DOI:
10.1039/D0RA07796H
(Paper)
RSC Adv., 2020,
10, 43994-44002
Random acceleration and steered molecular dynamics simulations reveal the (un)binding tunnels in adenosine deaminase and critical residues in tunnels†
Received
11th September 2020
, Accepted 27th November 2020
First published on 11th December 2020
Abstract
Adenosine deaminase (ADA) is an important enzyme related to purine nucleoside metabolism in human serum and various tissues. Abnormal ADA levels are related to a wide variety of diseases such as rheumatoid arthritis, AIDS, anemia, lymphoma, and leukemia and ADA is considered as a useful target for various diseases. Currently, ADA can be divided into open conformation and closed conformation according to the inhibitors of binding. As a consequence, we chose two inhibitors, namely, 6-hydroxy-1,6-dihydro purine nucleoside (PRH) and N-[4,5-bis(4-hydroxyphenyl)-1,3-thiazol-2-yl]hexanamide (FRK) to bind to ADA in the closed conformation or open conformation respectively. In this study, we performed the random acceleration molecular dynamics (RAMD) method, steered molecular dynamics (SMD) simulations and adaptive basing force (ABF) simulation to explore the unbinding tunnels and tunnel characteristics of the two inhibitors in ADA. Our results showed that PRH and FRK escaped from ADA using three main tunnels (namely, T1, T2, and T3). Inhibitors (PRH and FRK) escape through T3 more frequently and more easily. The results from ABF simulations confirm that the free energy barrier in T1 or T2 is larger than that in T3 when inhibitors dissociate from the ADA and have potential mean of force (PMF) depth. Moreover, in the complexes (ADA-PRH, ADA-FRK), we also found that the most active residue that remarkably contributed to the binding affinity is W117 in T3, and the residue played an important role in the unbinding tunnel for inhibitor leaving. Our theoretical study provided insight into the ADA inhibitor passway mechanism and may be a clue for potent ADA inhibitor design.
Introduction
Adenosine deaminase (ADA; EC 3.5.4.4) is an important enzyme in purine metabolism. ADA is widely distributed in various tissues of the human body and catalyzes the irreversible deamination of adenosine to inosine.1–5 ADA deficiency is related to various immune deficiency (SCID) diseases with profound depletion of T, B, and natural killer cell lineages, such as rheumatoid arthritis, an autoimmune disease in the body's immune system that mistakenly attacks the joints,1–5 and cancer.6–9 ADA inhibitors may change the concentration of adenosine specifically and may act as an anti-inflammatory, anti-HIV and anti-cancer drug, however, usually they have several side effects.10
ADA inhibitors are classified into two types: transition- and ground-state inhibitors.11,12 Transition-state inhibitors of ADA resemble a tetrahedral transition-state intermediate as a result of deamination.11 Ground-state inhibitors of ADA are similar to adenosine. Furthermore, these two types of inhibitors are met with a number of problems, such as toxicity, poor oral absorption, and rapid metabolism.13,14 ADA has two distinct conformations: closed and open forms, which bind to two types of inhibitors.15,16 The structural difference is due to an inhibitor-induced conformation change (Fig. 1a and b).
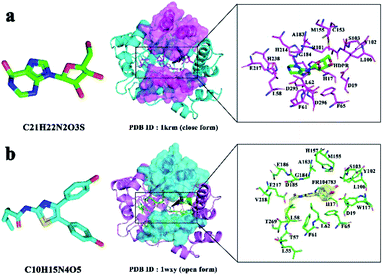 |
| Fig. 1 (a) Active residues in the ADA-PRH complex (PDB Id 1KRM). (b) Active residues in the ADA-FRK complex (PDB Id 1WXY). | |
Biological macromolecular channels are closely related to the biological function and structural stability of macromolecules and have important structural significance.17 In some enzymes the active site is relatively exposed to the surface, while in others the active site is deep in the core of the protein. For enzymes that hide the active site, the substrate must pass through a series of tunnels to bind to the active site, a structure that allows more potential protein–ligand interactions to occur than proteins that surface expose the active site. Structurally, an underground active site accessed by one or more tunnels increases the complexity of ligand binding or dissociation. Similarly, the dissociation of inhibitors of enzymes increases their complexity, and through the analysis of protein tunnels, we hope to obtain more effective and safe inhibitors. The active site residues and catalytic mechanism of ADA have been well studied, but the number and characteristics of the tunnels connecting the active site to the protein surface have not been widely characterized.
In the early days, drug development, selection, and optimization were mainly based on equilibrium thermodynamic constants such as KD or IC50 values, which to some extent demonstrated the affinity between compounds and the target, but failed to correctly evaluate the in vivo efficacy of the compounds, resulting in the failure of many compound development in phase II clinical trials. Residence time (RT) is an important parameter related to the safety and efficacy of drugs in vivo.18 The best drug candidate is determined by determining or adjusting the kinetic rate associated with RT.
Over the last few decades, computer-aided drug design has emerged to be a powerful technique playing a crucial role in the development of new drug molecules with less time and money.19 Herein, computer-aided drug design can be used to explore the interactions between ADA and different inhibitors, where it is a useful way to get new excellent inhibitors with low toxicity to regulate the function of ADA.
