DOI:
10.1039/D0RA04949B
(Paper)
RSC Adv., 2020,
10, 25929-25946
Bleomycin modulates amyloid aggregation in β-amyloid and hIAPP†
Received
4th June 2020
, Accepted 29th June 2020
First published on 9th July 2020
Abstract
Aberrant misfolding and amyloid aggregation, which result in amyloid fibrils, are frequent and critical pathological incidents in various neurodegenerative disorders. Multiple drugs or inhibitors have been investigated to avert amyloid aggregation in individual peptides, exhibiting sequence-dependent inhibition mechanisms. Establishing or inventing inhibitors capable of preventing amyloid aggregation in a wide variety of amyloid peptides is quite a daunting task. Bleomycin (BLM), a complex glycopeptide, has been widely used as an antibiotic and antitumor drug due to its ability to inhibit DNA metabolism, and as an antineoplastic, especially for solid tumors. In this study, we investigated the dual inhibitory effects of BLM on Aβ aggregation, associated with Alzheimer's disease and hIAPP, which is linked to type 2 diabetes, using both computational and experimental techniques. Combined results from drug repurposing and replica exchange molecular dynamics simulations demonstrate that BLM binds to the β-sheet region considered a hotspot for amyloid fibrils of Aβ and hIAPP. BLM was also found to be involved in β-sheet destabilization and, ultimately, in its reduction. Further, experimental validation through in vitro amyloid aggregation assays was obtained wherein the fibrillar load was decreased for the BLM-treated Aβ and hIAPP peptides in comparison to controls. For the first time, this study shows that BLM is a dual inhibitor of Aβ and hIAPP amyloid aggregation. In the future, the conformational optimization and processing of BLM may help develop various efficient sequence-dependent inhibitors against amyloid aggregation in various amyloid peptides.
Introduction
Amyloid peptides have several biological functions, varied sequences, and lengths, and their native 3D structures can misfold into β-sheet-rich amyloid aggregates, which are the pathological hallmarks of several neurodegenerative disorders, such as Alzheimer's disease (AD), Parkinson's disease (PD), and type 2 diabetes (T2D).1–3 Each of these disorders is linked to protein misfolding and amyloid aggregation of distinct amyloid peptides, such as amyloid beta (Aβ) in AD, alpha-synuclein (α-syn) in PD, and human islet amyloid polypeptide (hIAPP) in T2D. Although amyloid aggregation is a convoluted nucleation and polymerization mechanism with various conformational intermediates and transitions from disorganized monomers to transitional oligomers, protofilaments, and complex matured fibrils,4,5 it is commonly acknowledged that inhibiting the amyloid aggregation of misfolding amyloid peptides plays a significant role in understanding and curing these disorders.6–10
Previous attempts to discover or develop various types of inhibitors for the amyloid aggregation of intrinsically disordered peptides (IDPs) investigated small organic molecules (e.g., carbenoxolone,11,12 fullerene and fullerenols,13 fullerenemalonates,14 EGCG,15–20 oil palm phenolics,21 curcumin,22–32 oleuropein,33–35 and tanshinone36), various nanoparticles (e.g., GQD-T,37,38 CeONP@POMs,39 Res@SeNPs,40 L1T1-SeNPs,41 AgNPs,42–48 and IDA-NP49), selective peptide inhibitors (e.g., Aβ39–42,50 Aβ31–42,51 Aβ17–21,52 and Aβ16–20,53 including analogues54–56), and several polymers (e.g., polyA-FF-ME,57 poly-[amidoamine] dendrimer,58 and poly[acrylic acid]59). Most of these amyloid inhibitors act on specific amyloid peptides and show a higher capacity for inhibition based on sequence dependence. Very few of them have been studied to explore dual inhibition against the aggregation of amyloid peptides. As there is substantive evidence to prove that T2D, obesity, and prediabetic conditions of insulin resistance60 might stimulate AD growth.61–63 Patients with T2D are two to five folds more susceptible to develop AD,63,64 and the frequency of alteration from trivial cognitive damage to dementia instigated by AD is greater in people with a prior antiquity of T2D.65 Additionally, peripheral insulin resistance is more often in patients with AD than in neurologically asymptomatic matured cases,66 and the flawed brain insulin action in AD67 appears to be related with peripheral insulin resistance.68 Though, the process by which patients with AD deprived of T2D reveal peripheral insulin resistance, and whether peripheral or central dysregulation of insulin signaling starts the pathological measures in AD, are weakly understood.68
Non-neuronal tissues of several organs like liver, skeletal muscle, and pancreas vital for glucose regulation have been involved in Aβ aggregation.69–71 Also, human pancreas are found to be convoluted on expression of spliced tau isoforms,72 and an enhancement of hyperphosphorylated tau in the pancreatic tissue is linked with T2D.70 Hence, these facts point toward a strong link among the two diseases, and among peripheral and brain insulin resistance. Nevertheless, to our acquaintance the role of pancreas in AD pathogenesis has not yet been scrutinized. Recently, Paul et al. determined the anti-amyloidogenic effects of naphthoquinone tryptophan-based hybrid molecules against τ-derived aggregation peptide (PHF6), Aβ, and hIAPP, which are involved in AD and T2D.73 Genistein has also been found to show inhibitory activity against Aβ and hIAPP and has been utilized extensively as a cerebrovascular and anti-inflammatory drug because of its anti-acetylcholinesterase and antioxidation effects.74 A well-known natural compound, polyphenol (−)epigallocatechin gallate (EGCG), reportedly binds to a variety of amyloid peptides of κ-casein and α-synuclein, hindering their aggregation pathways and misfolding, and, therefore, exhibits prevalent inhibitory effects.75,76 Pentagalloyl glucose has also been proclaimed a dual inhibitor of Aβ and hIAPP, which prevents fibrillization in both Aβ and hIAPP and helps in the disassembly of existing Aβ fibrils.77,78 Mariano et al. introduced bis(hydroxyphenyl)-substituted thiophenes as selective dual inhibitors of both Aβ aggregation and tau kinase dual-specificity tyrosine phosphor-regulated kinase 1A (Dyrk1A).79 Fragment-based QSAR and molecular docking studies have been conducted to develop dual inhibitors among 20 1,4-dihydropyridine (DHP) derivatives against β-amyloid cleavage enzymes (BACE-1) and acetylcholine (AChE).80 Mancini et al. demonstrated that a component of brewed coffee, phenylindanes, help in the inhibition of Aβ and tau aggregation and are a probable mechanism by which coffee exhibits neuroprotective effects.81 Curcumin, obtained from turmeric, has also been found to have inhibitory effects, and the ability to disassemble and prevent the fibril formation of Aβ and α-synuclein in a dose-dependent, destabilizing manner.82 The major component of the herb danshen (Salvia miltiorrhiza Bunge), tanshinones, are also involved in amyloid aggregates' inhibition of Aβ and hIAPP, including disaggregate-achieved hIAPP and Aβ amyloid fibrils, and they defend cells from hIAPP- and Aβ-induced toxicity.36,83 Despite these discoveries, there is still no cure for these fatal neurodegenerative disorders, and the challenge remains for researchers to invent potent dual or multiple inhibitors against the amyloid aggregation and fibrillization of different amyloid peptides.
Drug repositioning (i.e., drug repurposing, reprofiling, or re-tasking) involves determining new uses for approved or investigational drugs that are further the opportunity of the real medical action.84 This approach offers several benefits over establishing a completely new drug for a particular purpose. The best and, perhaps, the most vital aspect of drug repositioning is that the risk of failure is quite low because reprofiled drugs have already undergone early phase trials in preclinical animal models and human testing and have been found to be sufficiently safe. Hence, they are less likely to fail, at least from a safety standpoint, in subsequent efficacy trails. Furthermore, the duration of drug development can be decreased since most of the preclinical trials, safety appraisals, and, in some cases, formulation development, have already been completed. Moreover, minimal investment is required for drug reprofiling, but costs may vary depending on the phase and mechanism of development of the reprofiling candidate.85 Recently, drug repurposing using the neuroinformatics approach was implemented to study the inhibitory mechanism of bexarotene, an anticancer drug against Aβ amyloid aggregation.86 Doxycycline (Doxy), a widely used antibiotic, has been found to exhibit neuroprotective effects by decreasing the progression and severity of disease in various experimental models of neurodegeneration by neutralizing these common features.87–94 Bortolanza et al. proposed repurposing tetracyclines, which are a multitarget antibiotic but also utilized to treat PD.95 Hayes et al. investigated their effects on arresting or decreasing amyloid plaques loaded in an AD mouse model after chronic treatment with carmustine.96
In this study, we investigated the dual inhibition effect against the amyloid aggregation of Aβ and hIAPP using both computational and experimental approaches. To achieve this goal, we performed drug repurposing through high-throughput virtual screening of the Food and Drug Administration (FDA)-approved drugs library against Aβ and hIAPP, followed by extra-precision docking to identify potent inhibitors of amyloid aggregation. We analyzed the most potent inhibitor (i.e., bleomycin [BLM]) using several energy and docking score-based parameters. Furthermore, atomistic conformational sampling using replica exchange molecular dynamics (REMD) simulations of Aβ and hIAPP both in the presence and absence of BLM suggests strong interactions among BLM and amyloid peptides (Aβ and hIAPP), and potent binding sites were identified that might be responsible for inhibiting the amyloid aggregation of these peptides by BLM. Additionally, experimental validations were performed by utilizing thioflavin T (ThT) fluorescence assay and transmission electron microscopy, which yielded results consistent with our computational studies. The repurposed drug (i.e., BLM) may serve as a leading structural template for the future development of BLM-based inhibitors of various amyloid peptides.
Material and methods
Drug repurposing
High throughput virtual screening of Aβ and hIAPP against the FDA-approved drugs library was performed using GLIDE,97 a module of Schrodinger.98 The 3D structures of AD-associated Aβ1–42 (PDB ID: 1Z0Q) and T2D-associated hIAPP1–37 (PDB ID: 2L86) were downloaded from the database RCSB PDB.99 The sequence 1DAEFRHDSGYEVHHQKLVFFAEDVGSNKGAIIGLMVGGVVIA42 was used for Aβ1–42 and 1KCNTATCATQRLANFLVHSSNNFGAILSSTNVGSNTY37 for hIAPP1–37; they were pre-processed, optimized, and minimized using Schrödinger's protein preparation wizard by enumerating missing atoms, amending the overlapping coordinates, assigning bond orders, adding hydrogen and minimizing energy via 0.30 Å RMSD, and optimizing the force field (i.e., OPLS 2005 (ref. 100)), as generally required for docking simulations.101 The FDA-approved drug library of 2162 compounds was prepared using the LigPrep102 module, and all probable states at pH 7 ± 2 were developed by employing an ionizer and confining it to the exact chirality of molecules. Twenty-five ligands were achieved per ligand molecule, and the results were saved for docking in the maestro format. Two-step docking was performed: high-throughput virtual screening (HTVS) and extra precision (XP) docking.103 Depending on the docking score, the presence of the same lead compound for both Aβ and hIAPP docking results were investigated. The protein-ligand interactions for the same lead compound separately with Aβ and hIAPP, were analyzed using LigPlot+104 and Chimera.105
REMD simulation
The same initial structures of Aβ and hIAPP prepared by the protein preparation wizard were utilized for atomistic conformational sampling using REMD in the presence and absence of the selected lead compound. Aβ and hIAPP were simulated at 500 K to obtain extended coil states and avoid bias in the initial secondary structures. Additionally, the amidation and acetylation of C and N terminals were performed to reduce the liability of sudden results from uncapped and non-neutralized ends of Aβ and hIAPP. The lead compound's 3D structure was downloaded from PubChem106 in SDF format, and its GROMACS coordinates and topology files were prepared using PRODRG.107
Four separate REMD simulation systems for both Aβ and hIAPP in the presence and absence of the same lead compound were performed using GROMACS.108 Newton's equation of motion was incorporated with a time step of 2 fs using the leapfrog algorithm integrator. van der Waals interactions were determined, along with short- and long-range electrostatics and the particle-mesh Ewald algorithm.109 It was combined with a fast Fourier transform algorithm to decrease the barriers of MD simulations. Periodic boundary conditions were also applied to improvise system size effects, and an OPLS force field was used to develop Aβ and hIAPP topologies. Furthermore, the water-model used as a solvent was the transferable intermolecular potential with three-point (TIP3P) rigid water,110 with the addition of charged ions to neutralize the charges of Aβ and hIAPP.
