DOI:
10.1039/C9RA07799E
(Paper)
RSC Adv., 2020,
10, 2027-2036
Integrated microbiome–metabolome analysis reveals novel associations between fecal microbiota and hyperglycemia-related changes of plasma metabolome in gestational diabetes mellitus†
Received
25th September 2019
, Accepted 21st December 2019
First published on 9th January 2020
Abstract
Gestational diabetes mellitus (GDM) has been associated with circulating metabolic disorders and alterations in gut microbiota, respectively. Although changes in gut microbiota contribute to metabolic diseases, the connections between gut microbiota and the circulating metabolic state in GDM remain largely undetermined. To investigate the associations between gut microbiota and the circulating metabolome of GDM, we enrolled 40 pregnant women (20 with GDM and 20 non-diabetic control), and performed multi-omics association (MOA) study on 16s rRNA sequencing of fecal microbiota and 1H-NMR profiling of the plasma metabolome. The results suggested that both fecal microbiota and the plasma metabolome of the enrolled pregnant women could be separated along the vector of hyperglycemia. A close correlation between fecal microbiota and the plasma metabolome of GDM was observed by MOA approaches. Redundancy Analysis identified five plasma metabolites (glycerol, lactic acid, proline, galactitol and methylmalonic acid) and 98 members of fecal microbiota contributing to the close correlation between the plasma metabolome and fecal microbiota. Further spearman rank correlation analysis revealed that four out of five of the identified plasma metabolites (except galactitol) were correlated with hyperglycemia. Co-occurring network analysis suggested that 15 out of 98 of the members of fecal microbiota were positively correlated with each other, forming a co-occurring cohort (mainly consisted of the phylum Firmicutes). The results of this study demonstrated that alterations in fecal microbiota were associated with hyperglycemia related changes of the plasma metabolome of women with GDM, suggesting novel therapies against gut microbiota to alleviate GDM.
Introduction
Gestational Diabetes Mellitus (GDM) has attracted worldwide concern for its increasing prevalence and various adverse outcomes for the pregnant women and for the fetus.1 Although diverse risk factors for GDM have been intensively studied, its pathogenesis remains incompletely understood,2 underscoring the importance of novel risk factors exploration on GDM.3
Gut microbiota has been correlated with GDM.4,5 Alterations of gut microbiota were observed to be discriminative features of pregnant women with GDM to those non-diabetic control.6 The ratio of GDM enriched bacteria to the control enriched bacteria is correlated with maternal hyperglycemia.4 Fecal transplant was sufficient to confer GDM features to the mouse models.5 Although gut microbiota has emerging as a potential source of biomarkers for GDM,2 the interactions between gut microbiota and the host in GDM remains largely unknown.
Metabolites are closely correlated with the hyperglycemia in GDM.7 Pregnancy presents a unique metabolic stress by metabolic hormones for fetal development and appropriate nutrient allocation between mother and fetus.8 Metabolomics studies in the past decade have provided insights into key metabolites involved in GDM.9 Higher plasma levels of triglycerides10 and β-hydroxybutyrate11 were observed in the pregnant women with GDM. Higher urine levels of 3-hydroxyisovalerate and 2-hydroxyisobutyrate were presented during the second trimester in women who eventually presented with GDM over those who had normal pregnancies.12 Perturbations in BCAA metabolism were also observed in pregnant women with GDM, resulting in higher levels of metabolites that decrease insulin sensitivity and impact β-cell functions.13
While the associations between gut microbiota and circulating metabolites in other types of diabetes have been intensively studied,14 whether alterations in gut microbiota mediate changes in circulating metabolites of GDM requires further investigations. In the present study, we performed microbiota–metabolome association studies to reveal the relationship between changes in gut microbiota and perturbations in circulating metabolome of GDM women.
Materials and methods
Subjects
A cohort of 40 pregnant women entered the Second Hospital of Shanxi Medical University (Shanxi Province, PR China) between May and September of 2018 were enrolled in this study, half of which were GDMs diagnosed with the criteria below. Women with known pre-existing diabetes, chronic or serious acute infections, abnormal liver or kidney function, cardiovascular hematological diseases were excluded. Fasting plasma was collected and an oral glucose tolerance test (OGTT) was performed during the 24th to 26th weeks of gestation. GDM was diagnosed according to the criteria of the International Association of Diabetes and Pregnancy Study Group, with at least one plasma glucose level being no less than the following thresholds: fasting, 5.1 mmol L−1, OGTT – 1 hour, 10.0 mmol L−1, OGTT – 2 hour, 8.5 mmol L−1. All experiments were performed in accordance with the guidelines in the Declaration of Helsinki. Experiments were approved by the ethics committee at the Second Hospital of Shanxi Medical University. Informed consents were obtained from human participants of this study.
Demographic data and sample collection
Demographic data for the enrolled pregnant women was collected by an interview at the same day with OGTT and sample collection, including height, age, body weight, blood pressure, fundal height, and abdominal circumference. Overnight fasting feces were collected during the fourth trimester of pregnancy (median: 34 gestational weeks), and immediately aliquoted and stored in a −86 °C deep freezer. Overnight fasting EDTA anticoagulated blood was collected at the same day, and centrifuged at 1500 g for 15 min at 4 °C. The extracted plasma sample was immediately aliquoted and stored in a −86 °C deep freezer.
Untargeted metabolic profiling
1H-NMR based untargeted metabolic profiling of the plasma sample was performed using the protocol described previously.15 Briefly, 450 μL plasma sample was added into 350 μL D2O (containing 0.05% TSP [3-trimethylsilyl-[2, 2, 3, 3-D4]-propionate] as internal standard). The mix was vortexed and centrifuged at 13
000g for 20 min at 4 °C. Six hundred microliter of the supernatant was transferred into a 5 mm NMR tube for spectrometry profiling. 1H-NMR spectrometry was performed using a 600 MHz AVANCE III NMR spectrometer (Bruker, BioSpin, Germany), with the following parameters: pulse sequence Carr–Purcell–Meiboom–Gill (CPMG), scanning times 64 scans, spectral size 65
536 points, spectral width 1235.7 Hz, pulse width 40.5 μs, relaxation delay 1.0 s. Spectra processing was performed by MestReNova (v8.0.1, Mestrelab Research, Santiago de Compostela, Spain). The phase and baseline were corrected manually and the chemical shift of TSP was calibrated at 0.00 ppm. The spectra region of δ 0.81 to δ 8.50 were segmented at 0.01 ppm width after exclusion of the region corresponding to residual water (δ 4.75 to δ 5.16). The obtained data was normalized to the total sum of spectra before further analysis.