In this study, we used the RAMD method and SMD simulations to explore the inhibitor unbinding tunnels in ADA and each tunnel's crucial residues. Our theoretical results may suggest a clue for ADA investigation.
Materials and methods
Protein preparation
We used two kinds of inhibitors combined with ADA, namely, 6-hydroxy-1,6-dihydro purine nucleoside (PRH) and N-[4,5-bis(4-hydroxyphenyl)-1,3-thiazol-2-yl]hexanamide (FRK). The initial structure of complex in the RAMD simulations was obtained from the RCSB Protein Data Bank (PDB Id 1KRM and 1WXY)16,20 (Fig. 1a and b). In 1KRM, ADA is presented in a closed form combined with the inhibitor PRH.20 In 1WXY, ADA is presented in an open form combined with the inhibitor FRK.16
Random acceleration molecular dynamics (RAMD) and reaction coordinates
RAMD was created to simplify and facilitate the simulation process and improve the accuracy and reliability of simulation results.21,22 RAMD is an excellent approach for determining the possible tunnels of a protein, and this technique has been applied to identify new tunnels in many systems.22–26 RAMD is improved on the basis of conventional simulation and steered molecular dynamics simulation. It applies force in either direction of the ligand to see whether the ligand can be shifted under the action of force. The force exerted on the ligand is expressed as:
where k is a constant; r0 is a unit vector to any direction.
where m is the number of steps, each force applied to the ligand molecule will maintain m steps, vmin is the minimum ligand velocity under this force, Δt is the time required for a step, rmin represents the minimum displacement of ligand motion in this direction. After m steps, if the average velocity of ligand movement is less than vmin the direction of force will change, and then it will move in a new direction.
Two complexes were used in NAnoscale Molecular Dynamics (NAMD) 2.10b1 version software using the CHARMM36 all-hydrogen force field.27–29 In our initial simulations, m = 10, 20, 30, 40; acceleration = 0.005, 0.01, 0.02, 0.03, 0.04, 0.05, 0.06, 0.08, 0.1; and rmin = 0.0005, 0.001, 0.002, 0.005, 0.008, 0.01, 0.02. In order to get better parameters, every combination of the parameters was simulated for 1 ns, and we chose the combination in which the inhibitor travelled in the protein for more than 30 ps. Eventually, we used the following parameters: m = 20; rmin = 0.005; and acceleration = 0.05 successfully ran 300 simulations for each inhibitor. All the results were visualized with Visual Molecular Dynamics (VMD) version 1.9.1.
Steered molecular dynamics (SMD) simulation
SMD is a simulation method for studying ligand (substrate and inhibitor) binding and separation, as well as some mechanical and energy properties in the simulation process.29 In the simulation of SMD, a time-dependent external force is applied to the ligand, exerting force on the ligand in the set direction, the ligand is finally separated from the receptor. The mechanism of action between ligand and receptor channels can be accurately judged by the time of action, the magnitude of the force, the energy required and the work done. Formula is as follows:
where the k is the elastic constants,
is controlled atomic displacement relative to its initial state, t is the time the action of forces.
The two complexes were used in NAMD 2.10b1 version software by using the CHARMM36 all-atom force field.27–29 Constant-velocity SMD simulations were performed in the present simulations. The pulling velocity was set to 0.01 Å·ps−1. A spring constant of 0.2 kcal mol−1 Å−2 was applied to the inhibitor's centre of mass. SMD simulations were performed from the snapshot structure at 10 ns. To obtain general results and eliminate contingency, we repeatedly stretched each tunnel for three times. The optimal tensile track in parallel experiments in each tunnel was selected for subsequent analysis of tensile force and tunnel residues, and we computed the maximum force for the inhibitor through the unbinding pathway.
Potential of mean force (PMF) with the adaptive-biasing-force (ABF) method
Potential of mean force (PMF) can characterize the substrate's free energy along it, which dictates the speed of transport and the selectivity. Park et al. have proposed a PMF reconstruction method from a limited number of SMD simulation trajectories for a channel system, which used the cumulated expansion to construct the PMF in this study. Formula is as follows:30,31
where σw2 = 〈W2〉 − 〈W〉2, kB is the Boltzmann constant, T is the temperature. In principle, PMF depth, ΔGoff is inversely proportional to koff and that is to say it is proportional to residence time (RT). The dissociation rate constant (koff) is the constant of the dissociation rate between the reaction ligand and the protein, and the reciprocal residual time (RT) is an important kinetic parameter that directly characterizes the interaction time between the drug molecule and the target protein, which is closely related to the efficacy of the drug. In general, a drug candidate may be more promising if it has a longer RT.
Adaptive biasing forces (ABF) can generate quasi-equilibrium trajectories from which the PMF can be deduced. Comparing with other several classic method like umbrella sampling (US), ABF has obvious advantages: ABF introduces the concept of bias force, greatly improves the sampling efficiency, and we don't need to estimate the shape of the free energy barrier in advance and height, also don't need to overlap between adjacent Windows. In this way, the error caused by weight addition and so on will not appear.
