, choline, PC/GPC, glucose, TMAO, Lactate, unsaturated lipid, tyrosine and histidine are different between ALL patients and healthy controls. In the BPP-LED spectra, we screened out some metabolites with statistical differences. These metabolites are almost all included in the differential metabolites screened from the CPMG spectra, and the trend of change is the same. Therefore, they will not be discussed separately. The result is shown in Table S3 (ESI†).
Table 1 Differential metabolites between healthy controls and ALL patients. s, singlet; d, doublet; q, quartet; dd, doublet of doublet; m: multiplet
Metabolites |
δ
1 H (ppm) |
Multiplicity |
P-Value |
Changes in ALL patients against healthy controls |
LDL |
1.25 |
m |
< 0.0001 |
↑ |
VLDL |
0.88 |
m |
< 0.0001 |
↑ |
Valine |
0.99 |
d |
< 0.0001 |
↓ |
Isoleucine |
1.005 |
d |
< 0.0001 |
↓ |
Alanine |
1.48 |
d |
0.0002 |
↓ |
NAc |
2.04 |
s |
0.0017 |
↑ |
Glutamate |
2.07 |
m |
0.0311 |
↑ |
Choline |
3.2, 3.665 |
s, m |
< 0.0001 |
↓ |
PC/GPC |
3.22 |
s |
< 0.0001 |
↓ |
Glucose |
3.23 |
dd |
0.0009 |
↓ |
TMAO |
3.26 |
s |
0.0190 |
↓ |
Lactate |
4.11 |
q |
0.0097 |
↓ |
Unsaturated lipid |
5.27 |
m |
0.0001 |
↑ |
Tyrosine |
6.90 |
dd |
0.0285 |
↓ |
Histidine |
7.77 |
s |
0.0005 |
↓ |
Trends of differential metabolites
The differences between groups can be visually presented through box-plot. The box-plot of the differential metabolites between healthy controls and ALL patients is shown in Fig. 3. The levels of differential metabolites vary significantly between healthy controls and ALL patients. Compared with all healthy controls, ALL patients show an increased concentration of LDL, VLDL, NAc, glutamic acid and unsaturated lipids, but a decreased concentration of valine, isoleucine, alanine, choline, PC/GPC, glucose, TMAO, Lactate, tyrosine and histidine. These differential metabolites may provide us with some relevant information about adult B-cell ALL.
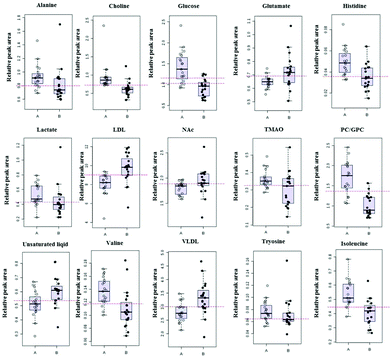 |
| Fig. 3 Box-plot of the differential metabolites between healthy controls (A, hollow circle) and ALL patients (B, solid circle). | |
In order to visualize the relationship between the samples and the differential metabolites, HCA was conducted (Fig. 4). The samples and the differential metabolites are hierarchically clustered simultaneously with high correlation being first gathered together. The upper horizontal axis of the graph shows the dendrogram of the samples. Basically, most samples from the healthy controls and the ALL patients are clustered together respectively. The left vertical axis of the figure shows a dendrogram of the differential metabolites. The differential metabolites in the same or similar metabolic pathways are first clustered together. Metabolites such as LDL, VLDL and unsaturated lipids belonging to the same metabolic pathway accumulate in the first layer. In addition, valine and isoleucine, which belong to branched chain amino acids, are in the same metabolic pathway and are cluttered in the first layer. Compared with healthy controls, the levels of the most differential metabolites in ALL patients are lower, such as valine, isoleucine, alanine, choline, etc. However, LDL, VLDL, NAc, glutamate and unsaturated lipid have higher concentrations. This result is consistent with the result of the box-plot.
