DOI:
10.1039/D1AN01569A
(Paper)
Analyst, 2022,
147, 178-186
An in vivo bioelectronic nose for possible quantitative evaluation of odor masking using M/T cell spatial response patterns†
Received
30th August 2021
, Accepted 8th November 2021
First published on 17th November 2021
Abstract
Odor masking is a prominent phenomenon in the biological olfactory perception system. It has been applied in industry and daily life to develop masking agents to reduce or even eliminate the adverse effects of unpleasant odors. However, it is challenging to assess the odor masking efficiency with traditional gas sensors. Here, we took advantage of the olfactory perception system of an animal to develop a system for the evaluation and quantification of odor masking based on an in vivo bioelectronic nose. The linear decomposition method was used to extract the features of the spatial response pattern of the mitral/tufted (M/T) cell population of the olfactory bulb of a rat to monomolecular odorants and their binary mixtures. Finally, the masking intensity was calculated to quantitatively measure the degree of interference of one odor to another in the biological olfactory system. Compared with the human sensory evaluation reported in a previous study, the trend of masking intensity obtained with this system positively correlated with the human olfactory system. The system could quantitatively analyze the masking efficiency of masking agents, as well as assist in the development of new masking agents or flavored food in odor or food companies.
1. Introduction
Odor masking is a significant aspect of odor interactions and plays a pivotal role in industry and human health. It is defined as the processing that modifies the quality of odor to make it more acceptable.1,2 In the olfactory systems of vertebrate animals, olfactory sensory neurons (OSNs) in the olfactory epithelium (OE) are activated by odor through the G-protein coupling pathway. Axons of OSNs expressing a given receptor converge to a few fixed glomeruli in the olfactory bulb (OB). Mitral/tufted (M/T) cells, called projection neurons, receive input from OSNs and send signals to the olfactory cortex.3 Previous studies indicated that odor masking occurs in the OE or OB.4–7 In OE, masking agents might inhibit cyclic nucleotide-gated (CNG) ion channels in the cilia of OSNs, and regulate the CNG level to achieve masking.5 Besides, M/T cells that receive lateral inhibition from periglomerular cells (PCs) and granule cells (GCs) would also participate in the odor masking process.6
It has been reported that the exposure of animals and humans to air pollutants will trigger fear memories, affect mood, and even cause psychological diseases such as anxiety-related disorders.8–10 Masking agents such as perfume or essential oils are utilized for decreasing the unpleasantness of smells,11 which is an important and common application of odor masking. The effect of odor masking can be assessed through direct (olfactory perception) and indirect (instrument) approaches.12,13 Directly, researchers evaluated the binary odor interaction effect with descriptions of odors14 or detection of the ratio of dilution to the threshold of odors by a group of trained panelists.12 If the masking effect occurs before olfactory perception (i.e. masking agents react with the odor compounds), a gas sensor is competent in evaluating the masking effect by detecting special odor substances.15 However, instruments are limited in assessing the masking efficiency due to the lack of biological perception. To overcome this limitation, researchers combine olfactometry and chemical analysis apparatus such as gas chromatography/mass spectrometry (GC/MS) to integrate the advantages of smell sense and analytical instruments.16,17 However, it will take a long time to train a person as an olfactory expert, and instruments are expensive. Moreover, many researchers achieved masking evaluation based on rats from the in vitro to in vivo level. The odor masking evaluation methods related to rodents include electrophysiology technology, imaging in the olfactory region of the mouse, and behavior tests of rats and mice.18–23 Previous studies have reported that rodents are suitable for the detection of odors as biosensors due to their sensitive sense of smell.24–26In vivo bioelectronic noses (in vivo bio-eNoses) have the potential to evaluate odor masking because of the perfect combination of olfactory perception and chemical analysis instruments. The phase encoding and lateral suppression of M/T cells have been utilized to study the odor masking efficiency, respectively.6,18 However, methods for analyzing the population response of M/T cells have rarely been reported. In addition, Takeuchi et al. have reported the human masking score of isovaleric acid.5 Therefore, odorants used as masking agents and masked odor were partly selected in fatty acids, ketones, and aldehydes mentioned in the former study so as to verify the reliability of the results from this study.
Here, we present an odor masking evaluation system for the detection and evaluation of odor masking efficiency by implanting a microwire array in the anteromedial olfactory bulb of rats. The evaluation system could be reused in nearly a month. We analyzed the aldehyde-, ketone-, and fatty acid-response patterns of M/T cells in the anteromedial OB. Then, we used the vector space decomposition method for quantifying the difference in firing patterns of M/T cells stimulated by monomolecular odorants and binary mixtures. Finally, we compared the masking evaluation result with the human olfactory assessment for verifying the feasibility of the system and the difference in the olfactory perception between humans and rats. The odor masking evaluation system, based on the in vivo bio-eNose, has the advantage of quantitatively evaluating the interactions of odorants with the biological olfactory system. Besides, it is competitive in examining the performance of novel masking agents and flavored food.
