DOI:
10.1039/D0RA05326K
(Paper)
RSC Adv., 2020,
10, 32548-32560
Metabolic characterisation of Magnetospirillum gryphiswaldense MSR-1 using LC-MS-based metabolite profiling†
Received
17th June 2020
, Accepted 23rd August 2020
First published on 2nd September 2020
Abstract
Magnetosomes are nano-sized magnetic nanoparticles with exquisite properties that can be used in a wide range of healthcare and biotechnological applications. They are biosynthesised by magnetotactic bacteria (MTB), such as Magnetospirillum gryphiswaldense MSR-1 (Mgryph). However, magnetosome bioprocessing yields low quantities compared to chemical synthesis of magnetic nanoparticles. Therefore, an understanding of the intracellular metabolites and metabolic networks related to Mgryph growth and magnetosome formation are vital to unlock the potential of this organism to develop improved bioprocesses. In this work, we investigated the metabolism of Mgryph using untargeted metabolomics. Liquid chromatography-mass spectrometry (LC-MS) was performed to profile spent medium samples of Mgryph cells grown under O2-limited (n = 6) and O2-rich conditions (n = 6) corresponding to magnetosome- and non-magnetosome producing cells, respectively. Multivariate, univariate and pathway enrichment analyses were conducted to identify significantly altered metabolites and pathways. Rigorous metabolite identification was carried out using authentic standards, the Mgryph-specific metabolite database and MS/MS mzCloud database. PCA and OPLS-DA showed clear separation and clustering of sample groups with cross-validation values of R2X = 0.76, R2Y = 0.99 and Q2 = 0.98 in OPLS-DA. As a result, 50 metabolites linked to 45 metabolic pathways were found to be significantly altered in the tested conditions, including: glycine, serine and threonine; butanoate; alanine, aspartate and glutamate metabolism; aminoacyl-tRNA biosynthesis and; pyruvate and citric acid cycle (TCA) metabolisms. Our findings demonstrate the potential of LC-MS to characterise key metabolites in Mgryph and will contribute to further understanding the metabolic mechanisms that affect Mgryph growth and magnetosome formation.
1. Introduction
Magnetotactic bacteria (MTB) are a diverse group of microorganisms which are able to produce intracellular nano-sized crystals of magnetite (Fe3O4) or greigite (Fe3S4) bound to a cytoplasmic membrane and packed in a chain-like structure namely, the magnetosomes.1 These biosynthesised magnetic nanoparticles present exquisite properties, such as exhibiting a narrow size distribution, ferrimagnetism, and high stability towards self-aggregation,1,2 and they can be functionalised in vivo using genetic engineering tools.3 Magnetosomes can be used in a wide range of biotechnological and biomedical applications, such as cancer therapy, magnetic resonance agents or bioremediation.2,4–6 However, their real-world application is hindered to a large extent by the low availability of such materials.
Magnetospirillum gryphiswaldense MSR-1 (Mgryph) is an MTB that can be grown at relatively high cell densities and is capable of producing up to 4% of its dry cell weight in the form of magnetosomes.7 Mgryph has been extensively used as an MTB model organism addressing genetics, physiology and magnetosome formation mechanism studies.8 Recently, advances in Mgryph bioprocessing9–11 and metabolic engineering12 have resulted in an increase of magnetosome yields, thus, paving the way for future magnetosome production at a large scale. However, magnetosome formation occurs under tightly controlled growth conditions. For example, Mgryph synthesises magnetosomes in an O2-limited environment as the biomineralisation of the magnetite is only viable under microaerobic conditions.3 The carbon source and its effect on cell growth and magnetosome formation have been widely studied in Mgryph.3,11 However, the central carbon metabolism (CCM) of Mgryph and the regulation of its metabolic pathways during the formation of the magnetosome and the cell growth is poorly understood at the cellular level. CCM is known to provide biomass precursors and energy through a carbon flux into different biochemical reactions. Previous studies suggested that the production of magnetosomes is an energy-dependent process as the ATPase13 and the magnetosome membrane protein MamK14 were found to be involved in the ferrous uptake by the MTB. Moreover, the cyclic AMP (cAMP) receptor protein (CRP), which is a global transcriptional regulator in prokaryotes, was found to play an important role in energy metabolism and in the formation of magnetosomes.15 However, further studies of the intermediates, such as the fluxes of the intracellular metabolites at different stages of the cell growth, and their complex networks, including CCM, by which magnetosomes are formed, remain unexplored in Mgryph. Such research can potentially enable unlocking of the mechanism whereby efficient cell growth and magnetosome production can be optimised.
Metabolomics using liquid chromatography-mass spectrometry (LC-MS) has the potential to provide an insight into cell metabolism and metabolic pathways.16,17 For instance, LC-MS was successfully used to monitor the intracellular metabolites of Clostridium autoethanogenum during gas fermentation, which enabled the optimisation of biofuel production using computational modelling.18 In addition, the growth medium analysis of bacterial cells using LC-MS provides comprehensive metabolic information which enables the study of the microenvironmental changes caused by the microbial activities under different culture conditions.19 Since the analysis of the extracellular metabolites in a growth medium using LC-MS requires minimum sample preparation (no complicated cell quenching and extraction procedures), this technique was used successfully to monitor metabolic changes in the growth media analysed from E. coli cultures investigating the efficient fermentation of fucose for bio-based products,20 and Clostridium acetobutylicum to study the use of polyketides to regulate cellular differentiation.21 However, to the best of our knowledge, no LC-MS studies have been performed for the metabolic characterisation of Mgryph. This technique can be employed to monitor the CCM and determine the affected pathways linked to the production of magnetosomes by Mgryph.
In this study, we first characterised the physiological properties of Mgryph grown under O2-limited and O2-rich conditions in shake flask cultures. Then, we applied untargeted metabolomics using LC-MS for profiling and identification of metabolites in the growth media. This was done in order to investigate the significantly altered metabolites and pathways in Mgryph. Our findings indicate that 50 metabolites linked to 45 metabolic pathways were found to be significantly altered in the tested conditions. This study paves the way for the application of LC-MS-based metabolomics to understand the metabolism of MTB, leading to the development of processes to achieve enhanced production of magnetosomes.
