DOI:
10.1039/C9MO00130A
(Research Article)
Mol. Omics, 2020,
16, 39-58
Regulatory network analysis of Paneth cell and goblet cell enriched gut organoids using transcriptomics approaches†
Received
16th August 2019
, Accepted 23rd October 2019
First published on 10th December 2019
Abstract
The epithelial lining of the small intestine consists of multiple cell types, including Paneth cells and goblet cells, that work in cohort to maintain gut health. 3D in vitro cultures of human primary epithelial cells, called organoids, have become a key model to study the functions of Paneth cells and goblet cells in normal and diseased conditions. Advances in these models include the ability to skew differentiation to particular lineages, providing a useful tool to study cell type specific function/dysfunction in the context of the epithelium. Here, we use comprehensive profiling of mRNA, microRNA and long non-coding RNA expression to confirm that Paneth cell and goblet cell enrichment of murine small intestinal organoids (enteroids) establishes a physiologically accurate model. We employ network analysis to infer the regulatory landscape altered by skewing differentiation, and using knowledge of cell type specific markers, we predict key regulators of cell type specific functions: Cebpa, Jun, Nr1d1 and Rxra specific to Paneth cells, Gfi1b and Myc specific for goblet cells and Ets1, Nr3c1 and Vdr shared between them. Links identified between these regulators and cellular phenotypes of inflammatory bowel disease (IBD) suggest that global regulatory rewiring during or after differentiation of Paneth cells and goblet cells could contribute to IBD aetiology. Future application of cell type enriched enteroids combined with the presented computational workflow can be used to disentangle multifactorial mechanisms of these cell types and propose regulators whose pharmacological targeting could be advantageous in treating IBD patients with Crohn's disease or ulcerative colitis.
Introduction
Gut barrier integrity is critically important for efficient nutrient absorption and maintenance of intestinal homeostasis1 and is maintained by the combined action of the various cell types lining the intestinal epithelium.2 These intestinal epithelial cells serve to mediate signals between the gut microbiota and the host innate/adaptive immune systems.3,4 Disruption of epithelial homeostasis along with dysregulated immune responses are some of the underlying reasons behind the development of inflammatory bowel disease (IBD) such as Crohn's disease (CD) and ulcerative colitis (UC).5 Therefore, a greater insight into the functions of intestinal cells will further our understanding of the aetiology of inflammatory gut conditions.
To date, various cell types have been identified in the intestinal epithelium based on specific functional and gene expression signatures. Paneth cells residing in the small intestinal crypts of Lieberkühn help to maintain the balance of the gut microbiota by secreting anti-microbial peptides, cytokines and other trophic factors.6 Located further up the intestinal crypts, goblet cells secrete mucin, which aggregates to form the mucus layer, which acts as a chemical and physical barrier between the intestinal lumen and the epithelial lining.7 Both of these cell populations have documented roles in gut-related diseases.8,9 Dysfunctional Paneth cells with reduced secretion of anti-microbial peptides have been shown to contribute to the pathogenesis of CD,10 while reduction in goblet cell numbers and defective goblet cell function has been associated with UC in humans.11
Recent studies have employed single cell transcriptomics sequencing of tissue samples to characterise the proportion and signatures of different epithelial cell types in the intestines of healthy and IBD patients.12–14 However, to provide deeper insights into the role of specific cell populations (such as Paneth cells and goblet cells) in IBD, in vitro models are required for in depth testing and manipulation. Such models can be used to study specific mechanisms of action, host-microbe interactions, intercellular communication, patient specific therapeutic responses and to develop new diagnostic approaches. Due to ease of manipulation, observation and analysis, organoid models, including small intestinal models (enteroids), are increasingly used in the IBD field.15–17 Enteroid cell culture systems employ growth factors to expand and differentiate Lgr5+ stem cells into spherical models of the small intestinal epithelia which recapitulate features of the in vivo intestinal tissue.18–20 It has been shown that these enteroids contain all the major cell types of the intestinal epithelium, and exhibit normal in vivo functions.21 These models, generated using intestinal tissue from mice, from human patient biopsies or from induced pluripotent stem cells (iPSCs), have proven particularly valuable for the study of complex diseases which lack other realistic models and exhibit large patient variability, such as IBD.22,23 Small molecule treatments have been developed that skew the differentiation of enteroids towards Paneth cell or goblet cell lineages, improving representation of these cells within the enteroid cell population.24,25 Specifically, differentiation can be directed towards the Paneth cell lineage through the addition of DAPT, which inhibits notch signaling, and CHIR99021, which inhibits GSK3β-mediated β-catenin degradation. Enteroid cultures enriched in goblet cells can be generated through the addition of DAPT and IWP-2, an inhibitor of Wnt signaling.25 Whilst these methods do not present single cell type resolution, they provide useful tools to study Paneth cell and goblet cell populations in the context of the other major epithelial cell types.26 A recent study by Mead et al. found that Paneth cells from enriched enteroids more closely represent their in vivo counterparts than those isolated from conventionally differentiated enteroids, based on transcriptomics, proteomics and morphologic data.27 Furthermore, we have shown that enteroids enriched for Paneth cells and goblet cells recapitulate in vivo characteristics on the proteomics level28,29 and that they are a useful tool for the investigation of health and disease related processes in specific intestinal cell types.29
Nevertheless, the effect of Paneth cell and goblet cell enrichment of enteroids on key regulatory landscapes has not been extensively characterised. In this study, we comprehensively profiled mRNAs, miRNAs and lncRNAs from mouse derived, 3D conventionally differentiated enteroids (control), Paneth cell enriched enteroids (PCeE) and goblet cell enriched enteroids (GCeE) to determine the extent to which these enteroids display increased Paneth cell and goblet cell signatures. We applied a systems-level analysis of regulatory interactions within the PCeEs and GCeEs to further characterise the effect of cell type enrichment and to predict key molecular regulators involved with Paneth cell and goblet cell specific functions. This analysis was carried out using interactions networks, which are a primary method to collate, visualise and analyse biological systems. These networks are a type of systems biology data representation, which aids the interpretation of -omics read-outs by contextualising genes/molecules of interest and identifying relevant signalling and regulatory pathways.30,31 In the presented analysis, the nodes of the interaction networks represent genes/molecules of interest from the transcriptomics data and the edges represent regulatory connections (molecular interactions) between the nodes inferred from databases. Studying regulatory interactions using interaction networks has proven useful to uncover how cells respond to changing environments at a transcriptional level, to prioritise drug targets and to investigate the downstream effects of gene mutations and knockouts.32–34
We used network approaches to interpret the PCeE and GCeE transcriptomic data by integrating directed regulatory connections from resources containing transcriptional and post-transcriptional interactions. This integrative strategy led us to define regulatory network landscapes altered by Paneth cell and goblet cell enrichment of enteroids, termed the network and GCeE network, respectively (Fig. 1). By incorporating known Paneth cell and goblet cell markers, we used these networks to predict master regulators of Paneth cell and goblet cell differentiation and/or maintenance in the enriched enteroids. Furthermore, we highlighted varying downstream actions of shared regulators between the cell types. This phenomenon, called regulatory rewiring, highlights the importance of changes in regulatory connections in the function and differentiation of specific cell types. Taken together, we show that cell type enriched enteroids combined with the presented network biology workflow have potential for application to the study of epithelial dysfunction and mechanisms of action of multifactorial diseases such as IBD.
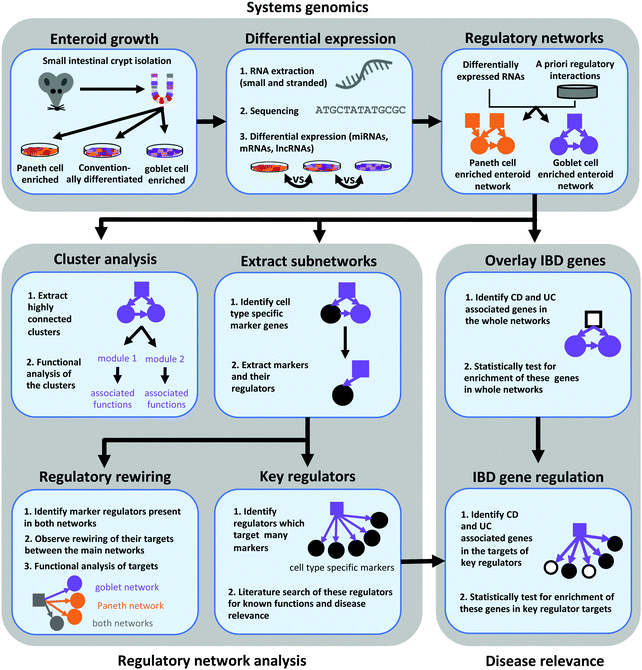 |
| Fig. 1 Schematic representation of the workflow used to infer and analyse regulatory network landscapes altered by Paneth cell and goblet cell enrichment of enteroids. PCeE/GCeE network – Paneth cell enriched enteroid/goblet cell enriched enteroid network; TF – transcription factor; lncRNA – long non-coding RNA; miRNA – microRNA; mRNA – messenger RNA; UC – ulcerative colitis; CD – Crohn's disease. | |
Results
Secretory lineages are over-represented in cell type enriched enteroids compared to conventionally differentiated controls
We generated 3D self-organising enteroid cultures in vitro from murine small intestinal crypts (Fig. S1, ESI†).18–20 In addition to conventionally differentiated enteroids, we generated enteroids enriched for Paneth cells and goblet cells using well-established and published protocols, presented in detail in the Methods.20,25 Bulk transcriptomics data was obtained from each set of enteroids to determine genes with differential expression resulting from enteroid skewing protocols. Differentially expressed genes were calculated by comparing the RNA expression levels (including protein coding genes, lncRNAs and miRNAs) of enteroids enriched for Paneth cells or goblet cells to those of conventionally differentiated enteroids. 4135 genes were differentially expressed (absolute log2 fold change ≥1 and false discovery rate ≤0.05) in the PCeE dataset, and 2889 were differentially expressed in the GCeE dataset (Fig. 2A–C and Table S1, ESI†). The larger number of differentially expressed genes (DEGs) in the PCeE data could be attributed to the highly specialised nature of Paneth cells.35,36 The majority of the DEGs were annotated as protein coding: 79% in the PCeE dataset and 84% in the GCeE dataset. In addition, we identified lncRNAs (PCeE, 11%; GCeE, 9%) and miRNAs (PCeE, 4%; GCeE, 2%) among the DEGs (Fig. 2B). Some of these DEGs were identified in both the PCeE and the GCeE datasets, exhibiting the same direction of change compared to the conventionally differentiated enteroid data. In total, 1363 genes were found upregulated in both the PCeE and the GCeE data, while 442 genes were found downregulated in both datasets (Fig. 2C). This result highlights considerable overlap between the results of skewing enteroids towards Paneth cells and goblet cells, and can be explained by the shared differentiation history and secretory function of both Paneth cells and goblet cells.
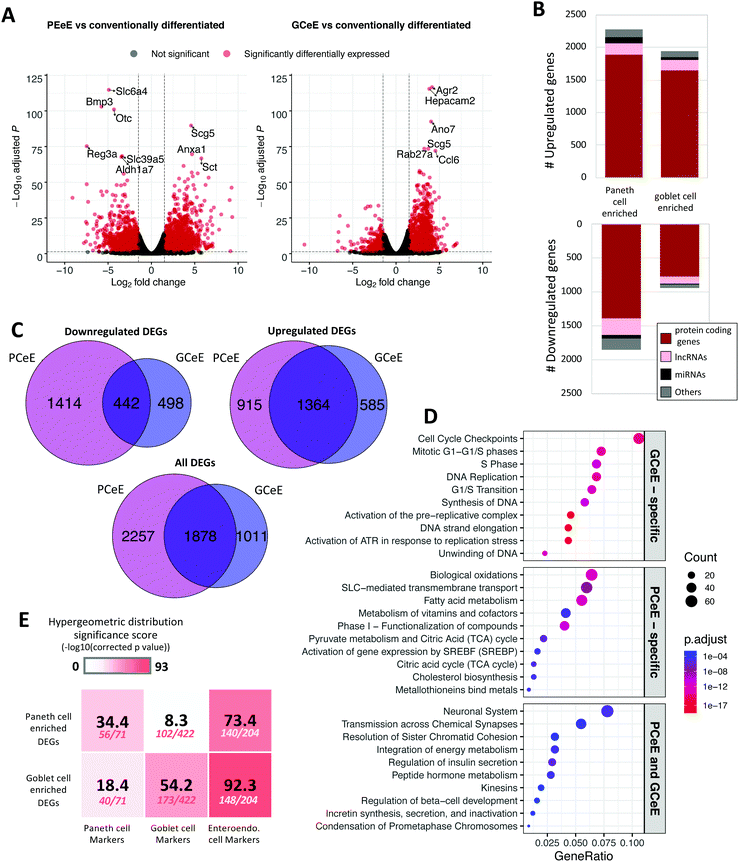 |
| Fig. 2 Differentially expressed genes in Paneth cell enriched enteroids (PCeEs) and goblet cell enriched enteroids (GCeEs) (compared to conventionally differentiated enteroids). (A) Volcano plots showing log2 fold change and adjusted p value for each gene following differential expression analysis of PCeEs (left) and GCeEs (right). Horizontal and vertical lines indicate the differential expression criteria cut offs (q value ≤ 0.05 and log2 fold change ≥|1|). (B) Number of differentially expressed genes (DEGs). miRNA = microRNA; lncRNA = long non-coding RNA; genes annotated as ‘other’ include pseudogenes and antisense genes. (C) Venn diagrams indicating the number of DEGs (passing the cut off criteria). (D) Top 10 Reactome pathways of the 50 most significant DEGs (by q value). (E) Enrichment of cell type specific marker genes in the DEG lists. Higher significance scores indicate greater enrichment. Number of markers in DEG list out of the total number of markers shown below significance score. Also see Tables S1 and S4 (ESI†). | |
Pathway analysis was employed to study functional associations of the DEGs (Fig. 2D). The PCeE-specific DEGs were associated with a number of metabolic pathways, including metabolism of vitamins and cofactors, pyruvate metabolism and citric acid (TCA) cycle and cholesterol biosynthesis. On the other hand, GCeE-specific DEGs were associated with the cell cycle through pathways such as cell cycle checkpoints, DNA replication and G1/S transition. Pathways associated with the shared DEGs included transmission across chemical synapses, integration of energy metabolism and a number of pathways linked to hormones. As hormone functions are characteristic of enteroendocrine cells, this analysis suggests that enteroendocrine cells are enriched in both the PCeEs and the GCeEs.