Multivariate pattern recognition analysis
Multivariate pattern recognition analysis was performed with SIMCA-P (v14.1, Umetrics AB, Umea, Sweden). Principle Component Analysis (PCA) was applied for assessment of the natural separation of the NMR data. Orthogonal projection to latent structures discriminant analysis (OPLS-DA) was performed to investigate the between-group difference by incorporating known classification information. The best-fitted OPLS-DA model was selected by a cross-validation of all models using a 200-cycle permutation test. The fitting validity and predictive ability of the selected OPLS-DA model were assessed by the parameters R2Y and Q2Y, respectively. The model performance of OPLS-DA was evaluated by sensitivity, specificity and accuracy through the SIMCA misclassification tool. Metabolite assignment was performed by searching the Human Metabolome Database (HMDB, http://www.hmdb.ca) and the Chenomx NMR suite (Chenomx Inc, Edmonton, Canada) with the chemical shift, coupling constant and peak type of the 1H-NMR features. Differential metabolites were defined as metabolites with differential between-group abundances, and simultaneously meet all of the following criteria: Importance for the Projection (VIP) > 1 in the selected OPLS-DA model,15 correlation coefficient (p(corr)) in S-plot analysis > 0.48 (through correlation coefficient table look-up), false discovery rate (fdr)-adjusted P < 0.05 in an independent-sample t-test. Metabolic pathway enrichment was performed by the MetaboAnalyst web portal (http://www.metaboanalyst.ca).
16s rDNA V3–V4 amplicon sequencing
Bacterial total DNA was extracted from the collected fecal sample using the HiPure Stool DNA Kit (Magen, Guangzhou, China) according to the manufacturer's instructions. The concentration and purity of the extracted DNA were determined by NanoDrop (Thermo Fisher, UD, USA) and agarose gel electrophoresis, respectively. V3–V4 region of 16s rDNA was selected and PCR amplified with the primer pairs: 341F: CCTACGGGNGGCWGCAG; 806R: GGACTACHVGGGTATCTAAT. The PCR cycling conditions was as follows, first round: initial denaturation at 94 °C for 2 minutes, 35 cycles of 98 °C for 10 seconds, 62 °C for 30 seconds and 68 °C for 30 seconds, followed by a final extension at 68 °C for 5 minutes. The PCR amplicons were purified with AmpureXp beads (Beckman Coulter, Life Sciences, CA, USA) and applied for the second round PCR amplification. Second round: initial denaturation at 94 °C for 2 minutes, 15 cycles of 98 °C for 10 seconds, 65 °C for 30 seconds and 68 °C for 30 seconds, followed by a final extension at 68 °C for 5 minutes. The amplicons of the second round PCR were purified with AmpureXp beads and quantified with Bioanalyzer DNA 1000 chip (Agilent Technologies, CA, USA). The DNA libraries were pair-end sequenced with the sequencing strategy PE300 by the MiSeq system (Illumina, San Diego, CA, USA).
Metagenome analysis
The raw reads of 16s rDNA sequencing data was filtered with the following parameters: maximum number of consecutive N 0, minimum sequence length 150 bp, minimum Q-score 20, maximum number of consecutive low-quality base calls allowed before truncating 3. The number of sequences per sample after filtration ranged from 93
720 to 204
203 (mean: 137
596, median: 133
597). Assembly of the pair-end sequencing reads was performed with FLASH v1.2.7. Operational taxonomic units (OTUs) were picked using UCLUST implemented in QIIME pipeline (v1.8.0) against the GreenGenes database (the May 2013 version, http://greengenes.secondgenome.com) at 97% identity. OTUs with relative abundance lower than 0.001% of the total OTUs were removed, leaving OTUs per sample ranged from 186 to 433 (mean: 305, median: 304). OTUs were normalized by the R package ALDEx version 2 (ALDEx2) before further multivariate and differential analysis.16 PCA and PCoA (by the R package ade4) were applied to evaluate the compositional changes of fecal microbiota in GDM. OTUs with significant between-group variations (adjusted P < 0.05) were selected by LEfSe. The metabolic potential of the altered microbiota was assessed by PICRUSt.
Co-inertia analysis (CIA)
CIA is a multivariate statistical technique, exploring the maximum co-variability between two datasets. CIA was performed by R software (v3.5.0, package vegan) to assess the co-variability between plasma metabolome and gut microbiota. The global similarity between the two datasets were evaluated by the parameter RV coefficient. The greater (scale 0–1) of the RV coefficient, the similar between the two datasets.
Procrustes analysis (PA)
PA was performed to assess the structural similarity between plasma metabolome and gut microbiota, based on superimposition of principal coordinates constructed from the distance matrices calculated from the square root of the Jensen–Shannon divergence.
Redundancy analysis (RDA)
To investigate the contributions of gut microbiota to the separation of plasma metabolome and vice versa, RDA was performed by R software (v3.5.0, package vegan) with default parameters. The fitness of each metabolite/OTU to an ordination of RDA was evaluated by envfit test.
Statistical analysis
Between-group statistical analyses were performed with two-tailed Student's t-test in SPSS 22.0. P values were adjusted with false discovery rate (fdr) correction in R (v3.5.0, package stats), and adjusted P < 0.05 were defined as statistically significant.
Data and codes availability
The raw 16s rDNA sequencing data have been deposited in the GenBank Sequence Read Archive with the BioProject ID PRJNA561655. The raw data of plasma metabolome are available upon request. Codes for CIA, PA and RDA are available upon request. Default parameters were used unless otherwise mentioned.
Results
Hyperglycemia in the pregnant women is a discriminating factor of the plasma metabolome
GDM is a complicated metabolic syndrome with hyperglycemia as one of the key features.1 Plasma metabolome reflects the outcome of hyperglycemia in the circulating system.17 To investigate the extent to which hyperglycemia could separate the plasma metabolome between women with GDM and the non-diabetic mellitus (NDM) control, we performed 1H-NMR based untargeted metabolomics analysis on plasma samples (Fig. 1). PCA was applied to investigate the clustering trends of metabolome between GDM and the NDM control, and to exclude possible outlier. A separation trend between GDM and the NDM control was observed in PCA score plot (Fig. 1a). OPLS-DA was further applied to discriminate women with GDM and the NDM control (Fig. 1b). The best-fitted OPLS-DA model was selected and validated by a cross-validation of all candidate models using a 200-cycle permutation test (Fig. 1d). The fit goodness (R2) and prediction ability (Q2) of the best-fitted model were 0.768 and 0.638, respectively. A clear separation between GDM and the NDM control was observed in the OPLS-DA model (Fig. 1b). The OPLS-DA model discriminated women with GDM from the NDM control with high sensitivity (0.95), specificity (0.95), accuracy (95%) and AUROC (0.95). These results suggested that changes occurred in the plasma metabolome of GDM.