2. Experimental
2.1 Chemicals and reagents
LC-MS grade methanol and acetonitrile were obtained from Fisher Scientific (Loughborough, UK) and VWR (West Sussex, UK), respectively. Ammonium carbonate, chemicals and all analytical standards were obtained from either Sigma-Aldrich (Gillingham, UK) or Fisher Scientific (Loughborough, UK) unless otherwise stated. Deionised water was prepared using a Milli-Q water purification system (Millipore, MA, USA). Five mixtures of 235 authentic standards were prepared at a concentration of 20 μM and used for the identification of the metabolites in the study. The list of the standards is available in Table S1 in the ESI.†
2.2 Strains, growth media and culture conditions of Mgryph
Mgryph MSR-1 was acquired from Deutsche Sammlung von Mikroorganismen und Zellkulturen GmbH culture collection (DSMZ, Braunschweig, Germany). Two types of Mgryph cells were obtained: (1) magnetosome and (2) non-magnetosome producing cells from microaerobic and aerobic bioreactor cultures, respectively, carried out under controlled conditions using a pH-stat fermentation strategy adapted from our previous study.22 Both cell types were further re-suspended in 2 mL of phosphate-buffered saline (PBS), inoculated into 38 mL of Flask Standard Medium (FSM) and grown at 30 °C in a shaker incubator at 150 rpm for 48 h. Magnetosome producing cells (n = 6) were grown in airtight O2-limited cultures while non-magnetosome producing cells (n = 6) were grown in aerobic cultures. The FSM was composed of 0.1 g L−1 yeast extract, 3 g L−1 soybean peptone, 3.5 g L−1 potassium L-lactate, 100 μM iron(III) citrate, 0.34 g L−1 NaNO3, 0.1 g L−1 KH2PO4, 0.15 g L−1 MgSO4·7H2O, 5 mL L−1 of EDTA-chelated trace element mixture and 2.38 g L−1 4-(2-hydroxyethyl)piperazine-1-ethane sulfonic acid (HEPES) buffer in deionized water; adjusted to pH 7.0 prior to autoclave sterilisation.
2.3 Polyhydroxyalkanoates imaging using fluorescence microscopy
Mgryph cells (100 μL) were taken from FSM cultures and stained with 5 μL of 0.1 mg mL−1 of pyrromethene-546 (Pyr-546) in dimethyl sulfoxide for polyhydroxyalkanoates (PHAs) imaging. A Zeiss Primo Star iLed microscope (Carl Zeiss Ltd., Cambridge, UK) fitted with a Zeiss AxioCam ERc 5s camera was used. Images were acquired within 1 min of fluorophore incubation and processed with the aid of Zeiss ZEN Lite 2012 software in auto exposure mode. Samples were excited with a Zeiss Led 470 nm light source and a 515 LP filter was employed for detection of Pyr-546 fluorescence.
2.4 Measurement of cell concentration and cellular magnetism
Culture optical densities were recorded at a wavelength of 565 nm (OD565) using an Evolution 300 UV-vis spectrophotometer controlled by Thermo Scientific™ VISIONpro™ software (Thermo Fisher Scientific, Hemel Hempstead, UK). Cellular magnetic response (Cmag) values were determined by OD565 measurements using a purpose-built magnetic measurement system mounted within the spectrophotometer as described elsewhere.22 Cmag values greater than “1” indicate the presence of the magnetic cells.
2.5 Transmission electron microscopy
The bacterial samples (2 μL) were drop cast on a graphene oxide on lacey carbon supported 300 mesh copper grid (GOLC300Cu50, EM Resolutions, Sheffield, UK) and vacuum-dried before analysis. Transmission Electron Microscopy (TEM) images were obtained using a JEM-2100F microscope (JEOL, Herts, UK) operated at 200 kV equipped with a Gatan Orius CCD camera (Pleasanton, USA).
2.6 Sample preparation for LC-MS
Samples (1 mL) were taken after 48 h of cultivation and were immediately centrifuged at 10
000g for 3 min at 4 °C. The spent media were then separated from the cell pellets and kept at −80 °C for LC-MS-based metabolite profiling. The spent medium samples of magnetosome (n = 6), non-magnetosome producing cells (n = 6) of Mgryph and the control FSM (n = 6) were prepared for LC-MS as follows: an equal volume of the thawed medium on ice (50 μL) from each culture was added to an equal volume of methanol (50 μL) (1
:
1 sample
:
methanol), vortexed for 30 s, cooled on ice for 2 min, centrifuged at 10
000g at 4 °C for 5 min and analysed immediately. Reagent blanks (n = 3) were prepared using 1
:
1 water
:
methanol following the same procedure excluding the sample. For metabolomics analysis, a sample was prepared by mixing 20 μL from each sample in the study and used as a pooled QC sample.
2.7 Analytical methodologies: LC-MS for metabolite profiling and LC-MS/MS for metabolite identification
LC-MS analysis was performed using a Q-Exactive Plus mass spectrometer (MS) equipped with Dionex U3000 UHPLC system (Thermo Fisher Scientific, Hemel Hempstead, UK). Metabolites in the samples (5 μL, 4 °C) were separated on a ZIC-pHILIC column (4.6 × 150 mm, 5 μm particle size, Merck SeQuant, Watford, UK) in which the column was maintained at a flow rate of 300 μL min−1 at a temperature of 45 °C for 24 min as previously described.23 The gradient started with 20% A (20 mM ammonium carbonate in water) and 80% of B (acetonitrile) and increased to 95% A over 15 min, then the composition was returned to its initial conditions in 2 min and the column was re-equilibrated for 7 min. The MS was operated in ESI+ and ESI− switching acquisition modes for LC-MS profiling of the samples and in data-dependent MS/MS (ddMS/MS) for identification for the analysis of the QC samples. For MS parameters, spray voltage was 4.5 kV (ESI+) and 3.5 (ESI−), and capillary voltage was 20 V (ESI+) and −15 V (ESI−). The sheath, auxiliary and sweep gas flow rates were 40, 5 and 1 (arbitrary unit), respectively, for both modes. Capillary and heater temperatures were maintained at 275 and 150 °C, respectively. Data were acquired for the LC-MS profiling with a resolution of 70
000 from m/z 70–1050. Top 5 ddMS/MS was performed on the QC sample (n = 3) at a resolution of 17
500 and a stepped normalised collision energy (NEC) of 20, 30 and 40.
The spent medium samples of the magnetosome, non-magnetosome producing cells and blank media (FSM, control) were randomised and analysed in a single LC-MS analytical run with the mixtures of authentic standards and reagent blanks. Pooled QC (n = 6) was injected at the beginning of the analysis to condition the column and after every 3–5 samples (n = 6) to check the stability, robustness, repeatability and performance of the analytical system.