2. Methods and experiments
2.1.
In vivo bio-eNose fabrication and electrophysiological recordings
The evaluation system consists of an in vivo bioelectronic nose, an acrylic box (30 × 20 × 40 cm), and a neural signal acquisition system. Firstly, to set up an in vivo bioelectronic nose, a homemade implantable microwire array was located in the anteromedial olfactory bulb, and the details are provided in section 2.2. A 16-channel electrode was made of 4 tetrodes (#761500, A-M Systems, USA) with an impedance of 300–500 KΩ at 1 kHz, a custom-made printed circuit board (PCB) for arranging micro-wires parallelly in both sides and a connector.27,28 To fix nichrome wires, PEG-4000 (Sigma-Aldrich, USA) was heated to 100 °C and covered on wires with a syringe needle (Fig. S1†). Secondly, an acrylic box was designed using SolidWorks 2017 (Dassault Systemes, USA) for odor testing. To eliminate power frequency interference, a copper net was covered on the outside of the box.
Neural activity from each channel was amplified at 1750 times gain, bandpass filtered at 0.5–7000 Hz, and sampled at 40 kHz with an OminiPlex Data Acquisition System (Plexon, Inc. USA). The continued spike signal was digitally filtered from a wide band signal with a bandpass filter between 300 and 7000 Hz. Spike sorting was performed with offline sorter 4 (Plexon, Inc., USA) to get spike trains.
2.2. Animal preparation and surgery
SD (Sprague Dawley) rats weighing 250–350 g at the start of the experiment were maintained on a 12 h light–dark cycle and housed individually. During the surgery, the head of the rat was attached to a stereotaxic instrument (RWD Inc., China), after being deeply anesthetized with pentobarbital (40 mg kg−1, ip). Meanwhile, 5 mL of atropine (1 mg mL−1, ip) was injected to prevent excessive secretion in the airways. According to the Paxinos and Watson's rat brain atlas,39 a cranial window (∼1.5 × ∼0.5 mm) above the right olfactory bulb (8.5–10 mm anterior to the bregma, 0.7 mm lateral to the midline suture) was drilled. The electrode was implanted into the olfactory bulb vertically (0.5–2 mm below the surface of the brain) at a low speed. Six skull screws were placed on the skull. The reference and ground wires of the electrode were tightly wrapped around the screws, respectively. Finally, dental cement was used to firmly anchor the electrode and screws on the skull. Dexamethasone was injected for anti-inflammatory treatment of cerebral edema (0.2–0.05 mg kg−1, subcutaneously, once a day for 3 days). The animals were allowed to recover for one or two weeks. All animal procedures were approved by the Zhejiang University Animal Care and Use Committee and the rules of the administration of affairs concerning experimental animals (1988) were followed.
2.3. Odor preparation and stimulation experiments
In this research, all odor reagents were purchased from Sigma-Aldrich (Shanghai) Trading Co., Ltd. For odor-masking evaluation, isovalerate, valeric acid, and butyric acid were set as the aversive odor group, while citral, beta-damascenone, and benzaldehyde were set as the masking agent group (Table 1).38 Mineral oil was used as a control odor. Odorant substances were independently dissolved in mineral oil at concentrations of 10−2 M, 10−3 M, and 10−4 M. Each odor solution was held in a 1.5 mL brown glass sample bottle. In a session, there were 4 stimuli groups including control, aversive odor, masking stimuli, and mixture groups. There were 20 trials in one session. The interval time of stimuli was 60 s to avoid odor adaptation. During a trial, an odor bottle containing 500 μL solution was placed about 1 cm in front of the nose, and it lasted 5 s for odor stimulation. The rat was under the anesthesia state in all stimulation experiments.
Table 1 Abbreviations and descriptions of odors presented in the study
Odor |
Abbreviations |
Descriptions |
Benzaldehyde |
BZA |
Bitter almond |
Butyric acid |
BTA |
Unpleasant, rancid |
Citral |
CIT |
Lemon-like odor |
Isovaleric acid |
IVA |
Penetrating odor |
Valeric acid |
VRA |
Unpleasant, penetrating |
β-Damascenone |
DMO |
Floral, fruity |
2.4. Data process
Spike train data were analyzed and processed offline in MATLAB (R2019b, Mathworks, USA) and Excel (version 2016, Microsoft Co., Ltd, USA). The spike signal was semi-automatically obtained by a double threshold method using the Offline Sorter (Plexon, Inc., USA). In this research, the PCA was used for dimension-reduction of the spike waveform data, and K-means was used to perform spike signal clustering to distinguish the neurons collected in each channel. The signal-to-noise ratio (SNR) of the spike signal was calculated using eqn (1). |  | (1) |
where σS and σN are the standard deviations of spikes and noise, respectively.