We choose the atom distance which also used in SMD to complete the ABF-PMF simulations. To enhance the efficiency of the ABF algorithm, the span of the reaction coordinate will be subdivided into equally spaced windows.
Results and discussions
Multiple unbinding pathways identified by RAMD simulations
RAMD, which is an effective approach for identifying the possible tunnel of macromolecules containing protein, has also been successfully applied in many systems.22–26,32 This study identified the unbinding pathways of two inhibitors (PRH and FRK) from ADA. For the two complexes, 300 simulations were performed to determine the trajectory and observe the unbinding pathway of the inhibitor with VMD. Table 1 shows the number of times each tunnel appears. Three main tunnels (T1–T3) were found in the ADA-inhibitor complex. These three tunnels most likely serve as the unbinding tunnel for inhibitors. Among them, T3 had higher occurrence frequencies for inhibitors unbinding. According to the basic principle of RAMD, we calculated the percentage of occurrences of each tunnel. The unbinding frequencies of T1–T3 in the ADA-PRH system were 17.3%, 17.3%, and 65.3%, respectively, whereas those in the ADA-FRK system were 21.3%, 23.0%, and 55.7%, respectively (Fig. 2a and b). In the ADA-inhibitor systems, T3 had the highest unbinding ratio both in the ADA-PRH and APH-FRK complex. Hence, T3 was the most favourable tunnel for PRH and FRK escape. T2 and T1 were few observed in the simulations.
Table 1 Summary of the RAMD simulations of ADA-PRH and ADA-FRK
Systems |
T1 |
T2 |
T3 |
ADA-PRH |
52 |
52 |
196 |
ADA-FRK |
64 |
69 |
167 |
 |
| Fig. 2 (a) Inhibitor unbinding pathways from ADA as revealed by RAMD simulations. (b) Egress frequency via each tunnel; the upper panel is ADA-PRH, and the lower panel is ADA-FRK. | |
Tunnel properties on the basis of SMD simulations
According to the result of RAMD, we can see the direction of each tunnel. In order to determine the tunnels direction, the vector direction was determined by taking a point on each protein and center of ligand. After repeated stretching direction confirmation, we finally determined the exact direction of the stretch. In the ADA-PRH complex, the traction direction of T1–T3 is set at the initial location of the ligand in the active site and the centre of the Cα atoms of the residues, Leu137, His17 and Val 16, respectively. In ADA-FRK complex, the directions were set at the inhibitor in the active site and the centre of the Cα atoms of the residues, Tyr102, His17, and Asp296, respectively. We conducted SMD simulations and acquired the trajectory file. We loaded the file into VMD and obtained the force, energy, and distance curve. The rupture forces of the three most frequently observed unbinding tunnels (T1, T2, and T3) were quantitatively estimated. SMD simulations were performed three times for each inhibitor via T1, T2, and T3 (Fig. S1†). SMD was used to further sample both inhibitors and residues along the same unbinding tunnel.32–37 The maximum force value (Fmax) extracted from the SMD simulation trajectories are listed in Table 2 and Fig. 3a, b. As shown in Fig. 3a and b.
Table 2 Fmax of inhibitors in ADA-PRH and ADA-FRK stretched along three tunnels respectively
Systems |
Tunnel |
Fmax(pN) |
ADA-PRH |
T1 |
9653 |
T2 |
10 496 |
T3 |
3147 |
ADA-FRK |
T1 |
2387 |
T2 |
2187 |
T3 |
2095 |
 |
| Fig. 3 Tension changes of inhibitors leaving each tunnel; (a) ADA-PRH (T1 (black), T2 (red), T3 (blue)). (b) ADA-FRK (T1 (black), T2 (red), T3 (blue)). | |
The dynamic process of SMD simulation and the change of interaction energy in PRH and FRK dissociation process were also demonstrated and recorded under the best simulation conditions mentioned above. The Lennard-Jones–Short Range (LJ–SR) and the Coulombic Potential–Short Range (Coul–SR) energies from 0 to 10 ns were being analysed to get the electrostatic energy and vdW energy (Fig. 4a–f and S2†). In three tunnels, during the period that the energy starts to change until the time that the all energies go to zero. The higher energy shown in the figure indicated that the bond is weaker and was more likely to disengage. For the ADA-PRH complex, T3 had the highest energy in the three tunnels during 1–2 ns. For the ADA-FRK complex, the energy of T3 was also highest in the three tunnels most of the time during 1.4–8 ns. This result indicated that the inhibitor is more easily released from the T3 tunnel.