To validate the cell types present in the enteroids, the expression of five previously reported major cell type specific markers were investigated across the enteroids using transcript abundances and RNA differential expression results (Table S2 and Fig. S2, ESI†). The control enteroids and the cell type enriched enteroids expressed all five investigated markers: Lgr5 (stem cells), ChgA (enteroendocrine cells), Muc2 (goblet cells), Lyz1 (Paneth cells) and Vil1 (epithelial cells). We observed an upregulation of Muc2, Lyz1 and ChgA and a downregulation of Lgr5 in PCeEs and GCeEs compared to the control enteroids, confirming the more pronounced differentiated status of the enteroids. In addition, a number of Paneth cell specific antimicrobial peptide genes were differentially expressed in the PCeE dataset, including Ang4, Reg3γ, Pla2g2a and Defa2 (Table S3, ESI†). Some of these genes were also differentially expressed in the GCeE dataset but with smaller log fold change values, e.g. Lyz1 and Ang4. Conversely, a number of goblet cell mucin related genes (including Muc2 and Tff3) were differentially expressed in both datasets although all genes exhibited a smaller increase in the PCeEs (Table S2, ESI†). Therefore, using primary cell type specific markers, antimicrobial peptide genes and mucin-related genes, we show that the enteroids contain all major cell types, and that Paneth cells are most upregulated in the PCeEs, while goblet cells are most upregulated in the GCeEs. We also note that both differentiation methods resulted in increases of other secretory cell types as well.
To further investigate secretory cell type specific signatures of the enteroids, we measured enrichment of secretory lineages in the upregulated DEG lists using additional marker genes of Paneth cells, goblet cells and enteroendocrine cells. While all tests were significant (hypergeometric model, q value ≤ 0.05), we identified greater enrichment of Paneth cell markers in the PCeE DEG list and goblet markers in the GCeE DEG list (Fig. 2E). This confirms that both enteroid enrichment protocols were successful in increasing the proportion of their target cell type, but also increased proportions of other secretory lineages, albeit to a lesser extent (Table S4, ESI†). This observation confirms previous studies that these enteroid differentiation protocols result in enteroendocrine enrichment in addition to Paneth cell and goblet cell enrichment.25,28
In conclusion, we have used image-based validation, pathway analysis and marker gene investigation to show successful enrichment of target cell types in the PCeE's and GCeE's. We also highlighted an additional increase in other secretory lineages, particularly enteroendocrine cells, as a result of both enrichment protocols.
Reconstruction of regulatory networks altered by enteroid differentiation skewing
To gain an understanding of the regulatory changes occurring when enteroids development is skewed, we applied a network biology approach, and identified regulator–target relationships within the DEG lists. First, we generated a large network of non-specific molecular interactions known to occurr in mice, by collating lists of published data (Table S6, ESI†). The resulting network (termed the universal network) consisted of 1
383
897 unique regulatory interactions connecting 23
801 molecular entities. All interactions within the network represent one of the following types of regulation: TF–TG, TF–lncRNA, TF–miRNA, miRNA–mRNA or lncRNA–miRNA. TF–TGs and TF–lncRNAs make up the majority of the network at 77% and 11% of all interactions, respectively. Due to its non-specific nature, this universal network contains many interactions not relevant for the current biological context. In order to get a clearer and valid picture of regulatory interactions occurring in our enteroids, we used the universal network to annotate the PCeE DEGs and GCeE DEGs with regulatory connections. Combining these connections, we generated specific regulatory networks for PCeEs and GCeEs, where every node is a DEG and every interaction has been observed in mice previously.
In total, the PCeE network, generated using differential expression data from the PCeEs compared to the conventionally differentiated enteroids, contained 37
062 interactions connecting 208 unique regulators with 3023 unique targets (Fig. 3A and Item S1, ESI†). The GCeE network, generated using differential expression data from the GCeEs compared to the conventionally differentiated enteroids, contained 19
171 interactions connecting 124 unique regulators with 2095 unique targets (Fig. 3A and Item S1, ESI†). 15.7% of all interactions (8856 out of 56
234) were shared between the PCeE and GCeE networks, however the interacting molecular entities in these interactions (termed nodes) did not all exhibit the same direction of differential expression between the networks (comparing PCeE or the GCeE data to the conventionally differentiated enteroid data). In each of the enriched enteroid regulatory networks, a particular gene was represented (as a node in the network) only once, but may have been involved in multiple different interactions. In different interactions, a single node could act either as a regulator or as a target and in different molecular forms, for example, as a lncRNA in one interaction and as a target gene in another.
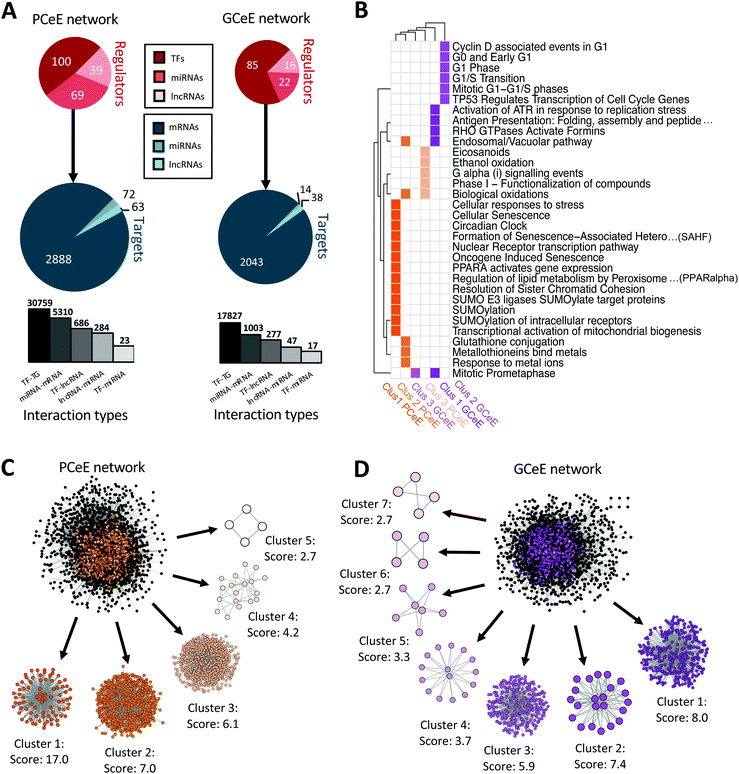 |
| Fig. 3 Summary and cluster analysis of regulatory network for Paneth cell enriched enteroid (PCeE) and goblet cell enriched enteroid (GCeE) datasets. (A) Summary of number of nodes and interactions in the whole PCeE (left) and GCeE (right) networks. Total number of each regulator type shown in red, number of each target type shown in blue. In the targets pie-chart, mRNAs represent protein coding genes and proteins, miRNAs represent miRNAs genes and lncRNAs represent lncRNA genes. Size of circles represents log10 (total unique regulators/targets). Bar chart represents the distribution of interaction types in the networks (log10 scale). (B) Heatplot of Reactome pathways significantly associated (q value ≤ 0.05) with each cluster of the PCeE (orange) and GCeE (purple) networks. Only the top 5 pathways shown for each group (or more where equal q values). Only the top 3 clusters had significantly associated pathways. Clusters labelled with rank and cell type and colours match the colour of the cluster shown in (C and D). (C and D) Visualisation of the PCeE and GCeE regulatory networks with their associated clusters. The cluster rank and score is given next to each cluster. Black nodes in the whole networks represent nodes which were not found in any cluster, whereas coloured nodes represent the cluster which they are part of. TF – transcription factor; miRNA – microRNA; lncRNA – long non-coding RNA. See Items S1, S2 and Table S5 (ESI†). | |
To further investigate the makeup of these networks, we employed cluster analysis to identify highly interconnected regions (possible regulatory modules) in the PCeE and GCeE regulatory networks. Using the MCODE software,37 we identified five distinct clusters in the PCeE network and seven distinct clusters in the GCeE networks. A total or 1314 nodes are present in the PCeE network clusters and 698 in the GCeE network clusters. Functional analysis identified Reactome pathways38 associated with each of the modules. Significant pathways (q value ≤ 0.05) were identified only for the highest ranked three modules from each network, with a total of 12 pathways shared between the PCeE and GCeE associated clusters (out of 32 associated with the PCeE clusters and 42 with the GCeE clusters) (Fig. 3B–D). Of particular note, the first cluster of the GCeE network has associations with the endosomal/vacuolar pathway and antigen presentation, the second cluster is associated with the cell cycle. Of the PCeE clusters, the first cluster is associated with a range of functions including nuclear receptor transcription pathway, regulation of lipid metabolism and senescence. The second is associated with response to metal ions and endosomal/vacuolar pathway and the third with G alpha (i) signalling events (Table S5, ESI†).
In conclusion, we have generated regulatory interaction networks, including transcriptional and post-transcriptional interactions, which illustrate the effect of skewing enteroid differentiation towards Paneth cell and goblet cell lineages.
Identification of potential cell type specific master regulators
Through pathway and marker analysis we predicted that our PCeE and GCeE datasets (i.e. DEG lists), and consequently our regulatory networks, contain signatures from the cell type of interest as well as additional noise from other secretory lineages. To focus specifically on the cell type specific elements of the networks, we used previously identified cell type specific markers to extract predicted Paneth cell and goblet cell regulators from our PCeE and GCeE networks. As cell type specific markers represent genes performing functions specific to a particular cell type, we expected that the regulators of these marker genes will have an important role in determining the function of said cell type. To identify these regulators, we extracted from the PCeE and GCeE networks, all relevant cell type specific markers and their direct regulators. These new networks were termed the Paneth cell subnetwork and goblet cell subnetwork respectively. The Paneth cell subnetwork contained 33 markers specific for Paneth cells with 62 possible regulators. The goblet cell subnework contained 150 markers with 63 possible regulators (Fig. 4 and Table S7, ESI†). Observing the ratio of regulators and markers, the Paneth cell subnetwork had, on average, 1.88 regulators for each marker. On the other hand, the goblet cell subnetwork exhibited only 0.42 regulators for each marker. The quantity of markers identified in each subnetwork (33 in the Paneth network and 150 in the goblet network) correlates with the number of marker genes identified by Haber et al.12 However, far fewer regulators were identified in the goblet cell subnetwork per marker than for the Paneth cell subnetwork. Whilst the underlying reason for this discrepancy is unknown, it could potentially be evidence of the complex regulatory environment required to integrate and respond to the arsenal of signals recognised by Paneth cells in comparison to goblet cells.35
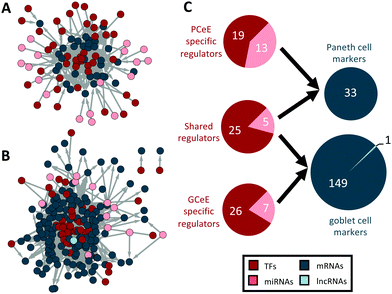 |
| Fig. 4 Regulator–marker subnetworks for Paneth cell and goblet cell datasets. (A and B) Paneth cell (A) and goblet cell (B) subnetworks. Nodes represent genes, transcription factors or RNAs and edges represent directed physical regulatory connections. Regulators are shown in red and pink. Cell type specific markers are shown in blue. (C) Summary of the number of nodes present in both the subnetworks. Paneth cell data above and goblet cell data below. Total number of each regulator type shown in red, number of each target type shown in blue. Regulators have been categorised based on their membership in the two subnetworks – shared regulators are present in both networks. In the targets pie-chart, mRNAs also represent protein coding genes. Size of circles represents log10 (total unique regulators/targets). TF – transcription factor; miRNA – microRNA; lncRNA – long non-coding RNA. | |
Of the 95 marker regulators, we identified approximately one-third (30/95) as present in both subnetworks (Fig. 4C). Given that the markers are different between the cell types, a regulator shared between the Paneth cell and goblet cell subnetworks must show an altered pattern of regulatory targeting in the two cell types. This phenomenon, referred to as regulatory rewiring, often results in functional differences of shared regulators in different environments39 – for example, in this case, between the Paneth cells and goblet cells.