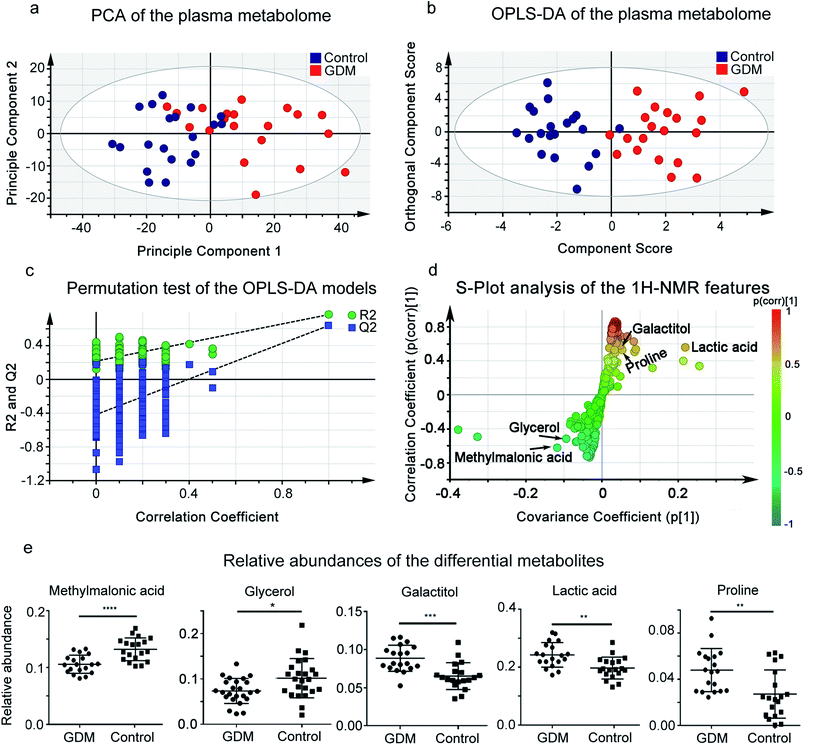 |
| Fig. 1 1H-NMR based plasma metabolomic analysis of the pregnant women. (a) Score plot of Principle Component Analysis (PCA) on plasma metabolome of the enrolled pregnant women in this study. PC1 and PC2 explain 57.97% of the total variances (48.50% and 9.47%, respectively). Red solid cycle, GDM group; blue solid cycle, non-diabetic control group. (b) Score plot of Orthogonal Projection to Latent Structures Discriminant Analysis (OPLS-DA) on plasma metabolome of the enrolled pregnant women in this study, based on the first predictive component (T scores [1]) and the first orthogonal component (orthogonal T scores [1]). The predictive and orthogonal variations in X explained by the model is 0.488 (R2X = 0.48). Total sum of variations in Y explained by the model is 0.768 (R2Y = 0.768). Prediction goodness of the model is 0.638 (Q2 = 0.638). (c) Permutation test of the OPLS-DA models. Plot of R2Y and Q2 from 200 permutation tests in OPLS-DA. The Y axis shows R2Y and Q2, the X axis shows the correlation of observed and permuted data. The two points on the upper-right correspond to R2Y and Q2 of the observed data set. Other points on the bottom-left correspond to R2Ys and Q2 of permuted data sets. The two plots suggested that the two models were well guarded against overfitting. (d) A representative OPLS-DA S-plot showing relative contribution of the 1H-NMR features to clustering of GDM group and the NDM control. This plot corresponds to Fig. 2b. Each point in the plot represents a 1H-NMR feature. The p[1] axis represents the magnitude of the 1H-NMR spectral features. (e) Relative abundances of the differential metabolites between the GDM group and the non-diabetic control group. The relative abundance of each metabolite was calculated by the original peak area against the total peak area of a sample. **P < 0.01; ***P < 0.0001; ****P < 0.00001. | |
To further select the differential metabolites between GDM and the NDM control, 1H-NMR features from the plasma metabolomic profiling were screened according to the criteria of differential metabolites defined in this study. A total of 15 features simultaneously met the three criteria, among which 5 metabolites were structurally identified (Table 1, Fig. 1 and S1†), including glycerol, glucose, lactic acid, proline, and methylmalonic acid. The relative abundances of the differential metabolites (Fig. 1e) suggested that methylmalonic acid and glycerol were decreased, while galactitol, lactic acid, and proline were elevated in the plasma metabolome of GDM.
Table 1 Differential plasma metabolites between women with GDM and the non-diabetic controla
Metabolites |
Chemical shift |
VIP |
p(corr) |
P-Value |
Adjusted P-value |
VIP: variable importance in the projection, p(corr): correlation coefficient. |
Methylmalonic acid |
1.22d, 3.14q |
1.10 |
−0.64 |
4.53 × 10−3 |
8.84 × 10−3 |
Proline |
2.34m, 3.33dt, 3.41dt, 4.12dd |
1.03 |
0.59 |
2.65 × 10−4 |
5.42 × 10−4 |
L-Lactic acid |
1.35d, 4.13q |
1.71 |
0.69 |
2.78 × 10−3 |
5.13 × 10−3 |
Glycerol |
3.55m, 3.64m |
1.14 |
−0.61 |
8.79 × 10−5 |
2.39 × 10−3 |
Galactitol |
3.97t |
3.27 |
0.72 |
5.80 × 10−4 |
4.85 × 10−2 |
Hyperglycemia discriminates the fecal microbiota of GDM from the non-diabetic control
Gut microbiota has been proved to be a key contributor to metabolic disorders of the host.18 To investigate the potential contributions of gut microbiota to GDM, we evaluated the compositional changes of fecal microbiota along the vector of hyperglycemia. By PCA (Fig. 2a) and PCoA (Fig. 2b), fecal microbiota of GDM was discriminated from that of the NDM control, suggesting compositional changes occurred along the vector of hyperglycemia. We also observed significant alterations in the alpha diversity (Fig. 2c, P = 0.039) and beta diversity (Fig. 2d, P = 3.41 × 10−6) of fecal microbiota between GDM and the NDM control. These results demonstrated that the fecal microbiota of GDM was altered with significant reduced alpha and beta diversity.