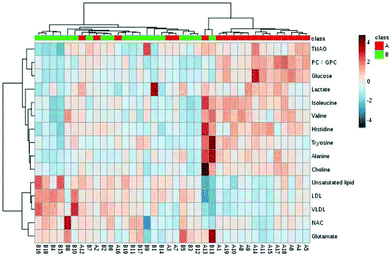 |
| Fig. 4 HCA heat map of the 15 significantly different metabolites between healthy controls (A, red) and ALL patients (B, green). | |
Screening of potential diagnostic biomarkers
Logistics regression belongs to probabilistic nonlinear regression analysis. It has strong recognition and prediction capabilities and is widely used to screen epidemiological risk factors. We used stepwise logistic regression with backward selection to screen the potential diagnostic biomarkers from the differential metabolites. The logistic regression model is excellent by analyzing the relevant parameters and evaluating the diagnostic efficacy of the equation (ESI,† Tables S4 and S5). Choline, tyrosine and unsaturated lipids were selected as the optimal combination for diagnosing ALL patients. Based on the data from ALL patients and healthy controls, the ROC curve of the combination of choline, tyrosine and unsaturated lipids yields an area under the curve of 0.970 to evaluate the overall diagnostic efficiency of these three metabolites (Fig. 5). The area under the ROC curve is closer to 1. Therefore, the diagnostic model composed of choline, tyrosine and unsaturated lipids has a higher diagnostic efficiency and has the potential to diagnose ALL patients and healthy controls.
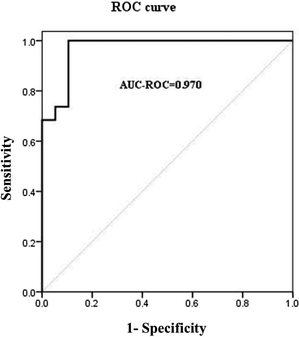 |
| Fig. 5 ROC curve for distinguishing the ALL patients from the healthy controls by the combination of choline, tyrosine and unsaturated lipids. | |
Discussion
Some reports have used metabolomics techniques to study ALL. However, as far as we know, no one has used metabolomics to specifically study adult ALL. The number of sample replicates in non-targeted metabolomics is greater than 6. If the number is too small, the multivariate statistical model is prone to over-fitting. In addition, the small sample size may cause some deviations in the results. For example, some metabolites with statistical differences may not be screened or some metabolites among the screened differential metabolites may be verified by a large sample as having no significant statistical difference. In this paper, we studied 38 bone marrow plasma samples including 19 adult B-cell ALL patients and 19 healthy controls using a 1H NMR-based metabolomics approach. The age and gender of the sample donor do not affect clustering. It has been proved that the OPLS-DA model constructed with 38 plasma samples has no over-fitting phenomenon by permutation test in multivariate analysis. Therefore, the multivariate statistical model should be effective. Although the robustness of the analysis may be increased in future studies using more samples, our research still provides evidence for the abnormal metabolism of adult B-cell ALL patients by comparing the metabolites of 38 plasma samples collected from 19 healthy controls and 19 ALL patients.
In this study, OPLS-DA was used to distinguish ALL patients from healthy controls. The two groups were well separated, and LDL, VLDL, NAc, glutamate, valine, isoleucine, alanine, choline, PC/GPC, glucose, TMAO, Lactate, unsaturated lipids, tyrosine and histidine made a great contribution to the separation. The variations of these differential metabolites were clearly shown in the box-plot and the HCA heat map. The metabolism of plasma is considered to be a collective “snapshot” reflecting the metabolic alteration throughout the body. Therefore, the physiological characteristics of the disease are known by studying plasma metabolites. According to the different metabolites we studied, multiple metabolic pathways of ALL patients have changed compared with healthy controls.