 |
| Fig. 4 Changes in the interaction energy between ADA and inhibitors during SMD simulation. In ADA-PRH. (a) Energy changes in T1; (b) energy changes in T2; (c) energy changes in T3. In ADA-FRK (d) energy changes in T1; (e) energy changes in T2; (f) energy changes in T3. | |
PMF calculations
The calculated PMF profiles for ADA-PRH and ADA-FRK complexes were depicted in Fig. 5. The free energy curve revealed the information about unbinding of the two ligands. With the departure of the inhibitor from the initial equilibrium position, the free energy value rapidly increased. In ADA-PRH, the PMF trends of the three tunnels correspond to Fig. 5a–c. The free energy barrier (the PMF depth, ΔGPMF,lowest
−
ΔGPMF,highest31) of the three tunnels were 64.12 kcal mol−1, 65.84 kcal mol−1, 30.54 kcal mol−1, respectively for T1, T2, T3. It's obvious that T3 required lowest energy. The same performance also can be gotten in ADA-FRK complex (Fig. 5d–f), the free energy barrier for T1, T2, T3 were 62.95 kcal mol−1, 66.9 kcal mol−1, 27.88 kcal mol−1. Another way to look at it, the PMF depth ∝ 1/koff and 1/koff was defined as the residence time (RT). Ligands were more likely to escape from T3, and FRK had shorter RT than PRH.
 |
| Fig. 5 PMF calculation along each tunnels for PRH (a–c) and FRK (d–f). | |
Key residues of PRH unbinding pathway along T3, T2, and T1
For the ADA-inhibitor complex, SMD simulations were used to identify the interactions spanning from hydrogen bonds that stabilize the secondary structure or electrostatic interactions between charged side chains at the atomic level to a long-range backbone or domain–domain specific structural properties at the macroscopic level. Once the peak force was obtained, the inhibitors interacted with some residues that may play an important role in inhibitor unbinding pathway. These residues would provide information for designing drugs to easily access the active binding site and enhance or inhibit the activity of the targeted protein.
In initial structure of ADA-PRH, PRH was held tightly in the binding pocket by His214, His238, Leu58, His15, His17, and Asp19 with hydrogen bonds (Fig. S3†). Figure shows that His17 made a π–π interaction with PRH.
T3 is the favourable pathway for PRH to escape from ADA. At 2.5 ns, the peak point, the hydrogen bonds between Asp19, Asp295, and His238 and PRH were retained, while other hydrogen bonds were broken (Fig. S4†). The π–π interaction between His17 and PRH was broken (Fig. 6a and d). These changes may weaken the interactions between PRH and ADA. However, strong hydrogen bonds with Glu217 and Gly184 were formed with PRH released from ADA. Thus, Gly184, Asp295, His238, and Glu217 were the major residues for the T3 unbinding pathway.
 |
| Fig. 6 (a) π–π interactions between His17 and PRH. (b–d) Distance between the centre of the imidazole group of PRH and the centre of the imidazole group of His17 via (b) T1, (c) T2, and (d) T3. | |
The peak of PRH via T2 unbinding pathway was at 8.5 ns (Fig. 3). All hydrogen bonds disappeared (Fig. S5†). In particular, Glu186 and Glu217 made new hydrogen bonds with PRH. The π–π interaction between His17 and PRH was broken (Fig. 6a and c). Gly216 formed one hydrogen bond with PRH. These hydrogen bonds interactions may prevent the release of PRH escaping from ADA. Thus, the major residues in T2 were Glu186, Glu217, and Gly216.
The active residues for PRH at the peak time (∼7.5 ns) in T1 were shown in Fig. S6.† At this time, all hydrogen bonds at the active pocket disappeared. The π–π interaction between His17 and PRH was broken (Fig. 6a and b). Moreover, new hydrogen bonds were formed (Glu186 and Arg156 made two hydrogen bonds with PRH, and Glu217, Val218, Leu58, and Asp185 formed a hydrogen bond with PRH). These hydrogen bonds may prevent the release of PRH from ADA via T1. Hence, Glu186, Arg156, Glu217, Val218, Leu58, and Asp185 were the important residues in T1.
In particular, His17 made a π–π interaction with PRH in the active pocket, and this interaction disappeared when PRH escaped from ADA via T3, T2, and T1 (Fig. 6a–d). Hence, PRH escaped from ADA via T3 and may break through less obstructions than those via T2 and T1.
Key residues of FRK unbinding along T3, T2, and T1
FRK was held in the binding pocket by Asp19 with a hydrogen bond initially (Fig. S7†). At the peak time (∼1.9 ns), the hydrogen bond between Asp19 and FRK was retained (Fig. S8†). In particular, Trp117 made a strong π–π interaction with FRK (Fig. 7a). These interactions (hydrogen bond and π–π interaction) may prevent the release of FRK leaving from ADA. Thus, Asp19 and Trp117 were the major residues for FRK via the T3 unbinding pathway.
 |
| Fig. 7 (a) π–π interactions between Trp117 and FRK in T3. (b) σ–π interactions between Leu62 and FRK in T2. (c) π–π interactions between Phe65 and FRK in T2. (d) σ–π interactions between Leu62 and FRK in T1. | |
As shown in Fig. 3b, the peak of FRK via the T2 unbinding pathway was at 1.9 ns. The hydrogen bond between Asp19 and FRK was retained (Fig. S9†). In particular, Phe65 formed a π–π interaction with FRK (Fig. 7c). Leu62 made an σ–π interaction with FRK (Fig. 7b). These interactions may prevent the release of PRH escaping from ADA. In T2, the major residues were Asp19, Phe65, and Leu62. The active residues for FRK via T1 were observed (Figs. S10† and 3b) at the peak time (∼2.0 ns). At this time, the hydrogen bond between Asp19 and FRK at the active pocket disappeared. Two new hydrogen bonds between Glu217 and Asp296 were formed. Leu62 made an σ–π interaction with FRK (Fig. 7d). These interactions may prevent the release of FRK from ADA via T1. Thus, Glu217, Asp296, and Leu62 were the important residues of FRK escaping from ADA via T1.