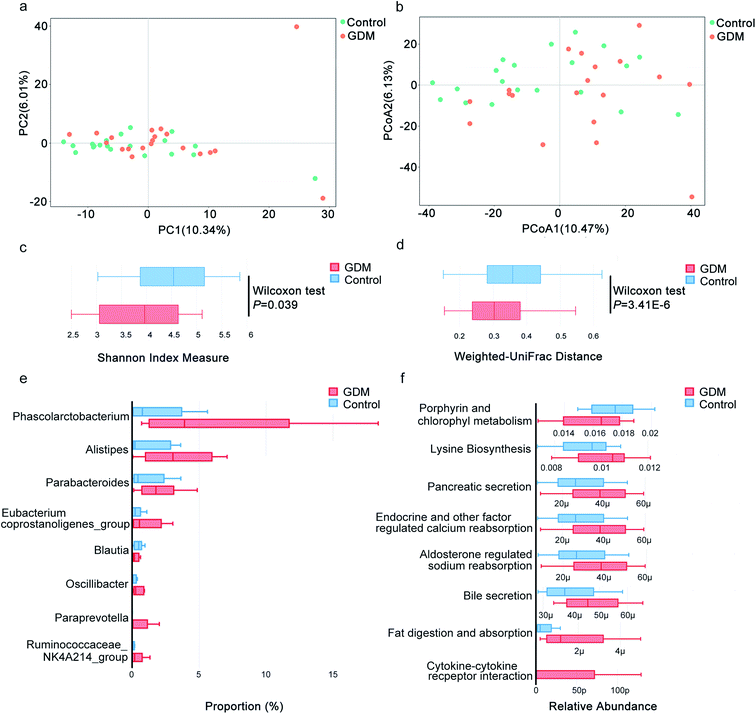 |
| Fig. 2 16s rRNA sequencing based fecal microbiota analysis of the pregnant women with GDM. (a) Principle Component Analysis (PCA) to study the natural separation of the fecal microbiota from the enrolled pregnant women. The first two principle components explained 16.35% of the total variations (10.34% and 6.01%, respectively). Orange solid cycle, GDM group; Green solid cycle, non-diabetic control group. (b) Principle Coordination Analysis (PCoA) of the fecal microbiota based on unweighted UniFrac distance. The first two coordinates explained 16.60% of the total variations (10.47% and 6.13%, respectively). Orange solid cycle, GDM group; green solid cycle, non-diabetic control group. (c) Shannon index for measurement of alpha diversity of the fecal microbiota. (d) Unweighted UniFrac for measurement of beta diversity of the fecal microbiota. (e) Bacteria genera with differential between-group abundances in the fecal microbiota of the pregnant women. Only genera with significantly altered between-group abundances (Wilcoxon test, adjust P < 0.05) were exhibited. Number on the coordinate axis indicates the number of OTU belonging to a genus. (f) Metabolic pathways predicted by Tax4Fun on bacteria genera with differential between-group abundances in the fecal microbiota of the pregnant women. Number on the coordinate axis indicates the relative abundance of a metabolic pathway calculated by the hit number of a pathway against the total hits. μ, 10 × 10−6; p, 10 × 10−12. | |
Among the genera with significant altered abundances in the fecal microbiota, Blautia was decreased in GDM, while a panel of bacterial genera were increased in GDM, including Phascolarctobacterium, Alistipes, Parabacteroides, Eubacterium coprostanoligenes_group, Oscillibacter, Paraprevotella and Ruminococcaceae NK4A214_group (Fig. 2e). Pathway analysis of the significant altered bacterial genera (Fig. 2f) revealed that porphyrin and chlorophyll metabolism was significantly decreased in GDM and a panel of metabolic pathways was elevated in GDM including lysine biosynthesis, pancreatic secretion, endocrine and other factor regulated calcium reabsorption, bile secretion, fat digestion and absorption and cytokine–cytokine receptor interaction. These results suggested that the fecal microbiota of GDM was featured with reduced diversity in overall composition and significantly elevated abundances of several bacteria genera.
Fecal microbiota correlated with the plasma metabolome in GDM
Because both of the fecal microbiota and the plasma metabolome of the enrolled pregnant women could be discriminated by hyperglycemia, we speculated that potential correlations occurred between these two profiling. We then applied multi-omics association approaches to evaluate the relationship between the fecal microbiota and the plasma metabolome (Fig. 3a and b). CIA is a multivariate statistical analysis that could assess relationships and trends in multiple high-dimensional omics data.19 Fig. 3a shows the projections of datasets from the enrolled pregnant women onto the first two principal components of CIA. The datasets of fecal microbiota and plasma metabolome are transformed into the same projection. The coordinates of the two datasets for each pregnant woman are connected by lines, the length of which indicates the divergence between the fecal microbiota and the plasma metabolome. The shorter the line, the higher the level of concordance. The CIA plot of the first two principal components (Fig. 3a) shows partially projected of the fecal microbiota and the plasma metabolome along the first axis (PC1, horizontal), and entirely projected of the two datasets along the second axis (PC2, vertical). The result of CIA suggested similar trends in fecal microbiota and plasma metabolome of the enrolled pregnant women (RV coefficient = 0.82, scale 0–1), indicating that some of the variant sources of biological information were similar. PA is a statistical shape analysis to analyze the distribution of a set of shapes and has been successfully applied to evaluate the relationships among multi-omics datasets.20 The result of PA indicated that the shape of the fecal microbiota partially fitted with that of the plasma metabolome (Fig. 3b). These results suggested that the fecal microbiota of GDM was correlated with the plasma metabolome.