First, the four REMD simulation systems were NPT equilibrated to obtain a static number of atoms and constant pressure and temperature (i.e., isotropic pressure for 1 bar and at 300 K), applying the Berendsen weak coupling thermostat and a barostat111 to accomplish equilibrated box dimensions at a compressibility of 4.5 × 10−5 bar−1. Also, NVT equilibrium conditions with a static number of atoms and a constant volume and temperature were employed via a Nose–Hoover thermostat112 and an advanced Hamiltonian combined superficially with the heat-bath to achieve precise thermodynamic ensembles. Separate peptide and water bonds were constrained via the linear constraint solver algorithm (LINCS)113 and SETTLE algorithm,114 respectively. Both algorithms use Lagrange multipliers and a symplectic integrator to constrain chemical bonds.
In these conditions, REMD simulations were performed to enhance conformational sampling. Several replicas were run with marginally alternative ensembles, swapping the coordinates of replicas within the ensembles periodically. In recent studies, temperatures were selected and distributed based on the exponential spacing law to perform REMD.115 Another method used in previous studies116 is the webserver at http://folding.bmc.uu.se/remd,117 which we used in this study for temperature selection in REMD simulations of each case of 32 replicas with a 20% average exchange probability within replica purses every 2 ps. The peptide in each of the four systems was simulated for 100 ns per replica, and further analysis was performed on the final trajectories.
REMD analysis
A series of trajectory analyses were performed using GROMACS tools and built-in scripts. First, energy components or the distance restraint inputs from the energy file were obtained using the GROMACS in-house command ‘g_energy’. We also confirmed that all physicochemical properties of the system had reached equilibrium, where their averages no longer altered as a function of time. The simplest way to measure stability was by calibrating the root mean square deviation (RMSD) using ‘g_rms’. Similarly, root-mean-square fluctuation (RMSF) analysis was performed using ‘g_rmsf’ to measure the peptides' flexibility, thereby detecting changes in C-alpha atoms' coordinates from their initial position. Hydrogen bonds were calculated using ‘g_hbond’ to detect the peptides' folding or unfolding behavior, peptides' interaction with the lead compound calculated with an oxygen–hydrogen–nitrogen angle of 30° or less, along with an oxygen–hydrogen distance of 2.5 Å or less.
Furthermore, to predict the compactness of Aβ and hIAPP, the radius of gyration (Rg) about the center of mass was measured using ‘g_gyrate’, and the peptides' end-to-end distance (Ree) was determined using ‘g_polymer’. Ree was calculated from the C-terminal's center of mass (i.e., the acetylated end) to the N-terminal's center of mass (i.e., the amidated end). Secondary structure analysis was also conducted using ‘do_dssp’, which performs pattern recognition of hydrogen-bonded and geometric characteristics. Cluster analysis of the peptides was also performed to predict the similar structures sampled together in the course of MD simulations using ‘g_cluster’ with a cutoff of 0.35 nm. Daura's118 algorithm was employed on MD trajectories, so the peptide conformations could be clustered together to compare several orientations of the protein backbone without terminals, and clusters were grouped together based on RMSD. The radial distribution function (RDF) of water and the lead compound corresponding to the peptides' surface and the lead compound's center of mass were determined using ‘g_rdf’.
Population density analysis
Population density analysis was performed using GNUPLOT 5.0, a convenient command-line tool used extensively for graphing and contouring in different operating systems. Population density analysis accounts for the well-known entities of associated processes and develops them across the landscape based on the quantity that is measured at each location and the spatial relationship of the locations of the measured quantities. The population density plots representing a function of Rg and Ree for each were plotted in GNUPLOT 5.0. Population density plots for salt-bridges among distant and nearby residues of the peptide were also analyzed.
Experimental conditions
Preparation of peptides. Aβ and hIAPP peptides with the sequences 1DAEFRHDSGYEVHHQKLVFFAEDVGSNKGAIIGLMVGGVVIA42 and 1KCNTATCATQRLANFLVHSSNNFGAILSSTNVGSNTY37 were purchased from GenPro Pvt., Ltd. (India) at purity levels of 98%. The mass, purity, and quantity of both these peptides were ascertained by performing analytical HPLC, combination matrix-assisted laser desorption/ionization time-of-flight mass spectrometry, and quantitative amino acid analyses.
Stock preparation of peptides
Aβ. A stock solution of Aβ was prepared as reported previously.119 Briefly, 1 mM of Aβ solution was prepared by dissolving the lyophilized Aβ peptide in 100% hexafluoroisopropanol (HFIP). Equal aliquots of Aβ solution were then distributed in sterile 1.5 ml micro-centrifuge tubes. HFIP was removed under vacuum using Speed Vac, and the dried peptide was then stored at −20 °C for future use. An aliquot of Aβ peptide was resuspended in dry DMSO prior to each experiment.
hIAPP. A stock solution of hIAPP was prepared as described previously by Cao et al.,120 with slight modifications. Briefly, 100% hexafluoroisopropanol (HFIP) was added to the lyophilized peptide and allowed to dissolve, yielding a final peptide solution of 615 μM. This solubilized peptide was filtered through a 0.2 μm filter, divided into aliquots in sterile micro-centrifuge vials, and stored at −20 °C until further experimentation.
Thioflavin-T (ThT) fluorescence kinetics
hIAPP. Amyloid aggregation was initiated by diluting the peptide to a final concentration of 16 μM in 20 mM Tris–HCl (pH 7.4) in the absence and presence of BLM. Peptide:BLM molar ratios of 1
:
1, 1
:
2, and 1
:
5 were used, as in the case of Aβ, to test the efficacy of BLM in controlling the fibrillation of hIAPP. The aggregation reaction was set up in triplicates for all the tested conditions in a Nunc F96 Microwell Plate (Thermo Scientific) at 25 °C with continuous rotation in a Perkin Elmer-Enspire multimode plate reader.To quantify amyloid fibrillation, ThT was added to each of the reaction wells at a final concentration of 25 μM at the beginning of the aggregation experiment, along with the other components. ThT fluorescence was monitored continuously at excitation and emission wavelengths of 450 nm and 485 nm, respectively, using the same instrument.
Additionally, efficiency of fibrillization inhibition and t-test statistical analysis was performed on the acquired ThT data, to scrutinize the effectiveness of BLM treatment in countering the individual aggregation of both these peptides. The test was conducted by comparing readings of the treated peptide samples (individually for all the tested molar concentration ratios) with those of the control aggregation sample, for both the peptides.
Transmission electron microscopy (TEM)
Visualization of the Aβ and hIAPP aggregation samples was performed by transmission electron microscopy. At the end of a kinetic run, a 10 μl sample was taken from all the tested treatments and allowed to adsorb on Formvar carbon-coated copper mesh grids (Agar Scientific Formvar 300 mesh). The samples were negatively stained with an aqueous solution of uranyl acetate, followed by washing with distilled water, and finally, air-dried before visualization on a JEM-2100F (JEOL, USA) transmission electron microscope operated at 200 kV.
Results
This study was designed to investigate the dual inhibition effect against the amyloid aggregation of Aβ and hIAPP, which are responsible for AD and T2D, respectively. The comprehensive computational and experimental approach was implemented mainly through drug repurposing, replica exchange molecular dynamics simulations, and biophysical characterizations of Aβ and hIAPP, both in the presence and absence of a common lead compound.
Selection of the most potent inhibitor through drug repurposing
The FDA-approved drug library of compounds showing docking scores greater than –6kcal mol−1 in HVTS was further employed for the XP docking protocol. GlideScore estimates the ligand binding free energy by considering force field conditions and other individual conditions rewarding or penalizing ligand binding. Therefore, most of the drugs docked with Aβ and hIAPP showed an XP GlideScore greater than −7 kcal mol−1. Among them, we found the common drug bleomycin (BLM) showed a good GlideScore of −8.38 and −9.47 kcal mol−1 for Aβ and hIAPP, respectively. BLM was selected for further analysis based on various ligand-binding parameters, such as glide_energy and glide_hbond (Table 1). Glide_hbond explains the sum of individual hydrogen bond scores between the ligand and target. More negative values for glide_hbond indicate stronger hydrogen bonds and the type of atoms, along with the geometry of atoms affecting the glide_hbond scores. Glide_evdw provides information about protein-ligand steric interactions. A combined value of ‘glide_evdw and glide_ecoul’ gives glide_energy. Glide_emodel is important for correlating various conformers and utilized by glide to select the best pose and rank of ligands. BLM was found to show a good glide_emodel value, suggesting a better pose for Aβ and hIAPP binding pockets.
Table 1 Result of XP docking parameters for Aβ + BLM and hIAPP + BLM
Glide parameters |
Aβ + BLM |
hIAPP + BLM |
GlideScore (kcal mol−1) |
−8.38 |
−9.47 |
Glide_evdw (kcal mol−1) |
−49.96 |
−60.16 |
Glide_ecoul (kcal mol−1) |
−30.63 |
−26.34 |
Glide_emodel (kcal mol−1) |
−80.14 |
−78.29 |
Glide_energy (kcal mol−1) |
−80.59 |
−86.50 |
Glide_hbond (kcal mol−1) |
−0.55 |
−1.09 |
A ligand–protein interaction diagram showing the binding residues for Aβ or hIAPP with BLM is shown in Fig. 1. The interactions among BLM and Aβ and hIAPP separately showed strong hydrogen bonding with residues Glu3, Asp7, Glu11, and Ser26, along with various hydrophobic interactions with residues Phe4, Gln15, Val18, Gln22, Asp23, Asn27, Ala30, Ile41, and Ala42 in case of Aβ, and similarly, residues Asn3, Arg11, Asn14, Ser28, Asn31, Asn35, and Tyr37 showed hydrogen bonds, including some hydrophobic interactions with residues Lys1, Leu12, Phe15, Asn21, Asn22, Ala25, Ser29, Thr30, and Ser34 for hIAPP. The quantitative analysis of hydrogen bond formation between Aβ and BLM, as well as hIAPP and BLM, is shown in Table 2. Here, all the hydrogen bond interactions have a bond length within 3.0–3.2 Å (Table 2).