2.8 Data analysis and metabolite identification
The acquired raw LC-MS datasets of the samples in the study were pre-processed for untargeted metabolomics, univariate analysis and metabolite identification with Compound Discoverer 3.1 SP1 software (Thermo Fisher Scientific, Hemel Hempstead, UK). Principal component analysis (PCA) and orthogonal partial least squares-discriminant analysis (OPLS-DA) were performed using Simca P+13 (Umetrics, Umeå, Sweden). The imported datasets were mean-centred and Pareto scaled. Cross-validation using the leave-one-out method was used to evaluate the robustness of the generated PCA and OPLS-DA by monitoring the fitness of model (R2X) for PCA, (R2Y) for OPLS-DA and predictive ability (Q2) values.24 Metabolite identification was performed using Compound Discoverer 3.1 SP1 software by matching accurate masses of the detected peaks with metabolites in Mgryph MSR-1 (MGRY1430440) BioCyc database, the retention times (RT) of the authentic standards (within 0.25 min shift) and mzCloud fragmentation database. Briefly, retention time range used was 3.2–23.5 min, mass range was m/z 70–1050, mass tolerance for peak picking and metabolite identification was ≤ 5 ppm for precursor and fragment ions, minimum peak intensity was 1.0 × 106, intensity tolerance was at 30%, area max was greater or equal to 3.0 × 106, maximum retention time shift was 0.25 min and activation energy tolerance was 5. The confidence in metabolite identification was assigned as level 1–4 based on the recommendation by the Chemical Analysis Working Group, Metabolomics Standards Initiative (MSI).25,26 In this classification, the metabolites identified using m/z, RT and/or MS/MS of reference standards were level 1, putatively annotated metabolites using m/z, RT and/or MS/MS from spectral library and no reference standards were level 2, putatively characterised metabolite classes were level 3 and unknowns were classified as level 4.
Variable importance in the projection (VIP) and |p(corr)| of the OPLS-DA were used to extract the metabolites responsible for the difference between the spent media of magnetosome and non-magnetosome producing cells. Student t-test adjusted with false discovery rate using the Benjamini–Hochberg approach27 for multiple testing problem were performed across all peak intensities detected in the samples.
2.9 Pathway analysis and metabolite network mapping
Pathway analysis, enrichment and network mapping of the significantly altered metabolites between magnetosome and non-magnetosome producing cells were performed using MetaboAnalyst 4.0.28 The normalised abundances of the metabolites were Pareto scaled and processed for metabolite enrichment and pathway analysis while fold changes were used for KEGG metabolite network mapping.
3. Results and discussion
3.1 Comparison of physiological changes of Mgryph grown under O2-limited and O2-rich culture conditions
To characterise the physiological differences between Mgryph grown with O2-rich and O2-limited conditions, we used cryostocks from bioreactors using a pH-stat feeding strategy. Magnetic and non-magnetic cryostocks were prepared in 5% DMSO using cells harvested from a bioreactor experiment grown under microaerobic (pO2 < 1%) and aerobic (pO2 > 5%) conditions, respectively. Magnetic (n = 6) and non-magnetic (n = 6) cryostocks were inoculated into fresh FSM (control) and grown in O2-limited and O2-rich conditions (free air exchange), respectively, at 30 °C on a shaker incubator (150 rpm). Both cultures had headspace volumes of 20% and OD565 and Cmag were measured after 48 h. PHAs and magnetosome contents were also measured (Fig. 1). Cells grown under O2-rich conditions grew better than O2-limited conditions. It was confirmed that cells grown under O2-rich conditions were not magnetic (Cmag = 1), whereas cells grown under O2-limited conditions were magnetic (Cmag = 1.82) (Fig. 1A). Strong Pyr-546 fluorescence and electron microscopy images indicated the presence of large quantities of PHAs in both cultures (Fig. 1B and C). TEM analysis confirmed the presence and absence of magnetosome chains in the O2-limited and O2-rich cultures, respectively. These results are in agreement with findings from our previous studies:29 using a 20% headspace volume allows relatively high growth under free air exchange conditions, whereas it maintains the magnetic properties of Mgryph in shake flask cultures.29 The presence of PHAs has been previously reported and might be due to the redirection of nutrients and energy competition between PHAs and magnetosome formation. In contrast, magnetosome formation is inhibited under aerobic conditions.30
 |
| Fig. 1 Effect of O2 limitation on the physiology of Mgryph. Cultures were grown under O2-limited and O2-rich conditions and characterised at 48 h. (A) OD565 (black bars) and cellular magnetism (Cmag; pale grey bars). Error bars are standard deviation (n = 6). Fluorescence of cells stained with Pyr-546 and transmission electron micrographs of magnetic (B) and non-magnetic (C) cells. | |
3.2 Performance of LC-MS for untargeted metabolomics
The performance of the LC-MS-based metabolite profiling of the culture medium samples of magnetosome and non-magnetosome producing cells, and FSM (control medium sample) was assessed using univariate and multivariate analysis of all the detected peaks in the samples. The LC-MS datasets generated 2556 peaks in which the variability based on the use of relative standard deviations (RSDs) across the average peak areas was less than 30% for 93.3% of all peaks present in the QC. The variability in the QC was lower than the recommended threshold of RSD < 30% for at least 70% of all peaks for metabolomics analysis.31 PCA was also used to assess the analytical performance of the method.32,33 The pooled QCs were closely clustered towards the centre of the PCA scores plot (Fig. 2). These results demonstrate satisfactory instrumental stability and validate the LC-MS analytical performance for untargeted metabolomics.
 |
| Fig. 2 PCA scores plot of FSM (flask standard media, control) and spent media of non-magnetosome (O2-rich) and magnetosome-producing (O2-limited) cells analysed with LC-MS (cross validation: R2X = 0.81 and Q2 = 0.61). | |
3.3 Data analysis
The mass ion pairs (RT, m/z) from the dataset generated in this study were exported with their normalised abundances for multivariate analysis in which PCA and OPLS-DA were used for modelling the differences and similarities between samples. PCA was used to give an unbiased overview of any possible trends and clusters within the sample datasets. PCA-class analysis was first performed to evaluate the similarity of samples within each class. Tight clusters with no significant differences were observed within each class, indicated by the poor PCA-class Q2 values (data not shown). This result demonstrates that the experimental setup, including the growth of microorganism, sample preparation and the analytical run, were carefully carried out, evidencing no outliers and adequate similarities within each class in the analysis.