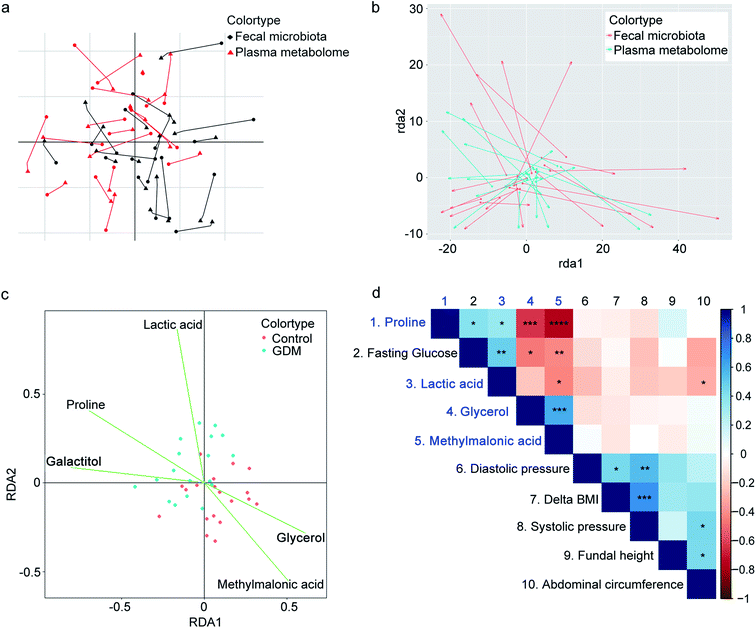 |
| Fig. 3 Multi-omics association studies on the fecal microbiota and the plasma metabolome of the pregnant women with GDM. (a) Co-inertia analysis (CIA) on the fecal microbiota and the plasma metabolome. The first two axes of CIA represent the fecal microbiota and the plasma metabolome datasets. Black solid cycle represents the fecal microbiota, red solid triangle represents the plasma metabolome. Edges link the fecal microbiota and the plasma metabolome from the same pregnant women. The length of the line is proportional to the divergence between the data from a same pregnant woman. The shorter the edge, the higher the level of concordance. (b) Procrustes analysis (PA) of the fecal microbiota and the plasma metabolome. (c) Redundancy analysis (RDA) on the differential plasma metabolites against the fecal microbiota of GDM. (d) Heatmap of the spearman rank correlations between the differential plasma metabolites and the clinical indices of GDM. * represents the results of statistical test: *0.05; **0.01; ***0.001; ****0.0001. | |
Correlation between fecal microbiota and plasma metabolome indicate potential mutual contributions.18 To investigate the contributions of plasma metabolites to the alterations of fecal microbiota, we performed RDA on the differential plasma metabolites against fecal microbiota (Fig. 3c). Four out of five of the differential plasma metabolites significantly contributed to the alterations in the fecal microbiota of GDM, including lactic acid, proline, methylmalonic acid, and glycerol (significant test of the correlation in the envfit test of RDA: Pr < 0.05, Table S1†). To further evaluate the correlations of GDM related clinical indices with the four metabolites contributing to the alterations of fecal microbiota, we performed spearman rank correlation (Fig. 3d). All of the four metabolites were significantly correlated with fasting glucose (Table S2†), while lactic acid was negatively correlated with abdominal circumference of the enrolled pregnant women (P = 0.03, ρ = −0.67). Refer to the relationship among the four differential metabolites, proline was positively correlated with lactic acid, and negatively correlated with glycerol and methylmalonic acid. Methylmalonic acid was positively correlated with glycerol, and negatively correlated with lactic acid and proline. These results suggested that hyperglycemia associated plasma metabolites were correlated with the alterations in GDM fecal microbiota.
Closely correlated members of fecal microbiota contributed to the alterations in plasma metabolome of GDM
To evaluate the potential contributions of fecal microbiota to the alterations of plasma metabolome of GDM, we performed RDA on all of the bacterial genera against the plasma metabolome. A total of 98 bacterial genera were observed to significantly contributed to the alterations of the plasma metabolome (Table S3†), of which 63.27% were Firmicutes, 16.33% were Bacteroidetes, 8.16% were Actinobacteria, 8.16% were Proteobacteria. To further investigate the correlations within the 98 bacterial genera, spearman rank correlation was performed and visualized with correlation network (Fig. 4). A total of 15 bacteria genera were observed to be significantly correlated with each other (Table S4†), of which 80% (12/15) were Firmicutes, 13.3% (2/15) were Bacteroidetes, 6.7% were Proteobacteria. These results suggested that connections among members of Firmicutes contributed to the alterations of the plasma metabolome of GDM.
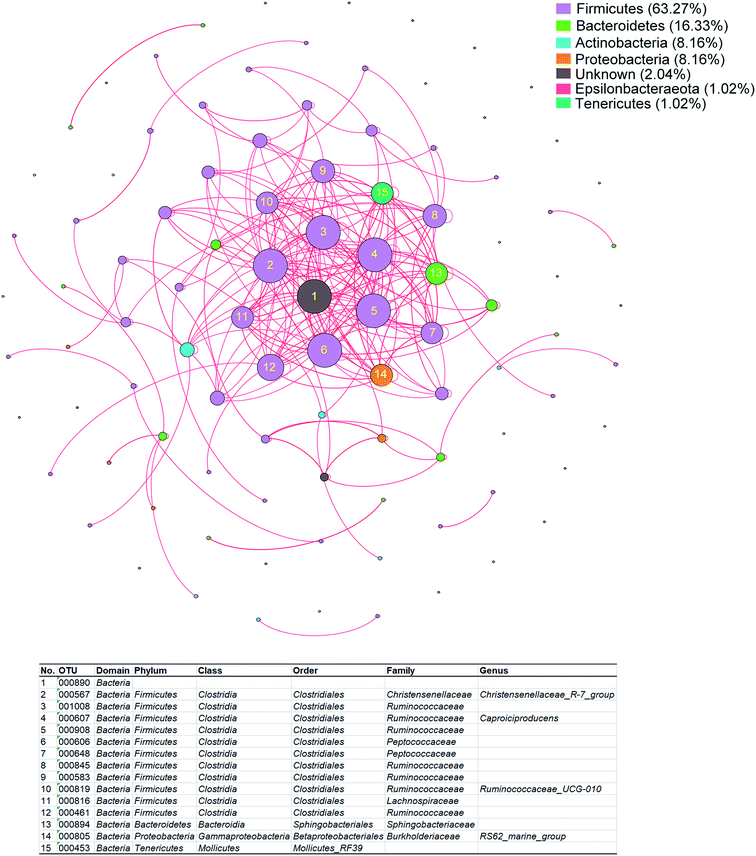 |
| Fig. 4 Co-occurring network of the bacteria genera contributing to the alterations in plasma metabolome of GDM. The co-occurring network was built based on spearman rank correlations among members of fecal microbiota that contributed to the alterations of plasma metabolome. The nodes were colored by bacteria genera. The size of each node is proportional to the number of connections. The edges were colored by the direction of correlation, red lines represent positive correlations, black lines represent negative correlations. The edge thickness is proportional to the strength of correlation. The color panel in the upper right represents the proportion of each phylum in the network. The significantly correlated nodes (correlation coefficient (ρ) > 0.6, and adjusted P < 0.05) were numbered, the assignment of which were listed in the table below. | |
Discussion
Although plasma metabolome and gut microbiota have been respectively correlated with GDM, the associations between these two profiling remain largely undetermined. In the present study, we performed 1H-NMR based plasma metabolomics, 16s rDNA sequencing of fecal microbiota, and multi-omics association approaches, to reveal a close correlation between fecal microbiota and plasma metabolome of GDM. Four hyperglycemia correlated plasma metabolites were observed to contribute to the alterations of fecal microbiota. While a total of 98 bacteria genera significantly contributed to the changes of the plasma metabolome in GDM, among which 15 genera were positively correlated with each other. The results of this study suggested that a population of Firmicutes of the fecal microbiota contributed to the GDM associated changes of the plasma metabolome.