Further investigation of the distinct regulator–marker interactions highlighted a gradient of regulator specificity. We generated matrices to visualise the markers controlled by each regulator in the goblet cell (Fig. 5A and C) and the Paneth cell (Fig. 5B) subnetworks. Each coloured square indicates that a marker (shown on the y-axis) is regulated by the corresponding regulator (shown on the x-axis). Squares are coloured blue if the associated regulator is shared between the Paneth cell and goblet cell subnetworks and orange if they are specific to one subnetwork. A collection of regulators (both subnetwork specific and shared) appear to regulate large proportions of the markers. For example, Ets1, Nr3c1 and Vdr regulate >50% of the markers in both the Paneth cell and the goblet cell subnetworks. Specific to the Paneth cell subnetwork, Cebpa, Jun, Nr1d1 and Rxra regulate >50% of the markers. Specific to the goblet cell subnetwork, Gfi1b and Myc regulate >50% of the markers. These regulators represent potential master regulators of differentiation or maintenance of the given cell types in the enriched enteroids. Referring back to the highly-interconnected clusters identified in the PCeE and GCeE networks (Fig. 3C and D), we find these predicted master regulators in different clusters. In the PCeE network, Cebpa, Nr1d1, Nr3c1 and Rxra are in cluster 1, Vdr is in cluster 2, Jun is in cluster 3 and Ets1 is unclustered. In the GCeE network, Ets1 and Myc are in cluster 1, Nr3c1 and Vdr are in cluster 2 and Gfi1b is in cluster 3. This suggests a wide range of central functions are carried out by this group of regulators, with possible divergence of roles between the Paneth cell and the goblet cell. In contrast to the predicted master regulators, regulators such as Mafk in the Paneth cell subnetwork and Spdef in the goblet cell subnetwork regulate only one marker. These regulators likely have more functionally specific roles.
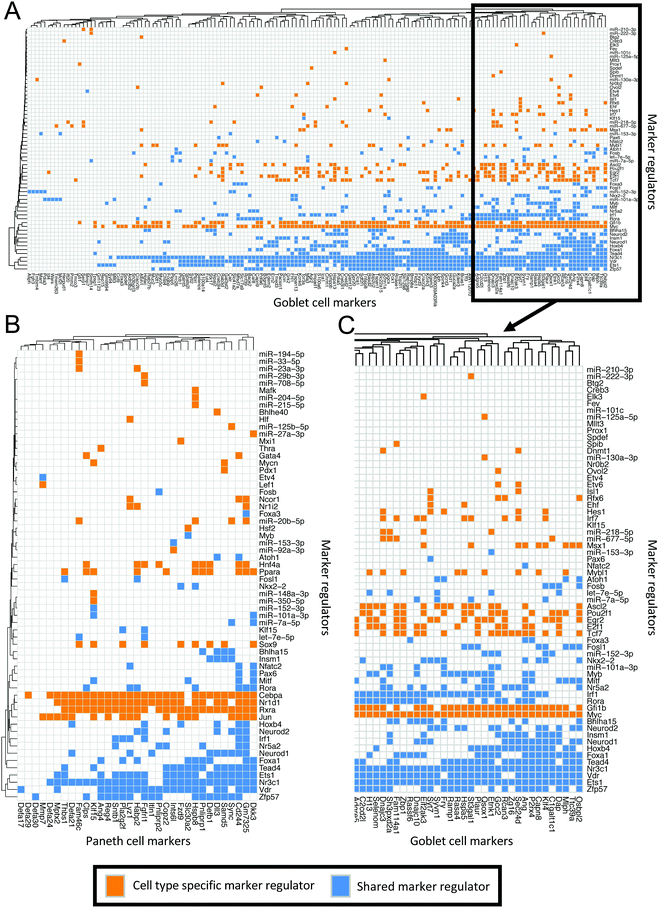 |
| Fig. 5 Matrices of interactions between markers and their regulators in the Paneth cell and goblet cell subnetworks. Regulators on y-axis, markers (regulator targets) on y-axis. Orange boxes indicate the interaction of a regulator and a marker where the regulator is only found in one of the two subnetworks. Blue boxes signify that the regulator is found in both the Paneth cell and the goblet cell subnetworks. (A) All goblet cell markers12 and their regulators in the goblet cell subnetwork. (B) All Paneth cell markers12 and their regulators in the Paneth cell subnetwork. (C) Sub-section of A showing the markers (and their regulators) which have the most regulatory connections. Interactions in Table S7 (ESI†). | |
Together, these results highlight potential regulators which likely play key roles in specification and maintenance of Paneth and goblet cells and their functions in cell type enriched enteroids.
Regulators of cell type specific markers exhibit rewiring between Paneth cells and goblet cells
Cell type specific markers, which carry out cell type specific functions, are inherently different between the Paneth cell and goblet cell subnetworks (mutually exclusive). Therefore, the regulators observed in both Paneth cell and goblet cell subnetworks (shared regulators) are expected to target different marker genes. To do this, the regulators must have different regulatory connections in the different cell types, a phenomenon termed ‘rewiring’.40 We extended the analysis to the original regulatory networks (PCeE and GCeE networks) to investigate whether any of the 30 identified shared regulators are rewired between the whole PCeE and the GCeE networks, and thus are highly likely to have different functions in the two types of enriched enteroids as well as between Paneth and goblet cells. To quantify rewiring of each of these regulators, we observed their targets in the PCeE and GCeE networks using the Cytoscape application, DyNet. DyNet assigns each regulator a rewiring score depending on how different their targets are between the two regulatory networks (Table S8, ESI†). Using these rewiring scores, we identified the five most rewired regulators (of 30) as Etv4, let-7e-5p, miR-151-3p, Myb and Rora. Functional enrichment analysis was carried out on the targets of these regulators to test whether the targets specific to the PCeE and GCeE networks have different functions (hypergeometric model, q value ≤ 0.1) (Table S9, ESI†). Across all five regulators the general trend indicated that targets specific to the PCeE network are associated with metabolism; targets specific to the GCeE network are associated with cell cycle and DNA repair. As pathway analysis carried out on the enteroid DEGs (Fig. 2D) identified the same phenomenon, this suggests that the rewired regulators could be key drivers of transcriptional changes during the skewing of enteroid differentiation towards Paneth cell or goblet cell lineages. In addition, given that the strongest signal of enriched enteroids represents their enriched cell type, we predict that these functions are key features of Paneth cells and goblet cells in the enteroids, and that the rewired regulators are important drivers of cell type specific functions.
Looking at the regulators in more detail, the GCeE specific targets of miR-151-3p, for example, are significantly enriched in functions relating to antigen presentation, cell junction organisation, Notch signalling and the calnexin/calreticulin cycle. None of these functions are enriched in the shared or PCeE targets. Of particular interest is the calnexin/calreticulin cycle, which is known to play an important role in ensuring proteins in the endoplasmic reticulum are correctly folded and assembled.41 Dysfunction of protein folding and the presence of endoplasmic reticulum stress are both associated with IBD.42–44 Therefore, we predict that miR-151-3p plays a role in the secretory pathway of goblet cells and could be an interesting target for IBD research. In addition, different functional profiles were also observed for the targets of Rora in the PCeE and GCeE regulatory networks: targets present in both networks are significantly associated with mitosis, whereas those specific to the PCeE network are associated with metabolism, protein localisation, nuclear receptor transcription pathway, circadian clock and hypoxia induced signalling. GCeE specific targets of Rora are connected to Notch signalling, cell cycle and signalling by Rho GTPases (associated with cell migration, adhesion and membrane trafficking) and interferon.
Altogether these observations show that some of the regulators of both Paneth cell and goblet cell marker genes have different targets (with different associated functions) between the PCeE and the GCeE networks. This suggests that regulatory rewiring occurrs between Paneth cell and goblet cell types.
Evaluating the disease relevance of the subnetwork specific master regulators
To investigate the function and relevance of the predicted master regulators in IBD, we carried out three analyses: (1) a literature search to check what is known about the identified master regulators; (2) an enrichment analysis to evaluate the disease relevant genes in the PCeE and GCeE networks and among the targets of the predicted master regulators; and finally, (3) a comparative analysis with human biopsy based single cell dataset to confirm the relevance of the data we identified PCeE and GCeE networks.
The literature search was carried out using the three groups of predicted master regulators: those specific to the Paneth cell markers (Cebpa, Jun, Nr1d1 and Rxra), those specific to the goblet cell markers (Gfi1b and Myc) and those which appear to regulate many of the markers of both cell types (Ets1, Nr3c1 and Vdr). We identified five genes (Ets1, Nr1d1, Rxra, Nr3c1 and Vdr) with associations to inflammation, autophagy and/or inflammatory bowel disease (IBD), as shown in Table 1. These genes correspond to 71% (5/7) of the Paneth cell associated master regulators and 60% (3/5) of the goblet cell associated master regulators. Interestingly, four of these genes (all apart from Ets1), encode nuclear hormone receptors.
Table 1 Literature associations relating to autophagy, inflammation and IBD for putative master regulators
Putative master regulator |
Autophagy/inflammation/IBD associations |
Ref. |
NR1D1 (REV-ERBa) |
Modulates autophagy and lysosome biogenesis in macrophages leading to antimycobacterial effects |
45
|
SNP rs12946510 which has associations to IBD, acts as a cis-eQTL for NR1D1 |
46
|
|
|
|
NR3C1 (glucocorticoid receptor) |
Associations with cellular proliferation and anti-inflammatory responses |
47
|
Exogenous glucocorticoids are heavily used as anti-inflammatory therapy for IBD |
48 and 49
|
ATG16L1, an autophagy related gene, was down-regulated in patients who do not respond to glucocorticoid treatment |
50 and 51
|
Transcriptionally regulates NFKβ1, a SNP affected gene in ulcerative colitis |
52 and 53
|
|
|
|
VDR (vitamin D receptor) |
Regulates autophagy in Paneth cells through ATG16L1 – dysfunction of autophagy in Paneth cells has been linked to Crohn's disease |
54 and 55
|
Induces antimicrobial gene expression in other cell lines |
56 and 57
|
Specific polymorphisms in the VDR genes have been connected to increased susceptibility to IDB |
58
|
A study looking at colonic biopsies of IBD patients observed reduced VDR expression compared to healthy biopsies |
59
|
Interacts with five SNP affected UC genes |
60 and 61
|
|
RXRa (retinoid X receptor alpha) |
Heterodimerizes with VDR (see above) |
62
|
|
ETS1 (ETS proto-oncogene 1) |
Important role in the development of hematopoietic cells and Th1 inflammatory responses |
63 and 64
|
Angiogenic factors in the VEGF-Ets-1 cascades are upregulated in UC and downregulated in CD |
65
|
IBD susceptibility gene |
66
|
Given the possible relationship between the identified master regulators and IBD, we tested the potential of the PCeE and GCeE regulatory networks to study the pathomechanisms of CD or UC. We checked for the presence of known CD or UC associated genes in the networks, using data from two studies of single nucleotide polymorphisms (SNPs)67,68 and one study of CD expression quantitative trait loci (eQTLs).69 Using hypergeometric significance tests, we found that the PCeE network was significantly enriched in all tested lists: genes with UC associated SNPs (13/47, p < 0.005), genes with CD associated SNPs (22/97, p < 0.005) and genes with CD associated eQTLs (290/1607, p < 0.0001) (Tables S10–S12, ESI†). On the other hand, we found that the GCeE network was significantly enriched in genes with UC associated SNPs (10/47, p < 0.005) but regarding CD, the genes with SNP associations were not significantly enriched (12/97, p = 0.11) and the genes with qQTL associations were enriched with a larger p value (p < 0.05) (Tables S10–S12, ESI†).