Clear clustering and separation were observed in the metabolic profiles of the spent media of magnetosome and non-magnetosome producing cells, and FSM controls in both PCA and OPLS-DA scores plots (Fig. 2 and 3A). As can be seen in Fig. 3B, a further comparative OPLS-DA of the spent media of magnetosome and non-magnetosome producing cells showed that they were clearly separated and clustered, indicating that the metabolic profile is more distinct from one class into another. Cross-validation of this OPLS-DA was excellent with a cumulative R2X of 0.76, R2Y of 0.99 and Q2 of 0.98; these values indicate that the models are less likely to be overfitted as they are higher than the recommended values of 0.50 of R2X, R2Y and Q2 for a robust model.24
 |
| Fig. 3 OPLS-DA scores plots of the spent media of magnetosome (O2-limited), non-magnetosome producing (O2-rich) cells and FSM controls analysed with LC-MS (cross-validation: (A) R2X = 0.83, R2Y = 0.99 and Q2 = 0.96; (B) R2X = 0.76, R2Y = 0.99 and Q2 = 0.98). | |
3.4 Feature selection and comparison of the significantly altered metabolites related to the presence of the magnetosomes
The 2556 metabolite features detected in the spent media of magnetosome compared to non-magnetosome producing cells (Fig. 4A) were submitted for metabolite identification using Compound Discoverer 3.1 SP1. The m/z, RT and/or MS/MS of these features were interrogated against the specific BioCyc database of Mgryph, the retention times (RT) of 235 authentic standards and the mzCloud fragmentation database. 423 metabolites were identified in which only 137 metabolites were reported with high confidence in identification (i.e. Level 1 and 2 only) based on MSI levels of identification25,26 as detailed earlier (Fig. 4B, Table S2 in the ESI†).
 |
| Fig. 4 Summary of the detected features, metabolite identification and pathway analysis of the significantly altered metabolites in the spent media of magnetosome compared to non-magnetosome producing cells. (A) Venn diagram presenting the common and the significantly changed features detected in the spent media samples, (B) pie-chart showing the significantly changed and the common number of identified metabolites (Level 1 and 2), putatively identified metabolites (Level 3 and 4) and the unidentified metabolite features between the two sets of samples; and (C) pathway analysis: the top pathways were ranked by the gamma-adjusted p-values for permutation per pathway (y-axis) and the total number of hits per pathway (x-axis). Colour graduated from white to yellow, orange and red, circle size (large > small) as well as the values of both x and y increase represents the degree of significance. | |
The significantly altered metabolites in the samples were selected using both multivariate (OPLS-DA) and univariate analysis (adjusted p-values). VIP and |p(corr)| of the OPLS-DA were used to extract the metabolites relevant to the separation of samples in the model. VIP scores estimate the importance of each variable in the projection used in OPLS-DA and is often used for variable selection, while |p(corr)| reflects the reliability of each VIP score in the model.34 Metabolites with adjusted p-value < 0.05, VIP > 1.0 and |p(corr)| > 0.4 were considered significantly altered metabolites (Fig. 4B). As a result, 50 metabolites, including amino acids, organic acids, energy molecules and nucleotides, were identified as significantly altered metabolites in the magnetosome- compared to non-magnetosome-producing cells. Fig. 5 shows a means of direct comparison of the levels of these metabolites; the full list of the key metabolites can be seen in Table S3 in the ESI.†
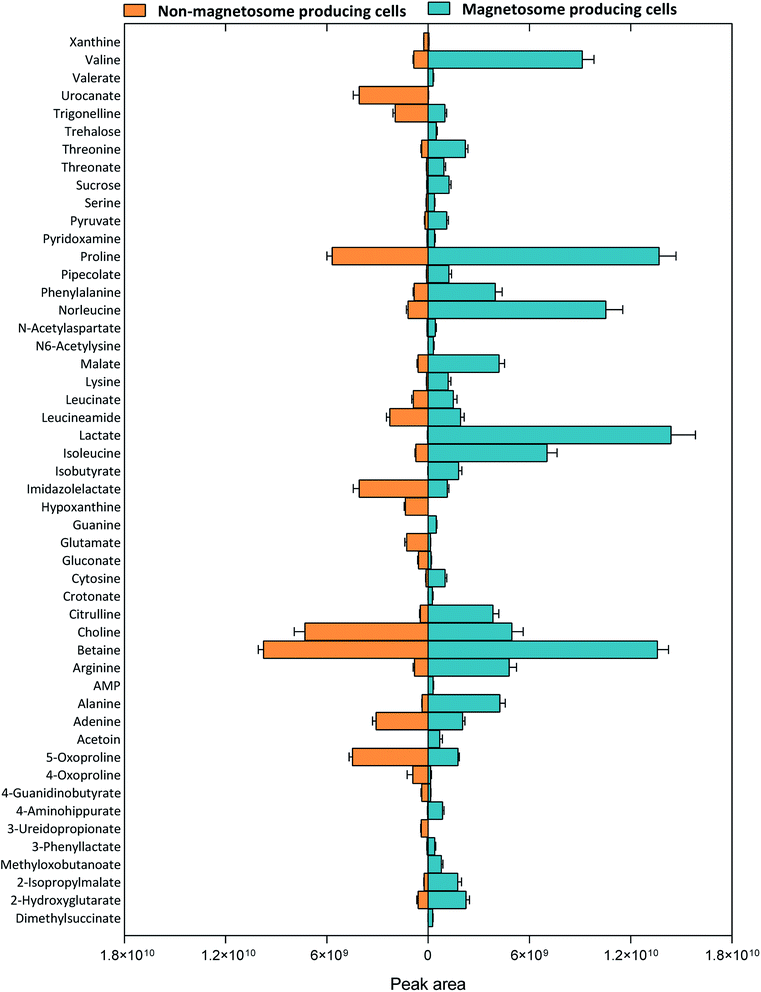 |
| Fig. 5 Relative levels expressed as peak area (arbitrary units) of significantly altered metabolites in the spent media of Mgryph grown on O2-limited cultures (n = 6, magnetosome producing cells) compared to O2-rich cultures (n = 6, non-magnetosome producing cells). | |
The level of AMP, lactate, pyruvate, acetoin, crotonate, pepicolate, trehalose and guanine were found to be high in the samples of magnetosome-producing cells compared to non-magnetosome producing cells (Table 1). This indicates that these metabolites were either excreted by Mgryph under the O2-limited growth conditions or, if already present in the growth media, they were consumed at a slower rate compared to the O2-rich cultures. The presence of higher level of lactate in the O2-limited cultures compared to O2-rich cultures is expected as it is the major carbon source component in FSM. The O2-limited cultures showed lower biomass than the O2-rich ones (Fig. 1A), hence, lower consumption of lactate. It is known that there is a correlation between Mgryph growth and lactate consumption.22 On the other hand, the presence of acetoin could be related to its secretion under microaerobic growth conditions and this has been previously described to occur in Bacillus subtilis35 grown under microaerobic conditions. The accumulation of AMP and pyruvate in the spent media of magnetosome-producing cells may suggest that lactate could be converted into pyruvate via lactate dehydrogenase and subsequently acetoin formation occurred via the pyruvate route.36 Our results suggest that this may act as an alternative energy and respiratory route during O2-limited growth of Mgryph. In addition, pepicolate was also found to serve as an osmoprotector and support the growth of E. coli under stressed conditions37 which may signal that Mgryph upregulated pepicolate to overcome its O2-limited growth. This is further evident by the accumulation of trehalose in the samples of magnetosome producing cells as endogenous trehalose was found to play an important role as an osmo- and stress-protector in E. coli.38 For further understanding of the metabolic changes in Mgryph during the formation of the magnetosomes, the list of significant metabolites was submitted for pathway analysis.