Alterations in levels of plasma metabolites have been correlated with GDM.21 As a biomarker of vitamin B12 deficiency,22 high blood level of methylmalonic acid is observed in the third trimester than during the other trimesters of pregnancy.23 Nevertheless, the non-pregnant adult possessed a higher level of methylmalonic acid.22 This study for the first time reported that pregnant women with GDM possessed lower level of methylmalonic acid than the NDM control. The fetal growth correlated maternal circulating glycerol was reported to be significantly higher in the plasma of women with GDM comparing to the NDM control,24 which is consistent with the finding of this study. Maternal blood lactate (the salt form of lactic acid) level has a significant role in determining the metabolic milieu of both mother and the fetus, which was reported to be significantly higher in GDM mothers than in the NDM control.25 The level of proline in the umbilical vein and artery of GDM was associated with hyperglycemia26 and was reported to be significantly increased in women with GDM,27 which is similar to the observation of this study. In accordance with the finding of this study, the level of galactitol was elevated in maternal serum, amniotic fluid and the fetal cord serum,28 suggesting that galactitol could cross the placental barrier and exert effects on the fetus. Although the abundance variations of the five differential metabolites observed in this study have been documented in women with GDM, further studies are required to reveal the sources of the metabolites and their action pathway to GDM.
Changes in gut microbiota have been correlated with GDM. Phascolarctobacterium is a butyrate-producing bacteria,29 higher level of which was reported to be positively correlated with insulin sensitivity.30 Despite this, a higher level of Phascolarctobacterium was observed in women with GDM,31 which is in accordance with the finding of the present study. Alistipes was positively correlated with fat intakes in pregnant women,6 which was reported to be enriched in the non-diabetic pregnant women,32 contrary to the observation of this study. Parabacteroides, as a potential next-generation probiotics in reverse obesity and insulin resistance, was reported to be enriched in women with GDM,31 similar to the finding of this study. Eubacterium coprostanoligenes is a cholesterol-reducing anaerobe,33 the enrichment of which in GDM was reported for the first time in the present study. Blautia was reported to be inversely correlated with the levels of insulin and hemoglobin A1C. In accordance with the observation of this study, the level of Blautia was reported to be increased in women with GDM.6 Ruminococcaceae is a dominant family in energy metabolism, and was strongly correlated with levels of adipokine and insulin. High abundance of family Ruminococcaceae in early pregnancy may be related to adverse metabolic health. In accordance with this, Ruminococcaceae NK4A214 was observed to be enriched in women with GDM in the present study, indicating a potential adverse effect to pregnancy. Oscillibacter is positively correlated with gut permeability and metabolic dysfunction in diet-induced obese mice.34 Contrary to the finding of this study, Oscillibacter was reported to be depleted in GDM women.32 Paraprevotella was reported to be strongly correlated with cardiovascular disease risk,35 which was enriched in the fecal microbiota of rats exposure to prenatal androgen, similar to the findings of the present study. Besides the associations of changes in microbiota with GDM, further studies are recommended to investigate the underlying biomedical significance of the alterations in the members of gut microbiota.
The associations between plasma metabolites and members of gut microbiota in GDM were rarely investigated. By far, only one study reported that the increased diversity and concentrations of beneficial gut microbes were associated with the metabolism of pregnant sows, suggesting that manipulating gut microbiota may potentially influence metabolism and healthy during pregnancy in sows.36 Nevertheless, the effects of gut microbiota on the plasma metabolites observed in this study (proline, lactic acid, galactitol and methylmalonic acid) have been reported previously in other cases except GDM. By comparing the conventionally raised and germ-free mice, Mardinoglu et al.37 reported that gut microbiota affected the host metabolism of proline. Lactic acid is a common metabolite of the gut microbiota, while microbiota-derived lactic acid activates production of reactive oxygen species by the intestinal NADPH oxidase Nox and shortens drosophila lifespan.38 Galactitol is a product of hepatic galactose metabolism, which has an inhibitory effect on the E. coli growing in minimal medium with glycerol.39 Galactitol is enriched in the gut of breast-fed infants, and substantially reduced in the plasma due to fasting intervention.40 Gut microbiota contribute a significant proportion of the substrate of the circulating methylmalonic acid, and is a potential target in management of methylmalonic acidemia (a rare inborn error of metabolism).41 In addition to the above associations between gut microbiota and plasma metabolites, the results of the present study suggested novel contributions of gut microbiota to variations of the plasma metabolites in women with GDM.
To avoid false positive rate commonly found in high-dimensional data,42 we applied adjusted P < 0.05 in selection of 1H-NMR features with significantly altered abundances. Although correlations between members of gut microbiota and metabolites of plasma metabolome in GDM have been observed in this study, these correlations are also influenced by diet and changes in metabolic health. Further biological validation studies are recommended to evaluate the contribution of gut microbiota to plasma metabolome in GDM excluding the influence of diet and metabolic status.
Conclusions
In conclusion, multi-omics association analysis in this study suggested that fecal microbiota was closely correlated with plasma metabolome in women with GDM. Changes in gut microbiota contributed to the altered abundance of hyperglycemia-related plasma metabolites, including proline, lactic acid, galactitol and methylmalonic acid. Among the bacteria genera contributing to the altered plasma metabolome in GDM, a cohort of 15 genera (mainly consisted of Firmicutes) was positively correlated with each other, suggesting synergistic effects of gut bacteria on the alterations in plasma metabolome of GDM.