Next we investigated whether any of the genes with UC or CD associated SNPs acts as regulators in the PCeE and GCeE networks. Of the genes with CD associated SNPs, one acts as a regulator in each network. Similarly, two genes with UC associated SNPs act as regulators in the networks. Specifically regarding CD associated genes, in the PCeE network, the gene Dbp regulates Bik, which encodes the BCL2 interacting killer, a pro-apoptotic, death promoting protein. In the GCeE network, Notch2 regulates Notch3 and Hes1. Specifically, regarding UC associated genes, in the PCeE network, Hnf4a regulates 994 genes/RNAs including nine Paneth cell markers (Cd244a, Fgfrl1, Clps, Habp2, Hspb8, Pnliprp1/2, Defb1, Mymx) and one other gene with UC associated SNPs (Tnfsf15). Additionally, a gene with UC associated SNPs, Nr5a2, was found in both the PCeE and GCeE networks regulating 389 and 276 genes/RNAs respectively. In the PCeE network Nr5a2 targets include 6 Paneth cell markers (Cd244a, Copz2, Pnliprp1/2, Sntb1, Mymx). Ultimately, the large number of targets of these regulatory UC associated genes suggests they have wide ranging effects on the regulatory network of Paneth and goblet cells. To further establish the relevance of the inferred PCeE and GCeE networks, we also found an over-representation of drug target associated genes in both the PCeE and GCeE networks (2683/16223 and 1918/16223 respectively, p < 0.0001), highlighting their potential for the study of therapeutic implications (Table S12, ESI†).
To investigate the link between predicted master regulators and IBD, we observed whether the genes with UC and CD associated SNPs are regulated by the predicted master regulators in the PCeE and GCeE networks (Table S13, ESI†). Given that Paneth cell dysregulation is classically associated with CD and goblet cell dysregulation/depletion with UC,70 we focused this analysis only on these pairings, examining CD genes amongst targets of Paneth cell predicted master regulators, and UC genes amongst targets of goblet cell predicted master regulators. In the PCeE network, we found 21 (of 22) of the CD genes were regulated by at least one of the seven Paneth cell predicted master regulators, while the targets of these master regulators were significantly enriched with CD genes in the PCeE network (p < 0.001). Similarly, we observed that all 10 UC genes in the GCeE network were regulated by at least one of the five goblet cell predicted master regulators, while the targets of these master regulators were significantly enriched with UC genes (p < 0.005).
To confirm the relevance of these predicted master regulators in a human system, a similar analysis was carried out using goblet cell differentially expressed genes from a recent single cell study of human inflamed UC colon biopsies.13 Using the top 100 differentially expressed genes, following conversion to mouse Ensembl identifiers, 20 were found to be targeted by the predicted goblet cell master regulators in the GCeE network. This represents a significant enrichment amongst all master regulator targets (p < 0.005) (Table S13, ESI†).
Ultimately, by integrating functional annotations obtained through literature searches, we show that the Paneth cell and goblet cell regulatory networks contain genes with direct and indirect associations with IBD. Furthermore, we find that the PCEe and GCeE networks and the targets of predicted master regulators are enriched with IBD associated genes – this finding is corroborated using human single cell data from UC colon biopsies. Consequently, these networks and the workflow to reconstruct and analyse them have great potential for the study of IBD pathomechanisms in specific intestinal cell types.
Discussion
By generating and integrating cell type enriched enteroid RNAseq datasets with regulatory networks, we characterised the regulatory environment altered by differentiation skewing. Through focusing on cell type specific markers, we used the regulatory networks to predict master regulators of Paneth cells and goblet cells and to highlight the role of regulatory rewiring in cell differentiation. Given the relevance of Paneth cell and goblet cell dysfunction in IBD, future application of cell type enriched enteroids combined with our network analysis workflow can be used to disentangle multifactorial mechanisms of IBD.
Analysis using known cell type specific markers confirmed that skewing differentiation of enteroids towards Paneth cells or goblet cells results in an increase in the target cell signatures at the transcriptomics level. In addition, signatures of enteroendocrine cells were increased in both differentiation protocols, albeit at a lower amount than the target cell type. This finding correlates with previous investigations at both the transcriptomic and proteomic levels25,27–29 and is likely due to the shared differentiation pathways of these secretory cells. Nevertheless, as enteroids contain a mixed population of cell types by nature and because intercellular communication is key to a functioning epithelium,19,71 the increased proportion of non-targeted secretory lineages should not be an issue for the application of these models to research. In fact, the enrichment of specific cell types is beneficial for enteroid-based research to increase the signal originating from a specific population of cells and to provide a larger population of cells of interest for downstream single cell analysis of enteroids, which is particularly beneficial when studying rare populations such as Paneth cells. This is valuable due to the lack of in vitro models for long-term culture of non-self-renewing small intestinal epithelial cells.72,73 Specifically, the comparison of ‘omics data from a cell type enriched enteroid to a conventionally differentiated enteroid enables generation of cell type signatures with more specificity than can be obtained otherwise – except through single cell sequencing. Single cell sequencing, however, comes at a greater financial cost and provides lower coverage which can be problematic for rare cell types and lowly expressed RNAs. Furthermore, a number of previous studies have shown that these cell type enriched enteroid models, which offer a simplified and manipulatable version of the intestinal environment, are useful for the investigation of health and disease related processes.26,28,29 It must be considered, however, that through applying chemical inhibitors to enteroids to enrich particular cell types, we may observe changes in gene expression which are related to the direct effects of the inhibitor but not to differentiation. Due to the nature of the inhibitors, it would be challenging to separate these effects. For example, CHIR99021, which is used for enriching Paneth cells, is a direct inhibitor of glycogen synthase kinase 3 (GSK-3). GSK-3 has a role in many cellular pathways including cellular proliferation and glucose regulation.74
Using a priori molecular interaction knowledge, we annotated differentially expressed genes (cell type enriched enteroids vs. conventionally differentiated enteroids) with regulatory connections. Collecting all connections together generated regulatory networks for the Paneth cell enriched enteroid (PCeE) and goblet cell enriched enteroid (GCeE) datasets. This approach to collating networks (regulatory or otherwise) has been used for a wide variety of research aims, such as the identification of genes functioning in a variety of diseases,75,76 the prioritisation of therapeutic targets77 and for a more general understanding of gene regulation in biological systems.78,79 The application of prior knowledge avoids the need for reverse engineering/inference of regulatory network connections, which is time consuming, computationally expensive and requires large quantities of high quality data.80 To investigate the substructure and functional associations of the generated PCeE and GCeE regulatory networks we applied a clustering approach. The identified clusters represent collections of highly interconnected nodes, which likely form regulatory modules. Functional analysis confirmed distinct functional associations between the clusters as well as between the networks. The observation that less than half of the network nodes exist in clusters is consistent with the view that regulatory networks are hierarchical and scale free with most genes exhibiting low pleiotropy.81,82
Given the observed additional increase in secretory lineages based on the DEGs of the enriched organoids, we chose to use cell type specific markers to extract interactions specific to Paneth cells and goblet cells from the generated regulatory networks. This enables further enrichment of Paneth cell and goblet cell signatures and reduction in noise in the networks due to the presence of other cell types in the enteroids. Using this approach, we identified possible regulators of cell type specific functions in Paneth cells and goblet cells. Some of these regulators were predicted to be important in both cell types, but exhibited differential targeting patterns between the PCeE and the GCeE networks, indicating rewiring of regulators between the cell types. This highlights apparent redundancy and/or cooperation of regulators which control similar cell type specific functions and shows the potential importance of regulatory rewiring in the evolution of cell type specific pathways and functions, something which has been shown previously to occurr.83,84 Functional analysis of the targets of the most rewired regulators (Etv4, let-7e-5p, miR-151-3p, Myb and Rora) highlights an overrepresentation of metabolism associated targets in the PCeE network and cell cycle associated targets in the GCeE network. A similar result was observed when functional analysis was carried out on genes with significantly different expression levels between the cell type enriched enteroids and the conventionally differentiated enteroids (Fig. 2B). This suggests that transcriptional changes during the skewing of enteroid differentiation could be driven by rewired regulators and that these functions are key features of Paneth cells and goblet cells in the enteroids. The latter is supported by current understanding that Paneth cells rely on high levels of protein and lipid biosynthesis for secretory functions,85 and they play an important role in metabolically supporting stem cells.86 Additionally, as terminally-differentiated cells do not undergo cell division, this result suggests that enteroid goblet cell signatures are derived from a large population of semi-differentiated goblet-like cells, a phenomenon previously observed in tissue sample based studies.13,87 Extension of our workflow to single cell sequencing of enteroid cells could validate these findings by providing greater cell type specificity. However, these techniques pose further technical and economic challenges.88,89 Specifically, a large number of organoids must be sequenced to mitigate cellular complexity and batch heterogeneity and powerful, reproducible and accurate computational pipelines are required to analyse such data.90
We predicted key regulators involved in differentiation or maintenance of Paneth cells and goblet cells in the enteroids: Cebpa, Jun, Nr1d1 and Rxra specific to Paneth cells, Gfi1b and Myc specific for goblet cells and Ets1, Nr3c1 and Vdr shared between them. Validation of these regulators poses significant challenges due to their wide expression and broad function range. If the master regulators are controlling differentiation as opposed to cell function maintenance, evaluating lineage arrest or delay can be carried out using a gene knockout or knock down. However the effects of pleiotropy will significantly hamper the results and such a study would require significant follow-up studies. On the other hand, if key regulators were predicted by applying the presented computational workflow to condition-specific organoids compared to control organoids (e.g. drug treated organoids vs. non-treated organoids), the validation would be much simpler. Literature investigation highlighted that many of the predicted master regulators, particularly those associated with Paneth cells, have connections to autophagy, inflammation and IBD (Table 1). While some of these associations are related to different cell types, we assume that if a gene can contribute to a specific function in one cell type it may also contribute in another. This finding suggests that dysregulation of key cell master regulators could contribute to IBD.
To further investigate this finding, we identified Crohn's disease (CD) and ulcerative colitis (UC) genes in the PCeE and GCeE networks. We found that CD associated genes are more strongly associated with the PCeE network than the GCeE network. Given that Paneth cell dysfunction is classically associated with CD, this finding highlights the relevance of the generated networks to the in vivo situation. In the PCeE network one SNP associated CD gene, Dbp, acts as a regulator. Dbp, encoding the D site binding protein, regulates Bik, which encodes the BCL2 interacting killer, a pro-apoptotic, death promoting protein. Interestingly, rate of apoptosis has been implicated in IBD disease mechanisms91 and has been associated with IBD drug response.92 Therefore, this finding highlights a possible regulatory connection between CD susceptibility genes and IBD pathology on a Paneth cell specific level. In the GCeE network, the SNP associated CD gene Notch2 acts as a regulator for Notch3 and Hes1. It has been previously demonstrated that this pathway can block glucocorticoid resistance in T-cell acute lymphoblastic leukaemia via NR3C1 (predicted master regulator).93 This is relevant to IBD given that glucocorticoids are a common treatment for IBD patients.49 Furthermore, this pathway has been previously associated with goblet cell depletion in humans94 commonly observed in IBD patients. Furthermore, we identified a significant enrichment of UC associated genes in both the PCeE and GCeE networks. The majority of UC associated genes identified in the networks (9/14) were present in both, suggesting that genetic susceptibilities of UC do not have a Paneth cell or goblet cell specific effect. Two of the identified UC associated genes act as regulators in the networks (Nr5a2 and Hnf4a), targeting hundreds of genes and thus suggesting a broad ranging effect on the networks. Building on the identified literature associations of predicted master regulators, we found that the targets of Paneth cell master regulators are enriched with CD associated genes, and the targets of the goblet cell master regulators are enriched with UC associated genes. This finding was further illustrated using UC associated goblet cell genes from a human biopsy study,13 highlighting the relevance of these findings in a human system. Ultimately, the observation of IBD susceptibility genes in the regulatory networks of these enteroids highlights possible application of this model system to study disease regulation in specific intestinal cell types, through understanding specific mechanistic pathways.
We have shown how network biology techniques can be applied to generate interaction networks representing the change in regulatory environments between two sets of enteroids. Here we presented this workflow, and by using transcriptomics data we characterised the effect of Paneth cell and goblet cell differentiation skewing protocols on enteroids. However. the described workflow could be applied to a variety of ‘omics datasets and enteroid conditions. For example, to test the response of enteroids to external stimuli, such as bacteria, and on enteroids grown from human-derived biopsies, enabling patient-specific experiments. The application of further ‘omics data-types to the described approach could generate a more holistic view of cellular molecular mechanisms, including the ability to observe post-translational regulation. In this study, we integrated miRNA and lncRNA expression datasets, in addition to mRNA data, as these molecules have been shown to perform critical regulatory and mediatory functions in maintaining intestinal homeostasis.46,68,95 However, only small proportions of the generated PCeE and the GCeE networks contained miRNA and lncRNA interactions (Fig. 2B), due to lack of published interaction information, particularly from murine studies. Both the application of human enteroid data and the future publication of high-throughput interaction studies involving miRNAs and lncRNAs will improve the ability to study such interactions. Nevertheless, these network approaches are beneficial for contextualising gene lists through annotating relevant signalling and regulatory pathways31 and we can use them to represent and analyse current biological knowledge, to generate hypotheses and to guide further research.