Linear decomposition was used for analyzing the difference of spatial response patterns of M/T cells evoked by the aversive odor, masking agent, and mixture groups.18 A model of linear decomposition is shown in Fig. 1d. The M/T cell response evoked by the odor was described with a change in the average firing rate between the stages before and during odor stimulation, defined as Δf in eqn (2). The response pattern was expressed by an n-dimensional column vector of the combination response of the M/T cell group, and n was equal to the number of M/T cells. A pattern of a masking agent is defined as
, while masked odor is defined as
.
denotes a pattern of mixture groups (eqn (3)). a and b in eqn (3) denote the projection coefficient on
and
, respectively. The n-dimensional column vector
in eqn (3)–(5), representing a different pattern between
and other groups, was perpendicular to the plane formed by
and
. The symbols
, a, and b were derived from eqn (3)–(5). Im, the intensity of odor masking, was calculated using eqn (6) for the quantitative evaluation of odor masking.
|  | (2) |
|  | (3) |
|  | (4) |
|  | (5) |
| 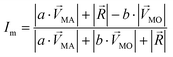 | (6) |
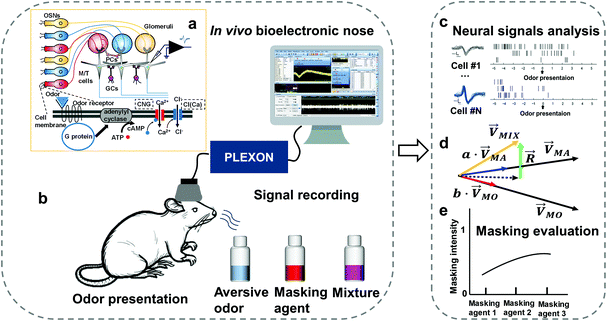 |
| Fig. 1 An odor masking evaluation system based on an in vivo bio-eNose. (a) Schematic of a G-protein coupling pathway of an odor receptor in the cilia of OSNs and the basic circuit diagram of the organization of neurons in the OE and OB. (b) The system consists of a rat, a neural signal acquisition device, and odor delivery modules. (c) Spike signal from M/T cells in the OB was sorted out by different waveforms. (d) Vector space decomposition model for quantifying the difference in multiple neural response patterns of the in vivo bio-eNose to the odorant and their binary mixture. (e) Evaluation of the masking efficiency of several masking agents. | |
2.5. Statistical analysis
All results and error bars were presented as the means ± SEM. Prism 8.0 (GraphPad, USA) was used for statistical analysis. A one-way ANOVA test was performed to examine significant differences between all groups. Statistical significance was accepted at p < 0.05. In all statistical graphs, ns = no significant, *p < 0.05, **p < 0.005, ***p < 0.0005, and ****p < 0.0001.
2.6. Histological verification
The brain of the rat was removed from the cranium following cardiac perfusion with physiological saline and 4% paraformaldehyde solution (PFA) in PBS. The brain was stored in 4% PFA at 4 °C overnight followed by continuous dehydration in 10%, 15%, and 20% sucrose in PBS at 4 °C. Before the frozen section, the brain was embedded in an OCT (optimal cutting temperature) compound. 26 μm coronal sections were obtained and mounted onto glass slides with a cryostat (Minux FS800A, RWD Inc., China). The tissue sections were stained by the Nissl method (C0117, Beyotime Biotechnology Inc., China). The electrode sites were observed under a light microscope.
3. Results and discussion
3.1. Construction of an odor masking evaluation system based on an in vivo bio-eNose
The in vivo bio-eNose odor masking system was composed of rats, an implanted microwire electrode, and a neural signal acquisition device (Fig. 1). The in vivo bio-eNose was used to detect response patterns to odorants and binary mixtures. Through the vector space decomposition method, decomposition components of the response pattern caused by the binary mixture were extracted to further evaluate and quantify the masking intensity of the masking agent to the masked odor. To realize the system, a microwire electrode was implanted in the olfactory bulb area that responds to the masked odor. The rat olfactory bulb exhibits regionalization of the odor response.29–31 Glomeruli respond to the fatty acids and aldehydes that were explored in this study and are located in the cluster A area of the rat olfactory bulb. The area is distributed in the anterior, middle, and lateral parts of OB.19 M/T cells receive inputs from glomeruli and can be detected by electrodes (Fig. 1a). Therefore, M/T cells in the anteromedial part of the OB served as outputs of the in vivo bio-eNose in this study.