A strobe image of PRH escaping from ADA via T3, T2, and T1 tunnel was shown Fig. 8a–c. According to the above results, PRH may have escaped from ADA via T3 and may break through less obstructions than those via T2 and T1.
 |
| Fig. 8 Strobe images of PRH (a–c) and FRK (d–f) escaping from ADA via T3, T2, and T1. | |
FRK escaped from ADA via T3, T2, and T1 tunnel, as shown in Fig. 8d–f. FRK may have escaped from ADA via T3 and may break through less obstructions than those via T2 and T1.
Alanine scanning for predicting the key residues in the peak of the three tunnels
Alanine scanning mutagenesis was used to analyze the function of specific amino acid residues on the surface of protein. SMD trajectories were employed to carry out binding free energy calculations and perform an alanine scanning of the peak site residues in six complexes by using FoldX software38 (Fig. 9a–c). The most active residues that remarkably contributed to the binding affinity was Trp117 for PRH-ADA via T3, Phe65 for PRH-ADA via T2, and Leu62 for PRH-ADA via T1. However, for the FRK-ADA at peak state, the results of alanine scanning revealed the energetic hot spots corresponding to different residues. In addition, in terms of same tunnel, different inhibitor in protein, Leu58 in T1, Glu186 in T2, Asp295, Glu257 and Gly184 in T3 showed wide difference. This interesting phenomenon can be further explored.
 |
| Fig. 9 Computational alanine scanning of the peak site residues of six complexes. Binding free energies and alanine scanning of residues are shown for (a) T1, (b) T2, (c) T3 in two systems. PRH-ADA is shown in purple bars and FRK-ADA is shown in blue bars. | |
Conservation of tunnel residues
It was much known that residues conservation rate in the superfamily of the protein is important for studying the interactions between protein and inhibitors. ADA belongs to adenosine/adenosine monophosphate (AMP) deaminase family.39 This protein family concludes ADA, ADA2, adenosine deaminase-like protein (ADAL), AMP deaminase (AMPD1, AMPD2 and AMPD3).40 The complete protein sequences of each protein were obtained from UniProt Knowledgebase and aligned via BioEdit software (version 7.2.5).41 The complete amino acid sequence alignment diagram is shown in Fig. S11† and the shade threshold was set as 50%. In the above, we got the residues in the three tunnels that had significant effect on inhibitor binding. To explore their conservatism in the family, we extracted fragments of each residue for comparison and calculated the identity percentage,42 as shown in the Table 3. The identity percentage of each amino acid in ADA is calculated by adding 1 to the number of proteins with the same residue as ADA and dividing by the total number of proteins. It can be concluded that Trp117, Glu186, His238, Asp295 are conservative in adenosine and AMP deaminases family proteins.8,43,44 Hence, Trp117 for PRH-ADA via T3 may play important roles in the unbinding tunnel for inhibitor leaving.
Table 3 Retention of ADA channel residues in other proteins
ADA |
ADA2 |
ADAL |
AMPD1 |
AMPD2 |
AMPD3 |
Percent identity |
Asp19 |
Cys157 |
Leu75 |
Ala316 |
Ser369 |
— |
0 |
Leu58 |
Tyr219 |
Leu138 |
Phe378 |
Phe431 |
Phe60 |
33% |
Leu62 |
Asp223 |
Leu142 |
Asp382 |
Asp435 |
Asp64 |
33% |
Phe65 |
Trp226 |
Phe145 |
Asn385 |
Asn438 |
Asn67 |
33% |
Trp117 |
Trp286 |
Trp197 |
Trp445 |
Trp498 |
Trp127 |
100% |
Arg156 |
Leu322 |
Arg236 |
Val491 |
Ile544 |
Ile173 |
33% |
Gly184 |
Gly348 |
Gly264 |
Asp522 |
Asp575 |
Asp204 |
50% |
Asp185 |
Arg349 |
Asp265 |
Asp253 |
Asp576 |
Asp205 |
83% |
Glu186 |
Glu350 |
Glu266 |
Glu524 |
Glu577 |
Glu206 |
100% |
Gly216 |
— |
Gly296 |
Pro580 |
Pro633 |
Pro262 |
33% |
Glu217 |
His378 |
Glu297 |
His581 |
His634 |
His263 |
33% |
His238 |
His406 |
His318 |
His603 |
His656 |
His285 |
100% |
Asp295 |
Asp463 |
Asp375 |
Asp658 |
Asp711 |
Asp340 |
100% |
Conclusions
ADA is involved in numerous purine metabolism processes. In previous studies, the unbinding tunnel of two different inhibitors remains unknown. In this study, we used RAMD and SMD simulations to explore the unbinding tunnel of two inhibitors (PRH and FRK) via ADA. Three main tunnels were most frequently observed, namely, T1, T2, and T3. Among them, T3 was the most favourable unbinding tunnel for two inhibitors. We also predicted and compared the critical residues in T1, T2, and T3 to ADA. Our results provide a reference for further studies on ADA and a theoretical basis for the development of subsequent ADA inhibitors.