The differences of plasma metabolites between adult B-cell ALL patients and healthy controls indicates changes in energy metabolism. Warburg had speculated that most tumor cells supply their own energy through glycolysis, while healthy cells have sufficient oxygen and do not rely on glycolysis. This metabolic change may be the fundamental cause of cancer.44,45 The process of glycolysis is likely to consume most of the blood glucose and release lactate. When the amount of glucose cannot meet the needs of cancer cells, glucogenic amino acids and lactate can meet the glucose consumption of tumor cells through gluconeogenesis. In our study, the levels of glucose, lactate and glucogenic amino acids including alanine, isoleucine, valine and tyrosine were lower in the ALL patients’ plasma. Perhaps the glucose in the ALL patients’ plasma is consumed to meet the energy requirements of cancer cells. In addition, gluconeogenesis has also been enhanced by consuming blood lactate and glucogenic amino acids in ALL patients to satisfy the demands of metabolism in tumor cells.46,47 This phenomenon has been reported in Acute Myeloid Leukemia.22
Glutamine is a free amino acid with the highest content in blood. It is considered to be the most important nitrogen source and respiratory material for mammals. However, glutamine is an essential amino acid for tumor cells. It is taken up by tumor cells as an important nitrogen and energy source. Glutamate is also used to synthesize purines and pyrimidine to support the biosynthesis of DNA and RNA. In order to satisfy the proliferation of tumor cells, the glutamine in blood is depleted. Decreased glutamine has been found in many tumors.22,48,49 However, the level of glutamine increased in our study. This phenomenon is probably due to the lack of glutamine in the blood, which leads to the up-regulation of glutamine synthase expression, and the body releases more glutamine into the blood to maintain the blood glutamine concentration. When glutamine is released at a higher concentration than what is consumed in blood, the level of blood glutamate will rise. The level of histidine fed to the tricarboxylic acid cycle (TCA cycle) by conversion to glutamine was decreased. It may also be a supplement to glutamine in the blood. The increase of glutamine in ALL is consistent with reports of other types of cancer.32,48,50
Lipid abnormalities were observed in this study. Adult ALL patients have higher levels of unsaturated lipids, VLDL and LDL, in plasma metabolites. This phenomenon is probably due to abnormal lipid metabolism in adult ALL patients. The proliferation of tumor cells requires the consumption of large amounts of biological energy, which is not only obtained from glucose by glycolysis, but also from lipids through β-oxidation. Due to the large amount of energy needed to meet cell proliferation, tumor cells will alter their lipid metabolism. Not only can it active lipid synthesis, but it can also enhance the mobilization of adipose tissue.51 Tumor cells obtain sufficient lipids through these two pathways, which leads to a decrease in the utilization rate of exogenous lipids. This may be the reason for the increased concentrations of unsaturated lipid, VLDL and LDL in the plasma of adult ALL patients.
Choline and its derivatives are important nutrients and the main components of cell membranes.52 The biosynthesis of cell membranes in tumor tissues is very active, and a large amount of choline is required as a raw material in the biosynthesis of cell membranes. The levels of choline and its derivatives in blood were elevated in many cancer patients compared to healthy controls.53,54 However, in our study, the levels of choline and PC/GPC were higher in the blood of adult ALL patients. It is probably because a large amount of choline and its derivatives in the blood are taken up by tumor cells to satisfy their own cell proliferation. The low level of choline and PC/GPC in the blood of adult B-cells is consistent with the results that have been reported in AML patients and childhood ALL patients.22,28
In our study, a decrease in the plasma concentration of TMAO was observed in adult B-cell ALL patients. Studies have shown that TMAO is closely related to the occurrence of cardiovascular diseases and other related diseases.55–57 TMAO in the blood can change the functions of platelets. It is able to make the platelets overreact to speed up clotting.57 Compared with healthy controls, adult B-cell ALL patients have a lower blood TMAO concentration, which is probably one of the reasons why a person with leukemia person cannot easily clot.