Conflicts of interest
The authors report no conflicts of interest in this work.
Acknowledgements
We thank the clinicians from Second Hospital of Shanxi Medical University for clinical sample collection. The study was supported by the National Major Science and Technology Projects (No. 2017ZX10201201-004-003), as well as the Young Scientists Fund of the National Natural Science Foundation of China (No. 81600951 and 81301441).
References
- W. L. Lowe Jr, D. M. Scholtens, L. P. Lowe, A. Kuang, M. Nodzenski, O. Talbot, P. M. Catalano, B. Linder, W. J. Brickman, P. Clayton, C. Deerochanawong, J. Hamilton, J. L. Josefson, M. Lashley, J. M. Lawrence, Y. Lebenthal, R. Ma, M. Maresh, D. McCance, W. H. Tam, D. A. Sacks, A. R. Dyer, B. E. Metzger and H. F.-u. S. C. R. Group, J. Am. Med. Assoc., 2018, 320, 1005–1016 CrossRef PubMed.
- N. Rodrigo and S. J. Glastras, J. Clin. Med., 2018, 7(6), pii: E120 CrossRef PubMed.
- G. E. Avalos, L. A. Owens, F. Dunne and A. D. Collaborators, Diabetes Care, 2013, 36, 3040–3044 CrossRef PubMed.
- J. Wang, J. Zheng, W. Shi, N. Du, X. Xu, Y. Zhang, P. Ji, F. Zhang, Z. Jia, Y. Wang, Z. Zheng, H. Zhang and F. Zhao, Gut, 2018, 67, 1614–1625 CrossRef CAS PubMed.
- O. Koren, J. K. Goodrich, T. C. Cullender, A. Spor, K. Laitinen, H. K. Backhed, A. Gonzalez, J. J. Werner, L. T. Angenent, R. Knight, F. Backhed, E. Isolauri, S. Salminen and R. E. Ley, Cell, 2012, 150, 470–480 CrossRef CAS PubMed.
- I. Ferrocino, V. Ponzo, R. Gambino, A. Zarovska, F. Leone, C. Monzeglio, I. Goitre, R. Rosato, A. Romano, G. Grassi, F. Broglio, M. Cassader, L. Cocolin and S. Bo, Sci. Rep., 2018, 8, 12216 CrossRef PubMed.
- T. Liu, J. Li, F. Xu, M. Wang, S. Ding, H. Xu and F. Dong, Anal. Bioanal. Chem., 2016, 408, 1125–1135 CrossRef CAS PubMed.
- W. Perng, S. L. Rifas-Shiman, S. McCulloch, L. Chatzi, C. Mantzoros, M. F. Hivert and E. Oken, Metabolism, 2017, 76, 11–22 CrossRef CAS PubMed.
- X. Mao, X. Chen, C. Chen, H. Zhang and K. P. Law, Clin. Chim. Acta, 2017, 475, 116–127 CrossRef CAS PubMed.
- M. G. Martineau, C. Raker, P. H. Dixon, J. Chambers, M. Machirori, N. M. King, M. L. Hooks, R. Manoharan, K. Chen, R. Powrie and C. Williamson, Diabetes Care, 2015, 38, 243–248 CrossRef PubMed.
- D. Dudzik, M. Zorawski, M. Skotnicki, W. Zarzycki, A. Garcia, S. Angulo, M. P. Lorenzo, C. Barbas and M. P. Ramos, J. Pharm. Biomed. Anal., 2017, 144, 90–98 CrossRef CAS PubMed.
- D. Sachse, L. Sletner, K. Morkrid, A. K. Jenum, K. I. Birkeland, F. Rise, A. P. Piehler and J. P. Berg, PLoS One, 2012, 7, e52399 CrossRef CAS PubMed.
- U. Andersson-Hall, C. Gustavsson, A. Pedersen, D. Malmodin, L. Joelsson and A. Holmang, J. Diabetes Res., 2018, 2018, 4207067 CrossRef PubMed.
- W. Zhou, M. R. Sailani, K. Contrepois, Y. Zhou, S. Ahadi, S. R. Leopold, M. J. Zhang, V. Rao, M. Avina, T. Mishra, J. Johnson, B. Lee-McMullen, S. Chen, A. A. Metwally, T. D. B. Tran, H. Nguyen, X. Zhou, B. Albright, B. Y. Hong, L. Petersen, E. Bautista, B. Hanson, L. Chen, D. Spakowicz, A. Bahmani, D. Salins, B. Leopold, M. Ashland, O. Dagan-Rosenfeld, S. Rego, P. Limcaoco, E. Colbert, C. Allister, D. Perelman, C. Craig, E. Wei, H. Chaib, D. Hornburg, J. Dunn, L. Liang, S. M. S. Rose, K. Kukurba, B. Piening, H. Rost, D. Tse, T. McLaughlin, E. Sodergren, G. M. Weinstock and M. Snyder, Nature, 2019, 569, 663–671 CrossRef CAS PubMed.
- J. S. Tian, X. T. Xia, Y. F. Wu, L. Zhao, H. Xiang, G. H. Du, X. Zhang and X. M. Qin, Sci. Rep., 2016, 6, 33820 CrossRef CAS PubMed.
- G. B. Gloor, J. M. Macklaim, V. Pawlowsky-Glahn and J. J. Egozcue, Front. Microbiol., 2017, 8, 2224 CrossRef PubMed.
- B. Jin, L. Liu, S. Zhang, X. Cao, Y. Xu, J. Wang and L. Sun, Metab. Syndr. Relat. Disord., 2017, 15, 439–449 CrossRef CAS PubMed.
- E. E. Canfora, R. C. R. Meex, K. Venema and E. E. Blaak, Nat. Rev. Endocrinol., 2019, 15, 261–273 CrossRef CAS PubMed.
- P. Bady, S. Doledec, B. Dumont and J. F. Fruget, Comptes Rendus Biol., 2004, 327, 29–36 CrossRef PubMed.
- J. Chong and J. Xia, Metabolites, 2017, 7(4), pii: E62 CrossRef PubMed.