In conclusion, we described an integrative systems biology workflow to compare regulatory landscapes between enteroids from different conditions, incorporating information on transcriptional and post-transcriptional regulation. We applied the workflow to compare Paneth cell and goblet cell enriched enteroids to conventionally differentiated enteroids and predicted Paneth cell and goblet cell specific regulators, which could provide potential targets for further study of IBD mechanisms. Application of this workflow to patient derived organoids, genetic knockout and/or microbially challenged enteroids, alongside appropriate validation and single cell sequencing if available, will aid discovery of key regulators and signalling pathways of healthy and disease associated intestinal cell types.
Methods
Animal handling
C57BL/6J mice of both sexes were used for enteroid generation. All animals were maintained in accordance with the Animals (Scientific Procedures) Act 1986 (ASPA).
Small intestinal organoid culture
Murine enteroids were generated as described previously.18,20,29 Briefly, the entire small intestine was opened longitudinally, washed in cold PBS then cut into ∼5 mm pieces. The intestinal fragments were incubated in 30 mM EDTA/PBS for 5 minutes, transferred to PBS for shaking, then returned to EDTA for 5 minutes. This process was repeated until five fractions had been generated. The PBS supernatant fractions were inspected for released crypts. The crypt suspensions were passed through a 70 μm filter to remove any villus fragments, then centrifuged at 300 × g for 5 minutes. Pellets were resuspended in 200 μl phenol-red free Matrigel (Corning), seeded in small domes in 24-well plates and incubated at 37 °C for 20 minutes to allow Matrigel to polymerise. Enteroid media containing EGF, Noggin and R-spondin (ENR; (18)) was then overlaid. Enteroids were generated from three separate animals for each condition, generating three biological replicates.
To chemically induce differentiation, on days two, five and seven post-crypt isolation, ENR media was changed to include additional factors for each cell type specific condition: 3 μM CHIR99021 (Tocris) and 10 μM DAPT (Tocris) [Paneth cells]; 2 μM IWP-2 (Tocris) and 10 μM DAPT [goblet and enteroendocrine cells].25
Small intestinal organoid immunofluorescence
On day eight post-crypt isolation, enteroids were fixed with 4% paraformaldehyde (PFA; Sigma-Aldrich) for 1 hour at 4 °C prior to permeabilization with 0.1% Triton X-100 (Sigma-Aldrich) and incubated in blocking buffer containing 10% goat serum (Sigma-Aldrich). Immunostaining was performed overnight at 4 °C using primary antibodies: mouse anti-E-cadherin (BD Transduction Laboratories), rabbit anti-muc2 (Santa Cruz) and rabbit anti-lysozyme (Dako), followed by Alexa Fluor-488 and -594 conjugated secondary antibodies (ThermoFisher Scientific). DNA was stained with DAPI (Molecular Probes). Images were acquired using a fluorescence microscope (Axioimager.M2, equipped with a Plan-Apochromat 63×/1.4 oil immersion objective) and analysed using ImageJ/FIJI V1.51.
RNA extraction
On day eight post-crypt isolation (allowing optimal cell type-enrichment as previously shown),25 enteroids were extracted from Matrigel (Corning, 356237) using Cell Recovery Solution (BD Bioscience, 354253), rinsed in PBS and RNA was extracted using miRCURY RNA Isolation Tissue Kit (Exiqon, 300115) according to the manufacturer's protocol.
Stranded RNA library preparation
The enteroid transcriptomics libraries were constructed using the NEXTflex™ Rapid Directional RNA-Seq Kit (PerkinElmer, 5138-07) using the polyA pull down beads from Illumina TruSeq RNA v2 library construction kit (Illumina, RS-122-2001) with the NEXTflex™ DNA Barcodes – 48 (PerkinElmer, 514104) diluted to 6 μM. The library preparation involved an initial QC of the RNA using Qubit DNA (Life technologies, Q32854) and RNA (Life technologies, Q32852) assays as well as a quality check using the PerkinElmer GX with the RNA assay (CLS960010). Ligated products were subjected to a bead-based size selection using Beckman Coulter XP beads (A63880). As well as performing a size selection this process removed the majority of unligated adapters. Prior to hybridisation to the flow cell the samples were amplified to enrich for DNA fragments with adapter molecules on both ends and to amplify the amount of DNA in the library. The strand that was sequenced is the cDNA strand. The insert size of the libraries was verified by running an aliquot of the DNA library on a PerkinElmer GX using the High Sensitivity DNA chip (PerkinElmer, CLS760672) and the concentration was determined by using a High Sensitivity Qubit assay and q-PCR. Libraries were then equimolar pooled and checked by qPCR to ensure the libraries had the necessary sequencing adapters ligated.
Small RNA library preparation
The small RNA libraries were made using the TruSeq Small RNA Library Prep Kits (Illumina, 15004197), six-base indexes distinguish samples and allow multiplexed sequencing and analysis using 48 unique indexes (Set A: indexes 1–12 (Illumina, RS-200-0012)), Set B: indexes 13–24 (Illumina, RS-200-0024), Set C: indexes 25–36 (Illumina, RS-200-0036), Set D: indices 37–48 (Illumina, RS-200-0048)) (TruSeq Small RNA Library Prep Kit Reference Guide (Illumina, 15004197 Rev.G)). The TruSeq Small RNA Library Prep Kit protocol is optimised for an input of 1 μg of total RNA in 5 μl nuclease-free water or previously isolated microRNA may be used as starting material (minimum of 10–50 ng of purified small RNA). Total RNA is quantified using the Qubit RNA HS Assay kit (ThermoFisher, Q32852) and quality of the RNA is established using the Bioanalyzer RNA Nano kit (Agilent Technologies, 5067-1511), it is recommended that RNA with an RNA Integrity Number (RIN) value ≥8 is used for these libraries as samples with degraded mRNA are also likely to contain degraded small RNA.
Library purification combines using BluePippin cassettes (Sage Science Pippin Prep 3% Cassettes Dye-Free (CDF3010), set to collection mode range 125–160 bp) to extract the library molecules followed by a concentration step (Qiagen MinElute PCR Purification, 28004) to produce libraries ready for sequencing. Library concentration and size are established using HS DNA Qubit and HS DNA Bioanalyser. The resulting libraries were then equimolar pooled and qPCR was performed on the pool prior to clustering.
Stranded RNA sequencing on HiSeq 100PE
The final pool was quantified using a KAPA Library Quant Kit (Roche, 07960140001), denatured in NaOH and combined with HT1 plus a 1% PhiX spike at a final running concentration of 10 pM. The flow-cell was clustered using HiSeq PE Cluster Kit v3 (Illumina, PE-401-3001) utilising the Illumina PE HiSeq Cluster Kit V3 cBot recipe V8.0 method on the Illumina cBot. Following the clustering procedure, the flow-cell was loaded onto the Illumina HiSeq2000 instrument following the manufacturer's instructions with a 101 cycle paired reads and a 7-cycle index read. The sequencing chemistry used was HiSeq SBS Kit v3 (Illumina, FC-401-3001) with HiSeq Control Software 2.2.68 and RTA 1.18.66.3. Reads in bcl format were demultiplexed based on the 6 bp Illumina index by CASAVA 1.8, allowing for a one base-pair mismatch per library, and converted to FASTQ format by bcl2fastq.
Small RNA sequencing on HiSeq rapid 50SE
The final pool was quantified using a KAPA Library Quant Kit (Roche, 07960140001), denatured in NaOH and combined with HT1 plus a PhiX spike at a final running concentration of 20 pM. The libraries were hybridized to the flow-cell using TruSeq Rapid Duo cBot Sample Loading Kit (Illumina, CT-403-2001), utilising the Illumina RR_TemplateHyb_FirstExt_VR method on the Illumina cBot. The flow-cell was loaded onto the Illumina HiSeq2500 instrument following the manufacturer's instructions with a 51-cycle single read and a 7 cycle index read. The sequencing chemistry used was HiSeq SBS Rapid Kit v2 (Illumina, FC-402-4022) with a single read cluster kit (Illumina, GD-402-4002), HiSeq Control Software 2.2.68 and RTA 1.18.66.3. Reads in bcl format were demultiplexed based on the 6 bp Illumina index by CASAVA 1.8, allowing for a one base-pair mismatch per library, and converted to FASTQ format by bcl2fastq.
Differentially expressed transcripts
The quality of stranded reads was assessed by FastQC software (version 0.11.4).96 All reads coming from technical repeats were concatenated together and aligned (in stranded mode, i.e. with ‘–rna-strandness RF’ flag) using HISAT aligner (version 2.0.5).97 Subsequently, a reference-based de novo transcriptome assembly was carried out for each biological repeat and merged together using StringTie (version 1.3.2) with following parameters: minimum transcript length of 200 nucleotides, minimum FPKM of 0.1 and minimum TPM of 0.1.98,99 Coding potential of each novel transcript was determined with CPC (version 0.9.2) and CPAT (version 1.2.2).100,101 From the novel transcripts, only non-coding transcripts (as predicted by both tools) were included in final GTF file. Gene and transcript abundances were estimated with kallisto (version 0.43.0).102 Sleuth (version 0.28.1) R library was used to perform differential gene expression.103 mRNAs and lncRNAs with an absolute log2 fold change of 1 and q value ≤0.05 were considered to be differentially expressed.
The small RNA reads were analysed using the sRNAbench tool within the sRNAtoolbox suite of tools.104 The barcodes from the 5′ end and adapter sequences from the 3′ end were removed respectively. Zero mismatches were allowed in detecting the adapter sequences with a minimum adapter length set at 10. Only reads with a minimum length of 16 and a read-count of 2 were considered for further analysis. The mice miRNA collection was downloaded from miRBase version 21.105 The trimmed and length filtered reads were then mapped to the mature version of the miRBase miRNAs in addition to the annotated version of the mouse genome (version mm10). No mismatches were allowed for the mapping. A seed length of 20 was used for the alignment with a maximum number of multiple mappings set at 10. Read-counts normalised after multiple-mapping were calculated for all the libraries. The multiple-mapping normalised read-counts from the corresponding cell type enriched enteroids were compared against the conventionally differentiated enteroids to identify differentially expressed miRNAs in a pair-wise manner using edgeR.106 miRNAs with an absolute log2 fold change of 1 and false discovery rate ≤0.05 were considered to be differentially expressed.
Differentially expressed genes were grouped by their presence in the PCeE dataset, the GCeE dataset or in both. Each group of differentially expressed genes was tested for functional enrichment (hypergeometric model, q value ≤ 0.1) based on Reactome and KEGG annotations using the ReactomePA R package38,107–109 following conversion from mouse to human identifiers using Inparanoid (v8).110,111
Enrichment of marker genes
Cell type specific marker gene lists were obtained a mouse single cell sequencing survey.12 The cell type specific signature genes for Paneth, goblet and enteroendocrine cell types were obtained from the droplet-based and the plate-based methods. Gene symbols were converted to Ensembl gene IDs using bioDBnet db2db.112
Hypergeometric distribution testing was carried out using a custom R script to measure enrichment of cell type specific marker genes in the differentially upregulated gene sets. To standardise the universal dataset, only markers which are present in the output of the Wald test (genes with variance greater than zero among samples) were used. Similarly, to enable fair comparisons, only differentially expressed protein coding genes and documented lncRNAs were used from the DEG lists, as was surveyed in the cell type specific marker paper. Bonferroni correction was applied to the hypergeometric distribution p values to account for multiple testing and significance scores were calculated using −log10 (corrected p value). For the mapping of marker genes to the interaction networks, no filters were applied.
Reconstruction of molecular networks
Mice regulatory networks containing directed regulatory layers were retrieved from multiple databases (Table S6, ESI†): miRNA–mRNA (i.e., miRNAs regulating mRNAs) and lncRNA–miRNA (i.e., lncRNAs regulating miRNAs) interactions were downloaded from TarBase v7.0113 and LncBase v2.0,114 respectively. Only miRNA–mRNA and lncRNA–miRNA interactions determined using HITS-CLIP115 experiments were considered. Regulatory interactions between transcription factors (TFs) and miRNAs (i.e. TFs regulating miRNAs) were retrieved from TransmiR v1.2,116 GTRD117 and TRRUST v2.118,119 Co-expression based inferences were ignored from all the above resources. Transcriptional regulatory interactions (i.e., TFs regulating target genes) were inferred using data from ORegAnno 3.0,61 GTRD and TRUSST. In cases where transcriptional regulatory interactions are derived from high-throughput datasets such as ChIP-seq, we attributed the regulatory interaction elicited by the bound transcription factor to genes which lie within a 10 kb window on either side of the ChIP-seq peak (ORegAnno) or meta-cluster (in the case of GTRD). TF–lncRNA interactions (i.e., TFs regulating lncRNAs) were also inferred based on the ChIP-seq binding profiles represented by meta-clusters in GTRD. We used only TF–lncRNA interactions within intergenic lncRNAs to avoid assigning false regulatory interactions due to the high number of instances where the lncRNAs overlap with protein-coding genes. In addition, no overlaps were allowed between the coordinates of the ChIP-seq peaks/meta-clusters and any gene annotation. Only if the first annotation feature within a 10 kb genomic window downstream to the ChIP-seq peak/meta-cluster was designated as an intergenic lncRNA, a regulatory interaction between the TF and the lncRNA was assigned. Bedtools120 was used for the custom analyses to look for overlaps between coordinates. All the nodes in the collected interactions were represented by their Ensembl gene IDs for standardization. A summary of the interactions collected from each resource and the quality control criteria applied is given in Table S6 (ESI†).