All the differential metabolites screened out from plasma may serve as candidate biomarkers. We used binary logistic regression to analyze the differential metabolites. Finally, we found that choline, tyrosine and unsaturated lipids as the optimal combination has the potential to diagnose adult B-cell ALL. The optimal combination of these three metabolites could distinguish ALL patients from healthy controls with the ROC curve AUC value of 0.970. Due to the limitations of the Logistic regression method and the complexity of clinical samples, whether it can be used as a biomarker requires further analysis for verification. Furthermore, it is possible that some of our ALL subjects had other disorders or treatments that may have affected their metabolome, and thus we recognize this as a potential limitation of our study.
Conclusions
This study shows the characteristics of plasma metabolism in adult patients with B-cell ALL through NMR-based metabolomics studies. The differential metabolites in the plasma between adult B-cell ALL patients and healthy controls demonstrate that adult B-cell ALL patients have abnormal metabolism pathways. Glycolysis and gluconeogenesis were enhanced, which may be used to support the rapid consumption of energy by tumor cells in adult B-cell ALL patients. Compared with healthy controls, adult B-cell ALL patients showed abnormalities of amino acid metabolism, fatty acid metabolism, and choline phospholipid metabolism.
Our study also showed the advantages of metabolomics technology in the identification and diagnosis of adult B-cell ALL patients. It can conduct a comprehensive study of the host's metabolic conditions to find abnormal metabolism that is closely related to the disease. Abnormal metabolism provides important clues for controlling disease progression and exploring new diagnostic and therapeutic evaluation methods for adult B-cell ALL patients.
Authors’ contributions
Conceptualization: Y. T., F. Y., H. S., J. X., Q. L., G. R., data curation: F. Y., J. X., formal analysis: F. Y., Q. L., funding acquisition: Y. T., H. S., Q. L., H. Z., investigation: F. Y., methodology: F. Y., J. X., G. R., Q. L., project administration: Y. T., resources: F. Y., G. R., supervision: Y. T., validation: F. Y., H. S., visualization: F. Y., Q. L., writing ± original draft: F. Y., H. S., Q. L., Y. T., H. Z., writing ± review & editing: F. Y., H. S., Q. L., Y. T., H. Z.
Compliance with ethical standards
The authors declare that we comply with ethical standards.
Conflicts of interest
The authors declared no competing interest.
Acknowledgements
This work was supported by the National Natural Science Foundation of China (Grant Numbers 21675162, 21602226, 21778058, 21977096, and 21977099), and Beijing Natural Science Foundation (7182189 and 7172248).
References
- I. Iacobucci, C. Papayannidis and A. Lonetti, Curr. Hematol. Malig. Rep., 2012, 7, 133–143 CrossRef.
- I. Iacobucci, C. T. Storlazzi, D. Cilloni, A. Lonettiand, E. Ottaviani and S. Soverini, Blood, 2009, 114, 2159–2167 CrossRef CAS.
- N. Gokbuget and D. Hoelzer, Semin. Hematol., 2009, 46, 64–75 CrossRef.
- R. Bassan and D. Hoelzer, J. Clin. Oncol., 2011, 29, 532–543 CrossRef.
- A. H. Goldstone, S. M. Richards and A. K. Fielding, Blood, 2008, 111, 5755 CrossRef CAS.
- G. Martinelli, I. Iacobucci and C. T. Storlazzi, J. Clin. Oncol., 2009, 27, 5202–5207 CrossRef CAS.
- J. K. Nicholson and J. C. Lindon, Nature, 2008, 455, 1054–1056 CrossRef CAS.
- J. K. Nicholson, J. C. Lindon and E. Holmes, Xenobiotica, 1999, 29, 1181–1189 CrossRef CAS.
- A. Di Leo, W. Claudino and D. Colangiuli, Ann. Oncol., 2007, 18, 8–14 CrossRef.
- H. van Wietmarschen, K. L. Yuan, C. Lu and P. Gao, J. Clin. Rheumatol., 2009, 15, 330–337 CrossRef.