Conflicts of interest
There are no conflicts to declare.
Acknowledgements
This work was supported by the Overseas Cooperation Project of Jilin Province [20200801069GH], the Science and Technology Research of Department of Education of Jilin Province (grant number: JJKH20180207KJ), the Graduate Innovation Fund of Jilin University (grant number: 101832018C180) and the Open Project of State Key Laboratory for Supramolecular Structure and Materials (grant number: SKLSSM202010). This work was performed at the High-Performance Computing Center of Jilin University.
References
- T. Tadashi, T. Kinoshita, M. Kuno and I. Nakanishi, A Highly Potent Non-Nucleoside Adenosine Deaminase Inhibitor:
Efficient Drug Discovery by Intentional Lead Hybridization, J. Am. Chem. Soc., 2004, 126(1), 34–35 CrossRef. - A. Valenzuela, J. Blanco, C. Callebaut, E. Jacotot, C. Lluis, A. G. Hovanessian and R. Franco, Adenosine Deaminase Binding to Human Cd26 Is Inhibited by HIV-1 Envelope Glycoprotein Gp120 and Viral Particles, J. Immunol., 1997, 158(8), 3721 CAS.
- H. Simpkins, A. Stanton and B. H. Davis, Adenosine Deaminase Activity in Lymphoid Subpopulations and Leukemias, J. Cancer Res., 1981, 41(8), 3107 CAS.
- J. F. Smyth, D. G. Poplack, B. J. Holiman, B. G. Leventhal and G. Yarbro, Correlation of Adenosine Deaminase Activity with Cell Surface Markers in Acute Lymphoblastic Leukemia, J. Clin. Invest., 1978, 62(3), 710–712 CrossRef CAS.
- M. B. Van der Weyden and W. N. Kelley, Adenosine Deaminase Deficiency and Severe Combined Immunodeficiency Disease, J. Life Sci., 1977, 20, 1645–1650 CrossRef CAS.
- M. Vedadi, L. Jocelyne, A. Jennifer, M. Amani, Y. Zhao, A. Dong, G. A. Wasney, M. Gao, T. Hills, B. Stephen, W. Qiu, S. Sharma, A. Diassiti, Z. Alam, M. Melone, A. Mulichak, A. Wernimont, J. Bray, P. Loppnau, O. Plotnikova, K. Newberry, E. Sundararajan, S. Houston, J. Walker, W. Tempel, A. Bochkarev, I. Kozieradzki, A. Edwards, C. Arrowsmith, D. Roos, K. Kain and R. Hui, Genome-Scale Protein Expression and Structural Biology of Plasmodium Falciparum and Related Apicomplexan Organisms, Mol. Biochem. Parasitol., 2007, 151(1), 100–110 CrossRef CAS.
- T. Terasaka, H. Okumura, K. Tsuji, T. Kato, I. Nakanishi, T. Kinoshita, Y. Kato, M. Kuno, N. Seki and Y. Naoe, Structure-Based Design and Synthesis of Non-Nucleoside, Potent, and Orally Bioavailable Adenosine Deaminase Inhibitors, J. Med. Chem., 2004, 47, 2728–2731 CrossRef CAS.
- T. Terasaka, T. Kinoshita, M. Kuno, N. Seki, K. Tanaka and I. Nakanishi, Structure-Based Design, Synthesis, and Structure–Activity Relationship Studies of Novel Non-Nucleoside Adenosine Deaminase Inhibitors, J. Med. Chem., 2004, 47(15), 3730–3743 CrossRef CAS.
- V. Sideraki, D. K. Wilson, L. C. Kurz, F. A. Quiocho and F. B. Rudolph, Site-Directed Mutagenesis of Histidine 238 in Mouse Adenosine Deaminase:
Substitution of Histidine 238 Does Not Impede Hydroxylate Formation, Biochemistry, 1996, 35(47), 15019–15028 CrossRef CAS. - X. G. Zhang, J. W. Liu, P. Tang, Z. Y. Liu, G. J. Guo, Q. Y. Sun and J. J. Yin, Identification of a New Uncompetitive Inhibitor of Adenosine Deaminase from Endophyte Aspergillus Niger Sp, Curr. Microbiol., 2018, 75(5), 565–573 CrossRef CAS.
- X. Tian, Y. Liu, J. Zhu, Z. Yu, J. Han, Y. Wang and W. Han, Probing Inhibition Mechanisms of Adenosine Deaminase by Using Molecular Dynamics Simulations, PLoS One, 2018, 13(11), e0207234 CrossRef.