We first mimicked the tetrode insertion using 0.6% agarose gels as a model for brain tissue.32Fig. 2a shows that the tetrodes were inserted into the gel without any visible deformation of the microelectrode. Microwire tetrodes were arranged on both sides of a custom-made PCB in a 2 × 2 arrangement. The distance between the tips of adjacent electrodes was about 200 μm (Fig. 2b). Then the tetrodes were used to obtain as much signal of multiple neurons as possible in the local area. A spike signal with a signal-to-noise ratio (SNR) larger than 4 (Fig. 2c and d), collected one week after the rat recovery, was appropriate for the analysis of odor response features without complex pattern recognition algorithms. It could be divided into three clusters by PCA and K-means methods (Fig. 2e). To test the long-time stability of the in vivo bio-eNose, the SNR of the signals recorded from day 2 to day 28 was calculated (Fig. 2f), and the result showed that the SNR was still greater than 4 on the 28th day. Thus the system could be reused for nearly a month. It is expected to further increase the usable time of the system through electrode surface modification and inflammation treatment.33,34
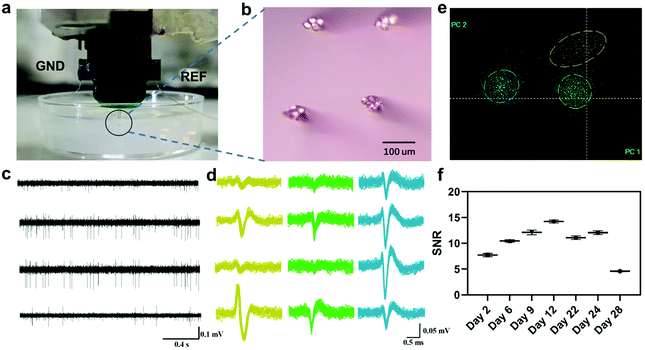 |
| Fig. 2 Neural signal extraction and quality test. (a) Homemade 4 × 4 micro-wire tetrodes inserted into 0.6% agarose gels. GND, ground wire; REF, reference wire. (b) The bottom view of the electrode showed a tip arrangement. Bar = 100 um. (c) A neural signal was extracted and low-frequency signals were filtered out from a tetrode. (d) Spike waveforms and (e) the PCA feature clustering graph of three neurons sorted from the signal shown in (c), respectively. (f) Signal-to-noise ratios of the neuron signal for different days (n = 3). | |
3.2. Response patterns of the odor masking evaluation system to monomolecular substances and binary mixtures
3.2.1. Verification of the odor response properties of the implantation area.
To explore the accuracy of implantation in a specific area that was sensitive to fatty acids, ketones, and aldehydes reported by previous studies,29 the system was tested with BZA, IVA, CIT, and DMO. Table 2 shows the statistics of the proportion of M/T cells sensitive to different odorants. Neurons that responded to benzaldehyde were observed in three rats with the electrode implanted in the anteromedial side of the OB. Meanwhile, in rat #2, the proportion of neurons responding to citral was up to 50%, while DMO and IVA both accounted for 40%. The site position was confirmed by histology (Fig. S2†). In general, it was possible for the zone with an electrode implanted to acquire a response signal induced by fatty acids, ketones, and aldehydes, and the results we obtained at the implantation site were consistent with those from previous studies.19,29
Table 2 Odor responses of the in vivo bio-eNose implanted in the anteromedial olfactory bulb
|
Rat #1 |
Rat #2 |
Rat #3 |
Mean |
BZA |
75% |
70% |
30% |
58% |
IVA |
0 |
40% |
25% |
22% |
CIT |
0 |
50% |
32% |
27% |
DMO |
0 |
40% |
23% |
21% |
3.2.2. Response properties of M/T cells activated by monomolecular odorants and binary mixtures.
This study mainly evaluated the masking effect between the two odors. It explored the difference between the response of the binary mixture to M/T cells and the independent stimulation of the two components. There were mainly three response patterns found in the study, including activation (Fig. 3a), inhibition (Fig. S3b† subgraph in the red rectangle) and no response. We firstly examined the response properties of M/T cells to odorants and binary mixtures. Fig. 3a shows the spike trains from the M/T cell of each trial during odor application to the in vivo bio-eNose, which explained how the benzaldehyde-selective neuron responded to other odors. In the case of a single odor, compared with the control group, the neuron was significantly activated by benzaldehyde, while other odors did not significantly activate the cell (Fig. 3b). However, in the case of binary mixtures containing benzaldehyde, the response of the IVA + BZA group was significantly higher than that of the BZA group, while the BTA + BZA group showed a decreasing trend (Fig. 3a and c). The former response was considered an enhancement, and the latter was a suppression response. The mixture of benzaldehyde and isovaleric acid produced a synergistic response, which may be caused by their similar response area in the OB.29
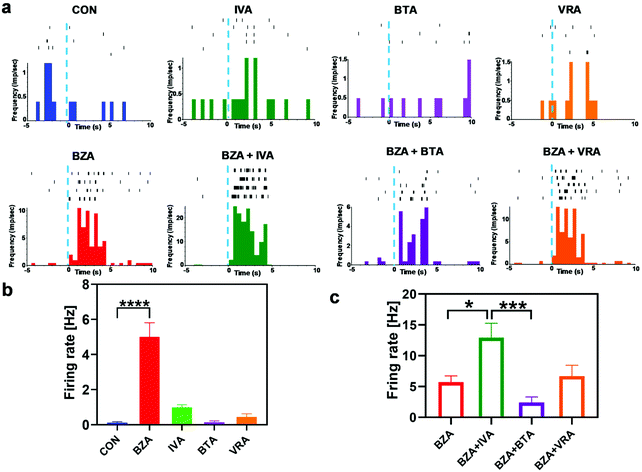 |
| Fig. 3 Response patterns of a BZA-responsive neuron to fatty acids, aldehydes, and binary mixtures containing BZA. (a) Raster plots of spike trains during odor application to the M/T cell of each trial. Peristimulus time histogram (PSTH) showing the average response under the raster plot of each group (bin = 500 ms). Blue dotted lines indicate the beginning time of odor stimulation. (b) and (c) Statistical plots of the firing frequency of the M/T cells responding to odorants and binary mixtures containing BZA, respectively (n = 10). | |
In addition, we also examined the response property of IVA-sensitive neurons induced by the stimulation of monomolecular odors and binary mixtures (Fig. 4). Cell #1 showed high specificity to IVA. Under the stimulation of BZA, CIT, and DMO, the response of cell #1 was not significantly different from that of the control group (Fig. 4a). Cell #2 was also significantly activated by benzaldehyde and citral except for DMO (Fig. 4b). Under the stimulation of the binary mixtures containing IVA, both cells showed inhibition responses. The response of cell #1 was suppressed by BZA and DMO groups (Fig. 4c), while the response of cell #2 was significantly inhibited by CIT and DMO groups (Fig. 4d).