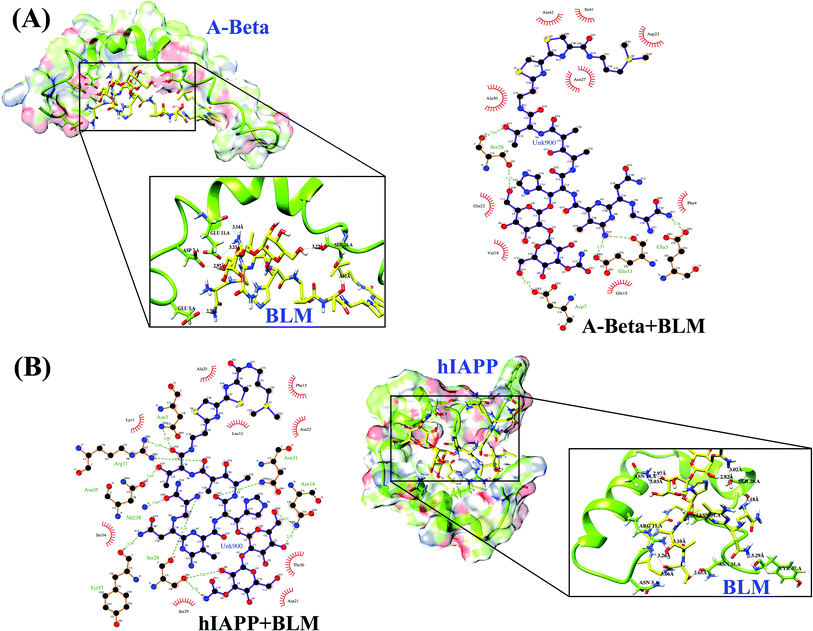 |
| Fig. 1 Protein ligand interaction (2D and 3D) diagram (A) Aβ and BLM (B) hIAPP and BLM. | |
Table 2 Intermolecular hydrogen bonds and hydrophobic residues showing close contact between Aβ and hIAPP with BLM
Interacting residue |
H-bond distance (Å) |
H-bond (D–H⋯A) |
Hydrophobic residues |
Aβ + BLM |
GLU 3.A OE2 – UNK900.hetN13 |
2.78 |
HOE2-H⋯N13 |
Phe4, Gln15, Val18, Gln22, Asp23, Asn27, Ala30, Ile41, Ala42 |
ASP 7.A OD2 – UNK900.hetO11 |
2.97 |
HOD2-H⋯O11 |
|
GLU 11.A OE2 – UNK 900.het N10 |
3.33 |
HOE2-H⋯N10 |
|
GLU 11.A O – UNK 900.het N10 |
3.14 |
HO-H⋯N10 |
|
SER 26.A OG – UNK 900.het O10 |
3.22 |
HOG-H⋯O10 |
|
SER 26.A O – UNK 900.het O17 |
3.01 |
HO-H⋯O17 |
|
![[thin space (1/6-em)]](https://www.rsc.org/images/entities/char_2009.gif) |
hIAPP + BLM |
ASN 3.A ND2 – UNK 900.het O18 |
3.06 |
HND2-H⋯O18 |
Lys1, Leu12, Phe15, Asn21, Asn22, Ala25, Ser29, Thr30, Ser34 |
ARG 11.A NH1 – UNK 900.het O18 |
3.26 |
HNH1-H⋯O18 |
|
ARG 11.A NH2 – UNK 900.het O16 |
3.10 |
HNH2-H⋯O16 |
|
ASN 14.A ND2 – UNK 900.het O6 |
2.97 |
HND2-H⋯O6 |
|
ASN 14.A ND2 – UNK 900.het O10 |
3.03 |
HND2-H⋯O10 |
|
SER 28.A O – UNK 900.het N13 |
3.18 |
HO-H⋯N13 |
|
SER 28.A OG – UNK 900.het O8 |
2.82 |
HOG-H⋯O8 |
|
SER 28.A OG – UNK 900.het N5 |
3.02 |
HOG-H⋯N5 |
|
ASN 31.A ND2 – UNK 900.het O12 |
2.85 |
HND2-H⋯O12 |
|
ASN 35.A OD1 – UNK 900.het O17 |
2.65 |
HOD1-H⋯O17 |
|
TYR 37.A O – UNK 900.het N12 |
3.29 |
HO-H⋯N12 |
|
REMD simulations analysis of Aβ and hIAPP in the presence and absence of BLM
The drug repurposing results presented above suggest that BLM has a strong potential to bind with Aβ and hIAPP and prevent their amyloid aggregation. To check the hypothesis, we performed REMD simulations to investigate conformational modulations, determine how BLM interacts with Aβ and hIAPP, and determine the specific interacting modes between BLM and Aβ and between BLM and hIAPP. REMD was performed for all four systems (i.e., Aβ, Aβ + BLM, hIAPP, and hIAPP + BLM) in explicit solvent environments, and for Aβ + BLM and hIAPP + BLM systems, BLM molecules were randomly added around Aβ and hIAPP with arbitrary orientations. REMD implemented a total of 32 replicas in each system for a wide range of temperatures, and the trajectories were analyzed at 300 K.
Simulation convergence estimation was achieved, and the average acceptance ratio was found to be more than 20% for each case. The convergence of the REMD simulation was investigated using trajectories, which revealed that the temperature space was explored widely by each replica throughout the simulation time. We also investigated the effect of BLM binding on the structural stability of Aβ and hIAPP peptides. A visual inspection of all REMD trajectories indicates that BLM interactions did not broadly disrupt the structural probity and secondary structure of Aβ and hIAPP, enabling them to maintain their folded structures either in the presence or absence of BLM.
RMSD analysis of the peptides gave insights into their structural conformation during REMD simulations, providing an explanation for the peptides' stability and determining whether the simulation had equilibrated. The average backbone RMSD for all Aβ and hIAPP systems varied (0.5–1.5 nm and 0.5–2.0 nm, respectively) and attained various conformations over the course of the REMD simulation time (Fig. 2A). The stable curve for the RMSD of the peptides during the course of the simulation shows that the REMD simulations were ideal for further analysis. Furthermore, the RMSF curve for each residue of Aβ and hIAPP was determined, and the peaks showed local residual fluctuations along with the peptide during the REMD simulations. Upon analyzing the RMSF curve, both N- and C-terminals residues were found to show more fluctuations than the other residues of Aβ and hIAPP (Fig. 2B). Moreover, the residues of Aβ and hIAPP in the presence of BLM were found to show relatively similar fluctuations as the Aβ or hIAPP system individually, except for hIAPP in the presence of BLM, which reduced residue fluctuation at the C-terminal.
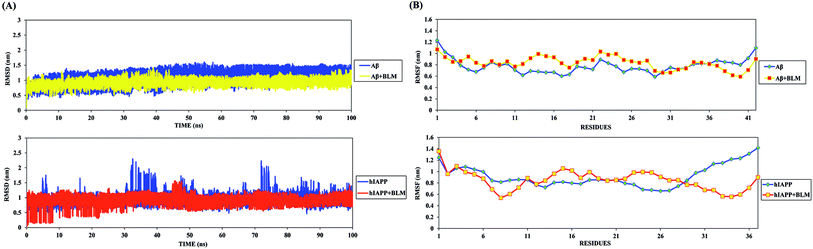 |
| Fig. 2 (A) Root mean square deviation (RMSD) plots for Aβ [top left] and hIAPP [bottom left] in presence and absence of BLM which demonstrates that the peptide adopted various conformations. (B) Root mean square fluctuation (RMSF) plots for all residues of Aβ [top right] and hIAPP [bottom right] in presence and absence of BLM. | |
Radius of gyration and peptide end-to-end distance population density analysis
Furthermore, the distribution of data-points for the entire REMD simulation time against the global parameters (i.e., the radius of gyration [Rg] and end-to-end peptide distance [Ree]) was determined by population density analysis for all four REMD simulations systems (Fig. 3). The Rg value was found to be about 1 nm and similar in all the four systems, while Ree was increased in the presence of BLM for the Aβ and hIAPP systems, from 2 to 3 nm and 0.5 to 2.5 nm, respectively. The densely populated region is represented in blue, which indicates most of the coordinates reside in that area, and yellow, green, red, and white show the less densely populated areas. The relative observation attained from population density graphs for all four systems confirms that the incorporation of BLM in Aβ or hIAPP resulted in shifting the densely populated region (i.e., the blue color shifted to higher coordinates). Table 3 shows the average Rg and Ree values measured for all four systems. These results clearly show that Aβ + BLM and hIAPP + BLM had higher Ree values, which led to the peptides being in extended unfolded conformations.
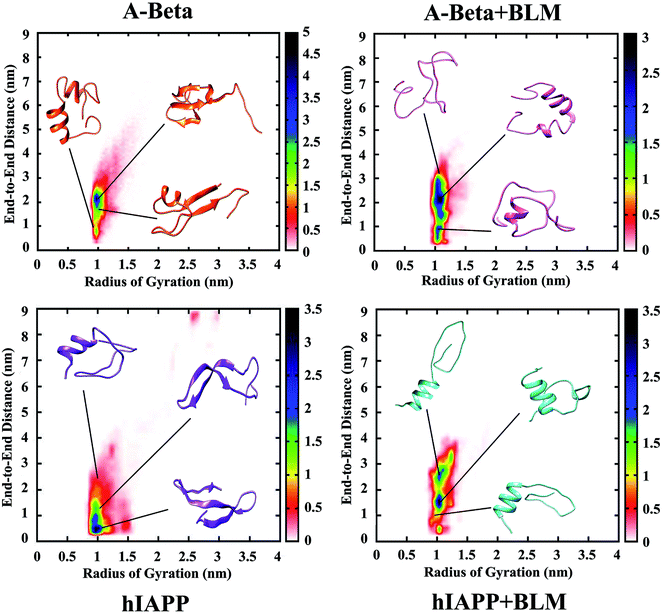 |
| Fig. 3 Population density analysis for Aβ and hIAPP in presence and absence of BLM, peptide end to end distance (Ree) i.e. C to N terminal and radius of gyration (Rg) around its center of mass. Blue part implies the heavily populated conformations, whereas red and yellow part indicates the limited populated conformations. | |
Table 3 Average Rg and Ree values measured for all four systems
Peptide in respective solvent/s |
Average Ree |
Average Rg |
Aβ |
2.316 nm |
1.084 nm |
Aβ + BLM |
2.510 nm |
1.155 nm |
hIAPP |
2.227 nm |
1.192 nm |
hIAPP + BLM |
2.366 nm |
1.189 nm |
Intra- and intermolecular hydrogen bond analysis
The quantitative analysis of intra- and intermolecular hydrogen bonds of Aβ or hIAPP with BLM were further analyzed by determining the probability% of hydrogen bonds. The presence of BLM led to the decreased formation of intramolecular hydrogen bonds and the increased formation of intermolecular hydrogen bonding with Aβ or hIAPP, respectively. As a control, both Aβ and hIAPP were shown to have a higher intramolecular hydrogen bond probability of 12.5% (27 hydrogen bonds) and 11.2% (13 hydrogen bonds), while Aβ + BLM and hIAPP + BLM were showed a lower intramolecular hydrogen bond probability of 8.2% (20 hydrogen bonds) and 10.8% (18 hydrogen bonds), respectively (Fig. 4A). The maximum intermolecular hydrogen bond probability was found to be 19.2% (2 hydrogen bonds) for Aβ + BLM and 18.9% (2 hydrogen bonds) for hIAPP + BLM (Fig. 4B).