Table 1 The top significantly affected metabolites and metabolic pathways in the magnetosome producing cells compared to non-producing cells
Metabolite |
Monoisotopic massa (Da) |
Formula |
Identificationb |
Mass error (ppm) |
log 2 fold change |
Adjusted p-valuec |
OPLS-DA p(corr) |
OPLS-DA VIP |
(1) Metabolites |
Detected accurate mass. Level of identification was based on MSI classification: (1) the metabolite was identified using m/z, RT and/or MS/MS of reference standards, (2) the metabolite was putatively annotated using m/z, RT and/or MS/MS from spectral library and no reference standards, (3) metabolite class was putatively characterised and (4) unknowns. p-Values were adjusted using Benjamini Hochberg FDR. |
AMP |
347.0631 |
C10H14N5O7P |
m/z, RT, MS/MS (1) |
0.44 |
9.1 |
3.82 × 10−15 |
1.00 |
1.17 |
Lactic acid |
90.0317 |
C3H6O3 |
m/z, RT, MS/MS (1) |
1.93 |
8.4 |
3.82 × 10−15 |
1.00 |
8.12 |
Trehalose |
342.1165 |
C12H22O11 |
m/z, MS/MS (2) |
0.81 |
8.2 |
3.82 × 10−15 |
0.99 |
1.50 |
Acetoin |
88.0526 |
C4H8O2 |
m/z, RT, MS/MS (1) |
1.48 |
7.7 |
3.82 × 10−15 |
0.96 |
1.75 |
Guanine |
151.0495 |
C5H5N5O |
m/z, RT (1) |
0.42 |
7.2 |
3.82 × 10−15 |
0.99 |
1.50 |
Isobutyric acid |
88.0526 |
C4H8O2 |
m/z, MS/MS (2) |
1.98 |
7.0 |
3.82 × 10−15 |
0.99 |
2.87 |
2,2-Dimethylsuccinic acid |
146.0581 |
C6H10O4 |
m/z, MS/MS (2) |
2.00 |
6.3 |
2.98 × 10−11 |
0.99 |
1.10 |
N6-Acetylysine |
188.1162 |
C8H16N2O3 |
m/z, RT |
0.30 |
6.0 |
3.82 × 10−15 |
0.99 |
1.20 |
Crotonic acid |
86.0369 |
C4H6O2 |
m/z, MS/MS (2) |
1.87 |
5.9 |
3.82 × 10−15 |
0.99 |
1.09 |
3-Methy-2-oxobutanoic acid |
116.0476 |
C5H8O3 |
m/z, RT, MS/MS (1) |
2.01 |
5.2 |
3.82 × 10−15 |
0.99 |
1.87 |
4-Aminohippuric acid |
194.0691 |
C9H10N2O3 |
m/z, RT (1) |
0.62 |
4.5 |
3.82 × 10−15 |
0.99 |
1.94 |
Sucrose |
342.1162 |
C12H22O11 |
m/z, RT, MS/MS (1) |
0.80 |
3.9 |
3.82 × 10−15 |
1.00 |
2.31 |
Valeric acid |
102.0683 |
C5H10O2 |
m/z, MS/MS (2) |
2.35 |
3.8 |
3.82 × 10−15 |
0.99 |
1.13 |
Pipecolic acid |
129.0790 |
C6H11NO2 |
m/z, MS/MS (2) |
0.58 |
3.6 |
3.82 × 10−15 |
0.99 |
2.28 |
Lysine |
146.1057 |
C6H14N2O2 |
m/z, RT (1) |
0.34 |
3.6 |
3.82 × 10−15 |
0.99 |
2.23 |
Alanine |
89.0477 |
C3H7NO2 |
m/z, RT, MS/MS (1) |
0.30 |
3.5 |
3.82 × 10−15 |
1.00 |
4.23 |
Valine |
117.0790 |
C5H11NO2 |
m/z, RT, MS/MS (1) |
0.27 |
3.4 |
3.82 × 10−15 |
1.00 |
6.17 |
Pyruvic acid |
88.0162 |
C3H4O3 |
m/z, MS/MS (2) |
2.12 |
2.5 |
7.26 × 10−12 |
0.99 |
2.05 |
Xanthine |
152.0337 |
C5H4N4O2 |
m/z, RT, MS/MS (1) |
1.52 |
−2.6 |
3.82 × 10−15 |
−1.00 |
1.02 |
4-Oxoproline |
129.0429 |
C5H7NO3 |
m/z, MS/MS (2) |
1.83 |
−2.7 |
6.89 × 10−7 |
−0.88 |
1.78 |
Glutamate |
147.0532 |
C5H9NO4 |
m/z, RT, MS/MS (1) |
0.25 |
−3.2 |
3.82 × 10−15 |
−0.99 |
2.28 |
3-Ureidopropionic acid |
132.0535 |
C4H8N2O3 |
m/z, MS/MS (2) |
0.95 |
−6.9 |
3.82 × 10−15 |
−0.99 |
1.38 |
Urocanic acid |
138.0430 |
C6H6N2O2 |
m/z, MS/MS (2) |
0.58 |
−7.6 |
3.82 × 10−15 |
−0.99 |
4.34 |
Hypoxanthine |
136.0386 |
C5H4N4O |
m/z, RT, MS/MS (1) |
0.55 |
−8.6 |
3.82 × 10−15 |
−0.99 |
2.49 |
Pathway |
Total number of metabolites |
p-value |
log(p-value) |
Adjusted p-valuec |
Pathway impact |
Pathway |
Hits |
(2) Metabolic pathways |
Pyruvate metabolism |
26 |
4 |
2.71 × 10−10 |
22.03 |
3.23 × 10−10 |
0.43 |
Arginine biosynthesis |
16 |
3 |
8.61 × 10−11 |
23.18 |
1.69 × 10−10 |
0.33 |
Alanine, aspartate and glutamate metabolism |
22 |
3 |
3.54 × 10−12 |
26.37 |
2.46 × 10−11 |
0.32 |
Glycine, serine and threonine metabolism |
33 |
5 |
1.11 × 10−13 |
29.83 |
2.98 × 10−12 |
0.28 |
Aminoacyl-tRNA biosynthesis |
45 |
10 |
1.68 × 10−10 |
22.51 |
2.22 × 10−10 |
0.2 |
Arginine and proline metabolism |
29 |
3 |
5.56 × 10−10 |
21.31 |
6.42 × 10−10 |
0.19 |
Butanoate metabolism |
17 |
2 |
7.08 × 10−13 |
27.98 |
1.06 × 10−11 |
0.17 |
D-Glutamine and D-glutamate metabolism |
7 |
1 |
5.46 × 10−12 |
25.93 |
2.46 × 10−11 |
0.17 |
Valine, leucine and isoleucine biosynthesis |
22 |
5 |
9.09 × 10−11 |
23.12 |
1.69 × 10−10 |
0.16 |
Methane metabolism |
26 |
3 |
7.29 × 10−11 |
23.34 |
1.69 × 10−10 |
0.15 |
Glyoxylate and dicarboxylate metabolism |
37 |
4 |
6.82 × 10−12 |
25.71 |
2.79 × 10−11 |
0.11 |
Purine metabolism |
73 |
5 |
2.73 × 10−11 |
24.33 |
8.46 × 10−11 |
0.1 |
Glycolysis/gluconeogenesis |
29 |
1 |
1.23 × 10−10 |
22.82 |
1.69 × 10−10 |
0.08 |
Citrate cycle (TCA cycle) |
20 |
2 |
7.02 × 10−11 |
23.38 |