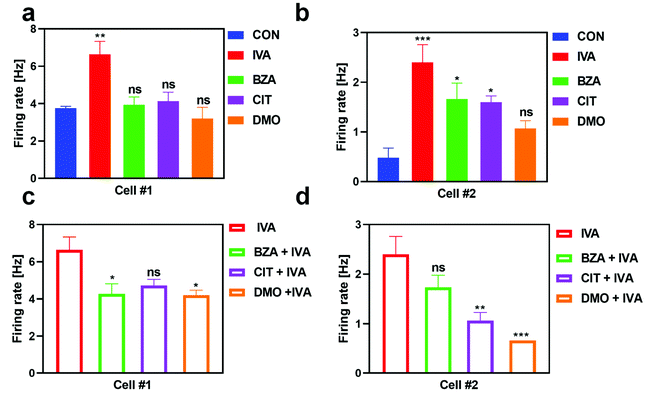 |
| Fig. 4 IVA-responsive neurons respond to odorants and binary mixtures containing IVA. (a) and (b) Statistical results of the firing frequency of cell #1 and cell #2 during the stimulation of odorants (n = 10), respectively. (c) and (d) A statistical graph of the firing frequency of cell #1 and cell #2 stimulated by binary mixtures containing isovaleric acid (n = 10). | |
The experimental results demonstrated that neurons activated by the specific odor had different response patterns such as suppression and enhancement to the binary mixture containing the masked odor. This phenomenon has also been observed in the OE by SCAPE microscopy. Several modulation mechanisms occurred at the receptor level including agonism, partial agonism, and enhancement.22 Odor masking might occur in the OE by receptor modulation or in the OB by lateral inhibition. M/T cells, as the major output neurons in the OB, not only convey the information from the OE and glomerular cells, but also receive lateral inhibition inputs from PCs and GCs. They have great potential to be used for masking effect evaluation because they contain more information than previous inputs. The information of odor could be represented as an N-dimensional vector of M/T cells in the OB, and N is the number of total M/T cells.35 Since the properties of distributing M/T cells from the same glomerular cells are similar, the number of M/T cells for odor coding is smaller than N,36 which makes it possible to extract masking information from the response patterns of a limited number of M/T cells.
3.3. Quantification of the intensity of odor masking
To quantitatively evaluate the masking intensity, we used the linear decomposition method to analyze the average changing firing rate of multiple neurons evoked by individual odor and binary mixtures. Fig. 5a shows the model of linear decomposition. The vector
is an n-dimensional vector composed of the average changing firing rate Δf of n M/T cells from the electrodes near odor 1 sensitive neurons.
and
, respectively, represent the n-dimensional vector composed of the response patterns of odor 2 and the binary mixture containing both odors. The three vectors were all scaled according to the magnitude of
, so that
was unitized.
was perpendicular to the plane where the vectors
and
were located, and was taken advantage of for measuring the difference between the mixture response and the spatial patterns of monomolecular odor responses.