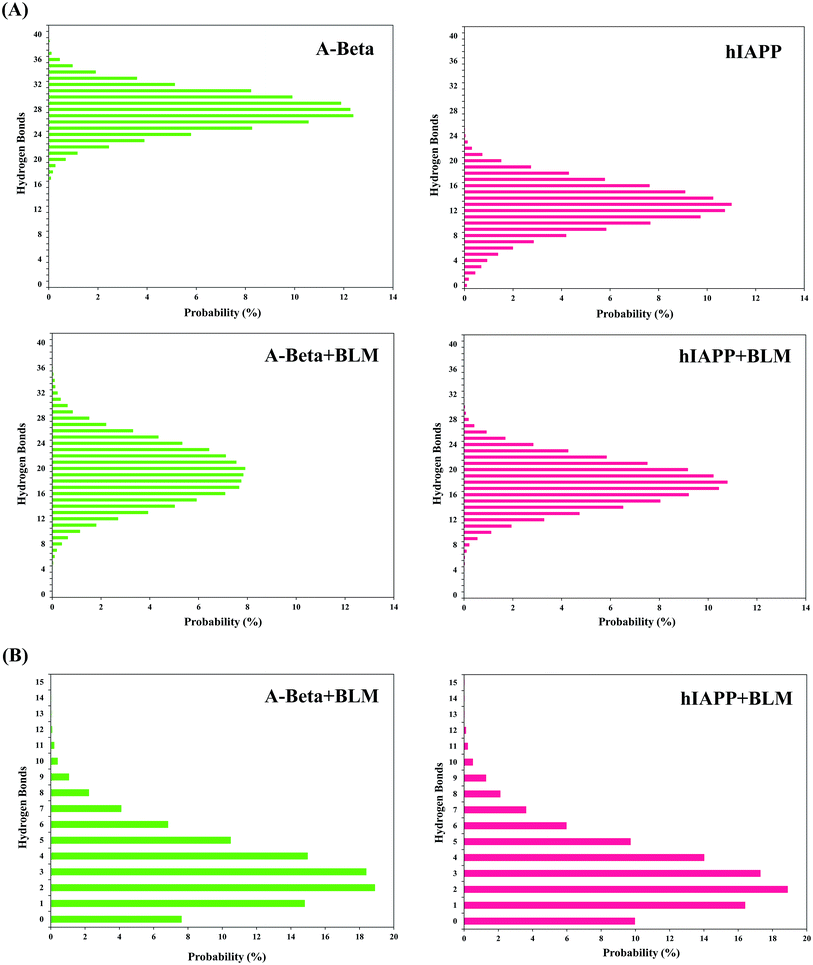 |
| Fig. 4 (A) Probability percentage (%) of intramolecular hydrogen bonds (C O to N–H) formation for Aβ and hIAPP in presence and absence of BLM. (B) Probability percentage (%) of intermolecular hydrogen bonds with BLM in Aβ and hIAPP. | |
Salt-bridge population density analysis
The presence of salt-bridges in Aβ was investigated for far-off residues (ASP23-LYS16) and adjacent residues (ASP23-LYS28), both in the presence and absence of BLM, and the population density plotted. Salt-bridge formation did not occur in hIAPP due to the absence of negatively charged or acidic amino acids (e.g., aspartic and glutamic acid) as the acidic side chains of the proteins. In Fig. 3, it is quite clear that Aβ alone was found to be folded in a compact form, which confirms more salt-bridges were formed between far-off residues (ASP23-LYS16) than adjacent residues (ASP23-LYS28). However, incorporating BLM into the Aβ + BLM system had the opposite effect on salt-bridge formation, as salt-bridge formation involved more adjacent residues (ASP23-LYS28) and fewer far-off residues (ASP23-LYS16). The Aβ and Aβ + BLM systems were relatable, as the Aβ + BLM system's salt-bridges were fewer in number than the Aβ system's, denoted by the blue region of the population density graph in Fig. 5. Therefore, depending on the relatable results for hydrogen bonds and salt-bridges, we detected that a superficial decrease in hydrogen bonds was managed by an increase in salt-bridges.
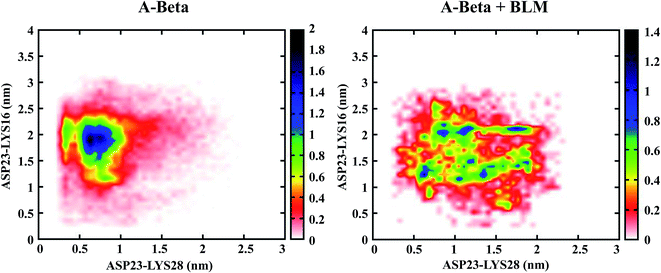 |
| Fig. 5 Population density analysis of monomeric salt bridges within ASP (D) and LYS (K) of Aβ in presence and absence of BLM. | |
Secondary structural characterization of Aβ and hIAPP in the presence and absence of BLM
Fig. 6A represents the secondary structures of Aβ and hIAPP, both in the presence and absence of BLM systems. For Aβ and hIAPP systems, the probabilities of coil structures are 31.5% and 38.5%, and for β-sheet structures, they are 19% and 29%, respectively. However, other secondary structures (i.e., β-bridge, bend, turn, α-helix, 5-helix, and 3-helix conformations) occurred less frequently for both Aβ and hIAPP in the absence of BLM. After adding BLM to Aβ and hIAPP systems, the coil structure probability increases from 31.5% to 44.5% (from 31.5% without BLM to 44.5% with BLM) for Aβ and remains similar, at about 38.3%, for the hIAPP + BLM system (38.5% without BLM in hIAPP system). The opposite happens for β-sheet probabilities, which decrease from 19% to 14% in the Aβ + BLM system and from 29% to 21% in the hIAPP + BLM system. The probability percentages of other secondary structures do not change much. These results illustrate that the BLM molecule decreases significantly the β-sheet structures that are considered the characteristic feature of amyloids, and thus, BLM helps reduce amyloid formation.
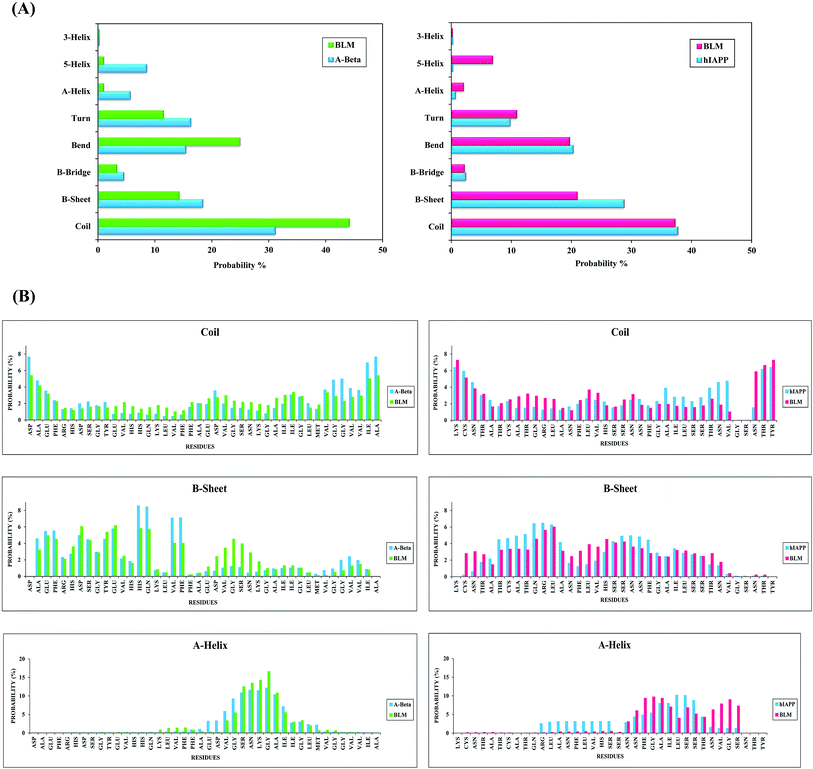 |
| Fig. 6 (A) Probability% of secondary structures formation for Aβ and hIAPP in presence and absence of BLM. (B) Detailed residue specific probability% of secondary structures [coil (top), β-sheet (middle), and α-helix (bottom)] for Aβ and hIAPP in presence and absence of BLM. | |
To obtain residue-based secondary structure details, we determined the probability percentages for secondary structures as a function of Aβ and hIAPP amino acid residues, which are shown in Fig. 6B. In the Aβ system, the N-terminal's hydrophobic residues, along with a few C-terminal residues, have a higher β-sheet propensity. Correspondingly, in the hIAPP system, residues 5–13 and 19–26 have a higher β-sheet propensity. After adding BLM, β-sheet propensity is reduced for the N-terminal's hydrophobic residues, along with some C-terminal residues, in the Aβ + BLM system, and similarly, in the hIAPP + BLM system, it is minimized for residues 5–13 and 19–26. However, coil propensity is higher for the Aβ + BLM system's residues than Aβ alone, while it is relatively similar for hIAPP with and without BLM. Furthermore, α-helix propensity was found more frequently for the C-terminal residues of the Aβ without BLM system, while it was lower in the C-terminal residues of the hIAPP without BLM system (Fig. 6B). Residue-specific probability percentages for other secondary structures, including β-bridge, bend, turn, 3-helix, and 5-helix, are reported in the ESI (Fig. S1).†
Clustering of Aβ and hIAPP with and without BLM, where the three/two-stranded anti-parallel cross β-sheet is transiently populated but diminished by BLM binding
An abundant conformational ensemble was gathered for each REMD-simulated temperature. To examine the three-dimensional (3D) structures of Aβ and hIAPP with and without BLM, we performed cluster analysis for the conformations developed in the presence and absence of BLM for all systems. Cluster analysis aids in determining the more populated structures and divides the low-temperature conformations generated by OPLS-employed REMD simulations into new clusters with related geometric attributes. Therefore, we captured the pertinent structural properties of all four systems by presenting definite structures that are representatives of their appropriate clusters. The conformations in all four systems (i.e., Aβ, Aβ + BLM, hIAPP, and hIAPP + BLM) were separated into various clusters using cluster parameters. The central axis of the top three most dominant clusters, including their ensembles, is depicted in Fig. 7. The Aβ and hIAPP systems show the most dominant conformations and have cluster probability percentages of 67.27% and 52.80%, respectively, showing compact folded conformation with β-sheet formation in the consecutive clusters that are dissimilar to the initial conformation. In the absence of the BLM molecule, in the first cluster, Aβ consists of a three-stranded cross β-sheet conformation (anti-parallel) and hIAPP consists of a two-stranded cross β-sheet conformation (anti-parallel), which, in the second cluster, forms a two-stranded cross four-β-sheet conformation (two β-sheets on each strand). However, adding BLM to the Aβ and hIAPP systems shows the most dominant conformations have a cluster probability percentage of 48.73% and 62.59%, respectively, showing less β-sheet-formation despite α-helix and coil formations. Hence, in the presence of BLM, Aβ and hIAPP mainly created coil rich conformations with the loss of β-sheet formation, which verifies that the BLM molecule prevents three-stranded anti-parallel β-sheets in the case of Aβ and two stranded anti-parallel four-β-sheets in case of hIAPP.