To generate PCeE and GCeE regulatory networks, interactions in this collated universal network were filtered using the differential expression data (Fig. 1). The assumption was made that if both nodes of a particular interaction were expressed in the RNAseq data, the interaction is possible. Furthermore, to filter for the interactions of prime interest, only nodes which were differentially expressed and their associated interactors were included in the regulatory networks.
Cluster analysis
Clusters of highly interconnected regions within the PCeE and GCeE regulatory networks were identified using the MCODE plugin within Cytoscape.37,121 Default settings were applied: degree cutoff = 2, haircut = true, node score cutoff = 0.2, k-core = 2 and max depth = 100. Clusters were visualised in Cytoscape.
The nodes of each cluster were tested for functional enrichment (hypergeometric model, q value ≤ 0.05) based on Reactome annotations using the ReactomePA R package38,107–109 following conversion from mouse to human identifiers using Inparanoid (v8).110,111 Cases where the number of nodes associated with a pathway <5 were considered not significant regardless of the q value. The top 5 significant Reactome pathways associated with each cluster were visualised using a heatplot generated in R (Fig. 3B). More than 5 pathways were visualised, where multiple Reactome pathways had equal q values.
Master regulator analysis
To identify potential master regulators of the Paneth cell and the goblet cell types, the upstream regulators of cell type specific markers (from ref. 12) were investigated. To do this, all markers were mapped to the relevant networks then subnetworks were extracted consisting of markers and their regulators (Table S7, ESI†).
Regulatory rewiring analysis
To calculate rewiring scores for regulators, sub-networks were extracted (from the PCeE and GCeE regulatory networks) containing just the regulator of interest and its downstream targets. For each regulator of interest, the subnetworks from the PCeE and GCeE networks were compared using the Cytoscape app DyNet.121,122 The degree corrected Dn score was extracted for each regulator and used to quantify rewiring of the regulator's downstream targets between the PCeE and GCeE regulatory networks. Functional analysis was carried out on the targets of the top five most rewired regulators. For each regulator, the targets were classified based on whether they are present in only the PCeE network, only the GCeE network or in both networks. Each group of targets was tested for functional enrichment (hypergeometric model, q value ≤ 0.1) based on Reactome and KEGG annotations using the ReactomePA R package38,107–109 following conversion from mouse to human identifiers using Inparanoid (v8).110,111
IBD and drug target associated genes
Genes associated with UC and CD based on single nucleotide polymorphisms were obtained from two studies.67,68 Additionally, the top 100 differentially expressed genes were obtained from goblet cell analysis of inflamed UC vs. healthy human colonic tissue from ref. 13. Genes were converted to Mouse Ensembl identifiers using Inparanoid (v8) and bioDBnet db2db.110–112 Additionally, to enable hypergeometric significant testing with the universal network as the background, only UC and CD genes present in the universal network are included in the analyses. eQTL datasets for CD were retrieved from ref. 69 while the list of targets related to drug-interactions was downloaded from ref. 123.
Quantification and statistical analysis
Statistical parameters including the exact value of n and statistical significance are reported in the figures and figure legends. n represents the number of enteroid biological replicates generated. Where relevant, data is judged to be statistically significant when Bonferroni corrected p value ≤0.01. Genes with absolute log2 fold change of ≥|1| and false discovery rate ≤0.05 were considered to be differentially expressed. Based on principal component analysis of transcript expression, one biological replicate from the Paneth cell enriched enteroids was identified as an outlier and removed (Fig. S3, ESI†). Where stated, the hypergeometric distribution model was used to calculate significance using R.
Data and software availability
Small and stranded RNA-seq data has been deposited in the European Nucleotide Archive (ENA) with accession numbers PRJEB32354 and PRJEB32366 respectively. Scripts to analyse the differentially expressed genes are available on GitHub: https://github.com/korcsmarosgroup/organoid_regulatory_networks.
Funding
This work was supported by a fellowship to TK in computational biology at the Earlham Institute (Norwich, UK) in partnership with the Quadram Institute (Norwich, UK), and strategically supported by the Biotechnological and Biosciences Research Council, UK grants (BB/J004529/1, BB/P016774/1 and BB/CSP17270/1). SRC, LH, TWi and TK were also funded by a BBSRC ISP grant for Gut Microbes and Health BB/R012490/1 and its constituent project(s), BBS/E/F/000PR10353 and BBS/E/F/000PR10355. ZM was supported by a PhD studentship from Norwich Medical School. PP was supported by the BBSRC grant BB/J01754X/1. AT and MO were supported by the BBSRC Norwich Research Park Biosciences Doctoral Training Partnership (grant BB/M011216/1). TWr and WH were supported by an MRC award (MR/P026028/1). Next-generation sequencing and library construction was delivered via the BBSRC National Capability in Genomics and Single Cell (BB/CCG1720/1) at Earlham Institute by members of the Genomics Pipelines Group.
Author contributions
EJJ, ZJM and IH worked on the transcriptomics pipeline development; AT, PS, TWr, JB and MO designed and carried out specific network analysis tasks; SRC, UM, PPP and TWi established and supervised the organoid enrichment work; LJH and FDP supervised the experimental and transcriptomics analysis work; WH, TWi and TK designed the study, supervised the experiments and the analysis. AT, PS, ZM, EJ, IH and TK wrote the manuscript.
Conflicts of interest
None.
Acknowledgements
The authors are grateful for the helpful discussions to the past and present members and visitors of the Wileman, Haerty and Korcsmaros groups.
References
- K. Zhang, M. W. Hornef and A. Dupont, The intestinal epithelium as guardian of gut barrier integrity, Cell. Microbiol., 2015, 17(11), 1561–1569 CrossRef CAS PubMed.
- R. Okumura and K. Takeda, Roles of intestinal epithelial cells in the maintenance of gut homeostasis, Exp. Mol. Med., 2017, 49(5), e338 CrossRef CAS PubMed.
- F. Gerbe and P. Jay, Intestinal tuft cells: epithelial sentinels linking luminal cues to the immune system, Mucosal Immunol., 2016, 9(6), 1353–1359 CrossRef CAS PubMed.
- B. A. Duerkop, S. Vaishnava and L. V. Hooper, Immune responses to the microbiota at the intestinal mucosal surface, Immunity, 2009, 31(3), 368–376 CrossRef CAS PubMed.
- M. Mokry, S. Middendorp, C. L. Wiegerinck, M. Witte, H. Teunissen and C. A. Meddens,
et al., Many inflammatory bowel disease risk loci include regions that regulate gene expression in immune cells and the intestinal epithelium, Gastroenterology, 2014, 146(4), 1040–1047 CrossRef CAS PubMed.
- C. L. Bevins and N. H. Salzman, Paneth cells, antimicrobial peptides and maintenance of intestinal homeostasis, Nat. Rev. Microbiol., 2011, 9(5), 356–368 CrossRef CAS PubMed.
- Y. S. Kim and S. B. Ho, Intestinal goblet cells and mucins in health and disease: recent insights and progress, Curr. Gastroenterol. Rep., 2010, 12(5), 319–330 CrossRef PubMed.
- M. Gersemann, E. F. Stange and J. Wehkamp, From intestinal stem cells to inflammatory bowel diseases, World J. Gastroenterol., 2011, 17(27), 3198–3203 Search PubMed.
- R. Okamoto and M. Watanabe, Role of epithelial cells in the pathogenesis and treatment of inflammatory bowel disease, J. Gastroenterol., 2016, 51(1), 11–21 CrossRef CAS PubMed.
- T.-C. Liu, B. Gurram, M. T. Baldridge, R. Head, V. Lam and C. Luo,
et al., Paneth cell defects in Crohn's disease patients promote dysbiosis, JCI Insight., 2016, 1(8), e86907 Search PubMed.
- M. Gersemann, S. Becker, I. Kübler, M. Koslowski, G. Wang and K. R. Herrlinger,
et al., Differences in goblet cell differentiation between Crohn's disease and ulcerative colitis, Differentiation, 2009, 77(1), 84–94 CrossRef CAS PubMed.
- A. L. Haber, M. Biton, N. Rogel, R. H. Herbst, K. Shekhar and C. Smillie,
et al., A single-cell survey of the small intestinal epithelium, Nature, 2017, 551(7680), 333–339 CrossRef CAS PubMed.
- C. S. Smillie, M. Biton, J. Ordovas-Montanes, K. M. Sullivan, G. Burgin and D. B. Graham,
et al., Intra- and Inter-cellular Rewiring of the Human Colon during Ulcerative Colitis, Cell, 2019, 178(3), 714–730 CrossRef CAS PubMed.
- K. Parikh, A. Antanaviciute, D. Fawkner-Corbett, M. Jagielowicz, A. Aulicino and C. Lagerholm,
et al., Colonic epithelial cell diversity in health and inflammatory bowel disease, Nature, 2019, 567(7746), 49–55 CrossRef CAS PubMed.
- R. G. Lindeboom, L. van Voorthuijsen, K. C. Oost, M. J. Rodríguez-Colman, M. V. Luna-Velez and C. Furlan,
et al., Integrative multi-omics analysis of intestinal organoid differentiation, Mol. Syst. Biol., 2018, 14(6), e8227 CrossRef PubMed.
- M. Noben, W. Vanhove, K. Arnauts, A. Santo Ramalho, G. Van Assche and S. Vermeire,
et al., Human intestinal epithelium in a dish: Current models for research into gastrointestinal pathophysiology, United Eur. Gastroenterol. J., 2017, 5(8), 1073–1081 CrossRef PubMed.
- M. R. Aberle, R. A. Burkhart, H. Tiriac, S. W. M. Olde Damink, C. H. C. Dejong and D. A. Tuveson,
et al., Patient-derived organoid models help define personalized management of gastrointestinal cancer, Br. J. Surg., 2018, 105((2), e48–e60 CrossRef CAS PubMed.
- T. Sato, R. G. Vries, H. J. Snippert, M. van de Wetering, N. Barker and D. E. Stange,
et al., Single Lgr5 stem cells build crypt-villus structures in vitro without a mesenchymal niche, Nature, 2009, 459(7244), 262–265 CrossRef CAS PubMed.
- T. Sato, J. H. van Es, H. J. Snippert, D. E. Stange, R. G. Vries and M. van den Born,
et al., Paneth cells constitute the niche for Lgr5 stem cells in intestinal crypts, Nature, 2011, 469(7330), 415–418 CrossRef CAS PubMed.
- T. Sato and H. Clevers, Growing self-organizing mini-guts from a single intestinal stem cell: mechanism and applications, Science, 2013, 340(6137), 1190–1194 CrossRef CAS PubMed.
- N. C. Zachos, O. Kovbasnjuk, J. Foulke-Abel, J. In, S. E. Blutt and H. R. de Jonge,
et al., Human enteroids/colonoids and intestinal organoids functionally recapitulate normal intestinal physiology and pathophysiology, J. Biol. Chem., 2016, 291(8), 3759–3766 CrossRef CAS PubMed.
- L. Schulte, M. Hohwieler, M. Müller and J. Klaus, Intestinal organoids as a novel complementary model to dissect inflammatory bowel disease, Stem Cells Int., 2019, 8010645 CAS.
- I. Dotti and A. Salas, Potential Use of Human Stem Cell-Derived Intestinal Organoids to Study Inflammatory Bowel Diseases, Inflammatory Bowel Dis., 2018, 24(12), 2501–2509 Search PubMed.
- H. F. Farin, J. H. Van Es and H. Clevers, Redundant sources of Wnt regulate intestinal stem cells and promote formation of Paneth cells, Gastroenterology, 2012, 143(6), 1518–1529 CrossRef CAS PubMed.
- X. Yin, H. F. Farin, J. H. van Es, H. Clevers, R. Langer and J. M. Karp, Niche-independent high-purity cultures of Lgr5+ intestinal stem cells and their progeny, Nat. Methods, 2014, 11(1), 106–112 CrossRef CAS PubMed.
- B. E. Mead and J. M. Karp, All models are wrong, but some organoids may be useful, Genome Biol., 2019, 20(1), 66 CrossRef PubMed.
- B. E. Mead, J. Ordovas-Montanes, A. P. Braun, L. E. Levy, P. Bhargava and M. J. Szucs,
et al., Harnessing single-cell genomics to improve the physiological fidelity of organoid-derived cell types, BMC Biol., 2018, 16(1), 62 CrossRef PubMed.