1.69 × 10−10 |
0.05 |
Pentose phosphate pathway |
26 |
2 |
3.02 × 10−12 |
26.53 |
2.46 × 10−11 |
0.04 |
3.5 Pathway analysis and metabolite network mapping
The significantly changed metabolites identified by comparing the magnetosome and non-magnetosome producing Mgryph cells were submitted for pathway analysis and network mapping using MetaboAnalyst 4.0.28 More than 40 metabolic pathways were found significantly affected in the magnetosome compared to non-magnetosome producing cells (Table S4 in the ESI†) in which glycine, serine and threonine; butanoate; alanine, aspartate and glutamate; aminoacyl-tRNA biosynthesis; and pyruvate and TCA metabolisms were highly affected (Fig. 4C, Table 1). Pathway network mapping in Fig. S1 in the ESI† highlighted the most significantly altered metabolite interactions. The map depicts interacting metabolites across the metabolic pathways identified through specific base-pair alignment. As can be observed in Fig. S1 in the ESI,† L-glutamic acid, pyruvic acid and adenosine monophosphate are the key metabolites showing the highest number of metabolite interactions across the metabolic networks.
3.6 The magnetosome and the amino acid metabolism
Amino acids are key carbon and energy sources for the growth of magnetotactic bacteria.22 They are essential for the growth of Mgryph and usually supplied in the culture using yeast extract which are rich in amino acids. Previous works showed that Mgryph was not be able to sustain growth above 1.5 OD565 when yeast extract was not included in the culture (not published). Such growth under limited amino acid source was approximately 10-fold lower than the highest biomass concentration reached in Mgryph.22 The levels of glutamine, ornithine, hypoxanthine, tryptophan, serine, phenylalanine, glycine, glutamate, 2-oxoglutarate and aspartate were found lower in the magnetosome producing cells compared to fresh media (FSM) (Fig. 6, Table S2 in the ESI†) indicating that these amino acids and their metabolites were significantly utilised during microaerobic growth of Mgryph. FSM contains large quantities of yeast extract and peptone, as detailed in the experimental section, necessary for the growth of Mgryph. Among all amino acids present in FSM, glutamate appeared to be slightly utilised in O2-rich growth conditions whilst it was heavily consumed under O2-limited conditions (Fig. 6, an enlarged version of the pathway map is also available in Fig. S2 in the ESI†), hence glutamate utilisation might be potentially linked to the formation of magnetosomes.
 |
| Fig. 6 Pathway mapping of metabolites detected in the spent medium samples of non-magnetosome (NM), magnetosome (M) producing Mgryph cells and control FSM. Green circle indicates that the metabolite was detected in the samples while the bar graph shows the relative abundance of metabolites expressed as peak areas (arbitrary units). | |
To further understand the decrease of amino acids in the O2-limited growth conditions in comparison to fresh FSM, it is necessary to take into account the proteome associated to magnetosomes and their formation: the conserved genomic magnetosome island (MAI) in Mgryph comprises 28 genes and their associated proteins which are involved in the magnetosome formation.8,39 This cellular machinery is “switched on” under microaerobic conditions.3 Therefore, lower levels of the amino acids, listed above, may indicate that these were utilised in the anabolic metabolism and synthesis of magnetosome-related proteins for structural or enzymatic purposes. Interestingly, it has been previously reported that aspartate, glutamate, proline and serine were the only amino acids significantly utilised by ε-proteobacteria Campylobacter jejuni during microaerobic growth.40 Also, the authors reported that the growth of an aspartase (aspA) mutant of Campylobacter jejuni (unable to utilise any amino acid except serine) and a Cj0762c (aspB) mutant (unable to utilise glutamate), were severely impaired. Recently, microaerobic growth conditions were found to upregulate amino acid synthesis genes in E. coli.41 Our findings, therefore, may indicate that the glycine, serine and threonine, and alanine, aspartate and glutamate metabolisms might be linked to the enhanced utilisation of these amino acids by Mgryph under O2-limited growth conditions where magnetosomes were formed.