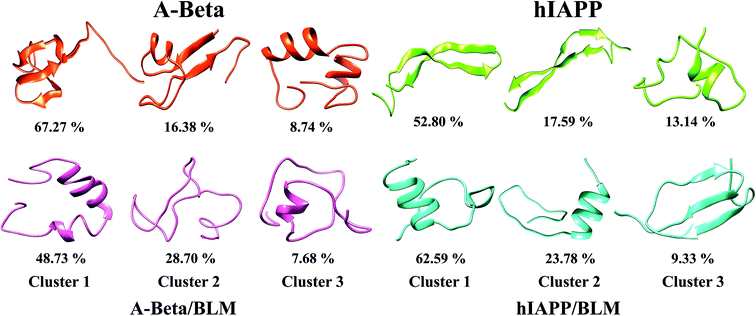 |
| Fig. 7 Ideal conformations of primary three highest populated clusters of Aβ and hIAPP raised in presence and absence of BLM. The corresponding time consumed in every conformation over production run of all system is determined by percentages. | |
BLM inhibits the aggregation and fibrillization of both Aβ and hIAPP: thioflavin fluorescence-based aggregation assays (ThT)
The efficacy of BLM in countering the aggregation of Aβ and hIAPP was tested by performing in vitro thioflavin-T (ThT) fluorescence assay. The level of ThT fluorescence gives a measure of the fibrillar load resulting from the aggregation of amyloidogenic proteins/peptides. The results of the ThT kinetics are shown in Fig. 8A and 9A; in the presence of BLM, both Aβ and hIAPP exhibit lower levels of ThT fluorescence.
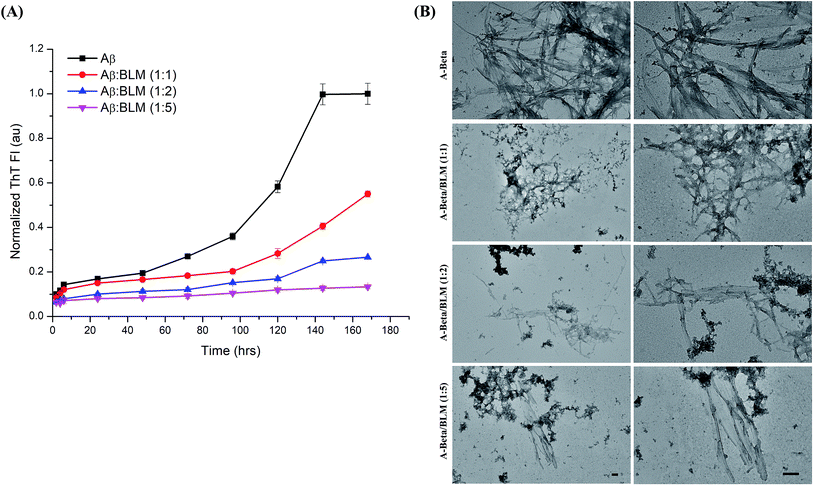 |
| Fig. 8 (A) ThioflavinT (ThT) fluorescence monitored showing kinetics mechanism of fibril formation for Aβ in presence and absence of BLM. (B) Transmission electron microscopy showing morphology of Aβ amyloid fibrils stained in uranyl acetate at 25 000 fold magnification and at 20 and 100 nm scale. | |
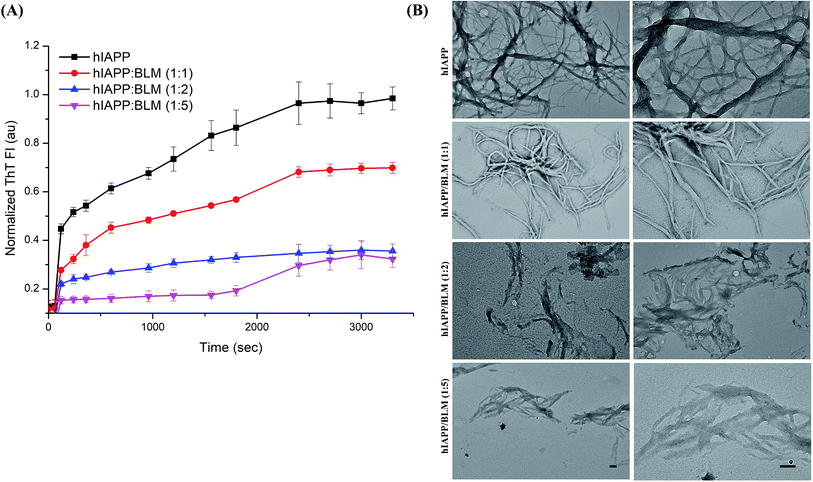 |
| Fig. 9 (A) ThioflavinT (ThT) fluorescence monitored showing kinetics mechanism of fibril formation for hIAPP in presence and absence of BLM. (B) Transmission electron microscopy showing morphology of hIAPP amyloid fibrils stained in uranyl acetate at 25 000 fold magnification and at 20 and 100 nm scale. | |
BLM increased the lag phase of Aβ amyloid aggregation, and this increase was much more pronounced at higher concentrations of the compound. At the maximum tested concentration of BLM (i.e., Aβ
:
BLM 1
:
5), the level of ThT fluorescence increased very little over time, suggesting minimal Aβ fibrillation.
In the case of hIAPP, lower test concentrations of BLM (i.e., hIAPP
:
BLM – 1
:
1 and 1
:
3) were not able to delay the exponential phase of amyloid aggregation, although lower levels of ThT fluorescence were detected when compared to hIAPP alone. At the maximum-tested concentration of BLM, a clear increase in the duration of lag was observed, but fluorescence levels rose significantly by the end of the kinetic run. Thus, BLM lowered the fibrillation of hIAPP but was less efficient in countering its aggregation when compared to Aβ (Fig. S2†).
The T-test statistical study revealed a high significance level for the compared populations. Results are presented for the ThT values obtained at the end of the kinetic run, for both Aβ and hIAPP. Entire calibrations were conceded in triplicates, with error bars demonstrating the standard deviation (Fig. S3†). The significance in all the cases is substantiated via p-value symbolizations (*p < 0.05, **p < 0.01, and ***p < 0.001).
BLM modified the secondary structures and morphologies of Aβ and hIAPP aggregates: transmission electron microscopy (TEM)
BLM's inhibitory effect on the aggregation of both Aβ and hIAPP was also visualized using TEM. The morphological characterization of the end-stage aggregates formed by these peptides in the presence and absence of BLM reveals that BLM was able to inhibit the fibrillation of Aβ and hIAPP in a concentration-dependent manner (Fig. 8B and 9B).
Both Aβ and hIAPP, when present alone, formed numerous larger-sized amyloid fibrils, in comparison to the aggregates formed by these peptides in the presence of BLM at all tested concentrations. In the presence of increasing molar concentrations of BLM, the size of Aβ and hIAPP amyloid fibrils diminished, and the abundance of amorphous off-pathway aggregates increased.
Discussion
The biochemical commonalities between AD and T2D would entail that trivial therapeutic approaches could be pursued. In this study, by performing computational and experimental techniques for AD and T2D, we determined the dual inhibitory actions against the amyloid aggregation of Aβ and hIAPP. FDA-approved drugs are considered the most vital resources for universal drug discovery and improvement because of the evidence that they are greater in number and have unique chemical diversity, and once selected for drug reprofiling, preclinical trials do not need to be repeated. In this study, we performed virtual screening of FDA-approved drugs to find potent inhibitors of amyloid aggregation in Aβ and hIAPP. Through docking analysis and refinement, we demonstrated that most of the ligands showed strong interactions. Among all the ligands, the most potent and common inhibitor of Aβ and hIAPP aggregation, bleomycin (BLM), was selected using various energy and docking score-based parameters.
BLM is a complex of associated glycopeptide antibiotics from Streptomyces verticillus, including bleomycin A2 and B2. It prohibits DNA metabolism and is utilized as an antineoplastic, primarily for solid tumors.122,123 It is a well-known antitumor and antibiotic in the treatment of squamous cell cancers, melanoma, sarcoma, testicular and ovarian cancer, and Hodgkin's and non-Hodgkin's lymphoma.124 Berg et al. reported that site-specific drug delivery administered by photochemical internalization intensifies the antitumor effect of BLM and leads to a therapeutic release that is entrapped in endocytic vesicles towards cytosol.125 BLMs are generally administrated therapeutically as a composite of various analogs denoted blenoxane, which consists primarily of bleomycin A2 and bleomycin B2.126,127 BLM's potency as an antitumor agent has also been established by, for example, the finding that omitting bleomycin from a multidrug regimen used to treat germ cell carcinomas resulted in decreased potency.128 It has also been found to be effective in the treatment of malignant pleural effusion. Nikbakhsh et al. assessed the efficacy of BLM in malignant pleural effusion treatment for 50 cases and showed that pleurodesis with BLM improved disease symptoms in about 88% of patients without causing serious complications.129 Furthermore, a combination of BLM and tranexamic acid was found to be an effective treatment for pleurodesis in patients suffering from malignant pleural effusion.130
After identifying a potent inhibitor, the characterization for the structures of short peptides (Aβ and hIAPP) and the interactions with BLM molecules are essential for the advancement of drugs that aim the preliminary fibrillation stage of Aβ and hIAPP aggregation. In this study, by implementing a 100 ns per replica all-atom explicit REMD simulation for atomistic conformational sampling, was performed to illustrate strong interactions between Aβ and BLM, as well as between hIAPP and BLM, and we investigated conformational ensemble modulations of both peptides (Aβ and hIAPP) and the key Aβ–BLM and hIAPP–BLM interactions. We implemented the REMD method to cross the potential energy barriers among unfolded conformations and increase convergence towards the native structure. To the preeminent of our information, this is the major all-atom explicit solvent REMD simulation research work on the full length Aβ and hIAPP peptides and the effect of BLM molecule. Beginning from unfolded coil forms, our REMD simulations illustrates that Aβ and hIAPP attains both compact and extended conformations. This atomistic simulation demonstrates that secondary structure modulations, primarily β-sheet formation and depletion mechanism of both Aβ and hIAPP, depend on the potent inhibitor, BLM, which leads to β-sheet destabilization, followed by α-helix destabilization. The presence of the β-hairpin comprising β-sheet in our REMD run claims the earlier proposition that the β-hairpin is the amyloidogenic precursor of Aβ and hIAPP.131,132 We observed an enhancement in Ree values when BLM was present in both Aβ and hIAPP systems, making the peptides form in a slightly unfolded and extended manner. We also observed a reduction in intramolecular hydrogen bonds and increased intermolecular hydrogen bonds with BLM in Aβ + BLM and hIAPP + BLM systems. In addition, secondary structure profiles reveal that BLM helped decrease β-sheet propensity, followed by the increased propensity of coil structures. For further validation purposes, we performed cluster analysis, in which the most dominant clusters showed an absence of β-sheet formation in BLM-treated Aβ and hIAPP systems.