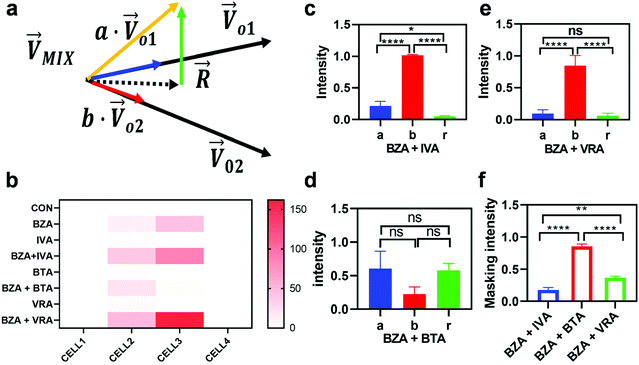 |
| Fig. 5 Feature extraction and quantification of odor response patterns. (a) A model of linear decomposition illustrating the decomposition of the binary mixture pattern on the directions of odorant patterns and another component. (b) Heat map of odor response patterns of four neurons. (c), (d), and (e) respectively represent the statistical graphs of IVA, BTA, and VRA groups of parameters a, b, and r obtained by the decomposition of the binary mixture containing BZA (n = 10). (f) The statistical analysis result of masking intensity shows the masking ability of three substances to BZA (n = 10). | |
We then studied the masking intensity of 10−4 M IVA, 10−4 M VRA, and 10−4 M BTA to 10−2 M BZA. Here, BZA is denoted as
, other odorants are denoted as
, and the binary mixtures containing BZA are denoted as
. Four M/T cells from one tetrode were used for the quantitative analysis, and the heat map of the cell population response is shown in Fig. 5b. Among the four cells, cell #2 and cell #3 could be significantly activated by the BZA group, while the BZA + BRA group showed the inhibition of cell #3, making the neuron firing pattern of this group different from those of other groups. We used the linear decomposition method to extract the feature parameters of the spatial response pattern of this group of the M/T cell population. Since the spatial response pattern to the BZA + IVA and BZA + VA groups was similar to that of the BZA group, the component intensity
was significantly higher than the component intensity in the other individual odor group
and the component intensity in the
direction
(Fig. 5c and e). There was no significant difference in the intensity of the spatial response patterns of the BZA + BTA group in three directions (Fig. 5d). Then, the masking intensity indexes of other odors to BZA were calculated according to eqn (6), and the results showed that the masking intensity index of BTA to BZA was higher than those of the other two groups, and the VRA group was higher than the IVA group (Fig. 5f). This was consistent with the results shown in the heat map (Fig. 5b).
3.4. Comparison of the odor masking results of the in vivo biological electronic nose and human sensory evaluation
Furthermore, we used the in vivo bio-eNose to evaluate the masking intensity of the odorants that have been reported in the human sensory evaluation.5 We explored the masking intensity values of 10−3 M BZA, 10−3 M CIT, and 10−3 M DMO to 10−4 M IVA. Then we compared them with the results of the human evaluation score. For humans, the odor masking score (0–5) of BZA, CIT, and DMO to IVA was 4.6, 3.9, and 2.4, respectively. The average changing firing rates of M/T cells from the electrodes near the IVA-sensitivity neurons were selected as the output parameters of the system. Fig. 6a shows the response heat map of the odor masking system to individual odorants and binary mixtures containing IVA.
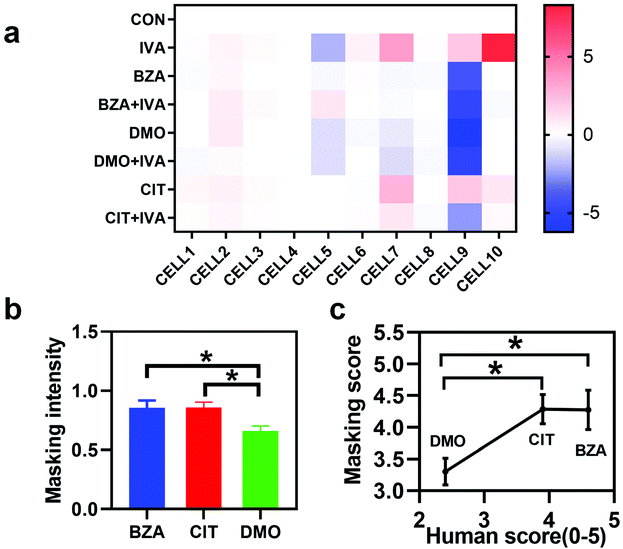 |
| Fig. 6 Comparison of the evaluation results of the in vivo bio-eNose based odor masking system with the human olfaction sensory system. (a) Response heat map of 10 neurons to odorants and binary mixtures containing isovaleric acid (n = 5). (b) The statistical analysis result of masking intensity shows the masking efficiency of three substances to IVA. (c) Comparison of human evaluation masking scores of IVA with the converted masking intensity value of the in vivo bio-eNose (n = 10). | |
Then we used the linear decomposition quantification method proposed in section 3.3, and the masking intensity of each odorant is shown in Fig. 6b. To be consistent with the human perception evaluation range, the calculated masking intensity value (0–1) was linearly scaled to the range of 0–5 (Fig. 6c). According to the comparison of masking evaluation results of the in vivo bio-eNose and human score, it was found that the result of the in vivo bio-eNose odor masking evaluation system was positively correlated with human sensory evaluation (Pearson correlation coefficient, r = 0.9466). Besides, the evaluation system showed that the masking scores of CIT and BZA were significantly higher than that of DMO. However, there was no difference in the masking intensity between BZA and CIT. Soh et al. have analyzed the degree of overlap in the response of rat's olfactory glomerular cells to similar monomolecular odorants as well as compound odors (such as isoamyl acetate and banana) and combined with human sensory evaluation experiments to explore the differences in odor perception between rats and humans.37 It showed that the response patterns of rats’ glomeruli were highly correlated with the human perception of odor similarity (r = 0.65–0.79),37 which proves that there is a partial similarity of olfactory perception between humans and rats. Combined with our results in this section, the in vivo bio-eNose mentioned in this study is capable of quantitatively assessing the odor masking effect using the spatial response patterns of M/T cells, and it could assist in the development of novel and efficient masking agents.