3.7 Protein biosynthesis in Mgryph
Aminoacyl-tRNAs are substrates for translation and are pivotal in determining how the genetic code is interpreted as amino acids, thus playing an important role in protein synthesis.42 Aminoacyl-tRNA biosynthesis pathways were significantly enhanced in the magnetosome producing culture, suggesting that they may be related to the biosynthesis of some proteins involved in magnetosome formation. These proteins may include those associated with the complex enzymatic and structural machinery in the iron biomineralisation and the formation of the magnetosome membrane which involves approximately 30 genes.43
3.8 Butyrate metabolism in Mgryph
Butanoate (butyrate) metabolism was significantly altered under O2-limited conditions in comparison to O2-rich conditions (Fig. 4C) and describes the metabolic fate of a number of short chain fatty acids/alcohols. Butanoate metabolism is linked to the formation of hydroxybutyrate, butyrate, acetone, succinate and crotonate. The butanoate metabolism is typically active in a variety of microorganisms that grow under microaerobic or anaerobic conditions, such as the obligate anaerobe Clostridium butyricum44 or lactic acid bacteria.45 As shown in Fig. 1, both cultures grown under O2-limited and O2-rich conditions accumulated large aggregates of PHAs consistent with previous studies in which limiting N2 and O2 under carbon excess resulted in high-level accumulation of PHAs in different bacteria species.46,47 Similar behaviour was also observed in our previous studies,29 whereby the relative content of PHAs decreased in microaerobic cultures after 48 h suggesting that Mgryph utilised PHAs as substrate. Therefore, the significant increase in the butanoate metabolism may indicate that PHAs were significantly utilised in the O2-limited cultures whereas the O2-rich cultures showed lower metabolism by this route.
4. Conclusion
In this study, metabolic characterisation of Mgryph spent media has been performed in magnetosome and non-magnetosome producing conditions using LC-MS. This led to the identification of differentially regulated metabolites in more than 40 metabolic pathways. Our findings indicate that these significant metabolic changes are possibly related to the energy and anabolic metabolism necessary for the growth, the degradation of PHAs and the synthesis of proteins involved in the formation of magnetosomes. This study will play a critical role in improving the understanding of the underlying metabolic mechanisms in Mgryph. Combined with stable isotope assisted approaches,18,48,49 this study can be extended to quantify the intracellular metabolite fluxes in specific stages of Mgryph growth, thus contributing to the further understanding of the metabolic mechanisms that affect the cell growth and the magnetosomes formation. In the long term, we envisage that the significant improvement of both, Mgryph growth and magnetosome production will depend to a large extend, in the application and combination of “omics” technologies along with high-yield bioprocessing strategies. This combination will ultimately accelerate the development of magnetosome-based products in a wide range of biotechnology and nanomedicine, such as drug delivery, magnetic hyperthermia and diagnostic applications.
Conflicts of interest
The authors declare no competing financial interest.
Acknowledgements
This work was supported by the NanoPrime EPSRC grant EP/R025282/1; Royal Society Research grant RGS\R1\191377; the Aston Institute of Materials Research (AIMR) Seed-corn grant; the Energy Research Accelerator (ERA) grant from Innovate UK and the Green Chemicals Beacon of Excellence, University of Nottingham. The authors acknowledge the assistance of the visiting student Jonathan Andraud of the Université Clermont Auvergne for the pre-culture experiments; and Tim W Overton and Owen RT Thomas of the University of Birmingham for enabling the access to the Cmag measurement system.
References
- D. L. Balkwill, D. Maratea and R. P. Blakemore, J. Bacteriol., 1980, 141, 1399–1408 CrossRef CAS.
- N. A. Frey, S. Peng, K. Cheng and S. Sun, Chem. Soc. Rev., 2009, 38, 2532–2542 RSC.
- U. Heyen and D. Schüler, Appl. Microbiol. Biotechnol., 2003, 61, 536–544 CrossRef CAS.
- S. Wilhelm, A. J. Tavares, Q. Dai, S. Ohta, J. Audet, H. F. Dvorak and W. C. W. Chan, Nat. Rev. Mater., 2016, 1, 16014 CrossRef CAS.
- I. Fischer, C. C. Hsu, M. Gärtner, C. Müller, T. W. Overton, O. R. Thomas and M. Franzreb, J. Chromatogr. A, 2013, 1305, 7–16 CrossRef CAS.
- P. Tajer-Mohammad-Ghazvini, R. Kasra-Kermanshahi, A. Nozad-Golikand, M. Sadeghizadeh, S. Ghorbanzadeh-Mashkani and R. Dabbagh, Bioprocess Biosyst. Eng., 2016, 39, 1899–1911 CrossRef CAS.
- K. Grünberg, E. C. Müller, A. Otto, R. Reszka, D. Linder, M. Kube, R. Reinhardt and D. Schüler, Appl. Environ. Microbiol., 2004, 70, 1040–1050 CrossRef.
- R. Uebe and D. Schüler, Nat. Rev. Microbiol., 2016, 14, 621–637 CrossRef CAS.
- A. Fernández-Castané, H. Li, O. R. T. Thomas and T. W. Overton, New Biotechnol., 2018, 46, 22–30 CrossRef.
- F. Guo, Y. Liu, Y. Chen, T. Tang, W. Jiang, Y. Li and J. Li, Appl. Microbiol. Biotechnol., 2011, 90, 1277–1283 CrossRef CAS.
- Y. Zhang, X. Zhang, W. Jiang, Y. Li and J. Li, Appl. Environ. Microbiol., 2011, 77, 5851–5856 CrossRef CAS.
- A. Lohße, I. Kolinko, O. Raschdorf, R. Uebe, S. Borg, A. Brachmann, J. M. Plitzko, R. Müller, Y. Zhang and D. Schüler, Appl. Environ. Microbiol., 2016, 82, 3032–3041 CrossRef.
- T. Suzuki, Y. Okamura, A. Arakaki, H. Takeyama and T. Matsunaga, FEBS Lett., 2007, 581, 3443–3448 CrossRef CAS.
- E. Ozyamak, J. Kollman, D. A. Agard and A. Komeili, J. Biol. Chem., 2013, 288, 4265–4277 CrossRef CAS.
- T. Wen, F. Guo, Y. Zhang, J. Tian, Y. Li, J. Li and W. Jiang, Sci. Rep., 2016, 6, 21156 CrossRef CAS.
- A. Abuawad, C. Mbadugha, A. M. Ghaemmaghami and D.-H. Kim, Metabolomics, 2020, 16, 33 CrossRef CAS.
- M. S. Nadeem, M. Razeeth, H. M. Z. Choudhry, F. Anwar, M. A. Zamzami, B. N. Murtaza, F. A. M. Al-Abbasi, M. I. Khan and A. R. Shakoori, J. Cell. Biochem., 2020, 121, 125–134 CrossRef CAS.