An in vitro assessment of BLM's effect on the aggregation of Aβ and hIAPP was examined via ThT fluorescence assays and TEM analysis. BLM was able to counter the aggregation of both the amyloidogenic peptides. In the case of Aβ, BLM increased the lag phase of aggregation, which might be a consequence of its stabilization of the native unfolded conformation of this peptide and further directs it towards off-pathway amorphous aggregates, which were also observed in our TEM analysis. BLM also impacted hIAPP fibrillation; although the delay in the exponential phase of aggregation was not observed, lower levels of hIAPP ThT fluorescence were detected in the presence of BLM throughout the time of aggregation, in comparison to the control reaction of hIAPP alone. On comparing the efficiency of BLM induced inhibition of fibrillization for both the peptides, it was observed that BLM is more effective in the case of Aβ. Analysis of the normalized ThT fluorescence values acquired at the end of the kinetic run for each of the peptides identified that, when Aβ and hIAPP are treated with equimolar concentrations of BLM, the percentage of inhibition in fibrillization detected is 45% and 30% respectively (Fig. S2†). Similarly, when the BLM concentration used is 5 times the molar concentration of the Aβ and hIAPP peptides, the percentage of inhibition observed was 87% and 69% respectively (Fig. S2†). The TEM analysis of hIAPP aggregates formed in the presence of BLM exhibited clear morphological changes in shape and size when compared to hIAPP alone, indicating BLM hinders the growth of hIAPP fibrils.
Inhibiting the formation of amyloid aggregates by amyloidogenic peptides/proteins is the most widely used approach sought by researchers for developing therapies against amyloid diseases. The Aβ–BLM and hIAPP–BLM interface scrutiny reveals that hydrophobic and hydrogen bond interactions synergistically play vital roles on the BLM-induced conformational shift of Aβ and hIAPP. The present study shows an all-atom interpretation of the conformational ensemble of Aβ and hIAPP and delivers the mechanistic acumens into the inhibitory process of BLM against Aβ and hIAPP aggregation. In this milieu, drug repurposing is an encouraging setting where a familiar remedy with novel anti-aggregating activity against amyloidogenic proteins could be used to treat such debilitating diseases. In this study, we identified BLM, a known antibiotic and anti-cancer drug, and determined its ability to inhibit the fibrillation of both Aβ and hIAPP peptides.
Author contributions
AK, RS, NS, PS and AG devised the mode of experimentation and its setup. AK, RS and NS performed the research work and addressed the manuscript.
Data availability statement
The data that support the findings of this study are available from the corresponding author upon reasonable request.
Conflicts of interest
No conflict of interest was declared by the authors regarding the content of this research article.
Acknowledgements
PS is grateful to TERI School of Advanced Studies, India for research and management. AG is grateful to Jawaharlal Nehru University for management of Advanced Instrumentation Research Facility (AIRF) and other facilities. AG is thankful to University Grant Commission, India for the Faculty Recharge position. NS is grateful to Department of Health Research (DHR), India for women scientist grant. AK and RS are contended to Indian Council of Medical Research (ICMR), India for the senior research fellowship.
References
- C. M. Dobson, T. P. Knowles and M. Vendruscolo, The amyloid phenomenon and its significance in biology and medicine, Cold Spring Harbor Perspect. Biol., 2019, a033878 Search PubMed.
- M. C. Owen, et al., Effects of in vivo conditions on amyloid aggregation, Chem. Soc. Rev., 2019, 48(14), 3946–3996 RSC.
- S. Giorgetti, C. Greco, P. Tortora and F. Aprile, Targeting amyloid aggregation: an overview of strategies and mechanisms, Int. J. Mol. Sci., 2018, 19, 2677 CrossRef PubMed.
- R. Crespo, F. A. Rocha, A. M. Damas and P. M. Martins, A generic crystallization-like model that describes the kinetics of amyloid fibril formation, J. Biol. Chem., 2012, 287, 30585–30594 CrossRef CAS PubMed.
- R. Nelson and D. Eisenberg, Structural models of amyloid-like fibrils, Adv. Protein Chem., 2006, 73, 235–282 CrossRef CAS PubMed.
- M. Zaman, A. N. Khan, S. M. Zakariya and R. H. Khan, Protein misfolding, aggregation and mechanism of amyloid cytotoxicity: an overview and therapeutic strategies to inhibit aggregation, Int. J. Biol. Macromol., 2019, 134, 1022–1037 CrossRef CAS PubMed.
- C. M. Dobson, Protein aggregation and its consequences for human disease, Protein Pept. Lett., 2006, 13, 219–227 CrossRef CAS PubMed.
- M. E. Larson and S. E. Lesné, Soluble Aβ oligomer production and toxicity, J. Neurochem., 2012, 120, 125–139 CrossRef CAS PubMed.
- J. Nasica-Labouze, et al., Amyloid β protein and Alzheimer's disease: when computer simulations complement experimental studies, Chem. Rev., 2015, 115, 3518–3563 CrossRef CAS PubMed.
- A. Morriss-Andrews and J.-E. Shea, Simulations of protein aggregation: insights from atomistic and coarse-grained models, J. Phys. Chem. Lett., 2014, 5, 1899–1908 CrossRef CAS PubMed.
- S. Sharma, B. Nehru and A. Saini, Inhibition of Alzheimer's amyloid-beta aggregation in vitro by carbenoxolone: Insight into mechanism of action, Neurochem. Int., 2017, 108, 481–493 CrossRef CAS PubMed.
- S. Sharma, N. Sharma, A. Saini and B. Nehru, Carbenoxolone Reverses the Amyloid Beta 1–42 Oligomer-Induced Oxidative Damage and Anxiety-Related Behavior in Rats, Neurotoxic. Res., 2019, 35, 654–667 CrossRef CAS PubMed.
- Z. Liu, et al., Distinct Binding Dynamics, Sites and Interactions of Fullerene and Fullerenols with Amyloid-β Peptides Revealed by Molecular Dynamics Simulations, Int. J. Mol. Sci., 2019, 20, 2048 CrossRef CAS PubMed.
- M.-H. Melchor, F.-G. Susana, G.-S. Francisco, R.-F. Norma and B.-I. Gustavo, Fullerenemalonates inhibit amyloid beta aggregation, in vitro and in silico evaluation, RSC Adv., 2018, 8, 39667–39677 RSC.
- K. Andrich and J. Bieschke, in Natural Compounds as Therapeutic Agents for Amyloidogenic Diseases, Springer, 2015, pp. 139–161 Search PubMed.
- K. Rezai-Zadeh, et al., Green tea epigallocatechin-3-gallate (EGCG) reduces β-amyloid mediated cognitive impairment and modulates tau pathology in Alzheimer transgenic mice, Brain Res., 2008, 1214, 177–187 CrossRef CAS PubMed.
- J. Bieschke, et al., EGCG remodels mature α-synuclein and amyloid-β fibrils and reduces cellular toxicity, Proc. Natl. Acad. Sci. U. S. A., 2010, 107, 7710–7715 CrossRef CAS PubMed.
- A. Franko, et al., Epigallocatechin gallate (EGCG) reduces the intensity of pancreatic amyloid fibrils in human islet amyloid polypeptide (hIAPP) transgenic mice, Sci. Rep., 2018, 8, 1116 CrossRef PubMed.
- Y. Mo, J. Lei, Y. Sun, Q. Zhang and G. Wei, Conformational ensemble of hIAPP dimer: insight into the molecular mechanism by which a green tea extract inhibits hIAPP aggregation, Sci. Rep., 2016, 6, 33076 CrossRef CAS PubMed.
- J. Zhang, et al., Epigallocatechin-3-gallate (EGCG)-stabilized selenium nanoparticles coated with Tet-1 peptide to reduce amyloid-β aggregation and cytotoxicity, ACS Appl. Mater. Interfaces, 2014, 6, 8475–8487 CrossRef CAS PubMed.
- R. P. Weinberg, et al., Oil palm phenolics inhibit the in vitro aggregation of β-amyloid peptide into oligomeric complexes, Int. J. Alzheimer’s Dis., 2018, 2018, 1–12 CrossRef PubMed.
- P. H. Reddy, et al., Protective effects of Indian spice curcumin against amyloid-β in Alzheimer's disease, J. Alzheimer's Dis., 2018, 61, 843–866 Search PubMed.
- S. Nasir Abbas Bukhari and I. Jantan, Synthetic
curcumin analogs as inhibitors of β-amyloid peptide aggregation: potential therapeutic and diagnostic agents for Alzheimer's disease, Mini-Rev. Med. Chem., 2015, 15, 1110–1121 CrossRef PubMed.
- F. Yang, et al., Curcumin inhibits formation of amyloid β oligomers and fibrils, binds plaques, and reduces amyloid in vivo, J. Biol. Chem., 2005, 280, 5892–5901 CrossRef CAS PubMed.
- P. Maiti and G. Dunbar, Use of curcumin, a natural polyphenol for targeting molecular pathways in treating age-related neurodegenerative diseases, Int. J. Mol. Sci., 2018, 19, 1637 CrossRef PubMed.
- R. A. Orlando, A. M. Gonzales, R. E. Royer, L. M. Deck and D. L. Vander Jagt, A chemical analog of curcumin as an improved inhibitor of amyloid Abeta oligomerization, PLoS One, 2012, 7, e31869 CrossRef CAS PubMed.
- L. Zhang, et al., The potential protective effect of curcumin on amyloid-β-42 induced cytotoxicity in HT-22 cells, BioMed Res. Int., 2018, 2018, 1–8 Search PubMed.
- V. P. Brahmkhatri, et al., Curcumin nanoconjugate inhibits aggregation of N-terminal region (Aβ-16) of an amyloid beta peptide, New J. Chem., 2018, 42, 19881–19892 RSC.
- A. Thapa, S. D. Jett and E. Y. Chi, Curcumin attenuates amyloid-β aggregate toxicity and modulates amyloid-β aggregation pathway, ACS Chem. Neurosci., 2015, 7, 56–68 CrossRef PubMed.
- P. H. Reddy, et al., Protective effects of a natural product, curcumin, against amyloid β induced mitochondrial and synaptic toxicities in Alzheimer's disease, J. Invest. Med., 2016, 64, 1220–1234 CrossRef PubMed.
- N. Ferreira, N. P. Gonçalves, M. J. Saraiva and M. R. Almeida, Curcumin: a multi-target disease-modifying agent for late-stage transthyretin amyloidosis, Sci. Rep., 2016, 6, 26623 CrossRef CAS PubMed.
- A. A. Reinke and J. E. Gestwicki, Structure–activity Relationships of amyloid beta-aggregation inhibitors based on curcumin: influence of linker length and flexibility, Chem. Biol. Drug Des., 2007, 70, 206–215 CrossRef CAS PubMed.
- J. G. Cordero, R. García-Escudero, J. Avila, R. Gargini and V. García-Escudero, Benefit of oleuropein aglycone for Alzheimer’s disease by promoting autophagy, Oxid. Med. Cell. Longevity, 2018, 2018, 1–12 CrossRef PubMed.
- L. Wu, P. Velander, D. Liu and B. Xu, Olive component oleuropein promotes β-cell insulin secretion and protects β-cells from amylin amyloid-induced cytotoxicity, Biochemistry, 2017, 56, 5035–5039 CrossRef CAS PubMed.
- M. Martorell, et al., Potential therapeutic effects of oleuropein aglycone in Alzheimer's disease, Curr. Pharm. Biotechnol., 2016, 17, 994–1001 CAS.