- C. Hellmuth, K. L. Lindsay, O. Uhl, C. Buss, P. D. Wadhwa, B. Koletzko and S. Entringer, Int. J. Obes., 2017, 41, 159–169 CrossRef CAS PubMed.
- L. R. Solomon, J. Am. Geriatr. Soc., 2013, 61, 577–582 CrossRef PubMed.
- R. Choi, S. Choi, Y. Lim, Y. Y. Cho, H. J. Kim, S. W. Kim, J. H. Chung, S. Y. Oh and S. Y. Lee, Nutrients, 2016, 8(12), pii: E797 CrossRef PubMed.
- U. M. Schaefer-Graf, K. Meitzner, H. Ortega-Senovilla, K. Graf, K. Vetter, M. Abou-Dakn and E. Herrera, Diabetic Med., 2011, 28, 1053–1059 CrossRef CAS PubMed.
- M. S. Huhtala, K. Tertti, O. Pellonpera and T. Ronnemaa, Diabetes Res. Clin. Pract., 2018, 146, 8–17 CrossRef CAS PubMed.
- Y. P. Lu, C. Reichetzeder, C. Prehn, K. von Websky, T. Slowinski, Y. P. Chen, L. H. Yin, B. Kleuser, X. S. Yang, J. Adamski and B. Hocher, Cell. Physiol. Biochem., 2018, 45, 625–638 CrossRef CAS PubMed.
- I. Cetin, M. S. de Santis, E. Taricco, T. Radaelli, C. Teng, S. Ronzoni, E. Spada, S. Milani and G. Pardi, Am. J. Obstet. Gynecol., 2005, 192, 610–617 CrossRef CAS PubMed.
- P. Briones, M. Giros and V. Martinez, J. Inherited Metab. Dis., 2001, 24, 79–80 CrossRef CAS PubMed.
- F. Liu, P. Li, M. Chen, Y. Luo, M. Prabhakar, H. Zheng, Y. He, Q. Qi, H. Long, Y. Zhang, H. Sheng and H. Zhou, Sci. Rep., 2017, 7, 11789 CrossRef PubMed.
- N. Naderpoor, A. Mousa, L. F. Gomez-Arango, H. L. Barrett, M. Dekker Nitert and B. de Courten, J. Clin. Med., 2019, 8(4), pii: E452 CrossRef PubMed.
- Y. S. Kuang, J. H. Lu, S. H. Li, J. H. Li, M. Y. Yuan, J. R. He, N. N. Chen, W. Q. Xiao, S. Y. Shen, L. Qiu, Y. F. Wu, C. Y. Hu, Y. Y. Wu, W. D. Li, Q. Z. Chen, H. W. Deng, C. J. Papasian, H. M. Xia and X. Qiu, Gigascience, 2017, 6, 1–12 CrossRef PubMed.
- M. K. W. Crusell, T. H. Hansen, T. Nielsen, K. H. Allin, M. C. Ruhlemann, P. Damm, H. Vestergaard, C. Rorbye, N. R. Jorgensen, O. B. Christiansen, F. A. Heinsen, A. Franke, T. Hansen, J. Lauenborg and O. Pedersen, Microbiome, 2018, 6, 89 CrossRef PubMed.
- T. A. Freier, D. C. Beitz, L. Li and P. A. Hartman, Int. J. Syst. Bacteriol., 1994, 44, 137–142 CrossRef CAS PubMed.
- Y. Y. Lam, C. W. Ha, C. R. Campbell, A. J. Mitchell, A. Dinudom, J. Oscarsson, D. I. Cook, N. H. Hunt, I. D. Caterson, A. J. Holmes and L. H. Storlien, PLoS One, 2012, 7, e34233 CrossRef CAS PubMed.
- T. N. Kelly, L. A. Bazzano, N. J. Ajami, H. He, J. Zhao, J. F. Petrosino, A. Correa and J. He, Circ. Res., 2016, 119, 956–964 CrossRef CAS PubMed.
- Y. J. Ji, H. Li, P. F. Xie, Z. H. Li, H. W. Li, Y. L. Yin, F. Blachier and X. F. Kong, J. Appl. Microbiol., 2019, 127, 867–879 CrossRef CAS PubMed.
- A. Mardinoglu, S. Shoaie, M. Bergentall, P. Ghaffari, C. Zhang, E. Larsson, F. Backhed and J. Nielsen, Mol. Syst. Biol., 2015, 11, 834 CrossRef PubMed.
- I. Iatsenko, J. P. Boquete and B. Lemaitre, Immunity, 2018, 49, 929–942 e925 CrossRef CAS PubMed.
- J. Barroso-Batista, A. Sousa, M. Lourenco, M. L. Bergman, D. Sobral, J. Demengeot, K. B. Xavier and I. Gordo, PLoS Genet., 2014, 10, e1004182 CrossRef PubMed.
- R. L. Washburn, J. E. Cox, J. B. Muhlestein, H. T. May, J. F. Carlquist, V. T. Le, J. L. Anderson and B. D. Horne, Nutrients, 2019, 11(2), pii: E246 CrossRef PubMed.
- A. Burlina, S. Tims, F. van Spronsen, W. Sperl, A. P. Burlina, M. Kuhn, J. Knol, M. Rakhshandehroo, T. Coskun, R. H. Singh and A. MacDonald, Expert Opin. Orphan Drugs, 2018, 6, 683–692 CrossRef CAS.
- R. Liu, J. Hong, X. Xu, Q. Feng, D. Zhang, Y. Gu, J. Shi, S. Zhao, W. Liu, X. Wang, H. Xia, Z. Liu, B. Cui, P. Liang, L. Xi, J. Jin, X. Ying, X. Wang, X. Zhao, W. Li, H. Jia, Z. Lan, F. Li, R. Wang, Y. Sun, M. Yang, Y. Shen, Z. Jie, J. Li, X. Chen, H. Zhong, H. Xie, Y. Zhang, W. Gu, X. Deng, B. Shen, X. Xu, H. Yang, G. Xu, Y. Bi, S. Lai, J. Wang, L. Qi, L. Madsen, J. Wang, G. Ning, K. Kristiansen and W. Wang, Nat. Med., 2017, 23, 859–868 CrossRef CAS PubMed.
Footnotes |
† Electronic supplementary information (ESI) available. See DOI: 10.1039/c9ra07799e |
‡ These authors contributed equally to this work. |
|
This journal is © The Royal Society of Chemistry 2020 |
Click here to see how this site uses Cookies. View our privacy policy here.