- L. Luu, Z. J. Matthews, S. D. Armstrong, P. P. Powell, T. Wileman and J. M. Wastling,
et al., Proteomic Profiling of Enteroid Cultures Skewed toward Development of Specific Epithelial Lineages, Proteomics, 2018, 18(16), e1800132 CrossRef PubMed.
- E. J. Jones, Z. J. Matthews, L. Gul, P. Sudhakar, A. Treveil and D. Divekar,
et al., Integrative analysis of Paneth cell proteomic and transcriptomic data from intestinal organoids reveals functional processes dependent on autophagy, Dis. Models Mech., 2019, 12, 3 Search PubMed.
- C. K. Kwoh and P. Y. Ng, Network analysis approach for biology, Cell. Mol. Life Sci., 2007, 64(14), 1739–1751 CrossRef CAS PubMed.
- T. Ideker and R. Nussinov, Network approaches and applications in biology, PLoS Comput. Biol., 2017, 13(10), e1005771 CrossRef PubMed.
- N. M. Luscombe, M. M. Babu, H. Yu, M. Snyder, S. A. Teichmann and M. Gerstein, Genomic analysis of regulatory network dynamics reveals large topological changes, Nature, 2004, 431(7006), 308–312 CrossRef CAS PubMed.
- M. G. P. van der Wijst, D. H. de Vries, H. Brugge, H.-J. Westra and L. Franke, An integrative approach for building personalized gene regulatory networks for precision medicine, Genome Med., 2018, 10(1), 96 CrossRef CAS PubMed.
- D. Módos, K. C. Bulusu, D. Fazekas, J. Kubisch, J. Brooks and I. Marczell,
et al., Neighbours of cancer-related proteins have key influence on pathogenesis and could increase the drug target space for anticancer therapies, NPJ Syst. Biol. Appl., 2017, 3, 2 CrossRef PubMed.
- T. S. Stappenbeck and D. P. B. McGovern, Paneth Cell Alterations in the Development and Phenotype of Crohn's Disease, Gastroenterology, 2017, 152(2), 322–326 CrossRef CAS PubMed.
- H. C. Clevers and C. L. Bevins, Paneth cells: maestros of the small intestinal crypts, Annu. Rev. Physiol., 2013, 75, 289–311 CrossRef CAS PubMed.
- G. D. Bader and C. W. Hogue, An automated method for finding molecular complexes in large protein interaction networks, BMC Bioinf., 2003, 4, 2 CrossRef PubMed.
- A. Fabregat, S. Jupe, L. Matthews, K. Sidiropoulos, M. Gillespie and P. Garapati,
et al., The reactome pathway knowledgebase, Nucleic Acids Res., 2018, 46(D1), D649–D655 CrossRef CAS PubMed.
- P. Han, C. Gopalakrishnan, H. Yu and E. Wang, Gene Regulatory Network Rewiring in the Immune Cells Associated with Cancer, Genes, 2017, 8, 11 Search PubMed.
- J. Park and H. H. Wang, Systematic and synthetic approaches to rewire regulatory networks, Curr. Opin. Syst. Biol., 2018, 8, 90–96 CrossRef PubMed.
-
M. R. Leach and D. B. Williams, Calnexin and Calreticulin, Molecular Chaperones of the Endoplasmic Reticulum – Madame Curie Bioscience Database – NCBI Bookshelf, 2013 Search PubMed.
- A. Kaser, A.-H. Lee, A. Franke, J. N. Glickman, S. Zeissig and H. Tilg,
et al., XBP1 links ER stress to intestinal inflammation and confers genetic risk for human inflammatory bowel disease, Cell, 2008, 134(5), 743–756 CrossRef CAS PubMed.
- A. Kaser and R. S. Blumberg, Endoplasmic reticulum stress in the intestinal epithelium and inflammatory bowel disease, Semin. Immunol., 2009, 21(3), 156–163 CrossRef CAS PubMed.
- A. Kaser, M. B. Flak, M. F. Tomczak and R. S. Blumberg, The unfolded protein response and its role in intestinal homeostasis and inflammation, Exp. Cell Res., 2011, 317(19), 2772–2779 CrossRef CAS PubMed.
- V. Chandra, E. Bhagyaraj, R. Nanduri, N. Ahuja and P. Gupta, NR1D1 ameliorates Mycobacterium tuberculosis clearance through regulation of autophagy, Autophagy, 2015, 11(11), 1987–1997 CrossRef CAS PubMed.
- A. H. Mirza, C. H. Berthelsen, S. E. Seemann, X. Pan, K. S. Frederiksen and M. Vilien,
et al., Transcriptomic landscape of lncRNAs in inflammatory bowel disease, Genome Med., 2015, 7(1), 39 CrossRef PubMed.
- R. H. Oakley and J. A. Cidlowski, The biology of the glucocorticoid receptor: new signaling mechanisms in health and disease, J. Allergy Clin. Immunol., 2013, 132(5), 1033–1044 CrossRef CAS PubMed.
- P. Rutgeerts, The use of oral topically acting glucocorticosteroids in the treatment of inflammatory bowel disease, Mediators Inflammation, 1998, 7(3), 137–140 CrossRef CAS PubMed.
- C. Prantera and S. Marconi, Glucocorticosteroids in the treatment of inflammatory bowel disease and approaches to minimizing systemic activity, Ther. Adv. Gastroenterol., 2013, 6(2), 137–156 CrossRef CAS PubMed.
- S. De Iudicibus, R. Franca, S. Martelossi, A. Ventura and G. Decorti, Molecular mechanism of glucocorticoid resistance in inflammatory bowel disease, World J. Gastroenterol., 2011, 17(9), 1095–1108 CrossRef CAS PubMed.
- K. Dubois-Camacho, P. A. Ottum, D. Franco-Muñoz, M. De la Fuente, A. Torres-Riquelme and D. Díaz-Jiménez,
et al., Glucocorticosteroid therapy in inflammatory bowel diseases: From clinical practice to molecular biology, World J. Gastroenterol., 2017, 23(36), 6628–6638 CrossRef CAS PubMed.
- A. Yemelyanov, J. Czwornog, D. Chebotaev, A. Karseladze, E. Kulevitch and X. Yang,
et al., Tumor suppressor activity of glucocorticoid receptor in the prostate, Oncogene, 2007, 26(13), 1885–1896 CrossRef CAS PubMed.
- H. Dinkel, K. Van Roey, S. Michael, M. Kumar, B. Uyar and B. Altenberg,
et al., ELM2016 – data update and new functionality of the eukaryotic linear motif resource, Nucleic Acids Res., 2016, 44(D1), D294–D300 CrossRef CAS PubMed.
- S. Wu, Y.-G. Zhang, R. Lu, Y. Xia, D. Zhou and E. O. Petrof,
et al., Intestinal epithelial vitamin D receptor deletion leads to defective autophagy in colitis, Gut, 2015, 64(7), 1082–1094 CrossRef CAS PubMed.
- D. Bakke, R. Lu, A. Agrawal, Y. Zhang and J. Sun, 18 myeloid vitamin d receptor signaling regulates Paneth cell function and intestinal homeostasis, Gastroenterology, 2018, 154(1), S41 CrossRef.
- T.-T. Wang, F. P. Nestel, V. Bourdeau, Y. Nagai, Q. Wang and J. Liao,
et al., Cutting edge: 1,25-dihydroxyvitamin D3 is a direct inducer of antimicrobial peptide gene expression, J. Immunol., 2004, 173(5), 2909–2912 CrossRef CAS PubMed.
- A. F. Gombart, N. Borregaard and H. P. Koeffler, Human cathelicidin antimicrobial peptide (CAMP) gene is a direct target of the vitamin D receptor and is strongly up-regulated in myeloid cells by 1,25-dihydroxyvitamin D3, FASEB J., 2005, 19(9), 1067–1077 CrossRef CAS PubMed.
- F. H. Pei, Y. J. Wang, S. L. Gao, B. R. Liu, Y. J. Du and W. Liu,
et al., Vitamin D receptor gene polymorphism and ulcerative colitis susceptibility in Han Chinese, J. Dig. Dis., 2011, 12(2), 90–98 CrossRef CAS PubMed.
- M. T. Abreu, V. Kantorovich, E. A. Vasiliauskas, U. Gruntmanis, R. Matuk and K. Daigle,
et al., Measurement of vitamin D levels in inflammatory bowel disease patients reveals a subset of Crohn's disease patients with elevated 1,25-dihydroxyvitamin D and low bone mineral density, Gut, 2004, 53(8), 1129–1136 CrossRef CAS PubMed.
- L. A. Bovolenta, M. L. Acencio and N. Lemke, HTRIdb: an open-access database for experimentally verified human transcriptional regulation interactions, BMC Genomics, 2012, 13, 405 CrossRef CAS PubMed.
- R. Lesurf, K. C. Cotto, G. Wang, M. Griffith, K. Kasaian and S. J. M. Jones,
et al., ORegAnno 3.0: a community-driven resource for curated regulatory annotation, Nucleic Acids Res., 2016, 44(D1), D126–D132 CrossRef CAS PubMed.
- D. J. Bettoun, T. P. Burris, K. A. Houck, D. W. Buck, K. R. Stayrook and B. Khalifa,
et al., Retinoid X receptor is a nonsilent major contributor to vitamin D receptor-mediated transcriptional activation, Mol. Endocrinol., 2003, 17(11), 2320–2328 CrossRef CAS PubMed.
- R. Grenningloh, B. Y. Kang and I.-C. Ho, Ets-1, a functional cofactor of T-bet, is essential for Th1 inflammatory responses, J. Exp. Med., 2005, 201(4), 615–626 CrossRef CAS PubMed.
- E. Mouly, K. Chemin, H. V. Nguyen, M. Chopin, L. Mesnard and M. Leite-de-Moraes,
et al., The Ets-1 transcription factor controls the development and function of natural regulatory T cells, J. Exp. Med., 2010, 207(10), 2113–2125 CrossRef CAS PubMed.
- S. Konno, M. Iizuka, M. Yukawa, K. Sasaki, A. Sato and Y. Horie,
et al., Altered expression of angiogenic factors in the VEGF-Ets-1 cascades in inflammatory bowel disease, J. Gastroenterol., 2004, 39(10), 931–939 CrossRef CAS PubMed.
- D. Li, T. Haritunians, A. Potdar and D. P. B. McGovern, 16 Genetic analysis identified novel loci associated with IBD, Inflammatory Bowel Dis., 2018, 24(suppl_1), S14–S14 CrossRef.
- L. Jostins, S. Ripke, R. K. Weersma, R. H. Duerr, D. P. McGovern and K. Y. Hui,
et al., Host-microbe interactions have shaped the genetic architecture of inflammatory bowel disease, Nature, 2012, 491(7422), 119–124 CrossRef CAS PubMed.
- K. K.-H. Farh, A. Marson, J. Zhu, M. Kleinewietfeld, W. J. Housley and S. Beik,
et al., Genetic and epigenetic fine mapping of causal autoimmune disease variants, Nature, 2015, 518(7539), 337–343 CrossRef CAS PubMed.
- A. F. Di Narzo, L. A. Peters, C. Argmann, A. Stojmirovic, J. Perrigoue and K. Li,
et al., Blood and Intestine eQTLs from an Anti-TNF-Resistant Crohn's Disease Cohort Inform IBD Genetic Association Loci, Clin. Transl. Gastroenterol., 2016, 7(6), e177 CrossRef CAS PubMed.
- M. Z. Cader and A. Kaser, Recent advances in inflammatory bowel disease: mucosal immune cells in intestinal inflammation, Gut, 2013, 62(11), 1653–1664 CrossRef CAS PubMed.
- C. A. Thorne, I. W. Chen, L. E. Sanman, M. H. Cobb, L. F. Wu and S. J. Altschuler, Enteroid monolayers reveal an autonomous WNT and BMP circuit controlling intestinal epithelial growth and organization, Dev. Cell, 2018, 44(5), 624–633 CrossRef CAS PubMed.
- D. P. Chopra, A. A. Dombkowski, P. M. Stemmer and G. C. Parker, Intestinal epithelial cells in vitro, Stem Cells Dev., 2010, 19(1), 131–142 CrossRef PubMed.
-
S. Lukovac and G. Roeselers, Intestinal crypt organoids as experimental models, in The Impact of Food Bioactives on Health: in vitro and ex vivo models. Cham (CH), ed. K. Verhoeckx, P. Cotter, I. López-Expósito, C. Kleiveland, T. Lea and A. Mackieet al., Springer, 2015 Search PubMed.
- B. W. Doble and J. R. Woodgett, GSK-3: tricks of the trade for a multi-tasking kinase, J. Cell Sci., 2003, 116(Pt 7), 1175–1186 CrossRef CAS PubMed.