- Q. Wang, et al., Tanshinones inhibit amyloid aggregation by amyloid-β peptide, disaggregate amyloid fibrils, and protect cultured cells, ACS Chem. Neurosci., 2013, 4, 1004–1015 CrossRef CAS PubMed.
- Y. Liu, L.-P. Xu, Q. Wang, B. Yang and X. Zhang, Synergistic Inhibitory Effect of GQDs–Tramiprosate Covalent Binding on Amyloid Aggregation, ACS Chem. Neurosci., 2017, 9, 817–823 CrossRef PubMed.
- Y. Liu, et al., Graphene quantum dots for the inhibition of β amyloid aggregation, Nanoscale, 2015, 7, 19060–19065 RSC.
- Y. Guan, et al., Ceria/POMs hybrid nanoparticles as a mimicking metallopeptidase for treatment of neurotoxicity of amyloid-β peptide, Biomaterials, 2016, 98, 92–102 CrossRef CAS PubMed.
- L. Yang, W. Wang, J. Chen, N. Wang and G. Zheng, A comparative study of resveratrol and resveratrol-functional selenium nanoparticles: inhibiting amyloid β aggregation and reactive oxygen species formation properties, J. Biomed. Mater. Res., Part A, 2018, 106, 3034–3041 CrossRef CAS PubMed.
- L. Yang, J. Sun, W. Xie, Y. Liu and J. Liu, Dual-functional selenium nanoparticles bind to and inhibit amyloid β fiber formation in Alzheimer's disease, J. Mater. Chem. B, 2017, 5, 5954–5967 RSC.
- H.-C. Lin, et al., From the Cover: Comparative Proteomics Reveals Silver Nanoparticles Alter Fatty Acid Metabolism and Amyloid Beta Clearance for Neuronal Apoptosis in a Triple Cell Coculture Model of the Blood–Brain Barrier, Toxicol. Sci., 2017, 158, 151–163 CrossRef CAS PubMed.
- M. Dehvari and A. Ghahghaei, The effect of green synthesis silver nanoparticles (AgNPs) from Pulicaria undulata on the amyloid formation in α-lactalbumin and the chaperon action of α-casein, Int. J. Biol. Macromol., 2018, 108, 1128–1139 CrossRef CAS PubMed.
- F. Ghasemi, M. R. Hormozi-Nezhad and M. Mahmoudi, Label-free detection of β-amyloid peptides (Aβ40 and Aβ42): a colorimetric sensor array for plasma monitoring of Alzheimer's disease, Nanoscale, 2018, 10, 6361–6368 RSC.
- M. Wang, A. Kakinen, E. H. Pilkington, T. P. Davis and P. C. Ke, Differential effects of silver and iron oxide nanoparticles on IAPP amyloid aggregation, Biomater. Sci., 2017, 5, 485–493 RSC.
- Y. Xing, et al., A sensitive and selective electrochemical biosensor for the determination of beta-amyloid oligomer by inhibiting the peptide-triggered in situ assembly of silver nanoparticles, Int. J. Nanomed., 2017, 12, 3171 CrossRef CAS PubMed.
- B. G. Anand, K. Dubey, D. S. Shekhawat and K. Kar, Capsaicin-coated silver nanoparticles inhibit amyloid fibril formation of serum albumin, Biochemistry, 2016, 55, 3345–3348 CrossRef CAS PubMed.
- V. Mangini, et al., Amyloid Transition of Ubiquitin on Silver Nanoparticles Produced by Pulsed Laser Ablation in Liquid as a Function of Stabilizer and Single-Point Mutations, Chem.–Eur. J., 2014, 20, 10745–10751 CrossRef CAS PubMed.
- H. Liu, X. Dong, F. Liu, J. Zheng and Y. Sun, Iminodiacetic acid-conjugated nanoparticles as a bifunctional modulator against Zn2+-mediated amyloid β-protein aggregation and cytotoxicity, J. Colloid Interface Sci., 2017, 505, 973–982 CrossRef CAS PubMed.
- K. L. Sciarretta, A. Boire, D. J. Gordon and S. C. Meredith, Spatial separation of β-sheet domains of β-amyloid: disruption of each β-sheet by N-methyl amino acids, Biochemistry, 2006, 45, 9485–9495 CrossRef CAS PubMed.
- E. A. Fradinger, et al., C-terminal peptides coassemble into Aβ42 oligomers and protect neurons against Aβ42-induced neurotoxicity, Proc. Natl. Acad. Sci. U. S. A., 2008, 105, 14175–14180 CrossRef CAS PubMed.
- C. Soto, M. S. Kindy, M. Baumann and B. Frangione, Inhibition of Alzheimer's amyloidosis by peptides that prevent β-sheet conformation, Biochem. Biophys. Res. Commun., 1996, 226, 672–680 CrossRef CAS PubMed.
- L. O. Tjernberg, et al., Arrest of β-amyloid fibril formation by a pentapeptide ligand, J. Biol. Chem., 1996, 271, 8545–8548 CrossRef CAS PubMed.
- T. Mohamed, S. S. Gujral and P. P. Rao, Tau derived hexapeptide AcPHF6 promotes beta-amyloid (Aβ) fibrillogenesis, ACS Chem. Neurosci., 2017, 9, 773–782 CrossRef PubMed.
- X. Zhou, et al., PEG modified graphene oxide loaded with EALYLV peptides for inhibiting the aggregation of hIAPP associated with type-2 diabetes, J. Mater. Chem. B, 2015, 3, 7055–7067 RSC.
- M. Tatarek-Nossol, et al., Inhibition of hIAPP amyloid-fibril formation and apoptotic cell death by a designed hIAPP amyloid-core-containing hexapeptide, Chem. Biol., 2005, 12, 797–809 CrossRef CAS PubMed.
- H. Skaat, R. Chen, I. Grinberg and S. Margel, Engineered polymer nanoparticles containing hydrophobic dipeptide for inhibition of amyloid-β fibrillation, Biomacromolecules, 2012, 13, 2662–2670 CrossRef CAS PubMed.
- E. N. Gurzov, et al., Inhibition of hIAPP Amyloid Aggregation and Pancreatic β-Cell Toxicity by OH-Terminated PAMAM Dendrimer, Small, 2016, 12, 1615–1626 CrossRef CAS PubMed.
- Y. Ma, et al., Swelling behaviors of porous lignin based poly (acrylic acid), Chemosphere, 2016, 163, 610–619 CrossRef CAS PubMed.
- K. Gudala, D. Bansal, F. Schifano and A. Bhansali, Diabetes mellitus and risk of dementia: a meta-analysis of prospective observational studies, J. Diabetes Invest., 2013, 4, 640–650 CrossRef PubMed.
- G. J. Biessels, S. Staekenborg, E. Brunner, C. Brayne and P. Scheltens, Risk of dementia in diabetes mellitus: a systematic review, Lancet Neurol., 2006, 5, 64–74 CrossRef.
- G. J. Biessels and F. Despa, Cognitive decline and dementia in diabetes mellitus: mechanisms and clinical implications, Nat. Rev. Endocrinol., 2018, 14, 591–604 CrossRef PubMed.
- A. Ott, et al., Diabetes mellitus and the risk of dementia: the Rotterdam study, Neurology, 1999, 53, 1937 CrossRef CAS PubMed.
- R. Peila, B. L. Rodriguez and L. J. Launer, Type 2 diabetes, APOE gene, and the risk for dementia and related pathologies: the Honolulu-Asia aging study, Diabetes, 2002, 51, 1256–1262 CrossRef CAS PubMed.
- J. A. Luchsinger, et al., Relation of diabetes to mild cognitive impairment, Arch. Neurol., 2007, 64, 570–575 CrossRef PubMed.
- G. Bucht, R. Adolfsson, F. Lithner and B. Winblad, Changes in blood glucose and insulin secretion in patients with senile dementia of Alzheimer type, Acta Med. Scand., 1983, 213, 387–392 CrossRef CAS PubMed.
- S. E. Arnold, et al., Brain insulin resistance in type 2 diabetes and Alzheimer disease: concepts and conundrums, Nat. Rev. Neurol., 2018, 14, 168 CrossRef CAS PubMed.
- N. Wijesekara, R. A. Gonçalves, F. G. De Felice and P. E. Fraser, Impaired peripheral glucose homeostasis and Alzheimer's disease, Neuropharmacology, 2018, 136, 172–181 CrossRef CAS PubMed.
- A. E. Roher, et al., Amyloid beta peptides in human plasma and tissues and their significance for Alzheimer's disease, Alzheimer's Dementia, 2009, 5, 18–29 CrossRef CAS PubMed.
- J. Miklossy, et al., Beta amyloid and hyperphosphorylated tau deposits in the pancreas in type 2 diabetes, Neurobiol. Aging, 2010, 31, 1503–1515 CrossRef CAS PubMed.
- C. L. Joachim, H. Mori and D. J. Selkoe, Amyloid β-protein deposition in tissues other than brain in Alzheimer's disease, Nature, 1989, 341, 226–230 CrossRef CAS PubMed.
- M. Maj, et al., The microtubule-associated protein tau and its relevance for pancreatic beta cells, J. Diabetes Res., 2016, 2016, 1–12 CrossRef PubMed.
- A. Paul, et al., Antagonistic activity of naphthoquinone-based hybrids towards amyloids associated with Alzheimer’s disease and type-2 diabetes, ACS Chem. Neurosci., 2019, 10(8), 3510–3520 CrossRef CAS PubMed.
- B. Ren, et al., Genistein: A Dual Inhibitor of Both Amyloid β and Human Islet Amylin Peptides, ACS Chem. Neurosci., 2018, 9, 1215–1224 CrossRef CAS PubMed.
- S. A. Hudson, H. Ecroyd, F. C. Dehle, I. F. Musgrave and J. A. Carver, (−)-Epigallocatechin-3-gallate (EGCG) maintains κ-casein in its pre-fibrillar state without redirecting its aggregation pathway, J. Mol. Biol., 2009, 392, 689–700 CrossRef CAS PubMed.
- D. E. Ehrnhoefer, et al., Green tea (−)-epigallocatechin-gallate modulates early events in huntingtin misfolding and reduces toxicity in Huntington's disease models, Hum. Mol. Genet., 2006, 15, 2743–2751 CrossRef CAS PubMed.
- N. E. de Almeida, et al., 1,2,3,4,6-Penta-O-galloyl-β-D-glucopyranose binds to the N-terminal metal binding region to inhibit amyloid β-protein oligomer and fibril formation, Int. J. Mass Spectrom., 2017, 420, 24–34 CrossRef CAS PubMed.
- E. Bruno, et al., IAPP aggregation and cellular toxicity are inhibited by 1,2,3,4,6-penta-O-galloyl-β-D-glucose, Amyloid, 2013, 20, 34–38 CrossRef CAS PubMed.
- M. Mariano, et al., First selective dual inhibitors of tau phosphorylation and beta-amyloid aggregation, two major pathogenic mechanisms in Alzheimer's disease, ACS Chem. Neurosci., 2014, 5, 1198–1202 CrossRef CAS PubMed.
- M. Goyal, et al., Development of dual inhibitors against Alzheimer’s disease using fragment-based QSAR and molecular docking, BioMed Res. Int., 2014, 2014, 1–12 CrossRef PubMed.