- J. L. Nierenberg, J. He, C. W. Li, X. Y. Gu and M. Y. Shi, Metabolomics, 2019, 15, 149 CrossRef.
- J. Maras, A. Bhatand and C. Junot, J. Gastroenterol. Hepatol., 2019, 34, 469 Search PubMed.
- J. Kim, S. C. Kang, N. E. Yoon, Y. Kim, J. Choi, N. Park, H. Jung, B. H. Jung and J. H. Ju, Stem Cell Res. Ther., 2019, 10, 319 CrossRef.
- A. V. Ahola-Olli, L. Mustelin and M. Kalimeri, Diabetologia, 2019, 62, 2298–2309 CrossRef CAS.
- S. W. Qi, X. Ouyang and L. Q. Wang, CTS: Clin. Transl. Sci., 2012, 5, 379–385 CAS.
- C. M. Rocha, J. Carrola and A. S. Barros, J. Proteome Res., 2011, 10, 4314–4324 CrossRef CAS.
- Y. S. Chung, G. Lin and T. Yen, Eur. J. Nucl. Med. Mol. Imaging, 2019, 46, 706 Search PubMed.
- A. Vignoli, D. M. Rodio and A. Bellizzi, Anal. Bioanal. Chem., 2017, 409, 1405–1413 CrossRef CAS.
- H. W. Gu, Z. Z. Panand and B. W. Xi, Anal. Chim. Acta, 2011, 686, 57–63 CrossRef CAS.
- A. P. de Santana, T. Jacomasso and D. S. Riter, Sci. Rep., 2017, 7, 42324 CrossRef.
- D. A. MacIntyre, B. Jimenez and E. J. Lewintre, Leukemia, 2010, 24, 788–797 CrossRef CAS.
- Y. H. Wang, L. M. Zhangand and W. L. Chen, J. Proteome Res., 2013, 12, 4393–4401 CrossRef CAS.
- K. E. Cano, L. Li, S. Bhatia and R. Bhatia, J. Proteome Res., 2011, 10, 2873–2881 CrossRef CAS.
- I. S. Gronningsaeter, H. K. Fredly and B. T. Gjertsen, Cells, 2019, 8, 1229 CrossRef CAS.
- Z. Zheng, P. Y. Liu and L. T. Xu, RSC Adv., 2018, 8, 14445–14453 RSC.
- H. Bhanot, M. M. Reddy and A. Nonami, Leukemia, 2015, 29, 1555–1563 CrossRef CAS.
- S. Tiziani, Y. Y. Kang and R. Harjanto, PLoS One, 2013, 8, e82859 CrossRef.
- Y. N. Bai, H. T. Zhangand and X. H. Sun, Clin. Chim. Acta, 2014, 436, 207–216 CrossRef CAS.
- S. G. Musharraf, A. J. Siddiquiand and T. Shamsi, Sci. Rep., 2016, 6, 30693 CrossRef CAS.
- Z. D. Liu, T. T. Zhou and X. Han, J. Transl. Med., 2019, 17, 38 CrossRef.
- L. M. Petrick, C. Schiffmanand and W. M. B. Edmands, Cancer Lett., 2019, 452, 71–78 CrossRef CAS.
- A. M. F. Tovar, N. V. M. Capille, G. R. C. Santos, B. C. Vairo, S. N. M. C. G. Oliveira, R. J. C. Fonseca and P. A. S. Mourao, Thromb. Haemostasis, 2012, 107, 903–915 CAS.
- H. Y. Liu, Z. Q. Zhang and R. J. Linhardt, Nat. Prod. Rep., 2009, 26, 313–321 RSC.
- J. Pinto, M. R. M. Domingues, E. Galhano, C. Pita, M. D. Almeida, I. M. Carreira and A. M. Gil, Analyst, 2014, 139, 1168–1177 RSC.
- F. M. Yang, J. Du and H. Zhang, PLoS One, 2017, 12, e0170896 CrossRef.