- G. Novarino, A. G. Fenstermaker, M. S. Zaki, M. Hofree, J. L. Silhavy and A. D. Heiberg,
et al., Exome sequencing links corticospinal motor neuron disease to common neurodegenerative disorders, Science, 2014, 343(6170), 506–511 CrossRef CAS PubMed.
- J. K. Huang, D. E. Carlin, M. K. Yu, W. Zhang, J. F. Kreisberg and P. Tamayo,
et al., Systematic evaluation of molecular networks for discovery of disease genes, Cell Syst., 2018, 6(4), 484–495 CrossRef CAS PubMed.
- S. Wachi, K. Yoneda and R. Wu, Interactome-transcriptome analysis reveals the high centrality of genes differentially expressed in lung cancer tissues, Bioinformatics, 2005, 21(23), 4205–4208 CrossRef CAS PubMed.
- H. Yu, N. M. Luscombe, J. Qian and M. Gerstein, Genomic analysis of gene expression relationships in transcriptional regulatory networks, Trends Genet., 2003, 19(8), 422–427 CrossRef CAS PubMed.
- J. Kubisch, D. Türei, L. Földvári-Nagy, Z. A. Dunai, L. Zsákai and M. Varga,
et al., Complex regulation of autophagy in cancer - integrated approaches to discover the networks that hold a double-edged sword, Semin. Cancer Biol., 2013, 23(4), 252–261 CrossRef PubMed.
- N. Vijesh, S. K. Chakrabarti and J. Sreekumar, Modeling of gene regulatory networks: a review, JBiSE, 2013, 06(02), 223–231 CrossRef.
- A.-L. Barabási and Z. N. Oltvai, Network biology: understanding the cell's functional organization, Nat. Rev. Genet., 2004, 5(2), 101–113 CrossRef PubMed.
- G. P. Wagner and J. Zhang, The pleiotropic structure of the genotype-phenotype map: the evolvability of complex organisms, Nat. Rev. Genet., 2011, 12(3), 204–213 CrossRef CAS PubMed.
- T. L. Davis and I. Rebay, Master regulators in development: Views from the Drosophila retinal determination and mammalian pluripotency gene networks, Dev. Biol., 2017, 421(2), 93–107 CrossRef CAS PubMed.
- M.-A. Mendoza-Parra, V. Malysheva, M. A. Mohamed Saleem, M. Lieb, A. Godel and H. Gronemeyer, Reconstructed cell fate-regulatory programs in stem cells reveal hierarchies and key factors of neurogenesis, Genome Res., 2016, 26(11), 1505–1519 CrossRef CAS PubMed.
- K. Cadwell, J. Y. Liu, S. L. Brown, H. Miyoshi, J. Loh and J. K. Lennerz,
et al., A key role for autophagy and the autophagy gene Atg16l1 in mouse and human intestinal Paneth cells, Nature, 2008, 456(7219), 259–263 CrossRef CAS PubMed.
- M. J. Rodríguez-Colman, M. Schewe, M. Meerlo, E. Stigter, J. Gerrits and M. Pras-Raves,
et al., Interplay between metabolic identities in the intestinal crypt supports stem cell function, Nature, 2017, 543(7645), 424–427 CrossRef PubMed.
- U. Paulus, M. Loeffler, J. Zeidler, G. Owen and C. S. Potten, The differentiation and lineage development of goblet cells in the murine small intestinal crypt: experimental and modelling studies, J. Cell Sci., 1993, 106(Pt 2), 473–483 Search PubMed.
- J. Jung and H. Jung, Methods to analyze cell type-specific gene expression profiles from heterogeneous cell populations, Anim. Cells Syst., 2016, 20(3), 113–117 CrossRef CAS.
- A. Brazovskaja, B. Treutlein and J. G. Camp, High-throughput single-cell transcriptomics on organoids, Curr. Opin. Biotechnol, 2019, 55, 167–171 CrossRef CAS PubMed.
- G. Chen, B. Ning and T. Shi, Single-Cell RNA-Seq Technologies and Related Computational Data Analysis, Front. Genet., 2019, 10, 317 CrossRef CAS PubMed.
- T. Nunes, C. Bernardazzi and H. S. de Souza, Cell death and inflammatory bowel diseases: apoptosis, necrosis, and autophagy in the intestinal epithelium, BioMed Res. Int., 2014, 218493 Search PubMed.
- H. A. Aghdaei, A. A. Kadijani, D. Sorrentino, A. Mirzaei, S. Shahrokh and H. Balaii,
et al., An increased Bax/Bcl-2 ratio in circulating inflammatory cells predicts primary response to infliximab in inflammatory bowel disease patients, United Eur. Gastroenterol. J., 2018, 6(7), 1074–1081 CrossRef CAS PubMed.
- P. J. Real, V. Tosello, T. Palomero, M. Castillo, E. Hernando and E. de Stanchina,
et al., Gamma-secretase inhibitors reverse glucocorticoid resistance in T cell acute lymphoblastic leukemia, Nat. Med., 2009, 15(1), 50–58 CrossRef CAS PubMed.
- X. Zheng, K. Tsuchiya, R. Okamoto, M. Iwasaki, Y. Kano and N. Sakamoto,
et al., Suppression of hath1 gene expression directly regulated by hes1 via notch signaling is associated with goblet cell depletion in ulcerative colitis, Inflammatory Bowel Dis., 2011, 17(11), 2251–2260 CrossRef PubMed.
- C. G. Chapman and J. Pekow, The emerging role of miRNAs in inflammatory bowel disease: a review, Ther. Adv. Gastroenterol., 2015, 8(1), 4–22 CrossRef PubMed.
-
S. Andrews, Babraham Bioinformatics – FastQC A Quality Control tool for High Throughput Sequence Data [Internet]. 2010 [cited 2018 Sep 28], available from: https://www.bioinformatics.babraham.ac.uk/projects/fastqc/.
- D. Kim, B. Langmead and S. L. Salzberg, HISAT: a fast spliced aligner with low memory requirements, Nat. Methods, 2015, 12(4), 357–360 CrossRef CAS PubMed.
- M. Pertea, G. M. Pertea, C. M. Antonescu, T.-C. Chang, J. T. Mendell and S. L. Salzberg, StringTie enables improved reconstruction of a transcriptome from RNA-seq reads, Nat. Biotechnol., 2015, 33(3), 290–295 CrossRef CAS PubMed.
- M. Pertea, D. Kim, G. M. Pertea, J. T. Leek and S. L. Salzberg, Transcript-level expression analysis of RNA-seq experiments with HISAT, StringTie and Ballgown, Nat. Protoc., 2016, 11(9), 1650–1667 CrossRef CAS PubMed.
- L. Kong, Y. Zhang, Z.-Q. Ye, X.-Q. Liu, S.-Q. Zhao and L. Wei,
et al., CPC: assess the protein-coding potential of transcripts using sequence features and support vector machine, Nucleic Acids Res., 2007, 35(Web Server issue), W345–W349 CrossRef PubMed.
- L. Wang, H. J. Park, S. Dasari, S. Wang, J.-P. Kocher and W. Li, CPAT: Coding-Potential Assessment Tool using an alignment-free logistic regression model, Nucleic Acids Res., 2013, 41(6), e74 CrossRef CAS PubMed.
- N. L. Bray, H. Pimentel, P. Melsted and L. Pachter, Near-optimal probabilistic RNA-seq quantification, Nat. Biotechnol., 2016, 34(5), 525–527 CrossRef CAS.
- H. Pimentel, N. L. Bray, S. Puente, P. Melsted and L. Pachter, Differential analysis of RNA-seq incorporating quantification uncertainty, Nat. Methods, 2017, 14(7), 687–690 CrossRef CAS PubMed.
- A. Rueda, G. Barturen, R. Lebrón, C. Gómez-Martín, Á. Alganza and J. L. Oliver,
et al., sRNAtoolbox: an integrated collection of small RNA research tools, Nucleic Acids Res., 2015, 43(W1), W467–W473 CrossRef CAS PubMed.
- A. Kozomara and S. Griffiths-Jones, miRBase: annotating high confidence microRNAs using deep sequencing data, Nucleic Acids Res., 2014, 42(Database issue), D68–D73 CrossRef CAS PubMed.
- M. D. Robinson, D. J. McCarthy and G. K. Smyth, edgeR: a Bioconductor package for differential expression analysis of digital gene expression data, Bioinformatics, 2010, 26(1), 139–140 CrossRef CAS PubMed.
- H. Ogata, S. Goto, K. Sato, W. Fujibuchi, H. Bono and M. Kanehisa, KEGG: kyoto encyclopedia of genes and genomes, Nucleic Acids Res., 1999, 27(1), 29–34 CrossRef CAS PubMed.
- G. Yu and Q.-Y. He, ReactomePA: an R/Bioconductor package for reactome pathway analysis and visualization, Mol. BioSyst., 2016, 12(2), 477–479 RSC.
- M. Kanehisa, M. Furumichi, M. Tanabe, Y. Sato and K. Morishima, KEGG: new perspectives on genomes, pathways, diseases and drugs, Nucleic Acids Res., 2017, 45(D1), D353–D361 CrossRef CAS PubMed.
- K. P. O’Brien, M. Remm and E. L. L. Sonnhammer, Inparanoid: a comprehensive database of eukaryotic orthologs, Nucleic Acids Res., 2005, 33(Database issue), D476–D480 CrossRef PubMed.
- E. L. L. Sonnhammer and G. Östlund, InParanoid 8: orthology analysis between 273 proteomes, mostly eukaryotic, Nucleic Acids Res., 2015, 43(Database issue), D234–D239 CrossRef CAS PubMed.
- U. Mudunuri, A. Che, M. Yi and R. M. Stephens, bioDBnet: the biological database network, Bioinformatics, 2009, 25(4), 555–556 CrossRef CAS PubMed.
- I. S. Vlachos, M. D. Paraskevopoulou, D. Karagkouni, G. Georgakilas, T. Vergoulis and I. Kanellos,
et al., DIANA-TarBasev7.0: indexing more than half a million experimentally supported miRNA:mRNA interactions, Nucleic Acids Res., 2015, 43(Database issue), D153–D159 CrossRef CAS PubMed.
- M. D. Paraskevopoulou, I. S. Vlachos, D. Karagkouni, G. Georgakilas, I. Kanellos and T. Vergoulis,
et al., DIANA-LncBasev2: indexing microRNA targets on non-coding transcripts, Nucleic Acids Res., 2016, 44(D1), D231–D238 CrossRef CAS PubMed.
- S. W. Chi, J. B. Zang, A. Mele and R. B. Darnell, Argonaute HITS-CLIP decodes microRNA-mRNA interaction maps, Nature, 2009, 460(7254), 479–486 CrossRef CAS PubMed.
- J. Wang, M. Lu, C. Qiu and Q. Cui, TransmiR: a transcription factor-microRNA regulation database, Nucleic Acids Res., 2010, 38(Database issue), D119–D122 CrossRef CAS PubMed.
- I. Yevshin, R. Sharipov, T. Valeev, A. Kel and F. Kolpakov, GTRD: a database of transcription factor binding sites identified by ChIP-seq experiments, Nucleic Acids Res., 2017, 45(D1), D61–D67 CrossRef CAS PubMed.
- H. Han, H. Shim, D. Shin, J. E. Shim, Y. Ko and J. Shin,
et al., TRRUST: a reference database of human transcriptional regulatory interactions, Sci. Rep., 2015, 5, 11432 CrossRef CAS PubMed.
- H. Han, J.-W. Cho, S. Lee, A. Yun, H. Kim and D. Bae,
et al., TRRUSTv2: an expanded reference database of human and mouse transcriptional regulatory interactions, Nucleic Acids Res., 2018, 46(D1), D380–D386 CrossRef CAS PubMed.
- A. R. Quinlan and I. M. Hall, BEDTools: a flexible suite of utilities for comparing genomic features, Bioinformatics, 2010, 26(6), 841–842 CrossRef CAS PubMed.
- P. Shannon, A. Markiel, O. Ozier, N. S. Baliga, J. T. Wang and D. Ramage,
et al., Cytoscape: a software environment for integrated models of biomolecular interaction networks, Genome Res., 2003, 13(11), 2498–2504 CrossRef CAS PubMed.
- I. H. Goenawan, K. Bryan and D. J. Lynn, DyNet: visualization and analysis of dynamic molecular interaction networks, Bioinformatics, 2016, 32(17), 2713–2715 CrossRef CAS PubMed.
- K. C. Cotto, A. H. Wagner, Y.-Y. Feng, S. Kiwala, A. C. Coffman and G. Spies,
et al., DGIdb 3.0: a redesign and expansion of the drug-gene interaction database, Nucleic Acids Res., 2018, 46(D1), D1068–D1073 CrossRef CAS PubMed.
Footnotes |
† Electronic supplementary information (ESI) available. See DOI: 10.1039/c9mo00130a |
‡ Equal contributions. |
|
This journal is © The Royal Society of Chemistry 2020 |