- R. S. Mancini, Y. Wang and D. F. Weaver, Phenylindanes in brewed coffee inhibit amyloid-beta and tau aggregation, Front. Neurosci., 2018, 12, 735 CrossRef PubMed.
- K. Ono and M. Yamada, Antioxidant compounds have potent anti-fibrillogenic and fibril-destabilizing effects for α-synuclein fibrils in vitro, J. Neurochem., 2006, 97, 105–115 CrossRef CAS PubMed.
- B. Ren, et al., Tanshinones inhibit hIAPP aggregation, disaggregate preformed hIAPP fibrils, and protect cultured cells, J. Mater. Chem. B, 2018, 6, 56–67 RSC.
- T. T. Ashburn and K. B. Thor, Drug repositioning: identifying and developing new uses for existing drugs, Nat. Rev. Drug Discovery, 2004, 3, 673 CrossRef CAS PubMed.
- A. Breckenridge and R. Jacob, Overcoming the legal and regulatory barriers to drug repurposing, Nat. Rev. Drug Discovery, 2019, 18, 1–2 CrossRef CAS PubMed.
- N. Bibi, S. R. Danish, A. Batool and M. Kamal, Inhibitory mechanism of an anticancer drug, bexarotene against amyloid β peptide aggregation: repurposing via neuroinformatics approach, Curr. Pharm. Des., 2019, 25(27), 2989–2995 CrossRef CAS PubMed.
- F. González-Lizárraga, et al., Repurposing doxycycline for synucleinopathies: remodelling of α-synuclein oligomers towards non-toxic parallel beta-sheet structured species, Sci. Rep., 2017, 7, 41755 CrossRef PubMed.
- F. V. Santa-Cecília, et al., Doxycycline suppresses microglial activation by inhibiting the p38 MAPK and NF-kB signaling pathways, Neurotoxic. Res., 2016, 29, 447–459 CrossRef PubMed.
- G. Forloni, et al., Preventive study in subjects at risk of fatal familial insomnia: innovative approach to rare diseases, Prion, 2015, 9, 75–79 CrossRef CAS PubMed.
- M. Lazzarini, et al., Doxycycline restrains glia and confers neuroprotection in a 6-OHDA Parkinson model, Glia, 2013, 61, 1084–1100 CrossRef PubMed.
- G. Forloni, M. Salmona, G. Marcon and F. Tagliavini, Tetracyclines and prion infectivity, Infect. Disord.: Drug Targets, 2009, 9, 23–30 CAS.
- A. Minagar, et al., Combination therapy with interferon beta-1a and doxycycline in multiple sclerosis: an open-label trial, Arch. Neurol., 2008, 65, 199–204 CrossRef PubMed.
- R. Kayed, et al., Common structure of soluble amyloid oligomers implies common mechanism of pathogenesis, Science, 2003, 300, 486–489 CrossRef CAS PubMed.
- G. Forloni, L. Colombo, L. Girola, F. Tagliavini and M. Salmona, Anti-amyloidogenic activity of tetracyclines: studies in vitro, FEBS Lett., 2001, 487, 404–407 CrossRef CAS PubMed.
- M. Bortolanza, et al., Tetracycline repurposing in neurodegeneration: focus on Parkinson's disease, J. Neural Transm., 2018, 125, 1403–1415 CrossRef CAS PubMed.
- C. D. Hayes, et al., Striking reduction of amyloid plaque burden in an Alzheimer's mouse model after chronic administration of carmustine, BMC Med., 2013, 11, 81 CrossRef CAS PubMed.
- Schrödinger LLC, Glide, v. 5.5, Schrödinger LLC, New York, 2009 Search PubMed.
- Glide, V. 5.5, Schrödinger. LLC, New York, NY, 2009 Search PubMed.
- S. K. Burley, et al., RCSB protein data bank: sustaining a living digital data resource that enables breakthroughs in scientific research and biomedical education, Protein Sci., 2018, 27, 316–330 CrossRef CAS PubMed.
- K. H. DuBay, et al., Accurate force field development for modeling conjugated polymers, J. Chem. Theory Comput., 2012, 8, 4556–4569 CrossRef CAS PubMed.
- M. Morrone Xavier, et al., SAnDReS a computational tool for statistical analysis of docking results and development of scoring functions, Comb. Chem. High Throughput Screening, 2016, 19, 801–812 Search PubMed.
- LigPrep, S. R. version 2.3, Schrödinger, LLC, New York, NY, 2009 Search PubMed.
- R. Palakurti, D. Sriram, P. Yogeeswari and R. Vadrevu, Multiple e-pharmacophore modeling combined with high-throughput virtual screening and docking to identify potential inhibitors of β-secretase (BACE1), Mol. Inf., 2013, 32, 385–398 CrossRef CAS PubMed.
- R. A. Laskowski and M. B. Swindells, LigPlot+: multiple ligand–protein interaction diagrams for drug discovery, J. Chem. Inf. Model., 2011, 2778–2786 CrossRef CAS PubMed.
- E. F. Pettersen, et al., UCSF Chimera—a visualization system for exploratory research and analysis, J. Comput. Chem., 2004, 25, 1605–1612 CrossRef CAS PubMed.
- S. Kim, et al., PubChem substance and compound databases, Nucleic Acids Res., 2015, 44, D1202–D1213 CrossRef PubMed.
- A. W. Schüttelkopf and D. M. Van Aalten, PRODRG: a tool for high-throughput crystallography of protein–ligand complexes, Acta Crystallogr., Sect. D: Biol. Crystallogr., 2004, 60, 1355–1363 CrossRef PubMed.
- M. J. Abraham, et al., GROMACS: high performance molecular simulations through multi-level parallelism from laptops to supercomputers, SoftwareX, 2015, 1, 19–25 CrossRef.
- U. Essmann, et al., A smooth particle mesh Ewald method, J. Chem. Phys., 1995, 103, 8577–8593 CrossRef CAS.
- W. L. Jorgensen, J. Chandrasekhar, J. D. Madura, R. W. Impey and M. L. Klein, Comparison of simple potential functions for simulating liquid water, J. Chem. Phys., 1983, 79, 926–935 CrossRef CAS.
- H. J. Berendsen, J. v. Postma, W. F. van Gunsteren, A. DiNola and J. Haak, Molecular dynamics with coupling to an external bath, J. Chem. Phys., 1984, 81, 3684–3690 CrossRef CAS.
- W. G. Hoover, Canonical dynamics: equilibrium phase-space distributions, Phys. Rev. A, 1985, 31, 1695 CrossRef PubMed.
- B. Hess, H. Bekker, H. J. Berendsen and J. G. Fraaije, LINCS: a linear constraint solver for molecular simulations, J. Comput. Chem., 1997, 18, 1463–1472 CrossRef CAS.
- S. Miyamoto and P. A. Kollman, Settle: an analytical version of the SHAKE and RATTLE algorithm for rigid water models, J. Comput. Chem., 1992, 13, 952–962 CrossRef CAS.
- R. Denschlag, M. Lingenheil and P. Tavan, Optimal temperature ladders in replica exchange simulations, Chem. Phys. Lett., 2009, 473, 193–195 CrossRef CAS.
- R. Qi, G. Wei, B. Ma and R. Nussinov, in Peptide Self-Assembly, Springer, 2018, pp. 101–119 Search PubMed.
- A. Patriksson and D. van der Spoel, A temperature predictor for parallel tempering simulations, Phys. Chem. Chem. Phys., 2008, 10, 2073–2077 RSC.
- X. Daura, et al., The β-peptide hairpin in solution: conformational study of a β-hexapeptide in methanol by NMR spectroscopy and MD simulation, J. Am. Chem. Soc., 2001, 123, 2393–2404 CrossRef CAS PubMed.
- A. Kumari, R. Rajput, N. Shrivastava, P. Somvanshi and A. Grover, Synergistic approaches unraveling regulation and aggregation of intrinsically disordered β-amyloids implicated in Alzheimer's disease, Int. J. Biochem. Cell Biol., 2018, 99, 19–27 CrossRef CAS PubMed.
- P. Cao, F. Meng, A. Abedini and D. P. Raleigh, The ability of rodent islet amyloid polypeptide to inhibit amyloid formation by human islet amyloid polypeptide has important implications for the mechanism of amyloid formation and the design of inhibitors, Biochemistry, 2010, 49, 872–881 CrossRef CAS PubMed.
- M. Szaruga, et al., Alzheimer’s-causing mutations shift Aβ length by destabilizing γ-secretase-Aβn interactions, Cell, 2017, 170, 443–456 CrossRef CAS PubMed , e414.
- J. S. Lazo, C. J. Boland and P. E. Schwartz, Bleomycin hydrolase activity and cytotoxicity in human tumors, Cancer Res., 1982, 42, 4026–4031 CAS.
- I. M. Lefterov, R. P. Koldamova, J. King and J. S. Lazo, The C-terminus of human bleomycin hydrolase is required for protection against bleomycin-induced chromosomal damage, Mutat. Res., Fundam. Mol. Mech. Mutagen., 1998, 421, 1–7 CrossRef CAS PubMed.
- S. M. Hecht, Bleomycin: new perspectives on the mechanism of action, J. Nat. Prod., 2000, 63, 158–168 CrossRef CAS PubMed.
- K. Berg, A. Dietze, O. Kaalhus and A. Høgset, Site-specific drug delivery by photochemical internalization enhances the antitumor effect of bleomycin, Clin. Cancer Res., 2005, 11, 8476–8485 CrossRef CAS PubMed.
- W. O. Foye, Cancer chemotherapeutic agents, Amer Chemical Society, 1995 Search PubMed.
- S. Hecht, Cancer chemotherapeutic agents, Am Chem Soc, Washington DC, 1995 Search PubMed.
- P. J. Loehrer Sr, D. Johnson, P. Elson, L. H. Einhorn and D. Trump, Importance of bleomycin in favorable-prognosis disseminated germ cell tumors: an Eastern Cooperative Oncology Group trial, J. Clin. Oncol., 1995, 13, 470–476 CrossRef CAS PubMed.
- N. Nikbakhsh, A. P. Amiri and D. Hoseinzadeh, Bleomycin in the treatment of 50 cases with malignant pleural effusion, Caspian J. Intern. Med., 2011, 2, 274 CAS.
- E. Ali and H. Mahmoud, Pleurodesis for malignant pleural effusions: A comparison of bleomycin or tranexamic acid alone versus a combination of both, Eur. Respir. J., 2013, 42, P3072 Search PubMed.
- W. Hoyer, C. Grönwall, A. Jonsson, S. Ståhl and T. Härd, Stabilization of a β-hairpin in monomeric Alzheimer's amyloid-β peptide inhibits amyloid formation, Proc. Natl. Acad. Sci. U. S. A., 2008, 105, 5099–5104 CrossRef CAS PubMed.
- S. Luca, W.-M. Yau, R. Leapman and R. Tycko, Peptide conformation and supramolecular organization in amylin fibrils: constraints from solid-state NMR, Biochemistry, 2007, 46, 13505–13522 CrossRef CAS PubMed.
Footnote |
† Electronic supplementary information (ESI) available. See DOI: 10.1039/d0ra04949b |
|
This journal is © The Royal Society of Chemistry 2020 |
Click here to see how this site uses Cookies. View our privacy policy here.