- L. Antonioli, R. Colucci, C. La Motta, M. Tuccori, O. Awwad, F. Da Settimo, C. Blandizzi and M. Fornai, Adenosine Deaminase in the Modulation of Immune System and Its Potential as a Novel Target for Treatment of Inflammatory Disorders, Curr. Drug Targets, 2012, 13(6), 842–862 CrossRef CAS.
- C. U. Lambe and D. J. Nelson, Pharmacokinetics of Inhibition of Adenosine Deaminase by Erythro-9-(2-Hydroxy-3-Nonyl)Adenine in Cba Mice, Biochem. Pharmacol., 1982, 31(4), 535–539 CrossRef CAS.
- R. P. Agarwal, Recovery of 2'-Deoxycoformycin-Inhibited Adenosine Deaminase of Mouse Erythrocytes and Leukemia L1210 in Vivo, J. Cancer Res., 1979, 39(4), 1425 CAS.
- V. Limongelli, L. Marinelli, S. Cosconati, C. La Motta, Michele and J. Parrinello, Sampling Protein Motion and Solvent Effect During Ligand Binding, Proc. Natl. Acad. Sci. U. S. A., 2012, 109(5), 1467–1472 CrossRef CAS.
- T. Kinoshita, I. Nakanishi, T. Terasaka, M. Kuno, N. Seki, M. Warizaya, H. Matsumura, T. Inoue, K. Takano and H. Adachi, Structural Basis of Compound Recognition by Adenosine Deaminase, J. Biochem., 2005, 44(31), 10562 CrossRef CAS.
- L. J. Kingsley and M. A. Lill, Substrate Tunnels in Enzymes: Structure-Function Relationships and Computational Methodology, Proteins, 2015, 83(4), 599–611 CrossRef CAS.
- D. A. Schuetz, W. E. A. de Witte, Y. C. Wong, B. Knasmueller, L. Richter, D. B. Kokh, S. K. Sadiq, R. Bosma, I. Nederpelt, L. H. Heitman, E. Segala, M. Amaral, D. Guo, D. Andres, V. Georgi, L. A. Stoddart, S. Hill, R. M. Cooke, C. De Graaf, R. Leurs, M. Frech, R. C. Wade, E. C. M. de Lange, I. A. P. Jzerman, A. Müller-Fahrnow and G. F. Ecker, Kinetics for Drug Discovery: An Industry-Driven Effort to Target Drug Residence Time, Drug Discovery Today, 2017, 22(6), 896–911 CrossRef CAS.
- B. Hassan, K. A. Mohammad, S. Roy, J. Mohammad Ashraf, M. Adil, M. H. Siddiqui, S. Khan, M. A. Kamal, I. Provazník and I. Choi, Computer Aided Drug Design: Success and Limitations, Curr. Pharm. Des., 2016, 22(5), 572–581 CrossRef.
- T. Kinoshita, N. Nishio, I. Nakanishi, A. Sato and T. Fujii, Structure of Bovine Adenosine Deaminase Complexed with 6-Hydroxy-1,6-Dihydropurine Riboside, Acta Crystallogr., Sect. A: Found. Crystallogr., 2003, 59(2), 299–303 CrossRef.
- X. Liu, X. Wang and H. Jiang, A Steered Molecular Dynamics Method with Direction Optimization and Its Applications on Ligand Molecule Dissociation, J. Biochem. Biophys. Methods, 2008, 70(6), 857–864 CrossRef CAS.
- S. K. Lüdemann, V. Lounnas and R. C. Wade, How Do Substrates Enter and Products Exit the Buried Active Site of Cytochrome P450cam? 1. Random Expulsion Molecular Dynamics Investigation of Ligand Access Channels and Mechanisms, J. Mol. Biol., 2000, 303(5), 797–811 CrossRef.
- H. Li, D. Min and Y. Liu, Essential Energy Space Random Walk Via Energy Space Metadynamics Method to Accelerate Molecular Dynamics Simulations, J. Chem. Phys., 2007, 127(9), 2183 CrossRef.
- L. Zheng and W. Yang, Essential Energy Space Random Walks to Accelerate Molecular Dynamics Simulations: Convergence Improvements Via an Adaptive-Length Self-Healing Strategy, J. Chem. Phys., 2008, 129, 181 Search PubMed.
- G. Ozer, F. V. Edward, Q. Stephen and R. Hernandez, Adaptive Steered Molecular Dynamics of the Long-Distance Unfolding of Neuropeptide Y., J. Chem. Theory Comput., 2010, 6(10), 3026 CrossRef CAS.
- J. Zhu, Yi Li, J. Wang, Z. Yu, Y. Liu, Y. Tong and W. Han, Adaptive Steered Molecular Dynamics Combined with Protein Structure Networks Revealing the Mechanism of Y68i/G109p Mutations That Enhance the Catalytic Activity of D-Psicose 3-Epimerase from Clostridium Bolteae, Front. Chem., 2018, 6, 437–37 CrossRef CAS.