- S. Schatschneider, S. Abdelrazig, L. Safo, A. M. Henstra, T. Millat, D.-H. Kim, K. Winzer, N. P. Minton and D. A. Barrett, Anal. Chem., 2018, 90, 4470–4477 CrossRef CAS.
- F. R. Pinu and S. G. Villas-Boas, Metabolites, 2017, 7, 43 CrossRef.
- J. Kim, Y. E. Cheong, I. Jung and K. H. Kim, Mar. Drugs, 2019, 17, 82 CrossRef CAS.
- N. A. Herman, S. J. Kim, J. S. Li, W. Cai, H. Koshino and W. Zhang, Nat. Commun., 2017, 8, 1514 CrossRef.
- A. Fernández-Castané, H. Li, O. R. T. Thomas and T. W. Overton, New Biotechnol., 2018, 46, 22–30 CrossRef.
- A. Surrati, R. Linforth, I. D. Fisk, V. Sottile and D. H. Kim, Analyst, 2016, 141, 3776–3787 RSC.
- L. Eriksson, E. Johansson, N. Kettaneh-Wold, C. W. J. Trygg and S. Wold, Multi- and Megavariate Data Analysis: Basic Principles and Applications, Umetrics AB, Umea, 2nd edn, 2006 Search PubMed.
- L. W. Sumner, A. Amberg, D. Barrett, M. H. Beale, R. Beger, C. A. Daykin, T. W. M. Fan, O. Fiehn, R. Goodacre, J. L. Griffin, T. Hankemeier, N. Hardy, J. Harnly, R. Higashi, J. Kopka, A. N. Lane, J. C. Lindon, P. Marriott, A. W. Nicholls, M. D. Reily, J. J. Thaden and M. R. Viant, Metabolomics, 2007, 3, 211–221 CrossRef CAS.
- L. W. Sumner, Z. Lei, B. J. Nikolau, K. Saito, U. Roessner and R. Trengove, Metabolomics, 2014, 10, 1047–1049 CrossRef CAS.
- Y. Benjamini and Y. Hochberg, J. R. Stat. Soc. Ser. B Methodol., 1995, 57, 289–300 Search PubMed.
- J. Chong, D. S. Wishart and J. Xia, Curr. Protoc. Bioinf., 2019, 68, e86 Search PubMed.
- A. Fernández-Castané, H. Li, O. R. T. Thomas and T. W. Overton, Sci. Rep., 2017, 7, 13118 CrossRef.
- X. Wang, H. Zheng, Q. Wang, W. Jiang, Y. Wen, J. Tian, J. Sun, Y. Li and J. Li, Front. Microbiol., 2019, 10, 1478 CrossRef.
- O. Begou, H. G. Gika, G. A. Theodoridis and I. D. Wilson, in Metabolic Profiling: Methods and Protocols, ed. G. A. Theodoridis, H. G. Gika and I. D. Wilson, Springer New York, New York, NY, 2018, pp. 15–26, DOI:10.1007/978-1-4939-7643-0_2.
- E. J. Want, I. D. Wilson, H. Gika, G. Theodoridis, R. S. Plumb, J. Shockcor, E. Holmes and J. K. Nicholson, Nat. Protoc., 2010, 5, 1005–1018 CrossRef CAS.
- H. G. Gika, G. A. Theodoridis, J. E. Wingate and I. D. Wilson, J. Proteome Res., 2007, 6, 3291–3303 CrossRef CAS.
- P. Yin, D. Wan, C. Zhao, J. Chen, X. Zhao, W. Wang, X. Lu, S. Yang, J. Gu and G. Xu, Mol. BioSyst., 2009, 5, 868–876 RSC.
- M. Wang, J. Fu, X. Zhang and T. Chen, Biotechnol. Lett., 2012, 34, 1877–1885 CrossRef.
- J. Zhu, A. Sánchez, G. N. Bennett and K.-Y. San, Metab. Eng., 2011, 13, 704–712 CrossRef CAS.
- G. Gouesbet, M. Jebbar, R. Talibart, T. Bernard and C. Blanco, Microbiology, 1994, 140, 2415–2422 CrossRef CAS.
- A. R. Strøm and I. Kaasen, Mol. Microbiol., 1993, 8, 205–210 CrossRef.
- K. Grünberg, E.-C. Müller, A. Otto, R. Reszka, D. Linder, M. Kube, R. Reinhardt and D. Schüler, Appl. Environ. Microbiol., 2004, 70, 1040–1050 CrossRef.
- E. Guccione, R. Leon-Kempis Mdel, B. M. Pearson, E. Hitchin, F. Mulholland, P. M. van Diemen, M. P. Stevens and D. J. Kelly, Mol. Microbiol., 2008, 69, 77–93 CrossRef CAS.
- K. Pandi, A. S. Chauhan, J. A. Gupta and A. S. Rathore, Appl. Microbiol. Biotechnol., 2020, 104(13), 5773–5785 CrossRef CAS.
- M. Ibba and D. Soll, Annu. Rev. Biochem., 2000, 69, 617–650 CrossRef CAS.
- O. Raschdorf, Y. Forstner, I. Kolinko, R. Uebe, J. M. Plitzko and D. Schüler, PLoS Genet., 2016, 12, e1006101 CrossRef.
- M. Salmela, T. Lehtinen, E. Efimova, S. Santala and R. Mangayil, Biotechnol. Biofuels, 2018, 11, 187 CrossRef.
- G. A. M. Cresci, P. C. Mayor and S. A. Thompson, FEMS Microbiol. Lett., 2017, 364 Search PubMed.
- A. C. Ward, B. I. Rowley and E. A. Dawes, Microbiology, 1977, 102, 61–68 CrossRef CAS.
- B. Kessler and B. Witholt, J. Biotechnol., 2001, 86, 97–104 CrossRef CAS.
- D.-H. Kim, F. Achcar, R. Breitling, K. E. Burgess and M. P. Barrett, Metabolomics, 2015, 11, 1721–1732 CrossRef CAS.
- D. J. Creek, M. Mazet, F. Achcar, J. Anderson, D. H. Kim, R. Kamour, P. Morand, Y. Millerioux, M. Biran, E. J. Kerkhoven, A. Chokkathukalam, S. K. Weidt, K. E. Burgess, R. Breitling, D. G. Watson, F. Bringaud and M. P. Barrett, PLoS Pathog., 2015, 11, e1004689 CrossRef.
Footnote |
† Electronic supplementary information (ESI) available. See DOI: 10.1039/d0ra05326k |
|
This journal is © The Royal Society of Chemistry 2020 |