4. Conclusions
In this study, we developed an odor masking evaluation and quantification system based on the in vivo bio-eNose technology. The linear decomposition method was used to extract the feature parameters of the spatial response pattern of the M/T cell population in the rat's olfactory bulb to monomolecular odors and binary mixtures. Finally, masking intensity values were calculated according to the feature parameters. They were applied to quantitatively measure the degree of interference of one odorant to another in the biological olfactory perception system. Notably, the masking intensity trend obtained with this system has a positive correlation with the human olfactory evaluation results. Therefore, the results indicate that this system has the potential to assist in the development of new masking agents or flavored foods in odor and food companies. Furthermore, it is expected to be used to quantitatively compare the differences in odor perception between humans and rats to study the differences in chemical perception between different species.
Authorship contributions
Qunchen Yuan: conceptualization, investigation, methodology, data curation, formal analysis, validation, visualization, writing – original draft, and writing – review and editing; Chunlian Qin: methodology, data curation, formal analysis, validation, and writing – review and editing; Yan Duan: methodology, data curation, and writing – review and editing; Nan Jiang: formal analysis, validation, and writing – review and editing; Mengxue Liu: data curation, validation, and writing – review and editing; Hao Wan: data curation, validation, writing – review & editing; Liujing Zhuang: conceptualization, writing – review and editing, supervision, and project administration; Ping Wang: conceptualization, writing – original draft, writing – review and editing, supervision, project administration, and funding acquisition.
Conflicts of interest
The authors declare no conflict of interest, financial or otherwise.
Acknowledgements
This work was supported by the National Natural Science Foundation of China (No. 31627801 and 31800826), National Key Research and Development Program of China (No. 2020AAA0105901), the Natural Science Foundation of Zhejiang Province (No. LGF19H180022, LBY21H180001 and LY21C100001), and the China Postdoctoral Science Foundation Funded Project (No. 2020M671728 and 2021T140605).
References
- W. S. Cain and M. Drexler, Ann. N. Y. Acad. Sci., 1974, 237, 427–439 CrossRef CAS PubMed
.
- W. S. Cain, Chem. Senses, 1975, 1, 339–352 CrossRef CAS
.
- K. Mori, H. Nagao and Y. Yoshihara, Science, 1999, 286, 711–715 CrossRef CAS PubMed
.
- T. Kurahashi, G. Lowe and G. Gold, Science, 1994, 265, 118–120 CrossRef CAS PubMed
.
- H. Takeuchi, H. Ishida, S. Hikichi and T. Kurahashi, J. Gen. Physiol., 2009, 133, 583–601 CrossRef CAS PubMed
.
- Y. K. Takahashi, S. Nagayama and K. Mori, J. Neurosci., 2004, 24, 8690–8694 CrossRef CAS PubMed
.
- D. Rokni, V. Hemmelder, V. Kapoor and V. N. Murthy, Nat. Neurosci., 2014, 17, 1225–1232 CrossRef CAS PubMed
.
- M. Hakim, A. R. Battle, A. Belmer, S. E. Bartlett, L. R. Johnson and F. Chehrehasa, Front. Mol. Neurosci., 2019, 12, 211 CrossRef PubMed
.
- Z. Pan, S. R. K. Kjaergaard and L. M. Lhave, Int. Arch. Occup. Environ. Health, 2003, 76, 598–604 CrossRef PubMed
.
- Y. Hirasawa, M. Shirasu, M. Okamoto and K. Touhara, Psychoneuroendocrinology, 2019, 106, 206–215 CrossRef PubMed
.
- A. Haehner, H. Maass, I. Croy and T. Hummel, Flavour Fragrance J., 2017, 32, 24–28 CrossRef
.
- K.-H. Kim, Sensors, 2010, 10, 7287–7302 CrossRef PubMed
.
- K.-H. Kim and S.-Y. Park, Atmos. Environ., 2008, 42, 5061–5070 CrossRef CAS
.
- D. G. Laing and M. E. Willcox, Chem. Senses, 1983, 7, 249–264 CrossRef CAS
.
- S. Abdikheibari, H.-M. Song, J.-I. Cho, S.-J. Kim, S.-C. Gwon, K. Park, B. Maluleque, N. Marleni, L. Shu and V. Jegatheesan, Int. Biodeterior. Biodegrad., 2016, 106, 25–33 CrossRef CAS
.