- D. Wang, H. Jin, J. Wang, S. Guan, Z. Zhang and W. Han, Exploration of the Chlorpyrifos
Escape Pathway from Acylpeptide Hydrolases Using Steered Molecular Dynamics Simulations, J. Biomol. Struct. Dyn., 2015, 34(4), 749–761 CrossRef.
- R. Salomon-Ferrer, D. A. Case and R. C. Walker, An Overview of the Amber Biomolecular Simulation Package, Wiley Interdiscip. Rev.: Comput. Mol. Sci., 2013, 3(2), 198–210 CAS.
- T. A. Darden, M. Y. Darrin and L. G. Pedersen., Particle Mesh Ewald: An log(N) Method for Ewald Sums in Large Systems, J. Chem. Phys., 1993, 98(12), 10089–10092 CrossRef CAS.
- S. Park and K. Schulten, Calculating Potentials of Mean Force from Steered Molecular Dynamics Simulations, J. Chem. Phys., 2004, 120(13), 5946 CrossRef CAS.
- S. Doudou, N. A. Burton and R. Henchman, Standard Free Energy of Binding from a One-Dimensional Potential of Mean Force, J. Chem. Theory Comput., 2009, 5(4), 909–918 CrossRef CAS.
- H. Jin, J. Zhu, Y. Dong and W. Han, Exploring the Different Ligand Escape Pathways in Acylaminoacyl Peptidase by Random Acceleration and Steered Molecular Dynamics Simulations, RSC Adv., 2016, 6, 10987–10996 RSC.
- D. S. Moore, C. Brines, H. Jewhurst, J. P. Dalton and I. G. Tikhonova, Steered Molecular Dynamics Simulations Reveal Critical Residues for (Un)Binding of Substrates, Inhibitors and a Product to the Malarial M1 Aminopeptidase, PLoS Comput. Biol., 2018, 14(10), e1006525 CrossRef.
- P.-C. Do, E. H. Lee and Ly Le, Steered Molecular Dynamics Simulation in Rational Drug Design, J. Chem. Inf. Model., 2018, 58(8), 1473–1482 CrossRef CAS.
- B.-L. Xiao, Y.-N. Ning, N.-N. Niu, Di Li, A. Moosavi-Movahedi, N. Sheibani and J. Hong, Steered Molecular Dynamic Simulations of Conformational Lock of Cu, Zn-Superoxide Dismutase, Sci. Rep., 2019, 9(1), 4353–53 CrossRef.
- J. Zhu, X. Li, S. Zhang, H. Ye, H. Zhao, H. Jin and W. Han, Exploring Stereochemical Specificity of Phosphodiesterase by Mm-Pbsa and Mm-Gbsa Calculation and Steered Molecular Dynamics Simulation, J. Biomol. Struct. Dyn., 2017, 35(14), 3140–3151 CrossRef CAS.
- W. Li, J. Fu, F. Cheng, M. Zheng, J. Zhang, G. Liu and Y. Tang, Unbinding Pathways of Gw4064 from Human Farnesoid X Receptor as Revealed by Molecular Dynamics Simulations, J. Chem. Inf. Model., 2012, 52(11), 3043–3052 CrossRef CAS.
- R. Guerois, J. E. Nielsen and L. Serrano, Predicting Changes in the Stability of Proteins and Protein Complexes: A Study of More Than 1000 Mutations, J. Mol. Biol., 2002, 320(2), 369–387 CrossRef CAS.
- G. Bojack, C. G. Earnshaw, R. Klein, S. D. Lindell, C. Lowinski and R. Preuss, Design and Synthesis of Inhibitors of Adenosine and Amp Deaminases, Org. Lett., 2001, 3(6), 839–842 CrossRef CAS.
- S. A. Maier, J. R. Galellis and H. E. Mcdermid, Phylogenetic Analysis Reveals a Novel Protein Family Closely Related to Adenosine Deaminase, J. Mol. Evol., 2005, 61(6), 776–794 CrossRef CAS.
- T. Hall, Bioedit: A User-Friendly Biological Sequence Alignment Editor and Analysis Program for Windows 95/98/Nt, Nucleic Acids Symp. Ser., 1999, 41, 95–98 CAS.
- P. Palenchar, M. Mount, C. Douglas and J. Dougherty, Using Genome-Wide Protein Sequence Data to Predict Amino Acid Conservation, Protein J., 2008, 27(6), 401–407 CrossRef CAS.
- T. Kinoshita, T. Tada and I. Nakanishi, Conformational Change of Adenosine Deaminase During Ligand-Exchange in a Crystal, Biochem. Biophys. Res. Commun., 2008, 373(1), 53–57 CrossRef CAS.
- K. Diederichs and G. E. Schulz, The Refined Structure of the Complex between Adenylate Kinase from Beef Heart Mitochondrial Matrix and Its Substrate Amp at 1.85 a Resolution, J. Mol. Biol., 1991, 217(3), 541–549 CrossRef CAS.
Footnote |
† Electronic supplementary information (ESI) available. See DOI: 10.1039/d0ra07796h |
|
This journal is © The Royal Society of Chemistry 2020 |