- M. AlaKorpela, Prog. Nucl. Magn. Reson. Spectrosc., 1995, 27, 475–554 CrossRef CAS.
- R. H. Barton, J. K. Nicholson and P. Elliott, Int. J. Epidemiol., 2008, 37, 31–40 CrossRef.
- O. Cloarec, M. E. Dumas and J. Trygg, Anal. Chem., 2005, 77, 517–526 CrossRef CAS.
- S. Wold, Technometrics, 1978, 20, 397–405 CrossRef.
- L. Eriksson, J. Trygg and S. Wold, J. Chemom., 2008, 22, 594–600 CrossRef CAS.
- M. R. O’Donnell, C. N. Abboud and J. Altman, J. Natl. Compr. Cancer Network, 2012, 10, 984–1021 CrossRef.
- H. R. Tang, Y. L. Wangand and J. K. Nicholson, Anal. Biochem., 2004, 325, 260–272 CrossRef CAS.
- H. C. Gao, B. J. Dong and J. M. Jia, J. Cancer Res. Clin. Oncol., 2012, 138, 753–761 CrossRef CAS.
- O. Warburg, F. Wind and E. Negelein, J. Gen. Physiol., 1927, 8, 519–530 CrossRef CAS.
- O. Warburg, Science, 1956, 123, 309–314 CrossRef CAS.
- V. E. Baracos and M. L. Mackenzie, J. Nutr., 2006, 136, 237s–242s CrossRef CAS.
- M. G. V. Heiden, L. C. Cantley and C. B. Thompson, Science, 2009, 324, 1029–1033 CrossRef.
- A. N. Zira, S. E. Theocharis and D. Mitropoulos, J. Proteome Res., 2010, 9, 4038–4044 CrossRef CAS.
- H. S. Lai, J. C. Lee and P. H. Lee, Semin. Cancer Biol., 2005, 15, 267–276 CrossRef CAS.
- Q. Huang, Y. X. Tanand and P. Y. Yin, Cancer Res., 2013, 73, 4992–5002 CrossRef CAS.
- J. A. Menendez and R. Lupu, Nat. Rev. Cancer, 2007, 7, 763–777 CrossRef CAS.
- S. H. Zeisel and J. K. Blusztajn, Annu. Rev. Nutr., 1994, 14, 269–296 CrossRef CAS.
- S. M. Ronen and M. O. Leach, Breast Cancer Res., 2001, 3, 36–40 CrossRef CAS.
- K. Glunde and N. J. Serkova, Pharmacogenomics, 2006, 7, 1109–1123 CrossRef CAS.
- C. C. Yue, X. D. Yang and J. Li, Biochem. Biophys. Res. Commun., 2017, 490, 541–551 CrossRef CAS.
- Z. N. Wang, E. Klipfelland and B. J. Bennett, Nature, 2011, 472, 57–82 CrossRef CAS.
- W. F. Zhu, J. C. Gregory and E. Org, Cell, 2016, 165, 111–124 CrossRef CAS.
Footnote |
† Electronic supplementary information (ESI) available: Fig. S1: 1H NMR spectra of plasma from healthy control and ALL; Fig. S2: typical BPP-LED 1H NMR spectra of plasma for healthy control and ALL patient; Fig. S3: the scores of the PCA for the controls (green circle) and the ALL patients (blue box) in CPMG; Fig. S4: multivariate analyses of BPP-LED 1H NMR spectra of healthy controls and ALL patients; Table S1: plasma samples used in this study; Table S2: assignments of plasma metabolites; Table S3: differential metabolites between healthy controls and ALL patients in BPP-LED spectra; Table S4: the related parameters of logistic regression equation; Table S5: the evaluation of diagnostic efficacy of Logistic regression model. See DOI: 10.1039/d0mo00067a |
|
This journal is © The Royal Society of Chemistry 2021 |
Click here to see how this site uses Cookies. View our privacy policy here.