- P. Lewkowska, B. Cieślik, T. Dymerski, P. Konieczka and J. Namieśnik, Environ. Res., 2016, 151, 573–586 CrossRef CAS PubMed
.
- Y. Niu, P. Wang, Z. Xiao, J. Zhu, X. Sun and R. Wang, Food Chem., 2019, 275, 143–153 CrossRef CAS PubMed
.
- P. Giraudet, F. Berthommier and M. Chaput, J. Neurophysiol., 2002, 88, 829–838 CrossRef PubMed
.
- Y. K. Takahashi, M. Kurosaki, S. Hirono and K. Mori, J. Neurophysiol., 2004, 92, 2413–2427 CrossRef CAS PubMed
.
- K. Osada, M. Hanawa, K. Tsunoda and H. Izumi, Chem. Senses, 2012, 38, 57–65 CrossRef PubMed
.
- S. Inagaki, R. Iwata, M. Iwamoto and T. Imai, Cell Rep., 2020, 31, 107814 CrossRef CAS PubMed
.
- L. Xu, W. Li, V. Voleti, D.-J. Zou, E. M. C. Hillman and S. Firestein, Science, 2020, 368, eaaz5390 CrossRef CAS PubMed
.
- L. Zhuang, X. Wei, N. Jiang, Q. Yuan, C. Qin, D. Jiang, M. Liu, Y. Zhang and P. Wang, Biosens. Bioelectron., 2021, 171, 112737 CrossRef CAS PubMed
.
- L. Zhuang, T. Guo, D. Cao, L. Ling, K. Su, N. Hu and P. Wang, Biosens. Bioelectron., 2015, 67, 694–699 CrossRef CAS PubMed
.
- T. Guo, L. Zhuang, Z. Qin, B. Zhang, N. Hu and P. Wang, Sens. Actuators, B, 2016, 225, 34–41 CrossRef CAS
.
- K. Gao, S. Li, L. Zhuang, Z. Qin, B. Zhang, L. Huang and P. Wang, Biosens. Bioelectron., 2018, 102, 150–156 CrossRef CAS PubMed
.
- Z. Qin, B. Zhang, L. Hu, L. Zhuang, N. Hu and P. Wang, Biosens. Bioelectron., 2016, 78, 374–380 CrossRef CAS PubMed
.
- Z. Qin, B. Zhang, K. Gao, L. Zhuang, N. Hu and P. Wang, Sens. Actuators, B, 2017, 239, 746–753 CrossRef CAS
.
- N. Uchida, K. Mori, Y. K. Takahashi and M. Tanifuji, Nat. Neurosci., 2000, 3, 1035–1043 CrossRef CAS PubMed
.
- B. A. Johnson, Z. Xu, S. S. Ali and M. Leon, J. Comp. Neurol., 2009, 514, 658–673 CrossRef CAS PubMed
.
- H. Matsumoto, K. Kobayakawa, R. Kobayakawa, T. Tashiro, K. Mori, H. Sakano and K. Mori, J. Neurophysiol., 2010, 103, 3490–3500 CrossRef CAS PubMed
.
- Z.-J. Chen, G. T. Gillies, W. C. Broaddus, S. S. Prabhu, H. Fillmore, R. M. Mitchell, F. D. Corwin and P. P. Fatouros, J. Neurosurg., 2004, 101, 314–322 Search PubMed
.
- J. E. Ferguson, C. Boldt and A. D. Redish, Sens. Actuators, A, 2009, 156, 388–393 CrossRef CAS PubMed
.
- J. W. Salatino, K. A. Ludwig, T. D. Kozai and E. K. Purcell, Nat. Biomed. Eng., 2017, 1, 862–877 CrossRef CAS PubMed
.
- G. Laurent, Nat. Rev. Neurosci., 2002, 3, 884–895 CrossRef CAS PubMed
.
- T. W. Chen, B. J. Lin and D. Schild, Proc. Natl. Acad. Sci. U. S. A., 2009, 106, 2401–2406 CrossRef CAS PubMed
.
- Z. Soh, M. Saito, Y. Kurita, N. Takiguchi, H. Ohtake and T. Tsuji, Chem. Senses, 2014, 39, 91–105 CrossRef PubMed
.
- S. Kim, J. Chen, T. Cheng, A Gindulyte, J. He, S. He, Q. Li, B. A. Shoemaker, P. A. Thiessen and B. Yu, Nucleic Acids Res., 2021, 49, D1388–D1395 CrossRef PubMed
.
-
G. Paxinos and C. Watson, The rat brain in stereotaxic coordinates: hard cover edition, Elsevier, 2006 Search PubMed
.
Footnote |
† Electronic supplementary information (ESI) available. See DOI: 10.1039/d1an01569a |
|
This journal is © The Royal Society of Chemistry 2022 |